- 1Department of Plant Pathology, College of Plant Science and Technology and the Key Lab of Crop Disease Monitoring and Safety Control in Hubei Province, Huazhong Agricultural University, Wuhan, China
- 2Department of Agricultural Botany, Faculty of Agriculture, Al-Azhar University, Cairo, Egypt
- 3State Key Laboratory of Agricultural Microbiology, College of Life Science and Technology, Huazhong Agricultural University, Wuhan, China
Antimicrobial peptides (AMPs) are effective against different plant pathogens and newly considered as part of plant defense systems. From prokaryotes to eukaryotes, AMPs can exist in all forms of life. SM-985 is a cationic AMP (CAMP) isolated from the cDNA library of Mexican teosinte (Zea mays ssp. mexicana). A computational prediction server running with different algorithms was used to screen the teosinte cDNA library for AMPs, and the SM-985 peptide was predicted as an AMP with high probability prediction values. SM-985 is an arginine-rich peptide and composed of 21 amino acids (MW: 2671.06 Da). The physicochemical properties of SM-985 are very promising as an AMP, including the net charge (+8), hydrophobicity ratio of 23%, Boman index of 5.19 kcal/mol, and isoelectric point of 12.95. The SM-985 peptide has amphipathic α-helix conformations. The antimicrobial activity of SM-985 was confirmed against six bacterial plant pathogens, and the MIC of SM-985 against Gram-positive indicators was 8 μM, while the MIC of SM-985 against Gram-negative indicators was 4 μM. The SM-985 interacting with the bacterial membrane and this interaction were examined by treatment of the bacterial indicators with FITC-SM-985 peptide, which showed a high binding affinity of SM-985 to the bacterial membrane (whether Gram-positive or Gram-negative). Scanning electron microscopy (SEM) and transmission electron microscopy (TEM) images of the treated bacteria with SM-985 demonstrated cell membrane damage and cell lysis. In vivo antimicrobial activity was examined, and SM-985 prevented leaf spot disease infection caused by Pst DC3000 on Solanum lycopersicum. Moreover, SM-985 showed sensitivity to calcium chloride salt, which is a common feature of CAMPs.
Introduction
Antimicrobial peptides (AMPs), defined as small-molecular-weight proteins, are regarded as one of the substitutes for traditional antibiotics since they are small molecules and often have a wide range of antimicrobial activity, and AMPs have always been acknowledged for their low relative cytotoxicity. The innate immune system of nearly all organisms produces AMPs, including bacteria (Hassan et al., 2012), animals (Hancock and Scott, 2000), and plants (Alland et al., 2005). AMPs represent the first line of defense against plant bacterial pathogens (Ebbensgaard et al., 2015). AMPs can be isolated from almost all plant parts (Nawrot et al., 2014). Teosinte includes a group of four annual Zea species (Galinat, 1969). Mexican teosinte Zea mays ssp. mexicana and maize are members of the same family and share the present accepted ancestor (Doebley, 1992). George Beadle was the first to suggest that teosinte is the wild ancestor of maize, and a few main genes chosen by the people of Mexico over the last 10,000 years may have domesticated teosinte to maize (Beadle, 1939, 1972). Breeding programs and germplasm classification reports have shown that, in general, cultivated plants have comparatively lower rates of resistance to abiotic and biotic stresses compared with their wild ancestors (Rosenthal and Dirzo, 1997). cDNA is a complementary DNA copy of mRNA that is generated by the enzyme reverse transcriptase. cDNA library construction is a powerful tool for determining cell- and tissue-specific gene expression. cDNA is prepared from mRNA, and it has no inverting sequences such as introns. Consequently, the DNA reflects both expressible RNA and gene products (proteins) (Ying, 2004). The large scale of cDNA library members, which can be thousands, makes the screening for AMPs quite challenging. However, in recent years, many studies have used the cDNA library method to clone AMPs (Ronèeviæ et al., 2018; Wu et al., 2019; Tavares et al., 2020) due to its advantages such as enhanced fragments of efficiently transcribed genes and cloned AMPs from cDNA library, which can be actively manufactured via bacterial expression systems (Sharma et al., 2014) due to the lack of introns that might pose a problem when the target is manufactured as a eukaryotic protein in bacteria. In our lab, we screened cDNA libraries for AMPs via expression systems (Kong et al., 2018; Wu et al., 2020), but this is also a time- and cost-consuming approach. In silico prediction is a time- and cost-effective approach for large-scale screening and detection of AMPs (Liu et al., 2017). The detection of AMPs from databases has drawn interest as a field of structural genomics and bioinformatics. Many techniques have been used to classify AMPs from databases, including local alignments, regular expression (REGEX), activity prediction by machine learning algorithms, as well as three-dimensional (3D) structure predictions (Porto et al., 2017). Well-constructed AMP databases provide good foundations for AMP prediction. Several prediction approaches have been suggested in recent decades (Porto et al., 2012), using several algorithms (Liu et al., 2017) centered on several parameters. For example, CAMPR3 is developing predictive methods for AMPs that rely on machine learning algorithms such as the random forest (RF), discriminant analysis (DA), and support vector machines (SVMs) (Thomas et al., 2010). APD3 offers useful information on the peptide discovery timeline, description, terminology, bibliography, statistics, and calculation methods. The APD allows the successful scanning, configuration, and prediction of AMPs (Zhou and Huang, 2015). The DBAASP developed a new simple algorithm of prediction based on the physicochemical characteristics in charge of the tendency of the peptide to interact with the anionic bacterial membrane, including hydrophobicity, amphiphilicity, and net charge (Vishnepolsky and Pirtskhalava, 2014). AMPs with a large amount of positively charged amino acids such as arginine have high net charge values and are called cationic AMPs (CAMPs). CAMPs have strong antimicrobial activity against bacterial pathogens, slow resistance formation, and fast action (Ciumac et al., 2019). The α-helical AMPs are potent agents in mediating plant defense due to the antibacterial effect (Montesinos, 2007; Keymanesh et al., 2009). Their primary mechanism is to destroy the outer and plasma pathogen membranes, prevent membrane invasion, or pore formation, leading to cell lysis (Holaskova et al., 2014). In addition, other antimicrobial mechanisms have been described to affect essential cellular processes involving DNA and protein synthesis, cell wall synthesis, protein folding, and enzymatic activity (Nicolas, 2009). Bacterial plant diseases are responsible for major losses of crops and agricultural goods, and their protection relies primarily on chemical pesticides (Agrios, 2004). Some of these bacterial diseases are bacterial wilt of tomato, bacterial canker of tomato, bacterial blight of rice, bacterial leaf streak, and leaf spot disease of tomato, and these diseases are caused by Ralstonia solanacearum (Murthy et al., 2019), Clavibacter michiganensis ssp. michiganensis (Tancos et al., 2013), Xanthomonas oryzae pv. oryzae (Sharma et al., 2017), Xanthomonas campestris pv. holcicola (Navi et al., 2002), and Pseudomonas syringae pv. tomato DC3000 (Xin and He, 2013), respectively. Several pesticides have been banned due to their negative influence on the environment. However, because of the lack of active compounds, certain plant diseases of economic significance have faced management difficulties.
This study aimed to isolate novel AMPs with effective antimicrobial activity against some plant bacterial pathogens (Gram-positive and Gram-negative) from the Mexican teosinte (Z. mays ssp. mexicana) and to investigate the mechanism underlying this antimicrobial activity.
Materials and Methods
cDNA Library Construction
Zea mays ssp. mexicana seeds were grown in peat moss pots in a growth chamber at 28°C and under 14-h light/10-h dark conditions. After 21 days of growth, teosinte leaves were inoculated with PDA disks of Rhizoctonia solani AG1-lA (maize leaf blight pathogen) that were 48 h old. The sampling was performed by collecting leaves samples at 10 different time points: 0, 6, 12, 24, 36, 48, 60, 72, 86, and 96 h after inoculation. The leaf samples were directly frozen in liquid nitrogen and stored in a -80°C freezer. cDNA library construction of teosinte (Z. mays ssp. mexicana) was performed according to general procedures: total RNA extraction, mRNA purification, cDNA synthesis, incorporation of cDNA into pBE-S vector, transformation into Escherichia coli HST08, and transformation into Bacillus subtilis SCK6 super competent cells. The complete steps of constructing the teosinte cDNA library are mentioned in the Supplementary Data, and adaptors used for construction of the cDNA library are mentioned in Supplementary Table S1. The total RNA, mRNA, and cDNA were visualized in a gel (Supplementary Figure S1). Random colonies were chosen from the cDNA library, and colony PCR was conducted using vector pBE-S general primers (Supplementary Table S2). The colony PCR protocol was started at 95°C for 5 min, followed by 28 cycles were performed (95°C for 30 s, 56°C for 30 s, 72°C for 55 s), and then 72°C for 10 min. After the PCR protocol was finished, the quality of the teosinte cDNA library was checked by gel electrophoresis.
cDNA Sequencing and Analysis
Colony PCR was performed for all recombinant colonies using pBE-S primers, and the colony PCR protocol was started at 95°C for 5 min, followed by 30 cycles were performed (95°C for 30 s, 56°C for 30 s, 72°C for 55 s), and then 72°C for 10 min. The band sizes were determined by gel electrophoresis. The PCR products were sent for sequencing (repeated three times) using the Sanger method (Sander et al., 1976) to determine the cDNA sequences, while the empty vector bands were eliminated. The Basic Local Alignment Search Tool (BLAST) was used at NCBI1, MM GDB2, and Maize GDB3 to confirm the sources of these cDNA sequences and their relationship to genus Z. mays. cDNA sequences were translated from nucleic acids to amino acid sequences using a translation server4 grounded on the codon sequence of each amino acid according to the NCBI codes (Osawa et al., 1992; Jukes and Osawa, 1993).
Bioinformatics Analysis
In silico Prediction-Based Screening for AMPs
To screen the teosinte cDNA library, computational prediction tools were used. The selected amino acid sequences were uploaded in the FASTA format into AMP prediction servers. For general AMP prediction, different prediction servers were used, including CAMPR35 (Waghu et al., 2016), APD36 (Wang, 2015), AMPA with threshold value 0.2257 (Torrent et al., 2012), DBAASP8 (Vishnepolsky and Pirtskhalava, 2014), and MLAMP9 (Lin and Xu, 2016). Specific AMP prediction servers have been used to narrow the spectrum of antimicrobial activities of the expected AMPs depending on the type of organism (anti-fungal, antibacterial or antiviral)10 (Joseph et al., 2012), iAMPpred11 (Meher et al., 2017), Antibp12 (Lata et al., 2007), and dbAMP13 (Jhong et al., 2019).
Peptide Characterization
The physicochemical properties of SM-985 peptide were predicted using two servers, APD3 (Wang, 2015) for the amino acid composition, hydrophobic ratio, Boman index, and molecular weight. Moreover, DBAASP (Vishnepolsky and Pirtskhalava, 2014) was used for the isoelectric point, net charge, and in vitro aggregation. The secondary structure of SM-985 was predicted using three different servers, PSIPRED14 (Buchan and Jones, 2019), I-TASSER15 (Roy et al., 2010), and PEPstrMOD16 (Singh et al., 2015). BLAST determined the similarity of SM-985 to other AMPs in five different AMPs databases: CAMPR3 (Waghu et al., 2016), APD3 (Wang, 2015), DRAMP (Fan et al., 2016), MLAMP (Lin and Xu, 2016), and dbAMP (Jhong et al., 2019).
3D Structure Prediction
The 3D structure of SM-985 was predicted by I-TASSER (Roy et al., 2010) and visualized using UCSF Chimera 1.14rc software. The 3D structure of SM-985 was validated by MolProbity (Williams et al., 2018) and ProSA-web (Wiederstein and Sippl, 2007). Conversely, the helical wheel diagram was designed by HeliQuest (Gautier et al., 2008).
Microbial Indicator Strains, Culture Media, and Bacterial Growth Conditions
In this study, the antimicrobial activity of SM-985 was screened against eight different bacterial indicators, such as the Gram-positive bacteria Clavibacter fangii, C. michiganensis ssp. michiganesis, and B. subtilis 168. Moreover, we used the Gram-negative bacteria P. syringae pv. tomato DC3000, R. solanacearum, X. campestris pv. holcicola, X. oryzae pv. oryzae, and E. coli BL21. Luria–Bertani (LB) medium was used to grow the genus Xanthomonas, King’s B (KB) medium was used to grow the genus Pseudomonas, and Nutrient agar (NA) medium was used to grow the remaining bacterial stains. Non-pathogenic bacterial indicators (B. subtilis 168 and E. coli BL21) were incubated at 37°C, while pathogenic bacterial indicators were incubated at 28°C.
SM-985 Peptide
SM-985 peptide was synthesized according to the Fmoc Solid Phase Peptide Synthesis (Fmoc SPPS) method at 97% purity, and peptide synthesis was performed by Genscript (United States) Co. Ltd. The company provided all the information on peptide characterization, including mass spectrometry and HPLC data.
Minimal Inhibition Concentration (MIC) and Minimal Bactericidal Concentration (MBC) Assays
The minimal inhibition concentration (MIC) and minimal bactericidal concentration (MBC) SM-985 peptide were determined against eight bacterial indicators, and these assays were carried out using the agar and broth dilution method (Wiegand et al., 2008) with some alterations. For each bacterial indicator, a single colony was gown in Mueller Hinton Broth (MHB) at 28 and 37°C for pathogenic and non-pathogenic bacterial indicators, respectively. The bacteria culture was diluted with MHB to reach a concentration of ∼1 × 106 colony-forming units (CFU)/ml. A stock of 256 μM SM-985 in MHB was prepared, and in a microtiter plate, six serial dilutions of SM-985 peptide were prepared in the microtiter wells: 128, 64, 32, 16, 8, and 4 μM. Each well containing the peptide solution and the growth control well (without SM-985) were inoculated with the bacterial suspension, and the final concentration of the bacterial cells was ∼1 × 105 CFU/ml. A sterile control was made without either bacterial or SM-985. The microtiter plate was incubated for 8 h at 28 and 37°C for pathogenic and non-pathogenic bacterial indicators, respectively. Serial dilutions were generated for the different SM-985 concentrations, growth control, and sterile control. The suitable dilution was plated on medium plates and incubated under the same conditions until colonies grew in the growth control. The MIC value was determined as the lowest SM-985 concentration that caused 80% inhibition of the growth control (Wu et al., 2014). The MBC value was calculated as the minimum SM-985 concentration that caused no bacterial growth (Kang et al., 2011).
