- 1Departamento de Biología Celular, Facultad de Ciencias, Universidad Nacional Autónoma de México, Mexico City, Mexico
- 2Departamento de Biología Molecular y Biotecnología, Instituto de Investigaciones Biomédicas, Universidad Nacional Autónoma de México, Mexico City, Mexico
- 3Departamento de Ciencias Naturales, Unidad Cuajimalpa, Universidad Autónoma Metropolitana, Mexico City, Mexico
- 4Laboratorio de Alelopatía, Departamento de Ecología Funcional, Instituto de Ecología, Universidad Nacional Autónoma de México, Mexico City, Mexico
- 5Instituto de Investigaciones en Ecosistemas y Sustentabilidad, Universidad Nacional Autónoma de México, Morelia, Mexico
The two-step model for plant root microbiomes considers soil as the primary microbial source. Active selection of the plant’s bacterial inhabitants results in a biodiversity decrease toward roots. We collected sixteen samples of in situ ruderal plant roots and their soils and used these soils as the main microbial input for single genotype tomatoes grown in a greenhouse. Our main goal was to test the soil influence in the structuring of rhizosphere microbiomes, minimizing environmental variability, while testing multiple plant species. We massively sequenced the 16S rRNA and shotgun metagenomes of the soils, in situ plants, and tomato roots. We identified a total of 271,940 bacterial operational taxonomic units (OTUs) within the soils, rhizosphere and endospheric microbiomes. We annotated by homology a total of 411,432 (13.07%) of the metagenome predicted proteins. Tomato roots did follow the two-step model with lower α-diversity than soil, while ruderal plants did not. Surprisingly, ruderal plants are probably working as a microenvironmental oasis providing moisture and plant-derived nutrients, supporting larger α-diversity. Ruderal plants and their soils are closer according to their microbiome community composition than tomato and its soil, based on OTUs and protein comparisons. We expected that tomato β-diversity clustered together with their soil, if it is the main rhizosphere microbiome structuring factor. However, tomato microbiome β-diversity was associated with plant genotype in most samples (81.2%), also supported by a larger set of enriched proteins in tomato rhizosphere than soil or ruderals. The most abundant bacteria found in soils was the Actinobacteria Solirubrobacter soli, ruderals were dominated by the Proteobacteria Sphingomonas sp. URGHD0057, and tomato mainly by the Bacteroidetes Ohtaekwangia koreensis, Flavobacterium terrae, Niastella vici, and Chryseolinea serpens. We calculated a metagenomic tomato root core of 51 bacterial genera and 2,762 proteins, which could be the basis for microbiome-oriented plant breeding programs. We attributed a larger diversity in ruderal plants roots exudates as an effect of the moisture and nutrient acting as a microbial harbor. The tomato and ruderal metagenomic differences are probably due to plant domestication trade-offs, impacting plant-bacteria interactions.
Introduction
Soil and plant root-associated bacteria are relevant for plant health, which has already been noticed in the beginning of the 20th century (Hiltner, 1904). It has been hypothesized that the microbiome could be related to crop quality (Hartmann et al., 2008). Soil is the most diverse microbial ecosystem, with up to 1011 bacterial cells per gram (Roesch et al., 2007). Soil properties such as pH, nutrient content, or moisture, and plant species can drive the soil microbiome composition (Fierer and Jackson, 2006; Lauber et al., 2009; Schlaeppi et al., 2014). Plants and soil interact at the rhizosphere, defined as the millimetric soil layer attached to plant roots. Plants play an active role in selecting their microbial inhabitants through root exudates, accounting from 5 to 20% of the photosynthetically fixed carbon and used by the microbes (Marschner et al., 2004). Plant-microbe interactions mainly occur in the rhizosphere (Berendsen et al., 2012). Some other known factors affecting the root microbial community structure are plant developmental stage (Inceoğlu et al., 2011), pathogen presence (Tian et al., 2015), and soil characteristics (Lundberg et al., 2012; Edwards et al., 2015). Plant-microbiome interaction has documented effects on plant growth and health; for example, the root microbiome composition has been associated with biomass increase in Arabidopsis thaliana (Swenson et al., 2000) and can also affect flowering time (Panke-Buisse et al., 2015).
A study of the A. thaliana microbiome using hundreds of plants and two soil sources concluded that root bacterial communities were strongly influenced by soil type (Lundberg et al., 2012). Microbial diversity was reduced in the rhizosphere compared to the surrounding soil, suggesting that plants filter and recruit a microbiome subset; these observations have led to the two-step model of microbiome selection (Bulgarelli et al., 2012, 2013). This model considers soil abiotic properties in the soil microbiome (first step), and specific plant-derived rhizodeposits contribute to selecting differential microbes in the rhizosphere and the endosphere (second step) (Bulgarelli et al., 2013). In the two-step model, α-diversity decreased in the following order: soils > rhizosphere > endosphere (Bulgarelli et al., 2013). However, a global-scale meta-analysis has reported that root microbiomes of multiple plant species (domesticated and wild) have a more substantial diversity than soils (Thompson et al., 2017).
This work explores the bacterial diversity by 16S rRNA gene massive amplicon sequencing and whole shotgun metagenomes to predict the protein diversity of 16 geochemically distinct Mexican soils, collected over a large geographical scale (Figure 1A and Table 1). The collected soils were chosen based on country-wide edaphological charts (INEGI, 2014). We explored the role of soil in microbiome structuring of in situ ruderal plants, growing above the collected soils with multiple species and at several plant developmental stages. The collected soils were used as the substrate in a greenhouse experiment for growing tomatoes (Solanum lycopersicum), eliminating plant genotype variability as well as developmental, climatic, and watering variables. Finally, testing diverse soil groups allowed us to explore the tomato core root microbiome, which follows the two-step model for root microbiome selection. The ruderal plants do not follow the two-step model and have a larger diversity than their source soils.
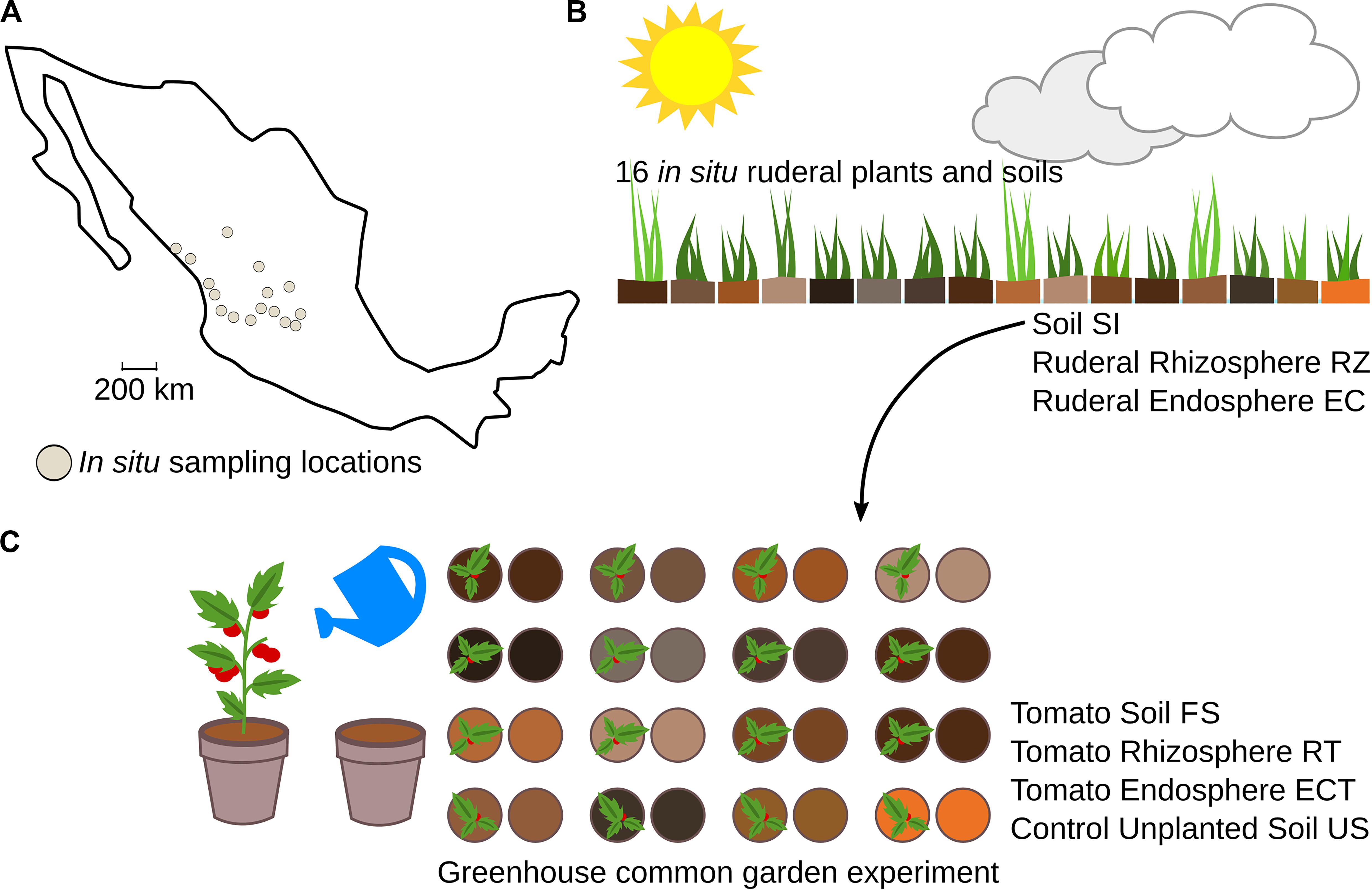
Figure 1. Experimental overview of the work. (A) In situ sampling locations; sampling points were selected according to edaphological charts. (B) The in situ plants were dependent on the weather and local environmental conditions, and we collected soil samples (SI) and roots of the dominant plant species in each locality. We extracted the rhizosphere (RZ) and endosphere (EC) metagenomic DNA. (C) A common garden experiment was conducted in a greenhouse; the soil (SI) was used as a microbial inoculum to reduce environmental variability. Plant diversity was eliminated using tomato, with constant watering, and finally, we collected roots (RT), endosphere (ECT), final soil (FS), and control unplanted soil (US).
Results
Soil Geochemical Description
Total nutrient concentration (C, N, P), pH, and Lang’s aridity index were calculated and considered as soil abiotic properties (Table 1). With the common garden experiment, we increased soil biological activity, reflected in the N and C overall increases after the experiment. We observed an increase in N concentrations in 12/16 samples, while total C increased in 11/16 samples, and P decreased in 7/16 samples (Table 1). Another explanation for the soil carbon enrichment is by plant root exudates (Canarini et al., 2019). Tomatoes planted in SLP1 and SIN2 exhibited a reduction in their total N concentrations; in SLP1, this is explained as plant biomass generation, and in SIN2, a coastal dune N was probably drained through watering (Table 1 and Supplementary Figure S2). Only two samples changed their pH profiles (Table 1). Ordination analysis showed clustering apart of source soils (SI) from final greenhouse soils (FS) and evidenced the modifications derived from the common garden experiment (Supplementary Figure S2A and Table 1).
Microbiome Diversity in the Source Soils, Ruderal Plants, and Tomatoes
A total of 106 amplicon libraries (16S rRNA gene V3-V4) were sequenced (Figure 1 and Supplementary Table S1). After quality control and assembly, 5,211,969 sequences were recovered. Subsequently 2,570,541 operational taxonomic units (OTUs; 97% identity) were clustered. After discarding singleton, mitochondrial, chloroplast, chimeras, and non-matching sequences, a total of 271,940 OTUs were the base for further analysis. The average in situ source soil (SI) OTU number (SI = 2,143) was lower than that in ruderal plants rhizospheres (RZ) and endosphere (EC) (RZ = 18,158; EC = 19,885 OTUs). Common garden final soils (FS) and control unplanted soils (US) had similar OTU averages (FS = 3,084; US = 2,882). The control soils (US) were pots with unplanted soil and output of the greenhouse experiment, thus receiving the same watering and homogeneous environmental conditions as final soils (FS), and tomato root microbiomes (RT and ECT). The rationale behind the common garden experiment is to impose a homogeneous treatment for all experimental units, in this case, regular watering and the same temperature, thereby eliminating local climatic variables of soil (temperature, humidity, altitude, precipitation). Lang’s aridity index is based on the historical data of precipitation and temperature in a site. The aridity index of soils is disrupted in the common garden experiment where the environmental conditions are homogenized. We hypothesize that control soils (US) have a larger number of OTUs than source soils (SI) either by reactivation of biological activity, increasing the abundance of microorganisms, and the proliferation of external sources of microbes such as the ones carried through air and water. Tomato rhizosphere (RT) and endosphere (ECT) samples had a higher OTU average than the SI, but a smaller average compared to FS (RT = 2,474 OTUs, EC = 2,088) (Supplementary Table S2).
