- 1Department of Plant and Wildlife Sciences, Brigham Young University, Provo, UT, United States
- 2Department of Biology and the Ecology Center, Utah State University, Logan, UT, United States
- 3Department of Geological Sciences, Brigham Young University, Provo, UT, United States
Stream bacterioplankton communities, a crucial component of aquatic ecosystems and surface water quality, are shaped by environmental selection (i.e., changes in taxa abundance associated with more or less favorable abiotic conditions) and passive dispersal (i.e., organisms’ abundance and distribution is a function of the movement of the water). These processes are a function of hydrologic conditions such as residence time and water chemistry, which are mediated by human infrastructure. To quantify the role of environmental conditions, dispersal, and human infrastructure (dams) on stream bacterioplankton, we measured bacterioplankton community composition in rivers from sub-alpine to urban environments in three watersheds (Utah, United States) across three seasons. Of the 53 environmental parameters measured (including physicochemical parameters, solute concentrations, and catchment characteristics), trace element concentrations explained the most variability in bacterioplankton community composition using Redundancy Analysis ordination. Trace elements may correlate with bacterioplankton due to the commonality in source of water and microorganisms, and/or environmental selection creating more or less favorable conditions for bacteria. Bacterioplankton community diversity decreased downstream along parts of the stream continuum but was disrupted where large reservoirs increased water residence time by orders of magnitude, potentially indicating a shift in the relative importance of environmental selection and dispersal at these sites. Reservoirs also had substantial effects on community composition, dissimilarity (Bray-Curtis distance) and species interactions as indicated by co-occurrence networks. Communities downstream of reservoirs were enriched with anaerobic Sporichthyaceae, methanotrophic Methylococcaceae, and iron-transforming Acidimicrobiales, suggesting alternative metabolic pathways became active in the hypolimnion of large reservoirs. Our results identify that human activity affects river microbial communities, with potential impacts on water quality through modified biogeochemical cycling.
Introduction
Bacterioplankton, the portion of stream microbial communities suspended within the water column, are a crucial component of stream ecosystems. Despite their importance, aquatic microbial communities are often treated as a black box in aquatic environments (Allison and Martiny, 2008). As land and water use alter biogeochemical fluxes and hydrological characteristics of aquatic ecosystems (Abbott et al., 2018; Blaszczak et al., 2019), understanding the drivers of microbial community composition is critical to assessing the scope of human impacts on freshwater systems (Lindström and Östman, 2011). Stream microbial communities are shaped by two interacting processes: environmental selection (Zwart et al., 2002; Fierer et al., 2007; Fierer and Lennon, 2011), and dispersal (Crump et al., 2007, 2012; Findlay, 2010; Savio et al., 2015; Albright and Martiny, 2018). Environmental selection leads to species sorting along stream habitats, with distinct microbial communities and interactions emerging across environmental gradients and habitat patches (Leibold et al., 2004; Niño-García et al., 2016). Dispersal, the movement of organisms from one environment or location into another, is particularly relevant for bacterioplankton, because these organisms are passively transported downstream with the constant movement of water.
The most important environmental factors for structuring bacterial communities are pH, salinity, temperature, and dissolved oxygen, because of their role in controlling, or being controlled by, cellular activity (Fierer and Jackson, 2006; Fierer et al., 2007; Doherty et al., 2017). Water residence time moderates the duration that bacterial communities are influenced by the environment (Ben Maamar et al., 2015; Abbott et al., 2016; Niño-García et al., 2016), potentially leading to feedback loops where bacteria engineer new conditions that select for an altered set of taxa (Figure 1). For example, longer residence times lead to anoxic conditions at and below the sediment-water interface as microbial decomposition exceeds reaeration rates (Baker et al., 2000; Zarnetske et al., 2011). Once anoxic conditions are created, alternative terminal electron acceptor pathways become activated, with many subsequent changes in water chemistry; for example, anoxic ecosystems switch from net bacterial nitrification to denitrification (Briggs et al., 2013; Oldham et al., 2013; Kolbe et al., 2019). However, dozens of environmental parameters, including physicochemical conditions and solute concentrations, affect the abundance of bacterial taxa due to selective pressure (i.e., species sorting). Trace elements, such as molybdenum (a cofactor in the enzyme nitrogenase) and rare earth elements like lanthanum, may stimulate growth at low concentrations, but may also be toxic at high concentrations (Herrmann et al., 2016; Smedley and Kinniburgh, 2017). Macronutrients and ions generally considered limiting for autotrophic organisms, such as nitrogen (N), phosphorus (P), and potassium (K), may be less important for explaining bacterial community composition, because bacterial metabolic pathways are able to metabolize even recalcitrant substrates into more bioavailable species (Zeglin, 2015). Organic matter quality and quantity in soils and streams also correlate with specific bacterial communities and ultimately bacterial activity across a broad range of climates and bedrock materials (Gabor et al., 2014; Ruiz-González et al., 2015; Miller et al., 2016). Explaining bacterioplankton community taxonomic composition with instantaneous stream chemistry conditions is complicated by temporal changes in the source and flow path of water entering a channel (Leff and Lemke, 1998; Dahlke et al., 2012; Moatar et al., 2017). Seasonal changes in hydrology and environmental parameters affect the concentration, form, and downstream availability of organic and inorganic matter necessary for, or inhibitory to, microbial function (Duff and Triska, 2000; Hendricks and White, 2000).
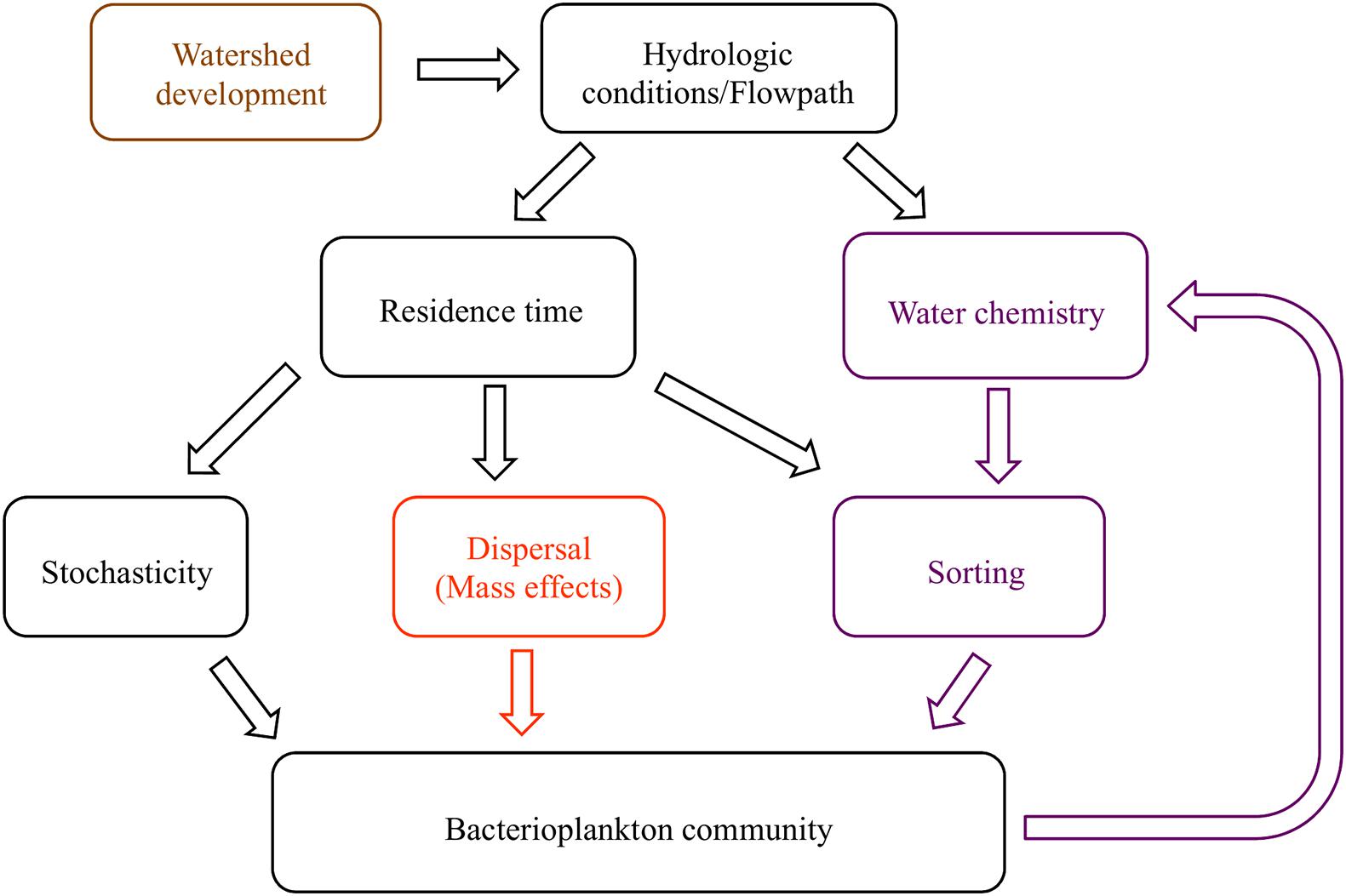
Figure 1. Conceptual diagram of the proposed relationships between hydrologic conditions and bacterioplankton communities. Residence time, a hydrologic condition related to flow velocity and volume, influences the extent that dispersal and stochastic processes alter community composition. Residence time also controls the extent to which environmental pressures act on communities to create species sorting, or selection. When dispersal is high, mass effects dominate; otherwise, dispersal limitation may occur. Bacterial metabolic activity affects water chemistry, creating a feedback loop within the model (hypothesis 1, purple). The community composition similarity along and between watersheds is related to longitudinal and lateral dispersal (hypothesis 2, orange). Anthropogenic changes to watersheds affect hydrologic conditions, and with repercussions on all aspects of the system (hypothesis 3, brown).
The effect of selective pressure by the environment on a community is constrained by which taxa are present through dispersal. Stream-lake networks are unique because of their dendritic nature, which creates specific patterns in biodiversity (Widder et al., 2014). Bacterioplankton dispersal in streams is constrained to directional flow paths and, like stream water chemistry, is ultimately the combination of longitudinal (i.e., upstream to downstream in channel), vertical (groundwater-surface-water exchange), and lateral (i.e., stream-bank exchange and tributaries) contributions (Covino, 2017). Headwater inputs and longitudinal connectivity are crucial for maintaining downstream community composition and potentially biogeochemical function; alpha and beta diversity are highest in low-order streams, and decrease as stream order increases (Crump et al., 2007; Besemer et al., 2013; Savio et al., 2015). River systems are naturally punctuated by lakes and other abrupt changes in the natural topography (Ward and Stanford, 1983). The role of artificial reservoirs in driving bacterioplankton communities has become increasingly important as they store an estimated four times the volume river water (2,000 km3, compared to 8,000 km3) with an additional 200,000 km3 in lakes (Shiklomanov, 1993; Messager et al., 2016; Abbott et al., 2019). Natural streams are less of a gradual longitudinal continuum and more often a series of highly distinct environments, particularly when considering microhabitats of bacteria, but lakes and reservoirs have been incorporated into a limited number of studies of riverine bacterial communities, including large rivers (Ruiz-González et al., 2013; Chen et al., 2020), and arctic and boreal lake networks (Adams et al., 2014; Niño-García et al., 2016).
Discontinuity in modern streams comes from both natural and human sources as humans have increasingly altered landscapes and introduced infrastructure along rivers that impede the flow of water and associated matter (Grimm et al., 2008; Hale et al., 2015; Grill et al., 2019). Links among bacterial communities, stream characteristics, and dispersal must consider the influence that urbanization exerts on natural processes due to increased infrastructure development to support growing populations in nearly every aspect of streams and rivers (Meybeck, 2003). Reservoirs and regulated lakes (natural lakes modified with infrastructure to provide managers with control over flow and lake elevation) behave differently than natural lakes (Döll et al., 2009), so understanding their unique impact on stream bacterial communities is crucial. Land use changes, such as agriculture, mining, forestry, and urbanization, may result in less direct changes to stream conditions by impacting hydrologic connections between upland, groundwater, and stream ecosystems (Rose and Peters, 2001; Meybeck, 2003). An estimated 77% of global land area is affected directly by land use change; indirect anthropogenic effects increase that number to 100% (Vitousek et al., 1997; Watson et al., 2018). Disturbance of the land surface affects water flowpath and chemistry, altering the microbial community in soils, aquifers, and surface waters (Covino, 2017; Moatar et al., 2017). Without more comprehensive understanding of the controls on stream bacterioplankton, it is almost impossible to account for the complex interactions between land use change, altered hydrology, and water chemistry, and alterations in bacterial communities (Fisher et al., 2015; Van Rossum et al., 2015; Hall et al., 2018).
Northern Utah streams provide an ideal setting to understand anthropogenic and seasonal impacts on bacterioplankton and the interactions between water chemistry and bacterial communities. Land uses in the region include agriculture, forestry, mining and urban development, with built infrastructure encompassing reservoirs, irrigation systems, cross-basin diversions and flood control to support human industries (Hall et al., 2015). To understand the effect of environmental conditions, dispersal mechanisms, and human infrastructure on stream bacterioplankton community composition, we collected bacterioplankton and a suite of environmental parameters from five locations along montane to urban gradients in three northern Utah watersheds, over three seasons (fall, spring, and winter). We hypothesized that: (1) bacterioplankton communities will correlate with standard water quality metrics, (pH, temperature, specific conductivity, and dissolved oxygen), more than other groups of variables (nutrients, major ions, trace elements and organic matter) because of their importance in regulating essential metabolic processes, unless basic physicochemical conditions are relatively homogeneous (Lindström et al., 2005; Fierer and Jackson, 2006; Niño-García et al., 2016; Henson et al., 2017); (2) Bacterial communities will be less diverse below reservoirs, possibly due to dispersal limitation or species sorting within reservoirs, corresponding to increased residence time (Lindström and Östman, 2011), and (3) the bacterioplankton communities in urban environments will be less diverse than sub alpine streams, possibly due to the homogenization of hydrologic conditions and flowpath (Figure 1).
Materials and Methods
Study Sites
Our project was conducted in three northern Utah, USA watersheds, selected as part of the iUTAH (innovative Urban Transitions and Aridregion Hydro-sustainability) project, funded by the NSF Established Program to Stimulate Competitive Research (EPSCoR) (Jones et al., 2017). The watersheds include Red Butte Creek, Logan River, and Provo River (Figure 2). Within these watersheds, long-term stream sampling sites were selected from subalpine (up to 2,368 m.a.s.l) elevations to low-elevation (down to 1,353 m.a.s.l.) urban and agricultural land uses to capture the effects of elevation and urbanization on water resources (Table 1). Snowmelt-dependent streams in the region flow from undeveloped mountains into densely populated valleys. In the three study watersheds, this transition is demarcated by dams of varying sizes built to meet urban and agricultural water demand in the semi-arid climate of the basins below. Logan River passes through a series of smaller impoundments compared to the other watersheds, with shallow reservoirs and much lower water residence times (Table 2). We included sites above and immediately downstream of the reservoirs to measure the effect of the introduced infrastructure on the bacterial community composition.
