- 1Guangdong Provincial Key Laboratory of Animal Molecular Design and Precise Breeding, Key Laboratory of Animal Molecular Design and Precise Breeding of Guangdong Higher Education Institutes, School of Life Sciences and Engineering, Foshan University, Foshan, China
- 2Institute of Animal Sciences, Chinese Academy of Agricultural Sciences, Beijing, China
- 3College of Animal Science, Shanxi Agricultural University, Shanxi, China
Myostatin (MSTN) functional inactivation can change the proportion of lean meat and fat content in pigs. While both genotype and microbial composition are known to affect the host phenotype, so far there has been no systematic study to detect the changes in the intestinal microbial composition and metabolome of MSTN single copy mutant pigs. Here, we used 16S rDNA sequencing and metabolome analysis to investigate how MSTN gene editing affects changes in the microbial and metabolome composition in the jejunum and the cecum of Large White pigs. Our results showed that Clostridium_sensu_stricto_1, Bifidobacterium, Lachnospiraceae_UCG-007, Clostridium_sensu_stricto_6, Ruminococcaceae_UCG-002, and Ruminococcaceae_UCG-004 were significantly upregulated; while Treponema_2 and T34_unclassified were significantly downregulated in the jejunum of MSTN pigs. Similarly, Phascolarctobacterium, Ruminiclostridium_9, Succinivibrio, Longibaculum, and Candidatus_Stoquefichus were significantly upregulated, while Barnesiella was significantly downregulated in the cecum of MSTN pigs. Moreover, metabolomics analysis showed significant changes in metabolites involved in purine, sphingolipid and tryptophan metabolism in the jejunum, while those associated with glycerophospholipid and pyrimidine metabolism were changed in the cecum. Spearman correlation analysis further demonstrated that there was a significant correlation between microflora composition and metabolites. Our analyses indicated the MSTN editing affects the composition of metabolites and microbial strains in the jejunum and the cecum, which might provide more useable nutrients for the host of MSTN± Large White pigs.
Introduction
Myostatin (MSTN) is a protein that inhibits muscle development (McPherron et al., 1997) and its inactivation interferes with fat deposition, which results in a higher proportion of lean meat (Zhao et al., 2005; Guo et al., 2009). Pigs are a major source of high-value animal protein, whereby farming animals of containing with MSTN loss-of-function mutations has become a research priority to facilitate the breeding of stock with better meat quality and higher economic value. Previous studies have reported the successful generation of healthy MSTN knockouts in different pig breeds, including Meishan (Qian et al., 2015), Erhualian (Wang et al., 2017), Large White/Landrace × Duroc (Rao et al., 2016) and Landrace pigs (Wang et al., 2015). The animals showed a reduced fat content and increased tenderness. Furthermore, pigs harboring naturally occurring single copy MSTN mutations have higher muscle mass and lower fat content compared to the wild-type genotype (Matika and Robledo, 2019). In fact, the phenotype of mutant MSTN pigs is affected by both double and single mutations that consistently the host leaner, with higher muscle and lower fat proportions. Therefore, these genotypes are likely to have significant future applications.
On the other hand, the gut microbial community is a complex system that co-exists inside each living body and associated with meat quality and body fat (Park et al., 2014). Yang et al. (2017) reported that microbial transplantation from pigs to mice changed the metabolic profiles of skeletal muscle (Yang et al., 2017). Mach et al. (2015) investigated the early establishment of the microbiome in pigs and identified enterotypes related to growth (Ramayo-Caldas et al., 2016), and (Xiao et al., 2016) reported the potential effects of different microbial profiles on lipid metabolism. Finally, Lu et al. (2018) reported a relationships between growth and carcass composition and specific microbial composition as well as alpha diversity (Lu et al., 2018). These observations demonstrate that gut bacteria affect host nutritional, physiological and immunological processes in various ways (Maltecca and Bergamaschi, 2020). Indeed, interference with intestinal microbial homeostasis is known to have downstream effects on intestinal metabolism (Alou et al., 2016; Lippert et al., 2017).
Given the above literatures, MSTN gene mutation can affect the proportion of lean meat and fat of pigs. And the gut microbial is associated with meat quality and body fat Nevertheless, up to data there is no a systematic study detecting the changes in intestinal microbial composition and metabolome of MSTN single mutant pigs. In this study, we used 16S rDNA gene sequencing and metabolome analysis to investigate changes in the microbial composition and metabolome in the jejunum and the cecum of MSTN edited Large White pigs.
Materials and Methods
Animals
The pigs had ad libitum access to a commercial pig diet and water throughout the study period. All experiments involving animals were approved by the Animal Welfare and Research Ethics Committee at the Institute of Animal Sciences, Chinese Academy of Agricultural Sciences (IAS2018-10). Animal care and treatment were complied with the standards described in the guidelines for the care and use of laboratory animals of the Institute of Animal Sciences of CAAS.
Sampling
To minimize the number of variables in the experiment, we selected six heterozygous mutants (average body weight: 114.4441 ± 3.6756 kg; average body length: 100.2235 ± 0.9389 cm) and six wild type (average body weight: 115.3689 ± 3.6499 kg; average body length: 114.4441 ± 3.6756 cm) 8 month old pigs from the offspring of the same MSTN+/− mutant boars (Supplementary Table 1). The animals were raised on the same farm with the same management. Feed came from Tianjin Taikang feed mill, and contained corn, fish meal, soybean meal, sodium chloride, amino acids, vitamins, trace elements, stone powder and calcium hydrogen phosphate; the nutrient levels of the diets can be found in Supplementary Table 2. The animals were electrocuted, and the jejunum and cecum contents were immediately removed.
Genotype Identification
Samples of piglets’ ear tissue were taken and preserved in alcohol. Total DNA was extracted according to the instructions of the animal tissue genomic DNA extraction Kit (TIANGEN, DP324). After that, the MSTN gene was amplified with 2 × Es Taq MasterMix (CoWin Biosciences). The PCR amplification products of MSTN (20 μL) were detected by 1.5% agar-gel electrophoresis. The PCR products (20 μL) were sequenced by Sangon Biotech (Shanghai) Co., Ltd.
16S rDNA Sequencing
DNA from fecal samples was isolated using the Stool DNA Kit (Omega, United States). The primers (F: 5′-ACTCCTACGGGAGGCAGCAG-3′; R: 5′-GGACTACHVGGGTWT-CTAAT-3′) were used in the PCR amplification of the V3–V4 region of the bacterial 16S rRNA gene. The 5′ ends of the primers were tagged with specific barcods per sample and sequencing universal primers. PCR amplification was performed in a total volume of 25 μL reaction mixture containing 25 ng of template DNA, 12.5 μL PCR Premix, 2.5 μL of each primer, and PCR-grade water. The size of the PCR products was confirmed with 2% agarose gel electrophoresis, purified with AMPure XT beads (Beckman Coulter Genomics, Danvers, MA, United States) and then quantified by Qubit (Invitrogen, CA, United States). The amplicon pools were prepared for sequencing, and the size and quantity of the amplicon library were assessed on an Agilent 2100 bioanalyzer (Agilent, United States) and with the Library Quantification Kit for Illumina (Kapa Biosciences, Woburn, MA, United States).
Amplicon libraries were sequenced on an Illumina MiSeq platform according to the manufacturer’s recommendations, which were provided by LC-Bio. Feature list and feature sequence were obtained by removing the background. Non-metric Multidimensional Scaling(NMDS) analysis was performed according to the weighted UniFrac distance metrics. The number of observed species and the indices of Chao 1 (species richness), Shannon and Simpson (diversity) were calculated to estimate alpha diversity. The PICRUSt software was used to predict the function of composition of samples.
The dataset for 16S rDNA sequencing was deposited in GenBank Sequence Read Archive (SRA) database and are available under the accession number PRJNA687099 (https://dataview.ncbi.nlm.nih.gov/object/PRJNA687099).
Untargeted Metabolomic Study
All fecal samples were thawed on ice, and metabolite were extracted with 50% methanol Buffer. The detailed extraction procedures can be found in (Yu et al., 2018). LC-MS analysis was performed on an ultra performance liquid chromatography (UPLC) system (SCIEX, United Kingdom) coupled with the high-resolution tandem mass spectrometer TripleTOF5600plus (SCIEX, United Kingdom). The Q-TOF was operated in both positive and negative ion modes.
The raw LC-MS data files were converted into mzXML format, and then processed by the XCMS, CAMERA and metaX (Li et al., 2018) toolbox, which was implemented in R. The online KEGG and HMDB databases were used to annotate the metabolites by matching the exact molecular mass data (m/z) of the samples with those from the database.
Student t-tests were used to calculate significant differences in metabolite concentrations between the two groups. We have implemented multiple test corrections by adjusting the P values using an FDR (Benjamini–Hochberg). Supervised PLS-DA was conducted through metaX in order to discriminate the different variables between groups. The metabolites with VIP > 1, P-value < 0.05 and fold change(FC) ≥ 1.5 or FC ≤ 0.5 were considered significantly different. Furthermore, significantly differentially abundant metabolites screened from untargeted metabolomics were imported into the MetaboAnalyst 4.0 database to perform pathway analysis.
