- 1Microbes in Food Chain, Quadram Institute Biosciences, Norwich Research Park, Norwich, United Kingdom
- 2SequenceAnalysis.co.uk, NRP Innovation Centre, Norwich, United Kingdom
- 3University of East Anglia, Norwich, United Kingdom
- 4Department of Veterinary Medicine, University of Cambridge, Cambridge, United Kingdom
- 5London School of Hygiene and Tropical Medicine, University of London, London, United Kingdom
- 6Cell Systems Modelling Group, Oxford Brookes University, Oxford, United Kingdom
Campylobacter jejuni, the most frequent cause of food-borne bacterial gastroenteritis, is a fastidious organism when grown in the laboratory. Oxygen is required for growth, despite the presence of the metabolic mechanism for anaerobic respiration. Amino acid auxotrophies are variably reported and energy metabolism can occur through several electron donor/acceptor combinations. Overall, the picture is one of a flexible, but vulnerable metabolism. To understand Campylobacter metabolism, we have constructed a fully curated, metabolic model for the reference organism M1 (our variant is M1cam) and validated it through laboratory experiments. Our results show that M1cam is auxotrophic for methionine, niacinamide, and pantothenate. There are complete biosynthesis pathways for all amino acids except methionine and it can produce energy, but not biomass, in the absence of oxygen. M1cam will grow in DMEM/F-12 defined media but not in the previously published Campylobacter specific defined media tested. Using the model, we identified potential auxotrophies and substrates that may improve growth. With this information, we designed simple defined media containing inorganic salts, the auxotrophic substrates, L-methionine, niacinamide, and pantothenate, pyruvate and additional amino acids L-cysteine, L-serine, and L-glutamine for growth enhancement. Our defined media supports a 1.75-fold higher growth rate than Brucella broth after 48 h at 37°C and sustains the growth of other Campylobacter jejuni strains. This media can be used to design reproducible assays that can help in better understanding the adaptation, stress resistance, and the virulence mechanisms of this pathogen. We have shown that with a well-curated metabolic model it is possible to design a media to grow this fastidious organism. This has implications for the investigation of new Campylobacter species defined through metagenomics, such as C. infans.
1. Introduction
1.1. Background
Campylobacter, the leading cause of acute bacterial gastroenteritis, is Gram-negative, microaerophilic, spiral-shaped, highly motile bacteria (Debruyne et al., 2008; Lastovica et al., 2014). The epidemiology in low to middle income countries is poorly studied but appears to be different from that in high-income countries (Platts-Mills and Kosek, 2014). Acquisition in high income countries is mainly through ingestion of contaminated poultry, milk, or water (Altekruse et al., 1998; Butzler, 2004; Ruiz-Palacios, 2007; Westrell et al., 2009). The genus Campylobacter can be divided into 28 species (Wilkinson et al., 2018) (and further into sub-species), that may vary in their temperature, oxygen, and nutrient requirements for growth. The most common species associated with campylobacteriosis is Campylobacter jejuni, with Campylobacter coli reported in 10–25% of cases. The disease is economically important, with an estimated cost to the UK economy at around £50 million (Tam and O'Brien, 2016). Under reporting of cases (Gibbons et al., 2014) and failure to consider sequelae (Mangen et al., 2016), however, makes this an underestimate. Disease symptoms in humans may vary, but in the UK, they include diarrhea, fever, and abdominal pain. The sequelae however include colitis, reactive arthritis, and Miller-Fisher and Guillain-Barré syndromes (Jacobs et al., 1996; Blaser and Engberg, 2008). Along with an increase in reported incidence, and improving diagnosis, resistance to antibiotics is becoming a concern (Châtre et al., 2010; Deckert et al., 2010; Gormley et al., 2010). The diagnosis of campylobacteriosis has depended, until recently, on culture-based methods, but these methods may not grow all Campylobacter spp. causing human infections (Buss et al., 2019; Bian et al., 2020). An understanding of how to grow these “non-culturable” Campylobacter spp. may change our understanding of campylobacterosis, particularly in low income countries where the burden is high and predominantly occurs in children (Platts-Mills and Kosek, 2014). The conditions used in diagnostic laboratories vary, but commonly include a gas mix of 5–10% O2, 5–10% CO2 and 80–85% N2 (with some mixes also containing H2), a temperature of 37°C or 42°C, with complex media containing blood, peptone, meat, or yeast extract (Hsieh et al., 2018). These media are not chemically defined, and may vary from batch to batch, making comparisons between strains and the growth conditions used for isolation difficult. The use of recently developed commercial PCR assays have improved diagnosis, but new variants would not be detected. As metagenomic methods are not yet freely available, a more permissive culture media is required for comprehensive burden studies and pathogen discovery.
1.1.1. Growth Culture
Until the introduction of selective agars (Skirrow, 1977; Lauwers et al., 1978), filtration techniques were used to isolate Campylobacter (Dekeyser et al., 1972; Butzler et al., 1973). An understanding of the microaerophilic nature of Campylobacter (Hoffman et al., 1979) improved the culture still further. The development of Campylobacter specific culture media (Corry et al., 1995; Davis and DiRita, 2008; Kim et al., 2016) now represents the state of the art, however, the availability of a permissive defined media for Campylobacter spp. remains elusive. Although defined media have been described (Dickgiesser and Czylwik, 1985; Tenover et al., 1985; Guccione et al., 2010; Alazzam et al., 2011), the use of complex, undefined media (Wright et al., 2009; Liu et al., 2012) persists, because of the need to culture a wide range of Campylobacter strains that demonstrate different auxotrophies (Dickgiesser and Czylwik, 1985; Tenover and Patton, 1987; Alazzam et al., 2011; Vorwerk et al., 2014). A method to investigate the ingredients required for a permissive media is clearly needed. One way to do this is to look at the metabolic requirements of campylobacter cells in terms of nutrient sources, biomass, and energy production.
1.1.2. Campylobacter Metabolism
Campylobacter spp. have considerable flexibility for energy production in terms of electron acceptors and donors that can be used in the electron transport chain (ETC); in addition to the ability to utilize O2 as an electron acceptor, nitrate, nitrite, and fumarate have also been described as alternatives. Campylobacter spp. would therefore appear to have the potential to carry out anaerobic respiration (Sellars et al., 2002; Kelly, 2008), however, many strains do not grow in the absence of oxygen, even in the presence of alternative electron acceptors (Sellars et al., 2002). The reason for this oxygen dependency is not absolutely certain but is likely to be related to oxygen dependent DNA synthesis enzymes (Jordan and Reichard, 1998; Kelly, 2008). The ETC also has a high degree of flexibility in terms of electron donors, with pyruvate, H2, formate, and succinate having been reported to fulfil this role (Sellars et al., 2002; Kelly, 2008). In contrast to the ETC, other areas of central metabolism are quite limited, when compared with that of most other gut bacteria. In particular, they lack transporters for many small carbohydrates, with two essential enzymes in the upper limb of the Embden–Meyerhof–Parnas pathway (glucokinase and phosphofructokinase) being absent. Most strains lack the Entner-Doudoroff pathway, and the oxidative steps of the oxidative pentose phosphate pathway are also absent (although the non-oxidative branch is present Velayudhan and Kelly, 2002; Line et al., 2010). The absence of carbohydrate utilization pathways suggests that amino acids and tricarboxylic acid (TCA) cycle intermediates act as the primary nutrient sources for Campylobacter jejuni(Westfall et al., 1986; Velayudhan et al., 2004; Del Rocio Leon-Kempis et al., 2006; Stahl et al., 2012; Hofreuter, 2014), but detailed knowledge as to how such substrates may be utilized, remains limited. Furthermore, the ability of this organism to survive right through the food chain, suggests it can utilize a variety of metabolic strategies. Our understanding of Campylobacter physiology, thus, remains limited, with most descriptions of Campylobacter physiology being based on a single isolate of Campylobacter jejuni NCTC11168 (Carrillo et al., 2004; Alazzam et al., 2011; Metris et al., 2011; Xu et al., 2015; van der Hooft et al., 2018).
1.2. Metabolic Modeling
Genome-scale metabolic models (GSMs) describe the metabolic interactions of an organism with its environment, based on a reaction network ultimately inferred from enzymes encoded by the genome. Their analyses enable an investigation of metabolic behavior of the whole system, rather than individual reactions and pathways, and can be used to identify mechanistic links between cellular genotype and metabolic phenotype.
Genome-scale metabolic models overview
Over the last two decades, GSMs have become a valuable tool to analyze cellular behavior under different biological conditions, to design drug targets, to investigate metabolic interactions in microbial communities, and to design defined growth media (reviewed in Liu et al., 2010; Zhang and Hua, 2016; Kim et al., 2017; Gu et al., 2019). GSMs can now be used to inform experimental design and to provide a rationale for experimental observations (Zampieri and Sauer, 2016; Villanova et al., 2017; van der Ark et al., 2018).
Construction of GSMs starts with the identification of enzymes and transport proteins from an annotated genome. Reactions catalyzed by enzymes thus identified, are further characterized by their stoichiometry and assumed (ir)reversibility. Such information can usually be obtained from a number of on-line databases including databases such as BioCyc (Caspi et al., 2015; Karp et al., 2017), MetaCyc (Caspi et al., 2013, 2017), KEGG (Ogata et al., 1999; Kanehisa and Goto, 2000; Kanehisa et al., 2006), BIGG (Schellenberger et al., 2009), SEED (DeJongh et al., 2007), and BRENDA (Barthelmes et al., 2007).