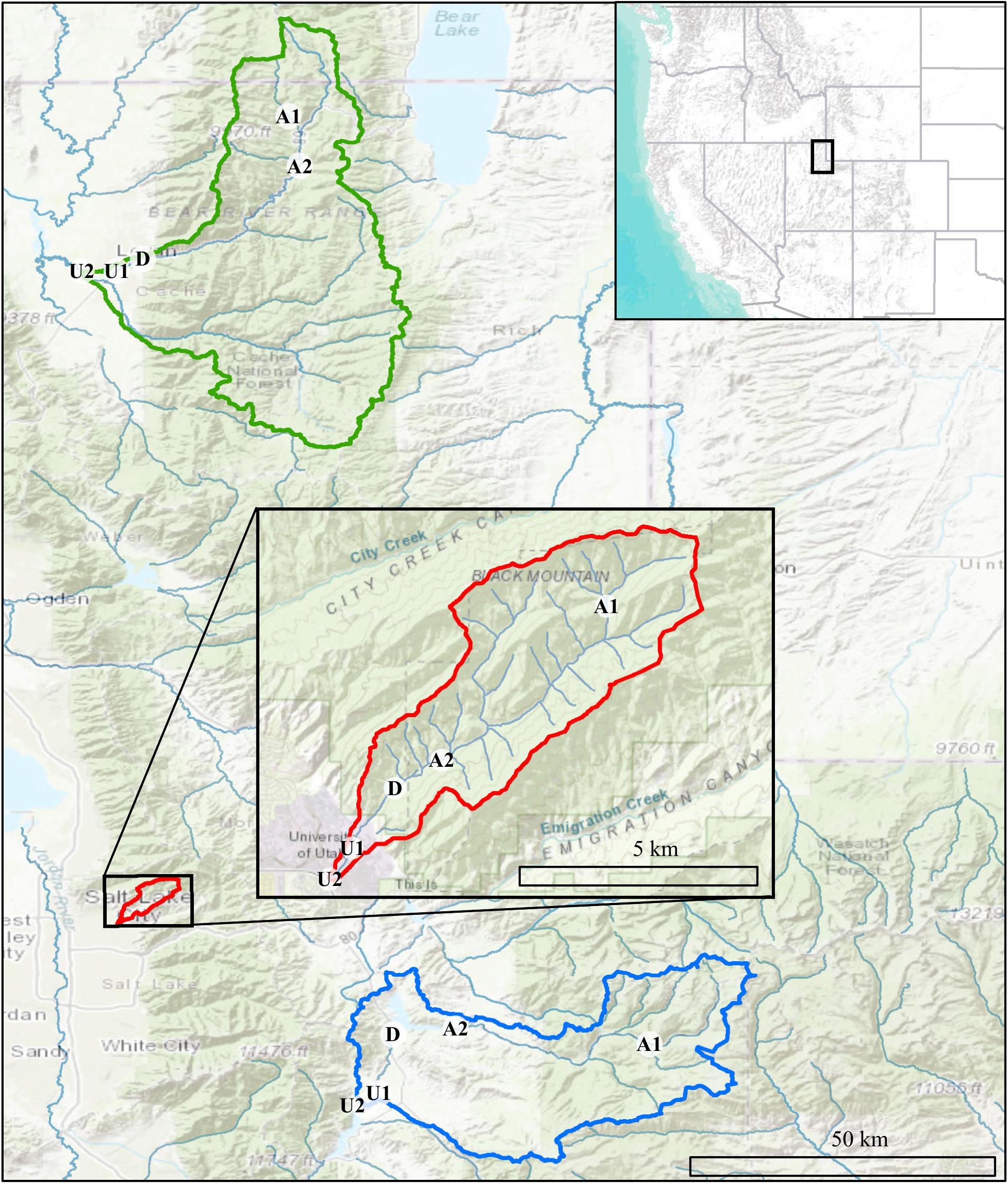
Figure 2. Map showing Logan (green), Red Butte (red), and Provo (blue) Watersheds in the Wasatch Range Metropolitan Area and locations within watersheds where bacterial communities and water quality data were collected. Location markers indicate position relative to man-made reservoirs and urban centers in all three watersheds: A, Above; D, below Dam; U, Urban. Site metadata is included in Table 1. Sources: ESRI, USGS, NOAA.
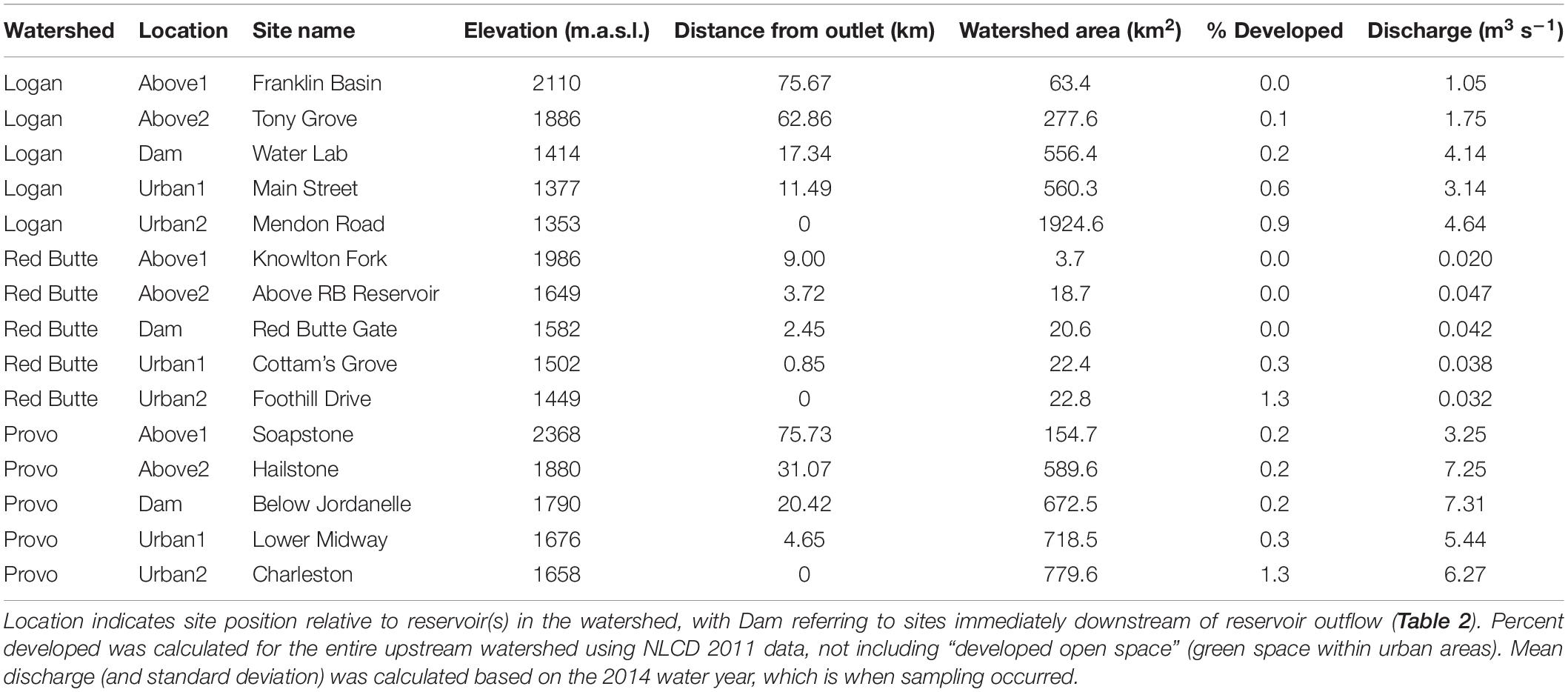
Table 1. Stream site characteristics in the Logan, Red Butte, and Provo River Watersheds (Figure 2).
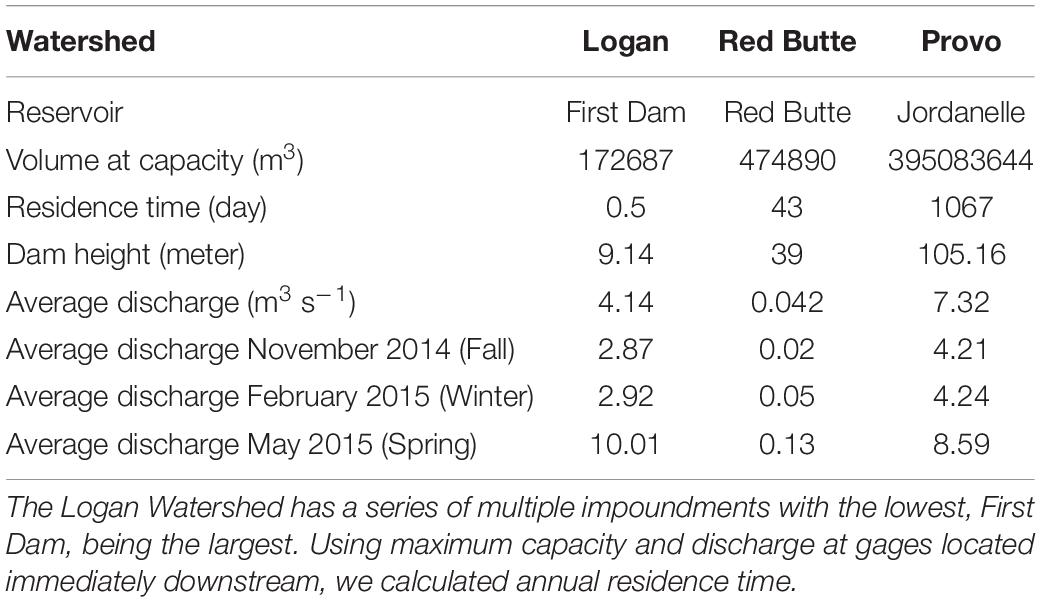
Table 2. Physical specifications and discharge of the reservoirs and dams within the three study reaches.
The degree and type of watershed development is variable within and among the three watersheds. The headwaters of each watershed are federal land and have some degree of protection from urbanization. Red Butte Creek has the most stringent protections; its headwaters are designated as a natural research preserve. The most impacted headwaters are in the Logan River watershed, which is opened to livestock grazing each summer (Hall et al., 2015). Each of the three watersheds’ valleys has a unique urban development type, allowing a comparison of a range of human activity. The Red Butte Creek watershed experienced urbanization beginning two centuries ago with Fort Douglas, one of the oldest permanently maintained developments in Utah; the subsequent construction of the University of Utah campus and residential areas exclude any present-day agricultural land use (Ehleringer et al., 1992). The Logan River watershed has valley sites that are a mixture of agriculture with some urban land uses, as the slow population growth in Cache County has resulted in a gradual shift from an agriculture-dominant to urbanized landscape. The middle region of the Provo River is also shifting from agricultural land use to newer urban development and was designated the fastest growing area in the country in 2016 (4.7% annual increase in population, US Census Bureau, 2017).
Bacterioplankton Community Composition
We designed a sampling regime to capture longitudinal urbanization gradients and the effect of seasonal changes in hydrology and environmental conditions on bacterioplankton communities. We collected water column samples at fifteen sites, including five locations (named for position relative to reservoirs) in each of the three study watersheds (Figure 2). Streams were sampled in November 2014 (Fall), which is dominated by low-flow conditions and subsequently high residence times, with leaf litter potentially providing organic matter subsidies to inorganic groundwater contributions; in February 2015 (Winter) to capture low-flow, snow-covered conditions when flows and inputs are likely most homogenous within and across watersheds; and in May 2015 (Spring) to capture peak runoff conditions, when residence times are lowest and lateral connectivity is high. We chose to collect suspended bacteria because these communities are highly responsive to urbanization- and season-driven changes in water residence time, and sampling them can capture spatial and temporal shifts in bacterial dispersal influenced by the surrounding watershed along the stream continuum. Focusing on bacterioplankton also allowed us to collect a more comprehensive sample and eliminate possible differences due to cross-site variable streambed material, which influences biofilm establishment based on size fraction and mineral type (Donlan, 2002; McCormick et al., 2014).
We used a metabarcoding approach for identifying bacterioplankton community composition. In the field, we filtered water onto 47-mm 0.2-μm PES Supor filters (Pall) using autoclaved filter cups (Nalgene) until filters were clogged, which varied between 100 and 700 mL (adapted from Somerville et al., 1989). We stored filters in cryovials in liquid nitrogen to immediately suspend microbial activity. We stored samples at −80°C until extracting with PowerSoil DNA extraction kits according to manufacturer instructions (MOBIO). We PCR-amplified the V4 region of the bacterial 16S rRNA gene with primer set 515F and 806R (Caporaso et al., 2011). After checking that amplification proceeded normally using gel electrophoresis, we purified and normalized samples (SequalPrep Normalization Plate Kit, Invitrogen). Samples were submitted to the Brigham Young University DNA Sequencing Center1 for 2 × 250 base pair paired-end sequencing on an Illumina HiSeq 2500 System. We processed sequences using a modified Mothur pipeline using SILVA-based reference alignment version 128 (Schloss et al., 2009; Quast et al., 2013). We calculated the relative abundances of bacterial taxa at the 97% operational taxonomic unit (OTU) similarity cutoff. All community inferences were based on 41 samples with 138,458 total sequences rarefied to 15,445 sequences, and 1,450 unique OTUs with samples possessing an average sequencing coverage of 90.1% ± 0.62 (mean and standard error). Four samples were removed during QA/QC due to low read counts including: Provo Above2 Fall, Logan Above1 Winter, Logan Dam Winter, and Logan Below1 Winter. All bacterial sequences are available at http://www.hydroshare.org/resource/48fc6871c51d436b83000a8d29ddb702, and code used in Mothur can be downloaded at https://github.com/erinfjones.
Environmental Factors
We quantified a wide range of environmental variables concurrent with bacterial sampling to identify which parameters correlated to community changes (see section “Environmental Drivers of Bacterioplankton Communities”). We measured standard water quality parameters (pH, water temperature, dissolved oxygen, conductivity) using either a YSI Quatro multiparameter probe or YSI EXO2 sonde (data downloaded from iUTAH web services using the R package WaterML; Kadlec et al., 2015; Jones et al., 2017). We analyzed nutrients potentially related to bacterial activity, including total nitrogen (TN, persulfate oxidation digestion and cadmium reduction method), total phosphorus (TP, persulfate oxidation digestion and ascorbic acid method), nitrate (EPA 353.2), ammonia (EPA 350.1), and dissolved orthophosphate (EPA 365.1) colorimetrically on an autoanalyzer (Astoria-Pacific). Total and volatile suspended solids were determined from combustion of pre-ashed Glass Fiber Filters (GF/F, Whatman) at 450°C for 2 h, and chlorophyll α was analyzed using ethanol extraction of filters followed by analysis on a handheld Turner Aquaflor fluorometer (Sartory and Grobbelaar, 1984; Steinman et al., 2017). Dissolved organic carbon (DOC), determined by acidification or sparging of inorganic carbon followed by combustion catalytic oxidation and NDIR detection, and total dissolved nitrogen (TDN), using the catalytic thermal decomposition/chemiluminescence method, were determined using the Shimadzu TOC analyzer. We measured major anion concentrations (F, Cl, and SO4) on a Dionex ICS-90 ion chromatograph. Major cations (Ca, K, Mg, Na) and trace elements (Ag, Al, As, B, Ba, Be, Cd, Ce, Co, Cr, Cs, Cu, Eu, Fe, La, Li, Lu, Mn, Mo, Nd, Ni, Pb, Rb, Sb, Se, Sm, Sr, Tb, Ti, Tl, U, V, Y, and Zn), which potentially shape bacterial community structure (Zeglin, 2015), were measured using an Agilent 7500ce quadrupole inductively coupled plasma mass spectrometer (ICP-MS, Goodsell et al., 2017). Stable isotopes in water (δ18O and δD), which we included with trace elements as a surrogate of water source (Follstad Shah et al., 2019), were measured on unfiltered aliquots using a Los Gatos Research Liquid Water Isotope Analyzer (LWIA-24d, Carling et al., 2015).
We characterized spectrofluorometric properties of dissolved organic matter (hereafter “organic matter”) from excitation emission matrices (EEMs) using a Horiba Aqualog spectrofluorometer (Horiba Scientific). EEMs were collected over excitation wavelengths 248–830 nm at 6 nm increments and over emissions 249.4–827.7 nm at 4.7 nm (8 pixel) increments. All samples were collected in Signal/Reference ratio mode to control for fluctuations in light source, and samples that exceeded 0.3 absorbance units at excitation 254 nm were diluted with deionized water. All samples were corrected for inner filter effects, Rayleigh scatter, and blank subtracted in MATLABTM (version 6.9; MathWorks) as described in Murphy et al. (2013). We calculated six indices from the EEMs, including: the beta:alpha index (BIX), where higher values represent more microbially derived DOM (Parlanti et al., 2000; Huguet et al., 2009); humification index (HIX) with higher values representing more humic-like material (Zsolnay et al., 1999); fluorescence index (FI), a ratio of fulvic- vs. humic-like organic matter (McKnight et al., 2001); TC index, the ratio of maximum fluorescence in the peak T region (protein-like) versus peak C region (humic-like), with higher values representing more protein-like organic matter, including WWTP effluent (Baker et al., 2008); SUVA254, specific ultraviolet absorbance at 254 nm, an indicator of aromaticity (Weishaar et al., 2003); and Total EEM intensity, which correlates to the concentration of organic carbon in the sample.