We found 586 shared bacterial genera between soils and roots (rhizosphere and endosphere) of tomatoes and ruderal plants (Figure 2A). The source soils had eight unique genera and shared most (98.78%) of their microbes with tomatoes or ruderal plants. The largest amount of unique genera (46.21%) was found for the ruderal plants, sharing the most bacterial genera with the tomato and the soils (53.78%). The tomato root microbiomes had 14 unique bacterial genera (1.9%), four were only shared with soils (0.53%), while most genera were shared with soils and ruderal plants (97.53%). The unique bacterial members found in plants and not found in soil may be a product of vertical inheritance of the plant microbiome (e.g., seed endophytes, Truyens et al., 2015; Shade et al., 2017). Another of our analyses showed that the tomato core microbiome had 51 bacterial genera, while ruderal plants and core soils had 187 and 16 bacterial genera, respectively. Cores were defined as detected genera in all of the sample types compared (RT, soils, and RZ). Complete information on unique and shared OTUs is available (Supplementary Table S3). In comparison to our result, another study identified a bacterial tomato rhizosphere core microbiome composed of 68 orders using different tomato cultivars in a single soil, and 27 orders using a single tomato genotype in five soils (Cheng et al., 2020).
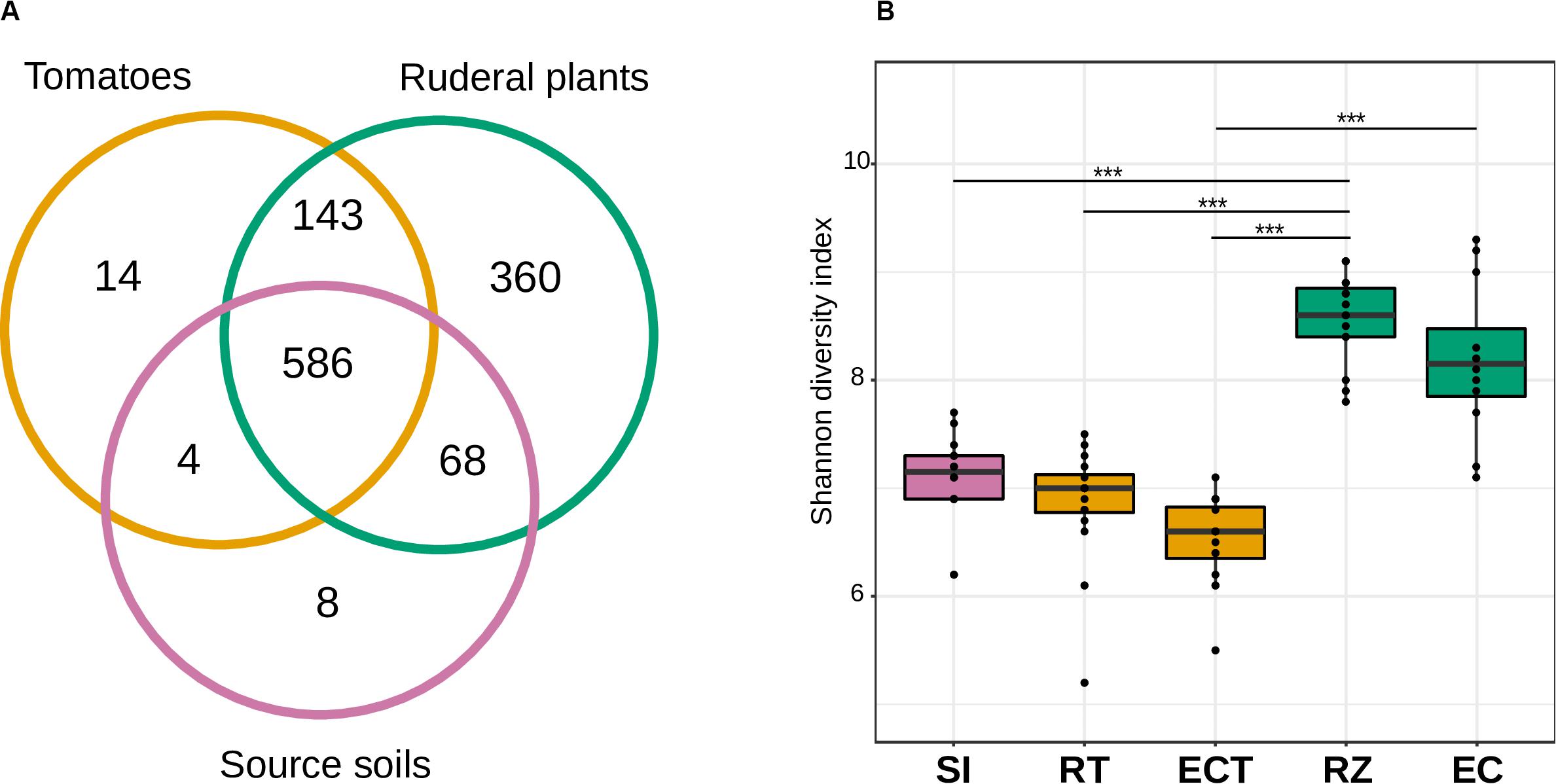
Figure 2. Alpha diversity and richness of the soil, rhizosphere, and endosphere of tomato and ruderal plants. (A) Venn diagram showing the number of shared bacterial genera in roots (endosphere + rhizosphere) and soils. (B) Boxplots showing the OTU Shannon diversity index (H&2032;) of source soils (SI), tomato rhizosphere (RT), tomato endosphere (ECT), in situ plants rhizosphere (RZ), and in situ ruderal plant endosphere (EC).
Ruderal Plants Rhizospheres Harbored a Larger Bacterial Diversity Than Soils and Tomatoes
We analyzed the α-diversity of soils, rhizospheres, and endosphere microbiomes through the Shannon diversity index (H′). Multiple studies have confirmed the two-step model of root microbiomes (Lundberg et al., 2012; Bulgarelli et al., 2013; Edwards et al., 2015). Here, we found that soils (H′ = 6.1–7.6) were more diverse than the tomato rhizosphere (H′ = 5.2–7.4) or the tomato endosphere (H′ = 5.5–7.1), thus fitting the two-step model for microbiome selection. However, when comparing the soil to the ruderal plant root microbiomes, higher H′ values were observed in the rhizosphere (H′ = 7.4–9.1) and even in their endosphere microbiomes (H′ = 7.0–9.2) compared to their soils (H′ = 6.1–7.6), not adjusting to the two-step model (Figure 2B and Supplementary Figure S3, Supplementary Table S2).
Ruderal plants might have a larger α-diversity because of the rhizosphere micro-environmental conditions, analogs to an oasis in the dry soil. However, previous reports show a larger diversity in rhizospheres than in soils comparing different biomes (Thompson et al., 2017). Marasco et al. (2018) found a higher 16S rRNA copies (qPCR) in the rhizosphere and rhizosheath than soil, of three species of dune colonizing speargrasses (Stipagrostis sabulicola, S. seelyae, and Cladoraphis spinosa). The larger rhizosphere and rhizoheath suggest an increase in microbial abundance and activity in these plants influenced microniches compared to soils. They also found that S. seelyae possessed a more substantial species richness in the rhizosphere than the bulk soil. Another study found that plants of Caragana microphylla can host rhizospheric microbial communities with larger Shannon diversity values in comparison to their bulk soils in particular sites or depending on the type of dunes (Gao et al., 2019). Additionally, some considerations must be made for ruderal plants since they are present in environments where they are not the only plant species but part of a plant community that could be broadening the rhizosphere effect. Different plant species or genotypes, as well as plant age, have been reported to attract specific bacterial communities (Baudoin et al., 2002; Marschner et al., 2004; Micallef et al., 2009). Additionally, plant communities and their richness and diversity growing in the soil affects belowground microbial community diversity, biomass, and respiration rates, thereby impacting plant diversity (Wu et al., 2019). Current agricultural management includes practices such as fertilizer-driven production, which decreases the importance of plant-microbe interactions when scavenging for nutrients (van der Heijden et al., 2008). The larger microbial diversity observed in ruderal plants shows the commitment of wild plants to their microbes, fostering plant-microbe relationships which are not observed in domesticated cultivars (Wissuwa et al., 2009). We have previously tested other non-domesticated plants, such as the aquatic carnivorous bladderwort Utricularia gibba (Alcaraz et al., 2016) and the bryophyte species Marchantia polymorpha and M. paleacea (Alcaraz et al., 2018); both showed less diversity in their root analogs (bladders, and rhizoids) than their soil sources, supporting the two-step model. The Marchantia microbiomes even allowed us to perform an extreme microbial selection due to the in vitro propagation of these plants, highlighting a reduced core of closely related microbial inhabitants (Alcaraz et al., 2018). Testing multiple plants, wild and domesticated, could reduce the gaps in understanding the microbiome structure loss as a domestication trade-off.
Plant Driven Selection of Bacterial Root Colonizers in Tomatoes and Ruderal Plants
We performed a α-diversity analysis based on the weighted UniFrac community distance matrix to dissect the role of soil in the establishment and structure of rhizo and endosphere microbiomes in both ruderal and S. lycopersicum plants (Figure 3). We observed that the microbiome (16S rRNA gene) distribution was largely driven by the host species. The weighted UniFrac dendrogram grouped the samples into three major clusters: Cluster (I) contains only tomato-associated microbiomes, cluster (II) includes soil and ruderal plant microbiomes, and a mixed cluster (III) includes soil, tomato, and ruderal plant microbiomes (Figure 3). The clustering of the three groups is supported by ANOSIM (R = 0.7257; p < 0.001; 999 permutations). Pairwise distance values were calculated between every sample in the weighted UniFrac dendrogram to evaluate the distance patterns and cohesion found inside and between each of the described clusters. The average internal distances were 0.5041 for cluster (I), 0.5058 for cluster (II), and 0.4787 for the cluster (III). The measured distance between any terminal node of cluster I against any tip in either cluster (II) or (III) was 0.6608. Most of the tomato samples were closer to each other than to their source soils. The tomato-associated cluster (Figure 3, cluster I) grouped 10/16 tomato rhizospheres, along with 13/16 of the tomato endospheres, suggesting a plant genotype-dependent role in root microbiome establishment. The closer α-diversity distance of ruderal plants to their soils, compared to tomatoes (Figure 3), showed the tomato host genotype associated microbiome selection having a larger effect than soil, lowering its overall α-diversity in a probable outcome of domestication trade-offs. A comparison of maize, its ancestor teosinte, and other Poaceae rhizosphere microbiomes showed correlations between microbiomes and host evolutionary distances (Bouffaud et al., 2014). The few tomatoes and ruderal samples that clustered closer to their source soils, were remarkably acid soils, indicating pH properties as microbiome structuring factor, as shown before (Fierer and Jackson, 2006; Männistö et al., 2007; Table 1).
