- 1State Key Laboratory of Pollution Control and Resource Reuse, School of the Environment, Nanjing University, Nanjing, China
- 2Lancaster Environment Centre, Lancaster University, Lancaster, United Kingdom
- 3School of Pharmacy and Biomedical Sciences, University of Central Lancashire, Preston, United Kingdom
- 4School of Environment, Tsinghua University, Beijing, China
Carbonaceous substances are fundamental organic nutrients for microbial metabolism and catabolism in natural habitats. Microbial abilities to sense, accumulate, and utilize organic carbonaceous substances in the complex nutrient environment are important for their growth and ecological functions. Bacterial chemotaxis is an effective mechanism for microbial utilization of carbonaceous substances under nutrient depletion conditions. Although bacterial accumulation and utilization to individual carbonaceous substance in long-term cultivation has been well studied, their selective affinity of mixed carbonaceous substances remains to be investigated, primarily because of technical limitations of conventional methods. Herein, we applied Raman microspectroscopy to identify chemotaxis-driven affinity and accumulation of four organic carbonaceous substances (glucose, succinate, acetate, and salicylate) by three bacterial strains (Acinetobacter baylyi, Pseudomonas fluorescence, and Escherichia coli). A. baylyi exhibited strong binding affinity toward glucose and succinate, whereas P. fluorescence and E. coli were preferentially responsive to glucose and acetate. For the first time, bacterial transient selectivity of carbonaceous substances was studied via interrogating Raman spectral alterations. Post-exposure to carbonaceous-substance mixtures, the three bacterial strains showed distinct selective behaviors. Stronger selective affinity enhanced the chemotaxis-related signal transduction in A. baylyi cells, whereas the carbonaceous substance signal transduction in E. coli was decreased by higher selective affinity. In P. fluorescence, there was no specific effect of selective affinity on signal transduction. Our study suggests that Raman microspectroscopy can successfully investigate and distinguish different scenarios of bacterial competitive and transient unitization of organic carbonaceous substances.
Introduction
Bacteria can employ diverse mechanisms to sense and accumulate chemical molecules in their natural environment (Adler, 1966; Sourjik and Wingreen, 2012). The bacterial chemotaxis system is a well-developed chemical gradient sensor, and motile chemotactic bacterial cells are able to follow concentration gradients of chemo-attractants, such as nutrients and other environmental stimuli, to select and accumulate them on cell membrane (Sourjik and Wingreen, 2012). Attractant nutrients include organic carbonaceous substances, organic nitrogen and other essential inorganic elements. Organic carbonaceous substances, e.g., sugar, organic acids and polyols, are fundamental nutrients for bacterial growth. Bacteria show positive and selective responses toward some specific carbonaceous substance molecules present in medium (Adler, 1966; Singh and Arora, 2001; Sourjik, 2004; Kirby, 2009; Sampedro et al., 2015). Glucose is a major carbonaceous substance exhibiting high attraction to microbes (Hazelbauer et al., 1969; Singh and Arora, 2001; Weert et al., 2002), and carbonaceous acids such as succinate and acetate can be used as chemo-attractant in bacterial chemotaxis assays (Dailey and Berg, 1993; Oku et al., 2014; Sampedro et al., 2015). Bacterial chemotaxis refers to the movement of cells toward attractants or repellents (Sampedro et al., 2015). Favorable attractants of carbonaceous acids lead to continuous movement promoting their further accumulation and utilization in bacterial cells (Sly et al., 1993). Microbial chemotaxis-related affinity toward carbonaceous substances is vital for their competitive advantages in natural habitats as it assists microbes in accessing carbonaceous nutrients, and determines the accumulation and utilization of carbonaceous nutrients in bacterial cells.
Bacterial chemotactic responses to carbonaceous substances are regulated by specific proteins. In Pseudomonas, methyl-accepting proteins (M) are believed to determine the specificity of the chemotactic response (Ferrández et al., 2002). MCP comprises the periplasmic ligand-binding region and the cytosolic methyl-accepting domain that allows sense and transfer of the chemical signal to bacterial cells (Ferrández et al., 2002; Alexander and Zhulin, 2007). The chemotaxis protein McpS in Pseudomonas putida KT2440 is the receptor-sensing tricarboxylic acid (TCA) cycle intermediate (Lacal et al., 2011), and the glucose-specific binding protein GLTB is characterized in P. aeruginosa (Sly et al., 1993). E. coli is a model strain for chemotaxis study (Sourjik, 2004; Sourjik and Wingreen, 2012), in which the CheA and CheW combine as a ternary complex to facilitate chemotactic responses to carbonaceous substances such as glucose and acetate (Maddock and Shapiro, 1993; Sourjik and Berg, 2000). It is reported that mcp, cheR, and chew-2 genes in Acinetobacter are responsible for chemoreceptor cluster formation (Wang and Shao, 2014). The flagella on the Acinetobacter cell membrane facilitate the movement toward carbonaceous molecules (Foster, 1962). However, mechanisms of glucose or succinate chemotaxis in Acinetobacter remain unknown.
Approaches used to study bacterial chemotaxis include swarm plates (Henrichsen, 1972), capillary assays (Berg and Turner, 1990), temporal stimulation of tethered cells (Block et al., 1983), and automated tracking of swimming cells (Shi et al., 2006; Kearns, 2010). These approaches are versatile in testing bacterial chemotaxis toward specific individual chemicals. They can successfully describe the movement of bacterial cells to a certain carbonaceous substance; however, they fail in distinguishing which molecule is chemotactically sensed and accumulated by bacterial cells in a mixture of substances. In contrast, Raman microspectroscopy can provide the vibrational information on chemicals acquired by bacteria (Li et al., 2017). It is a fast, reproducible and non-destructive approach, and widely applied in biological studies (Efrima and Zeiri, 2009). The effects of the co-existence of carbonaceous substances on bacterial sensing and accumulation require further investigation, because it represents a real-world scenario of carbonaceous substance utilization by bacteria and explains the microbial ecological functions in complicated natural habitats.
Well-developed to determine cell-molecule interactions and the microbial response to the environment (Efrima and Bronk, 1998; Guzelian et al., 2002; Wang et al., 2011; Zhou et al., 2015; Jin et al., 2017b), Raman microspectroscopy has been applied to investigate nanotoxicity in bacterial cells (Cui et al., 2013) and to determine the minimum inhibitory concentrations of antibiotics using heavy water labeling (Tao et al., 2017). Recently, the alkane chemotactic affinity of A. baylyi is studied via Raman spectral alterations to illustrate the intracellular bioaccumulation in these cells (Li et al., 2017). Although it is well-documented that Raman spectroscopy is sophisticated in discriminating spectral alterations of bacterial cells after long-term cultivation with different carbon sources (Maquelin et al., 2000; Schuster et al., 2000), the study of bacterial transient affinity and uptake of diverse carbonaceous substances via Raman spectra remains covered. The fast manners of biospectroscopy allow the measurement of binding affinity and accumulation of molecules on/inside bacterial cells, hinting at the possibility of characterizing the fingerprints of carbonaceous substances bioaccumulated by bacteria and to distinguish their transient and selective responses. To our knowledge, there is no such study exploring Raman spectral alterations in bacterial transient affinity toward and selective uptake from a mixture of organic carbonaceous substances.
Based on our recently developed Raman spectroscopic method for determining chemotactic affinity and accumulation of alkane molecules in A. baylyi cells (Li et al., 2017), this work further distinguishes the Raman spectral alterations and quantifies the bacterial transient affinity and accumulation of hydrophilic carbonaceous substances (glucose, succinate, acetate, and salicylate) after short-time exposure (30 min). By interrogating the selective affinity and accumulation in Acinetobacter baylyi, Pseudomonas fluorescence, and Escherichia coli toward individual carbonaceous substance and their mixtures via Raman spectra, we found the selective behavior of bacterial cells after transient exposure to carbonaceous substance mixtures. Additionally, Raman spectral alterations revealed the distinct mechanisms of chemotaxis-related signal transduction in the three bacterial strains. Signal transduction was enhanced or weakened by attractant binding affinity in A. baylyi or E. coli, respectively, whereas no significant effect of attractant binding affinity on the signal transduction was observed for P. fluorescence. Our results provide a novel, rapid and cost-effective Raman spectroscopic technique in distinguishing bacterial selective affinity and accumulation of various nutrients in situ, potentially feasible in characterizing bacterial metabolic and catabolic behavior in their natural habitats.
Materials and Methods
Bacterial Strains and Growth Conditions
The bacterial strains used in this study included Acinetobacter baylyi ADP1, Pseudomonas fluorescence, and Escherichia coli JM109 (Jin et al., 2017a). They were grown in minimal medium (MM) with 20 mM sodium succinate as the sole carbon source, shaking at 150 rpm and 30°C for 16 h. To prepare mineral medium, 1.0 g of (NH4)2SO4, 2.5 g of KH2PO4, 0.1 g of MgSO4⋅7H2O, 0.005 g of FeSO4⋅7H2O, 0.25 g of nitrilotriacetic acid, 0.55 g of NaOH and 1 mL of Bauchop and Elsden solution were mixed well in 1.0 L deionized water and autoclaved (Jia et al., 2016). Bacterial cells were harvested by centrifugation at 4,000 rpm for 4 min and washed three times using sterile deionized water. Finally, the cell pellets were separately suspended in MM without any carbon source for further experiments. Unless specified otherwise, all chemicals used in this study were of analytical grade and purchased from Sigma-Aldrich (United Kingdom).
Exposure to Carbonaceous Substances
The four studied carbonaceous substances included three organic acid salts (sodium succinate, sodium acetate, and sodium salicylate) and one carbohydrate (glucose). They were dissolved in MM to prepare the 1 M stock solution required. Bacterial suspensions were mixed with each carbonaceous substance stock solution to obtain a final concentration of 10 mM. The transient exposure was 30 min at 30°C for all treatments. From our previous study, all the three strains stayed in the lag phase for at least 2 h after re-cultivated in MM with different carbon sources and exhibited distinct spectral fingerprints comparing to those after the long-term cultivation (usually 12–16 h) (Jin et al., 2018). Their Raman spectral alterations, after transient exposure, were therefore entirely different from the alterations of bacterial cells after long-term cultivation with different carbon sources. Bacterial suspensions post-exposure to carbonaceous substances were centrifuged at 5,000 rpm for 5 min to remove the medium, and the cell pellets were washed once with sterile deionized water prior to Raman spectral acquisition.
