- 1Institute of Food Safety, Food Technology and Veterinary Public Health, University of Veterinary Medicine, Vienna, Austria
- 2Austrian Competence Center for Feed and Food Quality, Safety and Innovation FFoQSI GmbH, Tulln, Austria
A large part of foodborne outbreaks related to Listeria monocytogenes are linked to meat and meat products. Especially, recontamination of meat products and deli-meat during slicing, packaging, and repackaging is in the focus of food authorities. In that regard, L. monocytogenes persistence in multi-species biofilms is one major issue, since they survive elaborate cleaning and disinfection measures. Here, we analyzed the microbial community structure throughout a meat processing facility using a combination of high-throughput full-length 16S ribosomal RNA (rRNA) gene sequencing and traditional microbiological methods. Samples were taken at different stages during meat cutting as well as from multiple sites throughout the facility environment to capture the product and the environmental associated microbiota co-occurring with Listeria spp. and L. monocytogenes. The listeria testing revealed a widely disseminated contamination (50%; 88 of 176 samples were positive for Listeria spp. and 13.6%; 24 of 176 samples were positive for L. monocytogenes). The pulsed-field gel electrophoresis (PFGE) typing evidenced 14 heterogeneous L. monocytogenes profiles with PCR-serogroup 1/2a, 3a as most dominant. PFGE type MA3-17 contributed to the resilient microbiota of the facility environment and was related to environmental persistence. The core in-house microbiota consisted mainly of the genera Acinetobacter, Pseudomonas, Psychrobacter (Proteobacteria), Anaerobacillus, Bacillus (Firmicutes), and Chryseobacterium (Bacteroidota). While the overall microbial community structure clearly differed between product and environmental samples, we were able to discern correlation patterns regarding the presence/absence of Listeria spp. in both sample groups. Specifically, our longitudinal analysis revealed association of Listeria spp. with known biofilm-producing Pseudomonas, Acinetobacter, and Janthinobacterium species on the meat samples. Similar patterns were also observed on the surface, indicating dispersal of microorganisms from this multispecies biofilm. Our data provided a better understanding of the built environment microbiome in the meat processing context and promoted more effective options for targeted disinfection in the analyzed facility.
Introduction
Meat and meat products are potential vectors for foodborne pathogens, mostly Salmonella enterica, shiga toxin-producing Escherichia coli, Campylobacter spp., and Listeria monocytogenes (Fegan and Jenson, 2018; European Commission, 2020). Listeria monocytogenes causes listeriosis, a food-borne disease with a high case-fatality rate (15.6%) that has recently experienced a significant increase despite improvements of control measures [European Food Safety Authority and European Centre for Disease Prevention and Control (EFSA and ECDC), 2018]. A large part (5 out of 12) of foodborne outbreaks related to L. monocytogenes are linked to meat and meat products (EFSA Panel on Biological Hazards, 2019). Especially, recontamination of meat products and deli-meat during slicing, packaging, and repackaging is in the focus of the food control authorities (Churchill et al., 2019). The worldwide largest listeriosis outbreak in South Africa during 2017–2018 (1,060 listeriosis cases, 216 deaths) was traced back to a heat-treated meat product produced from a single meat producer (Allam et al., 2018; Kaptchouang Tchatchouang et al., 2020). Recent outbreaks have also been reported in Europe and the United States (European Centre for Disease Prevention and Control, European food safety authority, 2019; Center for Disease Control and Prevention, 2020).
The surge in listeriosis outbreaks has challenged the existing risk assessments and raised questions about the lifestyle of listeria species within the context of food processing environments (FPE). In that regard, the recurrent isolation and/or persistence of L. monocytogenes on food contact surfaces (FCS) and processing environments (non-FCS, NFCS) is directly related to its global spread (Nyarko et al., 2018; Harrand et al., 2020; Kaszoni-Rückerl et al., 2020). The ability of certain L. monocytogenes genotypes to adapt to FCS and NFCS in the FPE is of major concern. There are different factors that greatly influence the capability of L. monocytogenes to survive in FPE, such as their capability to adapt to low temperatures, sublethal concentrations of disinfectants, and high salt concentrations, and to grow in multi-species biofilms (Galié et al., 2018; Rodríguez-López et al., 2018; Alvarez-Ordóñez et al., 2019; Rodríguez-Melcón et al., 2019).
Insights on the behavior of L. monocytogenes in mono-or multi-species biofilms are gained from static or continuous flow models, but the presence of L. monocytogenes in FPE biofilms and the potential role of the co-existing microorganisms have not been fully explored (da Silva Fernandes et al., 2015; Dzieciol et al., 2016; Ripolles-Avila et al., 2019; Mendez et al., 2020; Rodríguez-López et al., 2020; Wagner et al., 2020). Pseudomonas, Serratia, Brochothrix, Psychrobacter, Acinetobacter, Staphylococcus, lactic acid bacteria, and aerobic and anaerobic spore-formers contribute to the resilient microbiota in meat processing plants (Stellato et al., 2016; Fagerlund et al., 2017). Understanding how the individual members of microbial communities influence each other is key in developing foodborne pathogen mitigation strategies (Van Reckem et al., 2020). It is therefore essential to investigate the microbial diversity in food processing plants and the potential relationships between the microbial community compositions with the occurrence of L. monocytogenes (Tan et al., 2019). The development of high-throughput sequencing technologies made it possible to study microbial populations in their natural environment, enabling researchers to gain snapshots of the world of microorganisms from broader and deeper perspectives (Cao et al., 2017; Van Reckem et al., 2020).
Several studies have reported the importance of the co-existing microbiota for L. monocytogenes establishment and survival. For example, Spor et al. (2020) reported that the composition of the soil microbiota is critical for the prevention of L. monocytogenes establishment. Streptomyces and Brevibacillus were described to produce secondary metabolites or bacteriocins with inhibitory effects on L. monocytogenes. Resident microorganisms of FPE (e.g., Lactobacillus plantarum, Pseudomonas fluorescens, Pseudomonas putida, Enterobacteriacae, or sulfide-producing bacteria) were shown to either protect or inhibit L. monocytogenes after sanitation (Rodríguez-López et al., 2018). This ambiguous behavior led us to investigate the characteristics of listeria presence in the context of whole microbial communities. We hypothesize that specific microbial community members are highly abundant in the presence of listeria in a meat cutting plant and act together to contribute to its persistence.
Therefore, we characterized the microbiological status, including the Listeria spp. and L. monocytogenes occurrence, by applying microbiological (ISO reference methods) and molecular biological methods [16S ribosomal RNA (rRNA) gene sequencing, L. monocytogenes serogroup PCR] in a meat cutting plant. Long read high-throughput sequencing technologies were applied to capture the complexity of microbial populations associated with the presence or absence of listeria. The study aims to provide insights into the presence of listeria and the associated microbiota during meat processing and indicates potential dynamic interactions between them. Thus, our results foster the development of novel concepts for control measures to reduce listeria presence on meat cuts delivered for the production of ready-to-eat meat products.
Materials and Methods
Facility Structure and Sampling
Samples for this study were taken at a large-scale meat cutting facility with a cutting capacity of 700 pork halves per hour in Austria in August 2017. Throughout the day, 10 carcass halves from three different producers (named A, B, and C) were monitored. Each half was marked upon arrival at the meat cutting plant during offloading at the cooling chamber and then subjected to primal cutting one by one, so that the same pieces could be followed and re-sampled throughout the entire processing chain (Supplementary Figure S1). At primal cutting each carcass half was cut into shoulder, leg, belly, and loin portions, which were then passed to a separate processing line. In this study, the leg and loin portions were focus of longitudinal sampling. The legs were pre-cut and held in the cooling chamber before re-joining the processing line. After cutting, the finished cuts were put in clean transport boxes, transported to the second floor, and vacuum packaged.
Carcass, meat, and environmental samples were taken with sterile polyurethane sponges using 100 cm2 templates (Item number SR-10NB-HDPUR-G, World Bioproducts, Woodinville, United States). First, a sterile template was placed on the sampling area then, wearing fresh sterile gloves, the hydrated sponge was taken out of the sample bag, swabbed for 10 s horizontally, then flipped and swabbed again for 10 s in vertical direction. Subsequently, the sponge was placed back into the sample bag, which was sealed and stored in the cooling chamber of the facility (4°C) until sampling was finished. Immediately after sampling, all samples were transported to the laboratory on ice to process them on the same day. Sponges were manually squeezed inside the bag to release fluid (~8 ml), which was pipetted into two separate 15 ml falcon tubes in equal proportions. One of the tubes was directly used for microbiological investigations and the other one was stored at −20°C for DNA-extraction on a later date. In total, 176 sponge samples were taken from different processing positions (product – P, n = 88; food contact surfaces – FCS, n = 48; personnel – PE, n = 32; non-FCS – NFCS, n = 8). Microbiome analysis were performed by using 16S rRNA gene amplicon sequencing on a total of 113 samples composed of 47 meat samples (carcass associated, n = 10; leg associated, n = 19; loin associated, n = 18) and 66 environmental associated samples (FCS, n = 32; PE n = 32; NFCS, n = 2). These 113 samples showed higher microbial loads according to the microbiological investigation and were therefore selected for the microbiome analysis.