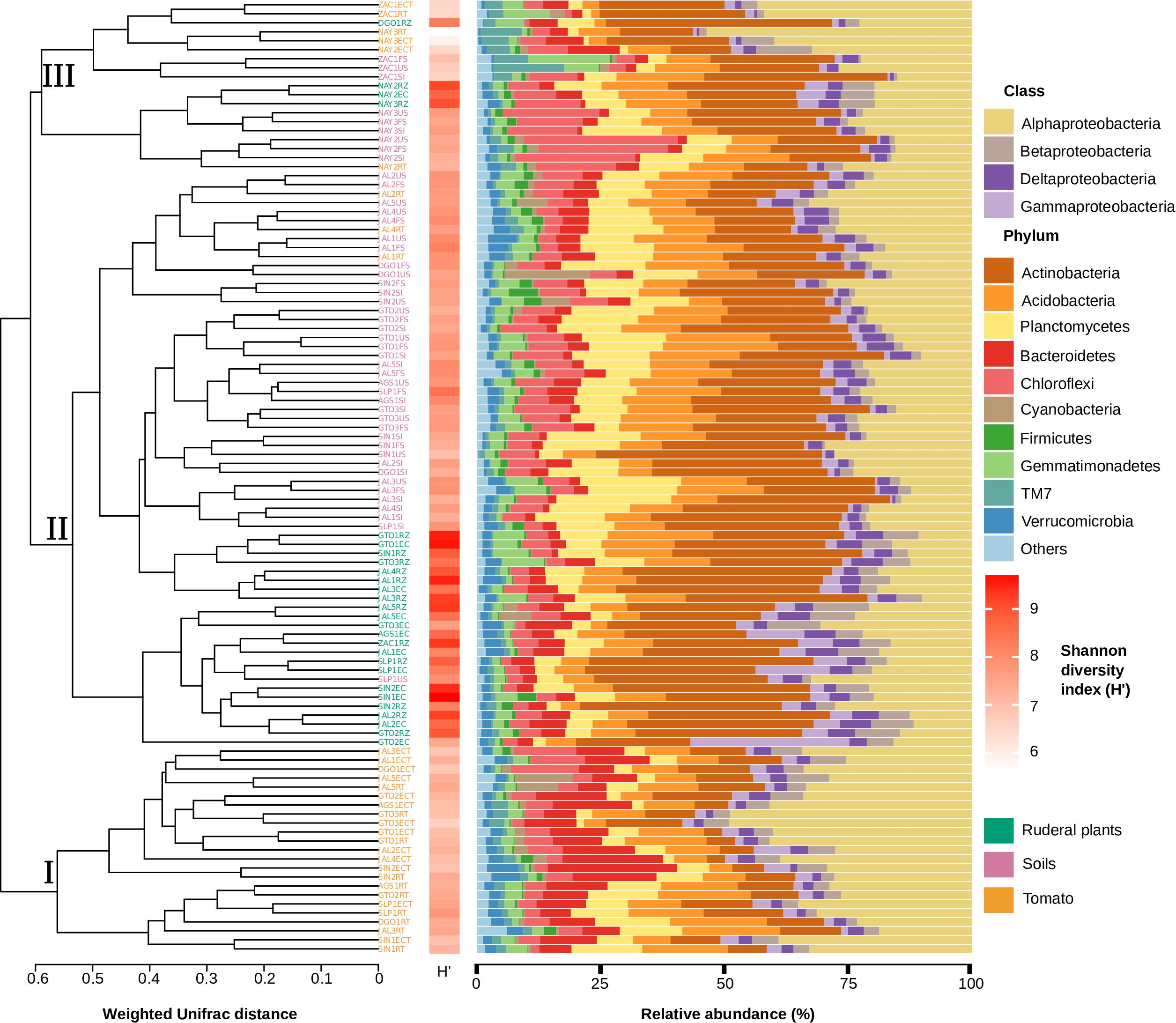
Figure 3. Host genotype and soil influence on microbial community structure (16S rRNA gene). On the left, a weighted Unifrac dendrogram shows α-diversity and phylogenetic similarity between soil, tomato, and ruderal plants. Each location is indicated at the dendrogram terminal nodes with a three-letter key for sampling location and suffix indicating type: initial source soil (SI), final soil (FS), unplanted soil (US), tomato rhizosphere (RT), tomato endosphere (ECT), ruderal plant rhizosphere (RZ), and ruderal plant endosphere (EC). Phyla diversity (H&2032;) in each sampled microbiome is shown as a horizontal heatmap. Bar plots show bacterial phyla relative abundance in each sample. Proteobacteria are shown at the class level in the bar plots.
Microbiome Phylogenetic Assignments and Differential Taxa in Soil, Ruderals, and Tomato
In situ samples of soils and ruderal plants were dominated by Actinobacteria, with a significantly (ANOVA p < 2e-16) lower abundance in tomato roots (Figure 3, Supplementary Figure S4 and Supplementary Table S5). Using the tomato (fixed plant genotype), we imposed a selective factor, since the plant-derived chemotactic signals and photosynthates should be similar, independent of the soil. Proteobacteria were significantly enriched in tomatoes (ANOVA p < 1.82e-15) compared to soils and ruderal plants. It seems that plants such as tomatoes as other agricultural species favor Proteobacteria (Correa-Galeote et al., 2018; Cordero et al., 2020), while ruderals and soils depend upon Actinobacteria. The class α-Proteobacteria was the most abundant in tomatoes, with significant enrichment (ANOVA p < 2e-16) compared to ruderal plants and soils. The β, γ, and δ-Proteobacteria were more abundant in ruderal plants (p < 0.05) than in tomatoes and soils. Bacteroidetes were enriched in tomato roots (ANOVA p < 1.34e-15) when compared to soils and ruderal plants (Figure 3, Supplementary Figure S4, and Supplementary Table S5). Each plant can attract and select specific microorganisms depending on plant-genotype-dependent chemical formulation of rhizodeposits and cell wall features, resulting in specificity for microbiome selection (Schlaeppi et al., 2014; Bulgarelli et al., 2015; Fitzpatrick et al., 2018). Additionally, in situ natural variations in the climatic conditions were reduced in the common garden experiment, tomato plants watered regularly, and minimized climatic variation. The larger abundance of Actinobacteria has practical explanations in plant interactions; there are reports of its use as biocontrol agents isolated from soil and rhizospheres, and they are secondary metabolite producers such as antibiotics or plant growth-promoting molecules such as indole acetic acid (El-Tarabily et al., 2009; Brader et al., 2014; Sreevidya et al., 2016). Actinobacteria differential abundance in both soils and ruderal plants can also be a product of environmental water limitations. Aridity increases the proportions of Actinobacteria in arid soils, while humid sites usually have larger Proteobacteria abundances (Neilson et al., 2017), probably because Proteobacteria have faster duplication times than Actinobacteria (Ramin and Allison, 2019).
We used DESeq2 to compare and identify significantly (p < 0.01, Bonferroni corrected) enriched OTUs (Supplementary Table S6). The overall diversity decrease in the tomato roots is consistent with the enrichment of certain bacterial groups capable of close plant interactions through specific molecular mechanisms (e.g., chemotaxis responsive, plant degradation enzymes) (Bais et al., 2006; Compant et al., 2010). We found six differential OTUs assigned as Sphingobium, Caulobacter, Asticcacaulis, Arthrospira, and Kaistobacter in the tomato rhizospheres compared to their source soils (Supplementary Figure S5A). These bacterial genera have been isolated from sources such as freshwater (Chen et al., 2013), soil (Costa et al., 2006), and rhizospheres (Young et al., 2008; Schreiter et al., 2014; Yang et al., 2016). The genera Caulobacter and Asticcacaulis are characterized by having at least one appendage or prostheca that protrudes from the cell envelope and can play a role in adhesion to solid substrates (Poindexter, 1981; Ong et al., 1990), a helpful attribute for the colonization of plant roots (Wheatley and Poole, 2018). Additionally, Caulobacter has been described as a hub taxa in the phyllosphere of Arabidopsis thaliana (Agler et al., 2016), remarking the possible importance of these taxa in plant-associated microbial communities. In contrast, in the endospheres, we found 14 enriched OTUs belonging to the same genera present in the rhizosphere and Agrobacterium and Lacibacter (Supplementary Figure S5B). The presence of OTUs assigned to the families Sphingomonadaceae and Bradyrhizobiaceae in roots of S. lycopersicum has been reported previously. Both Sphingomonadaceae and Bradyrhizobiaceae OTUs were reduced with plants inoculated with the pathogen Phytophthora parasitica, compared to healthy plants (Larousse et al., 2017). We found an overrepresentation of some Sphingobium and Rhizobium species, suggesting that their abundance could be used as a plant health proxy since we did not observe root rot symptoms in any of our individuals, as in other studies with healthy tomato plants (Satour and Butler, 1967; Lee et al., 2019). Moreover, in a previous work describing tomato roots, microbiomes, Sphingomonas, and Sphingobium were detected in more than 50% of the 16S rRNA gene OTUs (Lee et al., 2016). Sphingobium has been observed as the dominant genus in tomato roots elsewhere (Pii et al., 2016). The comparison between ruderal plant roots and soils showed 45 differentially abundant OTUs in the rhizospheres (Supplementary Figure S5C) and 31 enriched OTUs in the endosphere (Supplementary Figure S5D), most belonging to Actinobacteria (Supplementary Table S6). Additionally, we compared the sets of soils and their controls (Figure 1) and did not find any shared OTUs whose abundance differed significantly. Most of the ruderals in our study were grasses (Poaceae; Supplementary Figure S1), and recently it was reported that grasses rhizospheres were enriched in Actinobacteria under drought conditions (Naylor et al., 2017). Also, the loss of Actinobacteria abundance in tomato, a domesticated crop, compared to the soils and ruderal plants suggests that it could be a domestication trade-off, as previously suggested by a correlation between microbiome structure and host evolutionary history (Redford et al., 2010; Peiffer et al., 2013; Bouffaud et al., 2014).
Shotgun Metagenomic Diversity in Source Soils, Ruderal Plant Rhizospheres, and Tomato Rhizospheres
We sequenced 50.1 Gb in a total of 17 SI, RT, and RZ metagenomes. After quality control, we obtained 464,372,598 high-quality paired-end reads (μ = 27,316,035 ± 2,943,233 per sample), which were used as input to an assembly that yielded 12,677,118 contigs (μ = 745,713 ± 366,001 per sample), with an average N50 of 176 ± 51 bp and the longest contig average length of 45,645 bp. Subsequently, we were able to compute a total of 12,272,971 predicted peptides (μ = 708,835 ± 332,770 per sample) (Supplementary Table S7). Protein redundancy was reduced using proxy-genes of matches to known proteins and protein-clustering alignments (70% identity). After clustering and matching, protein annotation was performed using the M5NR database (see “Materials and Methods”), resulting in 3,147,929 proteins; only 411,432 (13.07%) were annotated based on homology against the M5NR database.
We compared the shared set of proteins between soils, ruderals, and tomatoes, resulting in a set of 43,305 proteins detected at least once for every sample type (Figure 4A). Most of the union set proteins (93%) were annotated. Tomatoes shared with the soils 8.49% of their predicted proteins, while ruderal plants shared 8.72% of the identified peptides with the soil. Tomatoes shared more coding genes with ruderal plants (8.85%) than with soil (8.49%) (Figure 4A). Different sets of proteins for each sample showed the largest novelty in soil (88.83%), followed by ruderal plants (87.46%) and tomatoes (86.36%) (Figure 4A). Although the largest number of unique proteins could be the result of an enthusiastic computer prediction, it was interesting that the tomato had the largest amount of annotated proteins (12.10%) compared to ruderals (9.97%) and soils (6.75%), maybe reflecting the larger previous genomic information in agricultural microbes, being scarcer in wild plants, and the soil microbes (Figure 4A). Complete lists of identified proteins are available as Supplementary Material (Supplementary Table S8).
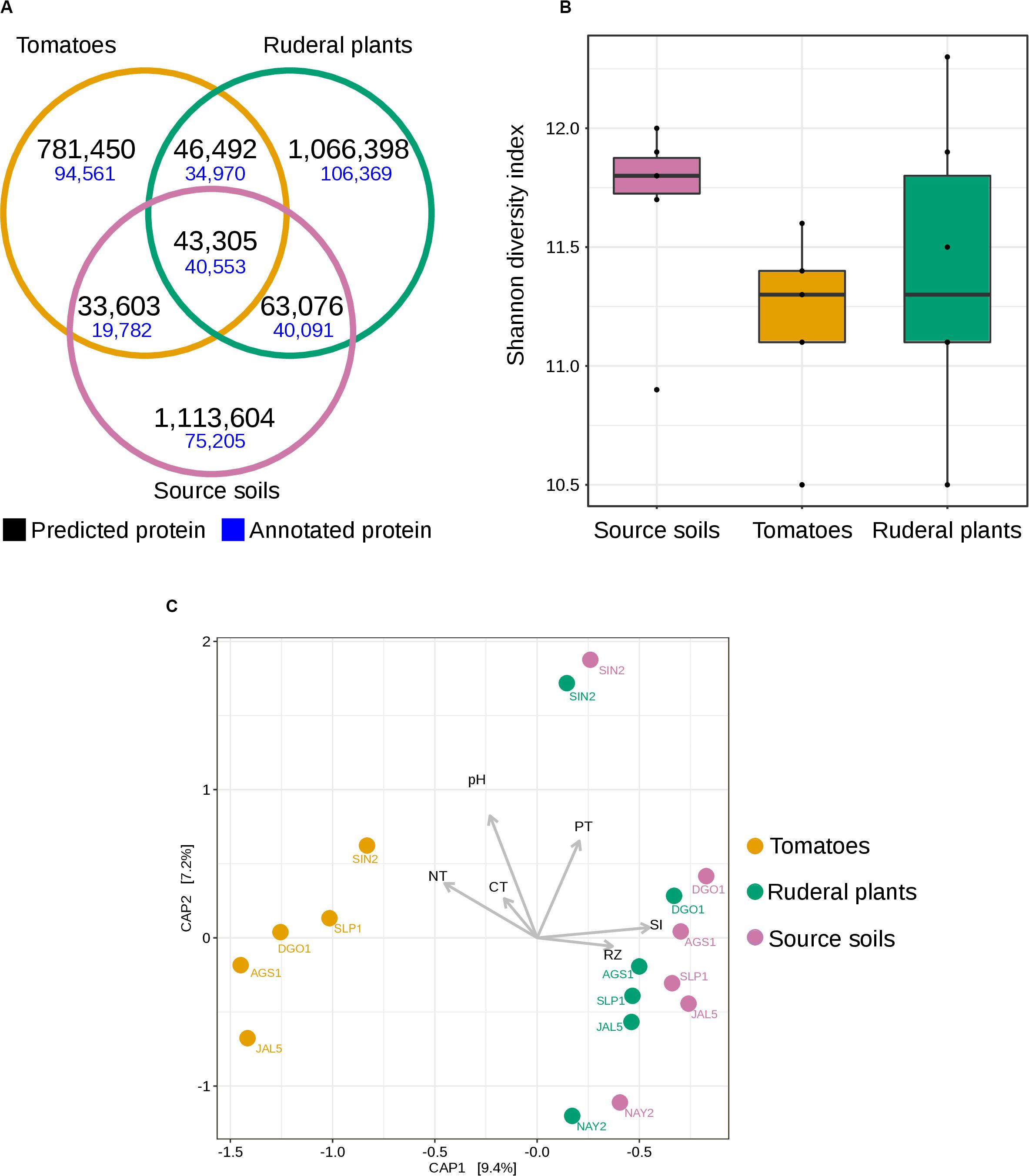
Figure 4. Shotgun metagenomics diversity of soil and rhizosphere microbial communities. (A) Venn diagram showing the number of shared and unique annotated protein families (70% identity) in soil and rhizosphere. (B) Boxplots showing the Shannon diversity index based on the total number of predicted proteins in soil and rhizosphere. (C) Constrained analysis of principal coordinates (CAP), calculated from the total number of predicted proteins for all sequenced soil and rhizosphere metagenomes using Bray-Curtis dissimilarity. Vectors display the environmental factors: CT, Total Carbon concentration; NT, Total Nitrogen concentration; PT, Total Phosphorus concentration; RZ, ruderal plant rhizosphere; SI, Initial soil.