EEMS were also used to resolve a 4 component PARAFAC model following protocols outlined in Supplementary Methods (Murphy et al., 2013). Components characterized as humic-like or protein like based on peak location and comparison to previously reported fluorophores in the OpenFluor library (Murphy et al., 2014). Component 1 represented percent organic matter within component 1 (humic-like, developed), component 2 (humic-like, forested), component 3 (protein-like, tryptophan-like, developed), and component 4 (protein-like, tyrosine and tryptophan, forested); and % Protein, the percent protein-like organic matter as indicated by the sum of components 3 and 4 (Supplementary Figure 1).
We also calculated two land use parameters that may explain changes in bacterioplankton communities: percent developed area (not including developed open space such as parks) and percent impervious surface, using 2011 National Land Cover Database (NLCD) and watersheds delineated from 10-m digital elevation models (DEM) from the Utah Automated Geographic Reference Center (AGRC) in ESRI ArcMap 10 (Van Rossum et al., 2015; Chen et al., 2018).
Statistical Analysis
Environmental Drivers of Bacterioplankton Communities
To evaluate the effect of environmental factors on the bacterial communities, we grouped variables into five categories and generated redundancy analyses (RDA) for each category. The five categories were standard field parameters (watershed, season, elevation,% impervious surface, pH, DO, temperature, specific conductivity, turbidity), nutrients (NH4, NO3, TDN, PO4, TN, DOC, SO4), major ions (Na, Mg, K, Ca, F, Cl), trace elements and isotopes (Li, B, Al, V, Mn, Fe, Co, Ni, Cu, Zn, As, Se, Rb, Sr, Y, Mo, Sb, Ba, La, Ce, Eu, Pb, U, δ18O, δD), and dissolved organic matter (BIX, HIX, FI, TC, SUVA254, Total intensity, % Protein, component 1, component 2, component 3, component 4). For each model, we calculated Pearsons correlation values and removed variables with multiple exceedances of R > 0.8 one at a time until all values were below < 0.8 to reduce autocorrelation. We selected marginally significant variables for each model (P < 0.1) using backwards step-wise regression using the R package vegan (Zelenı, 2011; Oksanen et al., 2015). We used RDA and not canonical correspondence analysis (CCA) after determining that our environmental variables followed a linear and not unimodal distribution (DCA axis lengths < 3.0, Lepš and Šmilauer, 2003). We calculated variance inflation factor (VIF), and removed any variables with VIF scores greater than 6 (James et al., 2013). For each RDA, we report adjusted R2 (the percent of variability in community composition explained, calculated by a PERMANOVA test of the model), constrained proportion (cp; the amount of variability in community composition explained with environmental variables, i.e., the constrained model, compared to without, i.e., the unconstrained model), and axis values (the percent of community composition explained by the first and second ordination axes). Ordination plots of models 1–5 are included in Supplementary Figures 2–6. Variables selected by RDA models 1–4 were combined and used in a sixth backward step-wise regression. Model 5, correlating dissolved organic matter characterization with communities, was generated using a subset of only 19 samples for which EEMs were available; to avoid reducing the number of samples included in the combined model or skewing results by attempting to interpolate missing values, we excluded organic matter variables in the combined model. Model 7 includes the sampling design factors. We also used variation partitioning to test the variability in community correlated to each matrix of environmental factors against principal coordinates of neighbor matrices (PCNM), which we calculated from distance matrices of latitude and longitude data (Buttigieg and Ramette, 2014). The variables selected by the combined model were rescaled and tested for differences between sites, watersheds, and seasons using ANOVA and TukeyHSD, histograms of residuals showed statistical assumptions were met.
Bacterioplankton Community Dynamics Along the Stream Continuum
Community similarity between watersheds, locations, and seasons was calculated using principal coordinate analysis (PCoA) of Bray-Curtis dissimilarities (phyloseq package; McMurdie and Holmes, 2013) and statistically tested using permutational multivariate analysis of variance (PERMANOVA) and visualized using ggplot2 (Wickham, 2016). To determine which of the watersheds, locations or seasons were different from the others, we used pairwise PERMANOVA tests, using the Holm method to correct for multiple comparisons (pairwise.Adonis R package; Martinez Arbizu, 2019). We tested for changes in bacterial diversity related to watershed, location, and season by calculating observed richness and Shannon diversity index. Values were graphed using box and whisker plots in R package ggplot2, with the box indicating interquartile range and whiskers showing the high and low extent of observations, with medians shown as a middle bar (Wickham, 2016).
Human Influence on Bacterioplankton Communities
We built community co-occurrence network models to compare the core community topology by analyzing samples grouped by watershed. The models were based on the maximal information coefficient (MIC) analysis in R package minerva (Reshef et al., 2011; Filosi et al., 2014). The nodes in the network models represent OTUs and edges represent significant co-occurrence connections that occur in at least 75% of samples in each watershed and have an MIC that is both > 0.7 and statistically significant (P < 0.01; Junker and Schreiber, 2011). We exported the graphs from R using igraph into Gephi (v. 0.8.2-beta; Bastian et al., 2009), where we visualized networks and calculated network statistics (Campbell, 2015).
To identify which taxa had unique patterns in abundance, we used analysis of composition of microbiomes (ANCOM) to determine which taxa (grouped by family) were significantly different in relative abundance between watersheds when controlled for location and season (Mandal et al., 2015). The results of the ANCOM were visualized using heatmap (R basic), where darker color indicates higher relative abundance. Samples and families are clustered by similarity in relative abundance, indicated by dendrograms on relevant axes (i.e., trees do not represent phylogeny of taxa). We calculated changes in percent relative abundance between different categories and reported means and standard deviations. All R code used for analysis is available at https://github.com/erinfjones/GAMUTdownload.
Results
Environmental Drivers of Bacterioplankton Communities
Of the six RDA models, encompassing 53 environmental variables, trace elements and isotopes explained the most community variation [R2 = 0.22, P = 0.001, df = 6, constrained proportion (cp) = 0.37; Table 3], while nutrients (R2 = 0.09, P = 0.001, df = 4, cp = 0.19) and major ions (R2 = 0.08, P = 0.001, df = 3, cp = 0.15) explained the least. Models incorporating standard field parameters (R2 = 0.15, P = 0.001, df = 5, cp = 0.25) and organic matter (R2 = 0.16, P = 0.001, df = 5, cp = 0.35) were intermediate in explaining bacterial community composition.
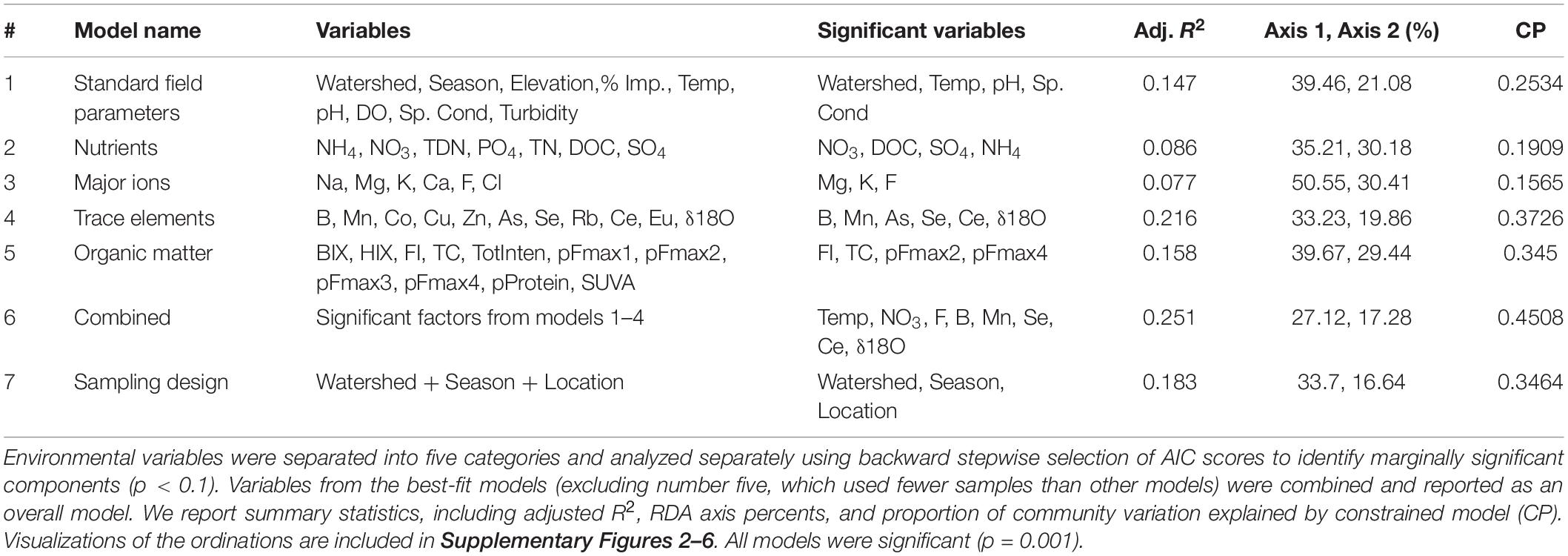
Table 3. Redundancy analysis (RDA) model results indicating the stream physiochemical variables structuring bacterial communities in streams across three watersheds (Logan, Red Butte, and Provo) and three seasons (Fall, Winter, and Spring).
The combined RDA model, incorporating significant variables from models one through four, included B, Ce, F, Mn, Se, NO3–-N, temperature, and δ18O, and explained more community variation than any other RDA (adj. R2 = 0.25, P = 0.001, df = 8, cp = 0.45; Table 3). Positive associations (represented by arrows indicating direction and magnitude of correlation between environmental factors and communities) occurred between high elevation Provo River bacterial taxa and Ce concentration, Red Butte Creek bacteria exiting the reservoir with Mn, and Logan stream bacteria, Se concentration, and temperature (Figure 3). Boron, F, and δ18O corresponded most with bacterial communities in Dam and Urban bacterioplankton communities in Red Butte and Provo watersheds. The parameters from the combined model explained 33% of the bacterial community variability (variation partition analysis, Supplementary Table 1). For comparison, the sampling design (Watershed + Location + Season) explained 43% of variation in the bacterial community.
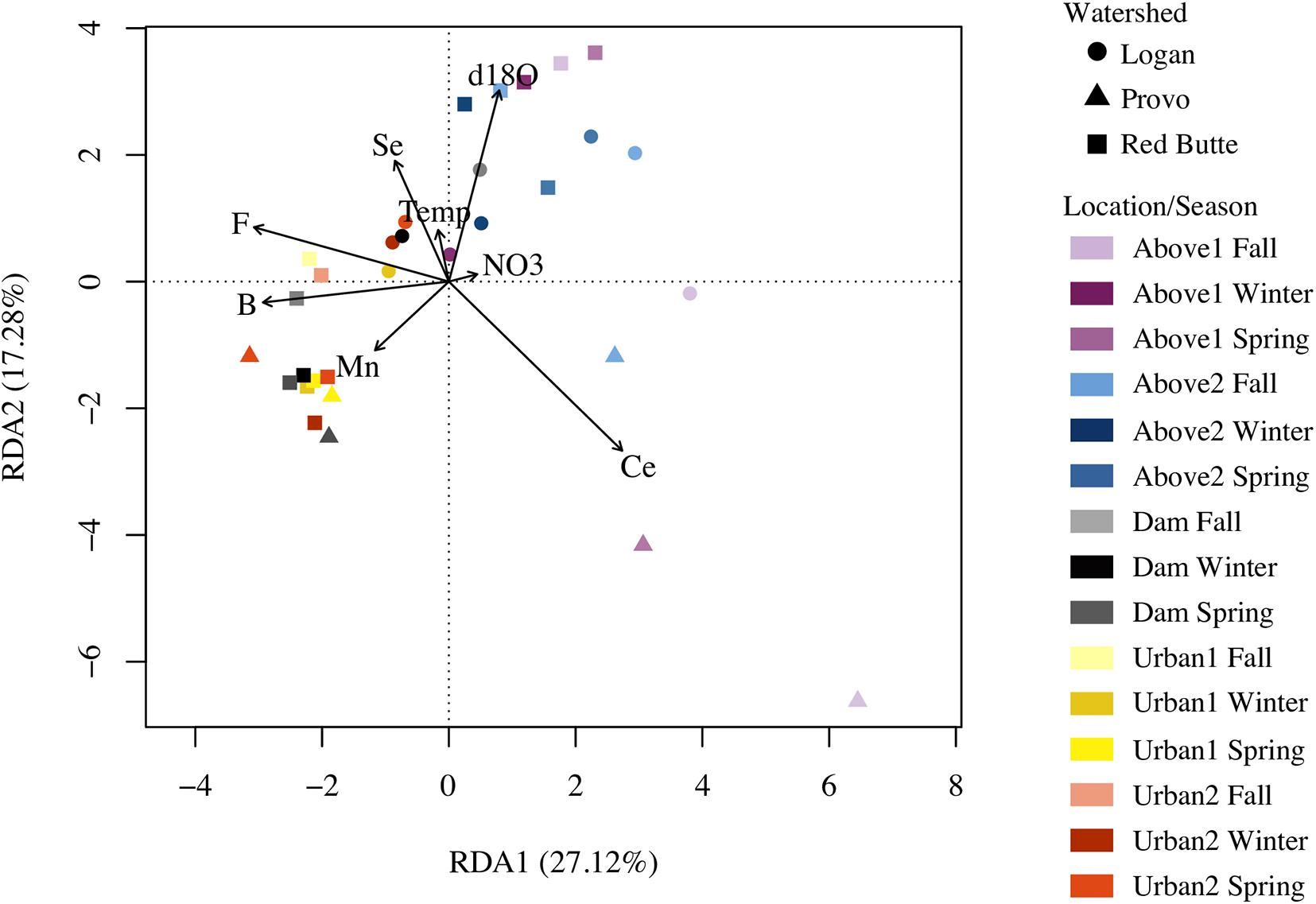
Figure 3. Redundancy analysis (RDA) plot relating stream environmental variables and bacterial communities in streams in three Utah watersheds (Red Butte Creek, Provo River, and Logan River) across three seasons (Fall, Winter, and Spring). Vectors are significant environmental factors, indicating positive correlation. Location indicates position relative to man-made reservoirs and urban centers. Variables were selected from the combination of four RDA models of standard field parameters, nutrients, major ions, and trace elements (Table 3).
Trace elements and the combined model correlated with the most amount of change in bacterioplankton community in the variation partitioning analysis (adj. R2 = 0.41 and 0.32; Table 4). Trace elements and the combined model had essentially no overlap in explanatory power between the environmental and spatial matrices (adj. R2 = −0.01). The overlap between variability explained by environmental and spatial factors was highest for standard field parameters, nutrients, and major ions (adj. R2 = 13–17%). Major ions explained no change in community composition that was not accounted for by the spatial matrix (adj. R2 = 0). Residual variability accounted for 48–80% of the community composition, indicating unaccounted for directional and stochastic processes.