Microbiological Investigation and DNA Extraction for Isolate Confirmation
The enumeration of aerobic mesophilic counts (AMC; ISO 4833-2:2013) and hygiene indicator bacteria (Enterobacteriaceae – EB; ISO 21528-2:2017 and Pseudomonadaceae – PS) was performed after preparing a 10-fold serial dilution in buffered peptone water, (Thermo Fisher Scientific Inc., Oxoid Ltd., Basingstoke, United Kingdom) up to dilution −108. The dilutions were plated in duplicates on Plate Count agar (PCA, Thermo Fisher Scientific Inc., Oxoid Ltd.), Violet Red Bile Glucose agar (VRBG, Thermo Fisher Scientific Inc., Oxoid Ltd.), and Glutamate Starch Phenol Red agar (GSP, Merck KGaA; Darmstadt, Germany) by surface plating technique. GSP and VRBG agar were incubated at 25 and 37°C for 24–48 h. PCA was incubated at 30°C for a maximum of 72 h. To determine the AMC/EB and PS counts/cm2, microbial colonies between 10 and 300 colony forming units (CFU) were included in the calculation. Presumptive EB and PS isolates (n = 5 each) were confirmed by Oxidase reaction, biochemical profiling up to genus level for PS, up to species level for EB (API 20E, API Rapid ID32E; bioMérieux Marcy-l’Étoile, France).
Listeria species detection and isolation was performed according to ISO 11290-1, modified by prolonged incubation of half Fraser enrichment to 48 h in order to increase the detection limit of the technique (ISO 11290-1, 2017). Swabs were enriched in 50 ml Half-Fraser broth (HFB; Merck KGaA) for 48 h at 30°C. After incubation, 0.1 ml HFB was transferred to 10 ml Fraser broth (FFB; Merck KGaA) and incubated for 48 h at 37°C. HFB and FFB were streaked on listeria agar acc. to Ottaviani and Agosti (ALOA; Merck KGaA). Up to five L. monocytogenes typical colonies and/or two Listeria spp., typical colonies without L. monocytogenes specific phosphatidylinositol-specific phospholipase C reaction were subcultivated on Tryptone Soy agar supplemented with 0.6% (wt/vol) yeast extract (TSAY; Oxoid Ltd.) for isolate based PCR confirmation and cryo-conservation. The DNA extraction for bacterial isolates was performed according a protocol published by Walsh et al. (2013). Briefly, 1–2 bacterial colonies were suspended in 100 μl 0.01 M Tris HCl Buffer (Sigma Aldrich Corp., St. Louis, MO, United States) and centrifuged for 5 min at 8,000 rpm. Subsequently, 400 μl Chelex® 100-Resin (BioRad, Hercules, CA, United States) was added to the bacterial suspension, heated for 10 min at 100°C on a thermoblock, and centrifuged at 14,000 × rpm for 5 s. The DNA supernatant was transferred to sterile tubes and stored at −20°C.
Listeria spp. PCR-Based Confirmation, and Listeria monocytogenes Serogroup PCR
Listeria spp. was differentiated by a PCR-multiplex approach targeting the invasion-associated protein (iap) gene. The PCR resulted in L. monocytogenes, Listeria innocua, the Listeria seeligeri-Listeria welshimeri-Listeria ivanovii group, or Listeria grayi, respectively (Bubert et al., 1999). The gel electrophoresis of PCR-amplicons was performed in a 1.5% agarose gel containing 0.5× Tris-Borate-EDTA (TBE) buffer and 3.5 μl peqGREEN DNA gel stain (VWR International, Radnor, United States), at 120 V for 25 min. The DNA standard Thermo Scientific™ GeneRuler™ 100 bp (Thermo Fisher Scientific Inc., Waltham, United States) was applied for fragment length comparison. The L. monocytogenes isolates were further confirmed by serogroup PCR according to Doumith et al. (2004) and Leclercq et al. (2011) by targeting the marker genes lmo0737, lmo1118, ORF2819, and ORF2110 and the Listeria spp. specific prs gene (Doumith et al., 2004; Leclercq et al., 2011).
Listeria monocytogenes Pulsed-Field Gel Electrophoresis
The genetic correlations among L. monocytogenes isolates were analyzed by pulsed-field gel electrophoresis (PFGE) according to CDC PulseNet standardized protocol (https://www.cdc.gov/pulsenet/PDF/listeria-pfge-protocol-508c.pdf; accessed on: 14-10-2020). The DNA fragments were digested with restriction enzyme AscI (50 U, 37°C, 4 h; Thermo Fisher Scientific Inc., Waltman, MA, United States) and electrophoretically separated using CHEF-DR III system (Bio-Rad Laboratories Inc., Hercules, CA, United States). The universal standard Salmonella ser. Braenderup H9812 was digested with 50 U XbaI (Thermo Fisher Scientific Inc.) at 37°C for 4 h. PFGE results were analyzed using BioNumerics 6.6 software (Applied Maths, Sint-Martens-Latem, Belgium), and similarity between the AscI macrorestriction profiles was determined with Dice coefficient (position tolerance 1.5%). Clustering and construction of dendrograms were performed by using the unweighted pair-group method with arithmetic averages (UPGMA). PFGE types were considered identical when the patterns were indistinguishable. The Simpson’s Index of diversity was calculated with the online tool of Comparing Partitions (http://www.comparingpartitions.info/; accessed on: 14-10-2020).
DNA-Extraction and 16S rRNA Gene Sequencing
Samples selected for microbiome analysis were centrifuged at 3,220 × rcf for 20 min, and the pellet was resuspended in 400 μl of 1 × phosphate buffered saline (PBS) before DNA-extraction, in order to increase microbial cell density and neutralize inhibiting sanitizers. The DNA was then extracted from 200 μl with the QIAamp® DNA Stool Mini Kit (Qiagen GmbH, Hilden, Germany) according to manufacturer instructions. The elution step of the protocol was modified; performed two times with 50 μl DEPC treated water instead of one time with 200 μl AE buffer. Negative controls (DEPC treated sterile water), one for each used kit, were extracted together with the rest of the samples. The DNA concentration of the samples was measured with the Qubit dsDNA HS Assay Kit and Qubit 2.0 Fluorometer (Invitrogen, Thermo Fisher Scientific, Oregon, United States).
The near-full-length 16S rRNA gene amplicon libraries were prepared and sequenced according to the official Pacbio guidelines1 at the Vienna Biocenter Core Facilities NGS Unit.2 Amplicons were prepared from 113 samples (plus three negative controls) using bacteria specific primers 27F (5'-AGRGTTYGATYMTGGCTCAG-3') and 1492R (5'-RGYTACCTTGTTACGACTT-3'). In a second round of amplification, barcodes were added with Pacbio Barcoded Universal primers, so that the amplicons could be multiplexed on three SMRT cells. Sequencing was carried out on a Pacbio Sequel machine with 2.1 chemistry.
Sequence Processing, Analysis, and Statistics
On average, each SMRT cell yielded 5,541,444 subreads which were used for circular consensus sequence (ccs) generation. The ccs command from the bioconda package pbccs v3.13 was run with the minimum predicted accuracy set to 0.99 and the minimum number of passes set to 3, resulting in a mean of 246,017 ccs reads per SMRT cell. The following sequence processing pipeline was then run on files from each SMRT cell individually. Sequences were demultiplexed and then further processed through the long-read DADA2 pipeline v1.14 in the R environment v3.6.2 (R Core Team, 2019) which measures the full-length 16S rRNA gene with single-nucleotide resolution and a near-zero error rate (Callahan et al., 2016, 2019). First, primers were trimmed with “removePrimers” and low-quality sequences were filtered using “filterAndTrim” with a maximum number of expected errors of 2. Then, the remaining reads were dereplicated and used to learn the error rates. Amplicon sequence variant (ASV) tables were generated after applying the sample inference algorithm to the dereplicated data. In the next step, individual ASV tables were merged and chimeras were removed. Finally, ASVs were classified to the SILVA rRNA database version SSU 138 (Quast et al., 2013).
All statistical analysis of the microbiome sequencing data was performed in R v3.6.2. Initial data exploration, filtering, and basic microbial community analysis were conducted using the R package phyloseq v1.22.3 (McMurdie and Holmes, 2013). Samples with low sequencing depth (less than 200 reads per sample) and ASVs represented by less than five reads were excluded from the analysis. Furthermore, potential contaminant ASVs were identified and removed by using the decontam v1.1.1 package, using a presence-based contaminant identification with a threshold of 0.5, which identifies all sequences that are more prevalent in negative controls than in positive samples as potential contaminants (Davis et al., 2018). Alpha diversity indices for the investigation of microbial communities’ richness (Chao1 index; Chao, 1984), diversity (Shannon index; Shannon, 1948), and evenness (Simpson index; Simpson, 1949) were calculated and compared with vegan v2.5-6 (Oksanen et al., 2011) with pairwise comparisons using Wilcoxon rank sum tests and Benjamini-Hochberg adjustment for p-values. For beta diversity, the “adonis” function was applied to calculate a permutational analysis of variance (PERMANOVA) with 5,000 permutations. The ASV counts of each sample were set as the dependent variable and the factors “Type,” “Position,” “Listeria,” and “Carcass” were used as independent variables. The dissimilarity in community composition was visualized in ampvis2 v2.6.6 by means of a non-metric MultiDimensional Scaling Analysis (NMDS) based on Bray-Curtis dissimilarities as a distance measure (Andersen et al., 2018). The relative abundances of individual taxa were calculated and illustrated as barplots (Phyla) or heatmaps (Top 50 ASVs) in phyloseq and ampvis2, respectively. Shared ASVs between meat samples entering and leaving the facility were computed with the function “amp_venn” with an abundance cutoff of 0.01 and a frequency cutoff of 10 in ampvis2. The cutoff values were chosen to exclude potential sequencing artifacts and include ASVs that were observed in the majority (80%) of samples from one sampling position.