The dose response of carbonaceous substance binding affinity and accumulation was measured by exposing bacterial suspensions to the carbonaceous substance with high binding affinity: succinate/glucose for A. baylyi and acetate/glucose for P. fluorescence and E. coli. After adding different volumes of carbonaceous substance stock solution to 1 mL bacterial suspensions to reach the final concentrations of 0, 1, 5, and 10 mM, the mixture was incubated for 30 min at 30°C, centrifuged at 5,000 rpm for 5 min and washed once with sterile deionized water prior to Raman spectra acquisition. Thirty-minute incubation at 30°C is the optimal exposure time for the most significant alterations in Raman spectra according to our previous study (Li et al., 2017) and was used in this study for Raman spectral acquisition.
To evaluate the transient and selective affinity response of each bacterial strain, the carbonaceous substance mixtures were prepared by mixing two carbonaceous substances, succinate/glucose for A. baylyi and acetate/glucose for P. fluorescence as well as E. coli; their final concentration was 10-mM:10-mM and 5-mM:10-mM, respectively. Bacterial cells were exposed to carbonaceous mixtures for 30 min at 30°C, followed by the same centrifugation and washing protocol as described above.
Chemotaxis Capillary Assay
To evidence bacterial chemotactic propensity toward each carbonaceous substance, the conventional capillary assay was performed, as previously described (Adler, 1973; Li et al., 2017). The capillary tube (internal diameter of 0.2 mm and length of 10 cm) was first plunged into the carbonaceous substance stock solution for 5 min until the liquid was drawn up to approximately 1 cm in the tube. The tube was then inserted into the bacterial suspension for 30 min at 30°C. In order to measure the carbonaceous substance affinity of different bacterial strains, quantitative polymerase chain reaction (qPCR) was used to quantify the 16S rRNA copy numbers of chemotactic bacteria on each capillary in triplicates. The 1-cm exterior from the open end of the capillary tube was plunged into qPCR buffer as the DNA template. The 10 μL qPCR buffer contained 1 μL of primer 341F (5′-CCTACGGGNGGCWGCAG-3′), 1 μL of primer 802R (5′-TACNVGGGTATCTAATCC-3′), 3 μL of molecular water and 5 μL of iTaqTM Universal SYBR® Green Supermix (BioRad, United States). The thermo cycling program was: initial denaturation at 94°C for 3 min; 34 amplification cycles of 94°C for 45 s, 52°C for 45 s, 72°C for 45 s, and fluorescence data acquisition at 80°C for 15 s. The qPCR standard curves were obtained with serial dilutions of quantified plasmid DNA containing the fragment of 16S rRNA genes.
Raman Spectral Acquisition
An InVia confocal micro-Raman system (Renishaw, Gloucestershire, United Kingdom) equipped with a 100 mW 785 nm excitation laser was employed for sample detection and Raman spectral acquisition, with a charged couple detector (CCD) and an attached microscope (Leica Microsystems, Milton Keynes, United Kingdom). The Raman system was calibrated using a Renishaw silicon calibration source before sample analysis. Ten microliter of washed cells were positioned onto a glass slide covered by aluminum foil and air-dried before Raman spectral acquisition (Cui et al., 2016). Twenty spectra per sample were randomly acquired using the 50 μm spectrometer entrance slit combined with a 1200 lines per mm diffraction grating (1 cm–1 spatial resolution), ×50 objective (0.75 numerical aperture; approximately 1 μm spatial resolution), 50% laser power (13 mW at samples), 10 s acquisition time and one accumulation within a spectral range from 500 to 2000 cm–1.
Computational Analysis
Unless specifically stated otherwise, Raman spectra were pre-processed using baseline correction and vector normalization prior to principal component analysis (PCA) and linear discriminant analysis (LDA) using the IRootLab toolbox based on Matlab (version R2013b, MathWorks, United States) (Trevisan et al., 2013). PCA was carried out to visualize the natural variance within the dataset and reduce the dimensionality of the multivariate data (Trevisan et al., 2013). The separation of individual spectral categories from negative control and pure carbonaceous substance classes was measured by exporting PCA-derived data. To attain inter-class separation and minimize intra-class differences, LDA was employed to extract inter-category discriminating features (Martin et al., 2007; Butler et al., 2015). Post-exposure to different concentrations of carbonaceous substances, the dispersion of individual Raman spectra to that of negative controls (carbonaceous substance concentration = 0 mM) and pure carbonaceous substance was calculated based on principal component (PC)1 and PC2, and visualized as dispersion indicator (DI) score plots (Li et al., 2017). In a DI score plot, the increasing DI value between two categorizes is proportional to dissimilarity (Ami et al., 2013; Li et al., 2017).
Results and Discussion
Bacterial Chemotaxis Toward Carbonaceous Substance via Capillary Assay
The chemotaxis capillary assay of organic carbonaceous substance proved the different chemotactic sensitivities and binding affinities of A. baylyi, P. fluorescence, and E. coli toward glucose, acetate, succinate, and salicylate, respectively. From the results of the capillary assay, A. baylyi exhibited a highly sensitive chemotactic response toward glucose and succinate. The accumulation of A. baylyi 16S rRNA genes was 6.38 × 107 and 6.75 × 107 copies/capillary for glucose and succinate, respectively, significantly higher than those in acetate and salicylate treatments [see Electronic Supporting Information (ESI) Supplementary Figure S1]. In carbonaceous substance capillary assays of P. fluorescence, glucose was a strong chemo-attractant, achieving 2.13 × 107 copies/capillary 16S rRNA genes (see ESI Supplementary Figure S1). P. fluorescence cells could also chemotactically sense acetate (1.18 × 107 copies/capillary) or succinate (7.54 × 106 copies/capillary), whereas only a small number of P. fluorescence cells accumulated in the salicylate capillary (1.29 × 106 copies/capillary), indicating the weak chemotaxis of P. fluorescence toward salicylate. The chemotactic behavior of E. coli was entirely different from that of A. baylyi and P. fluorescence, consistent with previous studies (Singh and Arora, 2001; Kirby, 2009; Sourjik and Wingreen, 2012; Sampedro et al., 2015). E. coli showed weak chemotaxis-related affinity to succinate, and the 16S rRNA copy numbers post-exposure to the succinate were approximately 50 or 100-fold lower than that of A. baylyi or P. fluorescence (see ESI Supplementary Figure S1). Alternatively, the highest accumulation was achieved when post-exposure to acetate (1.57 × 106 copies/capillary), whereas the accumulation was only 2.26 copies/capillary 16S rRNA genes in salicylate assay, showing the extremely weak salicylate attraction to E. coli.
Raman Spectral Characterization of Bacterial Transient Affinity to Carbonaceous Substances
Raman spectral alterations of the three bacterial strains post-exposure to four carbonaceous substances profile their transient binding affinity and cellular accumulation (Figure 1). Figure 2 further illustrates the PCA differentiation of the transient affinity and accumulation in A. baylyi, P. fluorescence, and E. coli for each organic carbonaceous substance. The three different color gradients of light-, medium-, and dark-red in PCA score plots represent weak, moderate, and strong levels of bacterial binding affinity toward different organic carbonaceous substances, respectively. After transient exposure (30 min) to the four carbon sources, distinct Raman spectra were identified for A. baylyi. Table 1 lists the predominant peaks in the Raman spectra of the pure A. baylyi, including 723 and 777 cm–1 (nucleic acids) (De Gelder et al., 2007), 1002 cm–1 (phenylalanine) (Li et al., 2017), 1238 and 1311 cm–1 (amino acids) (De Gelder et al., 2007), 1441 cm–1 (glycine) (Cui et al., 2013) and 1663 cm–1 (protein) (Li et al., 2017). Post-exposure to acetate or salicylate, the Raman spectra of A. baylyi remain unchanged, and the PCA of Raman spectra are indistinctive in comparison to the pure strains (Figures 1A, 2A). The PCA score plot indicates the obvious Raman alterations post-exposure to succinate (Figure 2A). The specific bands at 738, 1332, and 1446 cm–1 observed in the Raman spectra are attributed to C-H wag, sodium deformation and C-H bend in succinate, confirming the transient affinity and accumulation of succinate molecules in A. baylyi cells (Figure 1A) (Frost and Kloprogge, 2000; Dhanya et al., 2011). The PCA category comprising A. baylyi post-exposure to glucose is close to pure glucose, significantly different from the group of pure A. baylyi cells (Figure 2A). In Figure 1A, the glucose affinity and accumulation of A. baylyi contributes to the Raman spectral alterations, especially at 1338 cm–1 (-CH2 deformation), 1379 cm–1 (C-H bending and O-H bending) and 1454 cm–1 (-CH2 bending) (Vasko et al., 1972; Araujo-Andrade et al., 2005). These results are consistent with those of capillary assays, highlighting that A. baylyi has moderate chemotactic accumulation of succinate and glucose. It is worth noting that, compared to salicylate, the Raman spectral PCA categorization of A. baylyi post-exposure to acetate is closer to pure bacterial cells, whereas the capillary assays showed the opposite result that acetate attraction to A. baylyi was weaker than salicylate (see ESI Supplementary Figures S1, S2). The difference between the two approaches implies that the conventional capillary assay only evaluates the movement and accumulation of bacterial cells toward capillary, different from the detection of chemotaxis-driven accumulation and utilization of organic carbonaceous substances on/inside bacterial cells via Raman microspectroscopy. In addition, the cost of this Raman spectra acquisition was approximately $0.065 per sample (ESI Supplementary Table S1), showing its cost-effectiveness and feasibility for future practices. It hints at the applicability of employing Raman microspectroscopy to distinguish the vibrational fingerprints of organic carbonaceous substances on bacteria and further characterize the mechanisms of bacterial chemotaxis in complex environment.