In addition to defining the properties of internal reactions, it is, for all practical purposes, necessary to identify the properties of transport processes, representing the consumption and production of compounds in the assumed environment. Compounds present in the environment are referred to as external (or sometimes boundary) metabolites, otherwise they are referred to as internal metabolites. Reactions that only involve internal metabolites are therefore internal reactions, while those which involve internal and external metabolites are transport reactions. Although gene annotation with regard to enzymes is usually well-defined, annotation of transporters tends to be less comprehensive. Experimental investigations involving growth on defined media thus proves a useful independent method to infer the presence of transport processes, as well as providing useful data for subsequent analyses of the model.
Despite the fundamental importance of online databases, any reconstruction based solely upon these is likely to exhibit a number of problems, the most common of which are: missing gene-protein-reaction (GPR) associations, inconsistent naming of metabolites, and reaction identifiers, incorrect reaction stoichiometries, and reversibility, which propagates to the draft model. Thus, in order to represent a realistic representation of a given organism, an initial draft model will require refinement and curation by the user. The curation process usually begins with correcting reaction stoichiometries and reversibility to ensure that the model follows the law of mass and energy conservation, followed by checking consistency of identifiers, and identifying missing reactions by “gap-filling.” Such gap-filling may often lead to an updated gene annotation. One area of metabolism that requires special attention is the electron transport chain, to ensure that translocated protons are correctly assigned to their location in the cell or the environment.
Usually, large-scale metabolic models such as GSMs, are analyzed using constraint based linear programming (LP) approaches (Fell and Small, 1986; Varma and Palsson, 1993, 1994), an optimization technique that assigns fluxes to reactions, assuming that the system is at steady state, according to some objective function, and subject to one or more defined constraints. Typical objective functions are either maximization of growth rate, or minimization of total flux in the system, and constraints are used to apply upper or lower limits on reactions, and commonly to specify the export of one or more product as defined.
1.2.1. Aims of the Current Study
The aim of this study is to explore Campylobacter spp. nutrient requirements through the analysis of a newly created genome scale model of Campylobacter jejuni (M1Cam) with experimental validation. In doing so we define new, chemically defined, media compositions that will serve as a basis for further modeling and experimental studies of this organism.
2. Materials and Methods
2.1. Model Construction
The model was developed in an iterative manner, as illustrated in Figure 1, from the available gene sequence of Campylobacter jejuni M1cam (https://www.ncbi.nlm.nih.gov/nuccore/CP012149). Genes were predicted and annotated, and used to construct a strain specific Pathway/Genome database (PGDB) for Campylobacter jejuni M1cam. This was used as the basis for the model which was structured in a modular fashion as described by Poolman et al. (2009), Poolman et al. (2013), Hartman et al. (2014), and Ahmad et al. (2017). It consists of:
1. A “top-level” module defining some basic properties and importing the other modules as described;
2. A “PGDB reactions” module with a set of reactions directly imported from the strain-specific PGDB which was subjected to revision as described in 2.1.3.
3. “Media transporter” module describing the import and export of substrates to/from the medium.
4. “Biomass transporter” module describing the export of biomass precursors and metabolites produced as side products of biomass synthesis for which there are no known degradation pathways, also called “sink transporters.”
5. The ETC module describing stoichiometries of all ETC reactions.
6. Additional reactions required for the generation of biomass precursors, not reported in the PGDB.
2.1.1. Draft Reconstruction
Gene prediction and annotation was carried out using Prodigal (Hyatt et al., 2010) version 2.6.31 and Prokka (Seemann, 2014) version 1.142 respectively, with default cutoff scores.
The “Pathologic component” of Pathway Tools (Karp et al., 2002, 2015) version 23.0 and MetaCyc (Caspi et al., 2017) version 23.0 was used to generate the strain-specific Pathway/Genome databases (PGDB), from the annotated genome sequence. The pathway hole filler component (Green and Karp, 2004) of Pathway Tools was used to automatically fill pathway holes in PGDB. Pathways were inferred using the pathway prediction algorithm (Karp et al., 2011) with a default cutoff score of 0.15. Missing genes and GPR associations (see sections 3.1.1), were curated manually with reference to BLAST (Camacho et al., 2009) and the online databases KEGG (Ogata et al., 1999), Gene Ontology terms (Ashburner et al., 2000), BRENDA (Jeske et al., 2018), Pfam (El-Gebali et al., 2018), RHEA (Morgat et al., 2019), and String v11 accessed 2019 (Szklarczyk et al., 2018).
All the reactions from PGDB were extracted, using the ScrumPy (Poolman, 2006), metabolic modeling package, version 1254, to populate the “PGDB reactions” module described above, and to generate the initial draft model.
2.1.2. Defining System Boundaries
Media transporters were assigned for import of 33 organic substrates, out of 38 organic substrates in the DMEM/F-12 medium (Table S1). Transporters for the remaining five organic substrates, choline chloride, folic acid, lipoic acid, thymidine, and vitamin B12 (cobalamine) were not included because metabolic routes were not present for these metabolites in the PGDB. Transporters were assigned for exchange of the gases O2, CO2, H2, NH4 and H2S between the environment and the system and for the export of metabolic by-products, such as acetate, lactate, and succinate.
Biomass transporters were defined for the export of biomass components as individual components (amino acids, lipopolysacharides, peptidoglycan, nucleotides, vitamins, etc.), as described by Thiele et al. (2005) and Metris et al. (2011). Note that in contrast to these authors who represented biomass as a single lumped reaction, we assigned biomass transporters to individual biomass components, and subsequently set their relative fluxes to represent the observed biomass composition. There are five sink transporters added to the model for export of by-products from biomass synthesis [eg. adenosyl-4-methylthio-2-oxobutanoate produced during biotin synthesis (Metris et al., 2011)].
2.1.3. Curation and Theoretical Validation
The atomic balance of individual reactions were determined and all the reactions in the model were balanced with respect to carbon (C), nitrogen (N), phosphorus (P), and sulfur (S), iron (Fe), sodium (Na), oxygen (O), proton (H), and charge. Metabolites with unknown atomic compositions or generic compounds and reactions involved with such metabolites were replaced, where possible, by balanced reactions with known atomic composition of metabolites. For example, reactions involved with generic compounds (e.g., Alcohols) were replaced with specific metabolites and the stoichiometric coefficient balanced on both sides of the reaction. Reactions involved with non-metabolic species (e.g., tRNA, RNA) were excluded from the model. Similarly, reactions were corrected for reversibility so that the model is not able to generate energy (in the form of ATP and NAD(P)H) in absence of a mass flux through the system. Thus, the model is ensured to follow the laws of mass and energy conservation, and is free from stoichiometric inconsistencies (Gevorgyan et al., 2008).
2.2. Model Analysis
2.2.1. Linear Programming Formulation
Except where noted otherwise, model analysis was undertaken using appropriate variants of the linear program defined as:
Where v is the vector of all reaction fluxes and N is the stoichiometry matrix; the objective is to minimize the sum of all (absolute) flux values (including transporters), subject to the constraints: Nv = 0 (steady-state assumption), vi..j = bi..j defines flux in biomass transporters, as described in section 2.1.2, vk..l defines fluxes in the reactions importing media components, as described in section 2.1.2, vATPase = A defines flux in a hypothetical ATPase reaction in order to account for a growth and non-growth associated maintenance cost and vOtx ≤ Otxmax defines a maximum microaerophilic O2 consumption rate. A was assigned a value of 16 mmol/g DW/hr (Varma and Palsson, 1994), and Otxmax (= 5 mmol/g DW/hr) (Metris et al., 2011).
2.2.2. Identification of Substrate Auxotrophies
Substrate auxotrophies were determined, using the model, by repeatedly attempting to solve Equation (1) with individual media transporters (vk..l) set to zero, one at a time. Failure to obtain a solution was taken as demonstrating auxotrophy with respect to the component whose transporter was thus constrained.
2.3. Model Curation and Refinement From Experimental Observation
Although Campylobacter spp. was successfully cultured in the DMEM/F-12 medium (section 2.4), the initial model could not account for the production of all biomass components from these nutrients. These components were identified by modifying Equation (1) to account for the production of a single biomass component, sequentially. Additional reactions were then identified by their presence in other Campylobacter spp. specific biochemical databases in Biocyc and KEGG, literature surveys, and with reference to BLAST (Camacho et al., 2009). Once identified, these reactions were used to populate the “Additional Reactions” module described above.
Initial model analysis suggested that biotin and asparagine were essential nutrients, although this was found not to be the case in the experimental investigation. This discrepancy was due to gaps in the biosynthesis pathways of biotin and asparagine. Gap-filling reactions were identified, based on a literature study on biotin synthesis (Stok and Voss, 2000; Manandhar and Cronan, 2017) and BlastP analysis, and were added to the “Additional Reactions” module as above.
Conversely, initial model analysis suggested that niacinamide was not an essential nutrient, although subsequent experimental investigation showed that this was not the case. In order to identify spurious reactions in the model responsible for this heterotrophy, Equation (1) was modified to represent production of niacinamide, utilizing the other media components. Reactions in the resulting solution for which no GPR could be identified were removed, thus bringing model behavior into alignment with our experimentally observed behavior.