Correlation Analysis
We performed spearman correlation analysis on the differentiated metabolites screened by metabolomics and the significantly different genera obtained by 16S rDNA sequencing analysis.
Results
Genotype Identification
The pigs used in this study were selected from the offspring of healthy MSTN+/− mutant boars created by removing 11bp nucleotides (869-879), and introducing a single nucleotide A > G conversion at position 882 (Supplementary Figure 1A) and WT mothers. Since 6302 is a single copy mutant pig, the progeny will contain both MSTN+/− and WT pigs. Ear samples were collected one month after birth for genotyping. The WT DNA sequences showed single peaks throughout the MSTN gene, while the mutant DNA sequences showed multiple, overlapping, divergent base calls starting after position 868 (see Supplementary Figure 1B).
Microbiome of Intestinal Contents From WT and MSTN+/− Large White Pigs
We used a total of 24 samples consisting of the jejunum and the cecum content from WT and MSTN groups to study gut microbiota diversity through 16S rDNA (V3-V4 region) high-throughput sequencing. We evaluated differences in bacterial diversity between the MSNT and WT groups in both jejunum and cecum samples aligning these sequences and estimating alpha and beta diversity indices. The Chao1 index, Shannon and Simpson indexes, indicators of microbial richness were no statistically significant differences between groups (Figures 1A–C). β-diversity was assessed by Weighted UniFrac distance-based Non-metric Multidimensional Scaling (NMDS), which is a powerful ordination method that allows to uncover non-linear relationships between samples. The NMDS-based maps of the jejunum showed distinct differences between wild-type (WT_J) and MSTN -edited (MSTN_J) pigs (stress value = 0.02, Figure 1D). Similar distinct differences were observed when comparing cecum sample (stress value = 0.06, Figure 1F). Our results further showed that MSTN editing had no significant effect on the bacterial abundance and diversity of despite the aforementioned changes in the gut microbiota composition in the jejunum and the cecum of MSTN pigs.
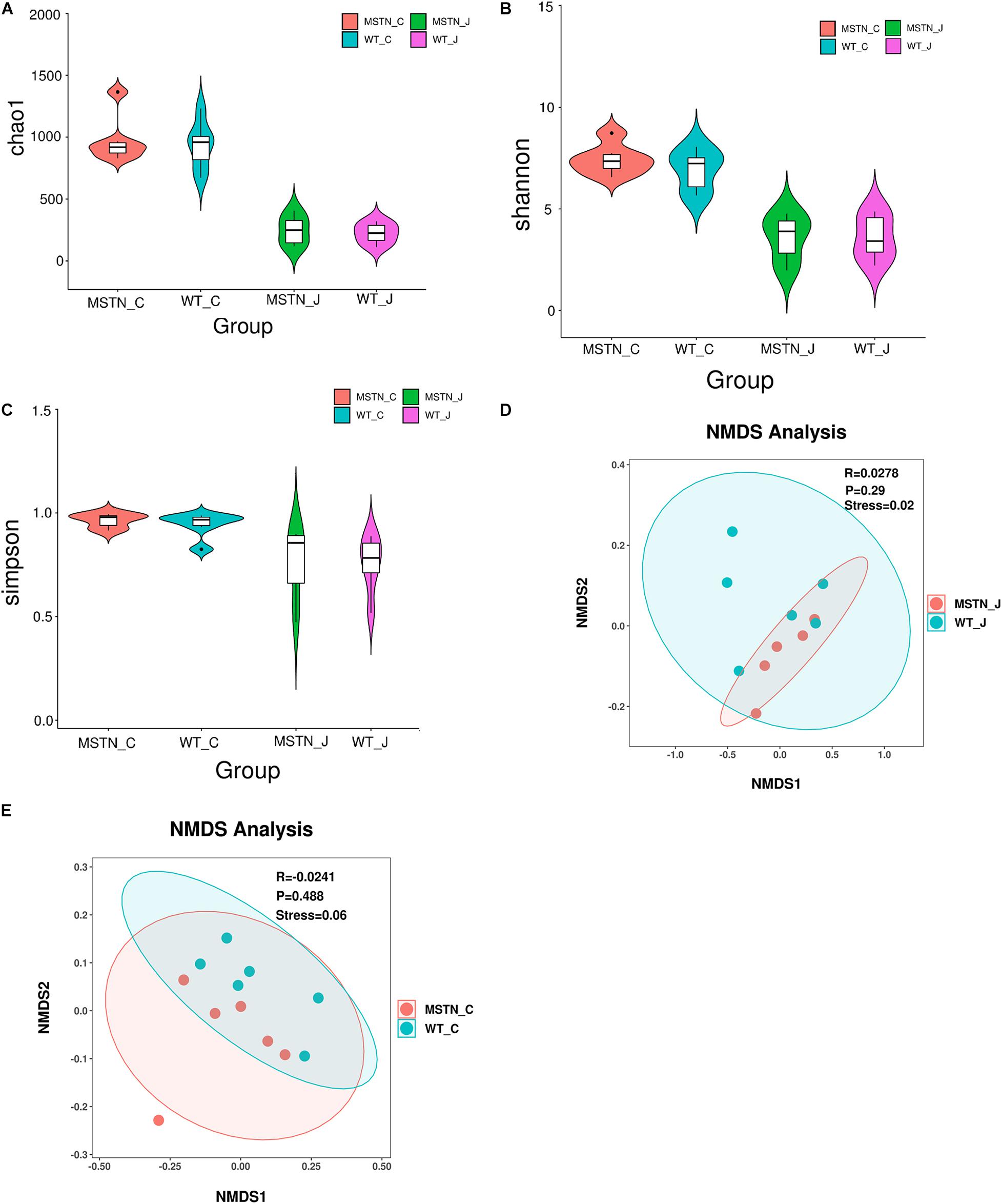
Figure 1. Gut microbiome diversity and structure analysis. (A–C) Violin plots showing chao1 (A), shannon (B), and simpson (C) of indices in both WT and MSTN+/− jejunum and cecum samples; (D,E) Non-metric multi-dimensional scaling (NMDS) ordination plot derived from weighted pairwise UniFrac distances in both WT and MSTN+/− jejunum (D), and cecum (E) samples. Stress values for ordination plot were <0.1, which indicates the accuracy of data representation in a two-dimensional space.
Next, we assessed the overall bacterial community compositions using heatmaps displaying the abundance of taxa at the phylum (Supplementary Figure 2A) and genus (Figure 2A) levels. At the phylum level, we found an downregulated of Spirochaetes in the jejunum of MSTN+/− pigs (Supplementary Figure 2B). At the genus level, Lactobacillus (44.99%), Clostridium_sensu_stricto (22.00%), Terrisporobacter (14.11%) and Turicibacter (3.08%) were the predominant genera in the MSTN jejunum; while Lactobacillus (39.63%), Escherichia-Shigella (14.87%), Brevundimonas (8.20%) and Clostridium_sensu_stricto (4.85%) were the most abundant in the WT jejunum. Wilcoxon Rank Sum Test was performed to compare the relative abundance of species in the two groups. Clostridium_sensu_stricto_1 (p = 0.0065), Bifidobacterium (p = 0.0374), Lachnospiraceae_UCG-007 (p = 0.0374), Clostridium_sensu_stricto_6 (p = 0.0061), Ruminococcaceae_UCG-002 (p = 0. 0.0495), and Ruminococcaceae_UCG-004 (p = 0. 0.0495) were significantly upregulated in MSTN_J;while Treponema_2 (p = 0.021) and T34_unclassified (p = 0.0028) were significantly downregulated (Figure 2B).