We compared the protein α-diversity using the Shannon diversity index (H′) based on the total number of predicted proteins (Supplementary Table S9). Soil diversity had a higher median (H′ = 11.8) than tomato diversity (H′ = 11.3) and ruderal plant diversity (H′ = 11.3), without significant differences (Figure 4B and Supplementary Figure S6). We hypothesize that domestication decreased the microbial diversity of the tomato root microbiome compared with that of grasses growing in the same soil. Plant domestication is targeted at meeting the requirements of humans, thereby decreasing plant genetic variability and generating crops dependent on humans (Doebley et al., 2006; Bulgarelli et al., 2013). Interestingly, the two-step model for root microbiota resembles the effects of reductive gene diversity in crop domestication (Doebley et al., 2006). Current agricultural management includes practices such as fertilizer-driven production, which decreases the importance of plant-microbe interactions when scavenging for nutrients (van der Heijden et al., 2008). Although it is not as descriptive with metagenome-predicted proteins, and it probably needs further refinement, maybe through linking the OTU abundance with pan-genomics and metagenomics to describe the genomic coding diversity (Delmont and Eren, 2018). To test the hypothesis that the tomato predicted metaproteome is divergent from those of the soil and ruderal plants, as suggested by the 16S α-diversity dendrogram (Figure 3), we performed a constrained analysis of principal coordinates (CAP) ordination (Figure 4C). We used the protein abundance as CAP input, and we constrained the analysis by sample type, pH, total N, C, and P. This metagenomic profiling of the microbial communities showed that ruderal plants and soils have a similar composition of predicted proteins (Figure 4C), differentiating them from tomato rhizospheres and highlighting the host-dependent selection. The CAP explained 16.6% of the total observed variance, with CAP1 (9.4%) splitting the tomatoes from ruderals and source soils. Ruderal plants were closer to the soils, but not mixed with them (CAP, Bray-Curtis distance, PERMANOVA 9,999 permutations, p < 1e-4). The split tomato and ruderal-soil groups are also supported by ANOSIM (R = 0.4568; p < 0.001; 999 permutations) (Figure 4C). Correlations with the measured geochemical variables with the two CAP axes showed positive correlations of P, source soils (SI), and ruderal plants (RZ), while negative correlations observed for pH, N, and C (Figure 4C).
Shotgun Metagenomics Taxa Assignments
We were able to bin and classify 38% ± 1.66 of the sequenced metagenomic reads to multiple taxa. Bacteria accounted for 88.14% of the identified matches, followed by 7.09% of Eukaryota, with almost half of the hits corresponding to Fungi (3.83%) (Supplementary Table S10). Further work will explore eukaryotic, archaeal, and viral diversity of the sequenced metagenomes. In soils, the most abundant bacterial species binned was Solirubrobacter soli (Actinobacteria), which was also highly abundant in ruderals and tomatoes. Sphingomonas sp. URHD0057 (α-Proteobacteria) were most abundant in ruderals, along with Solirubrobacter soli and the Rhizobiales Rhodoplanes sp. Z2-YC6860. The 16S data showed that Bacteroidetes were significantly enriched in tomato roots compared to soil and ruderals; metagenomic bins confirm the 16S rRNA gene trends. There are reports about bacterial groups’ enrichment, such as Bacteroidetes on wild plants and Proteobacteria on domesticated plants (Pérez-Jaramillo et al., 2018). Within the principal tomato metagenomic bins, we found Ohtaekwangia koreensis, Flavobacterium terrae, Niastella vici, Chryseolinea serpens the metagenome-assembled genome of a Chitinophagaceae bacterium IBVUCB2 as Bacteroidetes species.
We analyzed a functional summary of the sequenced metagenomes using the SEED subsystem gene ontology (Figure 5). The largest category was clustering-based subsystems, which include protein families that are quite diverse from the CRISPR, sugar metabolism, other known categories, and hypothetical proteins. We only found small differences (Tukey’s HSD) in iron acquisition metabolism (p = 0.07), cell wall and capsule genes (p = 0.06) between soils and tomatoes. We found significant differences (p = 0.017) between ruderals and tomatoes in sulfur metabolism genes (Figure 5).
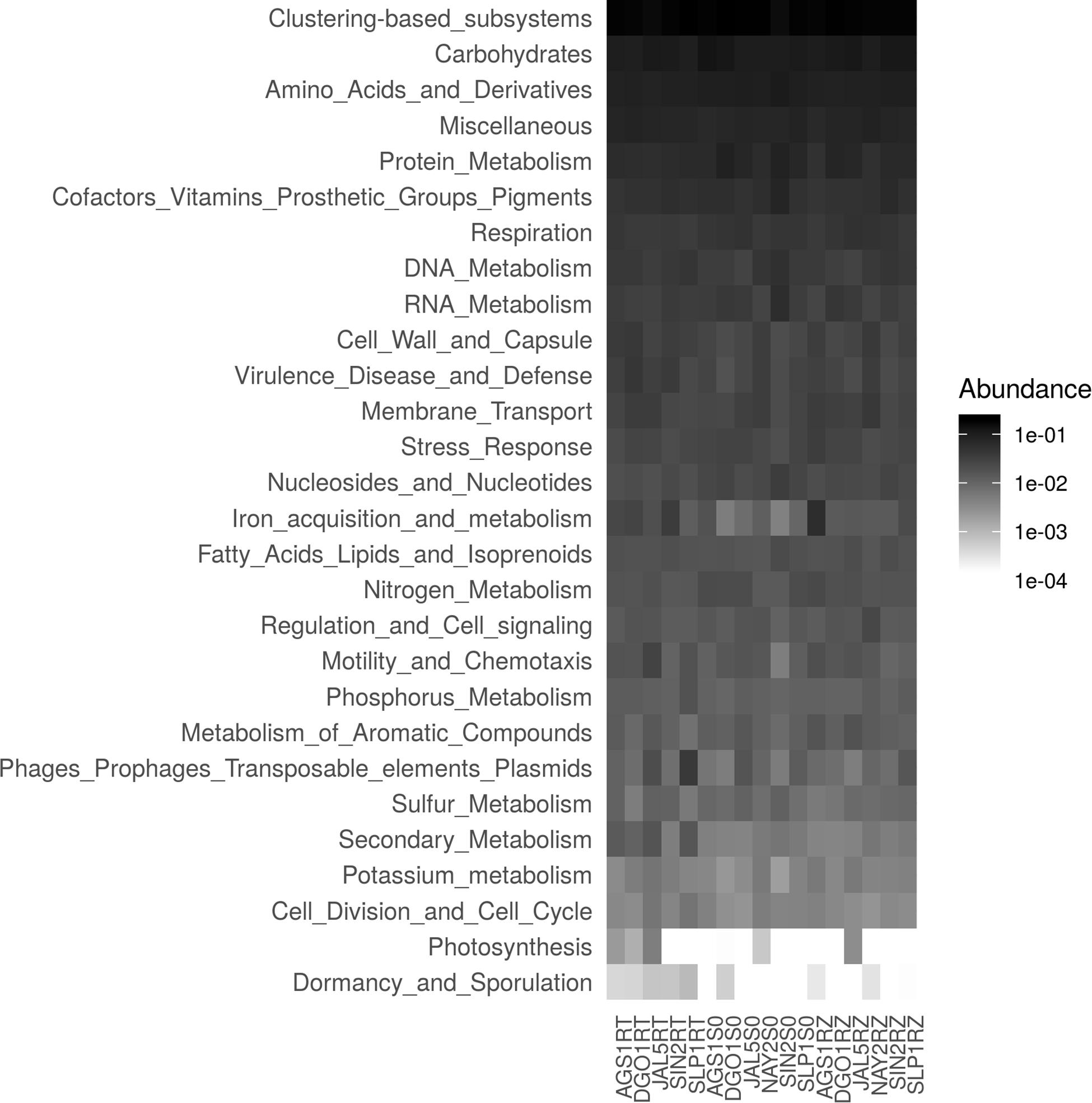
Figure 5. Summary of metagenomic functional profiles. Heatmap is describing the level 1 SEED subsystems ontology annotations in each row. Although only using the 138,627 M5NR matches to the SEED, representing 4.4% of the total dataset, it was helpful to describe the main molecular functions. Columns represent each metagenome, and the first four alphanumeric codes are for location; suffixes indicate sample type: RT is tomato rhizosphere metagenome, SI is source soil, and RZ represents ruderal plant rhizosphere metagenome.
Enriched Proteins in the Rhizospheres-Soil Comparison
Pairwise comparisons were made using DESeq2 to find significant (p < 0.001, Bonferroni) predicted protein enrichments. Comparing tomatoes (RT) and soils (SI), we identified 67 enriched proteins in RT involved in motility, chemotaxis, and biofilm formation (e.g., LuxR, CheY, diguanylate cyclase, CpaE), complex carbohydrate degradation (e.g., xyloglucanase, cellulase Cel5F), antibiotic resistance (e.g., β-lactamase class C), iron metabolism (e.g., TonB), and sporulation (e.g., SpoIIIE), as well as secretion system-related proteins (e.g., exo-sortase) (Supplementary Figure S7). The enrichment of Proteobacteria in tomato is in line with enriched genes such as motility and chemotaxis, widely distributed amongst α, β, and δ-Proteobacteria (Liu and Ochman, 2007). Motility traits are important for host colonization; this has been tested by mutagenesis in Pseudomonas fluorescens WCS36, reducing colonization efficiency of plant roots (de Weert et al., 2002), also reported for P. fluorescens SBW25 (Turnbull et al., 2001). Diguanylate cyclase and CpaE are involved in biofilm formation and pili production in Caulobacter crescentus (Skerker and Shapiro, 2000; Del Medico et al., 2020). Another interesting metabolic feature relevant for the plant-associated niche found in tomato roots is the enzyme xyloglucanase, involved in the degradation of xyloglucan. This heteropolysaccharide comprises up to one-quarter of the total carbohydrate content of terrestrial plant cell walls (Scheller and Ulvskov, 2010; Figure 6).