Minimal Lethal Concentration (MLC) Assay
Bacterial suspensions (∼1 × 106CFU/ml) were prepared using 10 mM phosphate buffer for each bacterial indicator (Van De Velde et al., 2010); the detailed steps of preparing the bacterial suspensions are mentioned in the Supplementary Methods. The bacterial suspension was treated with different final concentrations of SM-985 peptide, 128, 64, 32, 16, 8, 4, and 2 μM, in microtubes and labeled as treatments, while a microtube of the bacterial suspension was treated with dd water and labeled as a control. Both the treatments and control were incubated for 4 h (with gentle inversion each 1 h) at 28 and 37°C for pathogenic and non-pathogenic bacterial indicators, respectively. After incubation, serial dilutions were performed for both treatments and control. In medium plates in triplicate for both treatments and control, 100 μl of the suitable dilution (30–300 CFU per plate) was plated out, and then the plates were incubated until visible colonies grew at 28 and 37°C for pathogenic and non-pathogenic bacterial indicators, respectively. The MLC was determined as the minimum SM-985 concentration that caused no bacterial growth in the treated plates. This experiment was performed three times independently.
Cell Membrane Integrity Assay
Damage to the cytoplasmic membrane was assayed by propidium iodide (PI) uptake according to Van De Velde et al. (2010). For each bacterial indicator, a bacterial suspension ∼1 × 107 CFU/ml was prepared using 10 mM phosphate buffer (pH 7.0). The bacterial suspension was treated with 10 μM SM-985 and labeled as the treatment. A microtube with a similar volume of the bacterial suspension was treated with dd water and labeled as the control. Both the treatment and control were incubated for 4 h (with gentle inversion for each 1 h) at 28 and 37°C for pathogenic and non-pathogenic bacterial indicators, respectively. After incubation, PI dye was added to both the treatment and control microtubes at 10 μg/ml and fixed under dark conditions for 15 min. The bacterial cells were washed out with a 10 mM phosphate buffer (pH 7.0) two times by centrifugation at 5000 r/min to remove PI residues, and then resuspended in the buffer. An Olympus BX61 laser scanning confocal microscope (Wang et al., 2017) and flow cytometry Cytoflex lx (Beckman Coulter, Brea, CA, United States) (Kwon et al., 2019) were used to visualize and measure the PI uptake, respectively. The wavelengths of the excitation and emission were 535 and 617 nm, respectively. The flow cytometer data were analyzed using CyExpert 2.4 software.
FITC-Labeled-SM-985 Peptide
FITC-labeled-SM-985 peptide was synthesized by Genscript (United States) Co. Ltd. The FITC-labeled SM-985 peptide was dissolved as described for the SM-985 peptide, considering the change in molecular weight, and used to evaluate the interaction between SM-985 and the cytoplasmic membrane of each bacterial indicator (Zhu et al., 2015a). Similar cell membrane integrity assay procedures were preformed, but 4 μM FITC-SM-985 was used instead of SM-985. After incubation with FITC-SM-985, the bacterial cells were washed with 10 mM phosphate buffer (pH 7.0) to remove the peptide residues and resuspended in the buffer. An Olympus BX61 laser scanning confocal microscope was used to visualize FITC fluorescence at wavelengths of 488 and 500–530 nm, respectively. A cell killing assay was carried out on C. michiganesis ssp. michiganesis and P. syringae pv. tomato DC3000 to investigate the influence of the FITC tag on SM-985 antimicrobial activity. Both bacterial indicator suspensions (∼1 × 106 CFU/ml) were treated with 5 μM FITC-SM-985 for 4 h, while the control was treated with dd water. After incubation, serial dilutions were carried out for both the treatment and control. In medium plates in triplicate for both treatments and control, 100 μl of the suitable dilution (30–300 CFU per plate) was plated out, and then the plates were all incubated at 28°C until visible colony growth. Moreover, a cell membrane integrity assay was performed to verify the antimicrobial activity of 10 μM FITC-SM-985 peptide against seven bacterial indicators. The Leica TCS SP5 confocal microscope was used to determine both FTIC and PI fluorescence.
In vivo Antimicrobial Activity Assay
This experiment was carried out by a virulent bacterial strain of P. syringae pv. tomato DC3000 and two host Nicotiana benthamiana and Solanum lycopersicum. N. benthamiana plants grown in a growth room at 24°C (Yang L. Y. et al., 2018) (14/10 h, light/dark) for 5 weeks, while S. lycopersicum plants were grown at 28°C (14/10 h, light/dark) for 6 weeks. A bacterial suspension (∼1 × 106 CFU/ml) of Pst DC3000 was prepared. The in vivo AMP was assayed on both hosts in two ways. The first was performed on both hosts. SM-985 peptide was added to Pst DC3000 at a final concentration 5 μM, while in the control tube, Pst DC3000 was treated with dd water. Both the treatment and control were incubated at 28°C for 4 h (with gentle inversion for each 1 h). After incubation, both N. benthamiana and S. lycopersicum plants were inoculated with Pst DC3000 (treatment/control) using the infiltration method (Van De Velde et al., 2010; Vandenbossche et al., 2013) on the abaxial surface. The hypersensitive reaction was determined on N. benthamiana after 2 days, while necrosis symptoms on S. lycopersicum were determined after 4 days. The second was only performed on S. lycopersicum, and the same steps were followed as described above; the incubation, however, was performed on the plant surface. Both the treatment and control were sprayed directly on both adaxial and abaxial surfaces of plant leaves. The leaf spot disease symptoms were determined 6 days later, and both experiments were performed in triplicate.
Scanning Electron Microscopy (SEM)
This experiment was performed on C. michiganesis ssp. michiganesis as a representative of other bacterial indicators. A bacterial suspension (∼1 × 107 CFU/ml) was prepared and treated with 15 μM SM-985, while the control was treated with dd water; both treatment and control were incubated at 28°C (4 h with gentle inversion for each 1 h). Bacterial cells (treatment/control) were prepared to scanning electron microscopy (SEM) according to Wu et al. (2014) with some modifications, and they were handled in the same way. After incubation, the bacterial cells were collected and then fixed with 2.5% (v/v) of glutaraldehyde solution at room temperature for 2 h; then the solution of fixation was removed, and the bacterial cells were washed twice with 10 mM phosphate buffer (pH 7.0). The bacterial cells were dehydrated with 30, 50, 70, 90, and 100% ethanol solutions, respectively, and the bacterial pellet was dried under air for 20 min followed by a freeze dryer for 24 h until it achieved a powder form. Bacterial cells were lyophilized and coated with gold and then observed under a HITACHI SU8010 scanning electron microscope.
Transmission Electron Microscopy (TEM)
The transmission electron microscopy (TEM) assay was performed on C. michiganesis ssp. michiganesis as a representative of other bacterial indicators. The same steps as described for SEM were followed. However, after the incubation period, the bacterial cells were obtained and set to 2.5% (v/v) of the glutaraldehyde solution. The samples were then sent to the HZAU center of TEM (Wuhan, China) for preparation, and the bacterial cells were observed under a HITACHI H-7650 transmission electron microscope.
Influence of Calcium Chloride on SM-985 Antimicrobial Activity Assay
This assay was performed according to Van De Velde et al. (2010), with some modifications, on two bacterial indicators, Gram-positive bacteria C. michiganesis ssp. michiganesis, and Gram-negative bacteria P. syringae pv. tomato DC3000. For both bacterial indicators, a bacterial suspension (∼1 × 106 CFU/ml) was prepared, and calcium chloride salt was added to each bacterial suspension at four different final concentrations: 0, 5, 10, and 20 mM. As a final concentration, 5 μM SM-985 was added to the bacterial suspensions with four calcium chloride concentrations, after which they were incubated at 28°C for 4 h. After incubation, serial dilutions were performed. In medium plates in triplicate for both the treatments and control, 100 μl of the suitable dilution to obtain 30–300 CFU per plate was plated out and incubated at 28°C until visible colonies grew. Finally, the influence of calcium chloride salt on SM-985 activity was measured by counting the CFU of each salt concentration. All these procedures in the whole experiment were performed in triplicate.
Results
Teosinte cDNA Library
The cDNA insertions of teosinte were cloned into B. subtilis SCK6, and the constructed cDNA library consisted of 2500 single colonies. Most of the randomly picked up colony band sizes were more than 500 bp (empty vector band size) due to cDNA insertions, which indicated the high quality of the cDNA library (Supplementary Figure S2). As a result of the colony PCR of the whole cDNA library, more than 2000 colonies had cDNA insertions with varied band sizes. The sequencing results revealed similarities among the 2000 insertions, and to avoid repetition, 500 colonies were eliminated. The cDNA insertions showed 100% similarity with genus Zea according to the BLAST results against NCBI, MM GDB, and Maize GDB.
Bioinformatics Analysis
In silico AMP Prediction
After the translation of the 2000 cDNA inserts to amino acid sequences, each amino acid sequence was named based on size (the amino acid sequences were grouped under three groups of small sequences of 0–20 aa, medium sequences of 21–50 aa, and large sequences of greater than 50 aa), and the serial number of each sequence ranged from 1 to 2000. The 2000 sequences were screened for AMPs by the CAMPR3 prediction server (SVM algorithm), and based on the screening results, 30 sequences showed prediction values greater than 0.5 (Supplementary Table S3). SM-985 showed the height prediction value among the 30 sequences; the number of sequences filtered in each step is summarized in Figure 1A. Moreover, AMPA detected a stretch of essential antimicrobial amino acids from amino acid number 4 to number 20 within the SM-985 sequence, and this stretch had a propensity scale of 0.149 with 0% probability of misclassification. Generally, all predictions of SM-985 were positive (Supplementary Table S4) and all predictions indicated antibacterial activity (Supplementary Table S5).
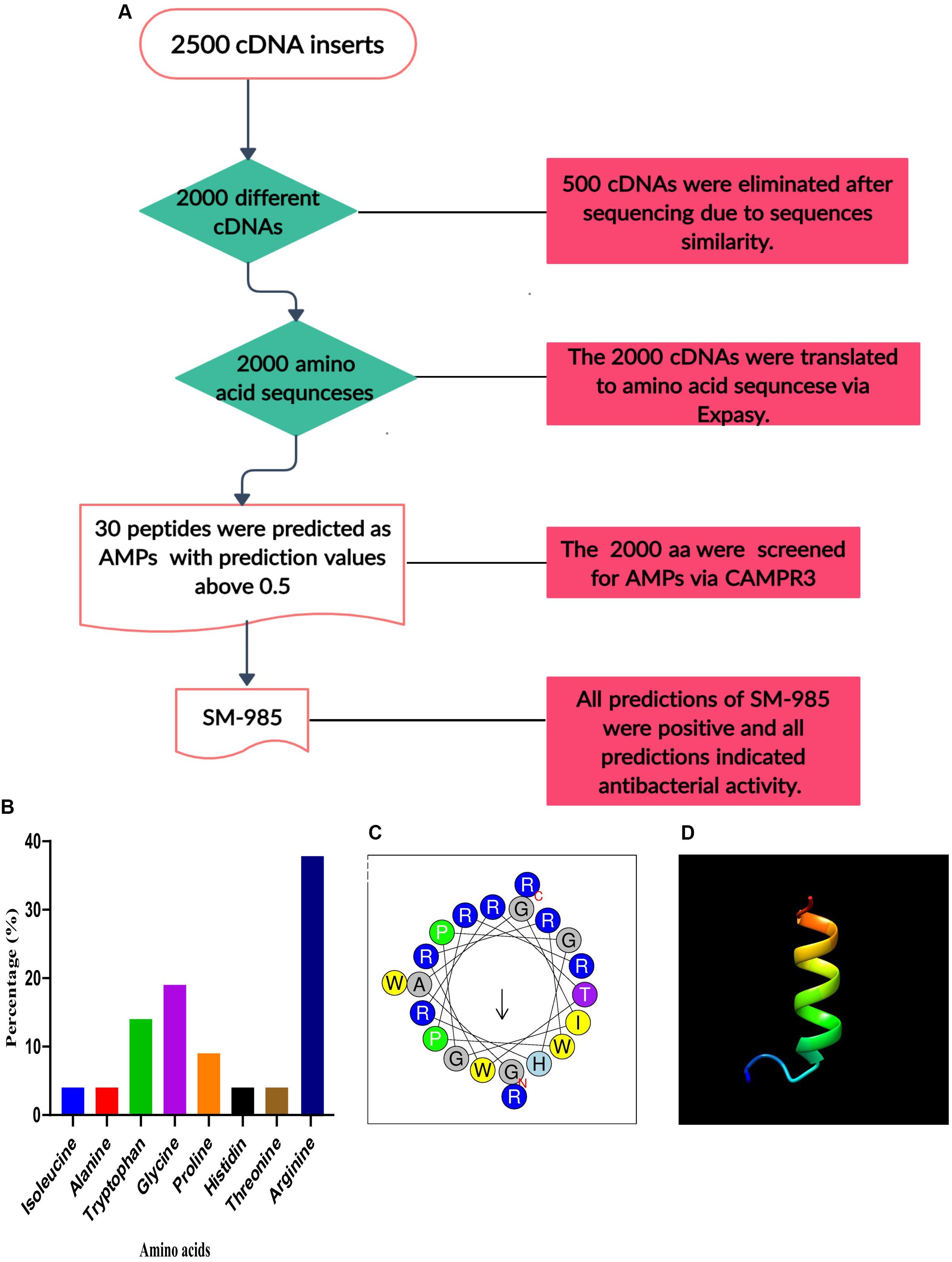
Figure 1. Bioinformatics analysis of SM-985. (A) Flow chart of peptide screening. (B) SM-985 composed of eight different amino acids, most with antimicrobial potentials, and rich in arginine (graph created with GraphPad Prism 8). (C) Helical wheel diagram of SM-985 peptide showing the amphipathic alpha-helix conformations. (D) The SM-985 predicted 3D structure contains an α-helix, as predicted by I-TASSER. The 3D structure model visualized using Chimera 1.14rc software. The 3D structure model of SM-985 was validated by ProSA-web software, the Z-score suggests that the peptide within a suitable area of structures. Regarding the MolProbity validation, the residues of SM-985 were within the favorable region.
Characteristics of SM-985 Peptide
Predicting SM-985 as AMP requires in-depth physicochemical sequence-based analysis. SM-985 is a small peptide with 21 amino acids; these 21 aa are composed of eight different amino acids as follows: arginine (R) 38%, glycine (G) 19%, tryptophan (T) 14%, proline (P) 9%, alanine (A) 4%, isoleucine (4%), histidine (4%), and threonine (4%). SM-985 is an arginine-rich peptide, according to APD3 (Figure 1B). The calculation of the physicochemical properties of SM-985 showed AMP properties due to the high net charge value (+8), high isoelectric point 12.95, hydrophobic ratio of 23%, protein-binding potential (Boman index) value of 5.19 kcal/mol, and in vitro aggregation value of zero. The secondary structure prediction by the servers PSIPRED, I-TASSER, and PEPstrMOD indicated that the SM-985 peptide contained an α-helix structure. The helical wheel diagram of SM-985 peptide had amphipathic α-helix conformations, in which the hydrophobic and the hydrophilic residues were placed on contradictory flanks of the α-helix (Figure 1C). The SM-985 peptide sequence showed no similarities to AMPs in the DRAMP, MLAMP, or dbAMP databases. Moreover, SM-985 had no significant similarities to either APD3 or CAMPR3. Thus, the BLAST results showed no similarity between the SM-985 peptide sequence and the other AMPs, confirming the novelty of the peptide.