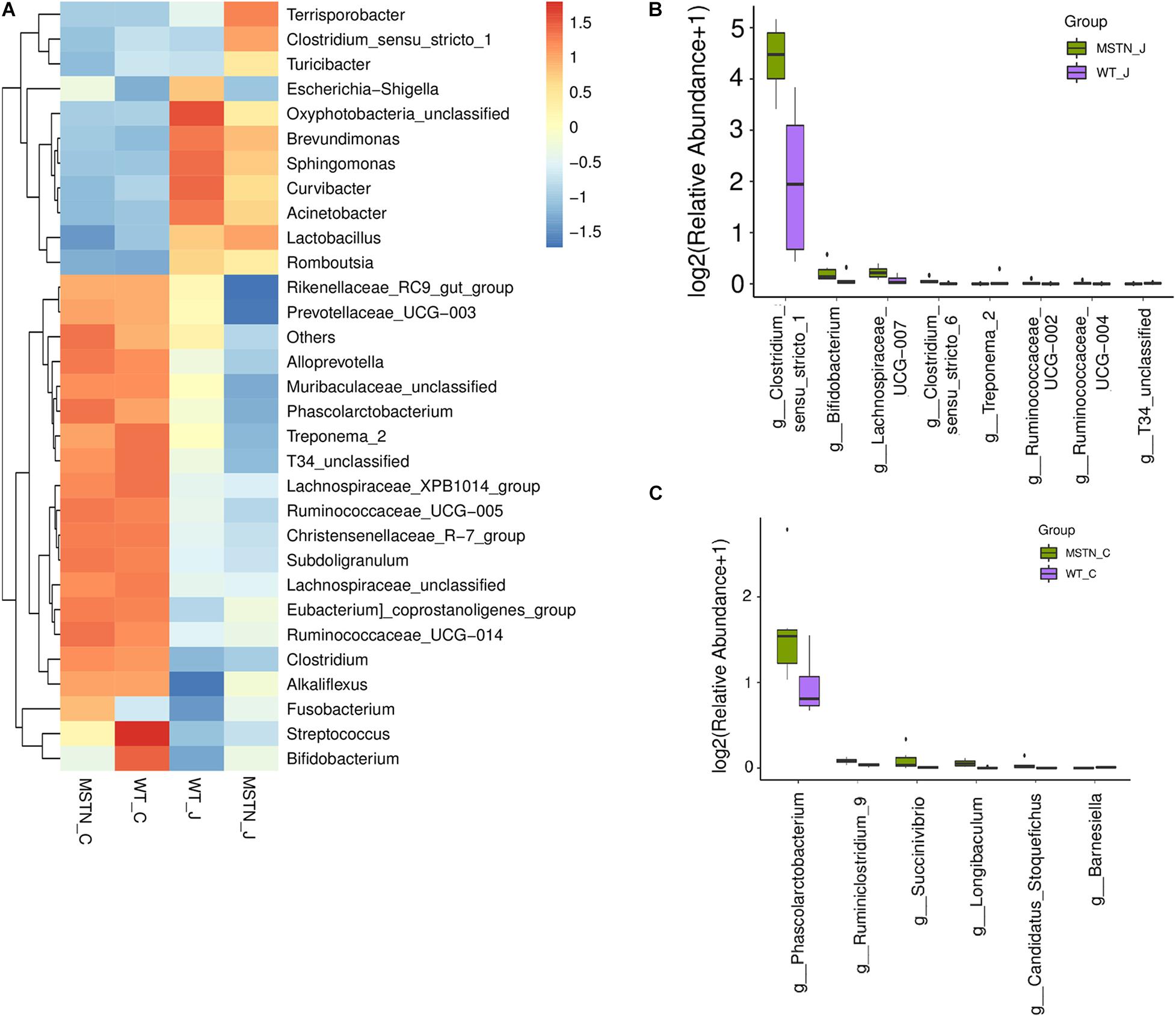
Figure 2. Generic differences between WT and MSTN groups. (A) Heat map of relative abundance of 30 genera. (B) Significantly different genera between WT and MSTN cecum samples. (C) Significantly different genera between WT and MSTN jejunum samples.
In cecum samples, Lactobacillus (15.59%), Ruminococcaceae_UCG-005 (10.13%), Escherichia-Shigella (4.40%) and Clostridium_sensu_stricto_1 (4.14%) were the most abundant genera in the MSTN_C group; while Lactobacillus (18.55%), Streptococcus (11.03%), Ruminococcaceae_UCG-005 (8.26%) and Clostridium_sensu_stricto_1 (5.22%)%) were the most frequent in the WT_C group. A comparison between these groups showed a significant upregulated of Phascolarctobacterium (p = 0.0374), Ruminiclostridium_9 (p = 0.0163), Succinivibrio (p = 0.0278), Longibaculum (p = 0.0033), and Candidatus_Stoquefichus (p = 0.0463) in the MSTN group. On the contrary, Barnesiella (p = 0.0201) was significantly downregulated (Figure 2C).
We predicted the functional potential of bacterial communities in WT and MSTN samples using the PICRUSt2 software (Langille et al., 2013). KEGG pathway analyses unveiled a total of 15 significant pathways were classified in MSTN jejunum samples (Figure 3A), in particular L-rhamnose degradation I (the most significant). Moreover, we were able to find 7 significantly different KEGG pathways in MSTN cecum samples, including acetyl-CoA fermentation to butanoate II, adenosylcobalamin salvage from cobinamide I, colanic acid building blocks biosynthesis, purine ribonucleosides degradation, superpathway of arginine and polyamine biosynthesis, superpathway of GDP-mannose-derived O-antigen building blocks biosynthesis and thiamin salvage II (Figure 3B).
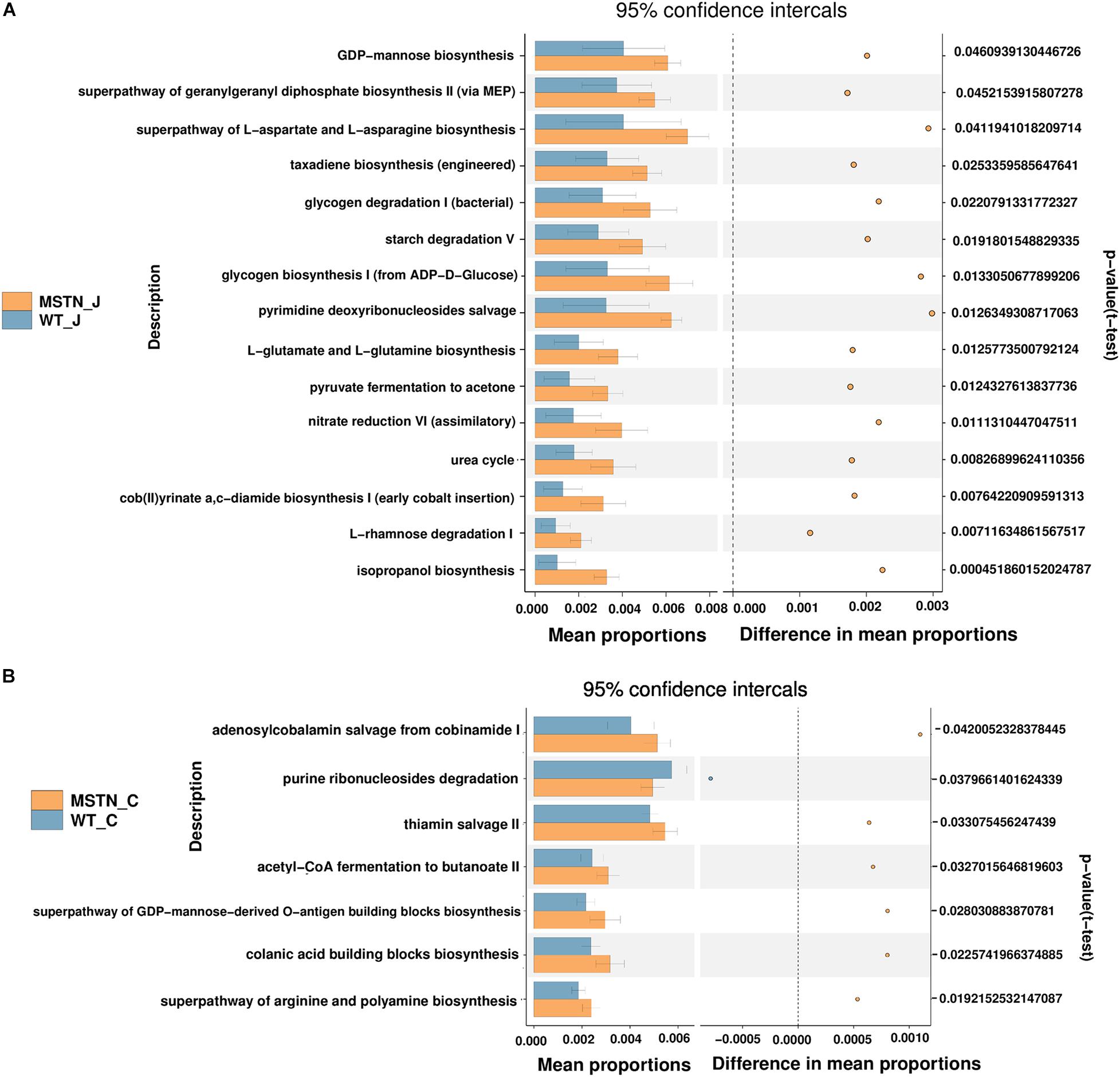
Figure 3. Comparison of PICRUSt-predicted functional pathways in the jejunum and the cecum between WT and MSTN+/− groups. (A,B) The extended error bars method in STAMP showing relative difference pathway in comparisons involving one hypervariable dataset and other datasets using Welch’s t-test (two sided).
Non-targeted Metabolomics of Fecal Samples Form WT and MSTN+/− Large White Pigs
To evaluate the metabolic changes occurring as a response to MSTN gene editing, we analyzed a total of 24 intestinal contents from the jejunum and cecum of WT and MSTN+/− Large White pigs (n = 6 for each group) by LC-MS-based untargeted metabolomics. This analysis allowed us to identify a total of 805 metabolites from experiments including both positive and negative ion modes. We then performed a partial least-squares-discriminant analysis (PLS-DA) to identify the altered metabolites in MSTN-edited Large White pigs. The PLS-DA model revealed significant differences between WT and MSTN+/− samples in the same intestinal tract (Figures 4A–D). Specifically, our PLS-DA model revealed metabolic profile differences between WT_J and MSTN_J (Figures 3A,B), WT_I and MSTN_I (Figures 4A,B), and WT_C and MSTN_C (Figures 4C,D), suggesting that MSTN gene editing leads to significant biochemical changes in the gut. The R2 and Q2 values of all mathematical models used are summarized in Supplementary Table 3.