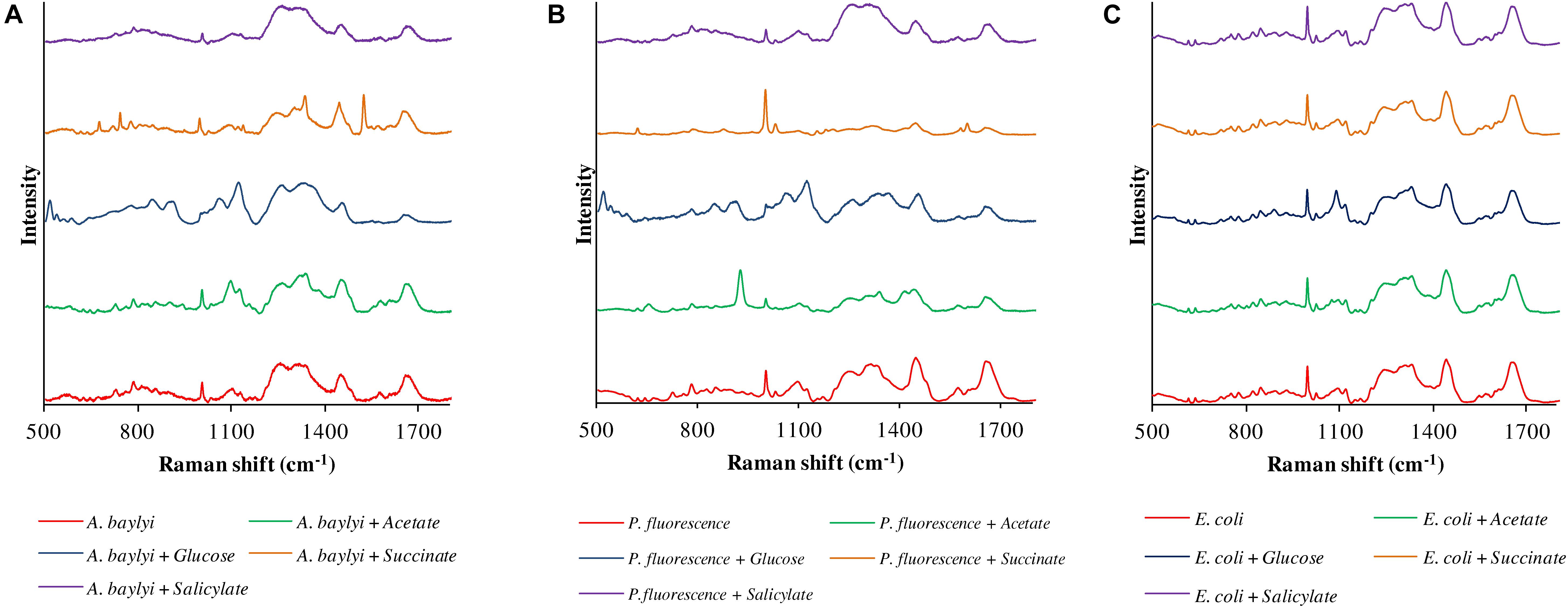
Figure 1. Raman spectra of A. baylyi (A), P. fluorescence (B) and E. coli (C), pre- and post-exposure to 10 mM acetate (green), glucose (blue), succinate (brown), and salicylate (purple). Twenty Raman spectra were randomly obtained per treatment.
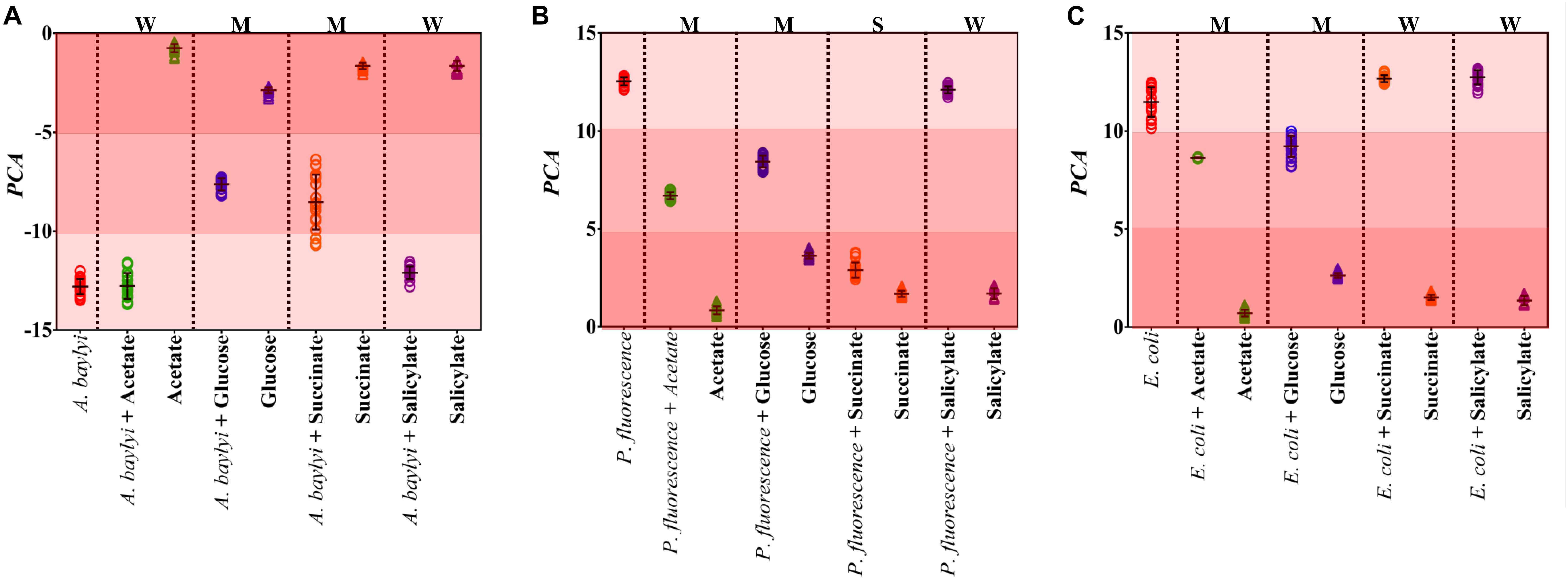
Figure 2. PCA score plots of A. baylyi (A), P. fluorescence (B) and E. coli (C) post-exposure to different organic carbonaceous substances including acetate (green), glucose (blue), succinate (orange), and salicylate (purple). In each plot, color gradients illustrate the level of bacterial binding affinity toward the four carbonaceous substances. Light-red indicates weak affinity (W), medium-red represents moderate affinity (M), and dark-red refers to strong affinity (S). Twenty Raman spectra were randomly obtained per treatment.
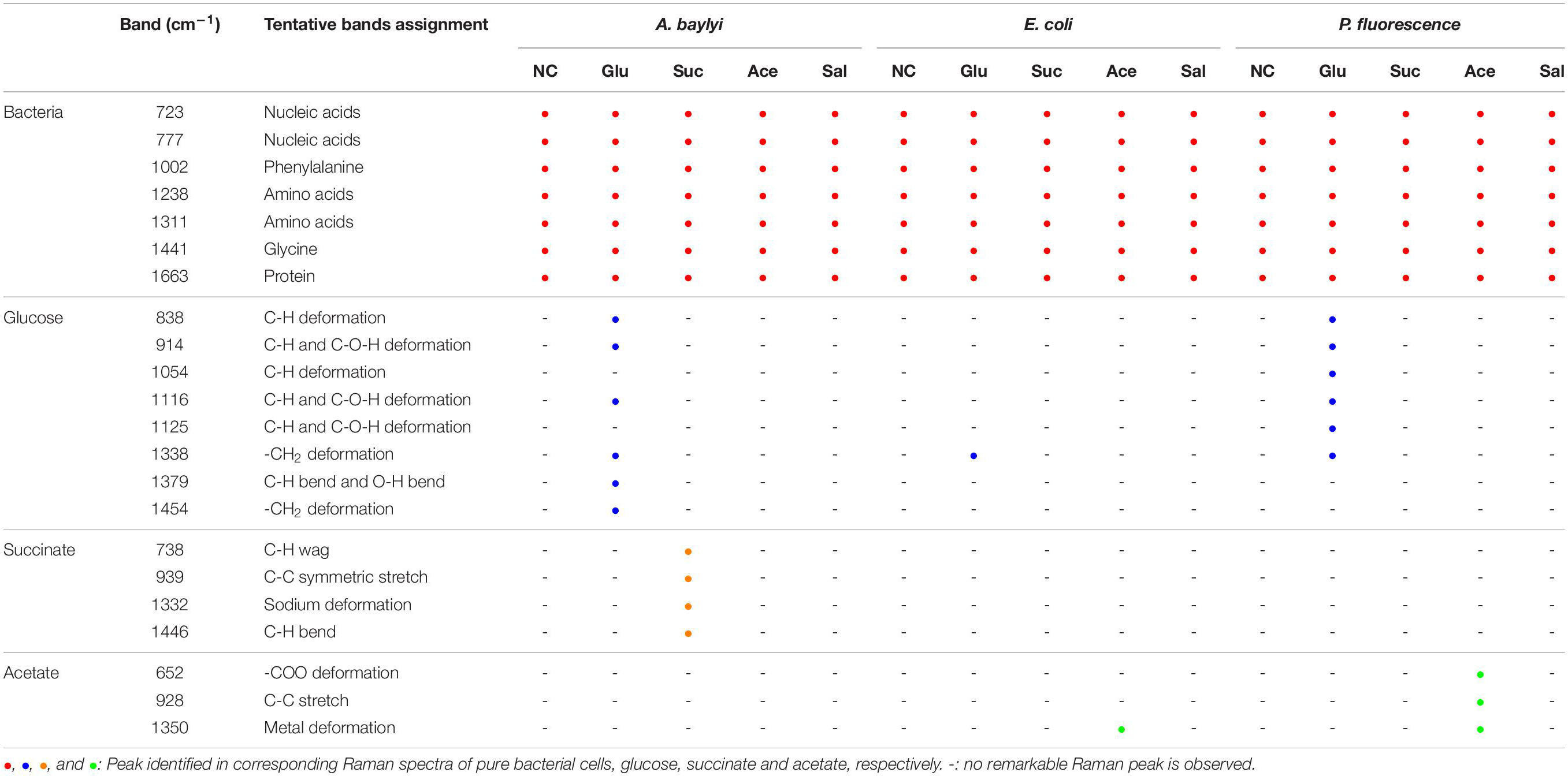
Table 1. Assignments of Raman bands of three bacteria strains pre- and post-exposure to four carbonaceous substances.