The 3D Structure of SM-985
The α-helical 3D structure model was predicted by the I-TASSER server (Figure 1D). To prevent mistakes in selecting the correct peptide structural model, the ProSA-web and MolProbity methods can be used to refine and verify peptide models. The α-helical 3D structure of SM-985 was within the suitable quality range. The Z-score value obtained using the ProSA-web software, which suggests that the majority of data points in a multidimensional NMR range are not signal-occupied locations (Wiederstein and Sippl, 2007), showed that the peptides were within a suitable area of structures, indicating that they have characteristics of native structures (Würz and Güntert, 2017). Regarding the MolProbity validation, the residues of SM-985 were within the favorable region (right-handed α-helix), indicating high structural reliability. Conversely, the SM-985 peptide generated 94.7% of its residues in the favorable region and 5.3% in the permitted region.
Determination of MICs and MBCs of SM-985 Peptide
The MIC and MBC of SM-985 were investigated against all the bacterial indicators. The lowest SM-985 concentration causing 80% growth inhibition (MIC) of the Gram-positive bacteria C. fangii, C. michiganesis ssp. michiganesis, and B. subtilis 168 was 8 μM, while the lowest SM-985 concentration causing no bacterial growth (MBC) was 16 μM. The MIC values of SM-985 against the Gram-negative bacterial indicators X. campestris pv. holcicola, X. oryzae pv. oryzae, P. syringae pv. tomato DC3000, R. solanacearum, and E. coli BL21 were 4 μM, <4 μM, <4 μM, 4 μM, and 8 μM, respectively. The MBC values of SM-985 were 16 μM, 4 μM, 4 μM, 8 μM, and 16 μM, respectively (Table 1).
The MLC of SM-985 Peptide Causes Complete Death for All Bacterial Indicators
This experiment was undertaken to assess the lowest concentration of SM-985 peptide, which causes complete death for all bacterial indicators, Gram-positive bacteria (C. fangii, C. michiganesis ssp. michiganesis, and B. subtilis 168) and Gram-negative bacteria (X. campestris pv. holcicola, X. oryzae pv. oryzae, P. syringae pv. tomato DC3000, R. solanacearum, and E. coli BL21) at a certain bacterial concentration (∼1 × 106 CFU/ml). The bacteria plates treated with SM-985 (treatment plates) did not show any visible growth with SM-985 peptide at concentrations of 128, 64, 32, 16, 8, 4, and 2 μM. In contrast, the plates of the control showed growing colonies in all bacterial indicators. Thus, ≤2, considered the minimal SM-985 concentration, caused no visible colonies (complete death) against all bacterial indicators (Figure 2). The colonies in the plates of the control were counted for all bacterial indicators (Table 2).
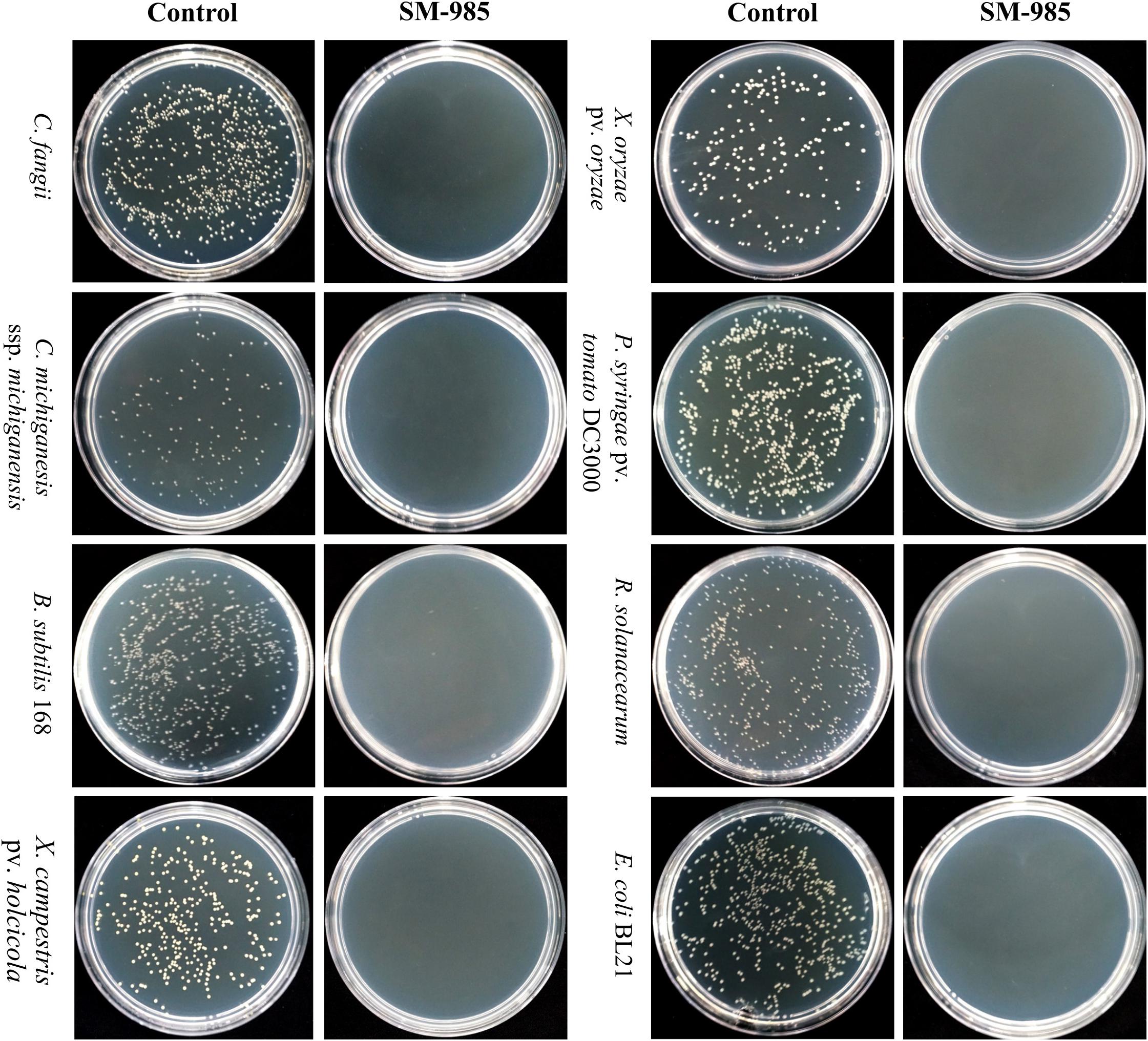
Figure 2. MLC of SM-985. No bacterial growth was noticed after all the bacterial indicators (Gram-negative and Gram-positive bacteria) were treated with SM-985 at ≤2 μM concentration for 4 h in phosphate buffer. However, the bacterial indicators grew well in the control treated with dd water.
SM-985 Increases Cell Membrane Permeability
PI dye uptake, which only can enter damaged cells, determines cell membrane damage. This experiment was performed on Gram-positive bacteria (C. fangii, C. michiganesis ssp. michiganesis, and B. subtilis 168) and Gram-negative bacteria (X. oryzae pv. oryzae, P. syringae pv. tomato DC3000, R. solanacearum, and E. coli BL21). An Olympus BX61 laser scanning confocal microscope was used to visualize PI uptake. Both cells treated with 10 μM SM-985 and controls were treated with PI dye. As a result, the bacterial cells treated with SM-985 were stained with PI and displayed red fluorescence of the PI dye, while the control bacterial cells showed no staining (Figure 3). The damage to the cell membrane was measured by Cytoflex lx (Beckman Coulter, Brea, CA, United States). There were two controls, the negative control representing bacterial cells without either SM-985 or PI dye, and the positive control representing bacterial cells without SM-985 and with PI dye. In this experiment, SM-985 disrupted the bacterial cell membrane, increasing the percentage of PI uptake more than both the negative and positive controls (Figure 4).
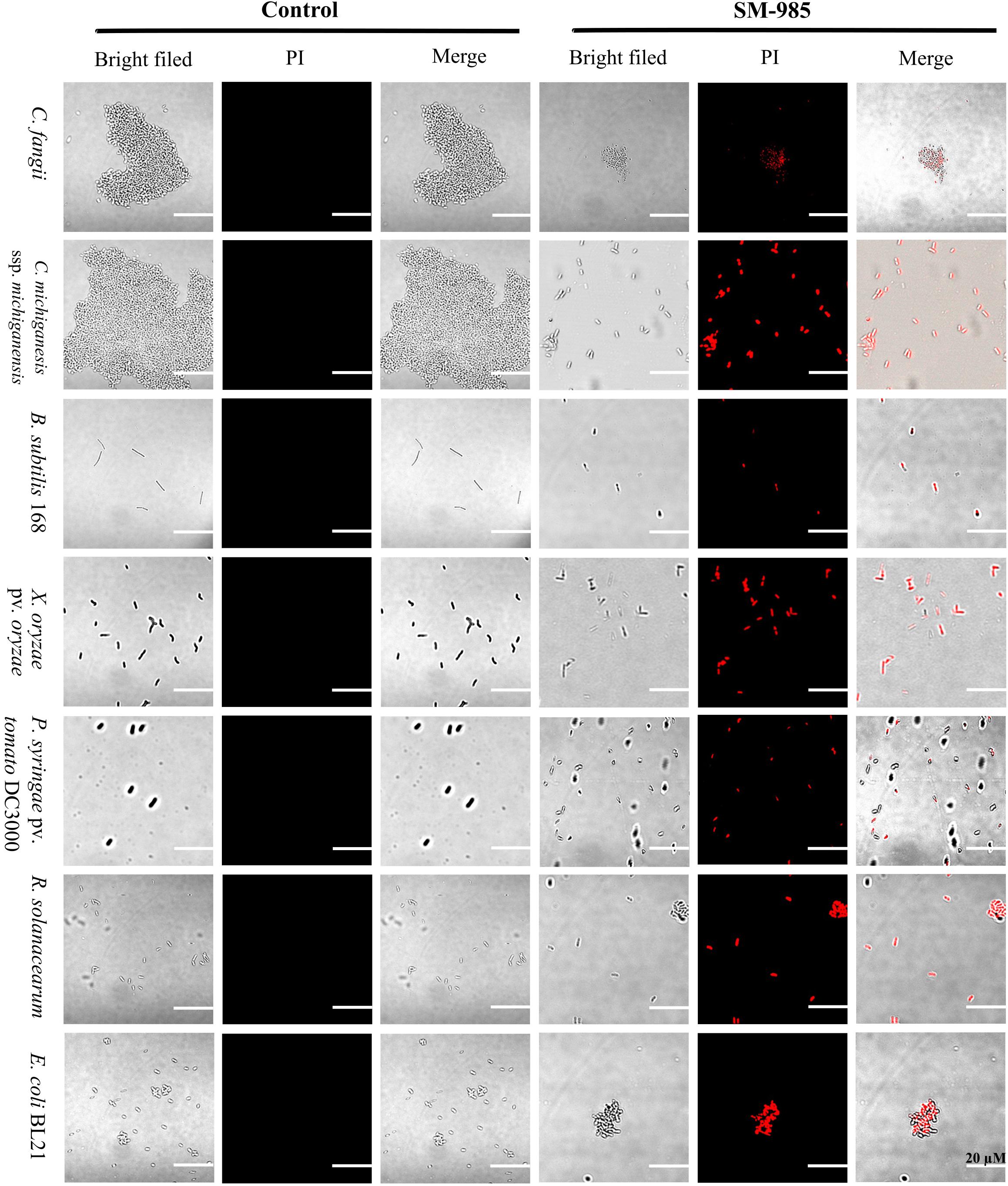
Figure 3. Visualizing the bacterial membrane permeability. The bacterial indicator, membranes (Gram-positive and Gram-negative) at a concentration of ∼1 × 107 CFU/ml, lost integrity after treatment with 10 μM SM-985 for 4 h. However, the control did not take up the PI stain. No fluorescence indicates invariable membrane integrity. The red fluorescence of the PI stain indicates membrane disintegration. An Olympus BX61 laser scanning confocal microscope was used to observe PI uptake. Scale bar: 20 μm.
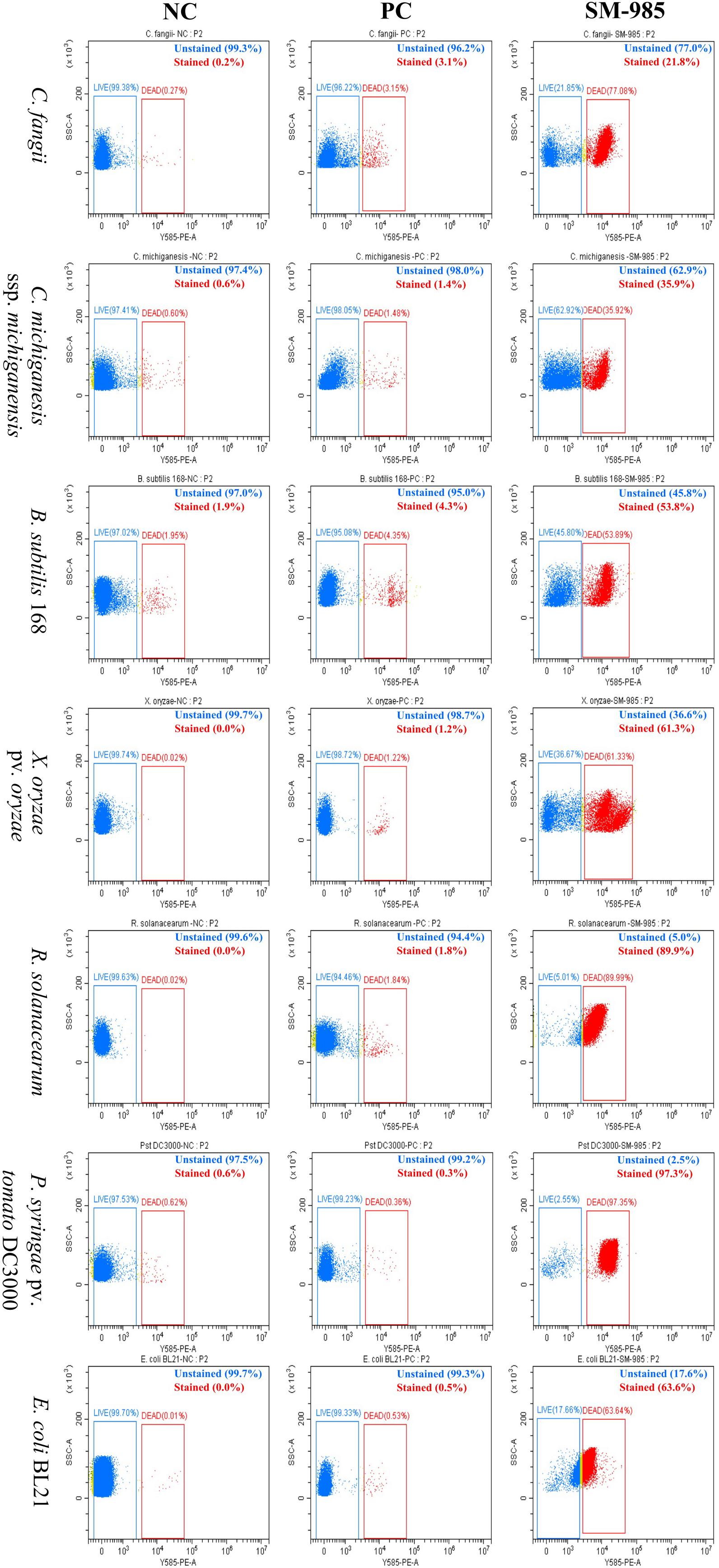
Figure 4. Quantitative analysis of bacterial membrane permeability. The PI uptake of the bacterial indicators (Gram-positive and Gram-negative) greatly increased after they were treated with 10 μM SM-985 for 4 h. The blue dots indicate unstained cells, while the red dots indicate stained cells. NC refers to the negative control and PC refers to the positive control. The Cytoflex lx machine was used to measure the PI uptake, and the data were analyzed with CyExpret 2.4 software.