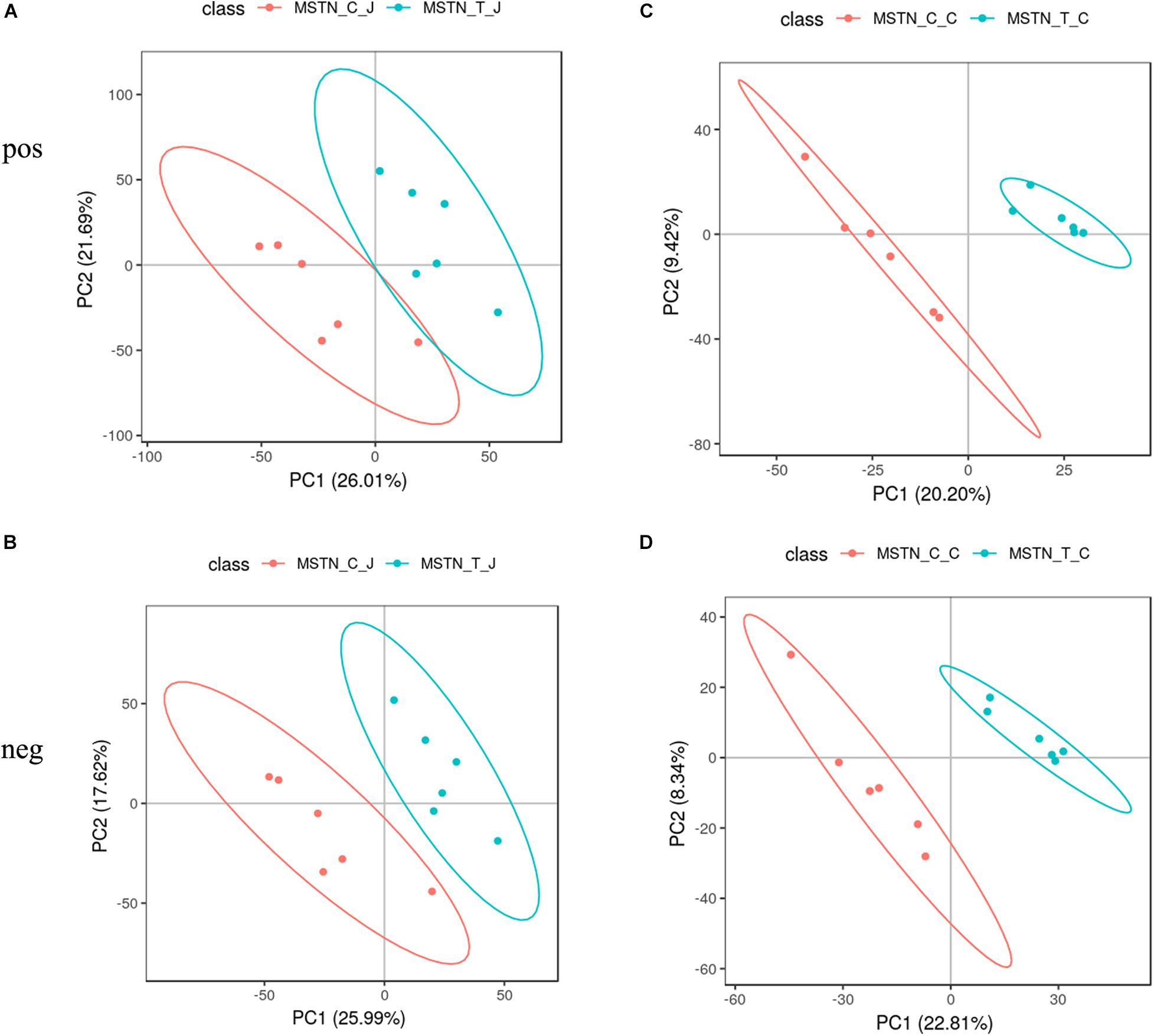
Figure 4. PLS-DA plot based on fecal metabolites. (A–D) PLS-DA plot of fecal metabolites detected in positive and negative mode between MSTN and WT pigs in the jejunum (A,B) and cecum (C,D).
We identified 15 differently enriched metabolites between MSTN_J and WT_J based on VIP values and relative abundance, of which 5 were up-regulated and 10 were down-regulated in MSTN_J samples (Table 1). These metabolites were mainly involved in purine, sphingolipid and tryptophan metabolism (Figure 5A).
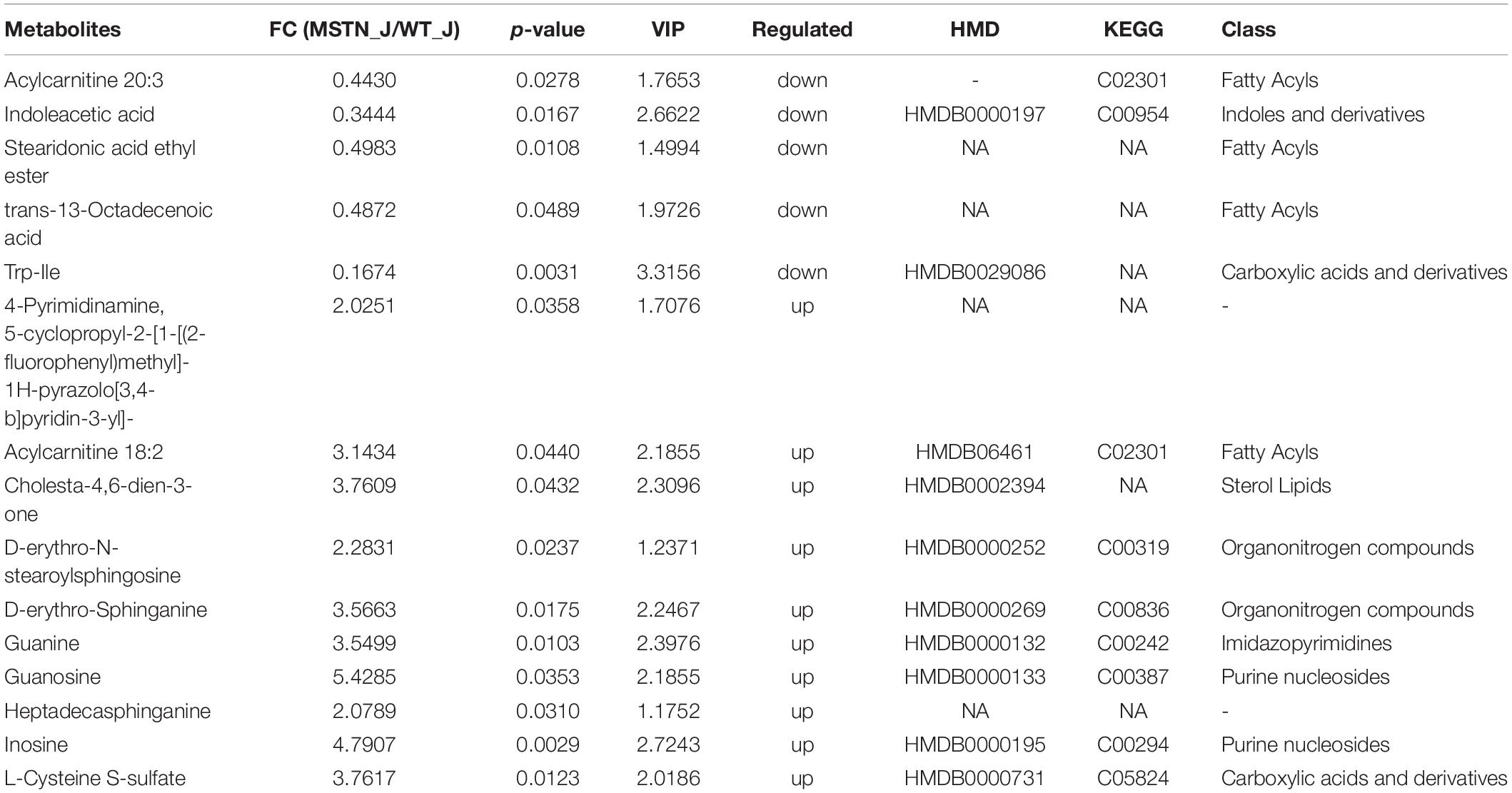
Table 1. Fold changes (FC) for LC-MS-based significant different metabolomics datasets for the MSTN_J and WT_J groups.