2.4. Experimental Conditions
2.4.1. Materials
Brucella broth was purchased from Sigma-Aldrich (Sigma-Aldrich, Poole, UK) and Brucella agar from BD (Becton, Dickinson U.K. Limited). Gibco Dulbecco's Modified Eagle Medium/Nutrient Mixture F-12 (DMEM/F-12) media with no added phenol red, was purchased from Thermo Fisher Scientific (Thermo Fisher Scientific, Darmstadt, Germany). All chemicals were of commercial analytical grade and obtained from Sigma-Aldrich. All solutions, except amino acids and vitamins, were made every week, sterile filtered (0.22 μM, Fisher-Scientific, UK), and kept at −4°C until needed. Amino acids and vitamins were prepared immediately before use.
2.4.2. Bacterial Strains Used
Campylobacter jejuni M1 has a published genome sequence (Friis et al., 2010) and is a well-studied laboratory model organism from a well-documented case of direct transmission between poultry and humans. The M1 used in this study was obtained from the Department of Veterinary Medicine, Cambridge University, and annotated as M1cam (de Vries et al., 2015), an “M1” strain which differs from the M1 reference strain (published by Friis et al., 2010) by 50 SNPs (insertions/deletions and true SNPs) (de Vries et al., 2015).
The other 7 strains, selected from different clades, according to Champion et al. (2005) and Stabler et al. (2013), were tested for their ability to grow in the final defined medium. These were: 81116 (NCTC11828), Calf 3, 11168H, 81-176, 12912, RM1221, and M1, obtained from the London School of Hygiene and Tropical Medicine. Strains were stored at −80°C using Protect - Multipurpose Microorganism Preservation System vials (Technical Service Consultants Ltd, Lancashire, UK) or MicrobankTM cryotubes (Pro-Lab Diagnostics, Merseyside, UK).
2.4.3. Culture Conditions
All strains were grown under microaerophilic conditions (10% O2 [v/v], 5% CO2 [v/v], 85% N2 [v/v]), using an anaerobic cabinet and gas supply (BOC Ltd, Surrey, UK), on Brucella broth agar plates, for 48 h at 37°C, except those tested in the Department of Veterinary Medicine, Cambridge University, which were grown at 42°C using a gas mix of hydrogen (5% O2 [v/v], 5% H2 [v/v], 5% CO2 [v/v], 85% N2 [v/v]), also using an anaerobic cabinet and gas supply (BOC Ltd, Surrey, UK).
All Campylobacter jejuni strains were harvested from agar plates and inoculated with a starting optical density (OD) OD600 of approximately 0.004 in Brucella broth, incubated with shaking (180 rpm) using the same temperature and gas supply indicated previously, to an OD600nm of 0.6 (approximately 2.8 × 109 CFU/ml). Cultures with OD600 different to 0.6 were standardized to that value using sterile PBS. After 20 h, 1.7 mL of this culture was collected, washed twice and resuspended in 1.7 mL of sterile PBS. Each one of the media tested in our study was inoculated in triplicate at 1% (100 μL in 10 mL of media), using Campylobacter jejuni washed cells. Cultures were then incubated microaerobically for 48 h with shaking (180 rpm), under the same temperature and gas conditions stated above, and growth was assessed daily, measuring OD600 in triplicate of each of the technical replicates, using a plate reader (FLUOstar® Omega, BMG Labtech Ltd, Aylesbury, UK). For this purpose, cultures were sampled in a class II cabinet and returned immediately to the anaerobic cabinet. All experiments performed in this study were repeated three times, each time using a new starting culture, prepared from glycerol stock, and with freshly made simple defined media.
2.4.4. Checks for Contamination
To confirm the identity of the experimental organisms, typical cellular morphology was checked using x1000 bright field microscopy of carbol fuchsin stained cultures and whole genome sequencing (WGS) was performed on selected isolates. Campylobacter jejuni M1cam genomic DNA for WGS was extracted using Maxwell® RSC Cultured Cells DNA Kit. DNA extracts were converted into a Nextera library for sequencing on an Illumina NextSeq 500 platform and genomes were assembled using Velvet de-novo genomic assembler and then subjected to multi-locus sequence typing (MLST).
2.4.5. Media Design and Confirmation of Auxotrophies
A modification of DMEM/F-12, not including glucose, was used as the starting point to develop our defined media for Campylobacter jejuni M1cam (Table S1). The inorganic substrates in our media were kept in the same concentrations present in the original DMEM/F-12 media formulation. To achieve a simple defined media, non-essential substrates identified by the model analysis, were gradually removed from the media, and growth, measured as OD, was compared to that of growth in Brucella broth (0.58 ± 0.06 at 24 h) and synthetic DMEM/F-12 media (0.38 ± 0.01 at 24 h). Removal of substrates in media design were compensated for the net amount of N and S, by increasing the concentration of remaining substrates proportionally to ensure that the total N and S was equal or higher than the original formulation.
Substrate auxotrophy was confirmed when removal of substrate could not support growth of M1cam. Growth was monitored over longer incubation times (up to 144 h) to confirm auxotrophy of the compounds tested. If no growth was obtained, experiments were repeated, to confirm results.
2.4.6. Media Testing
The media to be tested was made fresh on the day of the inoculation. After addition of all components and before adjusting the final volume to 100 mL using purified water, the pH of each of the minimal media tested in this study was measured using a pH meter [LAQUAtwin pH Meter PH-22 (HORIBA Advanced Techno Co., Ltd., Kyoto, Japan)] and adjusted to pH 7 using 1M NaOH or 1M HCl solutions. To maintain sterility, work was carried out in a class II microbiological safety cabinet, and 10 mL of media was filtered into each of the four 50 mL sterile cell culture flasks (Sigma-Aldrich, Poole, UK), closed with their filter caps and kept at 37°C until inoculation. Maintaining media at 37°C until inoculation proved to be very important to obtain reproducible results. Finally, in order to compare our results with previously published defined media, we also tested growth of Campylobacter jejuni M1cam in the defined media given by Guccione et al. (2010) and Alazzam et al. (2011).
3. Results
3.1. Model Building and Analysis
3.1.1. Pathway/Genome Databases
The automatically generated Campylobacter jejuni M1cam PGDB comprised of 228 pathways with a total 1,366 reactions, including 22 transport reactions, and 1,009 compounds. There were 291 reactions without any assigned gene and were inferred from pathway prediction algorithm. This also included reactions that were essential for biomass productions in the model. There were also genes that encoded metabolic enzymes but were not associated with reactions. The reason for the missing GPR association was mainly: (i) information necessary to attribute a particular reaction to specific gene was missing in the annotated file (ii) though the gene product had been annotated it was not detected by the Pathologic software. Reactions, particularly those inferred as essential for biomass production, without any gene association were revisited to establish GPR relation (see 2.1.1) where possible. Table S2 lists GPR relationships that have been curated in the PGDB. Curated PGDB, after being re-inferred using pathway prediction algorithm, comprises a total 262 pathways, 1,581 reactions, and 1,104 compounds (summarized in Table 1 along with other prokaryotic PGDBs) and is available from Pathway Tools Registry and https://data.quadram.ac.uk/dipali.singh/CJM1cam_DB/.
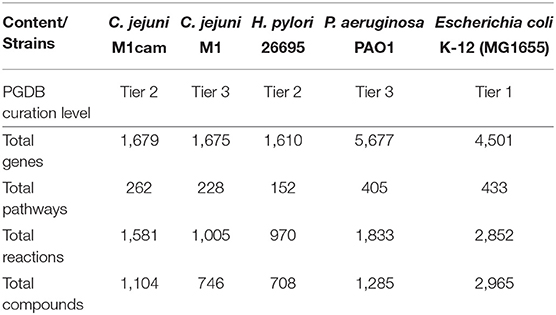
Table 1. Main content of C. jejuni M1cam Pathway/Genome database along with other prokaryotic databases.
3.1.2. Model Properties
The curated model consists of 994 reactions. The number is less than that of the initial draft model generated from the PGDB due to removal of reactions involved with non-metabolic species and those of unknown atomic composition, as described in 2.1.3. 76% of reactions in the model have gene associations, 6% are spontaneous, while the remainder are inferred by the gap filling algorithm or added after careful curation. The model includes 115 transporters, 968 internal metabolites and 93 external metabolites, summarized in Table 2.
3.1.3. Model Analysis—Biomass Production
The solution to Equation (1), with DMEM/F-12 media, has a total of 326 reactions, including transporters. There are 165 essential for biomass production: removal of any one of these results in Equation (1) having no feasible solution. The solution has no net import flux of amino acids, leucine, arginine, histidine, valine, asparagine, phenylalanine, alanine, threonine, tyrosine, tryptophan, and lysine (i.e., flux in these amino acid media transporters were equal to flux in their respective biomass transporters). There was, however, notable net import of pyruvate, as a carbon source, and the amino acids, aspartate, serine, glutamine, and proline; Acetate, succinate, NH4 and CO2 were among the excreted by-products. The model is able to account for ATP generation under anaerobic conditions through oxidative phosphorylation, using nitrate or fumarate electron acceptors. However, it is not able to generate biomass in the absence of O2.