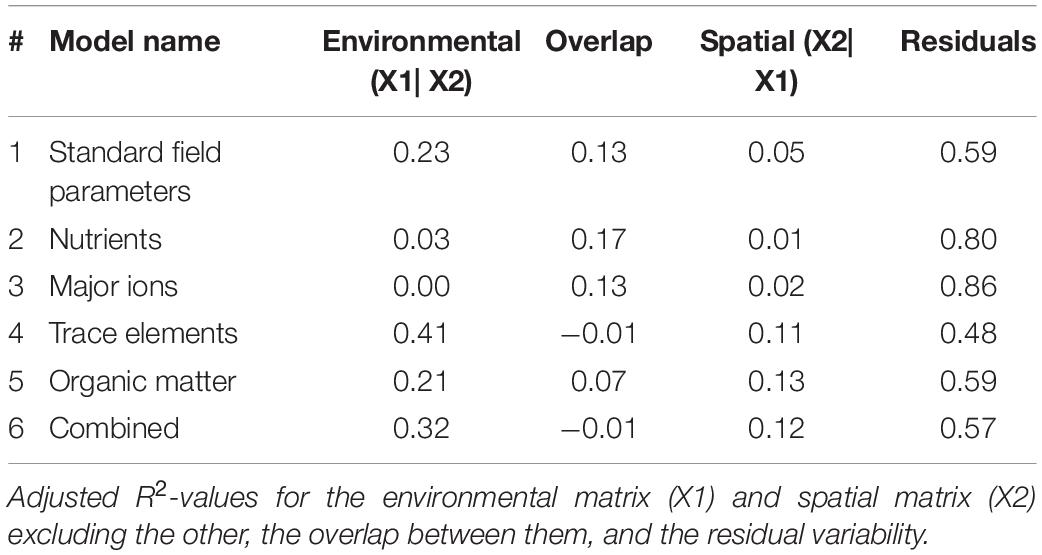
Table 4. Variation partitioning of bacterioplankton community by environmental variables and spatial data shows trace elements explain more changes in composition than any other environmental or spatial factors.
Variables used in the combined model (model 6) highlight the biogeochemical differences among watersheds and in urban environments (Figure 4). All parameters from the combined model except NO3– and temperature differed by watershed, regardless of season and location (ANOVA, P < 0.05). Red Butte had the highest concentrations of B and Se. Some solutes increased between montane and urban sites (B, F), while only Ce decreased from upstream to downstream (TukeyHSD, P-adj. < 0.05). Boron, F, Ce, Mn, and temperature differed by location regardless of watershed (ANOVA, P < 0.05, df = 4) and also had significant changes due to the interaction of watershed and season (ANOVA, P < 0.05, df = 4). Cesium, Mn, and temperature were the only parameters in model 6 that varied with season alone (ANOVA, P < 0.05, df = 2).
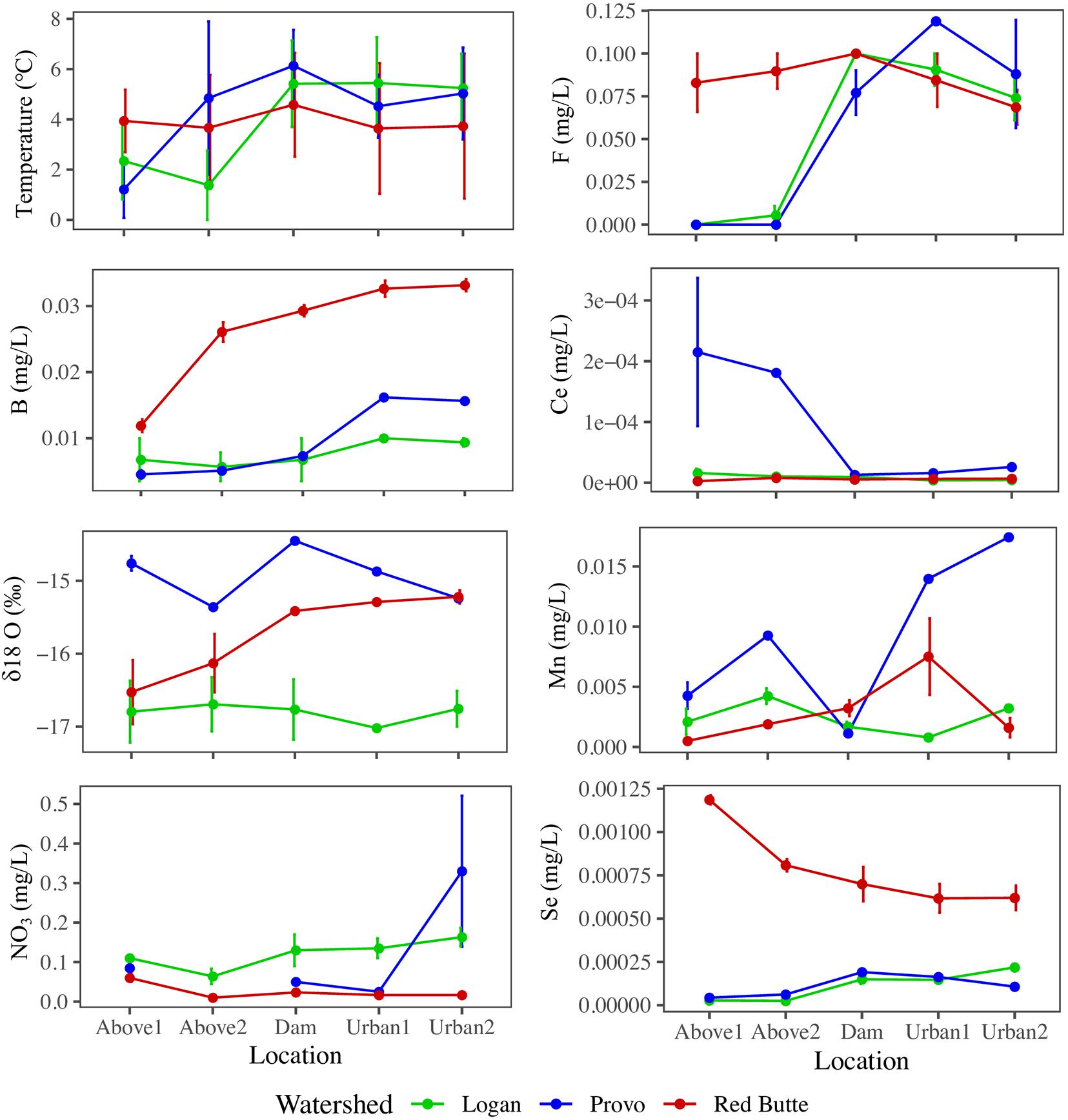
Figure 4. Longitudinal profiles of stream physiochemical variables selected by backward stepwise RDA ordination of bacterial communities in streams in three Utah watersheds (Red Butte Creek, Provo River, and Logan River) across three seasons (Fall, Winter, and Spring). Location indicates position relative to reservoirs and urban centers. Means and standard errors, where determinable, are shown (some data points were removed for QA/QC violations).
Bacterioplankton Community Dynamics Along the Stream Continuum
Bacterioplankton communities upstream of reservoirs were similar across all watersheds and seasons, despite large geographic distances and unique environmental conditions (Figure 5). Communities from the Logan watershed and upstream locations (Above1 and 2) in the Provo and Red Butte watersheds clustered together on the PCoA, indicating similar community compositions, but Dam sites in Provo and Red Butte watersheds, where dams increased water residence times by 2–4 orders of magnitude, were markedly unique. Downstream locations in the Red Butte watershed (Dam, Urban1 and 2) had a clear pattern of both location and season. Provo communities, where residence times in dams were longest, shifted further along Axis1 at Dam sites than Red Butte communities, then gradually moved back toward the Above and Logan watershed cluster downstream. Dam locations in Provo and Red Butte watersheds were most similar to the upstream community in May, when flows were highest and residence times lowest. The Logan watershed, despite covering the most stream miles (Table 1), was the most similar in community composition between sites.
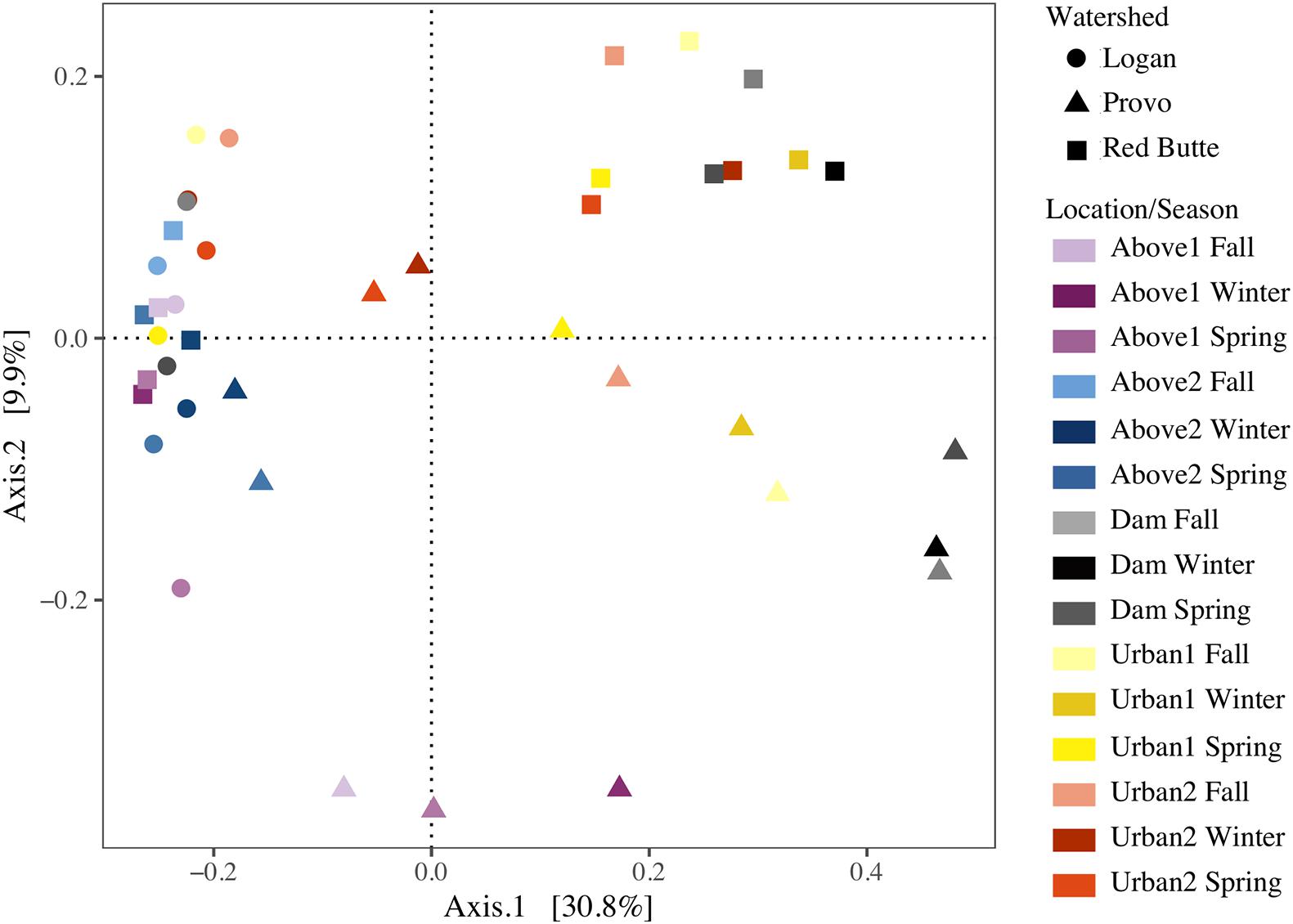
Figure 5. Reservoirs alter stream bacterial community composition from three watersheds in Utah’s Wasatch Range Metropolitan Area (Red Butte Creek, Provo River, and Logan River) across three seasons (Fall, Winter, and Spring). Graph represents a Principal Coordinate Analysis (PCOA) ordination, with each point representing a community of bacterioplankton classified at the 97% similarity from 16s rRNA gene amplicon sequencing of OTUs. Location indicates position relative to man-made reservoirs and urban centers. Points closer together are more similar (based on Bray-Curtis dissimilarity index), while points farther apart are dissimilar.
The effect of watershed and location relative to season in driving bacterioplankton community composition was confirmed by PERMANOVA and pairwise post hoc PERMANOVA tests. The differences in community composition observed between groups in the PCoA ordination were significant for all three main effects (Watershed, Season, and Location), as well as the interactions of watershed with season and location (PERMANOVA, Supplementary Table 2, P = 0.001). The R2-values for watershed, location, and their interaction were each around 21%, while the R2 values for seasonal changes were much lower, around 8%. Logan watershed was the least similar to Provo and Red Butte (22 and 18%), according to pairwise PERMANOVA tests (Table 5). All watersheds were different from each other, regardless of season and/or location (i.e., p-values did not change when either were added as strata to the command model). The PERMANOVA test was unable to differentiate seasons, although controlling for location or watershed made the difference between Spring and Winter significant.
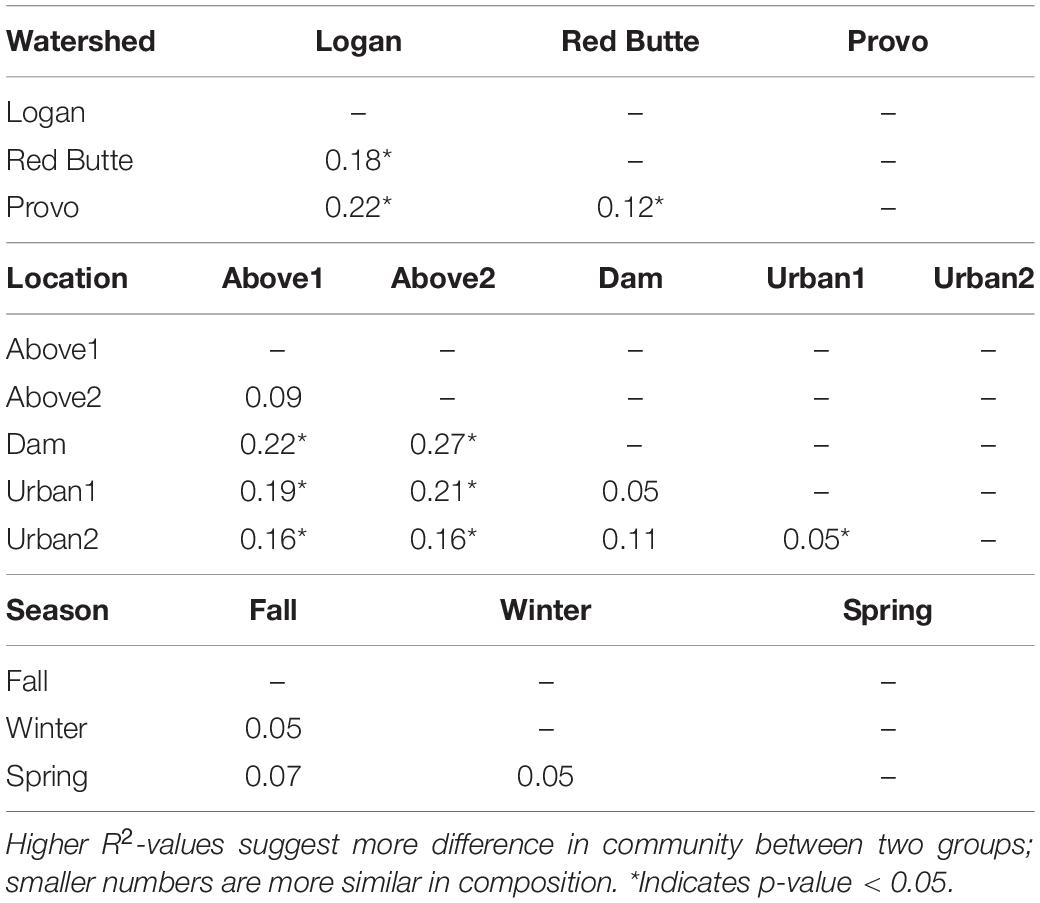
Table 5. Pairwise PERMANOVA results comparing bacterioplankton community composition across three watersheds, at five locations, and three seasons.
Both richness and diversity gradually decreased downstream in the Logan watershed, dropping by 18.7% (richness) and 8.7% (Shannon index) from upstream into the urban environment (Figure 6). Richness and diversity in Provo and Red Butte watersheds decreased slightly between Above1 and Above2 locations, then strikingly dropped at the Dam location. Diversity and richness then gradually increased downstream of the dam, despite moving into an urban environment, but remained slightly less diverse than the upstream communities. Richness and diversity were 25 and 11% higher for samples collected in Spring than Fall or Winter (TukeyHSD, adj. P < 0.05).