Owing to the close genomic and proteomic relationship to the order Pseudomonadales between A. baylyi and P. fluorescence, the Raman spectra of these two bacterial strains are similar (Barbe et al., 2004; Young et al., 2005; Vaneechoutte et al., 2006). However, the transient response of P. fluorescence toward four carbonaceous substances is different from A. baylyi. Significant alterations are found in P. fluorescence post-exposure to acetate and glucose (Table 1). Post-exposure to glucose (Figure 1B), Raman band of 1338 cm–1 attributed to -CH2 deformation is dominant. Additionally, three glucose peaks are detected, occurring at 1125 cm–1 (C-H and C-O-H deformations), 1054 cm–1 (C-H deformation), and 914 cm–1 (C-H and C-O-H deformations) (Vasko et al., 1972; Araujo-Andrade et al., 2005). The remarkable difference in Raman spectral PCA score plots of P. fluorescence between pre- and post-exposure to glucose also proves the high glucose binding affinity of this bacterial strain (Figure 2B). In acetate treatment, the specific 1350 cm–1 band is attributed to sodium deformation (Figure 1B), (Frost and Kloprogge, 2000) and the bands 928 cm–1 (C-C stretch) and 652 cm–1 (-COO deformation) are also apparent (Figure 1B) (Bickley et al., 1990). Figure 2B illustrates the close distance of Raman spectral PCA groups between pure acetate and P. fluorescence post-exposure to acetate, demonstrating the moderate acetate affinity and accumulation. In contrast, no specific Raman spectral biomarker was observed in P. fluorescence post-exposure to salicylate and the PCA score plots suggest a weak attraction of salicylate (Figures 1B, 2B).
Similar to the carbonaceous substance affinity and accumulation in P. fluorescence, E. coli exhibits positive affinity toward glucose and acetate. Some Raman spectral peaks of pure E. coli are identical to those of A. baylyi and P. fluorescence, including 1002 cm–1 (symmetric ring breathing of phenylalanine) (Wang et al., 2011; Li et al., 2017), 1238 and 1331 cm–1 (amino acid) (De Gelder et al., 2007), 1441 cm–1 (-CH2 deformation in glycine) and 1663 cm–1 (C=C stretching of bacterial proteins) (Figure 1C) (De Gelder et al., 2007; Li et al., 2017). The strong glucose affinity of E. coli is highlighted in the PCA score plots, and the -CH2 deformation Raman band at 1338 cm–1 indicates the accumulation of glucose molecules on/inside E. coli cells (Figure 1C and Table 1). Post-exposure to acetate, PCA scores of E. coli are close to that of pure acetate, demonstrating a strong acetate affinity (Figure 2C). In succinate or salicylate treatments, no Raman spectral alteration was found for E. coli, a consequence of weak affinity and accumulation of succinate or salicylate on E. coli, similar to the results in capillary assay.
To quantify the binding affinity and accumulation of different organic carbonaceous substances on/inside bacterial cells via Raman spectra, Dispersion indicator (DI) is employed in this study to represent the dissimilarity between different treatments. Post-exposure to glucose, all the bacterial strains (A. baylyi, P. fluorescence, and E. coli) exhibit positive affinity. The DI values of A. baylyi, P. fluorescence, and E. coli are 0.51, 0.72, and 0.22, respectively (see ESI Supplementary Figure S2). The results indicate moderate to strong binding affinity and cellular accumulation of glucose by all the strains. The Raman spectral DI values are 0.36 and 0.43 for A. baylyi and P. fluorescence post-exposure to succinate, respectively, both higher than that of E. coli (see ESI Supplementary Figure S2). It suggests weak bacterial attraction of succinate toward E. coli. Post-exposure to acetate, the DI values of P. fluorescence and E. coli are both higher than 0.5, indicating their strong acetate affinity. In salicylate treatment, all the DI values of A. baylyi, P. fluorescence, and E. coli are all lower than 0.30 and hint at weak salicylate affinity.
Raman microspectroscopy and capillary assay are different approaches to evaluate bacterial chemotaxis-driven affinity toward organic carbonaceous substances, using the distinct chemotactic indicators of DI and 16S rRNA copies per capillary, respectively. The capillary assay quantifies the chemotaxis-driven movement and accumulation of bacterial strains to carbonaceous substances, whereas Raman spectral alterations illustrate the vibrational fingerprints of carbonaceous substances on/inside bacterial cells caused by binding affinity and accumulation (Li et al., 2017). We therefore generated a semi-log linear regression relationship between DI scores in Raman spectral alterations and 16S rRNA copies in the capillary assay (Figure 3). The semi-log regression coefficient follows the order: A. baylyi (0.422) > P. fluorescence (0.308) > E. coli (0.271), indicating stronger chemotactic sensing and accumulation in A. baylyi and P. fluorescence than in E. coli. The R2 coefficient of determination is 0.5485 for E. coli, 0.6285 for A. baylyi and 0.8388 for P. fluorescence, showing good agreements between DI and 16S rRNA copies/capillary, acquired from Raman spectral alteration and capillary assay, respectively. These results validate the DI model in predicting bacterial chemotactic behavior, and DI is a feasible indicator to quantify carbonaceous substance chemotaxis-related affinity and accumulation on/inside bacterial cells.
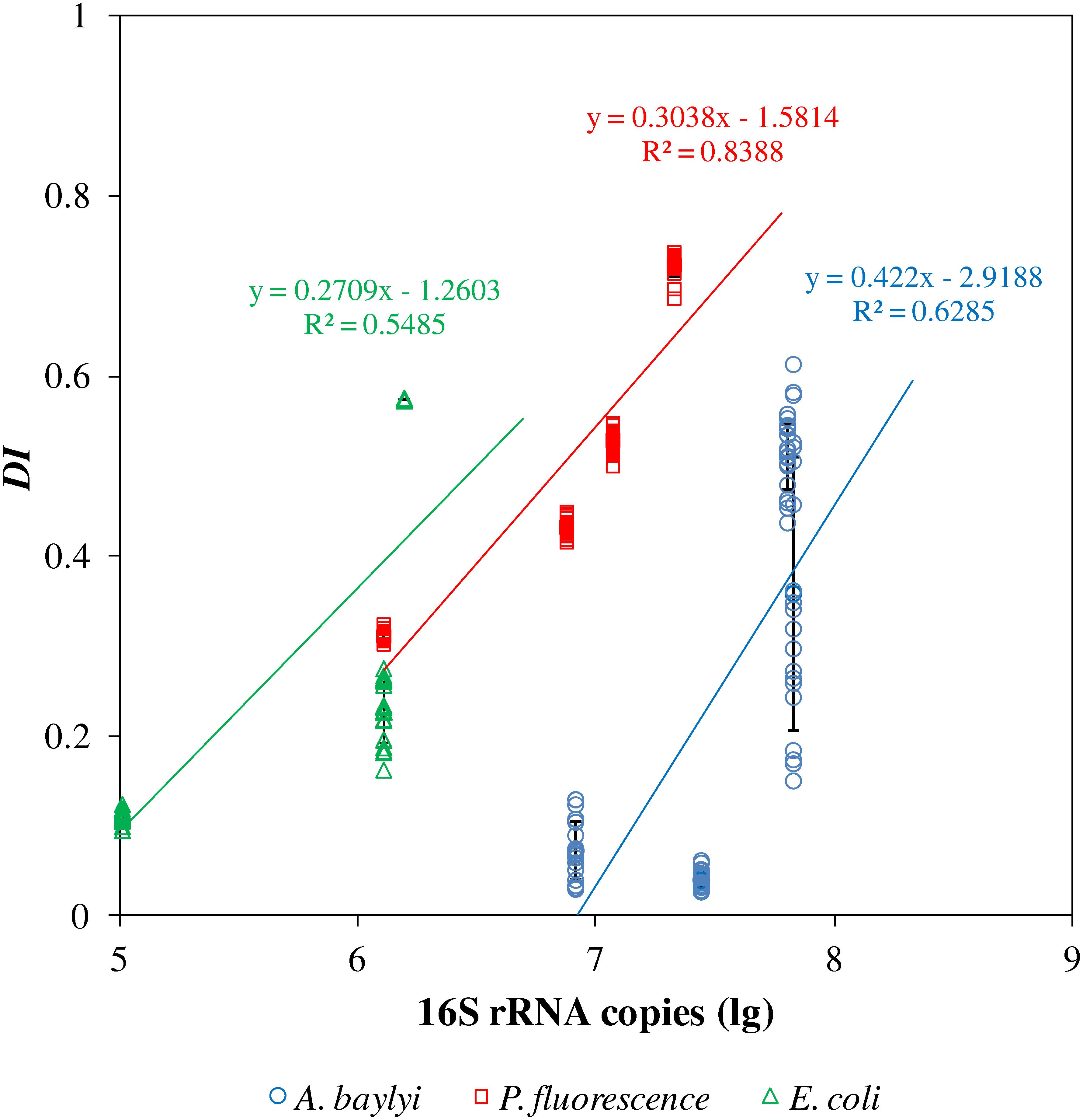
Figure 3. Semi-log linear regression of 16S rRNA copies per capillary and dispersion indicators (DI) of A. baylyi, P. fluorescence and E. coli post-exposure to different organic carbonaceous substances. R2 indicates the consistency of regression of DI and 16S rRNA copies per capillary.