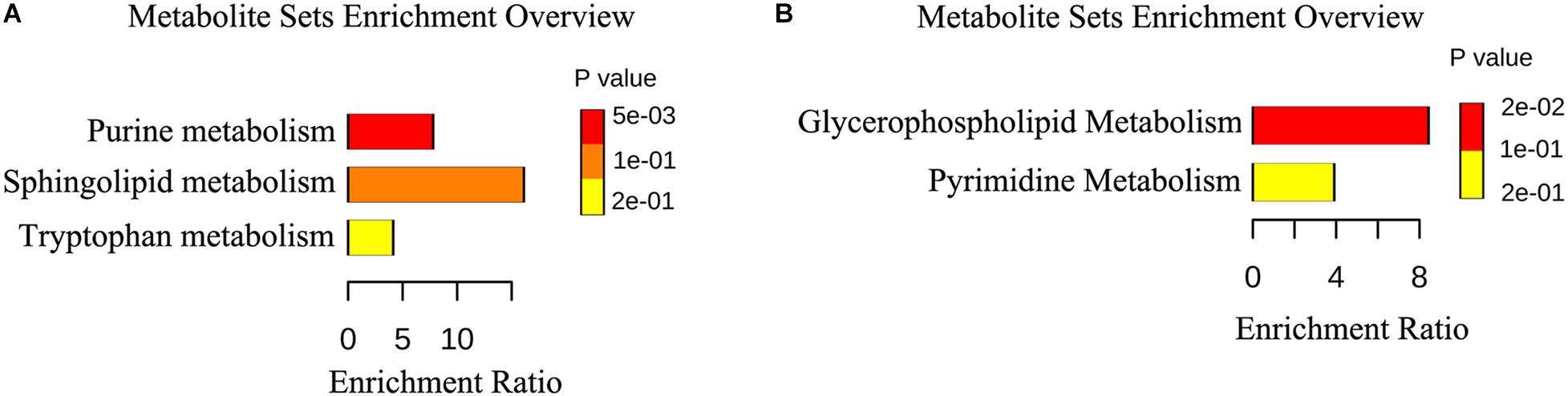
Figure 5. Metabolic pathway enrichment analysis. (A,B) Overview of metabolites that were changed in the jejunum (A) and the cecum (B) of MSTN pigs compared to WT.
Analogously, we found 22 differently enriched metabolites between the MSTN_C and WT_C samples, of which 7 were up-regulated and 14 were down-regulated in the former. Half of these metabolites were classified as lipids including fatty acyls, sterol lipids, prenol lipids and glycerophospholipids (Table 2). Functional analysis of these metabolites revealed that they were mainly involved in glycerophospholipid and pyrimidine metabolism (Figure 5B).
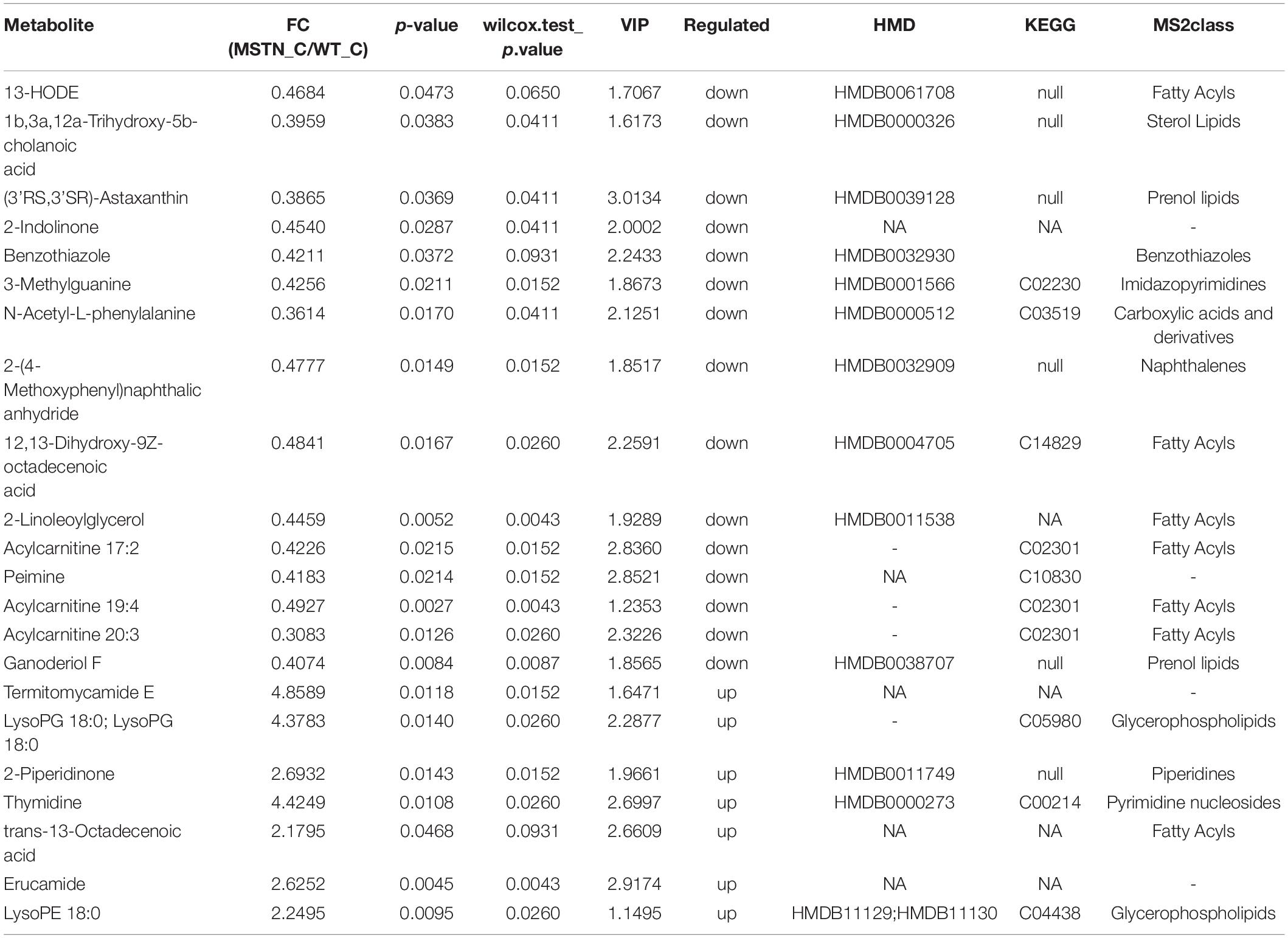
Table 2. Fold changes (FC) for LC-MS-based significant different metabolomics datasets for the MSTN_C and WT_C groups.
Correlation of Gut Microbiota With Fecal Metabolic Phenotype
We have also conducted correlation analyses between the changed relative abundance of gut bacterial taxa and altered fecal metabolites, which were visualized in a heatmap (Figure 6). In general, we found an agreement between the observed taxa enrichment and metabolites presence in the jejunum and samples. Network regulation analysis results of altered metabolites and genera in the jejunum and the cecum are showed in Supplementary Figure 3.
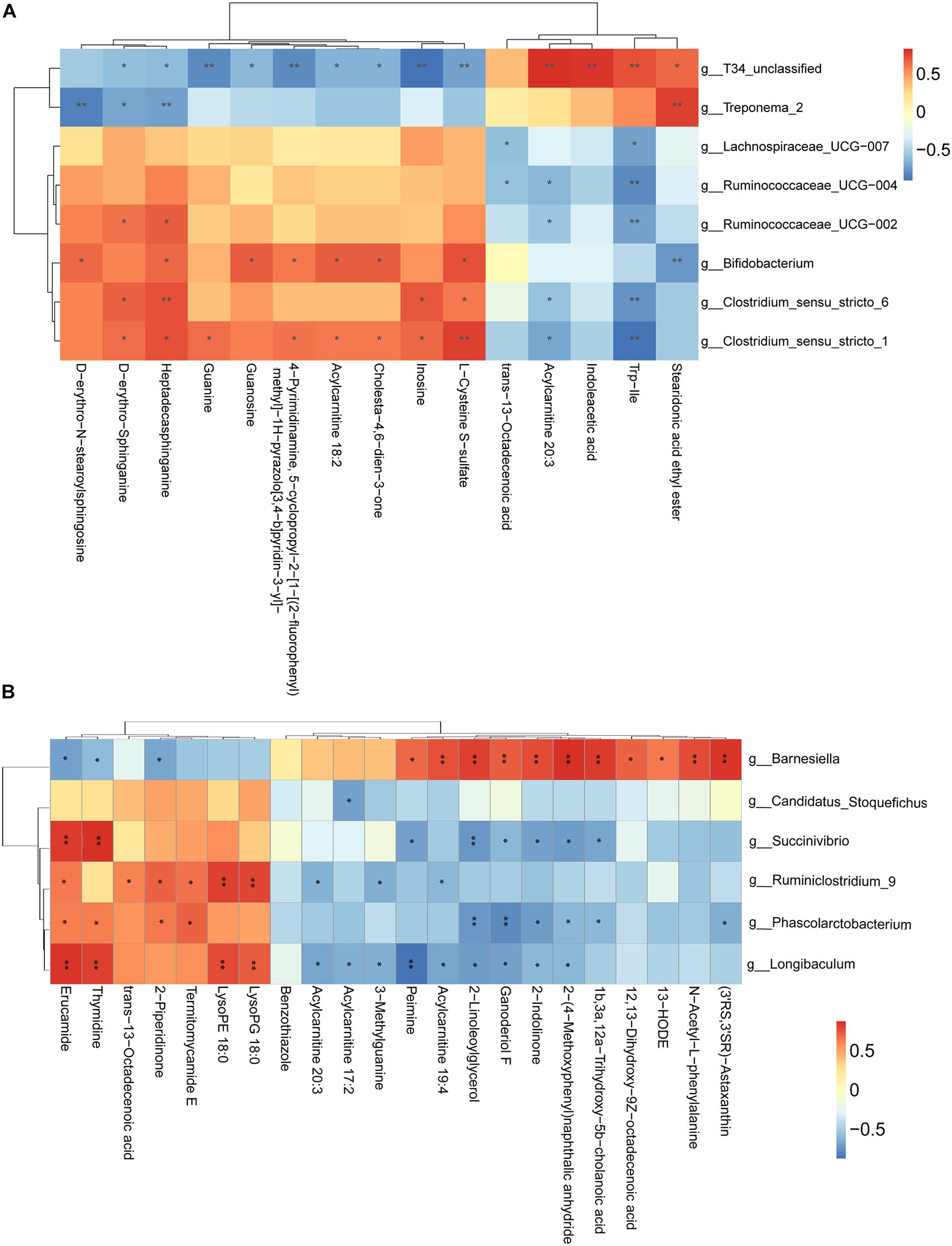
Figure 6. Correlation analysis between perturbed gut microbes and altered metabolites. (A,B) Pearson’s correlation analysis between perturbed genera and metabolite concentrations in the jejunum (A) and the cecum (B). Asterisks indicate significant correlations between MSTN and WT pigs. Cells are according to Spearman’s correlation coefficient between the significantly altered genera and metabolites; significantly positive correlation (P < 0.05), and significantly negative (P < 0.05) correlations are shown in red and blue, respectively. ∗P < 0.05, ∗∗P < 0.01.