Correlations between individual taxa and the presence of listeria were calculated by applying spearman rank correlations in the function “associate” from the package microbiome v1.11.2 (Lahti and Shetty, 2017). Furthermore, taxa with significant differential abundance between samples in which Listeria spp. were present or absent were identified and visualized with the R package DESeq2 v1.26 (Love et al., 2014). Only statistically significant differences (p < 0.05) were plotted. Chryseobacterium and Psychrobacter related ASVs that were identified in the DESeq2 analysis were aligned and classified using the Silva Incremental Aligner v1.2.11 including 25 neighbors per query sequence (Pruesse et al., 2012). The phylogenetic trees were calculated using the maximum likelihood method implemented in MEGA X with the number of bootstrap replicates criteria set to 500 (Kumar et al., 2018). The Kimura 2-parameter model of sequence evolution was used for tree reconstruction (Kimura, 1980).
Results
Aerobic Mesophilic and Pseudomonadaceae Counts Increased in the Course of Processing
The AMC, Pseudomonas (PS), and Enterobacteriaceae (EB) counts on meat samples were on average 4.96, 2.32, and 2.41 log CFU/cm2 at the time of their delivery to the cooling chamber at the meat cutting plant (AMC range 4.48–5.40 log CFU/cm2; PS range < 1.0–4.85 log CFU/cm2; EB range < 1.0–4.48 log CFU/cm2; Supplementary Figure S2).
Aerobic mesophilic counts increased by 2.38 log during cutting of leg samples (7.34 mean log CFU/cm2) to critical limits (as stated in DGHM, 2014; 6.7 log CFU/cm2) and by 0.22 log (5.18 mean log CFU/cm2) on loin samples. Environmental samples harbored 5.17 log CFU AMC/cm2 on average. Pseudomonas levels showed a similar distribution pattern across the facility, but had a higher variation among them, i.e., lower numbers at the beginning of the processing line (Cooling chamber: 2.32 log CFU/cm2), and higher numbers at the end (leg after packaging: 4.78 mean log CFU/cm2 and loin after packaging: 4.21 mean log CFU/cm2). On the contrary, Enterobacteriaceae had relatively high numbers in the cooling chamber (2.41 mean log CFU/cm2) but were not detected during meat cutting and packaging. On environmental samples, Enterobacteriaceae was detected in samples collected from the wall in the cooling chamber, the conveyor belt at prime dissection and on hands and shoes of employees at the locks. In 10.2% (9/88) and 13.6% (12/88) of meat samples, the Enterobacteriaceae and Pseudomonas spp. warning and guide values of the DGHM recommendation (5 and 6 log CFU/g) were exceeded.
Listeria spp. and Listeria monocytogenes Confirmation and Subtyping
All samples (n = 176) were tested for the presence of listeria species according to ISO 11290-1. Half of the samples were confirmed by PCR method as Listeria spp. (n = 88). About 82 (46.6%) and 24 (13.6%) of the samples were contaminated by apathogenic Listeria spp. and L. monocytogenes, respectively. Thereof, 18 samples (10.22%) contained both apathogenic listeria species and L. monocytogenes. The minority of samples was contaminated by exclusively L. monocytogenes (n = 6; 3.4%).
Interestingly, listeria was absent from carcass samples (producers A–C), hooks and wall on delivery to the cooling chamber, but was present due to cross-contamination events during meat cutting and packaging (Figure 1). Samples collected from the surface of equipment, machines or the processing environment showed a heterogeneous distribution of listeria, indicating a wide dissemination through the facility. Initial personnel associated samples (PE) tested positive for Listeria spp. and L. monocytogenes were shoes after passing the hygiene barrier. The saw and conveyor belt at meat cutting was the next contaminated environmental sampling site (FCS). At the station for fine cutting of the leg hook were heavily contaminated with Listeria spp. and L. monocytogenes (no negative sample). The leg was cross-contaminated from the environment at deboning, before and after packaging. Listeria spp. was present on conveyor belts, knives, and personnel associated samples (gloves, apron). Following sampling sites were tested Listeria spp. and L. monocytogenes positive at the station for fine cutting of the loin: the meat cuts, the ripping board (FCS), conveyors (FCS), aprons (PE), and the meat before packaging. Apathogenic Listeria spp. was detected on products after ripping, packaging, packaging film (FCS), and gloves (PE).
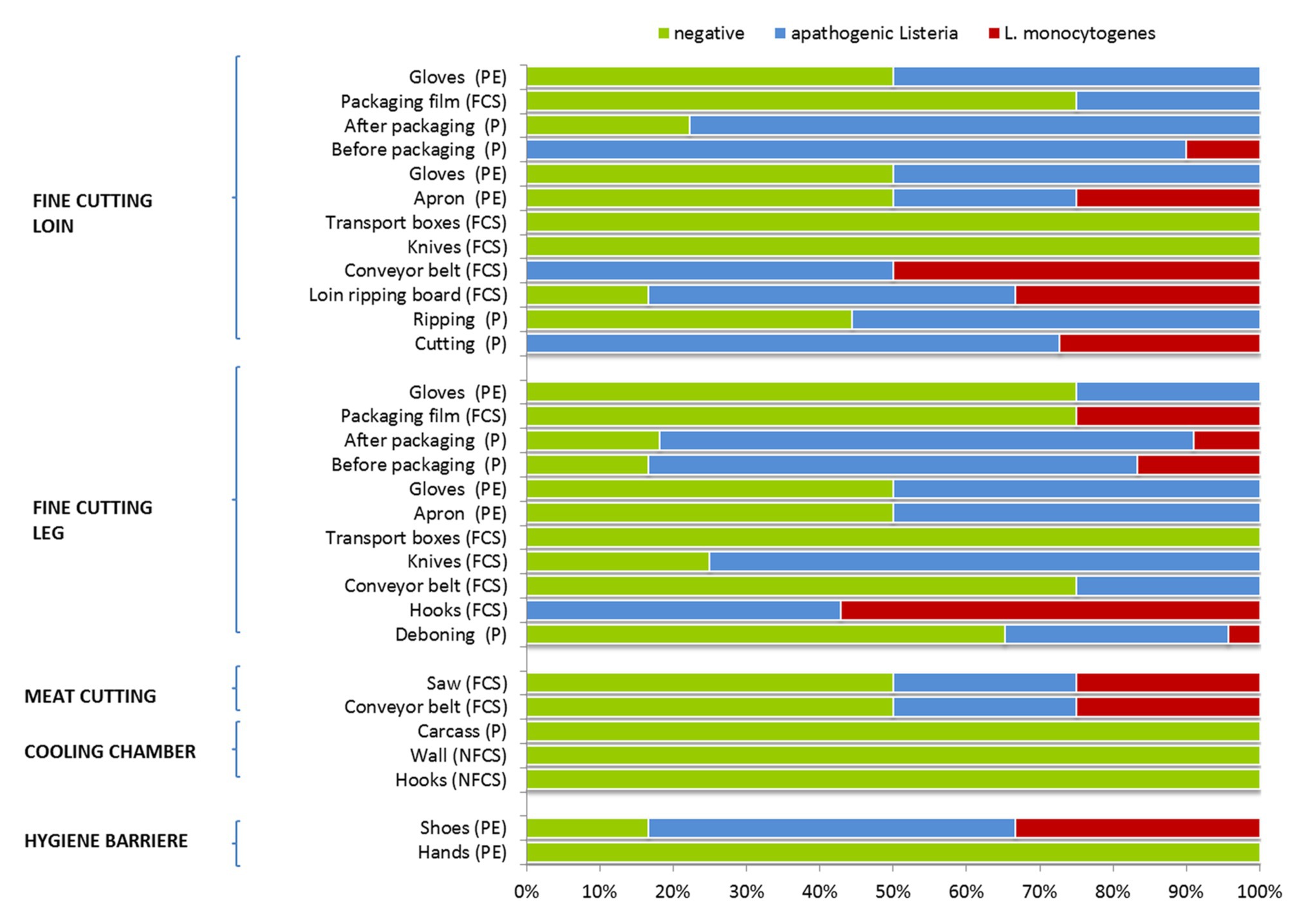
Figure 1. Occurrence of apathogenic listeria and Listeria monocytogenes during meat cutting. Number of samples positive or negative for Listeria spp. for each sampling position. Sample categories are abbreviated: “product” (P), “food contact surface” (FCS), “non-FCS” (NFCS), and “personnel” (PE).
The PCR serotyping of 64 L. monocytogenes isolates resulted to a majority in PCR serogroup 1/2a, 3a, except for one isolate (1/2c, 3c; both genetic lineage II; Figure 2). The PFGE typing applying AscI revealed 14 distinct L. monocytogenes profiles with a Simpson’s Index of Diversity of 0.679 (CI 95%, 0.559–0.798). About 54.2% (n = 13/24) of L. monocytogenes positive samples harbored up to two or three different PFGE types in parallel. The most abundant PFGE profile was MA3-17 (n = 35 isolates; 54.7%), followed by MA13-17 (n = 9; 14.1%), and MA6-17 (n = 5; 7.8%). The UPGMA cluster analysis applying the dice coefficient indicated a similarity of 90% for L. monocytogenes MA3-17 and MA5-17. Listeria monocytogenes PFGE profile MA3-17 was present on shoes after passing the hygiene barrier, on the saw during cutting (FCS), hooks at the fine cutting of legs (FCS), at the packaging site of legs, and at the fine cutting of loin (FCS-conveyor belt, PE-aprons), indicating cross-contamination from the environment and personnel as vectors. MA3-17 may already contribute to more than one cross-contamination event and can be related to environmental persistence. The PFGE profile MA13-17 only appeared during the fine cutting of the loin [including a personnel vector (apron)] and at the packaging site of the leg. Profile MA6-17 appeared initially at the hooks of the leg, at the leg after deboning and at packaging. Additionally, PFGE profile MA6-17 was found at the ripping board of the loin. The hooks of the leg indicated inefficient sanitation due to the presence of up to four different PFGE-profiles (MA3-17, MA5-17, MA6-17, and MA15-17). The shoe sanitation at the hygiene barrier did not properly remove PFGE-profiles MA3-17, MA7-17, and MA817. Saw and conveyor belts also contained heterogeneous L. monocytogenes PFGE-types.