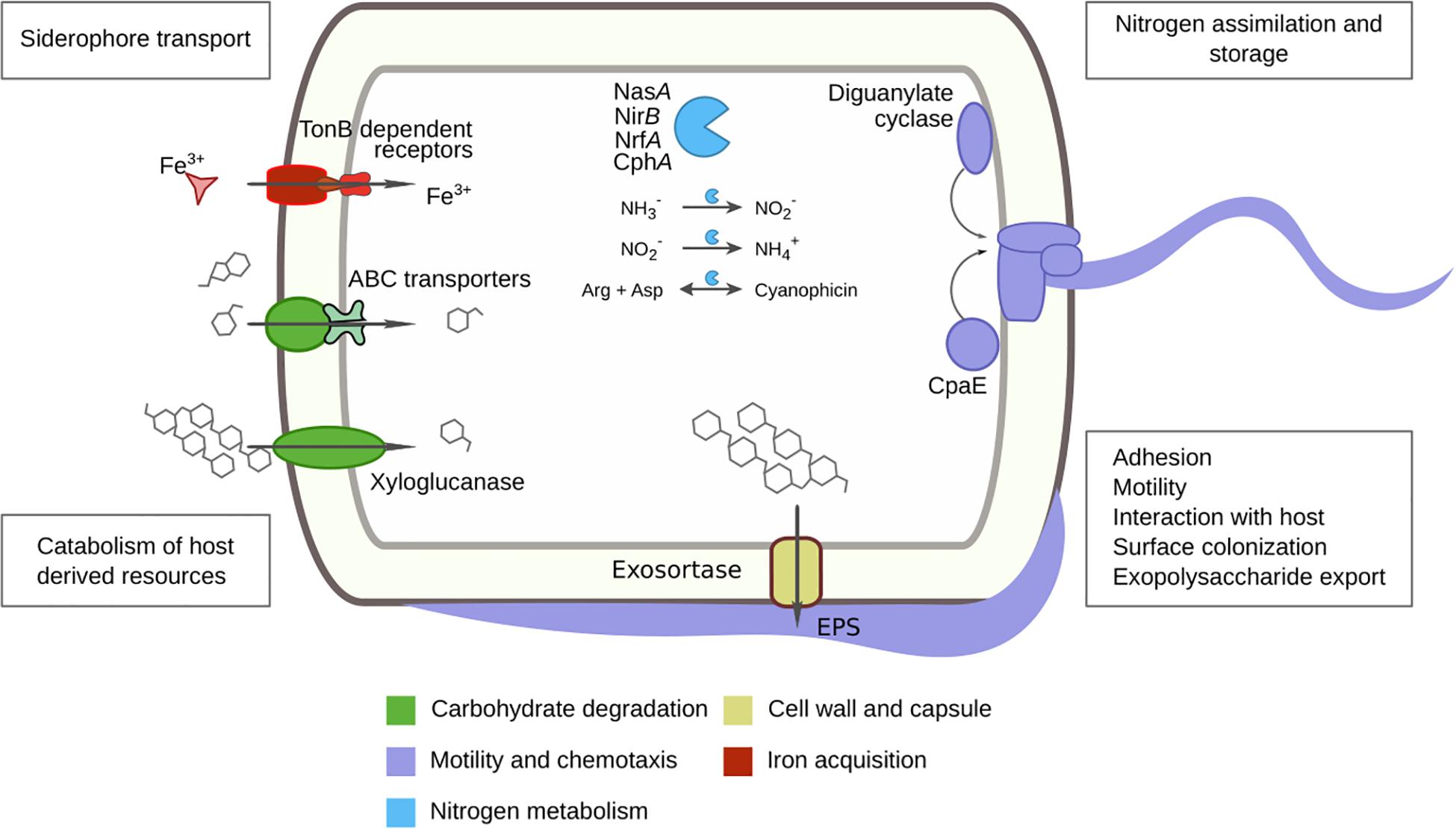
Figure 6. Tomato rhizosphere core metagenome and differential features with soil and ruderal plants summary. Predicted tomato core proteins and enriched tomato proteins are color-coded to the related cellular processes. The found proteins were previously reported as fundamental for plant-microbe interactions.
When comparing ruderals (RZ) and tomatoes (RT), we found 16 enriched proteins in RT and 11 in RZ (Supplementary Figure S8). The lowest number of enriched proteins between tomatoes and ruderals indicates that their shared set contains common features in plant-microbe interactions. Compared to RT, the RZ-enriched proteins included transporters and, interestingly, osmotic sensor components (e.g., osmosensitive K channel histidine kinase). The RT-enriched proteins, compared to RZ, included several peptidases (e.g., M17 leucyl aminopeptidase) and some horizontal gene transfer elements (e.g., integrase-recombinase, ISRSO17 transposase, bacteriophage N4 adsorption protein B). Interestingly, multiple similar proteins enriched in the RT-SI comparison were also enriched in the RT-RZ (e.g., β class C, glycoside hydrolases), remarking the host genotype filtering of RT. Finally, comparing RZ-SI registered only two RZ-enriched proteins, indicating the similarities between soil and ruderals (Supplementary Figure S9). The full list of overrepresented proteins for each comparison is available (Supplementary Table S11).
The Tomato Rhizosphere, Soil, and Ruderal Plant Core Metagenomes
It seems that the tomato was highly selective about its microbial inhabitants; we found 2,762 protein families ubiquitous in all tomato roots tested (Supplementary Figure S10). We used the protein annotation to reduce the dataset to 1,777 core proteins and only 1,353 exclusively in tomato (Supplementary Table S12). The core tomato metagenome was contrasting to the soil with only 162/639 and the ruderal metagenome with just 143/694 core-exclusive proteins. Some essential proteins were expected to be part of the core metagenomes and worked as controls for our searches, such as ribosomal proteins, DNA and RNA polymerases, gyrases, chaperonin GroEL, and we found them all within the tomato core metagenome. Within the tomato core metagenome, we found multiple strategies to cope with nitrogen, such as regulation genes via denitrification (nosZ) and nitrate reductase genes (nasA, nirB, and nrfA) to obtain ammonia (Figure 6). The high abundance of Actinobacteria in the source soils and the switch to a Proteobacteria dominance in the FS suggests processes such as biological nitrogen fixation and microbial biomass increments. Both Actinobacteria and Proteobacteria are capable of nitrogen fixation since their genomes contain nitrogenases (Boyd and Peters, 2013). Glutamate, glutamine synthetases, and their transferases were also detected in the RT core metagenome and could regulate amino acid synthesis and ammonia. Additional nitrogen storage proteins were detected, such as cyanophycin synthetase (CphA) and cyanophycinase, within the RT metagenomic core; cyanophycin is a non-ribosomal peptide built by aspartic acid and arginine. This reserve polymer regulates N and C and mediates N storage, providing bacterial fitness advantages under nitrogen fluctuations (Watzer and Forchhammer, 2018; Figure 6). Further, we found allantoinase and allantoate amidohydrolase genes, which are responsible for allantoin degradation to ammonia (Cruz-Ramos et al., 1997; Ma et al., 2016). Patatin-like phospholipase proteins were also found in the tomato core metagenome; they are phospholipases originally described in potato, but with abundant homologs in bacteria (Banerji and Flieger, 2004). Bacteria use patatins to target host cell membrane as effectors via the type III secretion system (Finck-Barbançon et al., 1997; Phillips et al., 2003; Sato et al., 2003) and are activated by ubiquitin (Anderson et al., 2015). The eukaryotic patatins are known to have antimicrobial activities (e.g., Phytophthora infestans inhibition) (Bártová et al., 2019). Tomato and potato, belonging to the family Solanaceae, interact with microbes via patatin and patatin-like proteins, and we will further explore plant-microbe interactions mediated by these proteins. While significantly enriched in ruderals, leucyl aminopeptidase was also ubiquitous in tomato metagenomes. Interestingly, leucine aminopeptidase A (LapA) is expressed in tomato after wounding and prevents foraging (e.g., Manduca sexta foraging tomato) (Fowler et al., 2009). LapA is also transcriptional and protein-responsive to microbial pathogens (Pautot et al., 1993; Pautot et al., 2001). The bacterial leucine aminopeptidases found in tomato metagenomes could be expanding the plant’s defensive response through LapA, but this is yet to be explored. The complete M5NR identifiers and core metagenomes are available (Supplementary Table S13).
Describing the tomato core microbiome and metagenome under multiple soils also allowed us to test the plant genotype filtering effect, evaluating selected microbes in diverse environments. With the current advances in synthetic biology, the tomato core metagenome could lead to a tomato root metagenomic chassis. This core metagenome could lead to microbe-complemented plant breeding programs aiming to reduce and optimize fertilizer use while increasing plant resilience, such as that observed in ruderal plants. Further possibilities could be the recovery of the domesticated missed root microbes from wild plants.
By using 16 geochemically diverse soils as microbial inputs for root colonization, we discarded the role of soil as the major structuring factor of root microbial communities, particularly of their coding genes. Further work is needed for detecting other environmental microbe sources than the soil for rhizosphere metagenomic diversity. Weather-dependent ruderal plant roots are a nutrient and moisture oasis for soil microbial communities with a higher taxonomic α-diversity. The tomato root microbiome followed the two-step model of microbiome acquisition. The reduced total protein number, along with significant enrichments in the tomato root metagenomes compared to ruderals and soils, suggests a tomato rhizosphere specialization and a possible domestication trade-off. Plants had been domesticated since the Neolithic age some 10,000 years ago (Purugganan and Fuller, 2009), and genomic changes in microbes linked to domestication processes have been documented (genome reduction, insertion sequences, and transposition expansions), such as the enriched genes found in RT (Mira et al., 2006). Our experimental setup showed that tomato enriched plant-microbe interaction genes (Figure 6). Altogether, our results show that tomato roots have a convergent, genotype driven, and reduced microbiome compared to their source soils, following the two-step selection model for the root microbiome. This is contrary to the ruderal plants, which exhibit a larger microbiome diversity than their soils, not following the two-step model.
Materials and Methods
Soil and Local Plant Roots Sampling
Edaphological charts were used to locate eight different soil groups, according to the United Nations FAO classification (IUSS Working Group WRB, 2015) from 16 different geographic locations described in Figure 1A and Table 1. In each location, 0.09 m1 quadrats were placed, and duplicate root samples were taken from the quadrant dominating plant species and the soil below them. We collected 2 kg of each soil into sterile plastic bags for the common garden experiment and biogeochemical analysis. All soil samples were taken from a depth not larger than 10 cm. The soil was kept at 4°C in a darkroom until greenhouse experiments were conducted 1 month later. In situ soils were collected for each soil group, poured into duplicate sterile centrifuge tubes (50 mL volume), and immediately field frozen in liquid nitrogen until storage into a −80°C freezer metagenomic DNA extraction.
Common Garden Experiment, Harvesting, and Sample Collection
The tomato seeds used were Solanum lycopersicum L. Cv. Río grande (Sun Seeds, Parma, ID, United States). Seeds were surface disinfected in 70% ethanol for 1 min, followed by a wash in 2.5% NaOCl for 2 min, and rinsed with sterile distilled water. Seeds were germinated in 1% agar for 96 h in a dark growth chamber at 27°C. Sprouts were aseptically transplanted into duplicated pots filled with the collected soils, two plants per pot were transplanted, summing four biological replicates; additionally, pots with each soil were prepared without plants which served as a control to track the changes in the soil microbiome composition due to the treatment applied in the greenhouse lacking the influence of plant development (US; Figure 1C). Pots were set in the greenhouse randomly, and plants were watered with tap water every other day and harvested after 60 days of growth. All soil samples (Figure 1) were collected in 50 mL sterile tubes and frozen at −80°C until metagenomic DNA extraction. Roots were separated from shoots to collect rhizosphere and endosphere samples by removing loose soil, followed by a washing and ultrasound procedure in 1X PBS buffer (137 mM NaCl; 2.7 mM KCl; 10 mM Na2HPO4; 1.8 mM KH2PO4) as described before (Lundberg et al., 2012). Tomato rhizosphere and endosphere metagenomic pellets were recovered through centrifugation (50mL tubes centrifuged at 1,300 g during10 min). Roots and shoots were oven-dried at 60°C for 24 h to measure plant biomass production. Due to low DNA extraction efficiency by this method in ruderal plant roots, they were cut and separated into ten 1.5 mL tubes, which received the same treatment as the 50 mL tubes. All sample pellets were frozen and kept −80°C until metagenomic DNA extraction.
Soil Geochemical Analyses
Initial and final soils were oven-dried for 24 h at 70°C. The pH was measured in deionized water (1:4 w:v) with a Corning digital pH meter. Total carbon was measured by coulometric combustion detection (Huffman, 1977) with a Total Carbon Analyzer (UIC Mod. CM 5012; Chicago, IL, United States). Total nitrogen was determined by a semi-Kjeldahl method and phosphorus by the molybdate colorimetric method after ascorbic acid reduction (Murphy and Riley, 1962) using a Bran-Luebbe Auto Analyzer III (Norderstedt, Germany). The Lang’s aridity index (Lang, 1920) of each site was calculated using historical data of mean annual precipitation and temperature for each sampling location, and data was consulted at the Atmospheric Sciences Center2 of UNAM. Non-metric multidimensional scaling (NMDS) of the samples was calculated with the geochemical data using the metaMDS function in the vegan R package (Oksanen et al., 2015) and plotted with ggplot2 (Wickham, 2009). Detailed statistical and bioinformatic methods are available at Github2.
Metagenomic DNA Processing and Massive Sequencing
The metagenomic DNA of all samples was extracted using the Mobio PowerSoil DNA extraction kit (MoBio, Carlsbad, CA, United States), following the manufacturer’s instructions. Briefly, for soils, ∼0.25 g were used for the extraction, for rhizosphere and endosphere pellets collected after washing and sonication of the roots were used respectively, as previously described (Lundberg et al., 2012). Then, the Mobio protocol was slightly modified to get extra DNA by heating the C6 elution solution to 60°C before eluting the DNA, and two 30 μL elutions were performed on the same spin filter. The same DNA was used for both amplicon and whole metagenome shotgun sequencing.
