- 1Scotland’s Rural College (SRUC), Edinburgh, United Kingdom
- 2Division of Genetics and Genomics, The Roslin Institute and R(D)SVS, University of Edinburgh, Edinburgh, United Kingdom
- 3Edinburgh Genomics, The Roslin Institute and R(D)SVS, University of Edinburgh, Edinburgh, United Kingdom
In this study, Bos Taurus cattle offered one high concentrate diet (92% concentrate-8% straw) during two independent trials allowed us to classify 72 animals comprising of two cattle breeds as “Low” or “High” feed efficiency groups. Digesta samples were taken from individual beef cattle at the abattoir. After metagenomic sequencing, the rumen microbiome composition and genes were determined. Applying a targeted approach based on current biological evidence, 27 genes associated with host-microbiome interaction activities were selected. Partial least square analysis enabled the identification of the most significant genes and genera of feed efficiency (VIP > 0.8) across years of the trial and breeds when comparing all potential genes or genera together. As a result, limited number of genes explained about 40% of the variability in both feed efficiency indicators. Combining information from rumen metagenome-assembled genomes and partial least square analysis results, microbial genera carrying these genes were determined and indicated that a limited number of important genera impacting on feed efficiency. In addition, potential mechanisms explaining significant difference between Low and High feed efficiency animals were analyzed considering, based on the literature, their gastrointestinal location of action. High feed efficiency animals were associated with microbial species including several Eubacterium having the genetic capacity to form biofilm or releasing metabolites like butyrate or propionate known to provide a greater contribution to cattle energy requirements compared to acetate. Populations associated with fucose sensing or hemolysin production, both mechanisms specifically described in the lower gut by activating the immune system to compete with pathogenic colonizers, were also identified to affect feed efficiency using rumen microbiome information. Microbial mechanisms associated with low feed efficiency animals involved potential pathogens within Proteobacteria and Spirochaetales, releasing less energetic substrates (e.g., acetate) or producing sialic acid to avoid the host immune system. Therefore, this study focusing on genes known to be involved in host-microbiome interaction improved the identification of rumen microbial genetic capacities and potential mechanisms significantly impacting on feed efficiency in beef cattle fed high concentrate diet.
Introduction
The FAO predicted that by 2050, the human population will grow to over 9 billion people, and in the same time frame, global meat consumption is projected to increase by 73% (FAO, 2019). Meat production from ruminants offers several advantages including the fact that ruminants convert feedstuffs into high-quality nutrients from materials that do not compete with human-edible food.
The bovine rumen microbiome is essential for feed digestion and beneficial for the hosting ruminant animal. In addition to nutrient absorption by the host, digestion is recognized as an important source of variation in cattle growth efficiency (Herd et al., 2004; Roehe et al., 2016). Such variation is also diet dependent and the importance of diet in determining the composition of the ruminal microbiome is now well-recognized (Rooke et al., 2014; Henderson et al., 2015).
Although, intensive food production using more concentrate diet instead of forage improved feed efficiency in ruminant production, also reducing methane emissions, it generated a stress on the rumen microbiome and the animal (Auffret et al., 2017; Wetzels et al., 2017). For example, both studies confirmed the influence of concentrate over forage diets to generate rumen dysbiosis in beef and dairy cattle. This disturbance is explained by a breach of robustness in microbiome composition and functionality also associated with a “bloom” of zoonotic pathogens especially within Proteobacteria, as similarly, found in humans (Brown et al., 2012).
In nature, most microorganisms are known to occur predominantly in consortia or biofilms, even on mucosal surfaces, involving a broad range of mechanisms for adhesion on the mucosa by beneficial and detrimental microorganisms (Tuson and Weibel, 2013).
It is known that ruminal and lower gut epithelia are dramatically different in term of structure and type of cells with epithelial-attached microorganisms predominantly detected in the lower gut (Steele et al., 2016). Although limited research focused on epithelial-attached microorganisms in ruminant (Malmuthuge et al., 2015), it is predicted to indirectly impact animal performance (Steele et al., 2016). Furthermore, microbial genes detected in the rumen and associated with activities specific of the lower gut like fucose sensing were previously detected in rumen digesta samples (Roehe et al., 2016; Auffret et al., 2017). However, there is lack of information on which microbial mechanisms can impact on cattle feed efficiency. Therefore, identifying and studying such microbial genetic capacities detected in the rumen could be helpful to predict microbial mechanisms impacting animal feed efficiency.
Microbial biofilms have been studied in human (Macfarlane and Dillon, 2007) and ruminants (Bang et al., 2014) and are known to mediate protective functions and enables communication between biofilm forming microorganisms (Macfarlane and Dillon, 2007). Although in general, biofilm studies focused on detrimental effects of mucosa-associated bacterial pathogens such as Escherichia, Shigella, Salmonella, and Treponema spp. (Mao et al., 2015; Song et al., 2018), the presence of biofilms in the rumen has also been reported in healthy ruminants (Pitta et al., 2016).
Other possible microbial mechanisms involved the presence of pili or flagella for surface hooking, different types of secretion systems for adhesion and transfer of toxins or metabolites, production of enzymes needed for the biofilm matrix or associated with toxic effect on the host (e.g., hemolysin; Ribet and Cossart, 2015). Finally, the mucosa surface in the intestine is covered by mucin composed of several sugars including fucose but also sialic acids that can serve for the growth of commensal or pathogenic species (Pickard and Chervonsky, 2015). Alternatively, their synthesis by mucosa-attached microorganisms can assist in avoiding the immune system (Sicard et al., 2017).
To sustain beef cattle production the necessity to reduce production costs mostly associated with animal nutrition by improving feed utilization has been one of the main objectives over the last years (Ramsey et al., 2005). Feed efficiency is often assessed as either feed conversion ratio (FCR) or residual feed intake (RFI) with RFI considered more appropriate to generate a measure of biological efficiency independent of production (Koch et al., 1963; Gunsett, 1984). Negative values of both indicators are indicators of high feed efficiency.
The importance of the rumen microbiome as one of the factors impacting on animal feed efficiency is recognized. Previous reports mostly focused on the microbial community composition (16S rRNA gene data) showing that variation in animal feed efficiency was explained by particular taxa (Myer et al., 2015; Shabat et al., 2016) instead of using the entire microbiome that means the microbial community and their microbial genes. Li et al. (2009) and Roehe et al. (2016) showed that the host genome shapes the rumen microbiota and could be used to identify differences in feed conversion efficiency. From these studies, a substantial lack of mechanistic understanding remained due to the inherent limitation to get information on microbial activities when using 16S rRNA gene sequencing. To alleviate this limitation, Ross et al. (2013) suggested to use metagenomic sequence information to obtain improved predictive models for the use of the microbiome for animal breeding purposes. In addition, Roehe et al. (2016) demonstrated the advantages of using microbial genes as proxies for feed conversion efficiency.
The rumen is an anaerobic microbial ecosystem with the ability to convert carbohydrates to short-chain, volatile fatty acids (VFA), which are absorbed by the animal and used in energy metabolism and protein synthesis. Furthermore, dihydrogen (H2) is also formed as a result of fermentation, and it is central to microbial metabolic activities. However, it is used by methanogenic archaea to reduce CO2 to methane (CH4; Hungate, 1967) leading to the loss of feed gross energy, estimated at 2–12% (Johnson and Johnson, 1995). Therefore, it is expected that high efficient cattle should produce less methane.
To the best of our knowledge, only a limited number of studies like Lima et al. (2019) identified microbial gene biomarkers for FCR and RFI. These authors applied a non-targeted approach for the selection of genes from metagenomics data. In the present study, a specific approach was applied selecting genes encoding for activities related to host–microbiome crosstalk and bacterial mobility and associating those with FCR and RFI and determine the microbial taxa carrying these genes based on metagenome-assembled genomes (MAGs) obtained by metagenomic sequencing (Stewart et al., 2018).
We hypothesized that differences observed in feed efficiency in apparently healthy cattle offered a high concentrate-based diet can be partly explained by differences in the rumen microbiome, particularly in microbial genera carrying genes related to adhesion and host-microbiome interaction. Rumen samples enriched in microbial genes known to be related to pathogenicity activities are detected in less feed efficient cattle. Genes carried by commensal bacteria associated with beneficial mechanisms occurring in the rumen but also those genes which mechanisms is explained for the lower gut (e.g., fucose sensing) are detected in high feed efficient cattle.
Therefore, the overall aim of our work was to identify important microbial genera and potential mechanisms in animal fed concentrate diet, having the capacity to explain differences in animal feed efficiency by calculating feed conversion ratio and residual feed intake. Importance of such mechanisms impacting on feed efficiency was quantified using statistical model. This could be an important step toward discrimination between beneficial and detrimental microorganisms both carrying genes associated with host interaction and to develop strategies targeting microorganisms with the aim of increasing animal feed efficiency using breeding or dietary intervention.
Materials and Methods
Ethics Statement
The animal experiment was conducted at the Beef and Sheep Research Centre of Scotland’s Rural College (SRUC, Edinburgh, United Kingdom). The experiment was approved by the Animal Experiment Committee of SRUC and was conducted in accordance with the requirements of the UK Animals (Scientific Procedures) Act 1986.
Animals, Experimental Design, and Animal Grouping
In our previous studies (Duthie et al., 2016, 2017), data on feed efficiency were obtained from a 2 × 2 factorial design experiment of breed types and diets using 72 steers each in two trial from purebred Luing and crossbred Charolais (CHx) steers. These animal trials were completed in 2012 and 2013 and more details are described in Supplementary Table S1 and Duthie et al. (2016, 2017). Prior to start of the experiment, all animals received the same diet type (Forage-based diet) and thereafter allocated to two different diets fed ad libitum containing (g/kg DM) ∼80 straw to 920 concentrate or 520 forage and 480 concentrate considered as high concentrate-based diet and a mixed diet, respectively. After a 5-week adaptation period to the diets, a 56-day test period for feed efficiency was carried out. Due to EU legislation, the application of antibiotics is prohibited for enhancing growth. In exceptional cases, animals were treated with antibiotics and then excluded from the trial. Information on the diets used in these animal trials was described in Duthie et al. (2016, 2017). Animals were fed ad libitum, the same allocated diet during adaptation period to the feed until they were slaughtered in the abattoir. There was no fastening period prior to sending the animals to slaughter. Gas emissions including methane were measured individually for 48 h in respiration chambers following the same procedure described in Rooke et al. (2014). Sample of ruminal digesta used to determine the microbiome were taken at slaughter between 4 and 14 weeks after the end of the feed efficiency monitoring period. This time gap between the end of the feed efficiency period and the collect of the rumen samples at the abattoir was due to monitoring of methane emissions in six available respiration chambers in which all cattle were recorded individually.
The methodology applied to collect the rumen digesta samples at the abattoir followed the same procedures previously described (Wallace et al., 2015; Roehe et al., 2016). Briefly, two rumen digesta (a mixed of solid and fluid) samples (~50 ml) were taken immediately after the rumen was opened to be drained prior to be stored at −80°C. The slaughter process results in well-mixed samples of rumen contents.