3.1.4. Model Analysis—Identification of Substrate Auxotrophy
Out of the 33 organic substrates tested in the model, 30 substrates were identified as non-essential. The remaining three substrates, methionine, pantothenate and niacinamide (after the curation based on experimental observation in 2.3) were identified as auxotrophic. The pathways for the de-novo biosynthesis of these metabolites were absent in the PGDB and thus the model. These included homoserine O-succinyltransferase and methionine synthase from the methionine biosynthesis pathway, 3-methyl-2-oxobutanoate hydroxymethyltrasferase, pantothenate synthetase, and 2-oxopantoate reductase from the pantothenate biosynthesis pathway, and nicotinate-nucleotide diphosphorylase and quinolinate synthetase from the niacinamide biosynthesis pathway.
3.1.5. Model Analysis—Specification of Minimal Nutrient Requirements
The model analysis suggests that growth can be supported with a medium containing only pyruvate, methionine, pantothenate, and niacinamide (in addition to inorganic salts). Furthermore, the fact that aspartate, serine, glutamine, and proline were imported in excess, relative to that required by the biomass composition, suggests that the presence of these compounds in the media would enhance growth.
3.2. Experimental Analysis
3.2.1. Media Design and Confirmation of Auxotrophies
A simplified defined media containing pyruvate, four amino acids (methionine, cysteine, serine and glutamine or glutamate), pantothenate, and niacinamide (along with inorganic salts) supported growth of C. jejuni M1cam. The removal of methionine, pantothenate and niacinamide from the DMEM/F-12 media (Table S1) resulted in no growth of M1cam, thus, confirming auxotrophies predicted by the model analysis.
As part of the media development, the concentration of sodium pyruvate, as a carbon source, was increased from 0.5 to 10 mM. In addition, the concentrations of auxotrophic substrates, niacinamide and pantothenate, were optimized to 33.1 and 9.4 μM, respectively (Table 3). The inorganic substrates were kept identical to those in DMEM/F-12 media. Amino acids cysteine, serine and glutamine or glutamate, although not essential for growth, had a significant growth promoting effect i.e., removal of any of these substrates from the media decreased the growth compared to DMEM/F-12 media. In contrast, removal of any other amino acids or vitamins did not have any significant effect on growth as compared to DMEM/F-12 media.
Thus, four simple defined media compositions were defined, where all four included the auxotrophic substrates, methionine, pantothenate, and niacinamide along with pyruvate as a carbon source, and with different combinations of non-essential but growth-improving organic substrates, presented in Table 3.
The best results, in terms of growth, were obtained when cysteine, serine, and glutamine or glutamate where present (MM1 and MM2 in Table 3). Individual removal of cysteine and serine from the media decreased growth to 0.41 ± 0.04 and 0.57 ± 0.01 at 48 h, respectively (MM3 and MM4 in Table 3). Glutamate and glutamine were exchangeable, having similar effects on growth (MM1 and MM2 in Table 3). However, removal of both substrates at the same time resulted in around 4.5 times lower OD (0.24±0.03 at 48 h, data not shown) when compared to MM1 and MM2. Providing only the essential auxotrophic substrates, i.e., methionine, pantothenate, and niacinamide, together with pyruvate as a carbon source and inorganic compounds, did not support growth (0.05 ± 0.02, data not shown). This confirmed the impact of each of the growth promoting amino acids in culturing Campylobacter jejuni M1cam. Although each of MM1 and MM2 had a similar effect on growth of Campylobacter jejuni M1cam, MM1 was selected as final defined media, as it had a higher starting pH and required less NaOH to reach pH 7.
Therefore, this media was tested for growth of 7 additional Campylobacter jejuni strains, with the experiments being performed in two different laboratories, the Department of Veterinary Medicine, Cambridge University and the London School of Hygiene and Tropical Medicine. All isolates tested in this study grew in the designed defined media MM1 by 48 h, using the conditions of growth as defined by each laboratory, resulting in a range of OD600 from 0.23 to 1.05 (Table 4). By 48 h, all strains had reached a plateau and the replicates were stable. The variation in growth was probably caused by different growth conditions and the genetics of the strains.
3.2.2. Testing Previously Published Campylobacter Specific Growth Media
We tested growth of M1cam on the previously published defined media by Guccione et al. (2010) and Alazzam et al. (2011) (MCLMAN media). Both formulations did not support growth of M1cam. MCLMAN media could support growth only after the addition of pantothenate, which was confirmed as an auxotrophic substrate for M1cam in our study. The OD at 600 nm of this culture (0.33 ± 0.03, data not shown) after 48 h of incubation, however, was still lower than the growth observed in our defined media (1.05 ± 0.06).
4. Discussion and Conclusion
In this study, we have constructed, a well-curated, strain-specific GSM of Campylobacter jejuni M1cam. We have examined the GSM to investigate the de-novo biosynthesis ability and substrate auxotrophy of the strain. We have experimentally validated the model results and curated the GSM for missing GPR associations or over-predicted reactions. A metabolic model guided the design of a simple defined media for the growth of Campylobacter jejuni. Additionally, we have shown that 8 diverse strains of Campylobacter jejuni are able to grow in the defined simple media.
4.1. Model Analysis
Our analysis showed that when all amino acids are available, leucine, arginine, histidine, valine, asparagine, phenylalanine, alanine, threonine, tyrosine, tryptophan, and lysine were imported at a rate equal to their demand for protein synthesis. However, the network described by the model has the ability for the de novo synthesis of these amino acids, as a solution to Equation 1 could still be found when their respective uptake transporters were blocked.
In contrast, aspartate, proline, serine, and glutamine were all taken up at a rate above that required for protein synthesis. It is worth noting that, without any constraint on media uptake, the model prefers uptake of aspartate, proline, serine, and glutamine, which are preferred by Campylobacter jejuni and are the most common amino acids found in chicken excreta (Parsons, 1984). In addition, the metabolic profile of Campylobacter jejuni liquid cultures' supernatant has shown significant depletion of these four amino acids from nutrient rich medium, reviewed in Hofreuter (2014).
Among all amino acids, serine has been most widely reported to be preferred by Campylobacter jejuni(Guccione et al., 2008; Wright et al., 2009; Gao et al., 2017). Glutamine utilization in Campylobacter jejuni is known to vary between strains: Campylobacter jejuni NCTC11168 has been reported not to utilize it (Hofreuter et al., 2008), while strains such as 81116 and 81-176 readily do (Hofreuter et al., 2006). Aspartate has been shown to be growth promoting under oxygen limitation (Guccione et al., 2008). Proline utilization has also been described at the stationary phase of Campylobacter jejuni growth, after the preferred nutrients become exhausted (Wright et al., 2009). These reports suggest that amino acid utilization varies between different strains of Campylobacter jejuni. It would therefore be necessary to build a model which represented that variation.
Whilst specific amino acids uptake from media remains to be tested experimentally for M1cam, similar experimental observations have been reported by Gao et al. (2017) where Campylobacter jejuni exhibits uptake capacity for amino acids such as arginine, cysteine, histidine, lysine, phenylalanine, or threonine that are directly incorporated into synthesized proteins rather than being catalyzed for energy or conversion to other intermediates.
Model analysis also reveals that Campylobacter jejuni M1cam has the ability for anaerobic respiration, however, it requires O2 for biomass. Although model observation is consistent with previous reports from Sellars et al. (2002), Kelly (2008), van der Stel et al. (2017), and van der Stel and Wösten (2019), this is a vast research topic in itself and details remain to be addressed experimentally.
4.2. Auxotrophic Substrates
The modeling and experimental results presented here, indicate that Campylobacter jejuni M1cam is auxotrophic for methionine, pantothenate, and niacinamide. In this study, we established that Campylobacter jejuni M1cam presents auxotrophy for methionine but not for cysteine. It can utilize methionine as a S source for cysteine biosynthesis as also reported in Helicobacter pylorii. The results presented here suggest that cysteine auxotrophy is strain dependent and not a conserved metabolic property in all Campylobacter jejuni strains as suggested by Vorwerk et al. (2014). On the contrary, we found that methionine auxotrophy is maintained in M1cam even under increased cysteine concentration. Cysteine, although not auxotrophic, is one of the growth improving components possibly because of its role in the de novo synthesis of proteins associated with FeS clusters, such as the serine dehydratase, pyruvate pyruvate:acceptor and oxoglutarate:acceptor oxidoreductases. Moreover, most Campylobacter jejuni, as a common feature of host associated epsilonproteobacteria, are unable to assimilate sulfate as the S source (Alazzam et al., 2011; Vorwerk et al., 2014) and to compensate for this limited anabolic capacity, it is reasonable to suggest that Campylobacter spp. retain auxotrophy (or prefer) S containing amino acids, methionine, and cysteine.