PCR amplification of the 16S rRNA gene was performed in duplicates, followed by the Illumina® MiSeq protocol for 16S metagenomic sequencing library preparation (Illumina 2013) using the 341F/805R primer pair targeting the V3-V4 regions with the Illumina sequencing adaptors in 5′ (341F: 5′-CCTACGGGNGGCWGCAG-3′; 805R: 5′-ACTACHVGGGTATCTAATCC 3′). PCR reactions were performed in a 20 μL volume, consisting of 0.16 μL Pfx polymerase (0.02U/μL) (Invitrogen, Thermo Fisher Scientific, Waltham, MA) 2μL buffer, 3 μL enhancer, 1.2 μL of each primer (5μM), 1.6 μL dNTPs (2.5 mM), 0.6 μL Mg2S04 (1.5μM), 9.2 μL PCR grade water and 2 μL DNA template. The PCR program for amplification was 95°C for 3 min, followed by five cycles of 94°C for 30 s, 55°C for 30 s, 68°C for the 30 s, followed by 25 cycles of 94°C for 5 s and 68°C for 30 s. The duplicate amplification products of each sample were pooled and purified with the SV Wizard PCR Purification kit (Promega, Madison, WI) following the manufacturer’s instructions. Amplicon library sequencing was done in the Illumina® MiSeq platform in a 2 × 300 paired-end configuration at the University Unit of Massive Sequencing and Bioinformatics3 of the Biotechnology Institute, UNAM, Mexico. Whole shotgun metagenome sequencing libraries were prepared using the Truseq PCR free library preparation kit for selected initial soils, ruderal plants, and S. lycopersicum rhizospheres, which were then sequenced with an Illumina HiSeq 2000 in a 2 × 100 bp reads, at the facilities of Macrogen, Korea4.
16S rRNA Gene Amplicon Sequence Analysis
The 16S rRNA protocol used in this work had been used previously and is detailed at GitHub5 (Alcaraz et al., 2018). In summary, gene amplicon libraries were quality inspected using Fastx Toolkit6 and trimmed to a 250 bp length. Trimmed paired-end reads were assembled using Pandaseq (Masella et al., 2012). The assembly was performed using a minimum overlap of 15 bp, the minimum output length of 250 bp, the maximum output length of 470 bp, and an alignment threshold of 95%. Finally, assembled sequences were filtered using a minimum PHRED score of 20. All the samples were concatenated and clustered into OTUs, using a 97% identity threshold with cd-hit-est (Li and Godzik, 2006). The taxonomy of representative sequences was assigned against Greengenes (De Santis et al., 2006) database with QIIME’s scripts (Caporaso et al., 2010). After taxonomic classification, singletons, and chimeras were removed as well as sequences corresponding to the mitochondria, chloroplast, and unassigned hits were filtered out. Finally, the representative OTU sequences were aligned with SSU-align (Nawrocki et al., 2009), and a phylogenetic tree was constructed with Fasttree (Price et al., 2009). Detailed statistical and bioinformatic methods are available at Github (See text footnote 2).
Metagenomic Shotgun Sequence Analysis
The quality control of whole shotgun metagenome sequences was done using Trimmomatic (Bolger et al., 2014), only paired-end matched reads were used for subsequent analysis. We filtered out metagenomic reads matching S. lycopersicum genome (NCBI BioProject: PRJNA66163), while soils and ruderal plants rhizosphere libraries were filtered against the Oryza sativa genome (NCBI BioProject: PRJNA122) with Bowtie2 (Langmead and Salzberg, 2012). Quality and host filtered metagenomic libraries were used to assemble individual metagenomes with metaSPADES (Nurk et al., 2017). High-quality reads were mapped against the metaSPADES contigs, and unmapped reads were subjected to a second assembly with Velvet (Zerbino and Birney, 2008). The resulting contigs from both assemblies were merged and used to predict ORFs and coding proteins with Prodigal (Hyatt et al., 2010). Annotation of predicted proteins was made against the M5NR database (Wilke et al., 2012) using DIAMOND (Buchfink et al., 2015) with the following parameters -f6 -e 1e-10 -k 10 -p1, retrieving Refseq (Pruitt et al., 2007) and SEED subsystems (Overbeek et al., 2014) annotations from M5NR matched identifiers. The abundance of each predicted protein was calculated by mapping the high-quality reads against the predicted ORFs with Bowtie2. All the predicted proteins were clustered using cd-hit (Li and Godzik, 2006) using a 70% identity threshold, and they were parsed into a biom formatted matrix, used as input for sets comparison using UpSetR (Conway et al., 2017). The binning of whole shotgun metagenomic reads was performed with Kaiju (Menzel et al., 2016). Detailed statistical and bioinformatic methods are available at Github2.
Diversity Analysis
The α and β-diversity of soils, rhizospheres, and endospheres from each site were calculated with phyloseq (McMurdie and Holmes, 2013), and vegan R (Oksanen et al., 2015) packages. Taxonomic α-diversity was assessed using a weighted Unifrac (Lozupone et al., 2006) distance matrix. Microbiomes were then hierarchically clustered with the hclust method using complete distances and clustering evaluated through the ANOSIM function. OTUs were clustered at the genus level, and Venn diagrams were used to compare the complete root system (rhizosphere + endosphere) microbiome composition of ruderal plants, S. lycopersicum, and initial soils using a web Venn diagram calculator7. Unique soil, ruderal plants, S. lycopersicum, and the ruderal plants-S. lycopersicum intersection taxonomic profiles were described at the phylum level based on OTU abundances.
Metabolic α-diversity was estimated through a constrained analysis of principal coordinates (CAP) analysis using Bray-Curtis dissimilarity based on the total abundance of predicted proteins. Differential OTUs and protein abundances comparing rhizospheres or endosphere against soils were calculated using DESeq2 (Love et al., 2014) with a Wald statistical test and a local fit of the data. For 16S rRNA data, OTUs were considered differentially abundant between groups using a p < 0.01, for metagenome predicted proteins, a p < 0.001 was used as a cut-off. Their 16S rRNA matches identified the collected ruderal plant species to NCBI’s NR database representing a variety of 5 different plant families, mainly grasses (Poaceae N = 10, Asteraceae N = 3, Lamiaceae N = 1, Fabaceae N = 1, and Fagales N = 1; Supplementary Figure S1). Detailed statistical and bioinformatic methods are available at Github2.
Data Availability Statement
The datasets generated for this study can be found in the NCBI SRA: https://www.ncbi.nlm.nih.gov/sra/PRJNA603590, https://www.ncbi.nlm.nih.gov/sra/PRJNA603586, https://www.ncbi.nlm.nih.gov/sra/PRJNA603603.
Author Contributions
HB, LS-G, MP, RC-O, FG-O, and LA conceived and designed the research. HB and LA wrote the manuscript. HB, SM-S, MP, and LA performed the field sampling. HB and SM-S performed the experiments. HB, SM-S, CH, MR, and LA analyzed the data. MP, RC-O, FG-O, and LA provided the reagents and material. HB, MP, and LA created the figures. All the authors read and approved the manuscript.
Conflict of Interest
The authors declare that the research was conducted in the absence of any commercial or financial relationships that could be construed as a potential conflict of interest.
Funding
HB was a doctoral student of Programa de Maestría y Doctorado en Ciencias Bioquímicas, Universidad Nacional Autónoma de México (UNAM); this study was performed in partial fulfillment of the requirements for the Ph.D. degree and received a fellowship from CONACYT (CVU-621156). CH, MR, and SM-S had graduate student fellowships from CONACyT. LA received funding from DGAPA-PAPIIT-UNAM grant IN221420 and SEP-CONACyT Ciencia Básica 237387. The funders had no role in study design, data collection, and analysis, decision to publish, or preparation of the manuscript.
Acknowledgments
We want to thank Rodrigo Velázquez Durán for his technical support with the soil physicochemical properties characterization. Selene Molina and Jazmín Blaz for their help in sample processing. Francisco González, who helped in the sampling of soils, and ruderal plants’ roots. We also thank Rodrigo García Herrera at LANCIS-Instituto de Ecología, UNAM, for his support in HPC computing.
Supplementary Material
The Supplementary Material for this article can be found online at: https://www.frontiersin.org/articles/10.3389/fmicb.2020.542742/full#supplementary-material
Complete Supplementary Material available at: https://github.com/genomica-fciencias-unam/Barajas-2020.
Abbreviations
EC, ruderal plant endosphere; ECT, tomato endosphere; FS, final soil; RT, tomato rhizosphere; RZ, ruderal plant rhizosphere; SI, Initial source soil; US, control unplanted soil.
Footnotes
- ^ https://github.com/genomica-fciencias-unam/Barajas-2020
- ^ http://uniatmos.atmosfera.unam.mx/ACDM/
- ^ http://www.uusmd.unam.mx
- ^ https://www.macrogen.com
- ^ https://genomica-fciencias-unam.github.io/SOP/
- ^ http://hannonlab.cshl.edu/fastx_toolkit/
- ^ https://bioinformatics.psb.ugent.be/webtools/Venn/
References
Agler, M. T., Ruhe, J., Kroll, S., Morhenn, C., Kim, S.-T., Weigel, D., et al. (2016). Microbial hub taxa link host and abiotic factors to plant microbiome variation. PLoS Biol. 14:e1002352. doi: 10.1371/journal.pbio.1002352
Alcaraz, L. D., Martínez-Sánchez, S., Torres, I., Ibarra-Laclette, E., and Herrera-Estrella, L. (2016). The metagenome of Utricularia gibba’s traps: into the microbial input to a carnivorous plant. PLoS One 11:e0148979. doi: 10.1371/journal.pone.0148979
Alcaraz, L. D., Peimbert, M., Barajas, H. R., Dorantes-Acosta, A. E., Bowman, J. L., and Arteaga-Vázquez, M. A. (2018). Marchantia liverworts as a proxy to plants’basal microbiomes. Sci. Rep. 8:12712.