Within this study, only animals (n = 72) receiving high concentrate-based diet within the two independent beef cattle trials of crossbred Charolais and purebred Luing were used.
The two feed efficiency indicators were calculated as follow: FCR is the ratio of feed intake to weight gain and provides an indication of the animal’s ability to convert feed to body weight. RFI is an estimation of the difference between actual feed intake and a predicted feed intake based on body weight and production following Savietto et al. (2014) calculation. Within 72 animals from 2 independent animal trials, half animals were classified as “Low” or “High” feed efficiency groups (Supplementary Table S1). The grouping between Low and High animals followed a balance designed including year of the animal trial (2012 and 2013) and breed (CHx and Luing). Significant differences in FCR and RFI between Low and High animal groups were subsequently confirmed (Figure 1). Immediately after the steers (within 2 h) left the respiration chambers, samples of ruminal fluid were obtained (one per animal) by inserting a tube (16 × 2700 mm Equivet Stomach Tube; Jørgen Kruuse A/S) nasally and aspirating manually. Approximately 50 ml of the fluid were strained through two layers of muslin and then deproteinised by adding 0.2 ml of metaphosphoric acid (215 g/l) and 0.1 ml of internal standard (10 ml 2-ethyl n-butyric acid/l) to determine volatile fatty acid (VFA) concentrations by HPLC analysis (1 ml) as described in Rooke et al. (2014).
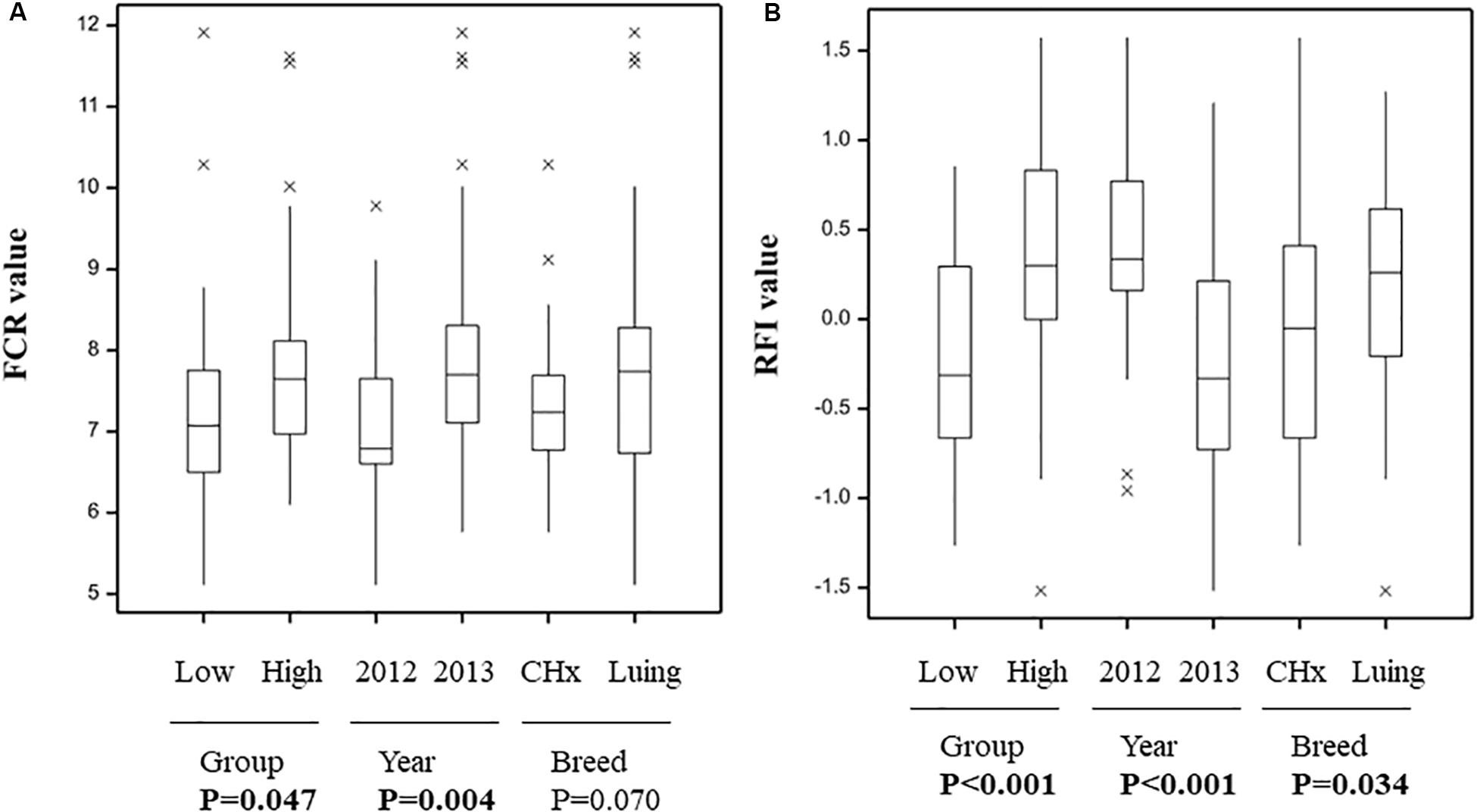
Figure 1. Variation in feed conversion ratio (A) and residual feed intake (B) between animals grouped based on feed efficiency indicators year and breed. P-value as indicator of significant difference (in bold when P < 0.05) between Low and High efficient animals.
Metagenomics Annotation and Analysis
To get information on the rumen microbial communities and their genes, all rumen samples were collected at the abattoir as previous results confirmed high microbiome similarity between rumen fluid samples collected using stomach tube and rumen digesta collected at the abattoir (Wallace et al., 2014; Snelling et al., 2019). DNA was extracted from the rumen digesta samples following the protocol from Yu and Morrison (2004) and was based on repeated bead beating with column filtration. The procedure is fully described in Rooke et al. (2014).
Illumina TruSeq libraries were prepared from genomic DNA and sequenced on an Illumina HiSeq 4000 instrument by Edinburgh Genomics (Edinburgh, United Kingdom). Paired-end reads (2 × 100 bp) were generated, resulting in between 8 and 15 GB per sample (between 40 and 73 million paired reads) with on average 73% passing quality check and being subsequently annotated. Bioinformatics analysis followed the same procedure as previously described in Roehe et al. (2016) and Wallace et al. (2015). In order to measure the abundance of known microbial genes in the rumen samples, reads from whole metagenome sequencing were aligned to the Kyoto Encyclopedia of Genes and Genomes (KEGG)1 database. The KEGG Orthologue groups (KO) of all hits that were equal to the best hit were examined. In the case we were unable to resolve the read to a single KO, the read was ignored; otherwise, the read was assigned to the unique KO. Statistical analysis of the metagenomics samples was based on the complete sample profiles as expressed by the pattern of metagenomic reads classified within KEGG orthologue groups with >90% similarity and belonging to a single KEGG orthologue (KO) groups. The alignment of the reads generated by whole metagenomic sequencing to the KEGG genes database resulted in identification of 4,427 microbial genes for each animal. Microbial genes were expressed in relative abundance (percentage) within animal and only those with a relative abundance greater than 0.001% (n = 1,630) were carried forward for downstream analysis.
For phylogenetic annotation, the genomic reads were aligned to a custom database using Kraken combining several databases including genomes from the Hungate 1,000 collection and metagenome-assembled genomes (MAGs) from beef rumen samples (Wood and Salzberg, 2014; Stewart et al., 2018). All taxonomic labels identified are subsequently described as the genus having the highest similarity with the identified genome or MAG and applying the same cutoff used in previous MAG study (Stewart et al., 2018) (estimated completeness ≥ 80% and estimated contamination ≤ 10%). As for microbial genes, microbial genera identified (n = 1,058) were normalized between animals expressing them as relative abundances only those with a relative abundance greater than 0.001% (n = 1,630) were carried forward for downstream analysis. The absence of “0” abundancies within the dataset was confirmed.
Following this step, 27 genes (within 1,630 genes), 42 phyla and 1,058 genera were selected for the statistical analysis. These microbial genes were selected based on their functions associated with different microbiome-host mucosa interaction mechanisms including flagella, pilus, secretion system, biofilm formation and fucose or sialic acid metabolism in order to answer to our hypotheses. The 27 genes were selected based on biological evidence that bacteria carrying such genes have the capacity to interact with the host. Based on our hypothesis, such genetic capacities could potentially affect animal performance in term of feed efficiency.
Information on the gene content within metagenome-assembled genomes (Stewart et al., 2018) was used to validate the microbial genera identified as highly important to explain variation in animal feed efficiency. MAGs with the highest % of similarity and query coverage using the Genome Taxonomy Database (GTDB) were selected as best hit and the most probable bacterial taxa carrying each particular gene studied.
Metagenomics raw sequencing data combined with metadata of the animal experiments can be downloaded from the European Nucleotide Archive under accession PRJEB10338 and PRJEB31266.
Statistical Analysis
Methodologies to analyze metagenomics data by General Linear Model (GLM; including year of the trial and breed type as fixed effects), Principal Coordinate Analysis (PCoA) and Canonical Variate Analysis (CVA) using Gen-Stat 16th edition (VSN International Ltd., United Kingdom), and Partial Least Square (PLS) analysis using SAS (Version 9.1 for Windows, SAS Institute Inc., Cary, NC, United States) were similar to those described in Auffret et al. (2017). Results were considered as significantly different when the P-value was P < 0.05. In order to identify the influence of microbial variables (genes or genera) and potential mechanisms that explained most of the variation in FCR and RFI, different Partial Least Square (PLS) models per gene were performed. Each model was built considering RFI or FCR as dependent variables, year and breed as fixed effects and each microbial gene as explanatory variables (Script in Supplementary Data). The most influential microbial genes or genera from each model that were important in explaining RFI or FCR were selected based on the variable importance for projection (VIP) criterion (Wold, 1995) whereby microbial genes with a VIP <0.8 contribute little to the prediction.
PLS analysis was first applied to identify the genes within the 27 preselected genes mostly explaining variation in FCR or RFI. This methodology was successfully applied to identified microbial biomarkers explaining variation in several beef cattle traits as shown in Auffret et al. (2017, 2018) and Lima et al. (2019). Secondly, a similar analysis was applied to determine the microbial genera mostly explaining variation in FCR or RFI. In parallel, using data from Stewart et al. (2018) the MAGs carrying the genes identified by PLS explaining variation in FCR or RFI were identified and such information was combined with the genera results to determine the microbial taxa carrying one particular gene identified as important to explain variation in RFI or FCR. Importance of individual VFA and acetate-to-propionate ratio was investigated using PLS analysis (breed and year as fixed effects) in addition to the 27 selected microbial genes. The acetate-to-propionate ratio was calculated and considered as a proxy for rumen pH, accepting that whilst the relationship between the two is generally strong, it is not exactly linear (Russell, 1998).
A Venn diagram was generated using Venny software (Oliveros, 2007) to compare the similarity in term of microbial genes or genera explaining most of the variability in FCR or RFI.