FITC-Labeled SM-985 Peptide Interacts With the Bacterial Cell Membrane
FITC-labeled SM-985 peptide was used to explore the SM-985 working mechanism as AMPs, and the bacterial cells were treated with FITC-labeled peptide at a low concentration for 3 h. Both Gram-positive bacteria (C. fangii, C. michiganesis ssp. michiganesis, and B. subtilis 168) and Gram-negative bacteria (X. campestris pv. holcicola, X. oryzae pv. oryzae, P. syringae pv. tomato DC3000, R. solanacearum, and E. coli BL21) showed green fluorescence under an Olympus BX61 laser scanning confocal microscope, according to the suitable laser wavelength of FITC green fluorescence (Figure 5). These results showed that FITC-SM-985 peptide interfered with the bacterial cell membrane. Two antimicrobial activity assays were conducted to investigate the impact of the FITC tag on SM-985 antimicrobial activity. A cell killing assay was performed on C. michiganesis ssp. michiganesis and P. syringae pv. tomato DC3000 as representatives of other indicators. Both bacterial indicator suspensions (∼1 × 106 CFU/ml) were treated with 5 μM FITC-SM-985 peptide for 4 h. However, the plates of the treated bacteria with FITC-SM-985 (treatment plates) did not show any visible growth, while the control plates showed growing colonies in both bacterial indicators (Table 3). Moreover, cell membrane integrity assay was conducted using PI uptake. Gram-positive bacteria (C. fangii, C. michiganesis ssp. michiganesis, and B. subtilis 168) and Gram-negative bacteria (X. oryzae pv. oryzae, P. syringae pv. tomato DC3000, R. solanacearum, and E. coli BL21) were stained with PI after treating each bacterial suspension (∼1 × 107 CFU/ml) with 10 μM FITC-SM985 peptide. Under a confocal microscope, the bacterial cells showed both green fluorescence of FITC and red fluorescence of PI dye (Figure 6).
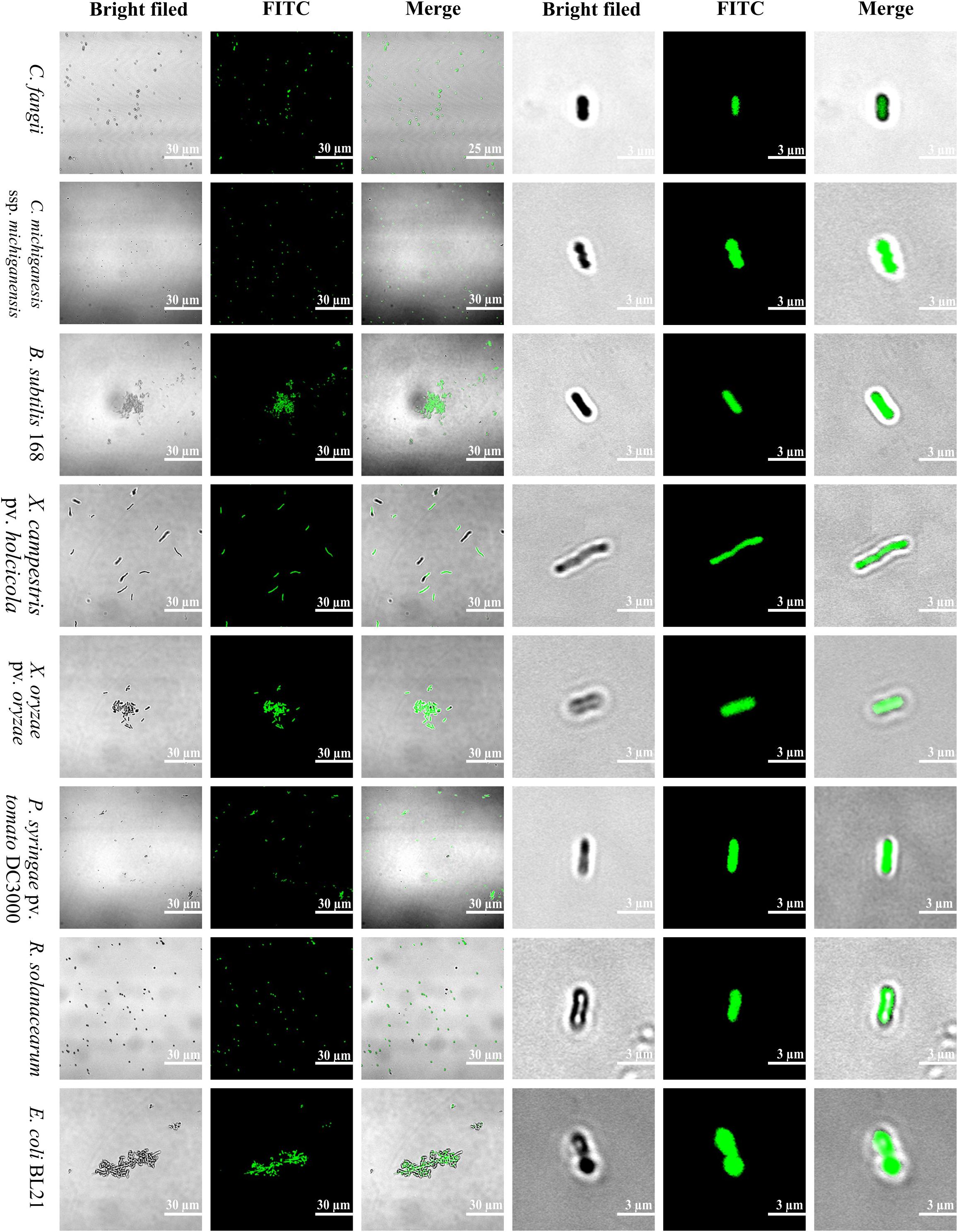
Figure 5. Localization of FITC-labeled SM-985. SM-985 showed a high affinity to bind to bacterial indicator (Gram-positive and Gram-negative) membranes after they were treated with 4 μM FITC-SM-985 for 4 h. The green fluorescence of the FITC tag indicates the interaction between SM-985 and bacterial membrane. The results were observed using an Olympus BX61 laser scanning confocal microscope. Scale bar: 30 and 3 μm.
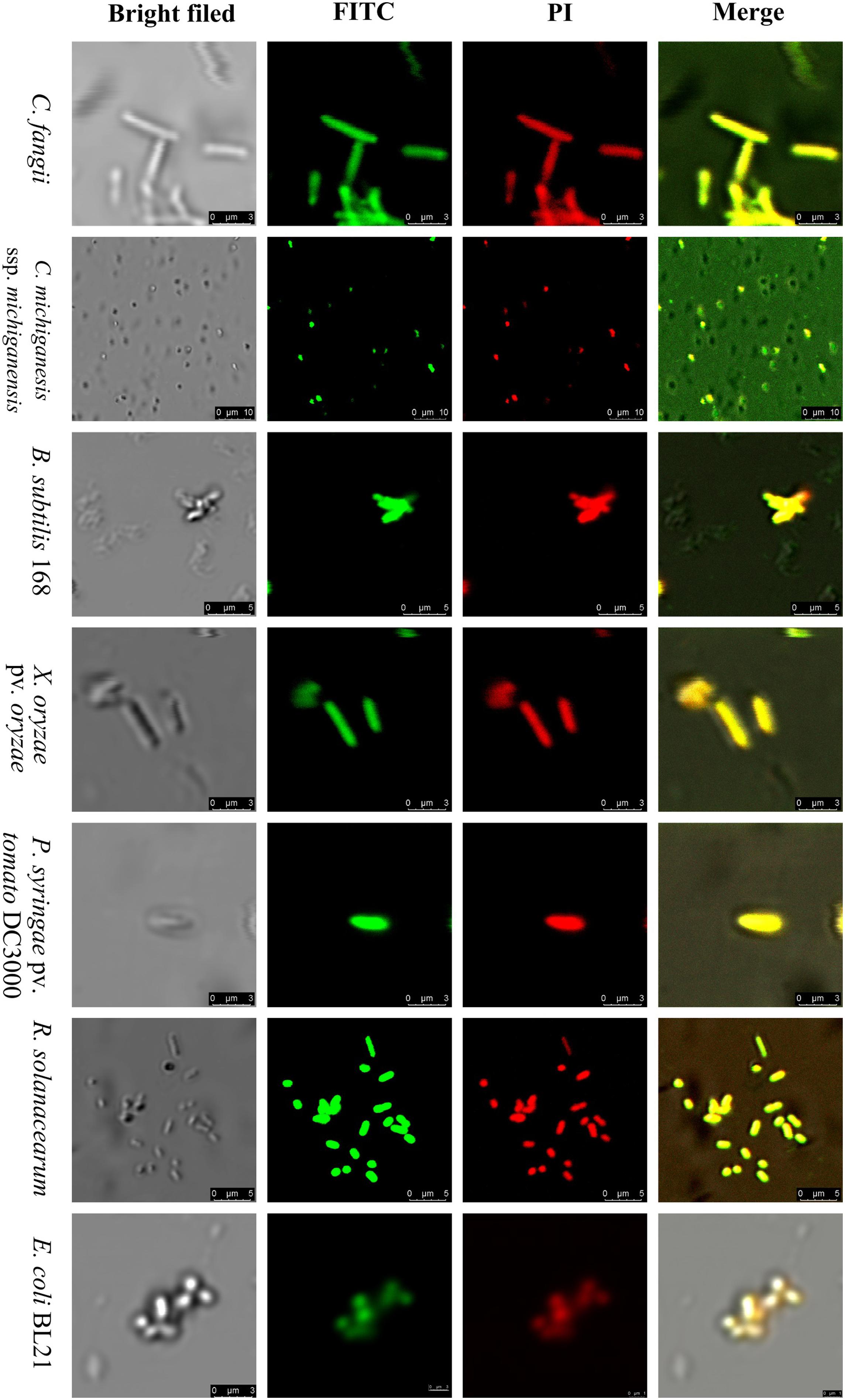
Figure 6. Bacterial membrane permeability by FITC-SM-985. The bacterial indicator membranes (Gram-positive and Gram-negative) lost their integrity after they were treated with 10 μM FITC-SM-985 for 4 h. The red fluorescence of the PI stain indicates membrane disintegration. The green fluorescence of the FITC tag indicates the interaction between SM-985 and bacterial membrane. The results were observed using a Leica TCS SP5 confocal microscope. Scale bar: 3, 5, and 10 μm.
In vivo Antimicrobial Activity of SM-985
Based on the ability of P. syringae pv. tomato DC3000 to cause leaf spot disease on S. lycopersicum and the hypersensitivity reaction (HR) on N. betnhamiana, the Pst DC3000 bacterial suspension (∼1 × 106 CFU/ml) was treated with 5 μM SM-985 for 4 h while the control was treated with dd water. Both hosts were inoculated with the treated and control bacterial cells using the infiltration technique. The treated Pst DC3000 bacterial cells could not induce disease symptoms on either S. lycopersicum or HR on N. betnhamiana. In contrast, the control Pst DC3000 bacterial cells induced disease symptoms on S. lycopersicum and HR on N. betnhamiana after 4 and 2 days, respectively (Figure 7). To simulate the real infection conditions of leaf spot disease, SM-985 was added to the Pst DC3000 bacterial suspension (∼1 × 106 CFU/ml) at a final concentration of 5 μM by direct spraying on the S. lycopersicum leaves. The Pst DC3000 treated with SM-985 did not show symptoms, while the control Pst DC3000 infected the S. lycopersicum leaves on both adaxial and abaxial surfaces and caused leaf spot symptoms (Figure 8).