Dose-Responsive Affinity and Accumulation of Carbonaceous Substances
The strong Raman spectral alterations of A. baylyi, P. fluorescence, and E. coli after transient exposure to carbonaceous substances allow one to quantify the binding affinity and cellular accumulation of organic carbonaceous molecules on/inside bacterial cells. The targeted carbonaceous substances are succinate and glucose for A. baylyi, and acetate and glucose for P. fluorescence and E. coli. Glucose had moderate to strong affinity and accumulation in the cells of all three strains. Thus, the intensity of characteristic peaks increased with glucose concentrations in all the treatments. The band at 1338 cm–1 (methylene deformation) was found in all the Raman spectra of A. baylyi post-exposure to glucose (see ESI Supplementary Figure S3). The Raman band of C-H and C-O-H deformation at 1116 cm–1 was positively correlated to the concentration of glucose in A. baylyi and P. fluorescence. Raman bands at 914 and 838 cm–1 bands assigned to C-H deformation were found in A. baylyi or P. fluorescence cells after transient exposure to 10-mM glucose. The DI score plots (Figures 4A,C,E) illustrate a significant discrimination between the groups of A. baylyi, P. fluorescence, or E. coli post-exposure to 1, 5, or 10 mM glucose. For all the three strains, the group of 1 mM glucose was significantly segregated from the negative control (0 mM glucose). The DI of E. coli post-exposure to 5 mM glucose was 0.073, of no difference with the 10 mM glucose treatment (p > 0.05). The results indicate a sensitive response of E. coli toward glucose, but the affinity and accumulation was weak at higher concentrations. In contrast, the DI values of A. baylyi or P. fluorescence post-exposure to glucose constantly increased with glucose concentrations (Figures 4A,C), owing to the increasing glucose molecules accumulated on or inside A. baylyi or P. fluorescence cells. As a classic chemo-attractant, glucose-binding affinity to MCPs is well-documented (Hazelbauer et al., 1969; Singh and Arora, 2001; Weert et al., 2002). Bacterial chemotaxis is a significant step for binding affinity and cellular accumulation of glucose molecules. MCPs mediate the sensitivity of chemotaxis-driven affinity via a two-component regulatory system comprising a sensor kinase CheA and a response regulator CheY (Weert et al., 2002; Sourjik, 2004). As the transmembrane signal transducers triggered by the concentrations of extracellular glucose, MCPs are responsible for glucose recognition and accumulation on bacterial cells (Aizawa et al., 2000; Weert et al., 2002). Increasing Raman spectral alterations are therefore measurable at higher concentration of glucose and quantified by DI scores.
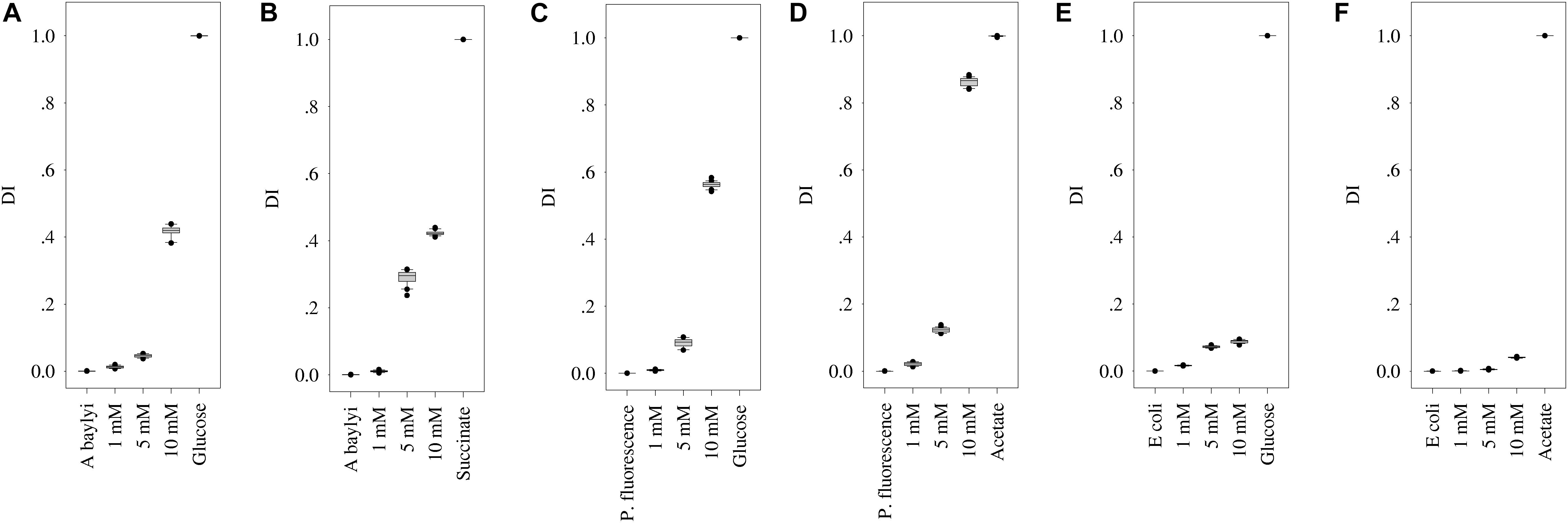
Figure 4. DI score plots of bacterial Raman spectra after transient exposure to different concentrations of organic carbonaceous substances. (A,B) for DI of A. baylyi cells post-exposure to glucose and succinate; (C,D) for DI of P. fluorescence post-exposure to glucose and acetate; (E,F) for DI of E. coli post-exposure to glucose and acetate. Twenty Raman spectra were randomly obtained per treatment.
For A. baylyi cells, we also observed the high binding affinity and cellular accumulation of succinate via Raman spectral alterations. The increasing concentrations of succinate raised the sodium deformation band at 1332 cm–1, and the Raman band of C-C symmetric stretch at 939 cm–1 was detectable when succinate concentration was >1 mM (see ESI Supplementary Figure S3). The 738 cm–1 band in A. baylyi post-exposure to 10 mM succinate was assigned to C-H wag. The DI score plot illustrates that the group of A. baylyi post-exposure to 1 mM succinate were separated from negative control group (0 mM), confirming their highly sensitive affinity and accumulation of succinate (Figure 4B). Additionally, the DI value of A. baylyi post-exposure to 5 mM succinate reached 0.29, significantly higher than that in 5 mM glucose treatment. It is reported that A. baylyi cells preferentially sense and accumulate straight chain dicarboxylic carbonaceous substances like succinate (Baumann et al., 1968; Parke et al., 2001). Therefore, succinate exhibits a higher attraction to A. baylyi especially at higher concentrations.
Post-exposed to acetate, Raman spectral alterations were detectable at 928 cm–1 (C-C stretch) in P. fluorescence and E. coli. The Raman bands at 652 cm–1 (sodium deformation) and 1350 cm–1 (carboxylic deformation) dominated the spectra of P. fluorescence post-exposure to 5 or 10 mM acetate (ESI Supplementary Figure S3). Figure 4D illustrates the increasing DI values with acetate concentrations, from 0.021 (1 mM) to 0.862 (10 mM). It indicates the strong response and binding affinity of P. fluorescence to acetate. The DI value of P. fluorescence post-exposure 10 mM acetate was much higher than that in 10 mM glucose (Figure 4D), although there was no significant difference between the categories of 5 mM acetate (0.09) and glucose (0.12). In addition, the DI group of P. fluorescence in 1 mM acetate treatment was entirely discriminated from that of the negative control (Figure 4D), but not for E. coli (Figure 4F). Only 10 mM acetate exhibits obvious attraction to E. coli from the DI score plot. The results suggest that P. fluorescence could recognize and accumulate 1 mM acetate, but the response sensitivity of E. coli toward acetate was 5 mM. Some previous studies have reported the strong acetate binding affinity in P. fluorescence, and the weak sensing and accumulation of acetate molecules in E. coli is attributed to the prolonged physiological adaptation in mineral medium (Paliy et al., 2003; Paliy and Gunasekera, 2007). Due to the low concentration of bivalent iron in mineral medium, the synthesis of enzymes is insufficient for acetate accumulation and uptake in E. coli.
Selective Affinity and Accumulation Toward Carbonaceous Substance Mixtures
Different to conventional capillary assay, which evaluates the movement and accumulation of bacterial cells toward carbonaceous substances, Raman microspectroscopy characterizes the chemotaxis-driven affinity, accumulation and utilization of organic carbonaceous substances on/inside bacterial cells via biospectral fingerprints. It allows one to distinguish which carbonaceous substance is selectively sensed and accumulated by bacterial cells and to discriminate the bacterial competitive affinity toward the mixtures of various carbonaceous substances. Figure 5 illustrates the LDA score plots of bacterial Raman spectra after transient exposure to individual and multiple carbonaceous substances, successfully evaluating the bacterial selective affinity and accumulation of individual carbonaceous substance in complicated nutrient environments.