In addition, Linear Discriminant Analysis (LDA) using the LDA function in R (version 3.5.1.) was applied on 13 microbial genes and 128 microbial genera, all identified by PLS as important to explain the variation observed in animals with significantly different RFI and FCR values.
A prediction accuracy value (%) was calculated as indicator of accurate identification of Low or High feed efficiency animals based on the selected genes or genera.
Results
Differences in Microbial Activities and Host Feed Efficiency Between Groups of Differently Feed Efficiency Animals
In this study, “High” animals have a low FCR and RFI values meaning they are efficient to convert feed into live weight gain, whilst “Low” animals performing in the opposite way.
All animals offered concentrate diet were selected and showed significant differences between groups of animals based on FCR or RFI (Figure 1), also confirming the grouping selection based on feed efficiency indicators and balanced for year of the trial and breed (Supplementary Table S1). Furthermore, significant differences between groups of animals based on years of the trial and breeds were found (Figure 1). Only the differences between breeds for FCR showed a tendency (P = 0.07).
Microbial activities associated with gas production including methane emissions (Supplementary Figure S1) or VFA (Supplementary Figure S2) were not significantly different between Low and High animals and the acetate-to-propionate ratio used as a proxy for rumen pH was not significantly different neither between the two groups of animals (Supplementary Figure S2C).
Variation in the Rumen Microbiome Composition Between Low and High Feed Efficiency Animals
The microbial community composition studied at the genus level showed a limited difference using Principal Coordinate Analysis (PCoA) between animals identified with Low- compared to High feed efficiency (Figure 2). This result was confirmed by Canonical Variate Analysis (CVA) as indicated by the overlapping of the 95% confidence circles between the two animal groups (Supplementary Figure S3).
Within 1,058 genera with a relative abundance above 0.001%, 379 genera were found significantly different (P< 0.05) by GLM based on the samples grouping (Supplementary Table S2). Genera within Proteobacteria phylum represent 35% of the 379 genera (Supplementary Figure S4) followed by genera within Firmicutes (16%) and Actinobacteria (17%). Within the 132 Proteobacteria genera, 32 were significantly higher in the Low feed efficiency group whilst 100 were dominant (P < 0.05) in the other group (Supplementary Table S2). Within Firmicutes and Bacteroidetes, Prevotella (Bacteroidetes) was the most dominant species significantly higher in the Low group like the Firmicutes genera Eubacterium, Sharpea, Clostridium, Lactobacillus, and Paenibacillus. Bifidobacterium and Ilumatobacter (Actinobacteria) were also significantly higher in the Low group. In the High feed efficiency group, genera associated with metabolic activities such as Succiniclasticum and Succinivibrio (succinate), Acidaminococcus (amino acids), Sarcina and Fibrobacter (cellulose) were all significantly more abundant.
Genera associated with Staphylococcus (Firmicutes), the protist Eimeria (Alveolata), Sphaerochaeta and Treponema (Spirochaetes), Vibrio and Lawsonia (Proteobacteria) known to be potential pathogens were all significantly more abundant in the High group.
Selected Rumen Microbiome Genes and Potential Mechanisms Explaining Variation in FCR and RFI
Genes selected for this study and associated with biofilm formation (K01335 and K01840), secretion system (K02005) and hemolysin synthesis were found significantly (P < 0.05) higher in the Low group by GLM analysis (Table 1). On the other hand, genes associated with sialic acid production (K01654), secretion systems (K01993 and K02454), pilus (K02283, K02652, K02653, K02662, and K02666), and flagellum (K02412), were significantly more abundant in High animals (Table 1).
Eight microbial genes explained 39 and 40% of the variability in FCR and RFI, respectively, as determined by Partial Least Square (PLS) analysis (Table 2). Genes associated with biofilm formation (K01840), Type I secretion system (K02005), hemolysin synthesis (K11068) and fucose sensing (K02429) showed a negative correlation with FCR whilst two genes encoding for general and Type IV of secretion systems (K02454 and K03205) and two genes encoding for Type IV pilus (K02652 and K02653) were positively correlated with FCR. Genes K02454, K02652, and K02653 were also found correlated with RFI (Figure 3A). Other genes positively correlated with RFI encoded for sialic acid synthesis (K01654) and flagellum (K02410) whilst those negatively correlated with RFI were associated with pilus assembly protein (K02283), flagellar hooking protein (K02396), and hemolysin synthesis (K06442).
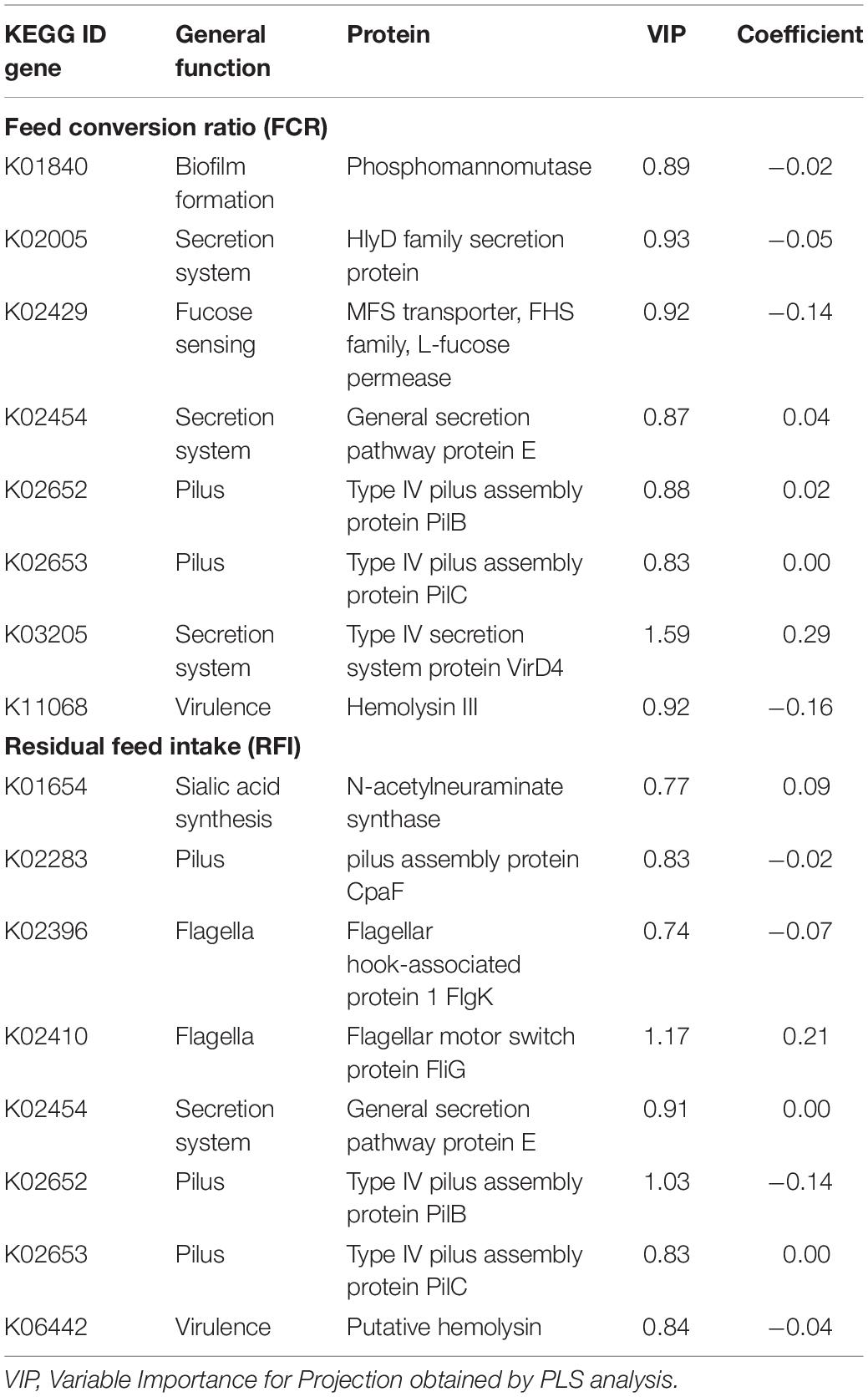
Table 2. Partial Least Square results for the microbial genes explaining the variability in feed conversion ratio and residual feed intake.
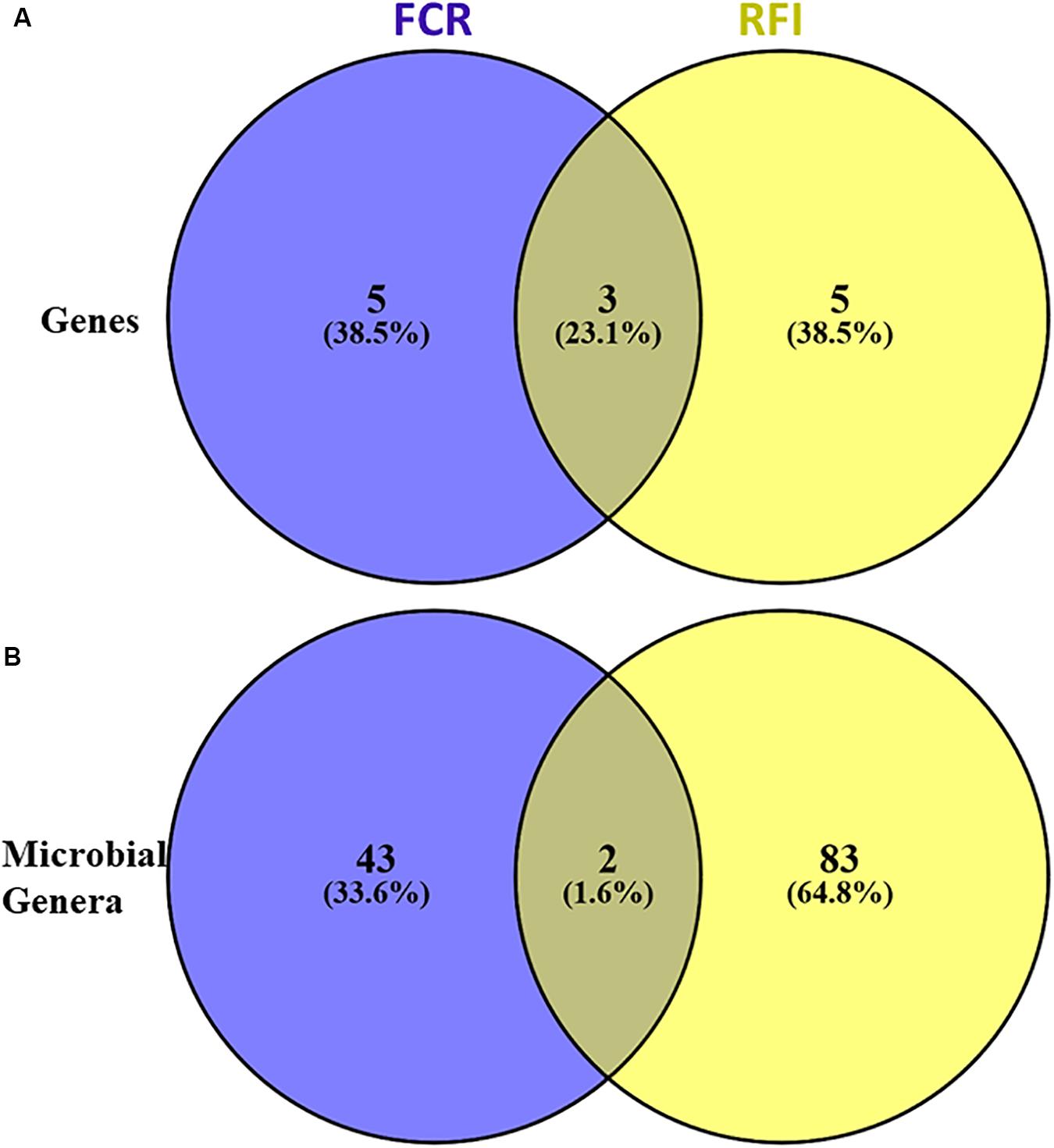
Figure 3. Venn diagram representing the microbial genes (A) and genera (B) associated with feed conversion ratio (FCR) and residual feed intake (RFI).