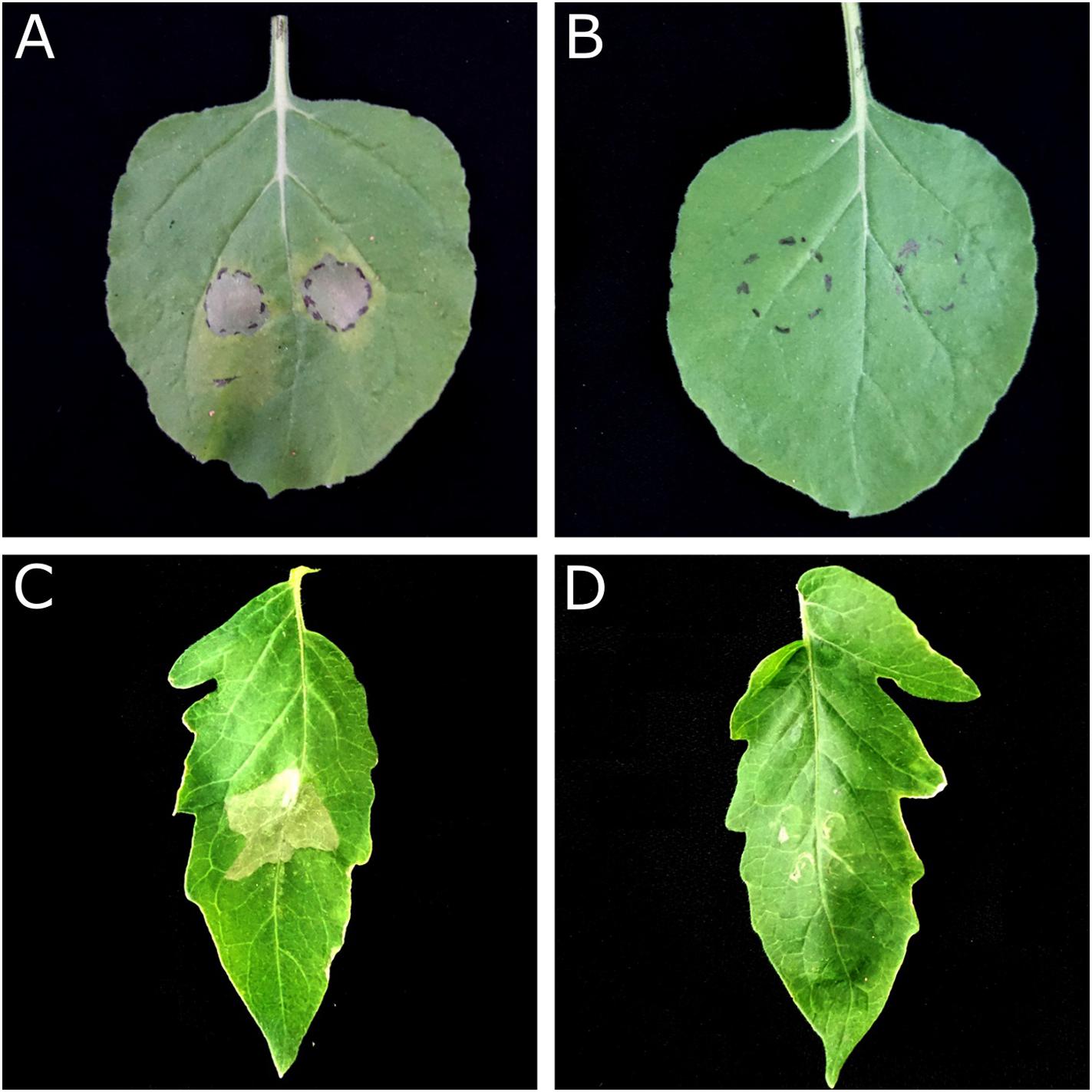
Figure 7. In vivo antimicrobial activity assay by the leaf infiltration method. Pst DC3000 at a concentration of ∼1 × 106 CFU/ml lost its ability to cause HR on N. benthamiana and necrosis on S. lycopersicum after treatment with 5 μM SM-985 for 4 h. (A) N. benthamiana treated with dd water (control). (B) N. benthamiana treated with SM-985. (C) S. lycopersicum treated with dd water (control). (D) S. lycopersicum treated with SM-985. The results were observed after 48 h for N. benthamiana and 96 h for S. lycopersicum.
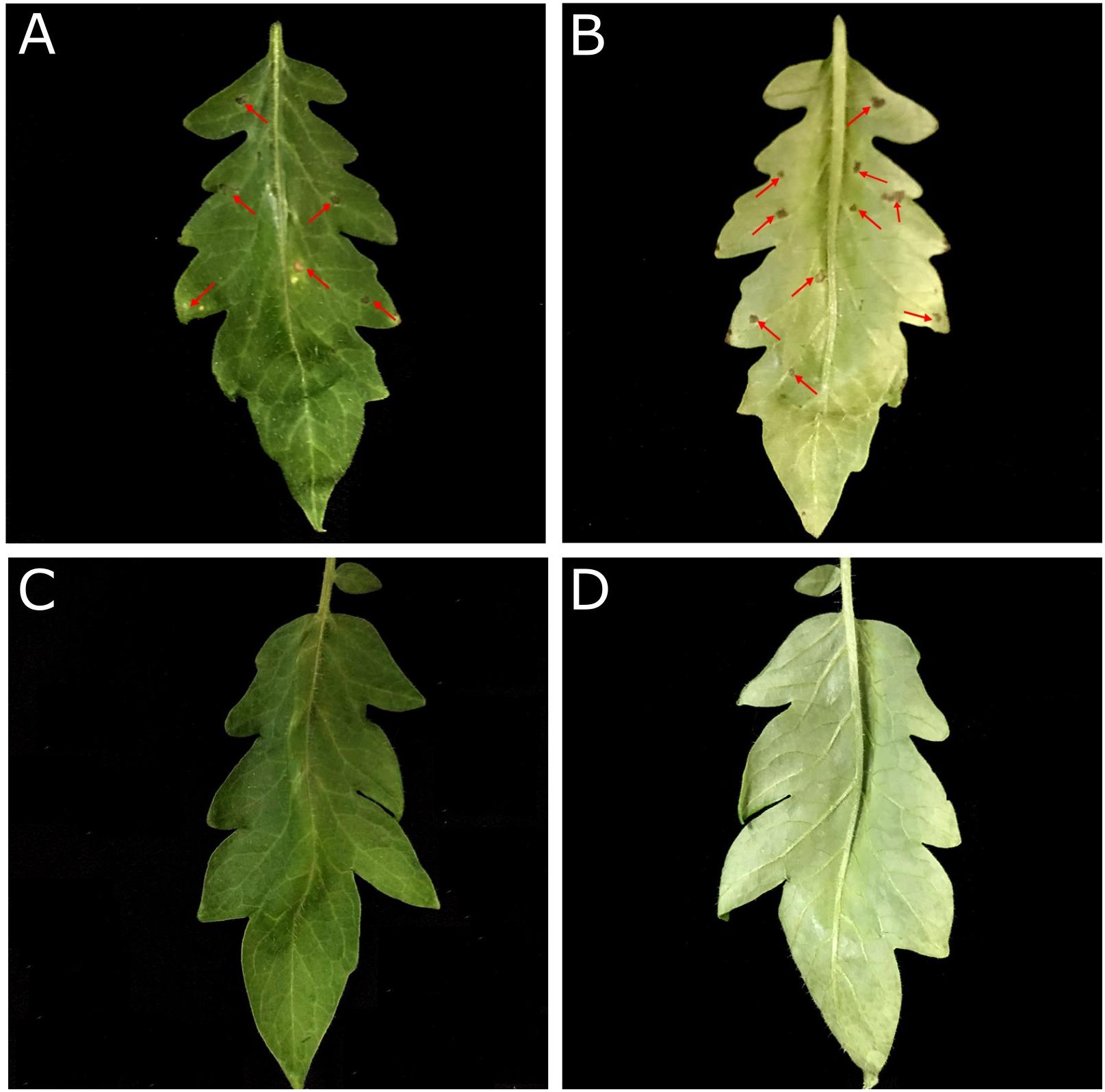
Figure 8. SM-985 prevented leaf spot infection on tomato. Pst DC3000 at a concentration of ∼1 × 106CFU/ml was treated with 5 μM SM-985, while the control was treated with dd water. Both the SM-985 treatment and control were directly sprayed on S. lycopersicum (adaxial and abaxial surfaces), and the results were observed after 6 days. (A) Control adaxial surface. (B) Control abaxial surface. (C) SM-985 adaxial surface. (D) SM-985 abaxial surface. The red arrows indicate leaf spots.
SM-985 Causes Distinct Damage to the Bacterial Cell Membrane
From previous results, it was determined that SM-985 damaged the cell membrane by increasing cell membrane permeability. SEM and TEM assays were performed on C. michiganesis ssp. michiganesis to observe the cell membrane damage after treating the bacteria cells with SM-985. The bacterial suspension (∼1 × 107 CFU/ml) was treated with 15 μM SM-985 for 4 h, while the control was treated with dd water. The SEM images showed the damaged and disrupted cell envelope of the treated C. michiganesis ssp. michiganesis. In contrast, the cell envelope of the control cells was intact and had a regular shape (Figure 9). Moreover, the TEM images revealed cell lysis, a damaged cell membrane, and the absence of cytoplasmic material in the treated C. michiganesis ssp. michiganesis. The cell membrane of the control cells was intact and smooth, and the cells were full of cytoplasmic material (Figure 9).
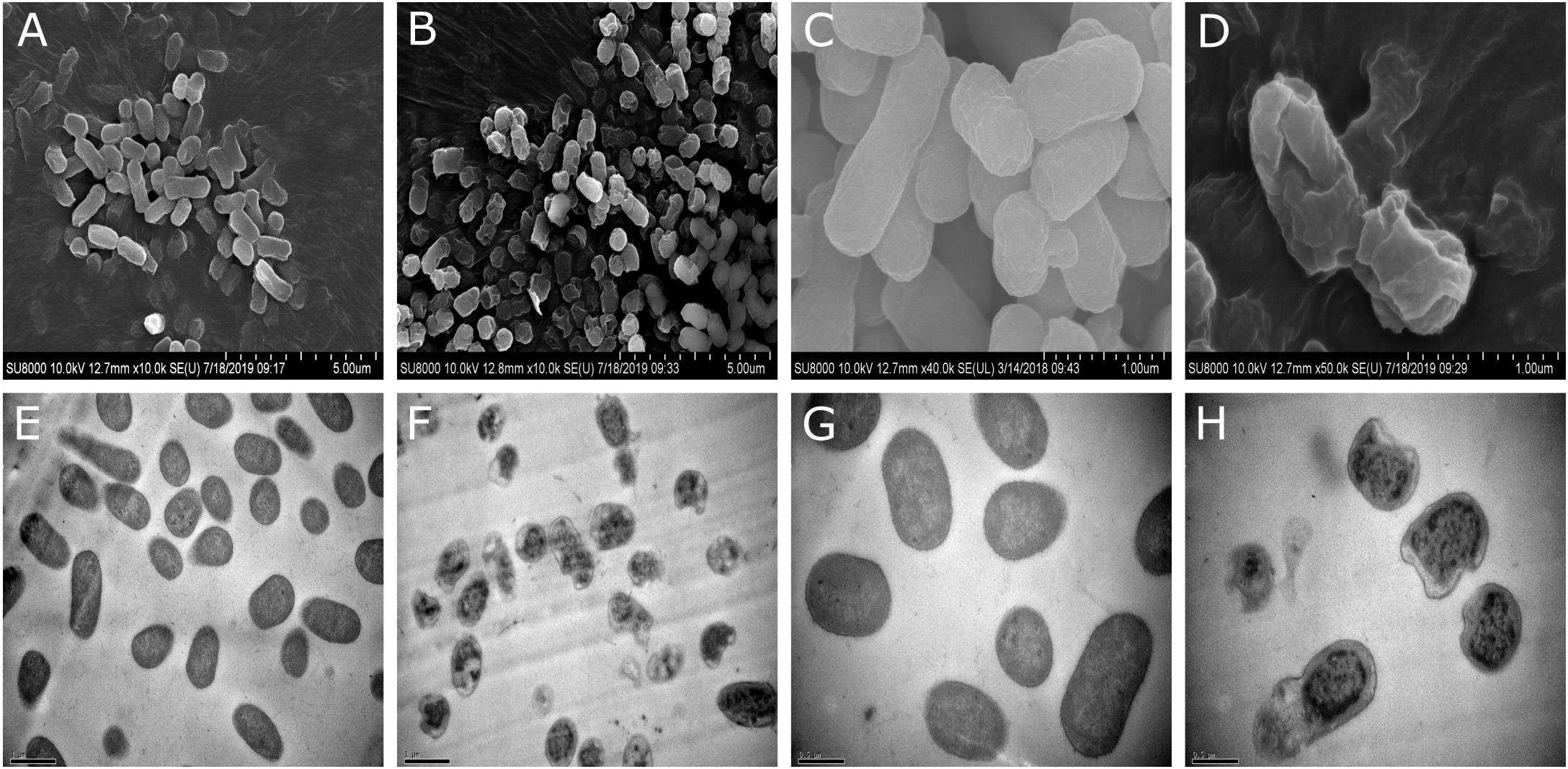
Figure 9. Cell membrane damage investigation by SEM and TEM. A bacterial suspension of ∼1 × 107 CFU/ml of C. michiganesis ssp. michiganesis was treated with 15 μM SM-9895 for 4 h. (A,C,E,G) The cytoplasmic membranes were intact, and the cells appeared normal in the control. (B,D,F,H) SM-985 caused damage to the cytoplasmic membrane and cell lysis. The results observed using a HITACHI SU8010 scanning electron microscope and HITACHI H-7650 transmission electron microscope.
Calcium Chloride Inhibits SM-985 Antimicrobial Activity
SM-985 peptide activity was affected by adding calcium chloride salt. The results of both the Gram-positive bacterial indicator C. michiganesis ssp. michiganesis and Gram-negative bacterial indicator P. syringae pv. tomato DC3000 showed that in 0 mM calcium chloride, SM-985 activity was very strong, with no visible colony growth. However, after addition of 5 mM calcium chloride, SM-985 activity was noticeably decreased. The salt concentration and SM-985 activity showed an inverse relationship. Thus, 10 mM calcium chloride caused more growing colonies than 5, and 20 mM caused more growing colonies than 10 mM. In summary, increasing the calcium chloride concentration resulted in more growing colonies and reduced the SM-985 antimicrobial activity (Figure 10).
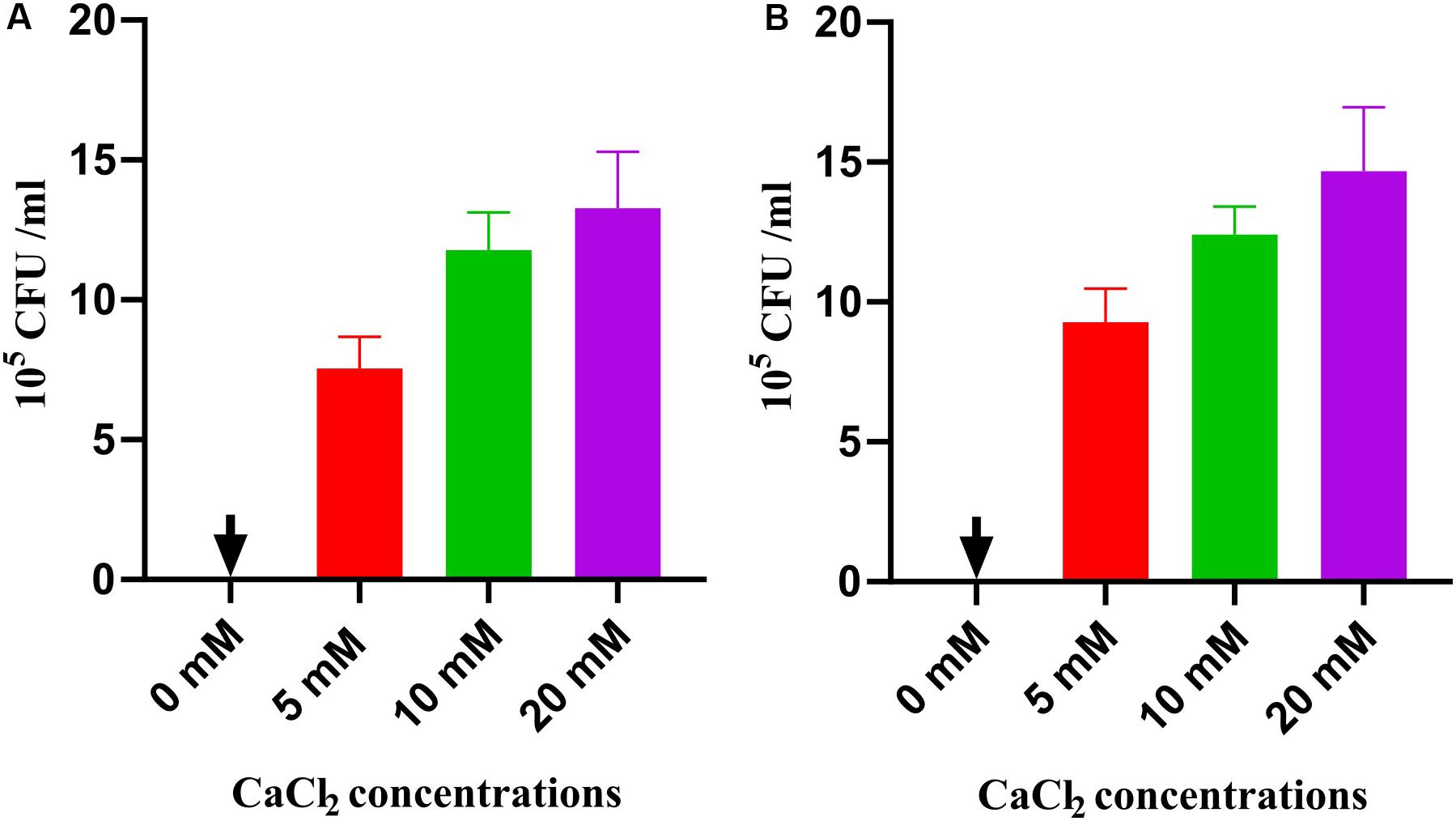
Figure 10. SM-985 sensitivity to CaCl2 salt. Bacterial suspension (CFU/ml) treated with 5 μM SM-985 for 4 h. CaCl2 salt was added at different concentrations. SM-985 antimicrobial activity was noticeably decreased after CaCl2 addition. The CaCl2 salt and SM-985 had an inverse relationship (A) C. michiganesis ssp. michiganesis. (B) Pst DC3000. Results with standard deviation are shown.