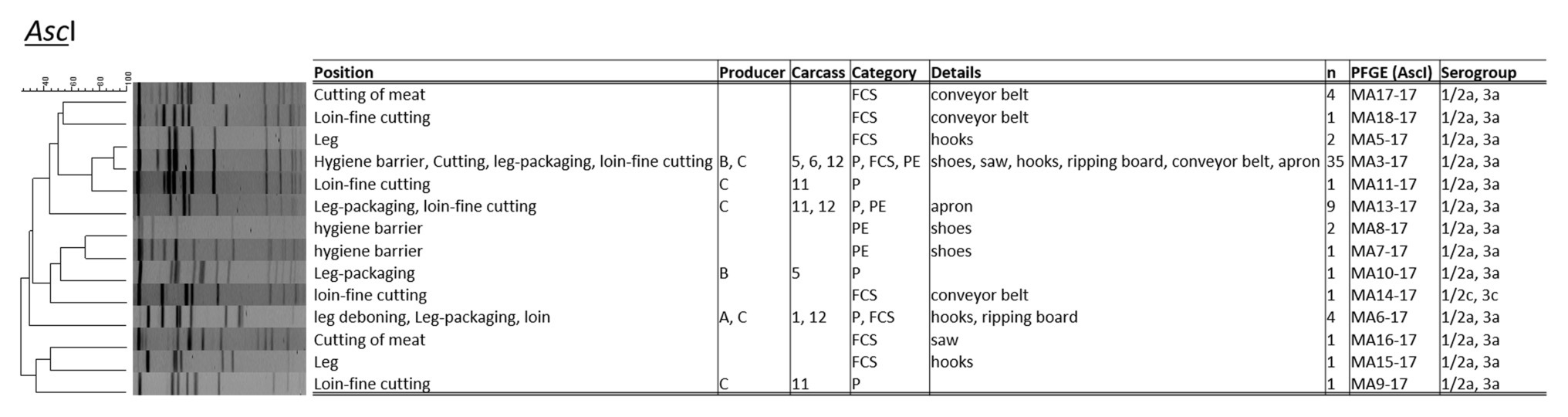
Figure 2. Pulsed-field gel electrophoresis (PFGE) cluster analysis with restriction enzyme AscI for L. monocytogenes (n = 58) isolated during meat cutting.
The Microbial Community Structure on Meat Changes During Processing
In total, 158,113 full-length 16S rRNA gene sequences and 1,373 ASVs passed our stringent quality, prevalence, and decontamination filtering thresholds, resulting in 1,777 sequences per sample on average. We estimated microbial biodiversity within samples using different alpha diversity indices (Chao1, Shannon, and Simpson index). Species richness (Chao1) was highest on meat samples taken at the beginning of the processing line and lowest for samples taken from the leg (Figure 3A). ASVs were similarly evenly distributed across all sampling positions, as shown by the Simpson index. The Shannon index shows that the species diversity was lower in leg samples compared to loin samples when abundance is considered. However, beta diversity analysis revealed that microbial communities on meat showed a strong patterning according to sampling position (Figure 3B). Samples taken at the cooling chamber cluster apart from leg and loin samples, which are also separated from each other. Interestingly, microbiomes of leg cutting samples were also different from microbiomes of leg packaging samples, whereas loin samples exhibited a similar microbial community structure throughout processing. To statistically test whether the microbial communities of different sample groups differ from each other, we calculated a permutational analysis of variance (Supplementary Table S1). The factors “Type” (meat or environmental sample) and “Position” (sampling positions) were the most significant factors (p < 0.001) responsible for differences in the microbiome. Moreover, the presence of listeria (Factor “Listeria”: present or not present) had a significant impact (p = 0.04879) on the structure of microbial communities. The factor “Carcass” (Meat from carcass A–C, etc.) was not significant, further substantiating that the microbiome of the same meat sample is not stable throughout processing and changes from start to end. The core microbiome on meat within the facility, defined here as all ASVs that occur in at least 10 samples with a minimum relative abundance of 0.01%, was comprised of 22 ASVs with a combined relative abundance of 17% across all samples (Supplementary Figure S3). Taxonomic classification of these 22 ASVs revealed Acinetobacter, Pseudomonas, Psychrobacter (Proteobacteria), Bacillus (Firmicutes), and Chryseobacterium (Bacteroidota) as the most represented genera (Supplementary Table S2).
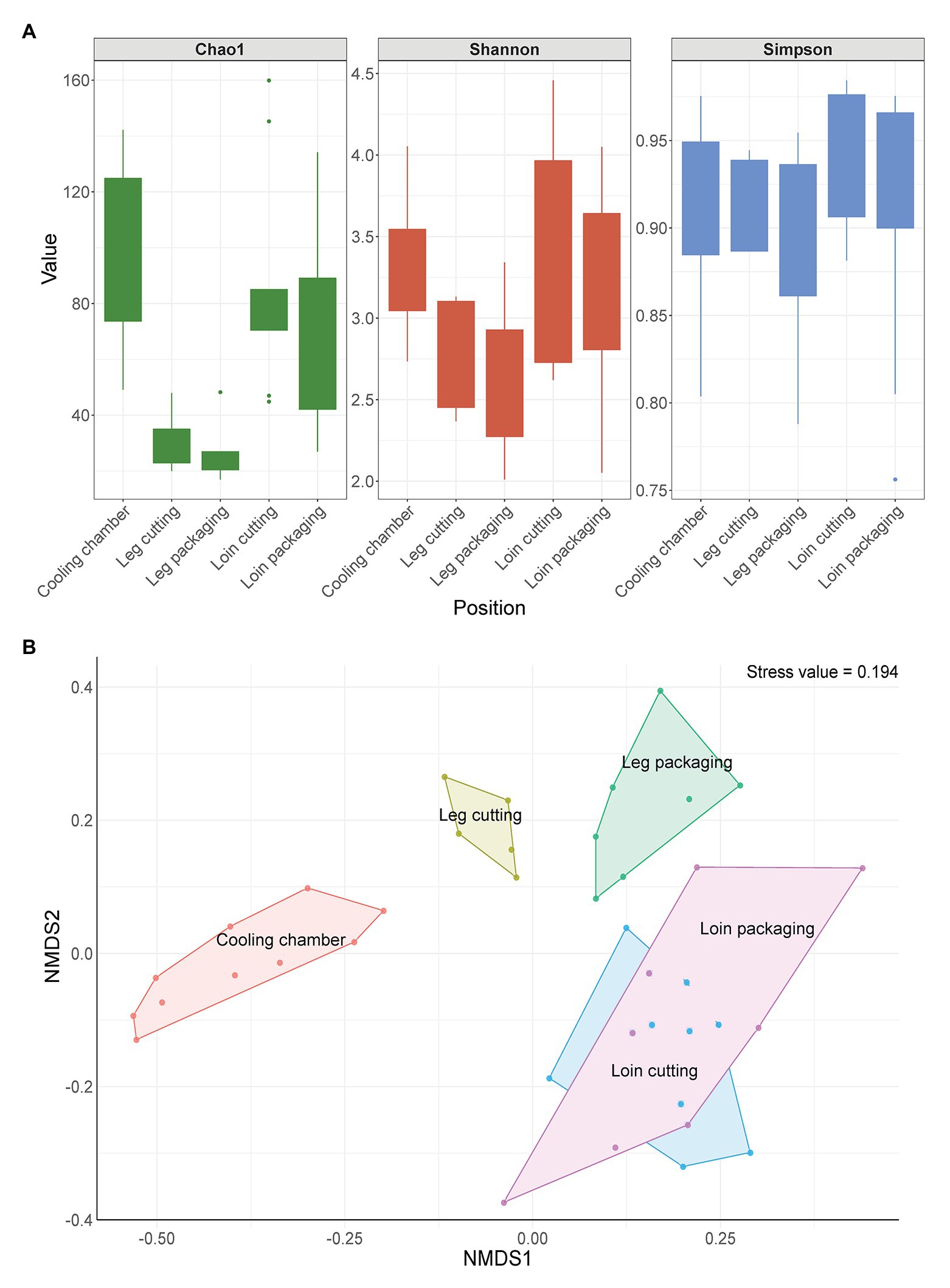
Figure 3. (A) Change in alpha diversity indices of meat samples over time. Boxes indicate the interquartile range (from 75 to 25th) of the data. Whiskers extend to the most extreme value within 1.5 times interquartile range and dots represent outliers beyond that range. (B) Non-metric multidimensional scaling (nMDS) plot of Bray-Curtis distances based on 16S ribosomal RNA (rRNA) gene libraries obtained from meat samples. Each point represents values from individual libraries with colors expressing meat samples from different positions along the processing line.