Inclusion of VFA data and the acetate-to-propionate ratio as proxy for the rumen pH into the PLS analysis in addition to the 27 selected genes did not improve the percentage of variation explained by the model for FCR and RFI, as indicated by VIP values of these additional variables being in general below 0.8 except for branched chain fatty acids showing a negative effect on RFI (Supplementary Table S3).
Identification of Potential Microbial Species Carrying Studied Genes and Explaining Variation in FCR and RFI
Using a PLS approach, a list of genera found highly correlated (VIP > 0.8) with the selected genes was identified (Supplementary Table S4) and this list was refined using gene content information from the MAGs previously generated in Stewart et al. (2018). In parallel, 45 and 85 genera were also found significantly correlated by PLS with FCR or RFI, respectively (Figure 3B), also explaining 60 and 52% of the variability observed in FCR and RFI, respectively (Supplementary Table S5).
Only two genera associated with Gordonibacter (Actinobacteria) and Sarcina (Firmicutes) were found both correlated with FCR and RFI.
In parallel to PLS analysis for the identification of biomarkers associated with FCR or RFI, LDA was applied to determine the prediction accuracy of Low compared to High feed efficiency animals and showed 81 or 90% of prediction accuracy using 13 genes (Figure 4A) or 148 microbial genera (Figure 4B), respectively.
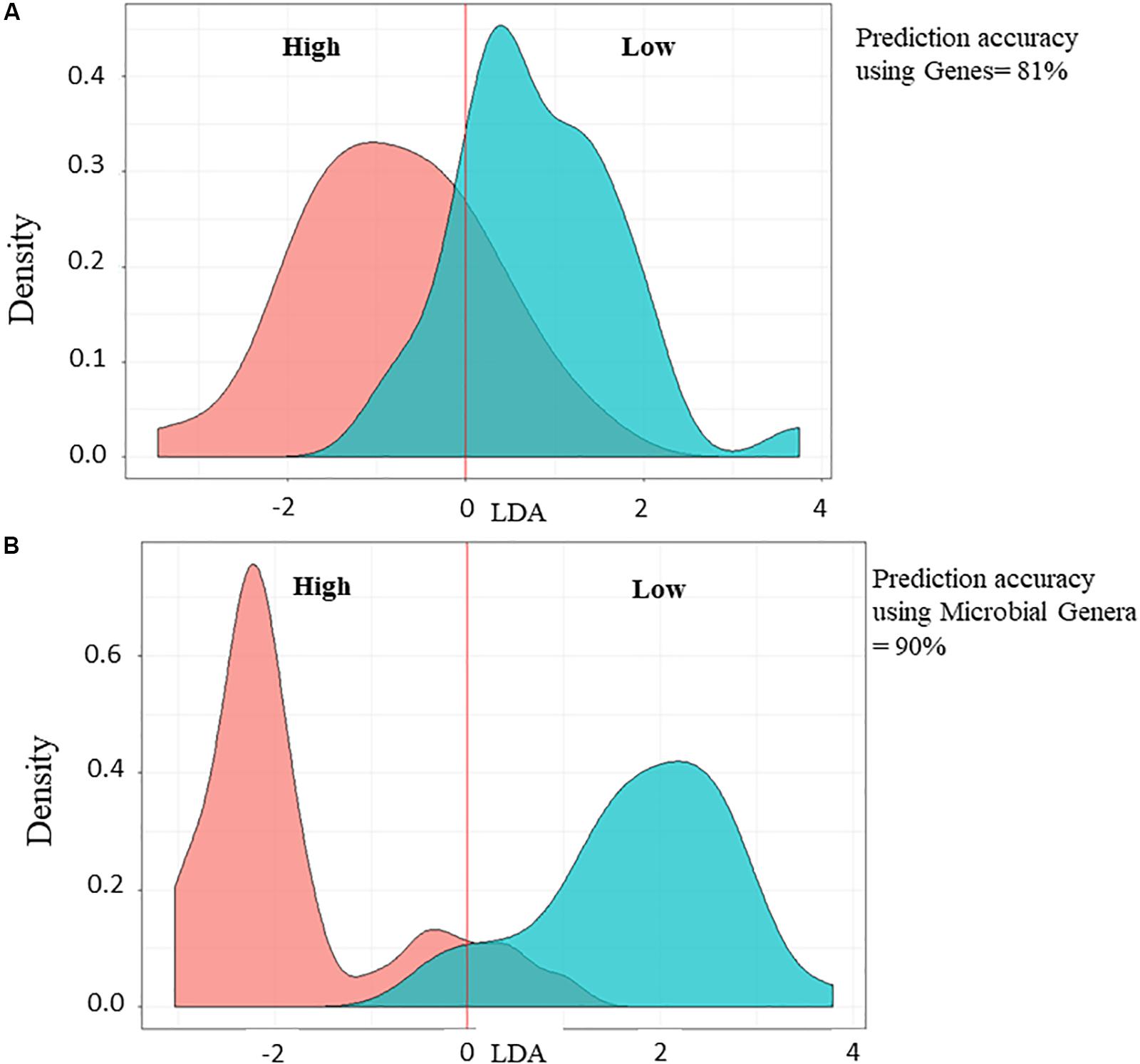
Figure 4. Prediction accuracy analysis using Linear Discriminant Analysis for the validation of the selected microbial genes (A) and genera (B). Low and High feed efficiency animals are represented in green curve and red curve, respectively. Percentage of prediction accuracy is indicated.
On average, 22 (±8) genera were significantly correlated with each gene and explained on average 52 ± 8% of the variability observed per gene. Over the 37 genera identified as highly correlated with at least one of the selected genes showing high correlation with FCR and/or RFI, 19 genera belonged to Proteobacteria and 8 Proteobacteria genera correlated with one of the genes showed a beneficial effect on feed efficiency (negative coefficient value). Genera within Actinobacteria (4/19) and Firmicutes (6/19) were also identified as important populations carrying genes related to adhesion activities.
The genera related to Eubacterium (Firmicutes) were found highly correlated with four genes encoding for the production of pilus (K02283 and K02652), flagella (K02396) and a putative hemolysin (K06442) and always negatively correlated with RFI (Table 4). Although Eubacterium was composed of 10 different species or variants based on GTDB database (Supplementary Table S6), species Q, H, and A were the most abundant constituting Eubacterium. Such diversity of species identified as important to explain variation in host feed efficiency was not observed in the other genera in our results (Supplementary Table S6).
Other genera identified as Succiniclasticum (Firmicutes, K01840 and K2005), Lactobacillus (Firmicutes, K02429), and Bifidobacterium (Actinobacteria, K11068) were all found negatively correlated with FCR or RFI. On the other hand, some genera were found highly correlated by PLS with two genes and also positively correlated with FCR or RFI (Tables 3, 4). For example, Desulfococcus and Variovorax within Proteobacteria and Sphaerochaeta (Spirochaetes) with genes involved in secretion system (K02454) and type IV pilus (K02653); the Spirochaetes Treponema was found positively correlated with FCR and genes involved in the formation of Type IV pilus (K02652) or secretion system (K03205). Finally, several genera within Proteobacteria were all found positively correlated with RFI and also highly correlated with genes encoding for sialic acid synthesis (K01654 with Lawsonia and Succinivibrio), pilus formation (K02283 with Pannonibacter) and flagella formation (K02410 with Providencia).
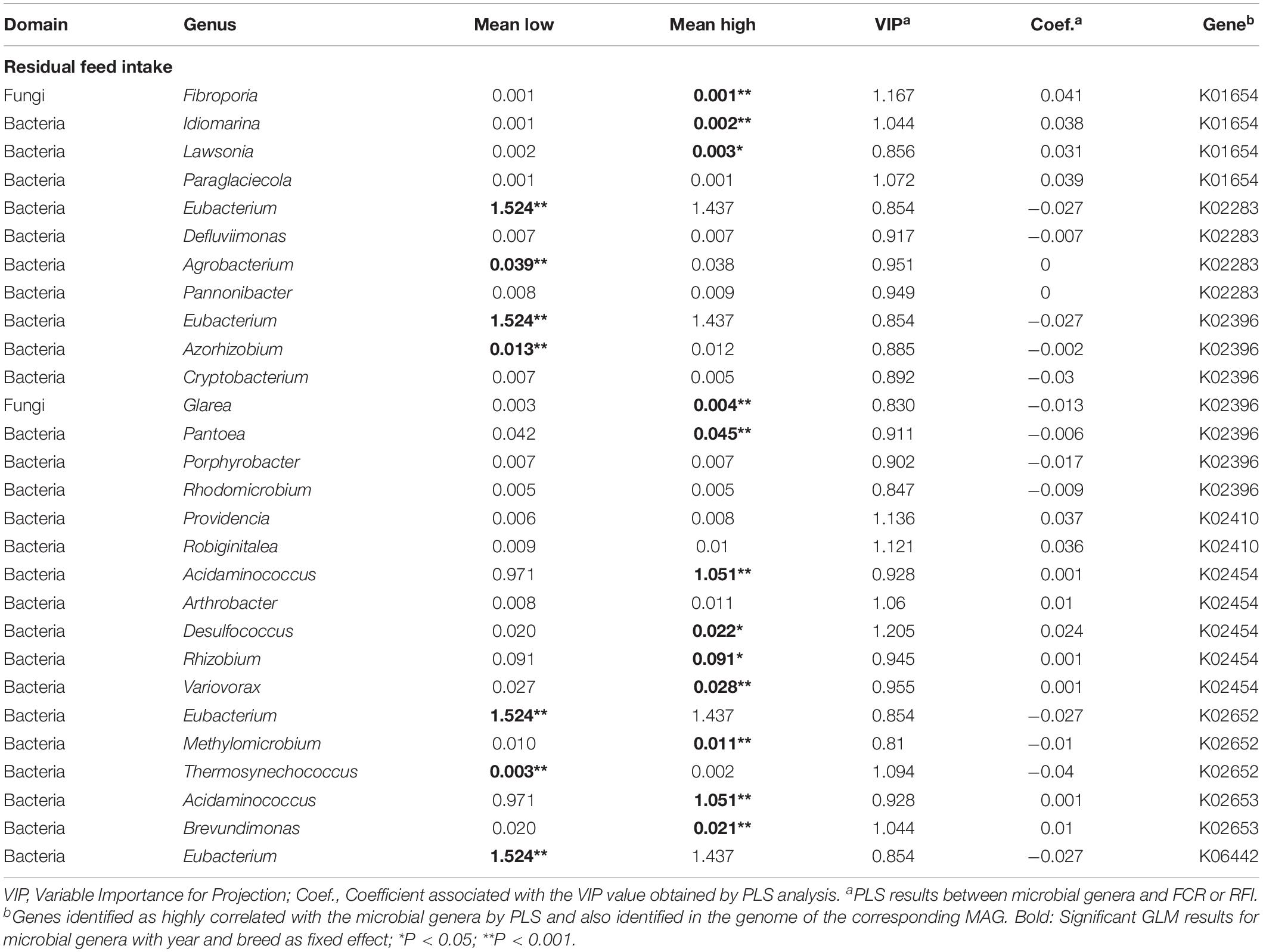
Table 3. Identification of microbial genera (including MAGs) and genes significantly correlated with residual feed intake.