In summary, we demonstrated that MSTN gene editing changed the structure and composition of the gut microbiome and altered fecal metabolic configuration in the jejunum and the cecum of MSTN+/− Large White pigs. Moreover, we showed that a strong correlation between the significant differential metabolites and significant changed genera in jejunum and cecum. Non-targeted metabolomics identified a number of metabolites that affected gene editing, possibly, at least in part, due to ecological changes in the microbiota.
Discussion
In this study, we aimed to determine the effects of MSTN gene editing on the fecal microbiome and metabolome. Accordingly, we employed an integrated approach consisting of 16S rDNA sequencing and LC-MS-based untargeted metabolomics to explore the phenotypical changes in gut microbiota and fecal metabolic composition in the jejunal and cecal of MSTN-edited Large White pigs.
Despite the overall similarities in the composition of the fecal microbiome of at a phylum level, Wilcoxon Rank Sum Test analyses revealed differences in the microbial profiles of MSTN-edited and WT groups, which indicate that MSTN gene editing is associated with shifts in the relative abundance of individual gut bacterial species. The alpha diversity of intestinal microbiota in the jejunum and the cecum was not observed between the two groups. However, we found that the beta diversity of MSTN intestinal contents was altered. Besides, several genus microbes in both the jejunum and cecum were seen to up/down regulated MSTN+/− Large White pigs. These findings somehow indicated that the MSTN gene editing not only changes the proportion of lean meat in Large White pigs (data no showed in this article), and also the composition of microorganisms in the gut. The result is inconsistent with a previous research using rectal feces of MSTN double-allele knockout Meishan pigs (Cui et al., 2019). The differences may arise from analyzing distinct pig species, different genotypes and from employing different methodological approaches for data analysis (e.g., QIIME 2 does not performed clustering based on sequence similarity but instead revises the sequencing errors of amplitors through dereplication, chimeric filtering and other methods to improve accuracy).
The alpha diversity of the microbiome, to some degree, is a health indicator of the host. In mice, social stress significantly changes microbial population (Bailey et al., 2010) and reduces the alpha diversity of gut microbiome (Bailey et al., 2011). Stress-induced cage rearing reduces gut microbial diversity in chickens as compared to free-range rearing (Chen et al., 2019), while heat stress results in decreased gut microbial diversity in Holstein dairy cows (Chen et al., 2018). Here, no changes in the intestinal microbial alpha diversity of MSTN gene editing pigs likely indicates no change in the health status of MSTN pigs.
The gut microbiota comprises numerous microorganisms, and changes in the gut microbial composition are influenced by both the host genotype and environmental factors (Neish, 2009; David et al., 2014). Results showed that 2 genotypes, GG and TT, in the rs16775833 within the DMRT1 gene can modulate the microbial community structure and are associated with the body weight of birds (Ji et al., 2020). The host’s genetic background, including the different isoforms of the apolipoprotein E (APOE) gene, can exert an influence over microbiota composition (Guardia-Escote et al., 2019). All pigs were sampled under the same feeding conditions throughout the experiment, but the gut microbial composition of MSTN pig jejunum and cecum microbes were altered, most likely due to changes in the MSTN gene. The jejunum is specialized in absorbing small nutrient particles via the enterocytes, and the mammal intestinal microbiome plays a key role in converting food into useable nutrients for the host. In the present study, concordant changes in both metabolite presence and microorganism composition in the jejunum were obtained. Clostridium_sensu_stricto_1, a genus belonging to butyrate-producing Clostridia bacteria, increased significantly in the MSTN group. Butyrate has a significant part in the development of intestinal epithelial cells and is essential for the energy supply of the host as the final unabsorbed polysaccharide metabolite in the fermented food of the intestinal microbiota (Pryde et al., 2002). Hence, our observations suggest an upregulation in the production of butyrate in the MSTN group that subsequently enhances energy metabolism. Bifidobacterium is associated with health promoting outcomes due to the production of bacteriocins (Martinez et al., 2013), and might contribute to improved metabolic end-products (e.g., acetate and lactate) (Alcon-Giner et al., 2020). Accordingly, the upregulated of Bifidobacterium in the MSTN group indicate that the production of acetate, lactate and bacteriocins is likely upregulated in MSTN group, which promotes energy metabolism and health. Interestingly, the main function of this genus is to ferment sugars into volatile fatty acids, CO2 and H2 (Berkessa et al., 2020), whereby an enrichment of Clostridium_sensu_stricto_6 in the MSTN group suggest that MSTN pigs might have enhanced sugar metabolism. The Ruminococcaceae_UCG-002 is a hydrolytic-acidogenic bacteria, that is known for its role in the organic compounds during cation exchange resin-induced hydrolysis of waste activated sludge. Some of these compounds include tryptophan-like and tyrosine-like proteins, amino acids, aliphatic, and metabolic intermediates (Xiao et al., 2020). The Ruminococcaceae_UCG-004 has been associated with intestinal permeability indices (Gao et al., 2019). The significant overrepresentation of these two genera in MSTN_J suggested that the absorptive capacity of the intestinal wall may be stronger, and that digestion of substances in the intestinal tract is more efficient compared to wild-type. The increase of Phascolarctobacterium in the cecum of MSTN-edited pigs with low fat content is consistent with previous results showing a negative correlation between Phascolarctobacterium and the percentage of body fat (Naderpoor et al., 2019). A decline in the abundance of Phascolarctobacterium has been associated with inflammatory diseases, including colorectal cancer, whereby this bacteria might reduce colonic inflammation, enhance protection of the colonic mucosa and provide nutrition to the colon cells. It is possible that similar processes occur in the cecum as a result of an increase in Phascolarctobacterium in MSTN samples.
The main function of the cecum is to absorb the remaining fluids and salts after intestinal digestion and absorption are completed, and to mix these contents with mucus, a lubricating substance. We identified several overrepresented genera in this intestinal segment. Among these is Ruminiclostridium_9, a bacterial group which has been previously connected to obesity and other metabolic disorders (Wang et al., 2019), although these results are regarded with caution. While some studies have proposed that obese mice have a higher abundance of Ruminiclostridium_9 in their fecal microbiota than the leaner counterparts (Hou et al., 2019; Luo et al., 2019; Wang et al., 2020), others have shown that Ruminiclostridium_9 is instead negatively correlated with obesity (Zhao et al., 2017; Zhu et al., 2018; Hu et al., 2019). Another example of a MSTN-edited upregulated group in the cecum is Succinivibrio, a bacteria that has high potential for fiber degradation (Hippe et al., 1999) and thus can improve metabolism. Enrichment of Succinivibrio in the cecum might also provide the host with additional nutrients.
In addition to alterations in the fecal composition of microbiota in MSTN-edited pigs, we implemented, for the first time, a comprehensive analysis of the fecal metabolome of the intestinal MSTN-edited samples. The disruption of the gut microbiota homeostasis subsequently affects intestinal metabolism (Alou et al., 2016; Lippert et al., 2017), whereby we envisioned that MSTN gene editing-associated changes in microbial composition might be followed by changes in the relative abundance of different metabolites in fecal samples. We were able to support this hypothesis by demonstrating differentially detected metabolites in both the jejunum and the cecum. We found differential metabolites in the contents of the ileum and cecum, and a lot of these were accounted for by fatty acids, sterol lipids and glycerophospholipids (Fahy et al., 2009). Functional analysis showed they were involved in metabolism of sphingolipid (jejunum) and glycerophospholipid (cecum). Both sphingolipid and glycerophospholipid metabolism belong to the lipid metabolism pathway. A recent study reported the protective roles of Huangjinya green tea extract against obesity, liver steatosis and insulin resistance in high-fat diet-fed mice, highlighting its favorable modulation on fecal metabolites of amino acids, sphingolipids, and bile acids in vivo(Li et al., 2020). Another study found that oral hydroxysafflor yellow can reduce obesity in mice and the changed metabolites by serum metabolomics analysis were mainly linked with the pathways of glycerophospholipid and sphingolipid metabolism (Liu et al., 2018). These studies suggest that the resistance to obesity in animals may cause changes in the lipid metabolic pathways. The ratio of fat of MSTN-edited pigs was 100% lower than that of WT ([| heterozygote value–original value of WT|]/original value of WT). Correlation analysis allowed us to identify several bacterial genera potentially implicated in the host metabolic. For instance, in the jejunum, T34_unclassified, Bifidobacterium and Clostridium_sensu_stricto_1 were positively correlated with the majority of metabolites that were changed in the MSTN pigs. In the cecum, Barnesiella abundance was negatively correlated with 3 metabolites and positively correlated with 11 metabolites, while Longibaculum was positively and negatively correlated with 4 metabolites and 9 metabolites, respectively. The strongly correlation between the differentially detected metabolites with flora composition Hence, it is possible that these changes are consistent with phenotype and are caused by alterations in the microflora.