Taxonomic Composition of Meat and Surface Samples During Meat Processing
The microbiome on meat was dominated by Firmicutes (43.35%), Proteobacteria (26.50%), Bacteroidota (17.26%), and Actinobacteriota (10.76%) when the carcasses were delivered to the facility (cooling chamber, Figure 4). While Actinobacteriota (leg packaging: 7.76%; loin packaging: 8.22%) remained stable over the course of processing, the relative abundance of Bacteroidota (5.42%; 2.00%) and Firmicutes (37.42%; 32.01%) decreased and Proteobacteria (49.41%; 56.79%) increased toward the end of the processing line. Primary phyla on surface samples were Firmicutes and Proteobacteria, although they displayed a diverse microbial community in general (Supplementary Figure S4). To resolve these differences on a finer scale, we looked at the 50 most abundant genera across all samples (Figure 5).
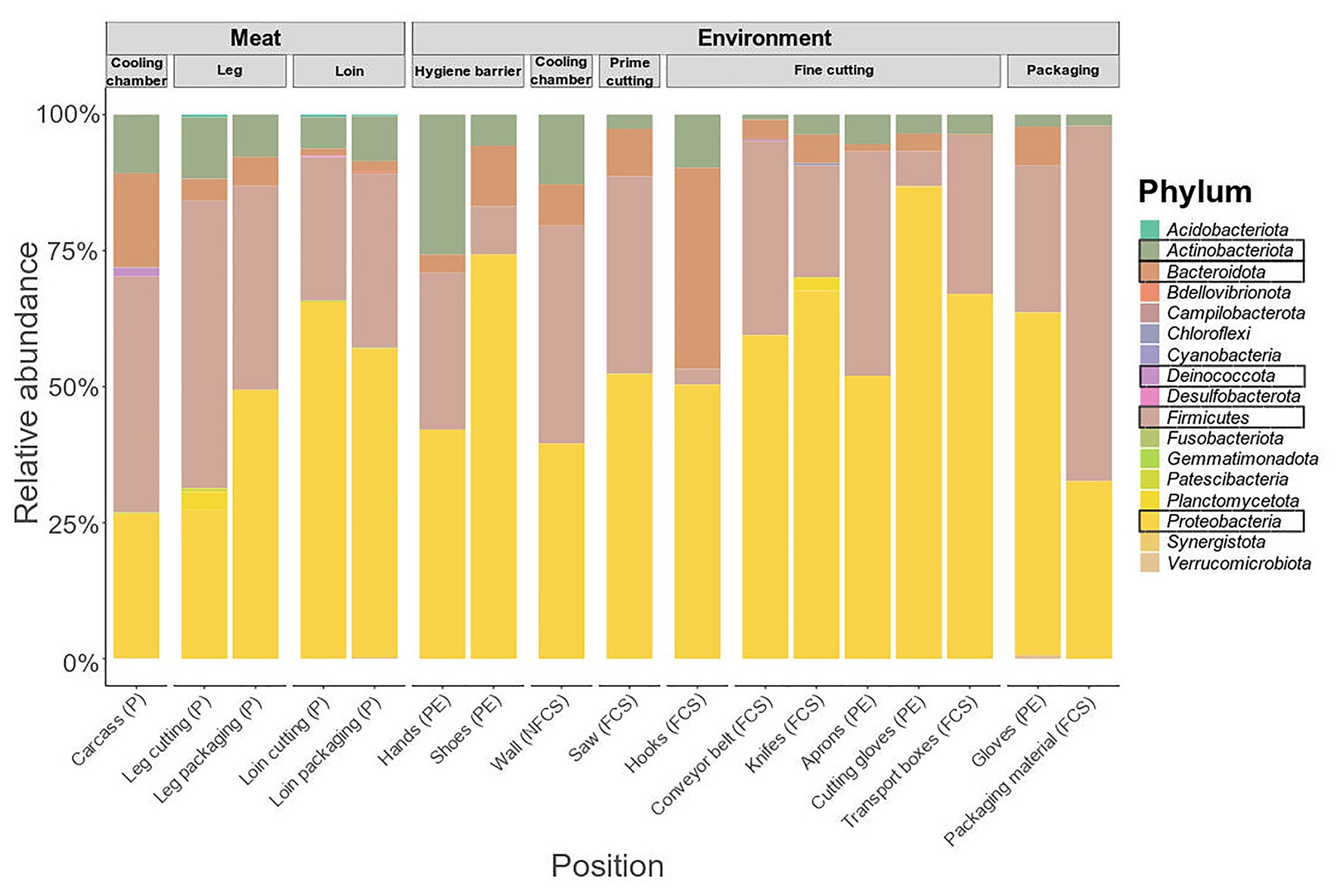
Figure 4. Phylum-level classification of 16S rRNA gene sequence reads parted by sampling position and type (meat or environment). Data represent average of amplicon sequence variant (ASV) counts from replicate libraries for each category. Sample categories are abbreviated: “product” (P), “food contact surface” (FCS), “non-FCS” (NFCS), and “personnel” (PE).
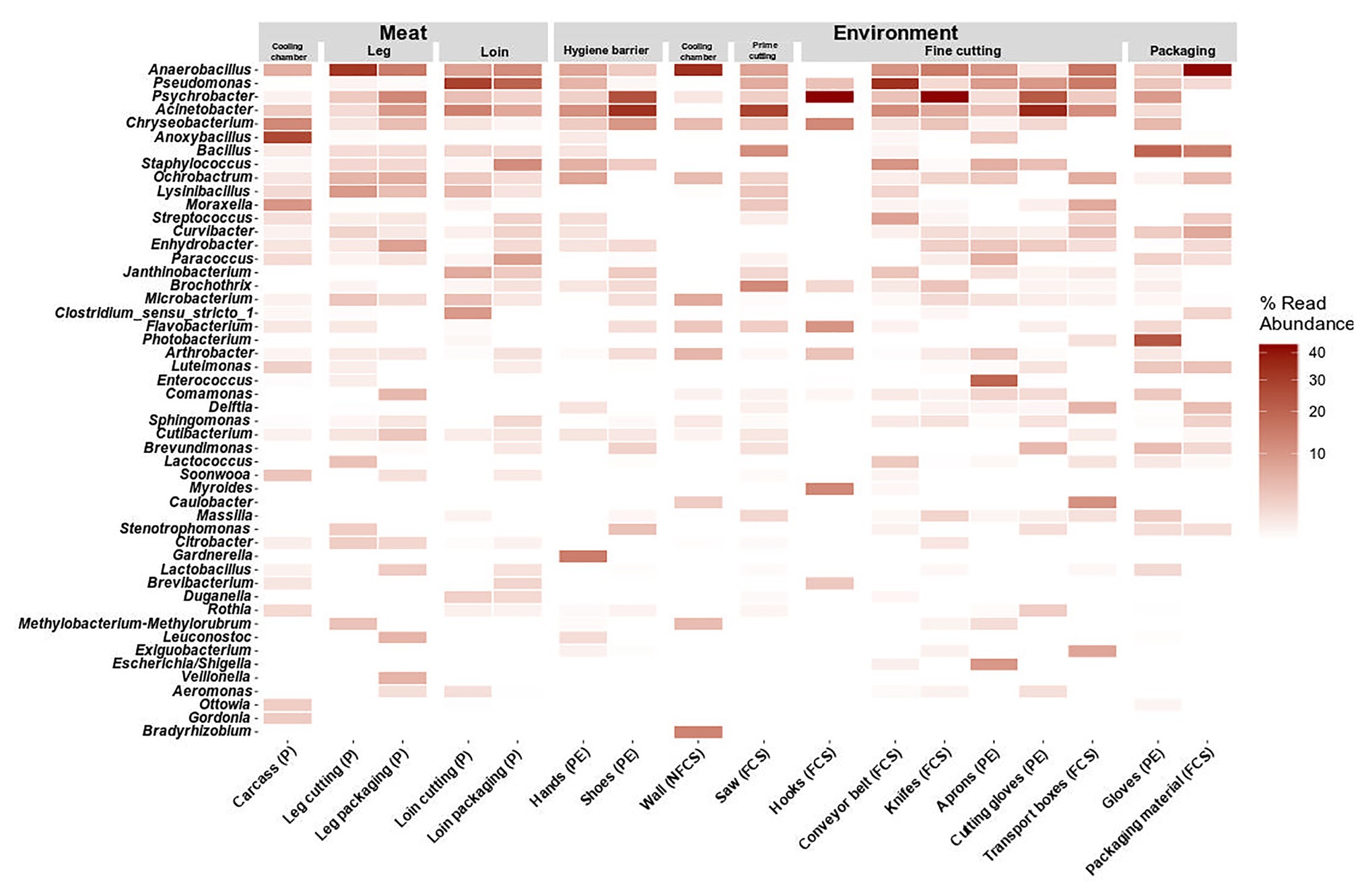
Figure 5. Heatmap of the top 50 most abundant genera grouped by position and split by type. Data represent average of ASV counts from replicate 16S rRNA gene libraries for each category. Sample categories are abbreviated: “product” (P), “food contact surface” (FCS), “non-FCS” (NFCS), and “personnel” (PE).
At large, genera that were detected on the meat were also found in one or more additional environmental samples (Pseudomonas, Psychrobacter, Acinetobacter, Chryseobacterium, Anaerobacillus, and Anoxybacillus). Pseudomonas was not present initially in the cooling chamber (P and NFCS), but was strongly associated to loin cutting and packaging (P, FCS, and PE) and conveyor belts (FCS) indicating cross-contamination on these stations. Psychrobacter and Acinetobacter were highly present in the FPE (shoes, saw, hooks-leg, and knives) pointing out environmental reservoirs. Anaerobacillus was most often observed on walls of the cooling chamber, leg, and packaging material. Bacillus was detected at the saw and at the last station (packaging). Selective hotspots for specific microbiota members were observed on the carcass in the cooling chamber (Anoxybacillus), on hands at hygiene barrier (Gardnerella) on aprons at the fine cutting (Enterococcus), and gloves at packaging (Arthrobacter).