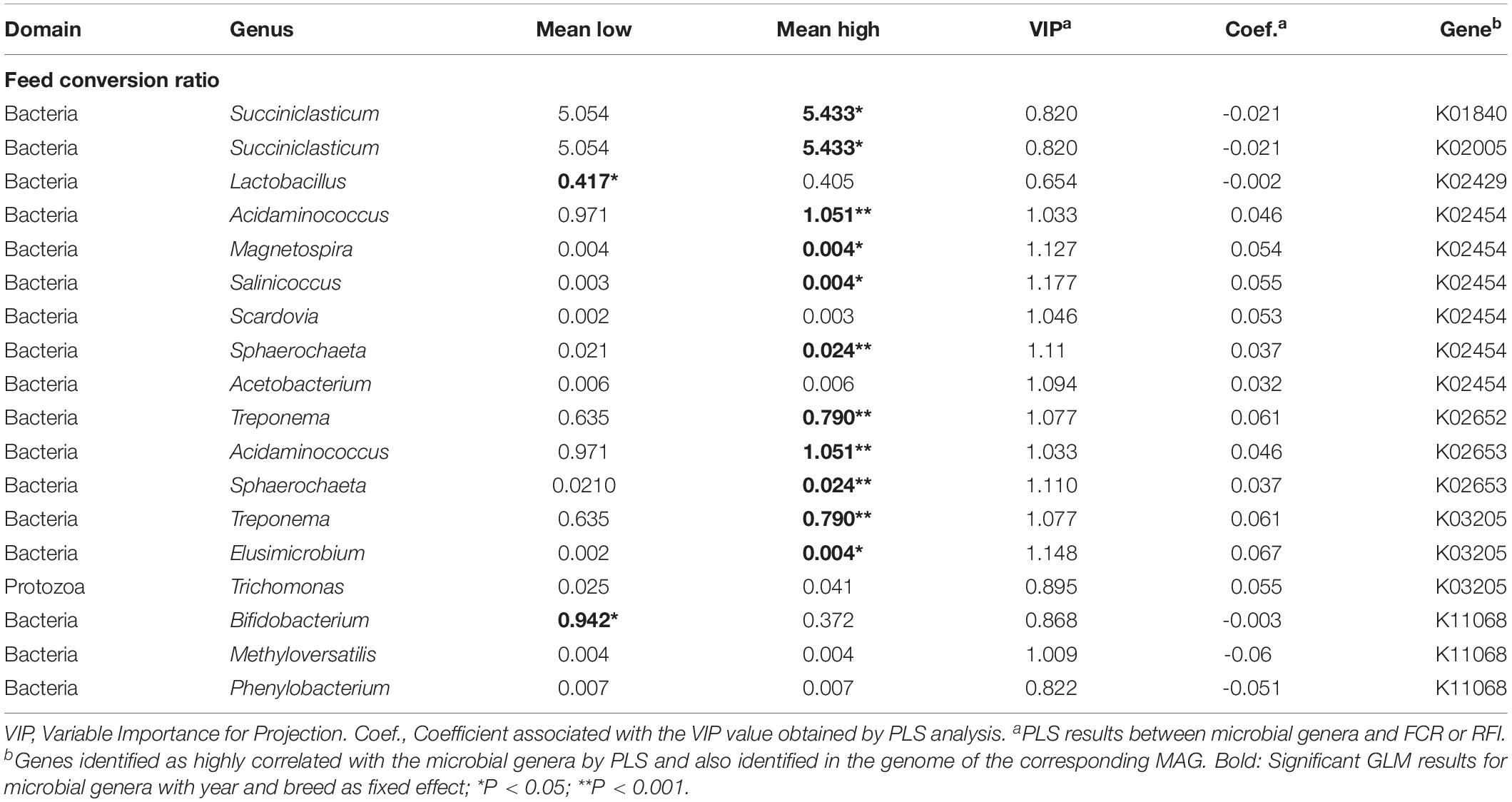
Table 4. Identification of microbial genera (including MAGs) and genes significantly correlated with feed conversion ratio.
Some other genera known to be involved in metabolite synthesis like the amino acid degrader Acidaminococcus (Firmicutes) was found positively correlated with FCR and RFI and also highly correlated with genes for the formation of secretion system (K02454) and Type IV pilus (K02653). Bacterial genera like Azorhizobium or Agrobacterium (both Proteobacteria) are generally interacting with plant tissue and in this study found highly correlated with flagella (K02396) or pilus (K02283) formation, respectively.
Discussion
This is one of the first reports that identified and demonstrates the importance of particular rumen microbial genetic capacities significantly affecting animal feed efficiency using a large dataset from two independent experiments.
Contribution of the Rumen Microbial Communities in Low and High Feed Efficient Beef Cattle
It is already well-known that inter-animal variation in feed efficiency exists among cattle of different breeds and receiving the same diet (Crowley et al., 2010; Cantalapiedra-Hijar et al., 2018). As a result, production cost can increase and was calculated in the current study (ca. on average 71 ± 32 Ł/animal between efficient and less efficient animals). Furthermore, grouping animals between low and high feed efficiency can help identifying possible microbial mechanisms explaining such variation (Roehe et al., 2016).
Our hypothesis was that differences observed in feed efficiency in cattle offered a high concentrate-based diet can be linked to differences in the rumen microbiome. Such animal-to-animal variation in feed efficiency was not significantly explained by differences in the entire rumen microbiota structure in agreement with previous work (reviewed in Cantalapiedra-Hijar et al., 2018). However, significant differences in feed efficiency between animal groups were mainly explained by a limited number of microbial genes and genera.
Some of the identified genera (e.g., Succiniclasticum, Eubacterium) were among the most dominant within the communities, partly contrasting with previous results indicating a lack of correlation between abundant taxa and feed efficiency (Hernandez-Sanabria et al., 2010, 2012). A possible explanation was a better characterization of the rumen microbiome following the recent advances in bioinformatics and culturomics (Seshadri et al., 2018; Stewart et al., 2018) and the release of thousands of new MAGs and complete rumen genomes used in this study. Furthermore, this study took advantages of such knowledge to identify the impact of microbial genes carried with certainty in the genome of rumen bacterial genera on host feed efficiency.
Importance of Microbial Biomarkers Explaining Differences in Feed Efficiency in Beef Cattle
Contrasting with previous reports mostly using microbial community composition data (16S rRNA gene sequencing), we used microbial gene information from metagenomics sequencing data to study the link between microbial genetic capacities and animal feed efficiency. Using a non-targeted approach, Lima et al. (2019) identified a limited number of microbial genes explaining between 63 and 65% of the variability in FCR and RFI. In this study, a limited number of microbial genes selected based on previous biological evidence (Pickard and Chervonsky, 2015; Ribet and Cossart, 2015; Pitta et al., 2016; Auffret et al., 2017) allowed to explain about 40% of variability in FCR and RFI.
It is known that high concentrate diets can induce gastrointestinal dysbiosis including acidosis (Nagaraja et al., 1998) and an increase in pathogenic bacteria carrying genes associated with host mucosa interaction and pathogenicity mechanisms (Auffret et al., 2017; Sicard et al., 2017) in some animals. Although most of the work on rumen dysbiosis is related to cattle health, we hypothesized that such microbial genetic capacities, enriched in the rumen microbiome of animals more susceptible to high concentrate diet, are one of the potential reasons leading to an overall reduction in animal performance (Ramsey et al., 2005; Qin et al., 2010; Elolimy et al., 2018).
The combination of different bioinformatic and modeling methodologies used in this study helped to identify potential microbial genetic capacities and mechanisms significantly affecting beef cattle feed efficiency. In addition, the use of both FCR and RFI was advantageous to identify these genera whilst partly differentiating the genes correlated with each indicator. Moreover, the microbial genes and genera identified by PLS as important to explain variation in FCR and RFI were also confirmed as good predictors using LDA (prediction accuracy between 81 and 90%) for the identification of Low compared to High feed efficiency animals.
Possible Microbial Mechanisms Detected in High Feed Efficient Beef Cattle
Possible mechanisms affecting beef cattle feed efficiency could be the result of microbial mechanisms (taxa and their metabolites) happening in the rumen or the lower gut as recent evidence suggests that both gastrointestinal sections can communicate (Steele et al., 2016). However, such interaction is not well-characterized and the exact mechanisms of gastrointestinal cross-talk in ruminants need further work.
Based on this study, several microbial mechanisms significantly more abundant in High efficient animals were identified and most of them could be involved in the rumen as well as in the lower intestine (see Table 2).
For example, the species related to Eubacterium were always significantly negatively correlated with RFI. Eubacterium is one of the dominant and major ruminal genera involved in ruminal cellulose degradation (Kozakai et al., 2007) and was found significantly more abundant in low RFI beef cattle (Elolimy et al., 2018). Similar result was described as diet dependent (concentrate) for low RFI animals (Hernandez-Sanabria et al., 2012). This genus characterized by a high diversity of potential species were confirmed carrying genes encoding for secretion system, hooking flagella and pilus formation both known to be widespread within bacteria and enhancing the capacity of the species to adhere on surface for epithelium colonization (Karuppiah et al., 2013; Green and Mecsas, 2016). In addition, some Eubacterium species produce butyrate and utilize acetate (Flint et al., 2007) both activities identified in high efficient animals also providing a higher energy source for the animal. As for Eubacterium, Succiniclasticum, and Lactobacillus are genera known to be involved in the synthesis of particular metabolites like propionate from succinate (Van Gylswyk, 1995) or lactate subsequently absorbed across the ruminal epithelium.