Auxotrophy for pantothenate, a precursor for CoA synthesis, is related to host specificity. The pantothenate biosynthesis gene (panB, panC, and panD) cluster lies in the hypervariable region (Pearson et al., 2003) and has been shown to be more frequently present in isolates from cattle compared to isolates from chickens (Sheppard et al., 2013). Campylobacter jejuni M1cam is the derivative of the human isolate Campylobacter jejuni M1 and is epidemiologically related to poultry strains (Friis et al., 2010; de Vries et al., 2015); thus, it retains pantothenate auxotrophy. There is notable differences in Campylobacter jejuni M1cam auxotrophy from that of the more commonly studied strain, Campylobacter jejuni NCTC 11168. The latter presents auxotrophy for methionine and cysteine; niacinamide, although being growth improving, is not essential and removal of pantothenate had no effect on growth in this strain (Alazzam et al., 2011). Campylobacter jejuni isolates 81176, on the other hand, present auxotrophy for cysteine but not methionine Vorwerk et al. (2014). Differences in substrate auxotrophy seems to be common among Campylobacter spp. Tenover and Patton (1987), based on 439 Campylobacter jejuni isolates and 46 Campylobacter coli isolates, have reported Cambylobacter auxotrophy for cysteine, cystine, arginine, proline, and methionine. The Dickgiesser and Czylwik (1985) study, based on 52 Campylobacter jejuni strains, reported methionine, cysteine, cystine, panthothenate, thiamine, and NAD as important substrates for auxotyping of strains.
4.3. Media Design
We have designed simple defined media specifically for Campylobacter jejuni M1cam which can support growth of 7 other Campylobacter jejuni strains tested in this study. The fact that previously reported MCLMAN media was designed based on Campylobacter jejuni NCTC11168 (Alazzam et al., 2011), which presents a different auxotrophy than Campylobacter jejuni M1cam, and did not include panthothenate, explains why Campylobacter jejuni M1cam growth was not supported in this media.
Our media, apart from Campylobacter jejuni M1cam specific auxotrophic substrates (methionine, pantothenate, niacinamide) and pyruvate as the carbon source, contains amino acids cysteine, serine, and glutamate/glutamine. The latter amino acids, though not essential for growth, had a significant growth promoting effect on Campylobacter jejuni M1cam under our experimental condition. Similar, reports have been shown for strains 81176 and NCTC11168 where substrates, though not essential, had to be added to improve growth (Alazzam et al., 2011; Vorwerk et al., 2014), although preference of these growth improving substance seems to vary between strains and culture conditions. This varying amino acid preference suggests their potential metabolic roles beyond nitrogen and the carbon source (Bièche et al., 2012). Thus, a detailed study on amino acid utilization is needed to increase our knowledge in nutrient acquisition and metabolism of Campylobacter. Although pyruvate transporters have not been identified, pyruvate has been shown to be used as an exogenous carbon source when present in growth medium (Mendz et al., 1997; Velayudhan and Kelly, 2002; Stahl et al., 2012; Wagley et al., 2014) and have a protective role in the presence of oxidative stress (Bolton et al., 1984; Hodge and Krieg, 1994). In our study, increasing the pyruvate concentration improved growth rate in C. jejuni M1cam. It should be noted that the media designed in this study was optimized to maximize the growth of C. jejuni M1cam. Further tests will be needed to optimize the growth of other strains, however it is clear that the designed media MM1 can support the growth of a variety of strains under different conditions.
4.4. Conclusion
By adopting a systems-biology approach and using a newly annotated genome of Campylobacter jejuni M1Cam, we have built and analyzed a strain specific genome-scale model of this organism. The analysis allowed us to identify specific auxotrophies, as well as compounds which are preferentially consumed, and this allowed us to develop a new media suitable for the study of M1Cam and other Campylobacter spp. strains. The model analysis also replicated the apparently paradoxical, but widely reported observation, that although Campylobacter spp. can operate the electron transport chain in the absence of oxygen, oxygen is nonetheless essential for growth. Experimentally confirming all aspects of our model results, in particular the generation of metabolic by-products in the media, was beyond the scope of the current contribution. However, we believe the experimental and modeling results we have presented here, when taken together, provide a solid foundation upon which to build further investigations of Campylobacter jejuni M1cam and related organisms.
Data Availability Statement
The datasets generated for this study can be found in the https://data.quadram.ac.uk/dipali.singh/CJM1cam_MM/, https://data.quadram.ac.uk/dipali.singh/CJM1cam_DB/. Additionally, Pathway/Genome database generated in this study is available from Pathway Tool Registry.
Author Contributions
NT and DS designed and supervised research plans with input from JW. DS constructed the biochemical database and genome-scale metabolic model. NT performed experiments for minimal media design with input from BP. LC performed gene predictions and annotations. ES and FN tested minimal media designed in this work in other strains. BD performed sequence analysis. MP and DS analyzed GSM and together with NT wrote the paper with revision from JW. All authors contributed to the article and approved the submitted version.
Funding
NT, LC, BP, ES, FN, MP, JW, and DS gratefully acknowledge the support of the Biotechnology and Biological Sciences Research Council (BBSRC); this research was funded by the BBSRC Institute Strategic Programme Microbes in the Food Chain BB/R012504/1 and its constituent project BBS/E/F/000PR10349 (Theme 2, Microbial Survival in the Food Chain). BD was funded by the Bill and Melinda Gates Foundation.
Conflict of Interest
LC is the director of SequenceAnalysis.co.uk and was paid to perform this work.
The remaining authors declare that the research was conducted in the absence of any commercial or financial relationships that could be construed as a potential conflict of interest.
Acknowledgments
Special thanks to Andrew Grant, from the Department of Veterinary Medicine, University of Cambridge, for donating the main strain used in this study, Campylobacter jejuni M1cam. We also deeply thank Ozan Gundogdu and Brendan Wren, from the London School of Hygiene and Tropical Medicine, for donating the other 7 strains to test final defined media in this study. From the Quadram Institute Biosciences Core Sequencing facility, we thank David Baker and Stephen Rudder, for the whole-genome sequence analysis of the genomic DNA of Campylobacter jejuni M1cam. And last, but not least, the authors would like to thank Zahra Omole for her technical assistance in the experiments performed in the London School of Hygiene and Tropical Medicine.
Supplementary Material
The Supplementary Material for this article can be found online at: https://www.frontiersin.org/articles/10.3389/fmicb.2020.01072/full#supplementary-material
Table S1. Composition of modified DMEM/F-12 media used in this study.
Table S2. List of Gene-Protein-Reaction relationships curated in the Campylobacter jejuni M1cam PGDB.
References
Ahmad, A., Hartman, H., Krishnakumar, S., Fell, D., Poolman, M., and Srivastava, S. (2017). A genome scale model of Geobacillus thermoglucosidasius (C56-YS93) reveals its biotechnological potential on rice straw hydrolysate. J. Biotechnol. 251, 30–37. doi: 10.1016/j.jbiotec.2017.03.031
Alazzam, B., Bonnassie-Rouxin, S., Dufour, V., and Ermel, G. (2011). MCLMAN, a new minimal medium for Campylobacter jejuni NCTC 11168. Res. Microbiol. 162, 173–179. doi: 10.1016/j.resmic.2010.09.024
Altekruse, S. F., Swerdlow, D. L., and Stern, N. J. (1998). Campylobacter Jejuni. Vet. Clin. N. Am. Food Anim. Pract. 14, 31–40. doi: 10.1016/S0749-0720(15)30277-2