Discussion
High losses caused every year by plant pathogens in addition to the restriction of the use of pesticides in several countries around the world have urged the development of alternative approaches as new antimicrobial agents (Giralt, 2014). This study focused on isolating novel AMPs with strong antimicrobial activity against several bacterial plant pathogens. A wild plant species, Z. mays ssp. mexicana, was chosen as the source of the AMPs. Teosinte is the wild ancestor of maize (Doebley, 1992). Some genes, such as resistance genes, may be lost during the domestication of plant species (Chaudhary, 2013), or the expression of certain genes linked to the resistance of plant diseases may be expressed more in wild plants such as teosinte than domesticated plants such as maize (Dávila-Flores et al., 2013; Szczepaniec et al., 2013). Therefore, Z. mays ssp. mexicana is a good candidate source for the isolation of AMPs.
Toward this aim, a cDNA library of teosinte was constructed. After sequencing, it was confirmed that Z. mays ssp. mexicana was the source of the cloned insertions via BLAST analysis. Based on the cDNA sequences, a translation tool was used to convert them to amino acid sequences. In silico predictive tools may be beneficial for big-scale screening and evaluation of new AMPs. Therefore, five servers were used to screen the amino acid sequences for potent AMPs. These servers utilize several algorithms to predict new AMPs (Liu et al., 2017), and this prediction is based on different parameters. More than one server was used to increase the prediction accuracy. According to the prediction results, SM-985 peptide showed the highest prediction values among the other amino acid sequences. SM-985 is a short peptide consisting of 21 amino acids and is similar to some well-known AMPs with a size range between 10 and 50 amino acids (Hamamoto et al., 2002). The short and simple sequence of an AMP may simplify its rapid construction, cut costs of synthesis, and accelerate translational applications (Ong et al., 2014). SM-985 is composed of eight different amino acids: arginine (R) 38%, glycine (G) 19%, tryptophan (T) 14%, proline (P) 9%, alanine (A) 4%, isoleucine (4%), histidine (4%), and threonine (4%). As a result, the APD3 database considered SM-985 as arginine-rich peptide due to its high content of arginine. Some studies have demonstrated that arginine is the key structure of cell-penetrating peptides (CPPs) (Takeuchi and Futaki, 2016; Murayama et al., 2017). Previous studies have shown that arginine-rich peptides appear to be closer to the lipid bilayer in simulations, validating their increased ability to initiate probable translocation events compared with variations of other peptides (Takeuchi and Futaki, 2016). In addition to arginine, SM-985 is composed of other amino acids, also all of which have potential antimicrobial activity. Several studies have mentioned the antimicrobial potential of tryptophan (Courrol et al., 2019), glycine (Halder et al., 2019), and proline (Mardirossian et al., 2019). SM-985 is a CAMP due to the high content of arginine (Zhang and Gallo, 2016).
SM-985 was predicted as an α-helical peptide with an α-helix secondary structure, and the α-helical conformation has been directly linked to the antimicrobial activity (Park et al., 2000). In previous studies, many AMPs with an α-helix structure have been reported (Bonduelle, 2018; Shen et al., 2018; Triana-Vidal et al., 2018) due to the helical structure, which plays an essential role in AMP activity, whereas the α-helix secondary structure assumes an amphipathic structure and presents a precise hydrophobic portion for membrane penetration (Zelezetsky and Tossi, 2006). The 3D structural model of SM-985 was predicted using the I-TASSER server, and this model was validated with ProSA-web and MolProbity software. The ProSA-web results showed that the SM-985 3D model was within the favorable region of structures, and these results were comparable to a previous study (Liscano et al., 2019). The results of MolProbity indicated that the peptide structure parameters remained within the limits of acceptable quality and stability (Beg et al., 2018).
Due to the high levels of positive amino acids such as arginine, the net charge of SM-985 is (+8). Most CAMPs have such a positive net charge, ranging from +4 to +8, which are ideal for biotic operations (Tossi et al., 2000). CAMPs bind to cytoplasmic membrane phospholipids by forming a forceful electrostatic link (Yount et al., 2006). In a previous study, the net charge of V13K analogs was decreased to less than +4, which inactivated the peptide, while higher antimicrobial activity was observed when they increased the net charge from +4 to +8. However, a further rise in net charges to +9 and +10 increased the toxicity of the peptide (Jiang et al., 2008).
Hydrophobicity is another important feature of AMP activity. Creating pores in the cytoplasmic membrane of the bacterial cell requires hydrophobic residues that interact with the lipid bilayer (the hydrophobic portion). Ultimately, this interaction results in the degradation of the bacterial cytoplasmic membrane. Low hydrophobicity may be insufficient to induce an effective interaction between the AMPs and cytoplasmic membranes, which decreases antimicrobial activity. Nevertheless, the exceptionally high hydrophobicity of AMPs, which leads to their auto-association, makes them unable to pass through the cell wall of bacteria. The hydrophobic ratio of SM-985 is 23%, which represents moderate hydrophobicity; moreover, the average hydrophobicity is vital for optimal antimicrobial activity (Chen et al., 2007). The helical wheel of SM-985 indicated amphipathic α-helix conformations, and an earlier study has shown that the positive polar face supports phospholipid binding when the AMP is attached to the cell membrane. At that point, via hydrophobic interactions, the non-polar face of the AMP inserts into the membrane (He et al., 2018). The Boman index of SM-985 is 5.19 kcal/mol, and the high positive Boman index increases the ability of SM-985 to bind to bacterial cell membrane proteins (He et al., 2018). Radzicka and Wolfenden established an early hydrophobic scale focused on the partitioning of small-molecule side-chain amino acid analogs between water and cyclohexane. The Boman index is essentially the average hydrophobic value measured using the Radzicka–Wolfenden scale (Radzicka and Wolfenden, 1988).
According to the BLAST results, SM-985 showed no significant similarity with other AMPs from three large AMPs databases, which strongly indicates that it is a new plant AMP from teosinte. The prediction results and physiochemical properties of SM-985 make it a promising AMP candidate. In our studies, we also confirmed the in silico results experimentally.
A critical function of AMPs is the direct killing of microbial targets. In our research, MICs and MBCs of SM-985 have been measured against a wide range of bacterial indicators, including Gram-positive bacteria (C. fangii, C. michiganesis ssp. michiganesis, and B. subtilis 168) and Gram-negative bacterial indicators (X. campestris pv. holcicola, X. oryzae pv. oryzae, P. syringae pv. tomato DC3000, R. solanacearum, and E. coli BL21). Interestingly, the MICs and MBCs values of SM-985 were higher against Gram-positive bacterial indicators than Gram-negative bacterial indicators. The MICs of SM-985 against Gram-positive and most Gram-negative indicators were 8 and 4 μM, respectively. Thus, Gram-positive bacterial indicators are more tolerant to SM-985 than Gram-negative bacterial indicators because of the cell envelop structure. There are more layers of peptidoglycan surrounding Gram-positive membranes than Gram-negative membranes (Silhavy et al., 2010), which might explain the difference in MIC and MBC values. It is interesting to note that Guavanin 2 peptide (Porto et al., 2018) shares common features with SM-985, both of which are arginine-rich α-helical peptides with no similar sequences in AMPs databases, and have a preference for Gram-negative bacteria. Moreover, a previous study examined NCR335 antimicrobial activity against two bacterial indicators. The MIC of CNR335 against Listeria monocytogenes (Gram-positive bacteria) was 32 μM, while the MIC of NCR335 against Salmonella enterica (Gram-negative bacteria) was 16 μM (Farkas et al., 2017). The next step in our research was to investigate the lowest concentration value of SM-985 that triggered direct killing of both Gram-positive and Gram-negative bacterial indicators using 10 mM phosphate buffer. The MLC of SM-985 was ≤2 μM, and we noticed that the MLC value was lower than the MIC and MBC values. Thus, SM-985 demonstrated lower antimicrobial efficacy with MIC and MBC agar dilution compared with the MLC determination process. The main reason for the observed difference was the nature of the place in which the antimicrobial activity was investigated. The agar dilution process uses MHB medium, which contains high divalent cation levels, whereas the 10 mM phosphate buffer used in the MLC system does not contain divalent cations. Divalent cation inhibition is considered to be a common feature of CAMPs (Farkas et al., 2017).
SM-985 increased the bacterial indicator membrane permeability, which was confirmed by PI uptake assay. Only dead bacterial cells with damaged cell membranes can take up PI dye, and both Gram-negative and Gram-positive indicators treated with SM985 were able to take up more PI dye than the control. Many studies have confirmed the cell membrane damage after treatment with AMPs by the PI uptake assay (Farkas et al., 2017; Velivelli et al., 2018; Yang M. et al., 2018). The positive net charge of SM-985 facilitates the interaction with the cell membrane phospholipid negatively charged groups (Fernandez et al., 2013). Therefore, SM-985 interacts with bacterial cell membranes to induce damage. As a result, the bacterial cell is able to take up the PI dye. The interaction between SM-985 and the bacterial cell membrane was confirmed by treating the bacterial indicators with FITC-SM-985, and as a result, SM-985 showed a strong affinity to the bacterial membrane (both Gram-positive and Gram-negative). These results are consistent with other studies on CAMPs (Farkas et al., 2014; Zhu et al., 2015b; Nagarajan et al., 2019). The effect of the FITC tag on SM-985 antimicrobial activity was investigated by performing antimicrobial activity assays. In the cell killing assay, FITC-SM-985 was able to kill Gram-positive C. michiganesis ssp. michiganesis and Gram-negative P. syringae pv. tomato DC3000. Moreover, in the PI uptake assay, PI dye uptake ability of all the bacterial indicators was increased after treating them with FITC-SM-985, and these results were agreement with a previous study (Van De Velde et al., 2010). From recent findings, even for the short amino acid sequence of SM-985, the FITC tag did not affect SM-985 antimicrobial activity.
Pst Dc3000 was treated with SM-985 at a concentration of 5 μM and then incubated for 4 h at 28°C. Then, N. betnhamiana and S. lycopersicum were inoculated with Pst DC3000 using the infiltration method. SM-985 prevented Pst DC3000 infection on N. betnhamiana and S. lycopersicum. The infection ability of Pst DC3000 was lost due to the incubation period of Pst DC3000 with SM-985. To simulate the real infection conditions, Pst DC3000 was treated with SM-985 at a concentration of 5 μM and then directly sprayed on S. lycopersicum without an incubation period. Pst DC3000 lost infection ability after it was treated with SM-985. It is known that leaf spot disease pathogens require a prolonged period (12–24 h) to initiate infection, and during this period, SM-985 interacts with the PsDC3000 membrane and damages it, leading to cell death. The in vivo antimicrobial activity of SM-985 opens the door for the application of SM-985 to protect plants against bacterial pathogens.
Scanning electron microscopy and TEM demonstrated the damaging effect of SM-985 on the bacterial membrane. The SEM images showed the damaged and disrupted cell envelope of the C. michiganesis ssp. michiganesis after it was treated with 15 μM SM-985 for 4 h. In contrast, the cell envelope of the control cells appeared intact with a regular shape. The TEM images demonstrated cell membrane lysis and the lack of cytoplasmic material in the treated C. michiganesis ssp. michiganesis. In contrast, the cell membrane of the control cells was intact and smooth, and the cells were full of cytoplasmic material. These results are comparable to many other studies used SEM and TEM to determine the damage present in the bacterial cell membrane after treatment with AMP (Wu et al., 2014; Zhang et al., 2018; Halder et al., 2019; Hou et al., 2019).
Calcium chloride impairs the antimicrobial activity of SM-985 on C. michiganesis ssp. michiganesis (Gram-positive bacteria) and P. syringae pv. tomato DC3000 (Gram-negative bacteria), and SM-985 shows sensitivity to different concentrations of calcium chloride. In fact, several studies have suggested a detrimental effect of divalent cations (Ca+2) on the antimicrobial activity of AMPs (Maisetta et al., 2008; Huang et al., 2011). The mechanism of SM-985 calcium chloride sensitively might be clarified as follows: the positive charge of calcium chloride disorganizes the interaction between the cationic SM-985 and the bacterial membrane (Zhu et al., 2015a). Other studies have indicated that salt sensitivity might affect AMP stability, which affects antimicrobial activity (Luo et al., 2018).
Conclusion
We screened a cDNA library from teosinte (Z. mays ssp. mexicana) for AMPs using in silico prediction tools, and SM-985 peptide was predicted as an AMP. SM-985 showed very promising physiochemical properties as an AMP with an arginine-rich α-helical structure. SM-985 showed wide spectrum antimicrobial activity against bacterial phytopathogens; Gram-positive and Gram-negative plant pathogenic bacteria were efficiently eliminated by cationic plant peptide SM-985. Thus, SM-985 was demonstrated to disrupt and damage the bacterial cell physical structure by increasing the membrane permeability through pore formation. In vivo antimicrobial activity was conducted, and SM-985 prevented leaf spot infection caused by Pst DC3000 on S. lycopersicum. In addition, SM-985 demonstrated sensitivity to calcium chloride acid, a typical characteristic of CAMPs.
Data Availability Statement
The raw data supporting the conclusions of this article will be made available by the authors, without undue reservation, to any qualified researcher.