From the rumen microbiome, two possible mechanisms (fucose sensing and hemolysin synthesis) recognized to specifically impact the lower gut were also identified. For example, Succiniclasticum and Lactobacillus genera both significantly more abundant in low FCR animals were confirmed carrying genes involved in biofilm formation, secretion system, and more importantly fucose sensing allowing the strains to adapt to rumen and intestinal conditions (Van Gylswyk, 1995; Myer et al., 2015). For example, Lactobacillus genus is generally more abundant in ruminants fed with high concentrate diet (Wells et al., 1997) and will degrade fucosylated mucin recovering intestinal mucosa (but not rumen; Steele et al., 2016) using the gene K02429 as a strategy develop by the host to feed symbiotic and commensal bacteria and to regulate bacterial intestinal colonization including pathogenic colonization (Pacheco et al., 2012; Pickard and Chervonsky, 2015). However, how these mechanisms are triggered from the rumen to the intestine need further research.
In addition of adhesion genes, Eubacterium, the lactate producer Bifidobacterium and Phenylobacterium genera have genes encoding for hemolysin which were also associated with low RFI (K06442) and low FCR (K11068). Although hemolysin is generally produced by pathogenic bacteria for nutrient acquisition by initiating host cell lysis, it has been suggested that hemolysin can promote activation of inflammasome signals reducing pathogen colonization in the intestine (Chen et al., 2018). Results related to Eubacterium might be a good example of possible gastrointestinal cross-talk from bacteria detected in the rumen having a possible effect on the lower gut. More work is needed to confirm this mechanism in the rumen and the lower gut.
Therefore, our results suggest that the influences of beneficial bacteria on animal feed efficiency can be mediated by direct bacteria-cell contacts or indirectly via bacterial metabolites, such as butyrate or propionate or antipathogenic compounds from commensal bacteria.
Possible Microbial Mechanisms Detected in Low Feed Efficient Beef Cattle
Identified mechanisms impacting host feed efficiency and located in the rumen involved two Spirochaeales genera, Treponema and Sphaerochaeta. These two genera were both positively correlated with FCR and genes associated with type IV pilus (K02652 and K02653) or secretion system (K03205). Both genera are known to inhabit the rumen with potential pathogenic activities (Smibert, 1984). Furthermore, some species within Treponema and Sphaerochaeta in the rumen showed pectinolytic activities instead of being pathogens (Xie et al., 2018) and also producing acetate, a lower energy source for the animal in comparison with butyrate and therefore potentially reducing feed efficiency (Stanton and Canale-Parola, 1980; Abt et al., 2012).
In addition to bacteria, the protozoan genus Trichomonas had a negative effect on feed efficiency (FCR). Generally, this genus dominates total protozoa in the rumen and the presence of protozoa compared to defaunated animals is known to reduce feed conversion efficiency in ruminants (Newbold et al., 2015). This genus was correlated with a type IV secretion system gene (K03205) one indicator of the presence of intra-ciliate Proteobacteria using T4SS to invade and survive in ruminal protozoa (Park and Yu, 2018). However, the importance of this relationship in explaining differences in feed efficiency is unclear and needs further work.
Contrasting with beneficial bacteria producing volatile fatty acids (VFA) such as butyrate or propionate, Acidaminococcus species are amino acid-fermenting bacteria generally located in the rumen (Cook et al., 1994). This genus through its metabolic activities in the rumen was reported to be involved in lower gut microbiome dysbiosis and gut disorder through amino acids metabolism disturbance (Lin et al., 2017). Moreover, this genus could have a contrasting impact on the physiological aspects of the host (Lin et al., 2017) like weight gain (Gough et al., 2015; Yun et al., 2017).
Other ruminal microbial species carrying genes or associated with mechanisms to colonize specifically the intestinal mucosa surface whilst avoiding the host immune system were identified and potentially having a negative impact on animal feed efficiency.
Five Proteobacteria genera were all positively correlated with high RFI. It included the motile enteric bacteria Providencia (K02410) which is an opportunistic pathogen in cattle normally infecting the urinary tract (Blaiotta et al., 2016). Also, the sulfate reducing bacterium Desulfococcus and Variovorax were both correlated with the T1SS gene (K02454) conferring the ability to release hemolytic toxin in a broad range of host cells (Thomas et al., 2014). Finally, Lawsonia was also identified and correlated with a sialic acid synthesis gene (K01654). To the best of our knowledge, this is one of the first times that Lawsonia known to be an obligate intracellular enteric pathogen in several animals including pig and horse (Vannucci and Gebhart, 2014) is reported in beef cattle. This genus seems to have developed a mechanism avoiding the host immune system by the production of sialic acid which is one of the main compounds constituting the mucus covering the intestine (Severi et al., 2007; Quintana-Hayashi et al., 2018). Similar mechanisms these genera may use to avoid the host immune system of the epithelia cells in the rumen. For example, Mann et al. (2018) showed that one microbial gene encoding for N-acetylneuraminate synthase and involved in sialic acid synthesis was highly expressed in the rumen microbiome in all cattle tested.
Link Between Methane Emissions and Feed Efficiency in Beef Cattle
In this study, animals were fed with high concentrate diet during the finishing period to increase feed efficiency and productivity. Such diet may reduce methane emissions compared to animals receiving forage diet (Duthie et al., 2017; Cantalapiedra-Hijar et al., 2018). One of the surprising results was the lack of significant difference in methane emissions between low and high feed efficient animals whilst expected (Fitzsimons et al., 2013). Furthermore, there were no differences in CO2 and H2 emissions and VFA concentrations between both animal groups. Although the link between methane emissions and feed efficiency was not the primary aim of this study, one possible explanation for this lack of difference in methane emissions could be related to VFA metabolisms and absorption. For example, microbial metabolisms releasing of butyrate or propionate instead of acetate is known to divert H2 away from methanogenesis potentially reducing methane emissions and improving ruminant feed efficiency (Zhou et al., 2009; Shabat et al., 2016). However, demonstrating a higher metabolite production like for individual VFA is challenging as the rate of absorption into the blood stream is directly under the control of rumen pH as well as metabolite concentration and therefore cannot be easily determined using rumen digesta samples (Dijkstra et al., 1993). Another explanation could be due to the gap between the recording period of feed efficiency and the allocated time in the respiration chambers leading to a weak or a lack of significant correlation (Mercadante et al., 2015; Alemu et al., 2017).
This study identified both microbial genetic capacities and the microorganisms carrying the genes related and explaining a significant variability (∼40%) observed in beef cattle feed efficiency. Although knowledge about the dynamics of exchange between communities from the digesta with mucosa or between rumen digesta and lower gut is limited (Malmuthuge et al., 2015; Steele et al., 2016), it is expected that populations within the rumen digesta will compete for space and nutrients with rumen epithelia tissue-attached communities (epimural communities) also releasing metabolites and therefore impacting on the host (Pitta et al., 2016; Zhan et al., 2019). However, we acknowledge that our results are a primary step in the identification of microbial parameters impacting on host feed efficiency prior to in vitro or in vivo validation. Furthermore, other factors related to microbiome (e.g., metabolic pathways) or host responses (e.g., metabolites absorption, immune responses or behavior) are important to explain variation in host feed efficiency (Cantalapiedra-Hijar et al., 2018; Lima et al., 2019).
Conclusion
In conclusion, microbial species carrying genes involved in adhesion and host-microbiome interaction in the rumen digesta seem to be important mechanisms explaining significant differences in animal feed efficiency but potentially sharing similar adhesion mechanisms (e.g., Type IV pilus). Based on our results, detrimental species were involved in sialic acid synthesis, and carried flagella for motility or type IV secretion system. Some others could be related to VFA and amino acid metabolisms. In contrast, beneficial bacteria, especially Eubacterium had the genetic capacities to form biofilms or to release hemolysin that could stimulate the immune system against pathogens in the lower gut Moreover, these species had type II secretion system and flagella for hooking and the capacity to degrade fucose as mucosal compound produced by the host intestinal epithelium.
Finally, more work is needed to better understand the dynamics and importance of exchange between microbial populations colonizing epithelial cells or the lumen during the gastrointestinal crosstalk. Such information could be used to develop molecular tools for the identification of possible probiotics or biomarkers, with the aim to improve animal production and health dietary intervention or animal breeding.
Data Availability Statement
The datasets generated for this study can be found in the Metagenomics raw sequencing data combined with metadata of the animal experiments can be downloaded from the European Nucleotide Archive under accession PRJEB10338 and PRJEB31266.
Ethics Statement
The animal experiment was conducted at the Beef and Sheep Research Centre of Scotland’s Rural College (SRUC, Edinburgh, United Kingdom). The experiment was approved by the Animal Experiment Committee of SRUC and was conducted in accordance with the requirements of the UK Animals (Scientific Procedures) Act 1986. Written informed consent was obtained from the owners for the participation of their animals in this study.
Author Contributions
MA and RR conceptualized the study. MA and MW carried out the formal analysis. MA wrote the manuscript. MA, RD, C-AD, RS, MW, and RR reviewed and edited the manuscript. All authors read and approved the final manuscript.
Funding
This project was supported by grants from the Biotechnology and Biological Sciences Research Council (BBSRC BB/N01720X/1, BB/N016742/1, BB/S006567/1, and BB/S006680/1) and the Scottish Government. This research was based on data from experiments funded by the Scottish Government as part of the 2011–2016 commission, Agriculture and Horticulture Development Board (AHDB) Beef & Lamb, Quality Meat Scotland (QMS), and Department for Environment Food & Rural Affairs (Defra).
Conflict of Interest
The authors declare that the research was conducted in the absence of any commercial or financial relationships that could be construed as a potential conflict of interest.
Acknowledgments
We thank Dr. Irene Cabeza Luna, Joana Lima, Laura Nicoll, Lesley Deans, and Claire Broadbent for the excellent technical support.
Supplementary Material
The Supplementary Material for this article can be found online at: https://www.frontiersin.org/articles/10.3389/fmicb.2020.01229/full#supplementary-material
Footnotes
References
Abt, B., Han, C., Scheuner, C., Lu, M., Lapidus, A., Nolan, M., et al. (2012). Complete genome sequence of the termite hindgut bacterium Spirochaeta coccoides type strain (SPN1T), reclassification in the genus Sphaerochaeta as Sphaerochaeta coccoides comb. nov. and emendations of the family Spirochaetaceae and the genus Sphaerochaeta. Stand. Genomic Sci. 6, 194–209. doi: 10.4056/sigs.2796069
Alemu, A. W., Vyas, D., Manafiazar, G., Basarab, J. A., and Beauchemin, K. A. (2017). Enteric methane emissions from low- and high-residual feed intake beef heifers measured using GreenFeed and respiration chamber techniques. J. Anim. Sci. 95, 3727–3737. doi: 10.2527/jas.2017.1501
Auffret, M. D., Dewhurst, R. J., Duthie, C.-A., Rooke, J. A., Wallace, R. J., Freeman, T. C., et al. (2017). The rumen microbiome as a reservoir of antimicrobial resistance and pathogenicity genes is directly affected by diet in beef cattle. Microbiome 5:159.