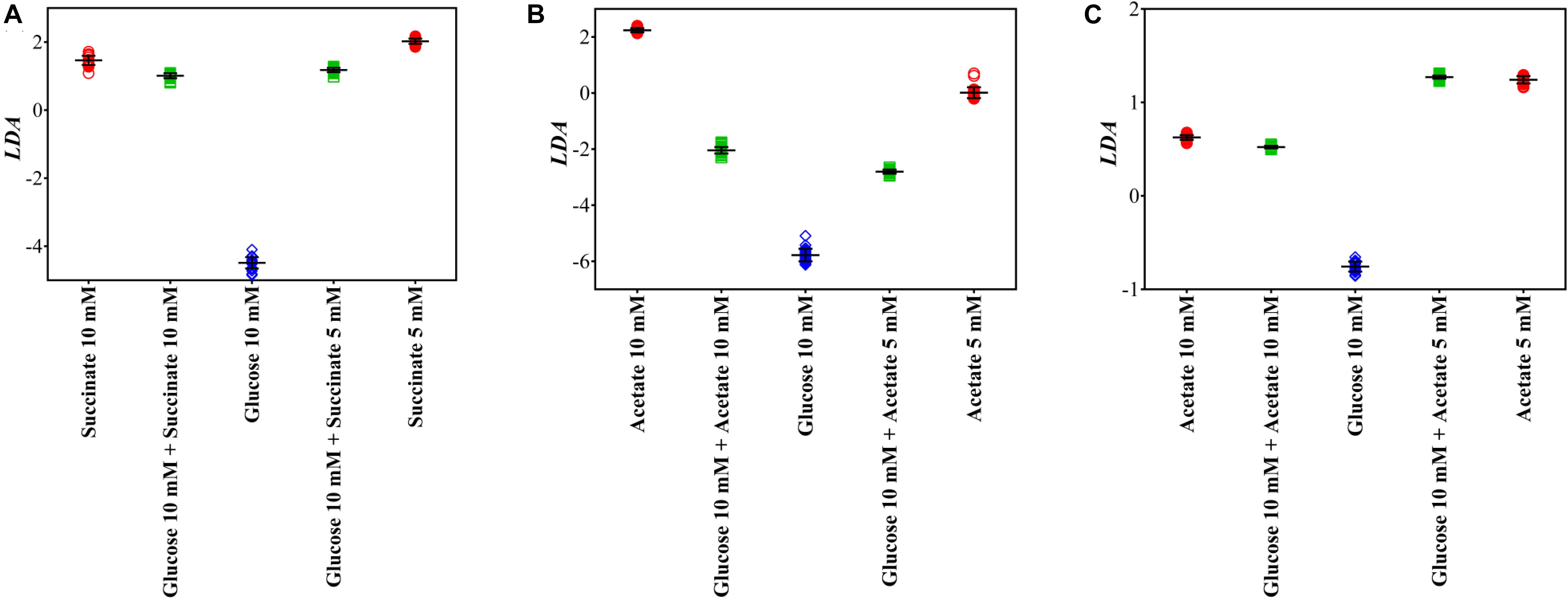
Figure 5. LDA score plots of A. baylyi, P. fluorescence, and E. coli after transient exposure to individual and multiple carbonaceous substances. (A) A. baylyi post-exposure to 10 mM succinate, 5 mM succinate, 10 mM glucose, and glucose-succinate (10 mM + 5 mM and 10 mM + 10 mM); (B) P. fluorescence post-exposure to 10 mM acetate, 5 mM acetate, 10 mM glucose, and glucose-acetate (10 mM + 5 mM and 10 mM + 10 mM); (C) E. coli post-exposure to 10 mM acetate, 5 mM acetate, 10 mM glucose, and glucose-acetate (10 mM + 5 mM and 10 mM + 10 mM).
Post-exposure to the glucose-succinate mixture (10 mM + 5 mM and 10 mM + 10 mM), the LDA categorization of A. baylyi cells is close to the groups of the same succinate concentration but markedly separated from the group of 10 mM glucose (Figure 5A). The difference in LDA position between LDA scores of multiple and individual carbonaceous substance treatments illustrates that only a small proportion of glucose molecules are sensed and accumulated on A. baylyi cells in the presence of both glucose and succinate (Figure 5A). Despite the decreasing concentration of succinate from 10 to 5 mM in the mixture, A. baylyi retains high binding affinity and accumulation of succinate. Namely, the molar ratio of succinate to glucose does not affect bacterial selectivity and the majority of A. baylyi cells have stronger binding affinity toward succinate. This result is consistent with the DI values of A. baylyi post-exposure to individual glucose or succinate. A. baylyi cells show noticeable affinity toward 5 mM succinate (Figure 4B), whereas the significant attraction of glucose is only detectable at 10 mM (Figure 4A). It hints a stronger succinate binding affinity than glucose, and succinate is selectively sensed and accumulated in A. baylyi in succinate-glucose regardless of the occurrence of glucose. Although the MCPs of A. baylyi can recognize and bind glucose molecules, they are reported to have stronger binding affinity toward succinate (Juni, 1978; Young et al., 2005; Wang et al., 2013). The higher succinate binding affinity up-regulates the chemotaxis signal transduction and promotes the activities of CheA autophosphorylation (Garrity and Ordal, 1997). As a result, the movement of A. baylyi cells is more positively promoted toward succinate than glucose, leading to the increasing and predominant affinity and accumulation of succinate.
From the LDA plots of P. fluorescence post-exposure to acetate or/and glucose (Figure 5B), LDA categorization of the glucose-acetate mixture groups, either 10 mM + 5 mM or 10 mM + 10 mM treatment, are both located with the same distance between the groups of individual acetate and glucose. Meanwhile, higher acetate concentration (10 mM) in the mixture does not significantly increase the selective affinity and accumulation of P. fluorescence toward acetate (Figure 5B). The results demonstrate a neutral competitive affinity and accumulation toward acetate and glucose by P. fluorescence. Compared to the results in DI score plots (Figures 4C,D) that the chemotactic behaviors of P. fluorescence are similar post-exposure to 5 mM acetate or glucose but significantly stronger for 10 mM acetate than 10 mM glucose, the neutral selectivity suggests different chemoreceptors for acetate and glucose in P. fluorescence. Previous studies report that Pseudomonas sp. have diverse MCP-like proteins and are strongly chemotactic toward a variety of attractants (Ramos, 2004). The chemoreceptor for acetate in Pseudomonas strains is McpS, a cluster II ligand binding region for TCA cycle intermediates (Lacal et al., 2011; Pineda-Molina et al., 2012; Sampedro et al., 2015). This acetate chemotaxis protein is different from that for glucose, but following the same downstream signal transduction pathway in P. fluorescence (Weert et al., 2002; Sampedro et al., 2015). Thus, post-exposure to acetate-glucose mixture, different MCPs exhibit limited effects on the chemotaxis signal transduction of other chemo-attractants.
Post-exposure to glucose or acetate, Raman spectral alterations show almost the same binding affinity and accumulation of these two carbonaceous molecules on E. coli cells from DI score plots (Figures 4E,F). We therefore speculate a similar LDA categorization of E. coli in glucose-acetate mixture comparing to that of P. fluorescence. However, Raman spectra LDA score plots illustrate that groups of E. coli treated with two acetate-glucose mixtures are remarkably close to those in individual acetate treatments (5 and 10 mM, Figure 5C), indicating a strong selection toward acetate rather than glucose. Additionally, the concentration of acetate does not affect the selective affinity, and there is a significant difference in Raman spectral LDA score plot for the two groups between acetate-glucose mixtures with 5 and 10 mM acetate. It is obvious that, post-exposure to glucose-acetate mixtures, E. coli exhibits a more sensitive response to acetate than glucose. The difference between individual and multiple exposure illustrates the distinct selective behaviors of E. coli cells in a complex carbonaceous substance circumstance. Namely, although E. coli has moderate chemotaxis-driven affinity and accumulation of glucose, similar as acetate, the glucose sensing is strongly inhibited by the presence of acetate. In E. coli cells, strong binding affinity decreases CheA autophosphorylation and weakens the chemotaxis signal transduction (Borkovich et al., 1989). In contrast, acetate can activate CheY phosphorylation via acetylation and promote E. coli chemotaxis (Dailey and Berg, 1993). Although acetylation is not essential for chemotaxis, it can offset the negative effects of CheA autophosphorylation in acetate-glucose mixture, and as a result, encourages acetate affinity and accumulation. Thus, increasing Raman spectral biomarkers of acetate on E. coli cells are identified post-exposure to acetate-glucose mixture, and our work for the first time successfully observes the significantly selective chemotaxis-driven affinity and accumulation between two carbonaceous substances with similar chemotactic behavior.
Raman spectra can measure bacterial binding affinity to individual carbonaceous substance and can also identify which molecule is sensed and accumulated on bacterial cells in a carbonaceous substance mixture. Our results suggest that Raman microspectroscopy is an effective tool in discriminating biospectral fingerprints and revealing the mechanisms of bacterial selective affinity and accumulation in complex nutrient environments. To our knowledge, and compare with the results of capillary assay, we believe the selective affinity and accumulation of bacteria toward the mixture of carbonaceous substances is related to the chemotactic regulatory mechanism. Comparing the different behaviors of the three bacterial strains toward individual or carbonaceous substance mixtures, we find three different types of chemo-attractants with distinct roles in MCPs recognition and signal transduction. When the signal transduction is not regulated by MCPs recognition and affinity, P. fluorescence toward glucose and acetate (neutral-chemo-attractant) as an example, there is no significant difference between individual and multiple carbonaceous substances. In the case of up-regulating chemotaxis signal transduction by MCPs recognition and affinity (succinate as active-chemo-attractant for A. baylyi), bacterial cells have strong selectivity and preferentially swim toward and accumulate active-chemo-attractant post-exposure to multiple carbonaceous substances. Conversely, if MCPs recognition and affinity down-regulates the signal transduction, like E. coli toward glucose as an inactive-chemo-attractant, bacterial binding affinity will prefer other types of chemo-attractants and less inactive-chemo-attractant is sensed. Bacterial chemotaxis is a complex biological behavior in seeking out and accessing chemical stimuli, and both chemo-attractant sensing and signal transduction play important roles in chemotactic behavior. As the chemoreceptor protein, MCPs are able to recognize the periplasmic cognate ligands and deliver the adaptive response through methylation and demethylation (Ferrández et al., 2002). The signal is subsequently transmitted to regulate CheA kinase, CheW scaffolding protein and CheY signal transmission protein to control flagellar motor response (Wadhams and Armitage, 2004; Mukherjee et al., 2016). Some mechanisms of chemotaxis signal transduction pathways have been discussed for several bacterial strains (Borkovich et al., 1989; Garrity and Ordal, 1997; Sampedro et al., 2015; Mukherjee et al., 2016). CheA autophosphorylation and CheY phosphorylation regulate flagella rotation, leading to the change in swimming direction or a tumble (Welch et al., 1993; Toker and Macnab, 1997; Wadhams and Armitage, 2004). Our results from Raman spectral alterations and multivariate analysis suggest diverse mechanisms of bacterial chemotactic selectivity, varying across bacterial species and carbonaceous substances. Post-exposure to multiple carbonaceous substances, the chemo-affinity and chemotaxis signal transduction both play remarkable roles in the bacterial chemotactic behavior (Sampedro et al., 2015). Raman microspectroscopy allows one to elucidate the possible mechanisms of cell-molecule interaction via distinguishing specific molecular biomarkers, especially in a complex nutrient environment.