Author Contributions
AQ and WD conceived research and designed the experiments. AQ conducted library construction and data analyses. AQ and FW performed gene screening and functional test experiments. AQ and WD wrote the manuscript with input from all co-authors.
Funding
This work was supported by the National Major Project for Transgenic Organism Breeding (2016ZX08003-001) and the Hubei Provincial Technology Innovation Program (2016ABA093).
Conflict of Interest
The authors declare that the research was conducted in the absence of any commercial or financial relationships that could be construed as a potential conflict of interest.
Acknowledgments
We would like to thank Drs. Guoying Wang and Yunjun Liu for comments and suggestions on the experimental design and data analysis.
Supplementary Material
The Supplementary Material for this article can be found online at: https://www.frontiersin.org/articles/10.3389/fmicb.2020.01353/full#supplementary-material
Footnotes
- ^ https://blast.ncbi.nlm.nih.gov/Blast.cgi
- ^ http://mmgdb.hzau.edu.cn/
- ^ https://www.maizegdb.org
- ^ https://web.expasy.org
- ^ http://www.camp.bicnirrh.res.in/
- ^ http://aps.unmc.edu/AP/main.php
- ^ http://tcoffee.crg.cat/apps/ampa/do:ampa
- ^ https://dbaasp.org/prediction
- ^ http://biotechlab.fudan.edu.cn/database/lamp/
- ^ http://www.bicnirrh.res.in/classamp/predict.php
- ^ http://cabgrid.res.in:8080/amppred/server.php
- ^ https://webs.iiitd.edu.in/raghava/antibp/index.html
- ^ http://csb.cse.yzu.edu.tw/dbAMP/
- ^ http://bioinf.cs.ucl.ac.uk/psipred/
- ^ https://zhanglab.ccmb.med.umich.edu/
- ^ http://osddlinux.osdd.net/raghava/pepstrmod/index.php
References
Agrios, G. (2004). Plant Pathology, 5th Edn. Cambridge, MA: Academic Press. doi: 10.1016/C2009-0-02037-6
Alland, C., Moreews, F., Boens, D., Carpentier, M., Chiusa, S., Lonquety, M., et al. (2005). RPBS?: a web resource for structural bioinformatics. Nucleic Acids Res. 33, 44–49. doi: 10.1093/nar/gki477
Beadle, G. (1939). Teosinte and the origin of maize. J. Hered. 30, 245–247. doi: 10.1093/oxfordjournals.jhered.a104728
Beg, M. A., Shivangi, Thakur, S. C., and Meena, L. S. (2018). Structural prediction and mutational analysis of Rv3906c gene of Mycobacterium tuberculosis H37Rv to determine its essentiality in survival. Adv. Bioinformatics 2018:6152014. doi: 10.1155/2018/6152014
Bonduelle, C. (2018). Secondary structures of synthetic polypeptide polymers. Polym. Chem. 9, 1517–1529. doi: 10.1039/c7py01725a
Buchan, D. W. A., and Jones, D. T. (2019). The PSIPRED protein analysis workbench: 20 years on. Nucleic Acids Res. 47, W402–W407. doi: 10.1093/nar/gkz297
Chaudhary, B. (2013). Plant domestication and resistance to herbivory. Int. J. Plant Genomics 2013:572784. doi: 10.1155/2013/572784
Chen, Y., Guarnieri, M. T., Vasil, A. I., Vasil, M. L., Mant, C. T., and Hodges, R. S. (2007). Role of peptide hydrophobicity in the mechanism of action of α-helical antimicrobial peptides. Antimicrob. Agents Chemother. 51, 1398–1406. doi: 10.1128/AAC.00925-06
Ciumac, D., Gong, H., Hu, X., and Lu, J. R. (2019). Membrane targeting cationic antimicrobial peptides. J. Colloid Interface Sci. 537, 163–185. doi: 10.1016/j.jcis.2018.10.103
Courrol, D. S. S., Lopes, C. R. B., Pereira, C. B. P., Franzolin, M. R., Silva, F. R., and Courrol, L. C. (2019). Tryptophan silver nanoparticles synthesized by photoreducti susceptible bacteria. Int. J. Tryptophan Res. 12:117864691983167. doi: 10.1177/1178646919831677
Dávila-Flores, A. M., DeWitt, T. J., and Bernal, J. S. (2013). Facilitated by nature and agriculture: performance of a specialist herbivore improves with host-plant life history evolution, domestication, and breeding. Oecologia 173, 1425–1437. doi: 10.1007/s00442-013-2728-2
Doebley, J. (1992). Mapping the genes that made maize. Trends Genet. 8, 302–307. doi: 10.1016/0168-9525(92)90261-2
Ebbensgaard, A., Mordhorst, H., Overgaard, M. T., Nielsen, C. G., Aarestrup, F. M., and Hansen, E. B. (2015). Comparative evaluation of the antimicrobial activity of different antimicrobial peptides against a range of pathogenic bacteria. PLoS One 10:e0144611. doi: 10.1371/journal.pone.0144611
Fan, L., Sun, J., Zhou, M., Zhou, J., Lao, X., Zheng, H., et al. (2016). DRAMP: a comprehensive data repository of antimicrobial peptides. Sci. Rep. 6, 1–7. doi: 10.1038/srep24482
Farkas, A., Maróti, G., Dürgo, H., Györgypál, Z., Lima, R. M., Medzihradszky, K. F., et al. (2014). Medicago truncatula symbiotic peptide NCR247 contributes to bacteroid differentiation through multiple mechanisms. Proc. Natl. Acad. Sci. U.S.A. 111, 5183–5188. doi: 10.1073/pnas.1404169111
Farkas, A., Maróti, G., Kereszt, A., and Kondorosi, É (2017). Comparative analysis of the bacterial membrane disruption effect of two natural plant antimicrobial peptides. Front. Microbiol. 8:51. doi: 10.3389/fmicb.2017.00051
Fernandez, D. I., Lee, T., Sani, M., Aguilar, M., and Separovic, F. (2013). Proline facilitates membrane insertion of the antimicrobial peptide maculatin 1. 1 via surface indentation and subsequent lipid disordering. Biophys. J. 104, 1495–1507. doi: 10.1016/j.bpj.2013.01.059
Galinat, W. (1969). The evolution under doestication of the maize ear: string cob maize. Mass Agric. Exper. Stat. Bull 577, 1–19.
Gautier, R., Douguet, D., Antonny, B., and Drin, G. (2008). HELIQUEST: a web server to screen sequences with specific α-helical properties. Bioinformatics 24, 2101–2102. doi: 10.1093/bioinformatics/btn392
Giralt, E. (2014). Small wonders: peptides for disease control. edited by Kanniah Rajasekaran, Jeffrey W. Cary, Jesse M. Jaynes and Emilio Montesinos. ChemMedChem 9:233. doi: 10.1002/cmdc.201300418
Halder, T., Upadhyaya, G., Roy, S., Biswas, R., Das, A., and Bagchi, A. (2019). Glycine rich proline rich protein from Sorghum bicolor serves as an antimicrobial protein implicated in plant defense response. Plant Mol. Biol. 101, 95–112. doi: 10.1007/s11103-019-00894-y
Hamamoto, K., Kida, Y., Zhang, Y., Shimizu, T., and Kuwano, K. (2002). Antimicrobial activity and stability to proteolysis of small linear cationic peptides with D-Amino acid. J. High Energy Phys. 46, 741–749. doi: 10.1007/JHEP122012025
Hancock, R. E. W., and Scott, M. G. (2000). The role of antimicrobial peptides in animal defenses. Proc. Natl. Acad. Sci. U.S.A. 97, 8856–8861. doi: 10.1073/pnas.97.16.8856
Hassan, M., Kjos, M., Nes, I. F., Diep, D. B., and Lotfipour, F. (2012). Natural antimicrobial peptides from bacteria: characteristics and potential applications to fight against antibiotic resistance. J. Appl. Microbiol. 113, 723–736. doi: 10.1111/j.1365-2672.2012.05338.x
He, J., Luo, X., Jin, D., Wang, Y., and Zhang, T. (2018). Identification, recombinant expression, and characterization of lhg2, a novel antimicrobial peptide of lactobacillus casei HZ1. Molecules 23, 1–18. doi: 10.3390/molecules23092246
Holaskova, E., Galuszka, P., Frebort, I., and Oz, M. T. (2014). Antimicrobial peptide production and plant-based expression systems for medical and agricultural biotechnology. Biotechnol. Adv. 33, 1005–1023. doi: 10.1016/j.biotechadv.2015.03.007
Hou, X., Feng, C., Li, S., Luo, Q., Shen, G., Wu, H., et al. (2019). Mechanism of antimicrobial peptide NP-6 from Sichuan pepper seeds against E. coli and effects of different environmental factors on its activity. Appl. Microbiol. Biotechnol. 103, 6593–6604. doi: 10.1007/s00253-019-09981-y
Huang, J., Hao, D., Chen, Y., Xu, Y., Tan, J., Huang, Y., et al. (2011). Inhibitory effects and mechanisms of physiological conditions on the activity of enantiomeric forms of an α-helical antibacterial peptide against bacteria. Peptides 32, 1488–1495. doi: 10.1016/j.peptides.2011.05.023
Jhong, J. H., Chi, Y. H., Li, W. C., Lin, T. H., Huang, K. Y., and Lee, T. Y. (2019). DbAMP: an integrated resource for exploring antimicrobial peptides with functional activities and physicochemical properties on transcriptome and proteome data. Nucleic Acids Res. 47, D285–D297. doi: 10.1093/nar/gky1030
Jiang, Z., Vasil, A. I., Hale, J. D., Hancock, R. E. W., Vasil, M. L., and Hodges, R. S. (2008). Effects of net charge and the number of positively charged residues on the biological activity of amphipathic α-helical cationic antimicrobial peptides. Biopolym. Pept. Sci. Sect. 90, 369–383. doi: 10.1002/bip.20911
Joseph, S., Karnik, S., Nilawe, P., Jayaraman, V. K., and Idicula-Thomas, S. (2012). ClassAMP: a prediction tool for classification of antimicrobial peptides. IEEE/ACM Trans. Comput. Biol. Bioinforma. 9, 1535–1538. doi: 10.1109/TCBB.2012.89
Jukes, T. H., and Osawa, S. (1993). Evolutionary changes in the genetic code. Comp. Biochem. Physiol. B 106, 489–494. doi: 10.1016/0305-0491(93)90122-l
Kang, C. G., Hah, D. S., Kim, C. H., Kim, Y. H., Kim, E., and Kim, J. S. (2011). Evaluation of antimicrobial activity of the methanol extracts from 8 traditional medicinal plants. Toxicol. Res. 27, 31–36. doi: 10.5487/TR.2011.27.1.031
Keymanesh, K., Soltani, S., and Sardari, S. (2009). Application of antimicrobial peptides in agriculture and food industry. World J. Microbiol. Biotechnol. 25, 933–944. doi: 10.1007/s11274-009-9984-7
Kong, X., Yang, M., Abbas, H. M. K., Wu, J., Li, M., and Dong, W. (2018). Antimicrobial genes from Allium sativum and Pinellia ternata revealed by a Bacillus subtilis expression system. Sci. Rep. 8, 1–12. doi: 10.1038/s41598-018-32852-x
Kwon, J. Y., Kim, M. K., Mereuta, L., Seo, C. H., Luchian, T., and Park, Y. (2019). Mechanism of action of antimicrobial peptide P5 truncations against Pseudomonas aeruginosa and Staphylococcus aureus. AMB Express 9:122. doi: 10.1186/s13568-019-0843-0
Lata, S., Sharma, B. K., and Raghava, G. P. S. (2007). Analysis and prediction of antibacterial peptides. BMC Bioinformatics 8:263. doi: 10.1186/1471-2105-8-263
Lin, W., and Xu, D. (2016). Imbalanced multi-label learning for identifying antimicrobial peptides and their functional types. Bioinformatics 32, 3745–3752. doi: 10.1093/bioinformatics/btw560
Liscano, Y., Salamanca, C. H., Vargas, L., Cantor, S., Laverde-Rojas, V., and Oñate-Garzón, J. (2019). Increases in hydrophilicity and charge on the polar face of alyteserin 1c helix change its selectivity towards gram-positive bacteria. Antibiotics 8:238. doi: 10.3390/antibiotics8040238
Liu, S., Fan, L., Sun, J., Lao, X., and Zheng, H. (2017). Computational resources and tools for antimicrobial peptides. J. Pept. Sci. 23, 4–12. doi: 10.1002/psc.2947
Luo, X., Liu, Y., Qin, Z., Jin, Z., Xu, L., Liu, Y., et al. (2018). Studies on the antibacterial activity and mechanism of antimicrobial peptides against drug-resistant bacteria. J. Biomed. Nanotechnol. 14, 601–608. doi: 10.1166/jbn.2018.2528
Maisetta, G., Di Luca, M., Esin, S., Florio, W., Brancatisano, F. L., Bottai, D., et al. (2008). Evaluation of the inhibitory effects of human serum components on bactericidal activity of human beta defensin 3. Peptides 29, 1–6. doi: 10.1016/j.peptides.2007.10.013
Mardirossian, M., Sola, R., Degasperi, M., and Scocchi, M. (2019). Search for shorter portions of the proline-rich antimicrobial peptide fragment Bac5(1–25) that retain antimicrobial activity by blocking protein synthesis. ChemMedChem 14, 343–348. doi: 10.1002/cmdc.201800734
Meher, P. K., Sahu, T. K., Saini, V., and Rao, A. R. (2017). Predicting antimicrobial peptides with improved accuracy by incorporating the compositional, physico-chemical and structural features into Chou’s general PseAAC. Sci. Rep. 7, 1–12. doi: 10.1038/srep42362
Montesinos, E. (2007). Antimicrobial peptides and plant disease control. FEMS Microbiol. Lett. 270, 1–11. doi: 10.1111/j.1574-6968.2007.00683.x
Murayama, T., Masuda, T., Afonin, S., Kawano, K., Takatani-Nakase, T., Ida, H., et al. (2017). Loosening of lipid packing promotes oligoarginine entry into cells. Angew. Chemie Int. Ed. 56, 7644–7647. doi: 10.1002/anie.201703578
Murthy, K. N., Soumya, K., Srinivas, C., and Niranjana, S. R. (2019). Evaluation of carica papaya leaf extracts for their efficacy on control of bacterial wilt of Tomato caused by Ralstonia solanacearum. Int. J. Curr. Microbiol. Appl. Sci. 8, 366–380. doi: 10.20546/ijcmas.2019.803.046
Nagarajan, D., Roy, N., Kulkarni, O., Nanajkar, N., Datey, A., Ravichandran, S., et al. (2019). W 76?: a designed antimicrobial peptide to combat carbapenem- and tigecycline-resistant Acinetobacter baumannii. Sci. Adv 5:eaax1946. doi: 10.1126/sciadv.aax1946
Navi, S., Bandyopadhyay, R., Thirumala Devi, K., and Reddy, D. (2002). Bacterial leaf streak of sorghum – a new report from india. Ismn 43, 61–63.