Auffret, M. D., Stewart, R., Dewhurst, R. J., Duthie, C.-A., Rooke, J. A., Wallace, R. J., et al. (2018). Identification, comparison, and validation of robust rumen microbial biomarkers for methane emissions using diverse Bos taurus breeds and basal diets. Front. Microbiol. 8:2642. doi: 10.3389/fmicb.2017.02642
Bang, C., Ehlers, C., Orell, A., Prasse, D., Spinner, M., Gorb, S. N., et al. (2014). Biofilm formation of mucosa-associated methanoarchaeal strains. Front. Microbiol. 5:353. doi: 10.3389/fmicb.2014.00353
Blaiotta, G., Di Cerbo, A., Murru, N., Coppola, R., and Aponte, M. (2016). Persistence of bacterial indicators and zoonotic pathogens in contaminated cattle wastes. BMC Microbiol. 16:87. doi: 10.1186/s12866-016-0705-8
Brown, K., DeCoffe, D., Molcan, E., and Gibson, D. L. (2012). Diet-induced dysbiosis of the intestinal microbiota and the effects on immunity and disease. Nutrients 4, 1095–1119. doi: 10.3390/nu4081095
Cantalapiedra-Hijar, G., Abo-Ismail, M., Carstens, G. E., Guan, L. L., Hegarty, R., Kenny, D. A., et al. (2018). Review: biological determinants of between-animal variation in feed efficiency of growing beef cattle. Animal 12, s321–s335. doi: 10.1017/S1751731118001489
Chen, S., Yang, D., Wen, Y., Jiang, Z., Zhang, L., Jiang, J., et al. (2018). Dysregulated hemolysin liberates bacterial outer membrane vesicles for cytosolic lipopolysaccharide sensing. PLoS Pathog. 14:e1007240. doi: 10.1371/journal.ppat.1007240
Cook, G. M., Rainey, F. A., Chen, G., Stackebrandt, E., and Russell, J. B. (1994). Emendation of the description of Acidaminococcus fermentans, a trans-aconitate- and citrate-oxidizing bacterium. Int. J. Syst. Bacteriol. 44, 576–578. doi: 10.1099/00207713-44-3-576
Crowley, J. J., McGee, M., Kenny, D. A., Crews, D. H. Jr., Evans, R. D., and Berry, D. P. (2010). Phenotypic and genetic parameters for different measures of feed efficiency in different breeds of Irish performance tested beef bulls. J. Anim. Sci. 88, 885–894. doi: 10.2527/jas.2009-1852
Dijkstra, J., Boer, H., Van Bruchen, J., Bruining, M., and Tamminga, S. (1993). Absorption of volatile fatty acids from the rumen of lactating dairy cows as influenced by volatile fatty acid concentration, pH and rumen liquid volume. Br. J. Nutr. 69, 385–396. doi: 10.1079/bjn19930041
Duthie, C.-A., Haskell, M., Hyslop, J. J., Waterhouse, A., Wallace, R. J., Roehe, R., et al. (2017). The impact of divergent breed types and diets on methane emissions, rumen characteristics and performance of finishing beef cattle. Animal 11, 1762–1771. doi: 10.1017/S1751731117000301
Duthie, C.-A., Rooke, J. A., Troy, S., Hyslop, J. J., Ross, D. W., Waterhouse, A., et al. (2016). Impact of adding nitrate or increasing the lipid content of two contrasting diets on blood methaemoglobin and performance of two breeds of finishing beef steers. Animal 10, 786–795. doi: 10.1017/S1751731115002657
Elolimy, A. A., Abdelmegeid, M. K., McCann, J. C., Shike, D. W., and Loor, J. J. (2018). Residual feed intake in beef cattle and its association with carcass traits, ruminal solid-fraction bacteria, and epithelium gene expression. J. Anim. Sci. Biotechnol. 9:67. doi: 10.1186/s40104-018-0283-8
Fitzsimons, C., Kenny, D. A., Deighton, M., Fahey, A. G., and McGee, M. (2013). Methane emissions and rumen fermentation variables of beef heifers differing in phenotypic residual feed intake. J. Anim. Sci. 91, 5789–5800. doi: 10.2527/jas.2013-6956
Flint, H. J., Duncan, S. H., Scott, K. P., and Louis, P. (2007). Interactions and competition within the microbial community of the human colon: links between diet and health. Environ. Microbiol. 9, 1101–1111. doi: 10.1111/j.1462-2920.2007.01281.x
Gough, E. K., Stephens, D. A., Moodie, E. E., Prendergast, A. J., Stoltzfus, R. J., Humphrey, J. H., et al. (2015). Linear growth faltering in infants is associated with Acidaminococcus sp. and community-level changes in the gut microbiota. Microbiome 3:24. doi: 10.1186/s40168-015-0089-2
Green, E. R., and Mecsas, J. (2016). Bacterial secretion systems: an overview. Microbiol. Spectr. 4:10.1128/microbiolspec.VMBF-0012-2015.
Gunsett, F. (1984). Linear index selection to improve traits defined as ratios. J. Anim. Sci. 59, 1185–1193. doi: 10.2527/jas1984.5951185x
Henderson, G., Cox, F., Ganesh, S., Jonker, A., Young, W., Collaborators, G. R. C., et al. (2015). Rumen microbial community composition varies with diet and host, but a core microbiome is found across a wide geographical range. Sci. Rep. 5:14567. doi: 10.1038/srep14567
Herd, R. M., Oddy, V. H., and Richardson, E. C. (2004). Biological basis for variation in residual feed intake in beef cattle. Review of potential mechanisms. Aust. J. Exp. Agric. 44, 423–430.
Hernandez-Sanabria, E., Goonewardene, L. A., Li, M., Mujibi, D. F., Stothard, P., Moore, S. S., et al. (2010). Correlation of particular bacterial PCR denaturing gradient gel electrophoresis patterns with bovine ruminal fermentation parameters and feed efficiency traits. Appl. Environ. Microbiol. 76, 6338–6350. doi: 10.1128/aem.01052-10
Hernandez-Sanabria, E., Goonewardene, L. A., Wang, Z., Durunna, O. N., Moore, S. S., and Guan, L. L. (2012). Impact of feed efficiency and diet on adaptive variations in the bacterial community in the rumen fluid of cattle. Appl. Environ. Microbiol. 78, 1203–1214. doi: 10.1128/AEM.05114-11
Hungate, R. E. (1967). Hydrogen as an intermediate in the rumen fermentation. Arch. Mikrobiol. 59, 158–164. doi: 10.1007/bf00406327
Johnson, K. A., and Johnson, D. E. (1995). Methane emissions from cattle. J. Anim. Sci. 73, 2483–2492. doi: 10.2527/1995.7382483x
Karuppiah, V., Collins, R. F., Thistlethwaite, A., Gao, Y., and Derrick, J. P. (2013). Structure and assembly of an inner membrane platform for initiation of type IV pilus biogenesis. Proc. Natl. Acad. Sci. U.S.A. 110, E4638–E4647. doi: 10.1073/pnas.1312313110
Koch, R., Swiger, L., Chambers, D., and Gregory, K. (1963). Efficiency of feed use in beef cattle. J. Anim. Sci. 22, 486–494.
Kozakai, K., Nakamura, T., Kobayashi, Y., Tanigawa, T., Osaka, I., Kawamoto, S., et al. (2007). Effect of mechanical processing of corn silage on in vitro ruminal fermentation, and in situ bacterial colonization and dry matter degradation. Can. J. Anim. Sci. 87, 259–267. doi: 10.4141/a06-028
Li, M., Penner, G. B., Hernandez-Sanabria, E., Oba, M., and Guan, L. L. (2009). Effects of sampling location and time, and host animal on assessment of bacterial diversity and fermentation parameters in the bovine rumen. J. Appl. Microbiol. 107, 1924–1934. doi: 10.1111/j.1365-2672.2009.04376.x
Lima, J., Auffret, M. D., Stewart, R. D., Dewhurst, R. J., Duthie, C.-A., Snelling, T. J., et al. (2019). Identification of rumen microbial genes involved in pathways linked to appetite, growth, and feed conversion efficiency in cattle. Front. Genet. 10:701. doi: 10.3389/fgene.2019.00701
Lin, R., Liu, W., Piao, M., and Zhu, H. (2017). A review of the relationship between the gut microbiota and amino acid metabolism. Amino Acids 49, 2083–2090. doi: 10.1007/s00726-017-2493-3
Macfarlane, S., and Dillon, J. F. (2007). Microbial biofilms in the human gastrointestinal tract. J. Appl. Microbiol. 102, 1187–1196. doi: 10.1111/j.1365-2672.2007.03287.x
Malmuthuge, N., Griebel, P. J., and Guan, L. L. (2015). The gut microbiome and its potential role in the development and function of newborn calf gastrointestinal tract. Front. Vet. Sci. 2:36. doi: 10.3389/fvets.2015.00036
Mann, E., Wetzels, S. U., Wagner, M., Zebeli, Q., and Schmitz-Esser, S. (2018). Metatranscriptome sequencing reveals insights into the gene expression and functional potential of rumen wall bacteria. Front. Microbiol. 9:43. doi: 10.3389/fmicb.2018.00043
Mao, S., Zhang, M., Liu, J., and Zhu, W. (2015). Characterising the bacterial microbiota across the gastrointestinal tracts of dairy cattle: membership and potential function. Sci. Rep. 5:16116. doi: 10.1038/srep16116
Mercadante, M. E. Z., de Melo Caliman, A. P., Canesin, R. C., Bonhila, S. F. M., Berndt, A., Frigheto, R. T. S., et al. (2015). Relationship between residual feed intake and enteric methane emission in Nellore cattle. Rev. Bras. Zootec. 44, 255–262. doi: 10.1590/s1806-92902015000700004
Myer, P. R., Smith, T. P. L., Wells, J. E., Kuehn, L. A., and Freetly, H. C. (2015). Rumen microbiome from steers differing in feed efficiency. PLoS One 10:e0129174. doi: 10.1371/journal.pone.0129174
Nagaraja, T. G., Galyean, M. L., and Cole, N. A. (1998). Nutrition and disease. Vet. Clin. North Am. Food Anim. Pract. 14, 257–277.
Newbold, C. J., de la Fuente, G., Belanche, A., Ramos-Morales, E., and McEwan, N. R. (2015). The role of ciliate protozoa in the rumen. Front. Microbiol. 6:1313. doi: 10.3389/fmicb.2015.01313
Oliveros, J. C. (2007). Venny. An Interactive Tool for Comparing Lists with Venn’s Diagrams. Available online at: https://bioinfogp.cnb.csic.es/tools/venny/index.html (accessed July, 2019).