The Presence of Listeria is Associated With Higher Abundances of Diverse Genera
Since the prevalence of listeria was identified as a significant factor explaining shifts in the microbial community structure, we were interested in which specific microorganisms were linked to the presence or absence of listeria. Thus, we calculated Spearman rank correlations for each individual taxon between the two sample groups (Figure 6). Acinetobacter and Janthinobacterium were positively associated with the presence of listeria on both meat and surface samples. Other bacteria, e.g., Brachybacterium and Carnobacterium were positively correlated to listeria only on surface samples. Pseudomonas was the only genus that showed positive correlation with listeria only in meat but not in environmental samples. Chryseobacterium, Moraxella, and Anoxybacillus (core microbiome) correlated positively with surface samples with listeria but negatively with meat samples with listeria. This opposing pattern might be explained by a varying behavior of different species or subpopulations of these genera. Thus, we looked into the differential abundance of individual ASVs, disregarding phylogenetic subsumptions. Our analysis revealed that Chryseobacterium ASVs (Seq1075, Seq1073, and Seq1076) were significantly higher abundant in meat samples in which listeria was absent, whereas other ASVs (Seq1120, Seq1137) were higher abundant in surface samples with listeria (Figure 7). Similarly, ASVs within the genus Psychrobacter had disparate associations with listeria in surface samples. Phylogenetic trees revealed that these ASVs represent diverse phylogenetic clades, suggesting that these interactions are species or even strain-specific (Supplementary Figures S5, S6).
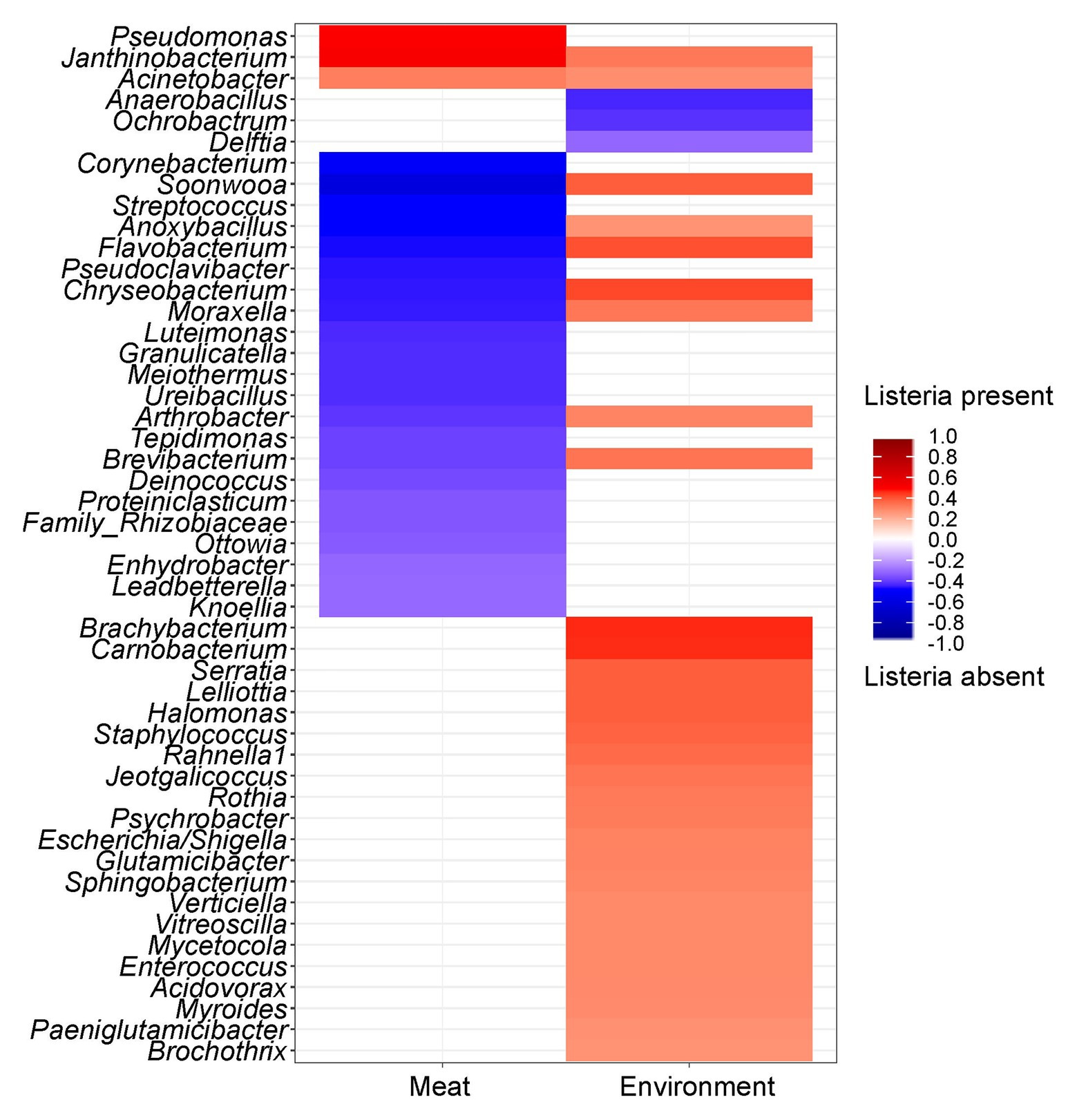
Figure 6. Heatmap showing the correlation of individual genera between samples with or without listeria. Red color shows a positive correlation and blue color illustrates a negative correlation with the presence of listeria. The saturation of the color indicates the strength of the correlation coefficient. Only significant (p ≤ 0.05) taxa are shown.
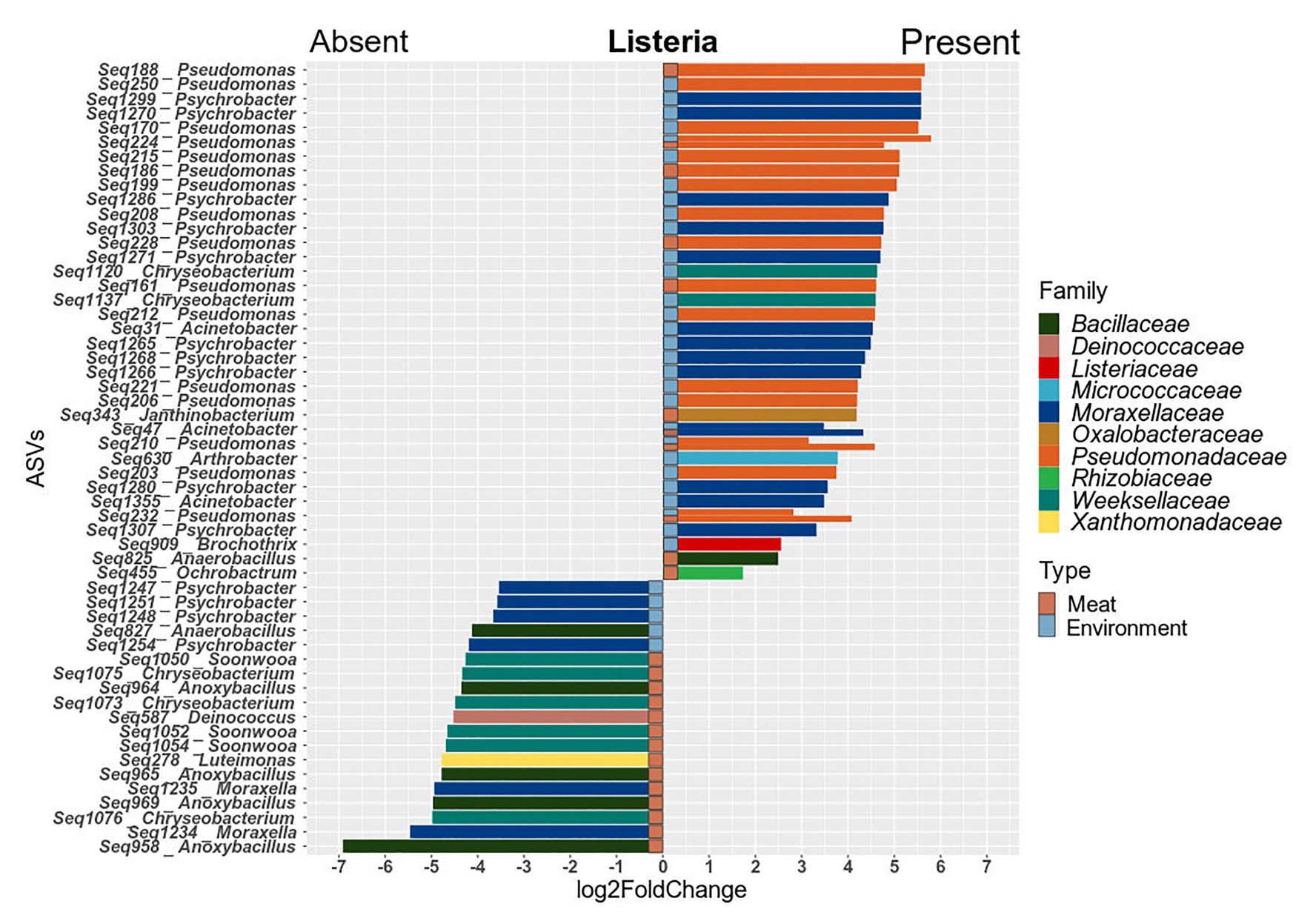
Figure 7. Barplots of ASVs that were significantly differentially abundant (p ≤ 0.05) between meat and surface samples with and without listeria. Positive values indicate higher abundance in samples with listeria and negative values depict higher abundance in samples where listeria is absent. Significant ASVs are plotted individually and colored according to their family-level classification.