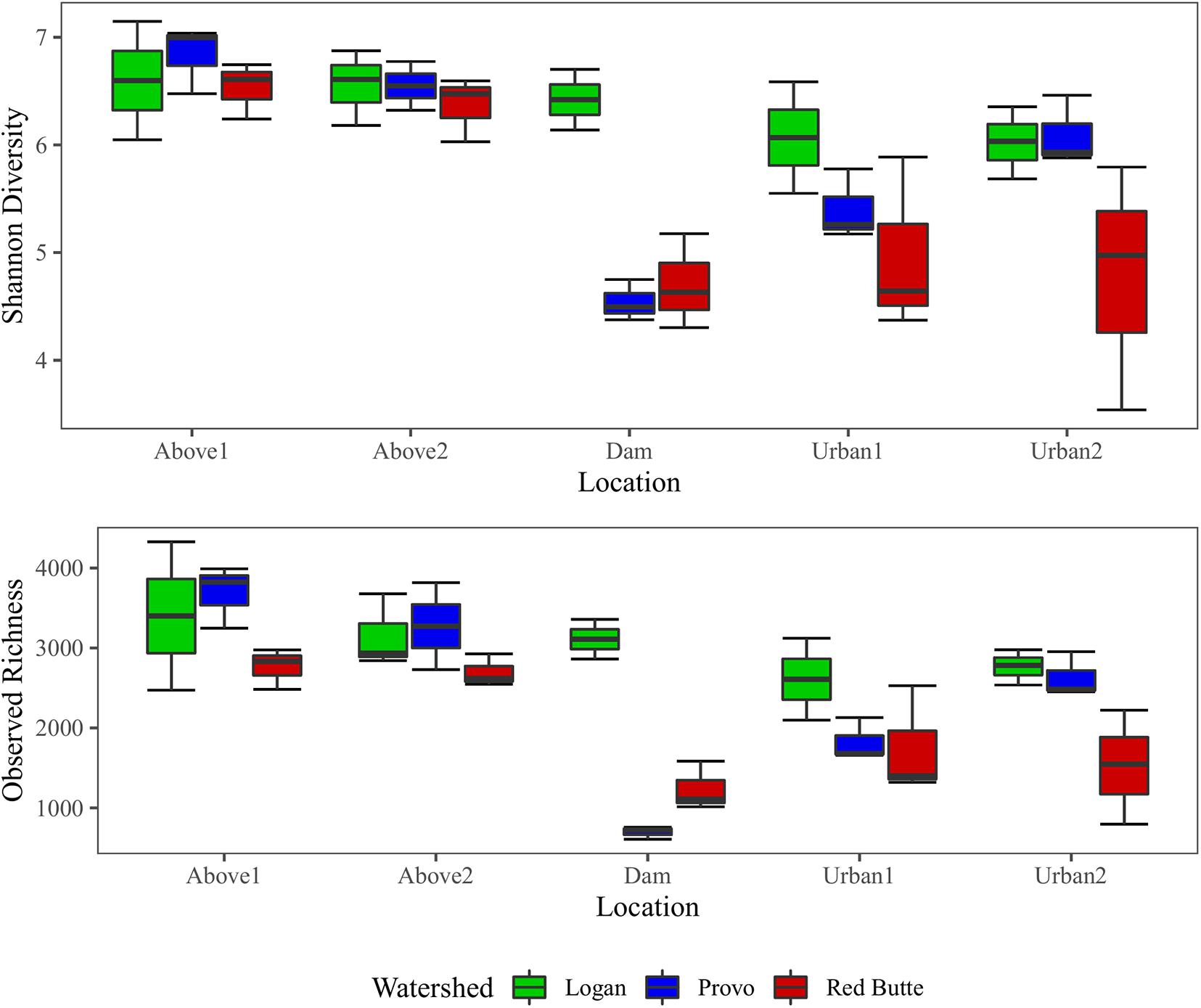
Figure 6. Observed richness and Shannon diversity of bacterial communities for three watersheds over three seasons in Wasatch Range Metropolitan Area (WRMA). Boxes depict interquartile ranges, with the center line on the median and whiskers showing the extent of values.
Human Influence on Bacterioplankton Communities
Large reservoirs had substantial effects on bacterioplankton richness, diversity, and community composition. Both species richness and diversity were highest in the Logan watershed, where reservoirs are shallow with much lower residence times (TukeyHSD, adj. P < 0.05). In the other two watersheds, where reservoir residence time was higher, bacterial diversity decreased by 25% and taxa richness by 67% from upstream to downstream of large reservoirs. Location was selected as a component of the RDA model (Table 3), and in the pairwise PERMANOVA tests the Above2 and Dam locations had the most dissimilarity of any comparison in bacterioplankton communities between main effects (27%, Table 5).
Development on the landscape was comparatively less influential on bacterioplankton richness, diversity, and community composition. Richness and diversity increased downstream of dams in Provo and Red Butte watersheds, despite moving into an urban environment, but remained slightly less diverse than the headwater community (Figure 6). Percent developed land use was not included by the step-wise RDA model selection (Table 3). Despite being the most developed and in different ways (densely urban versus mostly agricultural), Red Butte and Provo watersheds were the most similar (Table 5). Dam, Urban1 and Urban2 locations were the most similar, and differences between them were not significant regardless of whether comparisons were corrected for watershed and season (data not shown).
The community network structure was unique for each of the three watersheds. Logan watershed communities had the highest co-occurrence network complexity, with 3–4 times as many nodes as the other two watersheds (Figure 7 and Table 6). Logan and Provo watersheds had similar modularities (the degree to which clustering occurs, 0.856 and 0.767), mean path lengths (the average number of steps to connect each node, 6.739 and 6.029), and mean degrees (average number of edges for each node, 5.533 and 6.662). Red Butte watershed had nearly as many edges as Logan, despite having much fewer nodes; as a result, Red Butte had a much more tightly clustered network, with over 5 times as high density (0.074), 3 times as high degree (19.83) and 0.5 times as high modularity (0.397) as the other two watersheds.
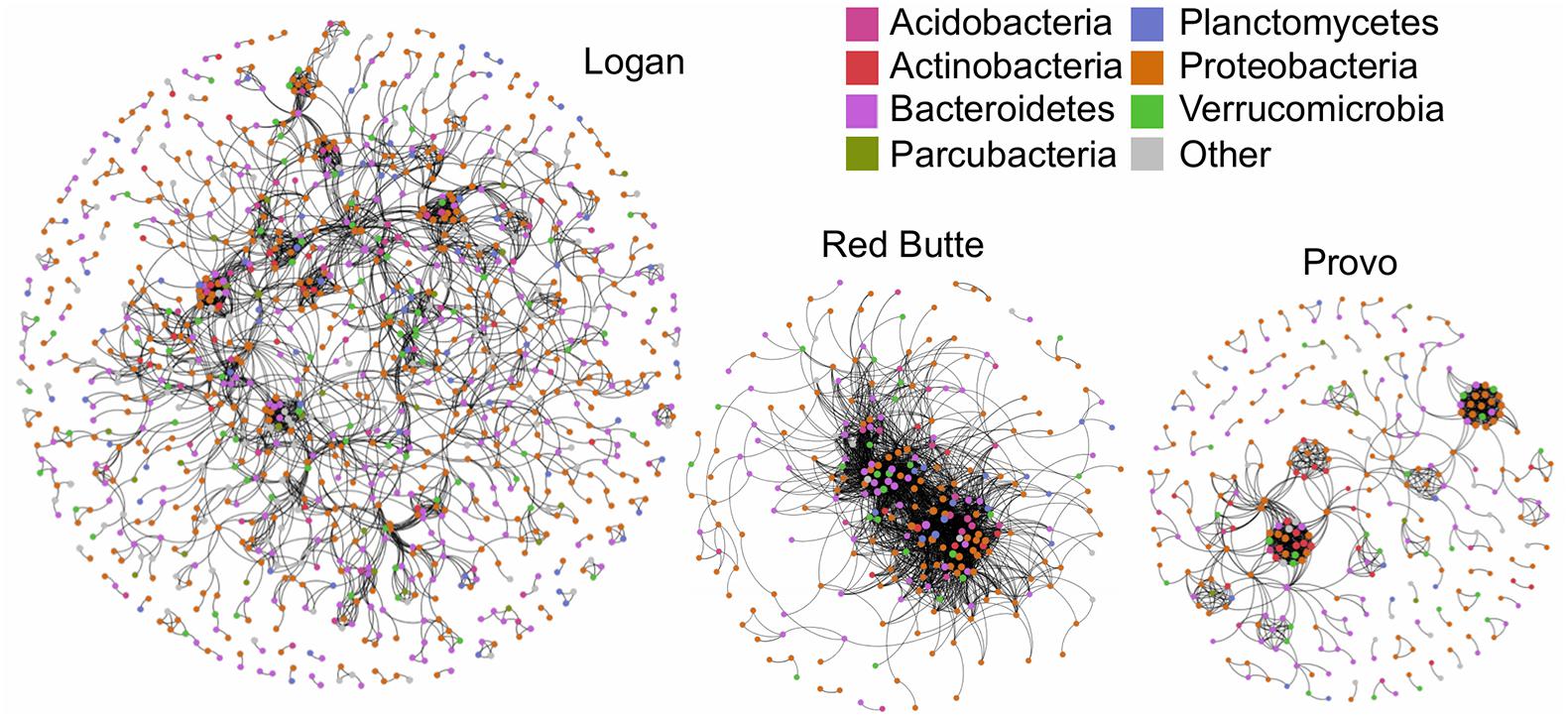
Figure 7. Network co-occurrence models for bacterial communities collected along elevation and urbanization gradients in three Utah watersheds (Red Butte Creek, Provo River, and Logan River) across three seasons (Fall, Winter, and Spring). Nodes indicate taxa (OTUs, taxa with 97% similar sequences) and edges connect where significant co-occurrence was detected in 75% of samples. Topologic statistics of the network are shown in Table 6.
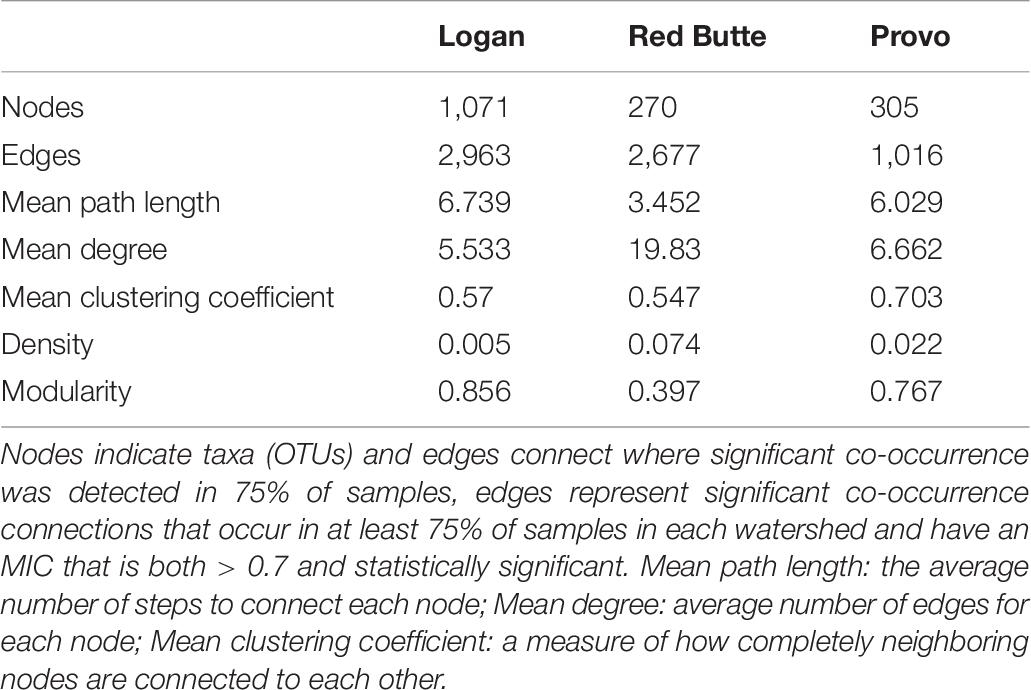
Table 6. Topological metrics calculated for network co-occurrence models of bacterial communities collected at five locations along an elevational gradient in three watersheds (Red Butte Creek, Provo River, and Logan River) across three seasons (Fall, Winter, and Spring).
Relative bacterial taxa abundance had unique distribution patterns in each watershed, correlating with changes in residence time (large reservoirs) more than changes in catchment land use. The ANCOM test (displayed using an abundance heatmap in Figure 8) returned taxa unique across watershed, location, and season. Similarities of taxa grouped by family expression patterns (shown by dendrogram on x-axis) matched the clusters in the PCoA ordination. Sporichthyaceae (Actinobacteria) was an important component of communities at Dam and Urban sites in Red Butte and Provo watersheds (16.1 ± 6.9%), while absent from Logan watershed and Above locations (1.1 ± 3.1%). Cryomorphaceae (Bacteroidetes) were also enriched directly below reservoirs, with 3.8 ± 2.7% in Red Butte and Provo Dam sites, and only 0.3 ± 0.1% in Logan and Above sites. Red Butte watershed had higher relative abundance of Acidimicrobiales (Actinobacteria) in February (0.57 ± 0.07%), while Provo communities in February were enriched with methanotrophic Methylococcaceae (Gammaproteobacteria, 1.4 ± 0.01%). Logan watershed and Above locations had higher densities of Cellvibrionales (Gammaproteobacteria), including over six times the relative abundance of Halieaceae (0.88 ± 0.56%) and three times the relative abundance of Cellvibrionaceae (0.77 ± 0.59%). The most abundant taxa, the aerobic, motile Comamonadaceae (Betaproteobacteria; Willems, 2014), was present in all samples at relative abundances between 5.5 and 53.4% (mean of 13.3%), but was removed by the ANCOM test because it was not differentially abundant between watershed, season, or location.