Ashburner, M., Ball, C., Blake, J., Botstein, D., Butler, H., Cherry, J., et al. (2000). Gene ontology: tool for the unification of biology. The gene ontology consortium. Nat. Genet. 25, 25–29. doi: 10.1038/75556
Barthelmes, J., Ebeling, C., Chang, A., Schomburg, I., and Schomburg, D. (2007). BRENDA, AMENDA and FRENDA: the enzyme information system in 2007. Nucleic Acids Res. 35(Suppl_1):D511–D514. doi: 10.1093/nar/gkl972
Bian, X., Garber, J. M., Cooper, K. K., Huynh, S., Jones, J., Mills, M. K., et al. (2020). Campylobacter abundance in breastfed infants and identification of a new species in the global enterics multicenter study. mSphere 5, e00735–19. doi: 10.1128/mSphere.00735-19
Bièche, C., de Lamballerie, M., Chevret, D., Federighi, M., and Tresse, O. (2012). Dynamic proteome changes in Campylobacter jejuni 81-176 after high pressure shock and subsequent recovery. J. Proteomics 75, 1144–1156. doi: 10.1016/j.jprot.2011.10.028
Blaser, M. J., and Engberg, J. (2008). “Clinical aspects of Campylobacter jejuni and Campylobacter coli infections,” in Campylobacter, 3rd Edn, eds I. Nachamkin, C. Szymanski, and M. Blaser (Washington, DC: American Society of Microbiology), 99–121. doi: 10.1128/9781555815554.ch6
Bolton, F., Coates, D., and Hutchinson, D. (1984). The ability of Campylobacter media supplements to neutralize photochemically induced toxicity and hydrogen peroxide. J. Appl. Bacteriol. 56, 151–157. doi: 10.1111/j.1365-2672.1984.tb04707.x
Buss, J., Cresse, M., Doyle, S., Buchan, B., Craft, D., and Young, S. (2019). Campylobacter culture fails to correctly detect Campylobacter in 30% of positive patient stool specimens compared to non-cultural methods. Eur. J. Clin. Microbiol. Infect. Dis 38, 1087–1093. doi: 10.1007/s10096-019-03499-x
Butzler, J., Dekeyser, P., Detrain, M., and Dehaen, F. (1973). Related vibrio in stools. J. Pediatr. 82, 493–495. doi: 10.1016/S0022-3476(73)80131-3
Butzler, J.-P. (2004). Campylobacter, from obscurity to celebrity. Clin. Microbiol. Infect. 10, 868–876. doi: 10.1111/j.1469-0691.2004.00983.x
Camacho, C., Coulouris, G., Avagyan, V., Ma, N., Papadopoulos, J., Bealer, K., et al. (2009). Blast+: architecture and applications. BMC Bioinform. 10:421. doi: 10.1186/1471-2105-10-421
Carrillo, C. D., Taboada, E., Nash, J. H. E., Lanthier, P., Kelly, J., Lau, P. C., et al. (2004). Genome-wide expression analyses of Campylobacter jejuni NCTC11168 reveals coordinate regulation of motility and virulence by flhA. J. Biol. Chem. 279, 20327–20338. doi: 10.1074/jbc.M401134200
Caspi, R., Billington, R., Ferrer, L., Foerster, H., Fulcher, C. A., Keseler, I. M., et al. (2015). The MetaCyc database of metabolic pathways and enzymes and the BioCyc collection of pathway/genome databases. Nucleic Acids Res. 44, D471–D480. doi: 10.1093/nar/gkv1164
Caspi, R., Billington, R., Fulcher, C. A., Keseler, I. M., Kothari, A., Krummenacker, M., et al. (2017). The MetaCyc database of metabolic pathways and enzymes. Nucleic Acids Res. 46, D633–D639. doi: 10.1093/nar/gkx935
Caspi, R., Dreher, K., and Karp, P. (2013). The challenge of constructing, classifying, and representing metabolic pathways. FEMS Microbiol. Lett. 345. doi: 10.1111/1574-6968.12194
Châtre, P., Haenni, M., Meunier, D., Botrel, M.-A., Calavas, D., and Madec, J.-Y. (2010). Prevalence and antimicrobial resistance of Campylobacter jejuni and Campylobacter coli isolated from cattle between 2002 and 2006 in France. J. Food Protect. 73, 825–831. doi: 10.4315/0362-028X-73.5.825
Champion, O. L., Gaunt, M. W., Gundogdu, O., Elmi, A., Witney, A. A., Hinds, J., et al. (2005). Comparative phylogenomics of the food-borne pathogen Campylobacter jejuni reveals genetic markers predictive of infection source. Proc. Natl. Acad. Sci. U.S.A. 102, 16043–16048. doi: 10.1073/pnas.0503252102
Corry, J. E., Post, D., Colin, P., and Laisney, M. (1995). Culture media for the isolation of campylobacters. Int. J. Food Microbiol. 26, 43–76. doi: 10.1016/0168-1605(95)00044-K
Davis, L., and DiRita, V. (2008). Growth and laboratory maintenance of Campylobacter jejuni. Curr. Protoc. Microbiol. Chapter 8:Unit 8A.1.1–8A.1.7. doi: 10.1002/9780471729259.mc08a01s10
de Vries, S., Gupta, S., Baig, A., L'Heureux, J., Pont, E., Wolanska, D., et al. (2015). Motility defects in Campylobacter jejuni defined gene deletion mutants caused by second-site mutations. Microbiology 161, 2316–2327. doi: 10.1099/mic.0.000184
Debruyne, L., Gevers, D., and Vandamme, P. (2008). “Taxonomy of the family Campylobacteraceae,” in Campylobacter, 3rd Edn, eds I. Nachamkin, C. Szymanski, and M. Blaser (Washington, DC: American Society of Microbiology), 3–25. doi: 10.1128/9781555815554.ch1
Deckert, A., Valdivieso Garcia, A., Reid Smith, R., Tamblyn, S., Seliske, P., Irwin, R., et al. (2010). Prevalence and antimicrobial resistance in Campylobacter spp. isolated from retail chicken in two health units in Ontario. J. Food Protect. 73, 1317–1324. doi: 10.4315/0362-028X-73.7.1317
DeJongh, M., Formsma, K., Boillot, P., Gould, J., Rycenga, M., and Best, A. (2007). Toward the automated generation of genome-scale metabolic networks in the seed. BMC Bioinform. 8:139. doi: 10.1186/1471-2105-8-139
Dekeyser, P., Gossuin-Detrain, M., Butzler, J. P., and Sternon, J. (1972). Acute enteritis due to related vibrio: first positive stool cultures. J. Infect. Dis. 125, 390–392. doi: 10.1093/infdis/125.4.390
Del Rocio Leon-Kempis, M., Guccione, E., Mulholland, F., Williamson, M. P., and Kelly, D. J. (2006). The Campylobacter jejuni PEB1a adhesin is an aspartate/glutamate-binding protein of an ABC transporter essential for microaerobic growth on dicarboxylic amino acids. Mol. Microbiol. 60, 1262–1275. doi: 10.1111/j.1365-2958.2006.05168.x
Dickgiesser, N., and Czylwik, D. (1985). Chemically defined media for auxotyping of Campylobacter jejuni. Z. Bakteriol. Mikrobiol. Hyg. Ser. A Med. Microbiol. Infect. Dis. Virol. Parasitol. 260, 57–64. doi: 10.1016/S0176-6724(85)80098-5
El-Gebali, S., Mistry, J., Bateman, A., Eddy, S., Luciani, A., Potter, S., et al. (2018). The PFAM protein families database in 2019. Nucleic Acids Res. 47, D427–D432. doi: 10.1093/nar/gky995
Fell, D. A., and Small, R. J. (1986). Fat synthesis in adipose tissue. An examination of stoichiometric constraints. Biochem. J. 238, 781–6. doi: 10.1042/bj2380781
Friis, C., Wassenaar, T. M., Javed, M. A., Snipen, L., Lagesen, K., Hallin, P. F., et al. (2010). Genomic Characterization of Campylobacter jejuni Strain M1. PLoS ONE 5:e12253. doi: 10.1371/journal.pone.0012253
Gao, B., Vorwerk, H., Huber, C., Lara-Tejero, M., Mohr, J., Goodman, A. L., et al. (2017). Metabolic and fitness determinants for in vitro growth and intestinal colonization of the bacterial pathogen Campylobacter jejuni. PLoS Biol. 15:e2001390. doi: 10.1371/journal.pbio.2001390
Gevorgyan, A., Poolman, M. G., and Fell, D. A. (2008). Detection of stoichiometric inconsistencies in biomolecular models. Bioinformatics 24, 2245–2251. doi: 10.1093/bioinformatics/btn425
Gibbons, C., Mangen, M.-J., Plass, D., Havelaar, A., Brooke, R., Kramarz, P., et al. (2014). Measuring underreporting and under-ascertainment in infectious disease datasets: a comparison of methods. BMC Public Health 14:147. doi: 10.1186/1471-2458-14-147
Gormley, F. J., Strachan, N. J., Reay, K., MacKenzie, F. M., Ogden, I. D., Dallas, J. F., et al. (2010). Antimicrobial resistance profiles of Campylobacter from humans, retail chicken meat, and cattle feces. Foodborne Pathog. Dis. 7, 1129–1131. doi: 10.1089/fpd.2009.0532
Green, M. L., and Karp, P. D. (2004). A Bayesian method for identifying missing enzymes in predicted metabolic pathway databases. BMC Bioinform. 5, 76–76. doi: 10.1186/1471-2105-5-76
Gu, C., Kim, G., Kim, W., Kim, T. Y., and Lee, S. Y. (2019). Current status and applications of genome-scale metabolic models. Genome Biol. 20:121. doi: 10.1186/s13059-019-1730-3
Guccione, E., Del Rocio Leon-Kempis, M., Pearson, B. M., Hitchin, E., Mulholland, F., Van Diemen, P. M., et al. (2008). Amino acid-dependent growth of Campylobacter jejuni: key roles for aspartase (ASPA) under microaerobic and oxygen-limited conditions and identification of ASPB (CJ0762), essential for growth on glutamate. Mol. Microbiol. 69, 77–93. doi: 10.1111/j.1365-2958.2008.06263.x