Overall, ASVs that were significantly higher abundant in the processing environment in parallel with listeria were associated to the genera Pseudomonas, Acinetobacter, Psychrobacter, Arthrobacter, and Brochothrix. In meat samples, the genera Pseudomonas, Acinetobacter, Janthinobacterium, Ochrobactrum, and Anaerobacillus entailed ASVs that were co-occurring with listeria. Highly abundant Pseudomonas ASVs which could be assigned to species level were P. fragi (Seq203, 232, 210, 215, 195, 200, 222-meat, and environment), P. psychrophila (Seq228), and P. fluorescens (Seq161). The latter were abundant on hooks, conveyor belts, loin cutting, and packaging in parallel with L. monocytogenes PFGE profiles MA3-17, MA5-17, MA13-17, and MA14-17.
Discussion
Frequent listeriosis outbreaks throughout the last decade notably underlined the necessity of research on the persistence and ecology of L. monocytogenes within diverse food processing plants (Buchanan et al., 2017). Many studies investigated L. monocytogenes prevalence and traced outbreaks using whole genome sequencing (WGS) of isolates (Jackson et al., 2016; Halbedel et al., 2018; Pietzka et al., 2019). However, more research that delivers community wide information is necessary in order to understand why L. monocytogenes was able to persist and contaminate food from FPE.
Currently, one of the main methods to inactivate L. monocytogenes in ready to-eat meat products is high pressure processing, although it can affect organoleptic properties of the meat (Teixeira et al., 2014). Developing effective risk management strategies is dependent on unraveling mechanisms of interactions between L. monocytogenes and the built-environment microbiome that contribute to the persistence and recontamination of FPE with L. monocytogenes. Here, we applied high-throughput full-length 16S rRNA gene sequencing to investigate the presence of Listeria spp. in a meat processing plant in the context of whole bacterial communities. Our results reveal co-occurrence of Listeria spp. and diverse members of the core community throughout processing.
The increase in AMC after the first processing steps indicate cross contamination events of microorganisms from the environment as well as the increase of psychrotrophic microbiota associated with the processed meat. Especially, at the leg cutting station AMC raised to critical limits (DGHM, 2014; >6.7 log CFU). The meat and environmental samples harbored higher Pseudomonadaceae in comparison to Enterobacteriaceae counts, except for the delivered carcass samples, after leg cutting and on shoes and on the wall of the cooling chamber (Supplementary Figure S2). However, species richness was lower at leg and loin cutting samples compared to samples taken at the beginning of the processing line (cooling chamber). In congruence with the shift in beta diversity, this indicates that the transfer of bacteria rapidly changes the overall composition of the microbiota between these steps to a community dominated by a few bacteria that are endemic in the facility. These results are in line with previous findings and together demonstrate that the facility-specific microbiome greatly affects the composition of the microbial community found on the final product (Bokulich and Mills, 2013; de Filippis et al., 2013; Hultman et al., 2015; Einson et al., 2018; Quijada et al., 2018; Zwirzitz et al., 2020). Among all samples, the primary phyla of surface samples were Firmicutes and Proteobacteria. In contrast, Rodríguez-López et al. (2020) identified Actinobacteriota and Firmicutes as the most dominant phyla in meat industry samples (Rodríguez-López et al., 2020).
The core in-house microbiota consisted mainly of the genera Acinetobacter, Pseudomonas, Psychrobacter (Proteobacteria), Bacillus (Firmicutes), and Chryseobacterium (Bacteroidota) which have been commonly isolated from other FPEs before (Stellato et al., 2015, 2016; Hascoët et al., 2019). Since all these genera are also highly abundant and include species that are frequently described as spoilage organisms, the widespread establishment of these bacteria throughout the facility is concerning (Odeyemi et al., 2020). In another study, bacterial communities from biofilms in four FPEs were characterized by 16S rRNA gene sequencing: Proteobacteria, Firmicutes, Actinobacteriota, and Bacteroidota represented over 94% of the operational taxonomic units, and Pseudomonas and Acinetobacter were the most dominant genera (93.47%; Caraballo Guzmán et al., 2020). Pseudomonas and Acinetobacter were the most frequently isolated genera surviving on conveyor belts after cleaning and disinfection in meat processing plants (Fagerlund et al., 2017). In our dataset, Pseudomonas was besides Anaerobacillus the most abundant genus throughout meat cutting and was involved in cross-contamination events from the environment. In detail, P. fragi, P. psychrophila, and P. fluorescens could be assigned to species level and were strongly linked to the FPE and meat cuts. This is in line with the findings from Stellato et al. (2017), who determined the most dominant Pseudomonas species in both meat and dairy processing environments (Stellato et al., 2017).
Listeria testing of meat, environmental, and personnel associated samples revealed a widely disseminated L. monocytogenes contamination (50% of samples Listeria spp. positive, 6% L. monocytogenes positive) throughout processing. Similar prevalence levels of listeria were observed in other processing environments as well (Muhterem-Uyar et al., 2015). Listeria was absent from carcass samples, hooks and wall in the cooling chamber, but was present due to cross-contamination events during meat cutting and packaging. The most prevalent L. monocytogenes serotype was 1/2a, 3a and was highly present in the FPE (Stessl et al., 2020). The PFGE typing evidenced 14 heterogenous L. monocytogenes profiles, with PFGE profile MA3-17 as most dominant. In future studies, listeria loads should be repeatedly recorded in order to get quantitative data and insights into the stability of the listeria population. To mitigate listeria contamination, operators could determine listeria levels prior to the supply to meat processing plants, using pooled surface samples per carcass and production environment. Carcasses from suppliers that more frequently contain low levels of listeria should not enter the processing area of low-processed edible meat products. In processing plants, where both heated and non-heated meat products are processed, environmental sampling for listeria should be intensified, with a focus on crossing points.
Pulsed-field gel electrophoresis MA3-17 contributed to the resilient microbiota of the facility environment and was related to environmental persistence as shoes after passing the hygiene lock were already positively tested. The hooks of the leg indicated inefficient sanitation due to the presence of up to four different PFGE-profiles and no L. monocytogenes negative sample. Saw and conveyor belts contained heterogenous L. monocytogenes PFGE-types. Furthermore, packaging material was tested positive for listeria highlighting the need of treating the packaging materials as a potential source of cross-contamination (Di Ciccio et al., 2020). Other studies compared the microbiome and the presence of L. monocytogenes in different FPEs and highlighted meat facilities as a common source of L. monocytogenes (Rodríguez-López et al., 2019). A wide variety of phylogenetic taxa co-occurred with L. monocytogenes (Psychromonas, Shewanella, Yersinia, and lactic acid bacteria). The authors concluded that L. monocytogenes is capable to co-exist with different bacteria in different ecological niches. Tan et al. (2019) observed L. monocytogenes persistence in the facility environment of an apple processing plant which was correlating with reduced bacterial diversity (Pseudomonas predominant) in comparison to other plants (Tan et al., 2019).
In general, the presence of listeria had a significant impact on the microbial community structure, affecting multiple taxa across the phylogenetic tree. Future studies are necessary to elucidate whether the presence of listeria drives these shifts in the microbial community, or rather the composition of the pre-existing community fosters suitable conditions for listeria to thrive. However, the ability of L. monocytogenes to survive and persist in biofilms of other bacteria has been proposed by others before (Fagerlund et al., 2017; Heir et al., 2018). Furthermore, Puga et al. (2018) have shown that L. monocytogenes is able to colonize biofilms formed by P. fluorescens in co-culture experiments leading to higher bacterial cell densities in vitro (Puga et al., 2018). Our study confirms that the same colonization progression occurs in the environment. We propose to perform similar experiments with the other bacteria that had positive correlations with listeria in this study. Especially, Acinetobacter and Janthinobacterium, another bacterium that had significantly higher abundances when listeria was present, are known to form biofilms and would be prime prospects to test more complex ecological interactions in controlled experiments. Based on our data, we hypothesize that listeria persists in multispecies biofilms formed by a consortium of Pseudomonas, Acinetobacter, Janthinobacterium, and other species in FPE.
Conclusion
Understanding how individual microbial community members of various FPE interact with each other will give us deeper insights into improved and targeted disinfection strategies. Diverse correlations from ASVs belonging to the same genus support that these interactions are highly specific and that a high taxonomic resolution is necessary when performing metagenomic analysis. Overall, our study suggests that interactions and symbiosis of microorganisms in addition to inherent genetic and environmental factors contribute to listeria persistence in FPE. Metagenomic and metatranscriptomic analysis as well as in vitro experiments are needed to gain more knowledge about the exact metabolic interactions and co-dependence of certain species and strains during biofilm formation and development in regard to L. monocytogenes prevalence.
Data Availability Statement
The datasets presented in this study can be found in online repositories. The names of the repository/repositories and accession number(s) can be found at: https://www.ebi.ac.uk/ena, PRJEB38260.
Author Contributions
BZ, SW, MW, and ES conceived and designed the study. Sampling was performed by BZ, SW, and BS. BZ, ST, MD, and BS performed the experiments. BZ, ED, and NQ developed bioinformatics pipelines. Data analysis and statistics were performed by BZ. BZ and BS wrote the manuscript. All authors contributed to the article and approved the submitted version.