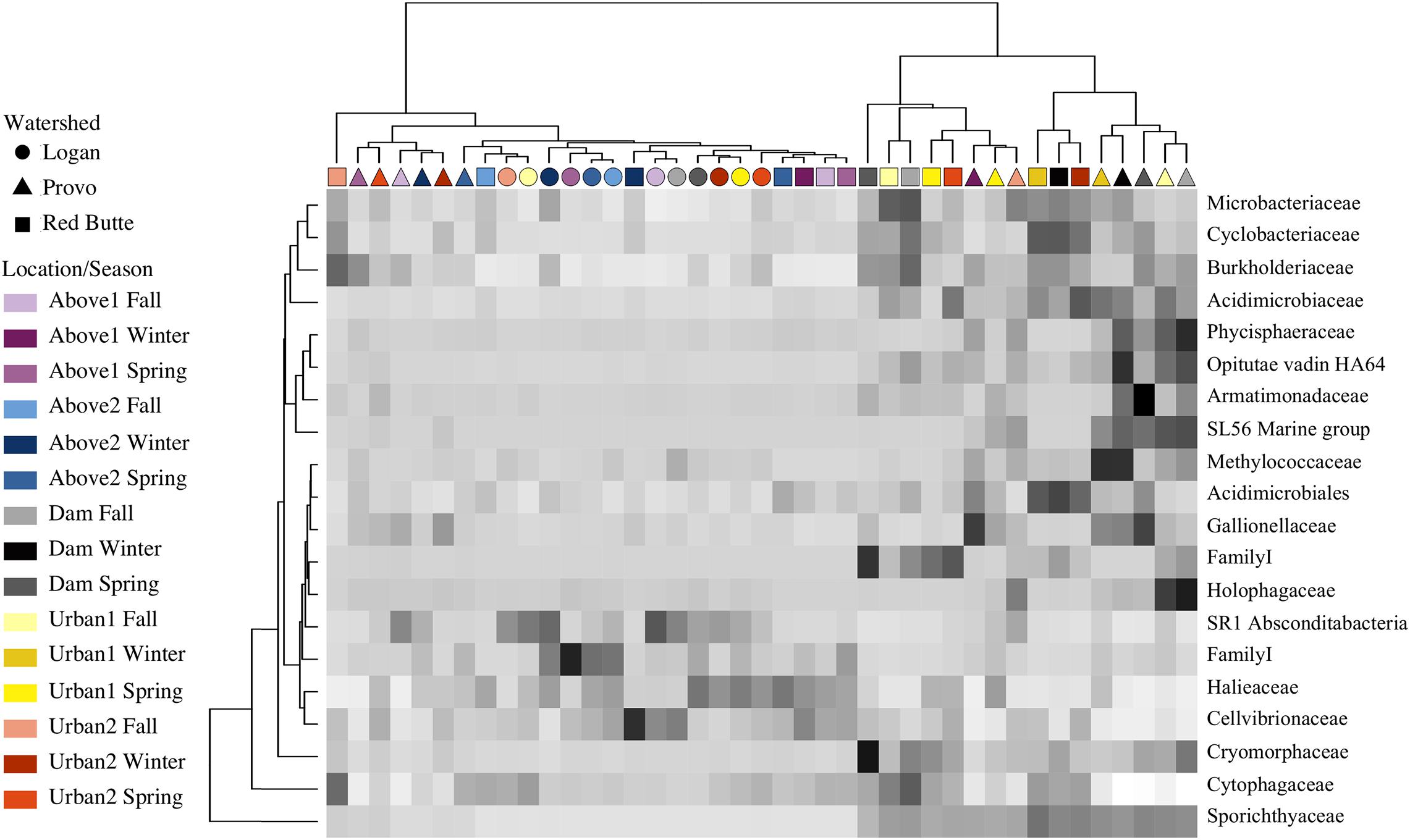
Figure 8. Heatmap showing ANCOM results of differentially abundant family for sites in three watersheds across three seasons (Fall, Winter, and Spring). Taxa were selected using a family relative abundance at least 0.5% for all samples. Location indicates position within watershed relative to man-made reservoirs, with Dam sites located immediately downstream of reservoir outlets (Table 1). Darker squares indicate higher relative abundance for bacterial families in each sample. Similarity between samples and taxa is indicated by dendrograms on each axis.
Discussion
Environmental Drivers of Bacterioplankton Communities
Contrary to our hypothesis, trace element concentrations, and not standard field parameters like pH and dissolved oxygen, were best correlated with bacterioplankton communities. This finding is contrary to observations in soil bacterial communities and longitudinal riverine studies on the Amazon and Mississippi Rivers (Doherty et al., 2017; Henson et al., 2017). However, a recent meta-analysis of stream bacteria compositions found that trace metals, when included in ordinations, always correlated with community composition (Zeglin, 2015). Many studies that report the importance of standard chemical and physical parameters do not include trace elements in their analyses, possibly overestimating the bacterial variability correlated to the standard variables and failing to quantify the role of other unmeasured parameters.
Trace elements may capture the variability among bacterial communities due to unique variations in geology, groundwater, and resultant stream chemistry better than the relatively narrow range of conditions in pH (7.24–8.5), dissolved oxygen (8.4–14.7 mg O2/L), and temperature (0–9.5°C) in these streams. The correlation between bacterial community composition and δ18O was 19%, which indicates the importance of the source of water (age indicated by enrichment of δ18O either in groundwater or within reservoirs). Other solutes in the combined model (F, B, Mn, Se, and Ce) may indicate natural weathering of rocks within groundwater as well as urban pollution (Buszka et al., 2007), but either mechanism ties bacterioplankton community composition with the ultimate source of stream water.
Statistical testing of the 53 variables returned many differences between the watersheds, locations and seasons (data not shown), but in some cases these differences failed to impact bacterial community composition, while variables with no statistical difference did affect composition. For example, nitrate concentrations were statistically indistinguishable between any factor of watershed, season or location, but correlated to 10% of bacterioplankton community variation (variation partitioning analysis of significant RDA Model 2), demonstrating that ecological significance may occur where statistical significance does not. Trace elements are no longer included in many water quality studies because their concentration is usually in a narrow range below toxicity levels for aquatic organisms at higher trophic levels, but the slight differences in concentration might be high enough to differentiate the multitude of unique microbial taxa (Herrmann et al., 2016; Smedley and Kinniburgh, 2017).
Our highest variation partitioning adj. R2-value was 52% (model 4, sum of environmental and spatial factors), meaning we were unable to attribute half of community composition to any factor, which is similar to R2-values reported in a meta-analysis of 22 environmental microbiology studies (Hanson et al., 2012). Stochasticity, including genetic drift (e.g., die-off, bacterivory) and mutation (Hanson et al., 2012; Evans et al., 2017) may contribute to differences in bacterioplankton community proportional to residence time, but we were unable to distinguish their relative impacts with this study design. Our correlation values may underestimate the impact of environmental variables and overestimate dispersal and stochastic mechanisms compared to benthic biofilm communities (Wang et al., 2017). Bacterial dormancy and horizontal gene transfer (Trevors et al., 1987; Jones and Lennon, 2010), priority effects (Evans et al., 2017), competition between organisms, and trophic interactions such as predation (Pernthaler, 2005), may also account for part of the 50% of community composition we were unable to associate with environmental conditions.
Bacterioplankton Community Dynamics Along the Stream Continuum
Our results appear to follow a pattern similar to other studies showing that the dominant dispersal pathway of stream bacterioplankton is via longitudinal transport from headwaters, where high lateral dispersal of bacteria from soil waters to streams helps form higher-diversity upstream communities that generally decrease in diversity downstream as environmental selection removes poorly adapted taxa (Crump et al., 2012; Besemer et al., 2013; Febria et al., 2015). Upstream of dams, diversity decreased with increasing stream distance as expected; however, the rapid decrease in diversity from above to below dams and then the gradual increase in diversity moving downstream demonstrated a deviation from typical patterns of bacterioplankton dispersal (Figure 6; Chen et al., 2018). The abrupt change in bacterioplankton community composition and diversity, presumably occurring within the reservoir, could be explained by a shift from dispersal-dominated community composition to a lower-diversity community shaped more by environmental selection. In reservoirs, longer residence times may separate bacterioplankton from both the longitudinal dispersal from upstream terrestrial soils (Lennon and Jones, 2011; Crump et al., 2012) and lateral dispersal from internal sources, such as lake sediments, that act as seed banks for microbial diversity (Comte et al., 2017). We observed a particularly atypical pattern along the stream continuum below reservoirs, with Provo and Red Butte community diversity increasing rather than decreasing downstream of dam sites. This downstream recovery in diversity could be caused by an increase in dispersal and decreased environmental selection as the bacterioplankton returned to a stream environment more influenced by dispersal and short residence times. Differences in stream-soil connectivity between these two watersheds may lend support to this idea; Red Butte Creek, which has a larger exposure ratio (surface area of bed:streamwater volume) than the Provo River, returned to the Above site PCoA cluster in much fewer stream kilometers than the Provo. Hydrologic variability, which is significantly decreased by static flows released from the reservoirs, may also play a role in structuring the bacterioplankton community indirectly through biofilm establishment (Widder et al., 2014).
Patterns in community similarity within and across watersheds could also support the idea that stream bacterioplankton communities are first dispersal-dominated and then increasingly influenced by environmental selection downstream. Most of the community similarity was attributed to watershed, possibly due to longitudinal transport of a common upstream-sourced community along the stream continuum. The lack of significant interaction between location and season (Supplementary Table 2) also indicates headwater-driven mass effects could play an important role in determining community composition, because an interaction would have indicated important contributions of unique local taxa based on changes in lateral sources and hydrologic connectivity through time. We expected to see a higher variability between communities due to seasonal changes in hydrologic conditions (Table 2), but a variation partitioning analysis of Model 7 determined only 7% of stream bacterial communities were associated with seasonal changes. Further, season was only significant in a pairwise PERMANOVA when controlled by watershed and/or location, suggesting that seasonal changes in residence time or lateral connectivity are less significant than expected (due to small and/or redundant contribution of bacterial taxa), unlike other stream bacterial communities that displayed strong seasonal changes (Leff et al., 1998; Yannarell et al., 2003; Crump and Hobbie, 2005; Zeglin, 2015; Niño-García et al., 2017).
Human Influence on Bacterioplankton Communities
Developed land use, as indicated by percent impervious surface, was not associated with changes in bacterioplankton communities (Table 3, % Imp), unlike previous studies identifying correlations between the two (Van Rossum et al., 2015; Chen et al., 2018). However, bacterial community composition was strongly affected by reservoirs, as demonstrated by large changes in communities where reservoir residence time was high in both Red Butte and Provo watersheds (Table 2 and Figure 5). Moving into the urban environment, communities became more similar to the Above locations, suggesting that either (1) high lateral dispersal occurred from downstream soil communities, which were not strongly affected by development in these watersheds, or (2) changes in environmental conditions promoted taxa being transported from above the reservoir to once again increase in abundance. The community network topology had watershed-specific responses that may have been due to differences in residence time: Red Butte watershed maintained a consistent, highly connected core community while in Provo, taxa were less connected and may be comprised of more generalist taxa functioning independently (Figure 7). The network topology may be an artifact of the shorter stream distance and catchment area in the Red Butte watershed, which affected clustering of co-occurrence networks in an Austrian catchment (Widder et al., 2014). The reduced network may also suggest higher rates of hydrologic disturbance (Widder et al., 2014) or species sorting (Ren et al., 2019) and imply changes in community function between watersheds (Fuhrman, 2009). The negligible effect of changes in land use at higher order streams suggests instream bacterial communities may not always be dramatically affected by land use changes or stream channelization in urban areas.
Many environmental factors are affected by reservoirs that might be responsible for the change in bacterioplankton community below reservoirs (Lindström et al., 2005; Comte et al., 2017). Drastic changes in bacterial communities are reported in artificial water systems (e.g., stormwater outfall, sewage, and 9-km long drinking water delivery pipe), but these changes were mostly attributed to biofilm development within pipes (Fisher et al., 2015; Van Rossum et al., 2015). Another potential factor is the removal of the suspended sediment load and particle-associated bacteria. As water slows, sediment drops from the water column and removes a subset of the overall taxa that might be dispersing attached to soil particles in swift currents (Atkinson et al., 1992; Doherty et al., 2017). However, turbidity was not a significant factor in the RDA selection, suggesting that this mechanism was not responsible for the changes observed (Table 3).
Large reservoirs may also impact bacterioplankton communities by altering substrate availability (Ruiz-González et al., 2015). Carbon substrate quality changes depending on source, and large, deep lakes have very different carbon sources and cycles than shallow streams. As more labile carbon is metabolized in reservoirs, DOC levels increase. DOC concentrations correlated in an RDA ordination with the sites downstream of reservoirs (Supplementary Figure 2), suggesting that the carbon substrate availability was related to changes in the bacterial community (Barberán and Casamayor, 2011). BIX values, indicating an increase in autochthonous production, also correlated with sites below reservoirs (Supplementary Figure 5). Cryomorphaceae, which increased in relative abundance below reservoirs, are chemoorganotrophs that are thought to metabolize these simple organic compounds (Bowman, 2014a). Oxygen is another metabolic component that likely controlled changes in taxa with increased residence time in reservoirs. Unfortunately, because outflow from reservoirs was quickly re-aerated, the oxygen levels measured were not representative of the environment where the community was formed, and our models did not detect correlations between community composition and oxygen concentrations (Figure 3). For example, samples collected below the larger reservoirs had substantially higher relative abundance of the facultative anaerobe Sporichthyaceae (Tamura, 2014), suggesting the anoxic hypolimnion played a role in driving community change. Sulfur concentrations also correlated with below reservoir samples, possibly due to oxidation of hydrogen sulfide created by sulfur-reducing bacteria in reservoir sediments. Increased Methylococcaceae relative abundance in Provo reservoir outflows potentially indicates both methanogenesis and methanotrophy were also responsible for some of the changes in community composition (Bowman, 2014b). These changes in taxa may also correspond to an increase in mobilized heavy metals from reservoir sediments; for example, Acidimicrobiales in Red Butte outflow indicates iron-reduction and iron-oxidation may be increased within the reservoir (Clark and Norris, 1996; Itoh et al., 2011). Changes in bacterial taxa may relate to activation of alternative metabolic pathways, especially those rare metabolic processes for which functional redundancy may be low (Comte and del Giorgio, 2010; Peura et al., 2012; Adams et al., 2014).
Conclusion
We tested three predictions regarding bacterioplankton community composition along a longitudinal-urbanization gradient, including multiple seasons and anthropogenic alterations to in-stream and landscape processes to determine the role of environmental conditions, water residence time, and human infrastructure on stream bacterioplankton community composition. Trace element concentrations explained more variability in bacterioplankton community composition than other environmental parameters, and should be included in more analyses of aquatic microbial ecology. Our findings followed previously established patterns showing that bacterioplankton diversity tends to gradually decrease downstream as environmental selection acts on dispersal-dominated communities, except in cases where large reservoirs drastically changed water residence time. Reservoirs and lakes (anywhere residence time changes) should be included in longitudinal stream bacteria studies; understanding changes in residence time may provide important context for other studies of bacterioplankton biogeography. Large reservoirs may have more impact on bacterioplankton communities than other aspects of watershed urbanization. More research is needed to quantify the magnitude of the effect of components of Figure 1, such as anthropogenic infrastructure and changes in residence time, on stream bacterial communities (e.g., when does longer residence time have increase or decrease alpha and beta diversity, and what controls the extent of that effect?). Understanding the drivers of bacteria and other microbial communities in streams will allow improved predictions of how watershed and stream development will affect biogeochemical processes and resultant water quality.
Data Availability Statement
The datasets generated for this study can be found in the Hydroshare repository https://doi.org/10.4211/hs.c0a1bb39015444ef86ace38ae6c44e77.
Author Contributions
EJ, ZA, and MB designed the study. EJ, NG, JK, and GC collected and analyzed samples. All authors helped write and review the manuscript.
Funding
This research was supported by NSF EPSCoR grant EPS 1208732 awarded to Utah State University, as part of the State of Utah Research Infrastructure Improvement Award. Additional support was provided by Brigham Young University in collaboration with the iUTAH EPSCoR Program. Any opinions, findings, and conclusions or recommendations expressed are those of the author(s) and do not necessarily reflect the views of the National Science Foundation.
Conflict of Interest
The authors declare that the research was conducted in the absence of any commercial or financial relationships that could be construed as a potential conflict of interest.
Acknowledgments
The many iUTAH technicians and assistants who helped collect and analyze data, including Joe Crawford, Dave Eirikkson, Dylan Dastrup, David Robinson, Timothy Goodsell, Dane Cook, Ben Rider, Ka-Voka Tokespeta, Scott Collins, Chris Cox, and Amber Jones.