Conclusion
In the present study, we applied Raman microspectroscopy to investigate the transient binding affinity and accumulation of organic carbonaceous substances on/inside bacterial cells after extremely short-term exposure (30 min). To the best of our knowledge, this is the first study successfully discriminating the vibrational fingerprints of carbonaceous substances on/inside bacterial cells and revealing the different mechanisms of selective accumulation and utilization via Raman spectra coupled with multivariate analysis. After transient exposure to carbonaceous substance, Raman spectral alterations can visualize the molecular biomarkers in biospectra and quantify the selective affinity and accumulation of carbonaceous molecules. Furthermore, the selective behaviors uncovered by Raman microspectroscopy provide deeper insights into the complicated association of MCPs recognition and chemotaxis signal transduction in different bacterial strains. The selective affinity and accumulation are important for bacterial access to adequate nutrients in their natural habitats, in which multiple carbonaceous substances co-exist. Our work suggests that Raman microspectroscopy is a robust and sensitive tool, with great potential in diagnosing bacteria-molecule interaction in situ at single cell level. Although the present study only proves the concept, it is feasible for non-destructive detection of living cells by real-time Raman spectroscopy in further study. Discriminating bacterial selective access to carbonaceous molecules in real world scenario, particularly for those uncultivable microbes, also helps our deeper understanding on microbial functions in natural environment.
Data Availability Statement
The datasets analyzed in this manuscript are not publicly available. Requests to access the datasets should be directed to the corresponding author.
Author contributions
DZ and FM designed the study. HL conducted the experiments, interpreted the data, and contributed to the data analysis. HL and DZ wrote the manuscript. FM, KJ, and DZ revised the manuscript.
Funding
The authors would like to thank the National Natural Science Foundation of China (Nos. 41301331 and 41977346) and Lancaster University FST Research Grant for financial support. HL was supported by the China Scholarship Council (CSC). DZ also acknowledges the support of Chinese Government’s Thousand Talents Plan for Young Professionals.
Conflict of Interest
The authors declare that the research was conducted in the absence of any commercial or financial relationships that could be construed as a potential conflict of interest.
Supplementary Material
The Supplementary Material for this article can be found online at: https://www.frontiersin.org/articles/10.3389/fmicb.2019.02215/full#supplementary-material
References
Adler, J. (1973). A method for measuring chemotaxis and use of the method to determine optimum conditions for chemotaxis by Escherichia coli. Microbiology 74, 77–91. doi: 10.1099/00221287-74-1-77
Aizawa, S.-I., Harwood, C. S., and Kadner, R. J. (2000). Signaling components in bacterial locomotion and sensory reception. J. Bacteriol. 182, 1459–1471. doi: 10.1128/jb.182.6.1459-1471.2000
Alexander, R. P., and Zhulin, I. B. (2007). Evolutionary genomics reveals conserved structural determinants of signaling and adaptation in microbial chemoreceptors. Proc. Natl. Acad. Sci. U.S.A. 104, 2885–2890. doi: 10.1073/pnas.0609359104
Ami, D., Mereghetti, P., and Doglia, S. M. (2013). “Multivariate analysis for fourier transform infrared spectra of complex biological systems and processes,” in Multivariate Analysis in Management, Engineering and the Sciences, eds L. Freitas, and A. P. B. R. de Freitas (London: InTechOpen).
Araujo-Andrade, C., Ruiz, F., Martínez-Mendoza, J. R., and Terrones, H. (2005). Infrared and raman spectra, conformational stability, ab initio calculations of structure, and vibrational assignment of α and β glucose. J. Mol. Struct. 714, 143–146. doi: 10.1016/j.theochem.2004.09.041
Barbe, V., Vallenet, D., Fonknechten, N., Kreimeyer, A., Oztas, S., Labarre, L., et al. (2004). Unique features revealed by the genome sequence of acinetobacter sp. ADP1, a versatile and naturally transformation competent bacterium. Nucleic Acids Res. 32, 5766–5779. doi: 10.1093/nar/gkh910
Baumann, P., Doudoroff, M., and Stanier, R. (1968). A study of the Moraxella group II. oxidative-negative species (genus acinetobacter). J. Bacteriol. 95, 1520–1541.
Berg, H. C., and Turner, L. (1990). Chemotaxis of bacteria in glass capillary arrays. Escherichia coli, motility, microchannel plate, and light scattering. Biophys. J. 58, 919–930. doi: 10.1016/s0006-3495(90)82436-x
Bickley, R., Edwards, H., Rose, S., and Gustar, R. (1990). A raman spectroscopic study of nickel (II) acetate, Ni (CH3COO) 2 and its aqueous and methanolic solutions. J. Mol. Struct. 238, 15–26. doi: 10.1016/0022-2860(90)85002-z
Block, S. M., Segall, J. E., and Berg, H. C. (1983). Adaptation kinetics in bacterial chemotaxis. J. Bacteriol. 154, 312–323.
Borkovich, K. A., Kaplan, N., Hess, J. F., and Simon, M. I. (1989). Transmembrane signal transduction in bacterial chemotaxis involves ligand-dependent activation of phosphate group transfer. Proc. Natl. Acad. Sci. U.S.A. 86, 1208–1212. doi: 10.1073/pnas.86.4.1208
Butler, H. J., Mcainsh, M. R., Adams, S., and Martin, F. L. (2015). Application of vibrational spectroscopy techniques to non-destructively monitor plant health and development. Anal. Methods 7, 4059–4070. doi: 10.1039/c5ay00377f
Cui, L., Butler, H. J., Martin-Hirsch, P. L., and Martin, F. L. (2016). Aluminium foil as a potential substrate for ATR-FTIR, transflection FTIR or raman spectrochemical analysis of biological specimens. Anal. Methods 8, 481–487. doi: 10.1039/c5ay02638e
Cui, L., Chen, P., Chen, S., Yuan, Z., Yu, C., Ren, B., et al. (2013). In situ study of the antibacterial activity and mechanism of action of silver nanoparticles by surface-enhanced raman spectroscopy. Anal. Chem. 85, 5436–5443. doi: 10.1021/ac400245j
Dailey, F. E., and Berg, H. C. (1993). Change in direction of flagellar rotation in Escherichia coli mediated by acetate kinase. J. Bacteriol. 175, 3236–3239. doi: 10.1128/jb.175.10.3236-3239.1993
De Gelder, J., De Gussem, K., Vandenabeele, P., and Moens, L. (2007). Reference database of raman spectra of biological molecules. J. Raman Spectros. 38, 1133–1147. doi: 10.1002/jrs.1734
Dhanya, V. S., Sudarsanakumar, M. R., Suma, S., Prasanna, S., Rajendra Babu, K., Suresh Kumar, B., et al. (2011). Growth and characterization of a new polymorph of lead succinate: a promising NLO material. J. Cryst. Growth 319, 96–101. doi: 10.1016/j.jcrysgro.2011.01.073
Efrima, S., and Bronk, B. (1998). Silver colloids impregnating or coating bacteria. J. Phys. Chem. B 102, 5947–5950. doi: 10.1021/jp9813903
Efrima, S., and Zeiri, L. (2009). Understanding SERS of bacteria. J. Raman Spectrosc. 40, 277–288. doi: 10.1002/jrs.2121
Ferrández, A., Hawkins, A. C., Summerfield, D. T., and Harwood, C. S. (2002). Cluster II che genes from Pseudomonas aeruginosa are required for an optimal chemotactic response. J. Bacteriol. 184, 4374–4383. doi: 10.1128/jb.184.16.4374-4383.2002
Frost, R., and Kloprogge, J. (2000). Raman spectroscopy of the acetates of sodium, potassium and magnesium at liquid nitrogen temperature. J. Mol. Struct. 526, 131–141. doi: 10.1016/s0022-2860(00)00460-9
Garrity, L. F., and Ordal, G. W. (1997). Activation of the CheA kinase by asparagine in bacillus subtilis chemotaxis. Microbiology 143, 2945–2951. doi: 10.1099/00221287-143-9-2945
Guzelian, A. A., Sylvia, J. M., Janni, J. A., Clauson, S. L., and Spencer, K. M. (2002). “SERS of whole-cell bacteria and trace levels of biological molecules,” in Proceedings of the SPIE 4577, Vibrational Spectroscopy-Based Sensor Systems, Boston, MA.
Hazelbauer, G. L., Mesibov, R. E., and Adler, J. (1969). Escherichia coli mutants defective in chemotaxis toward specific chemicals. Proc. Natl. Acad. Sci. U.S.A. 64, 1300–1307. doi: 10.1073/pnas.64.4.1300
Henrichsen, J. (1972). Bacterial surface translocation: a survey and a classification. Bacteriol. Rev. 36:478.
Jia, J., Li, H., Zong, S., Jiang, B., Li, G., Ejenavi, O., et al. (2016). Magnet bioreporter device for ecological toxicity assessment on heavy metal contamination of coal cinder sites. Sens. Actuators B Chem. 222, 290–299. doi: 10.1016/j.snb.2015.08.110
Jin, N., Paraskevaidi, M., Semple, K. T., Martin, F. L., and Zhang, D. Y. (2017a). Infrared spectroscopy coupled with a dispersion model for quantifying the real-time dynamics of kanamycin resistance in artificial microbiota. Anal. Chem. 89, 9814–9821. doi: 10.1021/acs.analchem.7b01765
Jin, N., Zhang, D., and Martin, F. L. (2017b). Fingerprinting microbiomes towards screening for microbial antibiotic resistance. Integr. Biol. 9, 406–417. doi: 10.1039/c7ib00009j
Jin, N., Semple, K. T., Jiang, L., Luo, C., Martin, F. L., and Zhang, D. (2018). Spectrochemical determination of unique bacterial responses following long-term low-level exposure to antimicrobials. Anal. Methods 10, 1602–1611. doi: 10.1039/c8ay00011e
Juni, E. (1978). Genetics and physiology of acinetobacter. Annu. Rev. Microbiol. 32, 349–371. doi: 10.1146/annurev.mi.32.100178.002025
Kearns, D. B. (2010). A field guide to bacterial swarming motility. Nat. Rev. Microbiol. 8, 634–644. doi: 10.1038/nrmicro2405
Kirby, J. R. (2009). Chemotaxis-like regulatory systems: unique roles in diverse bacteria. Annu. Rev. Microbiol. 63, 45–59. doi: 10.1146/annurev.micro.091208.073221
Lacal, J., Muñoz-Martínez, F., Reyes-Darías, J. A., Duque, E., Matilla, M., Segura, A., et al. (2011). Bacterial chemotaxis towards aromatic hydrocarbons in Pseudomonas. Environ. Microbiol. 13, 1733–1744. doi: 10.1111/j.1462-2920.2011.02493.x
Li, H., Martin, F. L., and Zhang, D. (2017). Quantification of chemotaxis-related alkane accumulation in acinetobacter baylyi using raman microspectroscopy. Anal. Chem. 89, 3909–3918. doi: 10.1021/acs.analchem.6b02297
Maddock, J. R., and Shapiro, L. (1993). Polar location of the chemoreceptor complex in the Escherichia coli cell. Science 259, 1717–1717.