Pacheco, A. R., Curtis, M. M., Ritchie, J. M., Munera, D., Waldor, M. K., Moreira, C. G., et al. (2012). Fucose sensing regulates bacterial intestinal colonization. Nature 492, 113–117. doi: 10.1038/nature11623
Park, T., and Yu, Z. (2018). Do ruminal ciliates select their preys and prokaryotic symbionts? Front. Microbiol. 9:1710. doi: 10.3389/fmicb.2018.01710
Pickard, J. M., and Chervonsky, A. V. (2015). Intestinal fucose as a mediator of host-microbe symbiosis. J. Immunol. 194, 5588–5593. doi: 10.4049/jimmunol.1500395
Pitta, D. W., Pinchak, W. E., Indugu, N., Vecchiarelli, B., Sinha, R., Fulford, J. D., et al. (2016). Metagenomic analysis of the rumen microbiome of steers with wheat-induced frothy bloat. Front. Microbiol. 7:689. doi: 10.3389/fmicb.2016.00689
Qin, J., Li, R., Raes, J., Arumugam, M., Burgdorf, K. S., Manichanh, C., et al. (2010). A human gut microbial gene catalogue established by metagenomic sequencing. Nature 464, 59–65. doi: 10.1038/nature08821
Quintana-Hayashi, M. P., Padra, M., Padra, J. T., Benktander, J., and Lindén, S. K. (2018). Mucus-pathogen interactions in the gastrointestinal tract of farmed animals. Microorganisms 6:E55. doi: 10.3390/microorganisms6020055
Ramsey, R., Doye, D., Ward, C., and McGrann, J. (2005). Factors affecting beef cow-herd costs, production, and profits. J. Agric. Appl. Econ. 37, 91–99. doi: 10.1017/s1074070800007124
Ribet, D., and Cossart, P. (2015). How bacterial pathogens colonize their hosts and invade deeper tissues. Microbes Infect. 17, 173–183. doi: 10.1016/j.micinf.2015.01.004
Roehe, R., Dewhurst, R. J., Duthie, C.-A., Rooke, J. A., McKain, N., Ross, D. W., et al. (2016). Bovine host genetic variation influences rumen microbial methane production with best selection criterion for low methane emitting and efficiently feed converting hosts based on metagenomic gene abundance. PLoS Genet. 12:e1005846. doi: 10.1371/journal.pgen.1005846
Rooke, J. A., Wallace, R. J., Duthie, C.-A., McKain, N., Motta de Souza, S., Hyslop, J. J., et al. (2014). Hydrogen and methane emissions from beef cattle and their rumen microbial community vary with diet, time after feeding and genotype. Br. J. Nutr. 112, 398–407. doi: 10.1017/S0007114514000932
Ross, E. M., Moate, P. J., Marett, L. C., Cocks, B. G., and Hayes, B. J. (2013). Metagenomic predictions: from microbiome to complex health and environmental phenotypes in humans and cattle. PLoS One 8:e73056. doi: 10.1371/journal.pone.0073056
Russell, J. B. (1998). The importance of pH in the regulation of ruminal acetate to propionate ratio and methane production in vitro. J. Dairy Sci. 81, 3222–3230. doi: 10.3168/jds.s0022-0302(98)75886-2
Savietto, D., Berry, D. P., and Friggens, N. C. (2014). Towards an improved estimation of the biological components of residual feed intake in growing cattle. J. Anim. Sci. 92, 467–476. doi: 10.2527/jas.2013-6894
Seshadri, R., Leahy, S. C., Attwood, G. T., Teh, K. H., Lambie, S. C., Cookson, A. L., et al. (2018). Cultivation and sequencing of rumen microbiome members from the Hungate1000 collection. Nat. Biotechnol. 36, 359–367. doi: 10.1038/nbt.4110
Severi, E., Hood, D. W., and Thomas, G. H. (2007). Sialic acid utilization by bacterial pathogens. Microbiology 153, 2817–2822. doi: 10.1099/mic.0.2007/009480-0
Shabat, S. K., Sasson, G., Doron-Faigenboim, A., Durman, T., Yaacoby, S., Berg Miller, M. E., et al. (2016). Specific microbiome-dependent mechanisms underlie the energy harvest efficiency of ruminants. ISME J. 10, 2958–2972. doi: 10.1038/ismej.2016.62
Sicard, J.-F., Le Bihan, G., Vogeleer, P., Jacques, M., and Harel, J. (2017). Interactions of intestinal bacteria with components of the intestinal mucus. Front. Cell. Infect. Microbiol. 7:387. doi: 10.3389/fcimb.2017.00387
Smibert, R. M. (1984). “Genus III treponema,” in Bergey’s Manual of Systematic Bacteriology, Vol. 1, eds N. R. Krieg and J. G. Holt (Baltimore, MD: Williams and Wilkins), 49–57.
Snelling, T. J., Auffret, M. D., Duthie, C.-A., Stewart, R. D., Watson, M., Dewhurst, R. J., et al. (2019). Temporal stability of the rumen microbiota in beef cattle, and response to diet and supplements. Anim. Microbiome 1:16.
Song, Y., Malmuthuge, N., Steele, M. A., and Guan, L. L. (2018). Shift of hindgut microbiota and microbial short chain fatty acids profiles in dairy calves from birth to pre-weaning. FEMS Microbiol. Ecol. 94:fix179. doi: 10.1093/femsec/fix179
Stanton, T. B., and Canale-Parola, E. (1980). Treponema bryantii sp. nov., a rumen spirochete that interacts with cellulolytic bacteria. Arch. Microbiol. 127, 145–156. doi: 10.1007/bf00428018
Steele, M. A., Penner, G. B., Chaucheyras-Durand, F., and Guan, L. L. (2016). Development and physiology of the rumen and the lower gut: targets for improving gut health. J. Dairy Sci. 99, 4955–4966. doi: 10.3168/jds.2015-10351
Stewart, R. D., Auffret, M. D., Warr, A., Wiser, A. H., Press, M. O., Langford, K. W., et al. (2018). Assembly of 913 microbial genomes from metagenomic sequencing of the cow rumen. Nat. Commun. 9:870. doi: 10.1038/s41467-018-03317-6
Thomas, S., Barry Holland, I., and Schmitt, L. (2014). The type 1 secretion pathway — the hemolysin system and beyond. Biochim. Biophys. Acta Mol. Cell Res. 1843, 1629–1641. doi: 10.1016/j.bbamcr.2013.09.017
Van Gylswyk, N. O. (1995). Succiniclasticum ruminis gen. nov. sp. nov., a rumen bacterium converting succinate to propionate as sole energy yielding mechanism. Int. J. Syst. Bacteriol. 45, 297–300. doi: 10.1099/00207713-45-2-297
Vannucci, F. A., and Gebhart, C. J. (2014). Recent advances in understanding the pathogenesis of Lawsonia intracellularis infections. Vet. Pathol. 51, 465–477. doi: 10.1177/0300985813520249
Wallace, R. J., Rooke, J. A., Duthie, C.-A., Hyslop, J. J., Ross, D. W., McKain, N., et al. (2014). Archaeal abundance in postmortem ruminal digesta may help predict methane emissions from beef cattle. Sci. Rep. 4:5892.
Wallace, R. J., Rooke, J. A., McKain, N., Duthie, C.-A., Hyslop, J. J., Ross, D. W., et al. (2015). The rumen microbial metagenome associated with high methane production in cattle. BMC Genomics 16:839. doi: 10.1186/s12864-015-2032-0
Wells, J. E., Krause, D. O., Callaway, T. R., and Russell, J. B. (1997). A bacteriocin-mediated antagonism by ruminal lactobacilli against Streptococcus bovis. FEMS Microbiol. Ecol. 22, 237–243. doi: 10.1111/j.1574-6941.1997.tb00376.x
Wetzels, S. U., Mann, E., Pourazad, P., Qumar, M., Pinior, B., Metzler-Zebeli, B. U., et al. (2017). Epimural bacterial community structure in the rumen of Holstein cows with different responses to a long-term subacute ruminal acidosis diet challenge. J. Dairy Sci. 100, 1829–1844. doi: 10.3168/jds.2016-11620
Wold, S. (1995). “PLS for multivariate linear modelling,” in Chemometric Methods in Molecular Design, ed. H. Van de Waterbeemd (Weinheim: VCH Publishers), 195–218.
Wood, D. E., and Salzberg, S. L. (2014). Kraken: ultrafast metagenomic sequence classification using exact alignments. Genome Biol. Evol. 15:R46.
Xie, X., Yang, C., Guan, L. L., Wang, J., Xue, M., and Liu, J. X. (2018). Persistence of cellulolytic bacteria Fibrobacter and Treponema after short-term corn stover-based dietary intervention reveals the potential to improve rumen fibrolytic function. Front. Microbiol. 9:1363. doi: 10.3389/fmicb.2018.01363
Yu, Z. T., and Morrison, M. (2004). Improved extraction of PCR-quality community DNA from digesta and fecal samples. Biotechniques 36, 808–812. doi: 10.2144/04365st04
Yun, Y., Kim, H. N., Kim, S. E., Heo, S. G., Chang, Y., Ryu, S., et al. (2017). Comparative analysis of gut microbiota associated with body mass index in a large Korean cohort. BMC Microbiol. 17:151. doi: 10.1186/s12866-017-1052-0
Zhan, K., Gong, X., Chen, Y., Jiang, M., Yang, T., and Zhao, G. (2019). Short-chain fatty acids regulate the immune responses via G protein-coupled receptor 41 in bovine rumen epithelial cells. Front. Immunol. 10:2042. doi: 10.3389/fimmu.2019.02042
Keywords: rumen microbiome, predicted microbial mechanisms, feed conversion efficiency, high concentrate diet, metagenomic sequencing
Citation: Auffret MD, Stewart RD, Dewhurst RJ, Duthie C-A, Watson M and Roehe R (2020) Identification of Microbial Genetic Capacities and Potential Mechanisms Within the Rumen Microbiome Explaining Differences in Beef Cattle Feed Efficiency. Front. Microbiol. 11:1229. doi: 10.3389/fmicb.2020.01229
Received: 17 January 2020; Accepted: 14 May 2020;
Published: 05 June 2020.
Edited by:
Alejandro Belanche, Experimental Station of Zaidín (EEZ), SpainReviewed by:
Sinead M. Waters, Teagasc Food Research Centre, IrelandRenee Maxine Petri, University of Veterinary Medicine Vienna, Austria
Copyright © 2020 Auffret, Stewart, Dewhurst, Duthie, Watson and Roehe. This is an open-access article distributed under the terms of the Creative Commons Attribution License (CC BY). The use, distribution or reproduction in other forums is permitted, provided the original author(s) and the copyright owner(s) are credited and that the original publication in this journal is cited, in accordance with accepted academic practice. No use, distribution or reproduction is permitted which does not comply with these terms.
*Correspondence: Marc D. Auffret, TWFyYy5BdWZmcmV0QHNydWMuYWMudWs=; bWFyY2F1ZmZyZXRAYW9sLmNvbQ==; Rainer Roehe, UmljaGFyZC5EZXdodXJzdEBzcnVjLmFjLnVr; UmFpbmVyLlJvZWhlQHNydWMuYWMudWs=
†Present address: Marc D. Auffret, Danone Nutricia Research, Innovation, Science & Nutrition Department, Gut & Microbiology Laboratories, Palaiseau Cedex, France