Supplementary Material
The Supplementary Material for this article can be found online at: https://www.frontiersin.org/articles/10.3389/fmicb.2020.491425/full#supplementary-material
Footnotes
References
Abbott, B. W., Baranov, V., Mendoza-Lera, C., Nikolakopoulou, M., Harjung, A., Kolbe, T., et al. (2016). Using multi-tracer inference to move beyond single-catchment ecohydrology. Earth Sci. Rev. 160, 19–42. doi: 10.1016/j.earscirev.2016.06.014
Abbott, B. W., Bishop, K., Zarnetske, J. P., Minaudo, C., Chapin, F. S., Krause, S., et al. (2019). Human domination of the global water cycle absent from depictions and perceptions. Nat. Geosci. 12, 533–540. doi: 10.1038/s41561-019-0374-y
Abbott, B. W., Moatar, F., Gauthier, O., Fovet, O., Antoine, V., and Ragueneau, O. (2018). Trends and seasonality of river nutrients in agricultural catchments: 18years of weekly citizen science in France. Sci. Total Environ. 624, 845–858. doi: 10.1016/j.scitotenv.2017.12.176
Adams, H. E., Crump, B. C., and Kling, G. W. (2014). Metacommunity dynamics of bacteria in an arctic lake: the impact of species sorting and mass effects on bacterial production and biogeography. Front. Microbiol. 5:82. doi: 10.3389/fmicb.2014.00082
Albright, M. B. N., and Martiny, J. B. H. (2018). Dispersal alters bacterial diversity and composition in a natural community. ISME J. 12, 296–299. doi: 10.1038/ismej.2017.161
Allison, S. D., and Martiny, J. B. (2008). Resistance, resilience, and redundancy in microbial communities. Proc. Natl. Acad. Sci. U.S.A. 105, 11512–11519. doi: 10.1073/pnas.0801925105
Atkinson, C. F., Aumen, N. G., Miller, G. L., and Ward, G. M. (1992). Influence of particle shapes and size distributions on fine particulate organic matter surface area in streams. J. North Am. Benthol. Soc. 11, 261–268. doi: 10.2307/1467646
Baker, A., Tipping, E., Thacker, S. A., and Gondar, D. (2008). Relating dissolved organic matter fluorescence and functional properties. Chemosphere 73, 1765–1772. doi: 10.1016/j.chemosphere.2008.09.018
Baker, M. A., Dahm, C. N., and Valett, H. M. (2000). “Anoxia, anaerobic metabolism biogeochemistry of the stream water- ground water interface,” in Streams and Ground Waters, eds J. B. Jones Jr. and P. J. Mulholland (San Diego, CA: Academic Press), 259–284. doi: 10.1016/b978-012389845-6/50012-0
Barberán, A., and Casamayor, E. O. (2011). Euxinic freshwater hypolimnia promote bacterial endemicity in continental areas. Microb. Ecol. 61, 465–472. doi: 10.1007/s00248-010-9775-6
Bastian, M., Heymann, S., and Jacomy, M. (2009). “Gephi: an open source software for exploring and manipulating networks,” Proceedings of the International AAAI Conference on Weblogs and Social Media, San Jose, CA.
Ben Maamar, S., Aquilina, L., Quaiser, A., Pauwels, H., Michon-Coudouel, S., Vergnaud-Ayraud, V., et al. (2015). Groundwater isolation governs chemistry and microbial community structure along hydrologic flowpaths. Front. Microbiol. 6:1457. doi: 10.3389/fmicb.2015.01457
Besemer, K., Singer, G., Quince, C., Bertuzzo, E., Sloan, W., and Battin, T. J. (2013). Headwaters are critical reservoirs of microbial diversity for fluvial networks. Proc. R. Soc. B Biol. Sci. 280:20131760. doi: 10.1098/rspb.2013.1760
Blaszczak, J. R., Delesantro, J. M., Urban, D. L., Doyle, M. W., and Bernhardt, E. S. (2019). Scoured or suffocated: urban stream ecosystems oscillate between hydrologic and dissolved oxygen extremes. Limnol. Oceanogr. 64, 877–894. doi: 10.1002/lno.11081
Bowman, J. P. (2014a). “The Family Cryomorphaceae,” in The Prokaryotes: Other Major Lineages of Bacteria and The Archaea, eds E. Rosenberg, E. F. DeLong, S. Lory, E. Stackebrandt, and F. Thompson (Berlin: Springer), 539–550. doi: 10.1007/978-3-642-38954-2_135
Bowman, J. P. (2014b). “The Family Methylococcaceae,” in The Prokaryotes: Gammaproteobacteria, eds E. Rosenberg, E. F. DeLong, S. Lory, E. Stackebrandt, and F. Thompson (Berlin: Springer), 411–440. doi: 10.1007/978-3-642-38922-1_237
Briggs, M. A., Lautz, L. K., Hare, D. K., and González-Pinzón, R. (2013). Relating hyporheic fluxes, residence times, and redox-sensitive biogeochemical processes upstream of beaver dams. Freshw. Sci. 32, 622–641. doi: 10.1899/12-110.1
Buszka, P. M., Fitzpatrick, J. A., Watson, L. R., and Kay, R. T. (2007). Evaluation of Ground-Water and Boron Sources by Use of Boron Stable-Isotope Ratios, Tritium, and Selected Water-Chemistry Constituents Near Beverly Shores, Northwestern Indiana, 2004. Reston, VA: U.S. Geological Survey.
Buttigieg, P. L., and Ramette, A. (2014). A guide to statistical analysis in microbial ecology: a community-focused, living review of multivariate data analyses. FEMS Microbiol. Ecol. 90, 543–550. doi: 10.1111/1574-6941.12437
Campbell, T. (2015). Tree Islands of Fertility Structure Bacterial Community Assembly and Functional Genes Contributing to Ecosystem Processes. All Theses and Dissertations. Available online at: https://scholarsarchive.byu.edu/etd/5241 (accessed February 27, 2017).
Caporaso, J. G., Lauber, C. L., Walters, W. A., Berg-Lyons, D., Lozupone, C. A., Turnbaugh, P. J., et al. (2011). Global patterns of 16S rRNA diversity at a depth of millions of sequences per sample. Proc. Natl. Acad. Sci. U.S.A. 108, 4516–4522. doi: 10.1073/pnas.1000080107
Carling, G. T., Tingey, D. G., Fernandez, D. P., Nelson, S. T., Aanderud, Z. T., Goodsell, T. H., et al. (2015). Evaluating natural and anthropogenic trace element inputs along an alpine to urban gradient in the Provo River, Utah, USA. Appl. Geochem. 63, 398–412. doi: 10.1016/j.apgeochem.2015.10.005
Chen, J., Wang, P., Wang, C., Wang, X., Miao, L., Liu, S., et al. (2020). Distinct assembly mechanisms underlie similar biogeographic patterns of rare and abundant Bacterioplankton in Cascade Reservoirs of a Large River. Front. Microbiol. 11:158. doi: 10.3389/fmicb.2020.00158
Chen, W., Wilkes, G., Khan, I. U. H., Pintar, K. D. M., Thomas, J. L., Lévesque, C. A., et al. (2018). Aquatic bacterial communities associated with land use and environmental factors in agricultural landscapes using a metabarcoding approach. Front. Microbiol. 9:2301. doi: 10.3389/fmicb.2018.02301
Clark, D. A., and Norris, P. R. (1996). Acidimicrobium ferrooxidans gen. nov., sp. nov.: mixed-culture ferrous iron oxidation with Sulfobacillus species. Microbiology 142, 785–790. doi: 10.1099/00221287-142-4-785
Comte, J., Berga, M., Severin, I., Logue, J. B., and Lindström, E. S. (2017). Contribution of different bacterial dispersal sources to lakes: Population and community effects in different seasons: bacterial dispersal in lakes. Environ. Microbiol. 19, 2391–2404. doi: 10.1111/1462-2920.13749
Comte, J., and del Giorgio, P. A. (2010). Linking the patterns of change in composition and function in bacterioplankton successions along environmental gradients. Ecology 91, 1466–1476. doi: 10.1890/09-0848.1
Covino, T. (2017). Hydrologic connectivity as a framework for understanding biogeochemical flux through watersheds and along fluvial networks. Geomorphology 277, 133–144. doi: 10.1016/j.geomorph.2016.09.030
Crump, B. C., Adams, H. E., Hobbie, J. E., and Kling, G. W. (2007). Biogeography of Bacterioplankton in Lakes and Streams of an Arctic Tundra Catchment. Ecology 88, 1365–1378. doi: 10.1890/06-0387
Crump, B. C., Amaral-Zettler, L. A., and Kling, G. W. (2012). Microbial diversity in arctic freshwaters is structured by inoculation of microbes from soils. ISME J. 6, 1629–1639. doi: 10.1038/ismej.2012.9
Crump, B. C., and Hobbie, J. E. (2005). Synchrony and seasonality in bacterioplankton communities of two temperate rivers. Limnol. Oceanogr. 50, 1718–1729. doi: 10.4319/lo.2005.50.6.1718
Dahlke, H. E., Easton, Z. M., Lyon, S. W., Walter, M. T., Destouni, G., and Steenhuis, T. S. (2012). Dissecting the variable source area concept–subsurface flow pathways and water mixing processes in a hillslope. J. Hydrol. 420, 125–141. doi: 10.1016/j.jhydrol.2011.11.052
Doherty, M., Yager, P. L., Moran, M. A., Coles, V. J., Fortunato, C. S., Krusche, A. V., et al. (2017). Bacterial biogeography across the amazon river-ocean continuum. Front. Microbiol. 8:882. doi: 10.3389/fmicb.2017.00882
Döll, P., Fiedler, K., and Zhang, J. (2009). Global-scale analysis of river flow alterations due to water withdrawals and reservoirs. Hydrol. Earth Syst. Sci. 13, 2413–2432. doi: 10.5194/hess-13-2413-2009
Donlan, R. M. (2002). Biofilms: microbial life on surfaces. Emerg. Infect. Dis. 8, 881–890. doi: 10.3201/eid0809.020063
Duff, J. H., and Triska, F. J. (2000). “Nitrogen biogeochemistry and surface-subsurface exchange in streams,” in Streams and Ground Waters, eds J. B. Jones and P. J. Mulholland (San Diego, CA: Academic Press).
Ehleringer, J. R., Arnow, L. A., Arnow, T., McNulty, I. B., McNulty, I. R., and Negus, N. C. (1992). Red butte canyon research natural area: history, flora, geology, climate, and ecology. Great Basin Nat. 52, 95–121. doi: 10.2307/41712704
Evans, S., Martiny, J. B. H., and Allison, S. D. (2017). Effects of dispersal and selection on stochastic assembly in microbial communities. ISME J. 11, 176–185. doi: 10.1038/ismej.2016.96
Febria, C. M., Hosen, J. D., Crump, B. C., Palmer, M. A., and Williams, D. D. (2015). Microbial responses to changes in flow status in temporary headwater streams: a cross-system comparison. Front. Microbiol. 6:522. doi: 10.3389/fmicb.2015.00522
Fierer, N., and Jackson, R. B. (2006). The diversity and biogeography of soil bacterial communities. Proc. Natl. Acad. Sci. U.S.A. 103, 626–631. doi: 10.1073/pnas.0507535103
Fierer, N., and Lennon, J. T. (2011). The generation and maintenance of diversity in microbial communities. Am. J. Bot. 98, 439–448. doi: 10.3732/ajb.1000498
Fierer, N., Morse, J. L., Berthrong, S. T., Bernhardt, E. S., and Jackson, R. B. (2007). Environmental controls on the landscape-scale biogeography of stream bacterial communities. Ecology 88, 2162–2173. doi: 10.1890/06-1746.1
Filosi, M., Visintainer, R., Riccadonna, S., Jurman, G., and Furlanello, C. (2014). Stability indicators in network reconstruction. PLoS One 9:e89815. doi: 10.1371/journal.pone.0089815
Findlay, S. (2010). Stream microbial ecology. J. North Am. Benthol. Soc. 29, 170–181. doi: 10.1899/09-023.1
Fisher, J. C., Newton, R. J., Dila, D. K., and McLellan, S. L. (2015). Urban microbial ecology of a freshwater estuary of Lake Michigan. Elementa 3:000064. doi: 10.12952/journal.elementa.000064
Follstad Shah, J. J., Jameel, Y., Smith, R. M., Gabor, R. S., Brooks, P. D., and Weintraub, S. R. (2019). Spatiotemporal variability in water sources controls chemical and physical properties of a semi-arid urban river system. JAWRA J. Am. Water Resour. Assoc. 55, 591–607. doi: 10.1111/1752-1688.12734
Fuhrman, J. A. (2009). Microbial community structure and its functional implications. Nature 459, 193–199. doi: 10.1038/nature08058
Gabor, R. S., Eilers, K., McKnight, D. M., Fierer, N., and Anderson, S. P. (2014). From the litter layer to the saprolite: chemical changes in water-soluble soil organic matter and their correlation to microbial community composition. Soil Biol. Biochem. 68, 166–176. doi: 10.1016/j.soilbio.2013.09.029
Goodsell, T. H., Carling, G. T., Aanderud, Z. T., Nelson, S. T., Fernandez, D. P., and Tingey, D. G. (2017). Thermal groundwater contributions of arsenic and other trace elements to the middle Provo River, Utah, USA. Environ. Earth Sci. 76:268. doi: 10.1007/s12665-017-6594-9
Grill, G., Lehner, B., Thieme, M., Geenen, B., Tickner, D., Antonelli, F., et al. (2019). Mapping the world’s free-flowing rivers. Nature 569, 215–221. doi: 10.1038/s41586-019-1111-9
Grimm, N. B., Faeth, S. H., Golubiewski, N. E., Redman, C. L., Wu, J., Bai, X., et al. (2008). Global change and the ecology of cities. Science 319, 756–760. doi: 10.1126/science.1150195
Hale, R. L., Turnbull, L., Earl, S. R., Childers, D. L., and Grimm, N. B. (2015). Stormwater infrastructure controls runoff and dissolved material export from arid urban watersheds. Ecosystems 18, 62–75. doi: 10.1007/s10021-014-9812-2
Hall, E. K., Bernhardt, E. S., Bier, R. L., Bradford, M. A., Boot, C. M., Cotner, J. B., et al. (2018). Understanding how microbiomes influence the systems they inhabit. Nat. Microbiol. 3, 977–982. doi: 10.1038/s41564-018-0201-z
Hall, S. J., Hale, R. L., Baker, M. A., Bowling, D. R., and Ehleringer, J. R. (2015). Riparian plant isotopes reflect anthropogenic nitrogen perturbations: robust patterns across land use gradients. Ecosphere 6:art200. doi: 10.1890/ES15-00319.1
Hanson, C. A., Fuhrman, J. A., Horner-Devine, M. C., and Martiny, J. B. H. (2012). Beyond biogeographic patterns: processes shaping the microbial landscape. Nat. Rev. Microbiol. 10, 497–506. doi: 10.1038/nrmicro2795
Hendricks, S. P., and White, D. S. (2000). “Stream and Groundwater Influences on Phosphorus Biogeochemistry-9,” in Streams and Ground Waters, eds J. J. Jones and P. J. Mulholland (Cambridge, MA: Academic Press).
Henson, M. W., Hanssen, J., Spooner, G., Flemming, P., Pukonen, M., Stahr, F., et al. (2017). Nutrient dynamics and stream order influence microbial community patterns along a 2914 km transect of the Mississippi River. bioRxiv [Preprint]. doi: 10.1101/091512
Herrmann, H., Nolde, J., Berger, S., and Heise, S. (2016). Aquatic ecotoxicity of lanthanum – A review and an attempt to derive water and sediment quality criteria. Ecotoxicol. Environ. Saf. 124, 213–238. doi: 10.1016/j.ecoenv.2015.09.033
Huguet, A., Vacher, L., Relexans, S., Saubusse, S., Froidefond, J. M., and Parlanti, E. (2009). Properties of fluorescent dissolved organic matter in the Gironde Estuary. Org. Geochem. 40, 706–719. doi: 10.1016/j.orggeochem.2009.03.002
Itoh, T., Yamanoi, K., Kudo, T., Ohkuma, M., and Takashina, T. (2011). Aciditerrimonas ferrireducens gen. nov., sp. nov., an iron-reducing thermoacidophilic actinobacterium isolated from a solfataric field. Int. J. Syst. Evol. Microbiol. 61, 1281–1285. doi: 10.1099/ijs.0.023044-0
James, G., Witten, D., Hastie, T., and Tibshirani, R. (2013). An Introduction to Statistical Learning. New York, NY: Springer. doi: 10.1007/978-1-4614-7138-7
Jones, A. S., Aanderud, Z. T., Horsburgh, J. S., Eiriksson, D. P., Dastrup, D., Cox, C., et al. (2017). Designing and implementing a network for sensing water quality and hydrology across mountain to urban transitions. JAWRA J. Am. Water Resour. Assoc. 53, 1095–1120. doi: 10.1111/1752-1688.12557
Jones, S. E., and Lennon, J. T. (2010). Dormancy contributes to the maintenance of microbial diversity. Proc. Natl. Acad. Sci. U.S.A. 107, 5881–5886. doi: 10.1073/pnas.0912765107
Junker, B. H., and Schreiber, F. (2011). Analysis of Biological Networks. Hoboken, NJ: John Wiley & Sons.