Maquelin, K., Choo-Smith, L.-P. I., Van Vreeswijk, T., Endtz, H. P., Smith, B., Bennett, R., et al. (2000). Raman spectroscopic method for identification of clinically relevant microorganisms growing on solid culture medium. Anal. Chem. 72, 12–19. doi: 10.1021/ac991011h
Martin, F. L., German, M. J., Wit, E., Fearn, T., Ragavan, N., and Pollock, H. M. (2007). Identifying variables responsible for clustering in discriminant analysis of data from infrared microspectroscopy of a biological sample. J. Comput. Biol. 14, 1176–1184. doi: 10.1089/cmb.2007.0057
Mukherjee, T., Kumar, D., Burriss, N., Xie, Z., and Alexandre, G. (2016). Azospirillum brasilense chemotaxis depends on two signaling pathways regulating distinct motility parameters. J. Bacteriol. 198, 1764–1772. doi: 10.1128/JB.00020-16
Oku, S., Komatsu, A., Nakashimada, Y., Tajima, T., and Kato, J. (2014). Identification of Pseudomonas fluorescens chemotaxis sensory proteins for malate, succinate, and fumarate, and their involvement in root colonization. Microb. Environ. 29, 413–419. doi: 10.1264/jsme2.ME14128
Paliy, O., Bloor, D., Brockwell, D., Gilbert, P., and Barber, J. (2003). Improved methods of cultivation and production of deuteriated proteins from E. coli strains grown on fully deuteriated minimal medium. J. Appl. Microbiol. 94, 580–586. doi: 10.1046/j.1365-2672.2003.01866.x
Paliy, O., and Gunasekera, T. S. (2007). Growth of E. coli BL21 in minimal media with different gluconeogenic carbon sources and salt contents. Appl. Microbiol. Biotechnol. 73, 1169–1172. doi: 10.1007/s00253-006-0554-8
Parke, D., Garcia, M., and Ornston, L. (2001). Cloning and genetic characterization of dca genes required for β-oxidation of straight-chain dicarboxylic acids in acinetobacter sp. strain ADP1. Appl. Environ. Microbiol. 67, 4817–4827. doi: 10.1128/aem.67.10.4817-4827.2001
Pineda-Molina, E., Reyes-Darias, J.-A., Lacal, J., Ramos, J. L., García-Ruiz, J. M., Gavira, J. A., et al. (2012). Evidence for chemoreceptors with bimodular ligand-binding regions harboring two signal-binding sites. Proc. Natl. Acad. Sci. U.S.A. 109, 18926–18931. doi: 10.1073/pnas.1201400109
Ramos, J. (2004). Pseudomonas, Vol 1. Genomics, Life Style and Molecular Architecture. New York, NY: Kluwer Academic Publishers.
Sampedro, I., Parales, R. E., Krell, T., and Hill, J. E. (2015). Pseudomonas chemotaxis. FEMS Microbiol. Rev. 39, 17–46. doi: 10.1111/1574-6976.12081
Schuster, K. C., Urlaub, E., and Gapes, J. (2000). Single-cell analysis of bacteria by Raman microscopy: spectral information on the chemical composition of cells and on the heterogeneity in a culture. J. Microbiol. Methods 42, 29–38. doi: 10.1016/s0167-7012(00)00169-x
Shi, L. Z., Nascimento, J., Chandsawangbhuwana, C., Berns, M. W., and Botvinick, E. L. (2006). Real-time automated tracking and trapping system for sperm. Microsc. Res. Tech. 69, 894–902. doi: 10.1002/jemt.20359
Singh, T., and Arora, D. K. (2001). Motility and chemotactic response of Pseudomonas fluorescens toward chemoattractants present in the exudate of Macrophomina phaseolina. Microbiol. Res. 156, 343–351. doi: 10.1078/0944-5013-00119
Sly, L. M., Worobec, E. A., Perkins, R. E., and Phibbs, P. V. Jr. (1993). Reconstitution of glucose uptake and chemotaxis in Pseudomonas aeruginosa glucose transport defective mutants. Can. J. Microbiol. 39, 1079–1083. doi: 10.1139/m93-163
Sourjik, V. (2004). Receptor clustering and signal processing in E. coli chemotaxis. Trends Microbiol. 12, 569–576. doi: 10.1016/j.tim.2004.10.003
Sourjik, V., and Berg, H. C. (2000). Localization of components of the chemotaxis machinery of Escherichia coli using fluorescent protein fusions. Mol. Microbiol. 37, 740–751. doi: 10.1046/j.1365-2958.2000.02044.x
Sourjik, V., and Wingreen, N. S. (2012). Responding to chemical gradients: bacterial chemotaxis. Curr. Opin. Cell Biol. 24, 262–268. doi: 10.1016/j.ceb.2011.11.008
Tao, Y., Wang, Y., Huang, S., Zhu, P., Huang, W. E., Ling, J., et al. (2017). Metabolic-activity-based assessment of antimicrobial effects by d2o-labeled single-cell raman microspectroscopy. Anal. Chem. 89, 4108–4115. doi: 10.1021/acs.analchem.6b05051
Toker, A. S., and Macnab, R. M. (1997). Distinct regions of bacterial flagellar switch protein FliM interact with FliG, FliN and CheY. J. Mol. Biol. 273, 623–634. doi: 10.1006/jmbi.1997.1335
Trevisan, J., Angelov, P. P., Scott, A. D., Carmichael, P. L., and Martin, F. L. (2013). IRootLab: a free and open-source MATLAB toolbox for vibrational biospectroscopy data analysis. Bioinformatics 29, 1095–1097. doi: 10.1093/bioinformatics/btt084
Vaneechoutte, M., Young, D. M., Ornston, L. N., De Baere, T., Nemec, A., Van Der Reijden, T., et al. (2006). Naturally transformable acinetobacter sp. strain ADP1 belongs to the newly described species acinetobacter baylyi. Appl. Environ. Microbiol. 72, 932–936. doi: 10.1128/aem.72.1.932-936.2006
Vasko, P., Blackwell, J., and Koenig, J. (1972). Infrared and raman spectroscopy of carbohydrates.: Part II: normal coordinate analysis of α-D-glucose. Carbohydr. Res. 23, 407–416.
Wadhams, G. H., and Armitage, J. P. (2004). Making sense of it all: bacterial chemotaxis. Nat. Rev. Mol. Cell Biol. 5, 1024–1037. doi: 10.1038/nrm1524
Wang, W., and Shao, Z. (2014). The long-chain alkane metabolism network of alcanivorax dieselolei. Nat. Commun. 5:5755. doi: 10.1038/ncomms6755
Wang, X. B., Nie, Y., Tang, Y. Q., Wu, G., and Wu, X. L. (2013). n-Alkane chain length alters dietzia sp. strain DQ12-45-1b biosurfactant production and cell surface activity. Appl. Environ. Microbiol. 79, 400–402. doi: 10.1128/AEM.02497-12
Wang, Y., Ravindranath, S., and Irudayaraj, J. (2011). Separation and detection of multiple pathogens in a food matrix by magnetic SERS nanoprobes. Anal. Bioanal. Chem. 399, 1271–1278. doi: 10.1007/s00216-010-4453-6
Weert, S. D., Vermeiren, H., Mulders, I. H. M., Kuiper, I., Hendrickx, N., Bloemberg, G. V., et al. (2002). Flagella-driven chemotaxis towards exudate components is an important trait for tomato root colonization by pseudomonas fluorescens. Mol. Plant Microbe. Int. 15, 1173–1180. doi: 10.1094/mpmi.2002.15.11.1173
Welch, M., Oosawa, K., Aizawa, S.-L., and Eisenbach, M. (1993). Phosphorylation-dependent binding of a signal molecule to the flagellar switch of bacteria. Proc. Natl. Acad. Sci. U.S.A. 90, 8787–8791. doi: 10.1073/pnas.90.19.8787
Young, D. M., Parke, D., and Ornston, L. N. (2005). Opportunities for genetic investigation afforded by acinetobacter baylyi, a nutritionally versatile bacterial species that is highly competent for natural transformation. Annu. Rev. Microbiol. 59, 519–551. doi: 10.1146/annurev.micro.59.051905.105823
Keywords: Acinetobacter baylyi, Pseudomonas fluorescence, Escherichia coli, chemotaxis, transient selectivity, Raman microspectroscopy
Citation: Li H, Martin FL, Jones KC and Zhang D (2019) Interrogating the Transient Selectivity of Bacterial Chemotaxis-Driven Affinity and Accumulation of Carbonaceous Substances via Raman Microspectroscopy. Front. Microbiol. 10:2215. doi: 10.3389/fmicb.2019.02215
Received: 08 May 2019; Accepted: 10 September 2019;
Published: 04 October 2019.
Edited by:
Marina G. Kalyuzhanaya, San Diego State University, United StatesReviewed by:
Wenbin Du, Chinese Academy of Sciences, ChinaJose Ruben Morones-Ramirez, Universidad Autónoma de Nuevo León, Mexico
Copyright © 2019 Li, Martin, Jones and Zhang. This is an open-access article distributed under the terms of the Creative Commons Attribution License (CC BY). The use, distribution or reproduction in other forums is permitted, provided the original author(s) and the copyright owner(s) are credited and that the original publication in this journal is cited, in accordance with accepted academic practice. No use, distribution or reproduction is permitted which does not comply with these terms.
*Correspondence: Dayi Zhang, zhangdayi@tsinghua.org.cn; zhangdayi@tsinghua.edu.cn