Guccione, E., Hitchcock, A., Hall, S. J., Mulholland, F., Shearer, N., Van Vliet, A. H. M., et al. (2010). Reduction of fumarate, mesaconate and crotonate by MFR, a novel oxygen-regulated periplasmic reductase in Campylobacter jejuni. Environ. Microbiol. 12, 576–591. doi: 10.1111/j.1462-2920.2009.02096.x
Hartman, H. B., Fell, D. A., Rossell, S., Jensen, P. R., Woodward, M. J., Thorndahl, L., et al. (2014). Identification of potential drug targets in Salmonella enterica sv. Typhimurium using metabolic modelling and experimental validation. Microbiology 160, 1252–1266. doi: 10.1099/mic.0.076091-0
Hodge, J., and Krieg, N. (1994). Oxygen tolerance estimates in campylobacter species depend on the testing medium. J. Appl. Bacteriol. 77, 666–673. doi: 10.1111/j.1365-2672.1994.tb02817.x
Hoffman, P. S., Krieg, N. R., and Smibert, R. M. (1979). Studies of the microaerophilic nature of Campylobacter fetus subsp. jejuni. I. Physiological aspects of enhanced aerotolerance. Can. J. Microbiol. 25, 1–7. doi: 10.1139/m79-001
Hofreuter, D. (2014). Defining the metabolic requirements for the growth and colonization capacity of Campylobacter jejuni. Front. Cell. Infect. Microbiol. 4:137. doi: 10.3389/fcimb.2014.00137
Hofreuter, D., Novik, V., and Galan, J. E. (2008). Metabolic diversity in Campylobacter jejuni enhances specific tissue colonization. Cell Host Microbe 4, 425–433. doi: 10.1016/j.chom.2008.10.002
Hofreuter, D., Tsai, J., Watson, R. O., Novik, V., Altman, B., Benitez, M., et al. (2006). Unique features of a highly pathogenic Campylobacter jejuni strain. Infect. Immunity 74, 4694–4707. doi: 10.1128/IAI.00210-06
Hsieh, Y.-H., Simpson, S., Kerdahi, K., and Sulaiman, I. M. (2018). A comparative evaluation study of growth conditions for culturing the isolates of Campylobacter spp. Curr. Microbiol. 75, 71–78. doi: 10.1007/s00284-017-1351-6
Hyatt, D., Chen, G.-L., LoCascio, P. F., Land, M. L., Larimer, F. W., and Hauser, L. J. (2010). Prodigal: prokaryotic gene recognition and translation initiation site identification. BMC Bioinform. 8:119. doi: 10.1186/1471-2105-11-119
Jacobs, B. C., van Doorn, P. A., TioGillen, A. P., Visser, L. H., van der Meché, F. G. A., et al. (1996). Campylobacter jejuni infections and antiGM1 antibodies in GuillainBarré syndrome. Ann. Neurol. 40, 181–187. doi: 10.1002/ana.410400209
Jeske, L., Placzek, S., Schomburg, I., Chang, A., and Schomburg, D. (2018). Brenda in 2019: a European elixir core data resource. Nucleic acids Res. 47, D542–D549. doi: 10.1093/nar/gky1048
Jordan, A., and Reichard, P. (1998). Ribonucleotide reductases. Annu. Rev. Biochem. 67, 71–98. doi: 10.1146/annurev.biochem.67.1.71
Kanehisa, M., and Goto, S. (2000). KEGG: Kyoto encyclopedia of genes and genomes. Nucleic Acids Res. 28, 27–30. doi: 10.1093/nar/28.1.27
Kanehisa, M., Goto, S., Hattori, M., Aoki-Kinoshita, K. F., Itoh, M., Kawashima, S., et al. (2006). From genomics to chemical genomics: new developments in KEGG. Nucleic Acids Res 34(Suppl_1):D354–D357. doi: 10.1093/nar/gkj102
Karp, P. D., Billington, R., Caspi, R., Fulcher, C. A., Latendresse, M., Kothari, A., et al. (2017). The BioCyc collection of microbial genomes and metabolic pathways. Brief. Bioinform. 20, 1085–1093. doi: 10.1093/bib/bbx085
Karp, P. D., Latendresse, M., and Caspi, R. (2011). The pathway tools pathway prediction algorithm. Stand Genomic Sci. 5, 424–429. doi: 10.4056/sigs.1794338
Karp, P. D., Latendresse, M., Paley, S. M., Ong, M. K. Q., Billington, R., Kothari, A., et al. (2015). Pathway tools version 19.0: integrated software for pathway/genome informatics and systems biology. Brief. Bioinform. 17, 877–890. doi: 10.1093/bib/bbv079
Karp, P. D., Paley, S., and Romero, P. (2002). The pathway tools software. Bioinformatics 18(Suppl_1):S225–S232. doi: 10.1093/bioinformatics/18.suppl_1.S225
Kelly, D. J. (2008). “Complexity and versatility in the physiology and metabolism of Campylobacter jejuni,” in Campylobacter, 3rd Edn, eds I. Nachamkin, C. Szymanski, and M. Blaser (Washington, DC: American Society of Microbiology), 41–61. doi: 10.1128/9781555815554.ch3
Kim, J., Oh, E., Banting, G. S., Braithwaite, S., Chui, L., Ashbolt, N. J., et al. (2016). An improved culture method for selective isolation of Campylobacter jejuni from wastewater. Front. Microbiol. 7:1345. doi: 10.3389/fmicb.2016.01345
Kim, W. J., Kim, H. U., and Lee, S. Y. (2017). Current state and applications of microbial genome-scale metabolic models. Curr. Opin. Syst. Biol. 2, 10–18. doi: 10.1016/j.coisb.2017.03.001
Lastovica, A. J., On, S. L. W., and Zhang, L. (2014). The Family Campylobacteraceae. Berlin; Heidelberg: Springer. doi: 10.1007/978-3-642-39044-9_274
Lauwers, S., Boeck, M. D., and Butzler, J. (1978). Campylobacter enteritis in Brussels. Lancet 311, 604–605. Originally published as Volume 1, Issue 8064. doi: 10.1016/S0140-6736(78)91045-0
Line, J., Hiett, K., Guard-Bouldin, J., and Seal, B. (2010). Differential carbon source utilization by Campylobacter jejuni 11168 in response to growth temperature variation. J. Microbiol. Methods 80, 198–202. doi: 10.1016/j.mimet.2009.12.011
Liu, L., Agren, R., Bordel, S., and Nielsen, J. (2010). Use of genome-scale metabolic models for understanding microbial physiology. FEBS Lett. 584, 2556–2564. doi: 10.1016/j.febslet.2010.04.052
Liu, X., Gao, B., Novik, V., and Galán, J. (2012). Quantitative proteomics of intracellular Campylobacter jejuni reveals metabolic reprogramming. PLoS Pathog. 8:e1002562. doi: 10.1371/journal.ppat.1002562
Manandhar, M., and Cronan, J. (2017). Pimelic acid, the first precursor of the Bacillus subtilis biotin synthesis pathway, exists as the free acid and is assembled by fatty acid synthesis: Bacillus subtilis biotin synthesis. Mol. Microbiol. 104, 595–607. doi: 10.1111/mmi.13648
Mangen, M.-J., Havelaar, A., Haagsma, J., and Kretzschmar, M. (2016). The burden of campylobacter-associated disease in six European countries. Microb. Risk Anal. 2–3:48–52. doi: 10.1016/j.mran.2016.04.001
Mendz, G. L., Ball, G. E., and Meek, D. J. (1997). Pyruvate metabolism in Campylobacter spp. Biochim. Biophys. Acta Gen. Subj. 1334, 291–302. doi: 10.1016/S0304-4165(96)00107-9
Metris, A., Reuter, M., Gaskin, D., Baranyi, J., and van Vliet, A. (2011). In vivo and in silico determination of essential genes of Campylobacter jejuni. BMC Genomics 12:535. doi: 10.1186/1471-2164-12-535
Morgat, A., Lombardot, T., Coudert, E., Axelsen, K., Neto, T. B., Gehant, S., et al. (2019). Enzyme annotation in UniProtKB using Rhea. Bioinformatics. 36, 1896–1901. doi: 10.1093/bioinformatics/btz817
Ogata, H., Goto, S., Sato, K., Fujibuchi, W., Bono, H., and Kanehisa, M. (1999). KEGG: Kyoto encyclopedia of genes and genomes. Nucleic Acids Res. 27, 29–34. doi: 10.1093/nar/27.1.29
Parsons, C. M. (1984). Influence of caecectomy and source of dietary fibre or starch on excretion of endogenous amino acids by laying hens. Brit. J. Nutr. 51, 541–548. doi: 10.1079/BJN19840059
Pearson, B., Pin, C., Wright, J., I'Anson, K., Humphrey, T., and Wells, J. (2003). Comparative genome analysis of Campylobacter jejuni using whole genome DNA microarrays. FEBS Lett. 554, 224–230. doi: 10.1016/S0014-5793(03)01164-5
Platts-Mills, J., and Kosek, M. (2014). Update on the burden of Campylobacter in developing countries. Curr. Opin. Infect. Dis 27, 444–450. doi: 10.1097/QCO.0000000000000091
Poolman, M. G. (2006). ScrumPy: metabolic modelling with Python. IEE Proc. Syst. Biol. 153, 375–378. doi: 10.1049/ip-syb:20060010
Poolman, M. G., Kundu, S., Shaw, R., and Fell, D. A. (2013). Responses to light intensity in a genome-scale model of rice metabolism. Plant Physiol. 162, 1060–1072. doi: 10.1104/pp.113.216762
Poolman, M. G., Miguet, L., Sweetlove, L. J., and Fell, D. A. (2009). A genome-scale metabolic model of Arabidopsis and some of its properties. Plant Physiol. 151, 1570–1581. doi: 10.1104/pp.109.141267
Ruiz-Palacios, G. M. (2007). The health burden of Campylobacter infection and the impact of antimicrobial resistance: playing chicken. Clin. Infect. Dis. 44, 701–703. doi: 10.1086/509936