Kadlec, J., StClair, B., Ames, D. P., and Gill, R. A. (2015). WaterML R package for managing ecological experiment data on a CUAHSI HydroServer. Ecol. Informatics 28, 19–28. doi: 10.1016/j.ecoinf.2015.05.002
Kolbe, T., de Dreuzy, J.-R., Abbott, B. W., Aquilina, L., Babey, T., Green, C. T., et al. (2019). Stratification of reactivity determines nitrate removal in groundwater. Proc. Natl. Acad. Sci. U.S.A. 116, 2494–2499. doi: 10.1073/pnas.1816892116
Leff, L. G., Leff, A. A., and Lemke, M. J. (1998). Seasonal changes in planktonic bacterial assemblages of two Ohio streams. Freshw. Biol. 39, 129–134. doi: 10.1046/j.1365-2427.1998.00269.x
Leff, L. G., and Lemke, M. J. (1998). Ecology of aquatic bacterial populations: lessons from applied microbiology. J. North Am. Benthol. Soc. 17, 261–271. doi: 10.2307/1467967
Leibold, M. A., Holyoak, M., Mouquet, N., Amarasekare, P., Chase, J. M., Hoopes, M. F., et al. (2004). The metacommunity concept: a framework for multi-scale community ecology. Ecol. Lett. 7, 601–613. doi: 10.1111/j.1461-0248.2004.00608.x
Lennon, J. T., and Jones, S. E. (2011). Microbial seed banks: the ecological and evolutionary implications of dormancy. Nat. Rev. Microbiol. 9, 119–130. doi: 10.1038/nrmicro2504
Lepš, J., and Šmilauer, P. (2003). Multivariate Analysis of Ecological Data Using CANOCO. Cambridge: Cambridge University Press.
Lindström, E. S., Agterveld, M. P. K.-V., and Zwart, G. (2005). Distribution of typical freshwater bacterial groups is associated with pH, temperature, and lake water retention time. Appl. Environ. Microbiol. 71, 8201–8206. doi: 10.1128/AEM.71.12.8201-8206.2005
Lindström, E. S., and Östman, Ö. (2011). The importance of dispersal for bacterial community composition and functioning. PLoS One 6:e25883. doi: 10.1371/journal.pone.0025883
Mandal, S., Van Treuren, W., White, R. A., Eggesbø, M., Knight, R., and Peddada, S. D. (2015). Analysis of composition of microbiomes: a novel method for studying microbial composition. Microb. Ecol. Health Dis. 26, 27663. doi: 10.3402/mehd.v26.27663
Martinez Arbizu, P. (2019). pairwiseAdonis: Pairwise Multilevel Comparison Using Adonis. Available online at: https://github.com/pmartinezarbizu/pairwiseAdonis (accessed March 6, 2019).
McCormick, A., Hoellein, T. J., Mason, S. A., Schluep, J., and Kelly, J. J. (2014). Microplastic is an abundant and distinct microbial Habitat in an Urban River. Environ. Sci. Technol. 48, 11863–11871. doi: 10.1021/es503610r
McKnight, D. M., Boyer, E. W., Westerhoff, P. K., Doran, P. T., Kulbe, T., and Andersen, D. T. (2001). Spectrofluorometric characterization of dissolved organic matter for indication of precursor organic material and aromaticity. Limnol. Oceanogr. 46, 38–48. doi: 10.4319/lo.2001.46.1.0038
McMurdie, P. J., and Holmes, S. (2013). phyloseq: an R package for reproducible interactive analysis and graphics of microbiome census data. PLoS One 8:e61217. doi: 10.1371/journal.pone.0061217
Messager, M. L., Lehner, B., Grill, G., Nedeva, I., and Schmitt, O. (2016). Estimating the volume and age of water stored in global lakes using a geo-statistical approach. Nat. Commun. 7:13603. doi: 10.1038/ncomms13603
Meybeck, M. (2003). Global analysis of river systems: from Earth system controls to Anthropocene syndromes. Philos. Trans. R. Soc. Lond. Ser. B Biol. Sci. 358, 1935–1955. doi: 10.1098/rstb.2003.1379
Miller, M. P., Boyer, E. W., McKnight, D. M., Brown, M. G., Gabor, R. S., Hunsaker, C. T., et al. (2016). Variation of organic matter quantity and quality in streams at Critical Zone Observatory watersheds. Water Resour. Res. 52, 8202–8216. doi: 10.1002/2016WR018970
Moatar, F., Abbott, B. W., Minaudo, C., Curie, F., and Pinay, G. (2017). Elemental properties, hydrology, and biology interact to shape concentration-discharge curves for carbon, nutrients, sediment, and major ions. Water Resour. Res. 53, 1270–1287. doi: 10.1002/2016wr019635
Murphy, K. R., Stedmon, C. A., Graeber, D., and Bro, R. (2013). Fluorescence spectroscopy and multi-way techniques, PARAFAC. Anal. Methods 5, 6557–6566. doi: 10.1039/C3AY41160E
Murphy, K. R., Stedmon, C. A., Wenig, P., and Bro, R. (2014). OpenFluor– an online spectral library of auto-fluorescence by organic compounds in the environment. Anal. Methods 6, 658–661. doi: 10.1039/C3AY41935E
Niño-García, J. P., Ruiz-González, C., and del Giorgio, P. A. (2016). Interactions between hydrology and water chemistry shape bacterioplankton biogeography across boreal freshwater networks. ISME J. 10, 1755–1766. doi: 10.1038/ismej.2015.226
Niño-García, J. P., Ruiz-González, C., and del Giorgio, P. A. (2017). Exploring the ecological coherence between the spatial and temporal patterns of Bacterioplankton in boreal lakes. Front. Microbiol. 8:636. doi: 10.3389/fmicb.2017.00636
Oksanen, J., Blanchet, F. G., Kindt, R., Legendre, P., Minchin, P. R., O’Hara, R. B., et al. (2015). Vegan: Community Ecology Package. Available online at: http://CRAN.R-project.org/package=vegan
Oldham, C. E., Farrow, D. E., and Peiffer, S. (2013). A generalized Damköhler number for classifying material processing in hydrological systems. Hydrol. Earth Syst. Sci. 17, 1133–1148. doi: 10.5194/hess-17-1133-2013
Parlanti, E., Wörz, K., Geoffroy, L., and Lamotte, M. (2000). Dissolved organic matter fluorescence spectroscopy as a tool to estimate biological activity in a coastal zone submitted to anthropogenic inputs. Org. Geochem. 31, 1765–1781. doi: 10.1016/S0146-6380(00)00124-8
Pernthaler, J. (2005). Predation on prokaryotes in the water column and its ecological implications. Nat. Rev. Microbiol. 3, 537–546. doi: 10.1038/nrmicro1180
Peura, S., Eiler, A., Bertilsson, S., Nykänen, H., Tiirola, M., and Jones, R. I. (2012). Distinct and diverse anaerobic bacterial communities in boreal lakes dominated by candidate division OD1. ISME J. 6, 1640–1652. doi: 10.1038/ismej.2012.21
Quast, C., Pruesse, E., Yilmaz, P., Gerken, J., Schweer, T., Yarza, P., et al. (2013). The SILVA ribosomal RNA gene database project: improved data processing and web-based tools. Nucleic Acids Res. 41, D590–D596. doi: 10.1093/nar/gks1219
Ren, L., Song, X., He, D., Wang, J., Tan, M., Xia, X., et al. (2019). Bacterioplankton Metacommunity processes across thermal gradients: weaker species sorting but stronger niche segregation in summer than in winter in a subtropical bay. Appl. Environ. Microbiol. 85:e02088-18. doi: 10.1128/AEM.02088-18
Reshef, D. N., Reshef, Y. A., Finucane, H. K., Grossman, S. R., McVean, G., Turnbaugh, P. J., et al. (2011). Detecting novel associations in large data sets. Science 334, 1518–1524. doi: 10.1126/science.1205438
Rose, S., and Peters, N. E. (2001). Effects of urbanization on streamflow in the Atlanta area (Georgia, USA): a comparative hydrological approach. Hydrol. Process. 15, 1441–1457. doi: 10.1002/hyp.218
Ruiz-González, C., Niño-García, J. P., Lapierre, J.-F., and del Giorgio, P. A. (2015). The quality of organic matter shapes the functional biogeography of bacterioplankton across boreal freshwater ecosystems. Glob. Ecol. Biogeogr. 24, 1487–1498. doi: 10.1111/geb.12356
Ruiz-González, C., Proia, L., Ferrera, I., Gasol, J. M., and Sabater, S. (2013). Effects of large river dam regulation on bacterioplankton community structure. FEMS Microbiol. Ecol. 84, 316–331. doi: 10.1111/1574-6941.12063
Sartory, D. P., and Grobbelaar, J. U. (1984). Extraction of chlorophyll a from freshwater phytoplankton for spectrophotometric analysis. Hydrobiologia 114, 177–187. doi: 10.1007/BF00031869
Savio, D., Sinclair, L., Ijaz, U. Z., Parajka, J., Reischer, G. H., Stadler, P., et al. (2015). Bacterial diversity along a 2600 km river continuum: river bacterioplankton diversity. Environ. Microbiol. 17, 4994–5007. doi: 10.1111/1462-2920.12886
Schloss, P. D., Westcott, S. L., Ryabin, T., Hall, J. R., Hartmann, M., Hollister, E. B., et al. (2009). Introducing mothur: open-source, platform-independent, community-supported software for describing and comparing microbial communities. Appl. Environ. Microbiol. 75, 7537–7541. doi: 10.1128/AEM.01541-09
Shiklomanov, I. A. (1993). “World fresh water resources,” in Water in Crisis: A Guide to the World’s Fresh Water Resources, ed. P. H. Gleick (Oxford: Oxford University Press), 13–24.
Smedley, P. L., and Kinniburgh, D. G. (2017). Molybdenum in natural waters: a review of occurrence, distributions and controls. Appl. Geochem. 84, 387–432. doi: 10.1016/j.apgeochem.2017.05.008
Somerville, C. C., Knight, I. T., Straube, W. L., and Colwell, R. R. (1989). SIMPLE, RAPID METHOD FOR DIRECT ISOLATION OF NUCLEIC-ACIDS FROM AQUATIC ENVIRONMENTS. Appl. Environ. Microbiol. 55, 548–554. doi: 10.1128/aem.55.3.548-554.1989
Steinman, A. D., Lamberti, G. A., Leavitt, P. R., and Uzarski, D. G. (2017). “Chapter 12 - Biomass and Pigments of Benthic Algae,” in Methods in Stream Ecology, Volume 1 (Third Edition), eds F. R. Hauer and G. A. Lamberti (Boston, MA: Academic Press), 223–241. doi: 10.1016/B978-0-12-416558-8.00012-3
Tamura, T. (2014). “The Family Sporichthyaceae,” in The Prokaryotes, eds E. Rosenberg, E. F. DeLong, S. Lory, E. Stackebrandt, and F. Thompson (Berlin: Springer), 883–888. doi: 10.1007/978-3-642-30138-4_182
Trevors, J. T., Barkay, T., and Bourquin, A. W. (1987). Gene transfer among bacteria in soil and aquatic environments: a review. Can. J. Microbiol. 33, 191–198. doi: 10.1139/m87-033
US Census Bureau (2017). Maricopa County Added Over 222 People Per Day in 2016. The United States Census Bureau. Available online at: https://www.census.gov/newsroom/press-releases/2017/cb17-44.html (accessed February 23, 2019).
Van Rossum, T., Peabody, M. A., Uyaguari-Diaz, M. I., Cronin, K. I., Chan, M., Slobodan, J. R., et al. (2015). Year-long metagenomic study of river microbiomes across land use and water quality. Front. Microbiol. 6:1405. doi: 10.3389/fmicb.2015.01405
Vitousek, P. M., Mooney, H. A., Lubchenco, J., and Melillo, J. M. (1997). Human domination of earth’s ecosystems. Science 277, 494–499. doi: 10.1126/science.277.5325.494
Wang, J., Meier, S., Soininen, J., Casamayor, E. O., Pan, F., Tang, X., et al. (2017). Regional and global elevational patterns of microbial species richness and evenness. Ecography 40, 393–402. doi: 10.1111/ecog.02216
Ward, J. V., and Stanford, J. (1983). The serial discontinuity concept of lotic ecosystems. Dyn. Lotic Ecosyst. 10, 29–42.
Watson, J. E. M., Venter, O., Lee, J., Jones, K. R., Robinson, J. G., Possingham, H. P., et al. (2018). Protect the last of the wild. Nature 563, 27–30. doi: 10.1038/d41586-018-07183-6
Weishaar, J. L., Aiken, G. R., Bergamaschi, B. A., Fram, M. S., Fujii, R., and Mopper, K. (2003). Evaluation of specific ultraviolet absorbance as an indicator of the chemical composition and reactivity of dissolved organic carbon. Environ. Sci. Technol. 37, 4702–4708. doi: 10.1021/es030360x
Widder, S., Besemer, K., Singer, G. A., Ceola, S., Bertuzzo, E., Quince, C., et al. (2014). Fluvial network organization imprints on microbial co-occurrence networks. Proc. Natl. Acad. Sci. U.S.A. 111, 12799–12804. doi: 10.1073/pnas.1411723111
Willems, A. (2014). “The Family Comamonadaceae,” in The Prokaryotes, eds E. Rosenberg, E. F. DeLong, S. Lory, E. Stackebrandt, and F. Thompson (Berlin: Springer), 777–851. doi: 10.1007/978-3-642-30197-1_238
Yannarell, A. C., Kent, A. D., Lauster, G. H., Kratz, T. K., and Triplett, E. W. (2003). Temporal patterns in bacterial communities in three temperate lakes of different Trophic Status. Microb. Ecol. 46, 391–405. doi: 10.1007/s00248-003-1008-9
Zarnetske, J. P., Haggerty, R., Wondzell, S. M., and Baker, M. A. (2011). Dynamics of nitrate production and removal as a function of residence time in the hyporheic zone. J. Geophys. Res. Biogeosci. 116:G01025. doi: 10.1029/2010JG001356
Zeglin, L. H. (2015). Stream microbial diversity in response to environmental changes: review and synthesis of existing research. Front. Microbiol. 6:454. doi: 10.3389/fmicb.2015.00454
Zelenı, D. (2011). Analysis of Community Ecology Data in R. Analysis of Community Ecology Data in R. Available online at: https://www.davidzeleny.net/anadat-r/doku.php/en:start (accessed March 7, 2019).
Zsolnay, A., Baigar, E., Jimenez, M., Steinweg, B., and Saccomandi, F. (1999). Differentiating with fluorescence spectroscopy the sources of dissolved organic matter in soils subjected to drying. Chemosphere 38, 45–50. doi: 10.1016/S0045-6535(98)00166-0
Keywords: bacterioplankton, dispersal, water chemistry, species sorting, urban, reservoir, community composition
Citation: Jones EF, Griffin N, Kelso JE, Carling GT, Baker MA and Aanderud ZT (2020) Stream Microbial Community Structured by Trace Elements, Headwater Dispersal, and Large Reservoirs in Sub-Alpine and Urban Ecosystems. Front. Microbiol. 11:491425. doi: 10.3389/fmicb.2020.491425
Received: 13 August 2019; Accepted: 04 November 2020;
Published: 26 November 2020.
Edited by:
James Cotner, University of Minnesota Twin Cities, United StatesReviewed by:
Jianjun Wang, Nanjing Institute of Geography and Limnology (CAS), ChinaSophie Crevecoeur, Environment and Climate Change, Canada
Jérôme Comte, Université du Québec, Canada
Copyright © 2020 Jones, Griffin, Kelso, Carling, Baker and Aanderud. This is an open-access article distributed under the terms of the Creative Commons Attribution License (CC BY). The use, distribution or reproduction in other forums is permitted, provided the original author(s) and the copyright owner(s) are credited and that the original publication in this journal is cited, in accordance with accepted academic practice. No use, distribution or reproduction is permitted which does not comply with these terms.
*Correspondence: Erin Fleming Jones, ZXJpbmZqb25lczNAZ21haWwuY29t