Conclusion
In conclusion, our study showed that MSTN gene editing alters the composition of metabolites and microbial communities in the jejunum and the cecum. Our results demonstrate that the different microbiome composition in the jejunal and cecal may provide more useable nutrients for the host of MSTN-edited pigs and influence the composition of metabolites.
Data Availability Statement
The datasets presented in this study can be found in online repositories. The names of the repository/repositories and accession number(s) can be found below: https://www.ncbi.nlm.nih.gov/sra/PRJNA687099.
Ethics Statement
The animal study was reviewed and approved by Animal Welfare and Research Ethics Committee at the Institute of Animal Sciences, Chinese Academy of Agricultural Sciences (CAAS).
Author Contributions
KL and HL designed and managed the project. YP and CC analyzed the data and performed all animal works and collected biological samples. YP wrote the manuscript. HL, YY, ZF, BL, HL, and KL revised the manuscript. All authors approved the final version of the manuscript.
Funding
This work was supported by the National Science Foundation for Young Scientists of China (31702088), the National Transgenic Breeding Project (2016ZX08006-001), the Guangdong Provincial Key Laboratory of Animal Molecular Design and Precise Breeding (2019B030301010), the Key Laboratory of Animal Molecular Design and Precise Breeding of Guangdong Higher Education Institutes (2019KSYS011), and Foshan University Initiative Scientific Research Program.
Conflict of Interest
The authors declare that the research was conducted in the absence of any commercial or financial relationships that could be construed as a potential conflict of interest.
Supplementary Material
The Supplementary Material for this article can be found online at: https://www.frontiersin.org/articles/10.3389/fmicb.2021.628685/full#supplementary-material
Supplementary Figure 1 | Genotype identification results. (A) The mutation domain’s specific sequence of MSTN mutant large white pigs. (B) Genome sequencing results of heterozygote MSTN mutant and wild-type large white pigs.
Supplementary Figure 2 | Phylum differences between WT and MSTN groups. (A) Heat map showing the relative abundance of Phyla. (B) Significantly different genera in WT and MSTN jejunum samples.
Supplementary Figure 3 | Altered metabolites and genera network regulation analysis results. (A,B) Network regulation analysis results of altered metabolites and genera in the jejunum (A) and the cecum (B). The different nodes in the figure represent different bacterial communities or metabolites. Bacterial communities are represented by the round shapes while metabolites are represented by triangles. Solid and dashed lines represent the positive and negative correlations between the flora and the metabolites, respectively.
Supplementary Table 1 | Information about of pigs in this study.
Supplementary Table 2 | Composition and nutrient levels of the diets (%, as-fed basis).
Supplementary Table 3 | The R2 and Q2 values for all mathematical models.
References
Alcon-Giner, C., Dalby, M. J., Caim, S., Ketskemety, J., Shaw, A., Sim, K., et al. (2020). Microbiota Supplementation with Bifidobacterium and Lactobacillus Modifies the Preterm Infant Gut Microbiota and Metabolome: An Observational Study. Cell Rep. Med. 1:100077. doi: 10.1016/j.xcrm.2020.100077
Alou, M. T., Lagier, J. C., and Raoult, D. (2016). Diet influence on the gut microbiota and dysbiosis related to nutritional disorders. Hum. Microbiome J. 1, 3–11. doi: 10.1016/j.humic.2016.09.001
Bailey, M. T., Dowd, S. E., Galley, J. D., Hufnagle, A. R., Allen, R. G., and Lyte, M. (2011). Exposure to a Social Stressor Alters the Structure of the Intestinal Microbiota: Implications for Stressor-Induced Immunomodulation. Brain Behav. Immun. 25, 397–407. doi: 10.1016/j.bbi.2010.10.023
Bailey, M. T., Dowd, S. E., Parry, N. M. A., Galley, J. D., Schauer, D. B., and Lyte, M. (2010). Stressor Exposure Disrupts Commensal Microbial Populations in the Intestines and Leads to Increased Colonization by Citrobacter rodentium. Infect. Immun. 78, 1509–1519. doi: 10.1128/iai.00862-09
Berkessa, Y. W., Yan, B., Li, T., Jegatheesan, V., and Zhang, Y. (2020). Treatment of anthraquinone dye textile wastewater using anaerobic dynamic membrane bioreactor: Performance and microbial dynamics. Chemosphere 238:124539. doi: 10.1016/j.chemosphere.2019.124539
Chen, S., Wang, J., Peng, D., Li, G., Chen, J., Gu, X., et al. (2018). Exposure to heat-stress environment affects the physiology, circulation levels of cytokines, and microbiome in dairy cows. Sci. Rep. 8:14606.
Chen, S., Xiang, H., Zhang, H., Zhu, X., Wang, D., Wang, J., et al. (2019). Rearing system causes changes of behavior, microbiome, and gene expression of chickens. Poultry Sci. 98, 3365–3376. doi: 10.3382/ps/pez140
Cui, W. T., Xiao, G. J., Jiang, S. W., Qian, L. L., Cai, C. B., Li, B., et al. (2019). Effect of ZFN-edited myostatin loss-of-function mutation on gut microbiota in Meishan pigs. PLoS One 14:e0210619. doi: 10.1371/journal.pone.0210619
David, L. A., Maurice, C. F., Carmody, R. N., Gootenberg, D. B., Button, J. E., Wolfe, B. E., et al. (2014). Diet rapidly and reproducibly alters the human gut microbiome. Nature 505, 559–563. doi: 10.1038/nature12820
Fahy, E., Subramaniam, S., Murphy, R. C., Nishijima, M., and Dennis, E. A. (2009). Update of the LIPID MAPS comprehensive classification system for lipids. J. Lipid Res. 50 Suppl(Suppl.), S9–S14.
Gao, Z., Wu, H., Zhang, K., Hossen, I., and Cao, Y. (2019). Protective effects of grape seed procyanidin extract on intestinal barrier dysfunction induced by a long-term high-fat diet. J. Funct. Foods 64:103663. doi: 10.1016/j.jff.2019.103663
Guardia-Escote, L., Basaure, P., Biosca-Brull, J., Cabré, M., and Colomina, M. T. (2019). APOE genotype and postnatal chlorpyrifos exposure modulate gut microbiota and cerebral short-chain fatty acids in preweaning mice. Food Chem. Toxicol. 135:110872. doi: 10.1016/j.fct.2019.110872
Guo, T., Jou, W., Chanturiya, T., Portas, J., Gavrilova, O., and McPherron, A. C. (2009). Myostatin inhibition in muscle, but not adipose tissue, decreases fat mass and improves insulin sensitivity. PLoS One 4:e4937. doi: 10.1371/journal.pone.0004937
Hippe, H., Hagelstein, A., Kramer, I., Swiderski, J., and Stackebrandt, E. (1999). Phylogenetic analysis of Formivibrio citricus, Propionivibrio dicarboxylicus, Anaerobiospirillum thomasii, Succinimonas amylolytica and Succinivibrio dextrinosolvens and proposal of Succinivibrionaceae fam. nov. Int. J. Syst. Bacteriol. 49(Pt 2), 779–782. doi: 10.1099/00207713-49-2-779
Hou, D., Zhao, Q., Yousaf, L., Khan, J., and Shen, Q. (2019). Consumption of mung bean (Vigna radiata L.) attenuates obesity, ameliorates lipid metabolic disorders and modifies the gut microbiota composition in mice fed a high-fat diet. J. Funct. Foods 64:103687. doi: 10.1016/j.jff.2019.103687
Hu, S., Xu, Y., Gao, X., Li, S., Jiang, W., Liu, Y., et al. (2019). Long-Chain Bases from Sea Cucumber Alleviate Obesity by Modulating Gut Microbiota. Mar. Drugs 17:17080455. doi: 10.3390/md17080455
Ji, J., Xu, Y., Luo, C., He, Y., Xu, X., Yan, X., et al. (2020). Effects of the DMRT1 genotype on the body weight and gut microbiota in the broiler chicken. Poultry Sci. 99, 4044–4051. doi: 10.1016/j.psj.2020.03.055
Langille, M. G. I., Zaneveld, J., Caporaso, J. G., McDonald, D., Knights, D., Reyes, J. A., et al. (2013). Predictive functional profiling of microbial communities using 16S rRNA marker gene sequences. Nat. Biotechnol. 31, 814–821. doi: 10.1038/nbt.2676
Li, M., Xu, J., Zhang, Y., Chu, S., Sun, S., Huo, Y., et al. (2020). Comparative analysis of fecal metabolite profiles in HFD-induced obese mice after oral administration of huangjinya green tea extract. Food Chemic. Toxicol. 145:111744. doi: 10.1016/j.fct.2020.111744
Li, Y., Fang, J., Qi, X., Lin, M., Zhong, Y., Sun, L., et al. (2018). Combined Analysis of the Fruit Metabolome and Transcriptome Reveals Candidate Genes Involved in Flavonoid Biosynthesis in Actinidia arguta. Int. J. Mol. Sci. 19:19051471. doi: 10.3390/ijms19051471
Lippert, K., Kedenko, L., Antonielli, L., Kedenko, I., Gemeier, C., Leitner, M., et al. (2017). Gut microbiota dysbiosis associated with glucose metabolism disorders and the metabolic syndrome in older adults. Benefic. Microbes 8, 1–12.