Anderson, D. M., Sato, H., Dirck, A. T., Feix, J. B., and Frank, D. W. (2015). Ubiquitin activates patatin-like phospholipases from multiple bacterial species. J. Bacteriol. 197, 529–541. doi: 10.1128/jb.02402-14
Bais, H. P., Weir, T. L., Perry, L. G., Gilroy, S., and Vivanco, J. M. (2006). The role of root exudates in rhizosphere interactions with plants and other organisms. Annu. Rev. Plant Biol. 57, 233–266. doi: 10.1146/annurev.arplant.57.032905.105159
Banerji, S., and Flieger, A. (2004). Patatin-like proteins: a new family of lipolytic enzymes present in bacteria? Microbiology 150, 522–525. doi: 10.1099/mic.0.26957-0
Bártová, V., Bárta, J., and Jarošová, M. (2019). Antifungal and antimicrobial proteins and peptides of potato (Solanum tuberosum L.) tubers and their applications. Appl. Microbiol. Biotechnol. 103, 5533–5547. doi: 10.1007/s00253-019-09887-9
Baudoin, E., Benizri, E., and Guckert, A. (2002). Impact of growth stage on the bacterial community structure along maize roots, as determined by metabolic and genetic fingerprinting. Appl. Soil Ecol. 19, 135–145. doi: 10.1016/s0929-1393(01)00185-8
Berendsen, R. L., Pieterse, C. M. J., and Bakker, P. A. H. M. (2012). The rhizosphere microbiome and plant health. Trends Plant Sci. 17, 478–486. doi: 10.1016/j.tplants.2012.04.001
Bolger, A. M., Lohse, M., and Usadel, B. (2014). Trimmomatic: a flexible trimmer for Illumina sequence data. Bioinformatics 30, 2114–2120. doi: 10.1093/bioinformatics/btu170
Bouffaud, M. L., Poirier, M. A., Muller, D., and Moënne-Loccoz, Y. (2014). Root microbiome relates to plant host evolution in maize and other Poaceae. Environ. Microbiol. 16, 2804–2814. doi: 10.1111/1462-2920.12442
Boyd, E. S., and Peters, J. W. (2013). New insights into the evolutionary history of biological nitrogen fixation. Front. Microbiol. 4:201. doi: 10.3389/fmicb.2013.00201
Brader, G., Compant, S., Mitter, B., Trognitz, F., and Sessitsch, A. (2014). Metabolic potential of endophytic bacteria. Curr. Opin. Biotechnol. 27, 30–37. doi: 10.1016/j.copbio.2013.09.012
Buchfink, B., Xie, C., and Huson, D. H. (2015). Fast and sensitive protein alignment using DIAMOND. Nat. Methods 12, 59–60. doi: 10.1038/nmeth.3176
Bulgarelli, D., Garrido-Oter, R., Münch, P. C., Weiman, A., Dröge, J., Pan, Y., et al. (2015). Structure and function of the bacterial root microbiota in wild and domesticated barley. Cell Host Microbe 17, 392–403. doi: 10.1016/j.chom.2015.01.011
Bulgarelli, D., Rott, M., Schlaeppi, K., Ver Loren van Themaat, E., Ahmadinejad, N., Assenza, F., et al. (2012). Revealing structure and assembly cues for Arabidopsis root-inhabiting bacterial microbiota. Nature 488, 91–95. doi: 10.1038/nature11336
Bulgarelli, D., Schlaeppi, K., Spaepen, S., van Themaat, E. V. L., and Schulze-Lefert, P. (2013). Structure and functions of the bacterial microbiota of plants. Annu. Rev. Plant Biol. 64, 807–838. doi: 10.1146/annurev-arplant-050312-120106
Canarini, A., Kaiser, C., Merchant, A., Richter, A., and Wanek, W. (2019). Root exudation of primary metabolites: mechanisms and their roles in plant responses to environmental stimuli. Front. Plant Sci. 10:157. doi: 10.3389/fpls.2019.00157
Caporaso, J. G., Kuczynski, J., Stombaugh, J., Bittinger, K., Bushman, F. D., Costello, E. K., et al. (2010). QIIME allows analysis of high-throughput community sequencing data. Nat. Methods 7, 335–336. doi: 10.1038/nmeth.f.303
Chen, H., Jogler, M., Rohde, M., Klenk, H. P., Busse, H. J., Tindall, B. J., et al. (2013). Sphingobium limneticum sp. nov. and Sphingobium boeckii sp. nov., two freshwater planktonic members of the family Sphingomonadaceae, and reclassification of Sphingomonas suberifaciens as Sphingobium suberifaciens comb. nov. Int. J. Syst. Evol. Microbiol. 63, 735–743. doi: 10.1099/ijs.0.040105-0
Cheng, Z., Lei, S., Li, Y., Huang, W., Ma, R., Xiong, J., et al. (2020). Revealing the variation and stability of bacterial communities in tomato rhizosphere microbiota. Microorganisms 8:170. doi: 10.3390/microorganisms8020170
Compant, S., Clément, C., and Sessitsch, A. (2010). Plant growth-promoting bacteria in the rhizo- and endosphere of plants: their role, colonization, mechanisms involved and prospects for utilization. Soil Biol. Biochem. 42, 669–678. doi: 10.1016/j.soilbio.2009.11.024
Conway, J. R., Lex, A., and Gehlenborg, N. (2017). UpSetR: an R package for the visualization of intersecting sets and their properties. Bioinformatics 33, 2938–2940. doi: 10.1093/bioinformatics/btx364
Cordero, J., de Freitas, J. R., and Germida, J. J. (2020). Bacterial microbiome associated with the rhizosphere and root interior of crops in Saskatchewan, Canada. Can. J. Microbiol. 66, 71–85. doi: 10.1139/cjm-2019-0330
Correa-Galeote, D., Bedmar, E. J., and Arone, G. J. (2018). Maize endophytic bacterial diversity as affected by soil cultivation history. Front. Microbiol. 9:484. doi: 10.3389/fmicb.2018.00484
Costa, R., Götz, M., Mrotzek, N., Lottmann, J., Berg, G., and Smalla, K. (2006). Effects of site and plant species on rhizosphere community structure as revealed by molecular analysis of microbial guilds. FEMS Microbiol. Ecol. 56, 236–249. doi: 10.1111/j.1574-6941.2005.00026.x
Cruz-Ramos, H., Glaser, P., Wray, L. V., and Fisher, S. H. (1997). The Bacillus subtilis ureABC operon. J. Bacteriol. 179, 3371–3373. doi: 10.1128/JB.179.10.3371-3373.1997
De Santis, T. Z., Hugenholtz, P., Larsen, N., Rojas, M., Brodie, E. L., Keller, K., et al. (2006). Greengenes, a chimera-checked 16S rRNA gene database and workbench compatible with ARB. Appl. Environ. Microbiol. 72, 5069–5072. doi: 10.1128/aem.03006-05
de Weert, S., Vermeiren, H., Mulders, I. H. M., Kuiper, I., Hendrickx, N., Bloemberg, G. V., et al. (2002). Flagella-driven chemotaxis towards exudate components is an important trait for tomato root colonization by Pseudomonas fluorescens. Mol. Plant Microbe Interact. 15, 1173–1180. doi: 10.1094/MPMI.2002.15.11.1173
Del Medico, L., Cerletti, D., Schächle, P., Christen, M., and Christen, B. (2020). The type IV pilin PilA couples surface attachment and cell-cycle initiation in Caulobacter crescentus. Proc. Natl. Acad. Sci. U.S.A. 117, 9546–9553. doi: 10.1073/pnas.1920143117
Delmont, T. O., and Eren, E. M. (2018). Linking pangenomes and metagenomes: the Prochlorococcus metapangenome. PeerJ 6:e4320. doi: 10.7717/peerj.4320
Doebley, J. F., Gaut, B. S., and Smith, B. D. (2006). The molecular genetics of crop domestication. Cell 157, 1309–1321. doi: 10.1016/j.cell.2006.12.006
Edwards, J., Johnson, C., Santos-Medellín, C., Lurie, E., Podishetty, N. K., Bhatnagar, S., et al. (2015). Structure, variation, and assembly of the root-associated microbiomes of rice. Proc. Natl. Acad. Sci. U.S.A. 112, E911–E920. doi: 10.1073/pnas.1414592112
El-Tarabily, K. A., Nassar, A. H., Hardy, G. E. S. J., and Sivasithamparam, K. (2009). Plant growth promotion and biological control of Pythium aphanidermatum, a pathogen of cucumber, by endophytic actinomycetes. J. Appl. Microbiol. 106, 13–26. doi: 10.1111/j.1365-2672.2008.03926.x
Fierer, N., and Jackson, R. B. (2006). The diversity and biogeography of soil bacterial communities. Proc. Natl. Acad. Sci. U.S.A. 103, 626–631. doi: 10.1073/pnas.0507535103
Finck-Barbançon, V., Goranson, J., Zhu, L., Sawa, T., Wiener-Kronish, J. P., Fleiszig, S. M. J., et al. (1997). ExoU expression by Pseudomonas aeruginosa correlates with acute cytotoxicity and epithelial injury. Mol. Microbiol. 25, 547–557. doi: 10.1046/j.1365-2958.1997.4891851.x
Fitzpatrick, C. R., Copeland, J., Wang, P. W., Guttman, D. S., Kotanen, P. M., and Johnson, M. T. J. (2018). Assembly and ecological function of the root microbiome across angiosperm plant species. Proc. Natl. Acad. Sci. U.S.A. 115, E1157–E1165. doi: 10.1073/pnas.1717617115
Fowler, J. H., Narváez-Vásquez, J., Aromdee, D. N., Pautot, V., Holzer, F. M., and Walling, L. L. (2009). Leucine aminopeptidase regulates defense and wound signaling in tomato downstream of jasmonic acid. Plant Cell 21, 1239–1251. doi: 10.1105/tpc.108.065029
Gao, J., Luo, Y., Wei, Y., Huang, Y., Zhang, H., He, W., et al. (2019). Effect of aridity and dune type on rhizosphere soil bacterial communities of Caragana microphylla in desert regions of northern China. PLoS One 14:e0224195. doi: 10.1371/journal.pone.0224195
Hartmann, A., Rothballer, M., and Schmid, M. (2008). Lorenz Hiltner, a pioneer in rhizosphere microbial ecology and soil bacteriology research. Plant Soil 312, 7–14. doi: 10.1007/s11104-007-9514-z
Hiltner, L. (1904). Über neuere Erfahrungen und Probleme auf dem Gebiete der Bodenbakteriologie unter besonderer Berücksichtigung der Gründüngung und Brache. Arbeit. Deut. Landw. Ges. 98, 59–78.
Huffman, E. W. D. (1977). Performance of a new automatic carbon dioxide coulometer. Microchem. J. 22, 567–573. doi: 10.1016/0026-265X(77)90128-X
Hyatt, D., Chen, G. L., LoCascio, P. F., Land, M. L., Larimer, F. W., and Hauser, L. J. (2010). Prodigal: prokaryotic gene recognition and translation initiation site identification. BMC Bioinformatics 11:119. doi: 10.1186/1471-2105-11-119
Inceoğlu, Ö., Al-Soud, W. A., Salles, J. F., Semenov, A. V., and van Elsas, J. D. (2011). Comparative analysis of bacterial communities in a potato field as determined by pyrosequencing. PLoS One 6:e23321. doi: 10.1371/journal.pone.0023321
INEGI (2014). Conjunto de Datos Vectoriales Edafológico, Escala 1:250000 Serie II. (Continuo Nacional), Escala: 1:250000, 2nd Edn. Aguascalientes: Instituto Nacional de Estadística y Geografía.
IUSS Working Group WRB (2015). World Reference Base for Soil Resources 2014, Update 2015. International Soil Classification System for Naming Soils and Creating Legends for Soil Maps. World Soil Resources Reports No. 106. Rome: FAO.
Lang, R. (1920). Verwitterung und Bodenbildung als Einfuehrung in die Bodenkunde. Stuttgart: Schweizerbart Science Publishers.
Langmead, B., and Salzberg, S. L. (2012). Fast gapped-read alignment with Bowtie 2. Nat. Methods 9, 357–359. doi: 10.1038/nmeth.1923
Larousse, M., Rancurel, C., Syska, C., Palero, F., Etienne, C., Industri, B., et al. (2017). Tomato root microbiota and Phytophthora parasitica-associated disease. Microbiome 5:56.
Lauber, C. L., Hamady, M., Knight, R., and Fierer, N. (2009). Pyrosequencing-based assessment of soil pH as a predictor of soil bacterial community structure at the continental scale. Appl. Environ. Microbiol. 75, 5111–5120. doi: 10.1128/aem.00335-09
Lee, S. A., Kim, Y., Kim, J. M., Chu, B., Joa, J.-H., Sang, M. K., et al. (2019). A preliminary examination of bacterial, archaeal, and fungal communities inhabiting different rhizocompartments of tomato plants under real-world environments. Sci. Rep. 9:9300. doi: 10.1038/s41598-019-45660-8
Lee, S. A., Park, J., Chu, B., Kim, J. M., Joa, J. H., Sang, M. K., et al. (2016). Comparative analysis of bacterial diversity in the rhizosphere of tomato by culture-dependent and -independent approaches. J. Microbiol. 54, 823–831. doi: 10.1007/s12275-016-6410-3
Li, W., and Godzik, A. (2006). Cd-hit: a fast program for clustering and comparing large sets of protein or nucleotide sequences. Bioinformatics 22, 1658–1659. doi: 10.1093/bioinformatics/btl158
Liu, R., and Ochman, H. (2007). Origins of flagellar gene: operons and secondary flagellar systems. J. Bacteriol. 189, 7098–7104. doi: 10.1128/jb.00643-07
Love, M. I., Huber, W., and Anders, S. (2014). Moderated estimation of fold change and dispersion for RNA-seq data with DESeq2. Genome Biol. 15:550.
Lozupone, C., Hamady, M., and Knight, R. (2006). UniFrac - an online tool for comparing microbial community diversity in a phylogenetic context. BMC Bioinformatics 7:371. doi: 10.1186/1471-2105-7-371
Lundberg, D. S., Lebeis, S. L., Paredes, S. H., Yourstone, S., Gehring, J., Malfatti, S., et al. (2012). Defining the core Arabidopsis thaliana root microbiome. Nature 488, 86–90. doi: 10.1038/nature11237
Ma, P., Patching, S. G., Ivanova, E., Baldwin, J. M., Sharples, D., Baldwin, S. A., et al. (2016). Allantoin transport protein, PucI, from Bacillus subtilis: evolutionary relationships, amplified expression, activity and specificity. Microbiology 162, 823–836. doi: 10.1099/mic.0.000266
Männistö, M. K., Tiirola, M., and Häggblom, M. M. (2007). Bacterial communities in arctic fields of Finnish lapland are stable but highly pH-dependent. FEMS Microbiol. Ecol. 59, 452–465. doi: 10.1111/j.1574-6941.2006.00232.x
Marasco, R., Mosqueira, M. J., Fusi, M., Ramond, J.-B., Merlino, G., Booth, J. M., et al. (2018). Rhizosheath microbial community assembly of sympatric desert speargrasses is independent of the plant host. Microbiome 6:215. doi: 10.1186/s40168-018-0597-y
Marschner, P., Crowley, D., and Yang, C. H. (2004). Development of specific rhizosphere bacterial communities in relation to plant species, nutrition and soil type. Plant Soil 261, 199–208. doi: 10.1023/B:PLSO.0000035569.80747.c5
Masella, A. P., Bartram, A. K., Truszkowski, J. M., Brown, D. G., and Neufeld, J. D. (2012). PANDAseq: paired-end assembler for Illumina sequences. BMC Bioinformatics 13:31. doi: 10.1186/1471-2105-13-31
McMurdie, P. J., and Holmes, S. (2013). Phyloseq: an R Package for reproducible interactive analysis and graphics of microbiome census data. PLoS One 8:e61217. doi: 10.1371/journal.pone.0061217
Menzel, P., Ng, K. L., and Krogh, A. (2016). Fast and sensitive taxonomic classification for metagenomics with Kaiju. Nat. Commun. 7:11257. doi: 10.1038/ncomms11257
Micallef, S. A., Shiaris, M. P., and Colón-Carmona, A. (2009). Influence of Arabidopsis thaliana accessions on rhizobacterial communities and natural variation in root exudates. J. Exp. Bot. 60, 1729–1742. doi: 10.1093/jxb/erp053
Mira, A., Pushker, R., and Rodríguez-Valera, F. (2006). The Neolithic revolution of bacterial genomes. Trends Microbiol. 14, 200–206. doi: 10.1016/j.tim.2006.03.001
Murphy, J., and Riley, J. P. (1962). A modified single solution method for the determination of phosphate in natural waters. Anal. Chim. Acta 27, 31–36. doi: 10.1016/s0003-2670(00)88444-5
Nawrocki, E. P., Kolbe, D. L., and Eddy, S. R. (2009). Infernal 1.0: inference of RNA alignments. Bioinformatics 25, 1335–1337. doi: 10.1093/bioinformatics/btp157
Naylor, D., Degraaf, S., Purdom, E., and Coleman-Derr, D. (2017). Drought and host selection influence bacterial community dynamics in the grass root microbiome. ISME J. 11, 2691–2704. doi: 10.1038/ismej.2017.118
Neilson, J. W., Califf, K., Cardona, C., Copeland, A., van Treuren, W., Josephson, K. L., et al. (2017). Significant impacts of increasing aridity on the arid soil microbiome. mSystems 2:e00195-16.