Schellenberger, J., Park, J. O., Conrad, T. M., and Palsson, B. O. (2009). BIGG: a biochemical genetic and genomic knowledgebase of large scale metabolic reconstructions. BMC Bioinform. 11:213. doi: 10.1186/1471-2105-11-213
Seemann, T. (2014). PROKKA: rapid prokaryotic genome annotation. Bioinformatics 30, 2068–2069. doi: 10.1093/bioinformatics/btu153
Sellars, M. J., Hall, S. J., and Kelly, D. J. (2002). Growth of Campylobacter jejuni supported by respiration of fumarate, nitrate, nitrite, trimethylamine-n-oxide, or dimethyl sulfoxide requires oxygen. J. Bacteriol. 184, 4187–4196. doi: 10.1128/JB.184.15.4187-4196.2002
Sheppard, S. K., Didelot, X., Meric, G., Torralbo, A., Jolley, K. A., Kelly, D. J., et al. (2013). Genome-wide association study identifies vitamin b5 biosynthesis as a host specificity factor in Campylobacter. Proc. Natl. Acad. Sci. U.S.A. 110, 11923–11927. doi: 10.1073/pnas.1305559110
Skirrow, M. B. (1977). Campylobacter enteritis: a “new” disease. British Med. J. 6078, 9–11. doi: 10.1136/bmj.2.6078.9
Stabler, R. A., Larsson, J. T., Al-Jaberi, S., Nielsen, E. M., Kay, E., Tam, C. C., et al. (2013). Characterization of water and wildlife strains as a subgroup of Campylobacter jejuni using DNA microarrays. Environ. Microbiol. 15, 2371–2383. doi: 10.1111/1462-2920.12111
Stahl, M., Butcher, J., and Stintzi, A. (2012). Nutrient acquisition and metabolism by Campylobacter jejuni. Front. Cell. Infect. Microbiol. 2:5. doi: 10.3389/fcimb.2012.00005
Stok, J. E., and Voss, J. J. D. (2000). Expression, purification, and characterization of biol: a carbon-carbon bond cleaving cytochrome p450 involved in biotin biosynthesis in Bacillus subtilis. Arch. Biochem. Biophys. 384, 351–360. doi: 10.1006/abbi.2000.2067
Szklarczyk, D., Gable, A., Lyon, D., Junge, A., Wyder, S., Huerta-Cepas, J., et al. (2018). String v11: protein-protein association networks with increased coverage, supporting functional discovery in genome-wide experimental datasets. Nucleic Acids Res. 47, D607–D613. doi: 10.1093/nar/gky1131
Tam, C., and O'Brien, S. (2016). Economic cost of Campylobacter, norovirus and rotavirus disease in the United Kingdom. PLoS ONE 11:e0138526. doi: 10.1371/journal.pone.0138526
Tenover, F. C., Knapp, J. S., Patton, C., and Plorde, J. J. (1985). Use of auxotyping for epidemiological studies of Campylobacter jejuni and Campylobacter coli infections. Infect. Immunity 48, 384–388. doi: 10.1128/IAI.48.2.384-388.1985
Tenover, F. C., and Patton, C. M. (1987). Naturally occurring auxotrophs of Campylobacter jejuni and Campylobacter coli. J. Clin. Microbiol. 25, 1659–1661. doi: 10.1128/JCM.25.9.1659-1661.1987
Thiele, I., Vo, T. D., Price, N. D., and Palsson, B. Ø. (2005). Expanded metabolic reconstruction of Helicobacter pylori (IIT341 GSM/GPR): an in silico genome-scale characterization of single- and double-deletion mutants. J. Bacteriol. 187, 5818–5830. doi: 10.1128/JB.187.16.5818-5830.2005
van der Ark, K. C. H., Aalvink, S., Suarez-Diez, M., Schaap, P. J., de Vos, W. M., and Belzer, C. (2018). Model-driven design of a minimal medium for Akkermansia muciniphila confirms mucus adaptation. Microb. Biotechnol. 11, 476–485. doi: 10.1111/1751-7915.13033
van der Hooft, J. J., Alghefari, W., Watson, E., Everest, P., Morton, F. R., Burgess, K. E., et al. (2018). Unexpected differential metabolic responses of Campylobacter jejuni to the abundant presence of glutamate and fucose. Metabolomics 14:144. doi: 10.1007/s11306-018-1438-5
van der Stel, A.-X., Boogerd, F. C., Huynh, S., Parker, C. T., van Dijk, L., van Putten, J. P. M., et al. (2017). Generation of the membrane potential and its impact on the motility, ATP production and growth in Campylobacter jejuni. Molecular Microbiol. 105, 637–651. doi: 10.1111/mmi.13723
van der Stel, A.-X., and Wösten, M. M. S. M. (2019). Regulation of respiratory pathways in campylobacterota: a review. Front. Microbiol. 10:1719. doi: 10.3389/fmicb.2019.01719
Varma, A., and Palsson, B. O. (1993). Metabolic capabilities of Escherichia coli: I. synthesis of biosynthetic precursors and cofactors. J. Theor. Biol. 165, 477–502. doi: 10.1006/jtbi.1993.1202
Varma, A., and Palsson, B. O. (1994). Stoichiometric flux balance models quantitatively predict growth and metabolic by-product secretion in wild-type Escherichia coli W3110. Appl. Environ. Microbiol. 60, 3724–31. doi: 10.1128/AEM.60.10.3724-3731.1994
Velayudhan, J., Jones, M. A., Barrow, P., and Kelly, D. J. (2004). L-serine catabolism via an oxygen-labile l-serine dehydratase is essential for colonization of the avian gut by Campylobacter jejuni. Infect. Immun. 72, 260–268. doi: 10.1128/IAI.72.1.260-268.2004
Velayudhan, J., and Kelly, D. J. (2002). Analysis of gluconeogenic and anaplerotic enzymes in Campylobacter jejuni: an essential role for phosphoenolpyruvate carboxykinase. Microbiology 148, 685–694. doi: 10.1099/00221287-148-3-685
Villanova, V., Fortunato, A. E., Singh, D., Bo, D. D., Conte, M., Obata, T., et al. (2017). Investigating mixotrophic metabolism in the model diatom Phaeodactylum tricornutum. Philos. Trans. R. Soc. B 372:1728. doi: 10.1098/rstb.2016.0404
Vorwerk, H., Mohr, J., Huber, C., Wensel, O., Schmidt-Hohagen, K., Gripp, E., et al. (2014). Utilization of host-derived cysteine-containing peptides overcomes the restricted sulphur metabolism of Campylobacter jejuni. Mol. Microbiol. 93, 1224–1245. doi: 10.1111/mmi.12732
Wagley, S., Newcombe, J., Laing, E., Yusuf, E., Sambles, C., Studholme, D., et al. (2014). Differences in carbon source utilisation distinguish Campylobacter jejuni from Campylobacter coli. BMC Microbiol. 14:262. doi: 10.1186/s12866-014-0262-y
Westfall, H. N., Rollins, D. M., and Weiss, E. (1986). Substrate utilization by Campylobacter jejuni and Campylobacter coli. Appl. Environ. Microbiol. 52, 700–705. doi: 10.1128/AEM.52.4.700-705.1986
Westrell, T., Ciampa, N., Boelaert, F., Helwigh, B., Korsgaard, H., Chrael, M., et al. (2009). Zoonotic infections in Europe in 2007: a summary of the EFSA-ECDC annual report. Eurosurveillance 14:19100.
Wilkinson, D., O'Donnell, A., Akhter, R., Fayaz, A., Mack, H., Rogers, L., et al. (2018). Updating the genomic taxonomy and epidemiology of Campylobacter hyointestinalis. Sci. Rep. 8:2393. doi: 10.1038/s41598-018-20889-x
Wright, J. A., Grant, A. J., Hurd, D., Harrison, M., Guccione, E. J., Kelly, D. J., et al. (2009). Metabolite and transcriptome analysis of Campylobacter jejuni in vitro growth reveals a stationary-phase physiological switch. Microbiology 155, 80–94. doi: 10.1099/mic.0.021790-0
Xu, F., Wu, C., Guo, F., Cui, G., Zeng, X., Yang, B., et al. (2015). Transcriptomic analysis of Campylobacter jejuni nctc 11168 in response to epinephrine and norepinephrine. Front. Microbiol. 6:452. doi: 10.3389/fmicb.2015.00452
Zampieri, M., and Sauer, U. (2016). Model-based media selection to minimize the cost of metabolic cooperation in microbial ecosystems. Bioinformatics 32, 1733–1739. doi: 10.1093/bioinformatics/btw062
Keywords: Campylobacter jejuni, genome-scale metabolic model, linear programming, defined growth media, metabolism, auxotrophy, metabolic network
Citation: Tejera N, Crossman L, Pearson B, Stoakes E, Nasher F, Djeghout B, Poolman M, Wain J and Singh D (2020) Genome-Scale Metabolic Model Driven Design of a Defined Medium for Campylobacter jejuni M1cam. Front. Microbiol. 11:1072. doi: 10.3389/fmicb.2020.01072
Received: 31 January 2020; Accepted: 29 April 2020;
Published: 19 June 2020.
Edited by:
Nicolae Corcionivoschi, Agri-Food and Biosciences Institute (AFBI), United KingdomReviewed by:
Monique Zagorec, INRA Centre Angers-Nantes Pays de la Loire, FranceOdile Tresse, INRA Centre Angers-Nantes Pays de la Loire, France
Copyright © 2020 Tejera, Crossman, Pearson, Stoakes, Nasher, Djeghout, Poolman, Wain and Singh. This is an open-access article distributed under the terms of the Creative Commons Attribution License (CC BY). The use, distribution or reproduction in other forums is permitted, provided the original author(s) and the copyright owner(s) are credited and that the original publication in this journal is cited, in accordance with accepted academic practice. No use, distribution or reproduction is permitted which does not comply with these terms.
*Correspondence: John Wain, am9obi53YWluQHF1YWRyYW0uYWMudWs=