Nawrot, R., Barylski, J., Nowicki, G., Broniarczyk, J., Buchwald, W., and Goździcka-Józefiak, A. (2014). Plant antimicrobial peptides. Folia Microbiol. 59, 181–196. doi: 10.1007/s12223-013-0280-4
Nicolas, P. (2009). Multifunctional host defense peptides: intracellular-targeting antimicrobial peptides. FEBS J. 276, 6483–6496. doi: 10.1111/j.1742-4658.2009.07359.x
Ong, Z. Y., Cheng, J., Huang, Y., Xu, K., Ji, Z., Fan, W., et al. (2014). Effect of stereochemistry, chain length and sequence pattern on antimicrobial properties of short synthetic β-sheet forming peptide amphiphiles. Biomaterials 35, 1315–1325. doi: 10.1016/j.biomaterials.2013.10.053
Osawa, S., Jukes, T. H., Watanabe, K., and Muto, A. (1992). Recent evidence for evolution of the genetic code. Microbiol. Rev. 56, 229–264. doi: 10.1128/mmbr.56.1.229-264.1992
Park, C. B., Yi, K. S., Matsuzaki, K., Kim, M. S., and Kim, S. C. (2000). Structure-activity analysis of buforin II, a histone H2A-derived antimicrobial peptide: the proline hinge is responsible for the cell-penetrating ability of buforin II. Proc. Natl. Acad. Sci. U.S.A. 97, 8245–8250. doi: 10.1073/pnas.150518097
Porto, W. F., Irazazabal, L., Alves, E. S. F., Ribeiro, S. M., Matos, C. O., Pires, ÁS., et al. (2018). In silico optimization of a guava antimicrobial peptide enables combinatorial exploration for peptide design. Nat. Commun 9:1490. doi: 10.1038/s41467-018-03746-3
Porto, W. F., Pires, A. S., and Franco, O. L. (2017). Computational tools for exploring sequence databases as a resource for antimicrobial peptides. Biotechnol. Adv. 35, 337–349. doi: 10.1016/j.biotechadv.2017.02.001
Porto, W. F., Silva, O. N., and Franco, O. L. (2012). “Prediction and rational design of antimicrobial peptides,” in Protein Structure, ed. E. Faraggi, (London: InTech), doi: 10.5772/38023
Radzicka, A., and Wolfenden, R. (1988). Comparing the polarities of the amino acids: side-chain distribution coefficients between the vapor phase, cyclohexane, 1-octanol, and neutral aqueous solution. Biochemistry 27, 1664–1670. doi: 10.1021/bi00405a042
Ronèeviæ, T., Gerdol, M., Spazzali, F., Florian, F., Mekiniæ, S., Tossi, A., et al. (2018). Parallel identification of novel antimicrobial peptide sequences from multiple anuran species by targeted DNA sequencing. BMC Genomics 19:827. doi: 10.1186/s12864-018-5225-5
Rosenthal, J. P., and Dirzo, R. (1997). Effects of life history, domestication and agronomic selection on plant defence against insects: evidence from maizes and wild relatives. Evol. Ecol. 11, 337–355. doi: 10.1023/A:1018420504439
Roy, A., Kucukural, A., and Zhang, Y. (2010). I-TASSER: a unified platform for automated protein structure and function prediction. Nat. Protoc. 5, 725–738. doi: 10.1038/nprot.2010.5
Sander, F., Goulson, A. R., and Road, H. (1976). A rapid method for determining sequences in DNA by primed synthesis with DNA polymerase. J. Mol. Bid. 94, 441–448. doi: 10.1016/0022-2836(75)90213-2
Sharma, K., Mishra, A. K., Mehraj, V., and Duraisamy, G. S. (2014). Advances and applications of molecular cloning in clinical microbiology. Biotechnol. Genet. Eng. Rev. 30, 65–78. doi: 10.1080/02648725.2014.921501
Sharma, P., Bora, L. C., Puzari, K. C., Baruah, A. M., and Baruah, R. (2017). Review on bacterial blight of rice caused by Xanthomonas oryzae pv. oryzae?: different management approaches and role of Pseudomonas fluorescens as a potential biocontrol agent. Int. J. Curr. Microbiol. Appl. Sci. 6, 982–1005. doi: 10.20546/ijcmas.2017.603.117
Shen, W., He, P., Xiao, C., and Chen, X. (2018). From antimicrobial peptides to antimicrobial poly(α-amino acid)s. Adv. Healthc. Mater. 7, 1–20. doi: 10.1002/adhm.201800354
Silhavy, T. J., Kahne, D., and Walker, S. (2010). The bacterial cell envelope. Cold Spring Harb. Perspect. Biol. 2:a000414. doi: 10.1101/cshperspect.a000414
Singh, S., Singh, H., Tuknait, A., Chaudhary, K., Singh, B., Kumaran, S., et al. (2015). PEPstrMOD: structure prediction of peptides containing natural, non-natural and modified residues. Biol. Direct 10, 1–19. doi: 10.1186/s13062-015-0103-4
Szczepaniec, A., Widney, S. E., Bernal, J. S., and Eubanks, M. D. (2013). Higher expression of induced defenses in teosintes (Zea spp.) is correlated with greater resistance to fall armyworm, Spodoptera frugiperda. Entomol. Exp. Appl. 146, 242–251. doi: 10.1111/eea.12014
Takeuchi, T., and Futaki, S. (2016). Current understanding of direct translocation of arginine-rich cell-penetrating peptides and its internalization mechanisms. Chem. Pharm. Bull. 64, 1431–1437. doi: 10.1248/cpb.c16-00505
Tancos, M. A., Chalupowicz, L., Barash, I., Manulis-Sasson, S., and Smart, C. D. (2013). Tomato fruit and seed colonization by Clavibacter michiganensis subsp. michiganensis through external and internal routes. Appl. Environ. Microbiol. 79, 6948–6957. doi: 10.1128/AEM.02495-13
Tavares, L. S., de Souza, V. C., Schmitz Nunes, V., Nascimento Silva, O., de Souza, G. T., Farinazzo Marques, L., et al. (2020). Antimicrobial peptide selection from Lippia spp leaf transcriptomes. Peptides 129:170317. doi: 10.1016/j.peptides.2020.170317
Thomas, S., Karnik, S., Barai, R. S., Jayaraman, V. K., and Idicula-Thomas, S. (2010). CAMP: a useful resource for research on antimicrobial peptides. Nucleic Acids Res. 38, 774–780. doi: 10.1093/nar/gkp1021
Torrent, M., Di Tommaso, P., Pulido, D., Nogués, M. V., Notredame, C., Boix, E., et al. (2012). AMPA: an automated web server for prediction of protein antimicrobial regions. Bioinformatics 28, 130–131. doi: 10.1093/bioinformatics/btr604
Tossi, A., Sandri, L., and Giangaspero, A. (2000). Amphipathic, α-helical antimicrobial peptides. Biopolym. Pept. Sci. Sect. 55, 4–30. doi: 10.1002/1097-0282200055:1<4::AID-BIP30>3.0.CO;2-M
Triana-Vidal, L. E., Castro, M. S., Pires Júnior, O. R., Álvares, A. C. M., de Freitas, S. M., Fontes, W., et al. (2018). Dendropsophin 1, a novel antimicrobial peptide from the skin secretion of the endemic Colombian frog Dendropsophus columbianus. Nat. Prod. Res. 32, 1383–1389. doi: 10.1080/14786419.2017.1346646
Van De Velde, W., Zehirov, G., Szatmari, A., Debreczeny, M., Ishihara, H., Kevei, Z., et al. (2010). Plant peptides govern terminal differentiation of bacteria in symbiosis. Science 327, 1122–1126. doi: 10.1126/science.1184057
Vandenbossche, R., Demedts, B., Vanderhaeghen, R., and Goossens, A. (2013). Chapter 18: transient expression assays in tobacco protoplasts. Jasmonate Signal. 1011, 227–239. doi: 10.1007/978-1-62703-414-2
Velivelli, S. L. S., Islam, K. T., Hobson, E., and Shah, D. M. (2018). Modes of action of a Bi-domain plant defensin MtDef5 against a bacterial pathogen Xanthomonas campestris. Front. Microbiol. 9:934. doi: 10.3389/fmicb.2018.00934
Vishnepolsky, B., and Pirtskhalava, M. (2014). Prediction of linear cationic antimicrobial peptides based on characteristics responsible for their interaction with the membranes. J. Chem. Inf. Model. 54, 1512–1523. doi: 10.1021/ci4007003
Waghu, F. H., Barai, R. S., Gurung, P., and Idicula-Thomas, S. (2016). CAMPR3: a database on sequences, structures and signatures of antimicrobial peptides. Nucleic Acids Res. 44, D1094–D1097. doi: 10.1093/nar/gkv1051
Wang, G. (2015). Improved methods for classification, prediction, and design of antimicrobial peptides. Methods Mol. Biol. 1268, 43–66. doi: 10.1007/978-1-4939-2285-7_3
Wang, Q., Yang, S., Liu, J., Terecskei, K., Abrahám, E., Gombár, A., et al. (2017). Host-secreted antimicrobial peptide enforces symbiotic selectivity in Medicago truncatula. Proc. Natl. Acad. Sci. U.S.A. 114, 6854–6859. doi: 10.1073/pnas.1700715114
Wiederstein, M., and Sippl, M. J. (2007). ProSA-web: interactive web service for the recognition of errors in three-dimensional structures of proteins. Nucleic Acids Res. 35, 407–410.
Wiegand, I., Hilpert, K., and Hancock, R. E. W. (2008). Agar and broth dilution methods to determine the minimal inhibitory concentration (MIC) of antimicrobial substances. Nat. Protoc. 3, 163–175. doi: 10.1038/nprot.2007.521
Williams, C. J., Headd, J. J., Moriarty, N. W., Prisant, M. G., Videau, L. L., Deis, L. N., et al. (2018). MolProbity: more and better reference data for improved all-atom structure validation. Protein Sci. 27, 293–315. doi: 10.1002/pro.3330
Wu, J., Abbas, H. M. K., Li, J., Yuan, Y., Liu, Y., Wang, G., et al. (2020). Cell membrane-interrupting antimicrobial peptides from isatis indigotica fortune isolated by a bacillus subtilis expression system. Biomolecules 10:30. doi: 10.3390/biom10010030
Wu, X., Wang, Z., Li, X., Fan, Y., He, G., Wan, Y., et al. (2014). In vitro and in vivo activities of antimicrobial peptides developed using an amino acid-based activity prediction method. Antimicrob. Agents Chemother. 58, 5342–5349. doi: 10.1128/AAC.02823-14
Wu, Y., Wang, L., Zhou, M., Chen, T., and Shaw, C. (2019). Phylloseptin-PBa1, -PBa2, -PBa3: three novel antimicrobial peptides from the skin secretion of Burmeister’s leaf frog (Phyllomedusa burmeisteri). Biochem. Biophys. Res. Commun. 509, 664–673. doi: 10.1016/j.bbrc.2018.12.156
Würz, J. M., and Güntert, P. (2017). Peak picking multidimensional NMR spectra with the contour geometry based algorithm CYPICK. J. Biomol. 67, 63–76. doi: 10.1007/s10858-016-0084-3
Xin, X.-F., and He, S. Y. (2013). Pseudomonas syringae pv. tomato DC3000: a model pathogen for probing disease susceptibility and hormone signaling in plants. Annu. Rev. Phytopathol. 51, 473–498. doi: 10.1146/annurev-phyto-082712-102321
Yang, L. Y., Yang, S. L., Li, J. Y., Ma, J. H., Pang, T., Zou, C. M., et al. (2018). Effects of different growth temperatures on growth, development, and plastid pigments metabolism of tobacco (Nicotiana tabacum L.) plants. Bot. Stud. 59:5. doi: 10.1186/s40529-018-0221-2
Yang, M., Zhang, C., Zhang, M. Z., and Zhang, S. (2018). Beta-defensin derived cationic antimicrobial peptides with potent killing activity against gram negative and gram positive bacteria. BMC Microbiol. 18:54. doi: 10.1186/s12866-018-1190-z
Ying, S. Y. (2004). Complementary DNA libraries: an overview. Appl. Biochem. Biotechnol Part B Mol. Biotechnol. 27, 245–252. doi: 10.1385/MB:27:3:245
Yount, N. Y., Bayer, A. S., Xiong, Y. Q., and Yeaman, M. R. (2006). Advances in antimicrobial peptide immunobiology. Biopolymers 84, 435–458. doi: 10.1002/bip.20543
Zelezetsky, I., and Tossi, A. (2006). Alpha-helical antimicrobial peptides-using a sequence template to guide structure-activity relationship studies. Biochim. Biophys. Acta Biomembr. 1758, 1436–1449. doi: 10.1016/j.bbamem.2006.03.021
Zhang, X., Shi, C., Liu, Z., Pan, F., Meng, R., Bu, X., et al. (2018). Antibacterial activity and mode of action of ε-polylysine against Escherichia coli O157:H7. J. Med. Microbiol. 67, 838–845. doi: 10.1099/jmm.0.000729
Zhou, P., and Huang, J. (2015). Computational Peptidology, Vol. 1268. Totowa, NJ: Humana Press, 1–333. doi: 10.1007/978-1-4939-2285-7
Zhu, X., Shan, A., Ma, Z., Xu, W., Wang, J., Chou, S., et al. (2015a). Bactericidal efficiency and modes of action of the novel antimicrobial peptide T9W against Pseudomonas aeruginosa. Antimicrob. Agents Chemother. 59, 3008–3017. doi: 10.1128/AAC.04830-14
Keywords: antimicrobial peptide, AMP prediction, bacterial pathogens, membrane damage, teosinte
Citation: Qutb AM, Wei F and Dong W (2020) Prediction and Characterization of Cationic Arginine-Rich Plant Antimicrobial Peptide SM-985 From Teosinte (Zea mays ssp. mexicana). Front. Microbiol. 11:1353. doi: 10.3389/fmicb.2020.01353
Received: 28 March 2020; Accepted: 26 May 2020;
Published: 19 June 2020.
Edited by:
Adi Avni, Tel Aviv University, IsraelReviewed by:
Igor V. Komarov, Taras Shevchenko National University of Kyiv, UkraineWilliam Farias Porto, Dom Bosco Catholic University, Brazil
Copyright © 2020 Qutb, Wei and Dong. This is an open-access article distributed under the terms of the Creative Commons Attribution License (CC BY). The use, distribution or reproduction in other forums is permitted, provided the original author(s) and the copyright owner(s) are credited and that the original publication in this journal is cited, in accordance with accepted academic practice. No use, distribution or reproduction is permitted which does not comply with these terms.
*Correspondence: Wubei Dong, ZHdiQG1haWwuaHphdS5lZHUuY24=
†ORCID: Wubei Dong, orcid.org/0000-0001-8513-8751