Liu, J., Yue, S., Yang, Z., Feng, W., Meng, X., Wang, A., et al. (2018). Oral hydroxysafflor yellow A reduces obesity in mice by modulating the gut microbiota and serum metabolism. Pharmacol. Res. 134, 40–50. doi: 10.1016/j.phrs.2018.05.012
Lu, D., Tiezzi, F., Schillebeeckx, C., McNulty, N. P., Schwab, C., Shull, C., et al. (2018). Host contributes to longitudinal diversity of fecal microbiota in swine selected for lean growth. Microbiome 6:4. doi: 10.1186/s40168-017-0384-1
Luo, Q., Cheng, D., Huang, C., Li, Y., Lao, C., Xia, Y., et al. (2019). Improvement of Colonic Immune Function with Soy Isoflavones in High-Fat Diet-Induced Obese Rats. Molecules 24:1139. doi: 10.3390/molecules24061139
Mach, N., Berri, M., Estellé, J., Levenez, F., Lemonnier, G., Denis, C., et al. (2015). Early-life establishment of the swine gut microbiome and impact on host phenotypes. Environ. Microbiol. Rep. 7, 554–569. doi: 10.1111/1758-2229.12285
Maltecca, C., and Bergamaschi, M. (2020). The interaction between microbiome and pig efficiency: A review. J. Anim. Breed Genet. 137, 4–13. doi: 10.1111/jbg.12443
Martinez, F. A., Balciunas, E. M., Converti, A., Cotter, P. D., and de Souza Oliveira, R. P. (2013). Bacteriocin production by Bifidobacterium spp. Rev. Biotechnol. Adv. 31, 482–488. doi: 10.1016/j.biotechadv.2013.01.010
Matika, O., and Robledo, D. (2019). Balancing selection at a premature stop mutation in the myostatin gene underlies a recessive leg weakness syndrome in pigs. PLoS Genet. 15:e1007759. doi: 10.1371/journal.pgen.1007759
McPherron, A. C., Lawler, A. M., and Lee, S. J. (1997). Regulation of skeletal muscle mass in mice by a new TGF-beta superfamily member. Nature 387, 83–90. doi: 10.1038/387083a0
Naderpoor, N., Mousa, A., Gomez-Arango, L. F., Barrett, H. L., Nitert, M. D., and Courten, B. D. (2019). Faecal Microbiota Are Related to Insulin Sensitivity and Secretion in Overweight or Obese Adults. J. Clin. Med. 8:452. doi: 10.3390/jcm8040452
Neish, A. S. (2009). Microbes in gastrointestinal health and disease. Gastroenterology 136, 65–80. doi: 10.1053/j.gastro.2008.10.080
Park, S. J., Kim, J., Lee, J. S., Rhee, S. K., and Kim, H. (2014). Characterization of the fecal microbiome in different swine groups by high-throughput sequencing. Anaerobe 28, 157–162. doi: 10.1016/j.anaerobe.2014.06.002
Pryde, S. E., Duncan, S. H., Hold, G. L., Stewart, C. S., and Flint, H. J. (2002). The microbiology of butyrate formation in the human colon. FEMS Microbiol. Lett. 217, 133–139. doi: 10.1111/j.1574-6968.2002.tb11467.x
Qian, L., Tang, M., Yang, J., Wang, Q., Cai, C., Jiang, S., et al. (2015). Targeted mutations in myostatin by zinc-finger nucleases result in double-muscled phenotype in Meishan pigs. Rep 5:14435.
Ramayo-Caldas, Y., Mach, N., Lepage, P., Levenez, F., Denis, C., Lemonnier, G., et al. (2016). Phylogenetic network analysis applied to pig gut microbiota identifies an ecosystem structure linked with growth traits. ISME J. 10, 2973–2977. doi: 10.1038/ismej.2016.77
Rao, S., Fujimura, T., Matsunari, H., Sakuma, T., Nakano, K., Watanabe, M., et al. (2016). Efficient modification of the myostatin gene in porcine somatic cells and generation of knockout piglets. Mol. Reprod. Dev. 83, 61–70. doi: 10.1002/mrd.22591
Wang, K., Ouyang, H., Xie, Z., Yao, C., Guo, N., Li, M., et al. (2015). Efficient Generation of Myostatin Mutations in Pigs Using the CRISPR/Cas9 System. Sci. Rep. 5:16623. doi: 10.1038/srep16623
Wang, K., Tang, X., Xie, Z., Zou, X., Li, M., Yuan, H., et al. (2017). CRISPR/Cas9-mediated knockout of myostatin in Chinese indigenous Erhualian pigs. Transgenic Res. 26, 799–805. doi: 10.1007/s11248-017-0044-z
Wang, P., Gao, J., Ke, W., Wang, J., and Hu, X. (2020). Resveratrol reduces obesity in high-fat diet-fed mice via modulating the structure and metabolic function of the gut microbiota. Free Radic. Biol. Med. 156, 83–98. doi: 10.1016/j.freeradbiomed.2020.04.013
Wang, R., Li, S., Jin, L., Zhang, W., and Wu, C. (2019). Four-week administration of nicotinemoderately impacts blood metabolic profile and gut microbiota in a diet-dependent manner. Biomed. Pharmacother. 115:108945. doi: 10.1016/j.biopha.2019.108945
Xiao, K., Abbt-Braun, G., and Horn, H. (2020). Changes in the characteristics of dissolved organic matter during sludge treatment: A critical review. Water Res. 187:116441. doi: 10.1016/j.watres.2020.116441
Xiao, L., Estellé, J., Kiilerich, P., Ramayo-Caldas, Y., Xia, Z., Feng, Q., et al. (2016). A reference gene catalogue of the pig gut microbiome. Nat. Microbiol. 1:16161. doi: 10.1038/nmicrobiol.2016.161
Yang, H., Huang, X., Fang, S., He, M., Zhao, Y., Wu, Z., et al. (2017). Unraveling the Fecal Microbiota and Metagenomic Functional Capacity Associated with Feed Efficiency in Pigs. Front. Microbiol. Front. Microbiol. 8:1555. doi: 10.3389/fmicb.2017.01555
Yu, C., Luo, X., Zhan, X., Hao, J., Zhang, L., Yb, L. S., et al. (2018). Comparative metabolomics reveals the metabolic variations between two endangered Taxus species (T. fuana and T. yunnanensis) in the Himalayas. BMC Plant Biol. 18:197. doi: 10.1186/s12870-018-1412-4
Zhao, B., Wall, R. J., and Yang, J. (2005). Transgenic expression of myostatin propeptide prevents diet-induced obesity and insulin resistance. Biochem. Biophys. Res. Commun. 337, 248–255. doi: 10.1016/j.bbrc.2005.09.044
Zhao, L., Zhang, Q., Ma, W., Tian, F., Shen, H., and Zhou, M. (2017). A combination of quercetin and resveratrol reduces obesity in high-fat diet-fed rats by modulation of gut microbiota. Food Funct. 8, 4644–4656. doi: 10.1039/c7fo01383c
Keywords: myostatin, microbiome, metabolome, jejunum, cecum, pigs
Citation: Pei Y, Chen C, Mu Y, Yang Y, Feng Z, Li B, Li H and Li K (2021) Integrated Microbiome and Metabolome Analysis Reveals a Positive Change in the Intestinal Environment of Myostatin Edited Large White Pigs. Front. Microbiol. 12:628685. doi: 10.3389/fmicb.2021.628685
Received: 13 November 2020; Accepted: 01 February 2021;
Published: 17 February 2021.
Edited by:
Shyam Sundar Paul, ICAR-Directorate of Poultry Research (DPR), IndiaReviewed by:
Xihong Zhou, Institute of Subtropical Agriculture, Chinese Academy of Sciences, ChinaBi E. Tan, Hunan Agricultural University, China
Copyright © 2021 Pei, Chen, Mu, Yang, Feng, Li, Li and Li. This is an open-access article distributed under the terms of the Creative Commons Attribution License (CC BY). The use, distribution or reproduction in other forums is permitted, provided the original author(s) and the copyright owner(s) are credited and that the original publication in this journal is cited, in accordance with accepted academic practice. No use, distribution or reproduction is permitted which does not comply with these terms.
*Correspondence: Kui Li, bGlrdWlAY2Fhcy5jbg==; Hua Li, b2todWFsaUBmb3N1LmVkdS5jbg==