Nurk, S., Meleshko, D., Korobeynikov, A., and Pevzner, P. A. (2017). MetaSPAdes: a new versatile metagenomic assembler. Genome Res. 27, 824–834. doi: 10.1101/gr.213959.116
Oksanen, J., Blanchet, F. G., and Kindt, R. (2015). Vegan: Community Ecology Package. R Package Version 2.3-0. Available online at: https://cran.r-project.org/web/packages/vegan/index.html (accessed March 4, 2015).
Ong, C. J., Wong, M. L., and Smit, J. (1990). Attachment of the adhesive holdfast organelle to the cellular stalk of Caulobacter crescentus. J. Bacteriol. 172, 1448–1456. doi: 10.1128/jb.172.3.1448-1456.1990
Overbeek, R., Olson, R., Pusch, G. D., Olsen, G. J., Davis, J. J., Disz, T., et al. (2014). The SEED and the rapid annotation of microbial genomes using subsystems technology (RAST). Nucleic Acids Res. 42, 206–214. doi: 10.1093/nar/gkt1226
Panke-Buisse, K., Poole, A. C., Goodrich, J. K., Ley, R. E., and Kao-Kniffin, J. (2015). Selection on soil microbiomes reveals reproducible impacts on plant function. ISME J. 9, 980–989. doi: 10.1038/ismej.2014.196
Pautot, V., Holzer, F. M., Chaufaux, J., and Walling, L. L. (2001). The induction of tomato leucine aminopeptidase genes (LapA) after Pseudomonas syringae pv. tomato infection is primarily a wound response triggered by coronatine. Mol. Plant Microbe Interact. 14, 214–224. doi: 10.1094/MPMI.2001.14.2.214
Pautot, V., Holzer, F. M., Reisch, B., and Walling, L. L. (1993). Leucine aminopeptidase: an inducible component of the defense response in Lycopersicon esculentum (tomato). Proc. Natl. Acad. Sci. U.S.A. 90, 9906–9910. doi: 10.1073/pnas.90.21.9906
Peiffer, J. A., Spor, A., Koren, O., Jin, Z., Tringe, S. G., Dangl, J. L., et al. (2013). Diversity and heritability of the maize rhizosphere microbiome under field conditions. Proc. Natl. Acad. Sci. U.S.A. 110, 6548–6553. doi: 10.1073/pnas.1302837110
Pérez-Jaramillo, J. E., Carrión, V. J., de Hollander, M., and Raaijmakers, J. M. (2018). The wild side of plant microbiomes. Microbiome 6:143. doi: 10.1186/s40168-018-0519-z
Phillips, R. M., Six, D. A., Dennis, E. A., and Ghosh, P. (2003). In vivo phospholipase activity of the Pseudomonas aeruginosa cytotoxin ExoU and protection of mammalian cells with phospholipase A 2 inhibitors. J. Biol. Chem. 278, 41326–41332. doi: 10.1074/jbc.M302472200
Pii, Y., Borruso, L., Brusetti, L., Crecchio, C., Cesco, S., and Mimmo, T. (2016). The interaction between iron nutrition, plant species and soil type shapes the rhizosphere microbiome. Plant Physiol. Biochem. 99, 39–48. doi: 10.1016/j.plaphy.2015.12.002
Poindexter, J. S. (1981). The caulobacters: ubiquitous unusual bacteria. Microbiol. Rev. 45, 123–179. doi: 10.1128/mmbr.45.1.123-179.1981
Price, M. N., Dehal, P. S., and Arkin, A. P. (2009). FastTree: computing large minimum evolution trees with profiles instead of a distance matrix. Mol. Biol. Evol. 26, 1641–1650. doi: 10.1093/molbev/msp077
Pruitt, K. D., Tatusova, T., and Maglott, D. R. (2007). NCBI reference sequences (RefSeq): a curated non-redundant sequence database of genomes, transcripts and proteins. Nucleic Acids Res. 35(Suppl. 1), D61–D65. doi: 10.1093/nar/gkl842
Purugganan, M. D., and Fuller, D. Q. (2009). The nature of selection during plant domestication. Nature 457, 843–848. doi: 10.1038/nature07895
Ramin, K. I., and Allison, S. D. (2019). Bacterial trade-offs in growth rate and extracellular enzymes. Front. Microbiol. 10:2956. doi: 10.3389/fmicb.2019.02956
Redford, A. J., Bowers, R. M., Knight, R., Linhart, Y., and Fierer, N. (2010). The ecology of the phyllosphere: geographic and phylogenetic variability in the distribution of bacteria on tree leaves. Environ. Microbiol. 12, 2885–2893. doi: 10.1111/j.1462-2920.2010.02258.x
Roesch, L. F. W., Fulthorpe, R. R., Riva, A., Casella, G., Hadwin, A. K. M., Kent, A. D., et al. (2007). Pyrosequencing enumerates and contrasts soil microbial diversity. ISME J. 1, 283–290. doi: 10.1038/ismej.2007.53
Sato, H., Frank, D. W., Hillard, C. J., Feix, J. B., Pankhaniya, R. R., Moriyama, K., et al. (2003). The mechanism of action of the Pseudomonas aeruginosa-encoded type III cytotoxin, ExoU. EMBO J. 22, 2959–2969. doi: 10.1093/emboj/cdg290
Satour, M. M., and Butler, E. E. (1967). A root and crown rot of tomato caused by Phytophthora capsici and P. parasitica. Phytopathology 57, 510–515.
Schlaeppi, K., Dombrowski, N., Oter, R. G., Ver Loren Van Themaat, E., and Schulze-Lefert, P. (2014). Quantitative divergence of the bacterial root microbiota in Arabidopsis thaliana relatives. Proc. Natl. Acad. Sci. U.S.A. 111, 585–592. doi: 10.1073/pnas.1321597111
Schreiter, S., Ding, G. C., Heuer, H., Neumann, G., Sandmann, M., Grosch, R., et al. (2014). Effect of the soil type on the microbiome in the rhizosphere of field-grown lettuce. Front. Microbiol. 5:144. doi: 10.3389/fmicb.2014.00144
Shade, A., Jacques, M.-A., and Barret, M. (2017). Ecological patterns of seed microbiome diversity, transmission, and assembly. Curr. Opin. Microbiol. 37, 15–22. doi: 10.1016/j.mib.2017.03.010
Skerker, J. M., and Shapiro, L. (2000). Identification and cell cycle control of a novel pilus system in Caulobacter crescentus. EMBO J. 19, 3223–3234. doi: 10.1093/emboj/19.13.3223
Sreevidya, M., Gopalakrishnan, S., Kudapa, H., and Varshney, R. K. (2016). Exploring plant growth-promotion actinomycetes from vermicompost and rhizosphere soil for yield enhancement in chickpea. Braz. J. Microbiol. 47, 85–95. doi: 10.1016/j.bjm.2015.11.030
Swenson, W., Wilson, D. S., and Elias, R. (2000). Artificial ecosystem selection. Proc. Natl. Acad. Sci. U.S.A. 97, 9110–9114. doi: 10.1073/pnas.150237597
Thompson, L. R., Sanders, J. G., McDonald, D., Amir, A., Ladau, J., Locey, K. J., et al. (2017). A communal catalogue reveals Earth’s multiscale microbial diversity. Nature 551, 457–463. doi: 10.1038/nature24621
Tian, B. Y., Cao, Y., and Zhang, K. Q. (2015). Metagenomic insights into communities, functions of endophytes, and their associates with infection by root-knot nematode, Meloidogyne incognita, in tomato roots. Sci. Rep. 5:17087. doi: 10.1038/srep17087
Truyens, S., Weyens, N., Cuypers, A., and Vangronsveld, J. (2015). Bacterial seed endophytes: genera, vertical transmission and interaction with plants. Environ. Microbiol. Rep. 7, 40–50. doi: 10.1111/1758-2229.12181
Turnbull, G. A., Morgan, J. A. W., Whipps, J. M., and Saunders, J. R. (2001). The role of bacterial motility in the survival and spread of Pseudomonas fluorescens in soil and in the attachment and colonisation of wheat roots. FEMS Microbiol. Ecol. 36, 21–31. doi: 10.1111/j.1574-6941.2001.tb00822.x
van der Heijden, M. G. A., Bardgett, R. D., and Van Straalen, N. M. (2008). The unseen majority: soil microbes as drivers of plant diversity and productivity in terrestrial ecosystems. Ecol. Lett. 11, 296–310. doi: 10.1111/j.1461-0248.2007.01139.x
Watzer, B., and Forchhammer, K. (2018). Cyanophycin synthesis optimizes nitrogen utilization in the unicellular cyanobacterium Synechocystis sp. strain PCC 6803. Appl. Environ. Microbiol. 84:e01298-18.
Wheatley, R. M., and Poole, P. S. (2018). Mechanisms of bacterial attachment to roots. FEMS Microbiol. Rev. 42, 448–461. doi: 10.1093/femsre/fuy014
Wilke, A., Harrison, T., Wilkening, J., Field, D., Glass, E. M., Kyrpides, N., et al. (2012). The M5nr: a novel non-redundant database containing protein sequences and annotations from multiple sources and associated tools. BMC Bioinformatics 13:141. doi: 10.1186/1471-2105-13-141
Wissuwa, M., Mazzola, M., and Picard, C. (2009). Novel approaches in plant breeding for rhizosphere-related traits. Plant Soil 321, 409–430. doi: 10.1007/s11104-008-9693-2
Wu, H., Xiang, W., Ouyang, S., Forrester, D. I., Zhou, B., Chen, L., et al. (2019). Linkage between tree species richness and soil microbial diversity improves phosphorus bioavailability. Funct. Ecol. 33, 1549–1560. doi: 10.1111/1365-2435.13355
Yang, Z., Yang, W., Li, S., Hao, J., Su, Z., Sun, M., et al. (2016). Variation of bacterial community diversity in rhizosphere soil of sole-cropped versus intercropped Wheat field after harvest. PLoS One 11:e0150618. doi: 10.1371/journal.pone.0150618
Young, C. C., Arun, A. B., Kämpfer, P., Busse, H. J., Lai, W. A., Chen, W. M., et al. (2008). Sphingobium rhizovicinum sp. nov., isolated from rhizosphere soil of Fortunella hindsii (Champ. ex Benth.) Swingle. Int. J. Syst. Evol. Microbiol. 58, 1801–1806. doi: 10.1099/ijs.0.65564-0
Keywords: plant microbiome, soil microbiome, rhizosphere metagenomics, core metagenome, domesticated plants, ruderal plants, common garden experiment
Citation: Barajas HR, Martínez-Sánchez S, Romero MF, Álvarez CH, Servín-González L, Peimbert M, Cruz-Ortega R, García-Oliva F and Alcaraz LD (2020) Testing the Two-Step Model of Plant Root Microbiome Acquisition Under Multiple Plant Species and Soil Sources. Front. Microbiol. 11:542742. doi: 10.3389/fmicb.2020.542742
Received: 13 March 2020; Accepted: 11 September 2020;
Published: 09 October 2020.
Edited by:
Alok Kumar Srivastava, National Bureau of Agriculturally Important Microorganisms (ICAR), IndiaReviewed by:
Murali Gopal, Central Plantation Crops Research Institute (ICAR), IndiaSiu Mui Tsai, University of São Paulo, Brazil
Copyright © 2020 Barajas, Martínez-Sánchez, Romero, Álvarez, Servín-González, Peimbert, Cruz-Ortega, García-Oliva and Alcaraz. This is an open-access article distributed under the terms of the Creative Commons Attribution License (CC BY). The use, distribution or reproduction in other forums is permitted, provided the original author(s) and the copyright owner(s) are credited and that the original publication in this journal is cited, in accordance with accepted academic practice. No use, distribution or reproduction is permitted which does not comply with these terms.
*Correspondence: Luis D. Alcaraz, bGFsY2FyYXpAY2llbmNpYXMudW5hbS5teA==