Funding
The competence center FFoQSI is funded by the Austrian ministries BMVIT, BMDW, and the Austrian provinces Niederoesterreich, Upper Austria and Vienna within the scope of COMET – Competence Centers for Excellent Technologies. The programme COMET is handled by the Austrian Research Promotion Agency FFG.
Conflict of Interest
The authors declare that the research was conducted in the absence of any commercial or financial relationships that could be construed as a potential conflict of interest.
Supplementary Material
The Supplementary Material for this article can be found online at: https://www.frontiersin.org/articles/10.3389/fmicb.2021.632935/full#supplementary-material
Footnotes
References
Allam, M., Tau, N., Smouse, S. L., Mtshali, P. S., Mnyameni, F., Khumalo, Z. T. H., et al. (2018). Whole-genome sequences of Listeria monocytogenes sequence type 6 isolates associated with a large foodborne outbreak in South Africa, 2017 to 2018. Genome Announc. 6:e00538–18. doi: 10.1128/genomeA.00538-18
Alvarez-Ordóñez, A., Coughlan, L. M., Briandet, R., and Cotter, P. D. (2019). Biofilms in food processing environments: challenges and opportunities. Annu. Rev. Food Sci. Technol. 10, 173–195. doi: 10.1146/annurev-food-032818-121805
Andersen, K. S., Kirkegaard, R. H., Karst, S. M., and Albertsen, M. (2018). “Ampvis2: An R Package to Analyse and Visualise 16S RRNA Amplicon Data.” BioRxiv [Preprint]. 299537. doi: 10.1101/299537
Bokulich, N. A., and Mills, D. A. (2013). Improved selection of internal transcribed spacer-specific primers enables quantitative, ultra-high-throughput profiling of fungal communities. Appl. Environ. Microbiol. 79, 2519–2526. doi: 10.1128/AEM.03870-12
Bubert, A., Hein, I., Rauch, M., Lehner, A., Yoon, B., Goebel, W., et al. (1999). Detection and differentiation of Listeria Spp. by a single reaction based on multiplex PCR. Appl. Environ. Microbiol. 65, 4688–4692. doi: 10.1128/AEM.65.10.4688-4692.1999
Buchanan, R. L., Gorris, L. G. M., Hayman, M. M., Jackson, T. C., and Whiting, R. C. (2017). A review of Listeria monocytogenes: an update on outbreaks, virulence, dose-response, ecology, and risk assessments. Food Control 75, 1–13. doi: 10.1016/j.foodcont.2016.12.016
Callahan, B. J., McMurdie, P. J., Rosen, M. J., Han, A. W., Johnson, A. J. A., and Holmes, S. P. (2016). DADA2: high-resolution sample inference from illumina amplicon data. Nat. Methods 13, 581–583. doi: 10.1038/nmeth.3869
Callahan, B. J., Wong, J., Heiner, C., Oh, S., Theriot, C. M., Gulati, A. S., et al. (2019). High-throughput amplicon sequencing of the full-length 16S RRNA gene with single-nucleotide resolution. Nucleic Acids Res. 47:e103. doi: 10.1093/nar/gkz569
Cao, Y., Fanning, S., Proos, S., Jordan, K., and Srikumar, S. (2017). A review on the applications of next generation sequencing technologies as applied to food-related microbiome studies. Front. Microbiol. 8:1829. doi: 10.3389/fmicb.2017.01829
Caraballo Guzmán, A., González Hurtado, M. I., Cuesta-Astroz, Y., and Torres, G. (2020). Metagenomic characterization of bacterial biofilm in four food processing plants in Colombia. Braz. J. Microbiol. 51, 1259–1267. doi: 10.1007/s42770-020-00260-x
Center for Disease Control and Prevention (2020). Outbreak of listeria infections linked to deli meats. Available at: https://www.cdc.gov/listeria/outbreaks/delimeat-10-20/index.html (Accessed January 4, 2021).
Chao, A. (1984). Nonparametric estimation of the number of classes in a population. Scand. J. Stat. 11, 265–270. doi: 10.2307/4615964
Churchill, K. J., Sargeant, J. M., Farber, J. M., and O’Connor, A. M. (2019). Prevalence of Listeria monocytogenes in select ready-to-eat foods-deli meat, soft cheese, and packaged salad: a systematic review and meta-analysis. J. Food Prot. 82, 344–357. doi: 10.4315/0362-028X.JFP-18-158
da Silva Fernandes, M., Kabuki, D. Y., and Kuaye, A. Y. (2015). Behavior of Listeria monocytogenes in a multi-species biofilm with Enterococcus Faecalis and Enterococcus Faecium and control through sanitation procedures. Int. J. Food Microbiol. 200, 5–12. doi: 10.1016/j.ijfoodmicro.2015.01.003
Davis, N. M., Proctor, D. M., Holmes, S. P., Relman, D. A., and Callahan, B. J. (2018). Simple statistical identification and removal of contaminant sequences in marker-gene and metagenomics data. Microbiome 6:226. doi: 10.1186/s40168-018-0605-2.
De Filippis, F., La Storia, A., Villani, F., and Ercolini, D. (2013). Exploring the sources of bacterial spoilers in beefsteaks by culture-independent high-throughput sequencing. PLoS One 8:e70222. doi: 10.1371/journal.pone.0070222
DGHM (2014). “Mikrobiologische Richt – Und Warnwerte Zur Beurteilung von Lebensmitteln.” 2014. Available at: http://www.dghm-richt-warnwerte.de (Accessed January 28, 2021).
Di Ciccio, P., Rubiola, S., Grassi, M. A., Civera, T., Abbate, F., and Chiesa, F. (2020). Fate of Listeria monocytogenes in the presence of resident cheese microbiota on common packaging materials. Front. Microbiol. 11:830. doi: 10.3389/fmicb.2020.00830
Doumith, M., Buchrieser, C., Glaser, P., Jacquet, C., and Martin, P. (2004). Differentiation of the major Listeria monocytogenes serovars by multiplex PCR. J. Clin. Microbiol. 42, 3819–3822. doi: 10.1128/JCM.42.8.3819-3822.2004
Dzieciol, M., Schornsteiner, E., Muhterem-Uyar, M., Stessl, B., Wagner, M., and Schmitz-Esser, S. (2016). Bacterial diversity of floor drain biofilms and drain waters in a Listeria monocytogenes contaminated food processing environment. Int. J. Food Microbiol. 223, 33–40. doi: 10.1016/j.ijfoodmicro.2016.02.004
EFSA Panel on Biological Hazards (2019). Whole genome sequencing and metagenomics for outbreak investigation, source attribution and risk assessment of food-borne microorganisms. EFSA J. 17:e05898. doi: 10.2903/j.efsa.2019.5898
Einson, J. E., Rani, A., You, X., Rodriguez, A. A., Randell, C. L., Barnaba, T., et al. (2018). A vegetable fermentation facility hosts distinct microbiomes reflecting the production environment. Appl. Environ. Microbiol. 84:e01680–18. doi: 10.1128/AEM.01680-18
European Centre for Disease Prevention and Control, European food safety authority (2019). Multi-country outbreak of Listeria monocytogenes sequence type 6 infections linked to ready-to-eat meat products Available at: https://www.ecdc.europa.eu/sites/default/files/documents/Listeria-rapid-outbreak-assessment-NL-BE.pdf (Accessed November 25, 2019).
European Commission (2020). “The rapid alert system for food and feed (RASFF).” Available at: https://ec.europa.eu/food/safety/rasff_en (Accessed January 28, 2021).
European Food Safety Authority and European Centre for Disease Prevention and Control (EFSA and ECDC) (2018). The European union summary report on trends and sources of zoonoses, zoonotic agents and food-borne outbreaks in 2017. EFSA J. 16:e05500. doi: 10.2903/j.efsa.2018.5500
Fagerlund, A., Møretrø, T., Heir, E., Briandet, R., and Langsrud, S. (2017). Cleaning and disinfection of biofilms composed of Listeria monocytogenes. Appl. Environ. Microbiol. 83:e01046–17. doi: 10.1128/AEM.01046-17
Fegan, N., and Jenson, I. (2018). The role of meat in foodborne disease: is there a coming revolution in risk assessment and management? Meat Sci. 144, 22–29. doi: 10.1016/j.meatsci.2018.04.018
Galié, S., García-Gutiérrez, C., Miguélez, E. M., Villar, C. J., and Lombó, F. (2018). Biofilms in the food industry: health aspects and control methods. Front. Microbiol. 9:898. doi: 10.3389/fmicb.2018.00898
Halbedel, S., Prager, R., Fuchs, S., Trost, E., Werner, G., and Flieger, A. (2018). Whole-genome sequencing of recent Listeria monocytogenes isolates from Germany reveals population structure and disease clusters. J. Clin. Microbiol. 56:e00119–18. doi: 10.1128/JCM.00119-18
Harrand, A. S., Jagadeesan, B., Baert, L., Wiedmann, M., and Orsi, R. H. (2020). Evolution of Listeria monocytogenes in a food processing plant involves limited single-nucleotide substitutions but considerable diversification by gain and loss of prophages. Appl. Environ. Microbiol. 86:e02493–19. doi: 10.1128/AEM.02493-19
Hascoët, A. S., Ripolles-Avila, C., Guerrero-Navarro, A. E., and Rodríguez-Jerez, J. J. (2019). Microbial ecology evaluation of an Iberian pig processing plant through implementing Sch sensors and the influence of the resident microbiota on Listeria monocytogenes. Appl. Sci. 9:461. doi: 10.3390/app9214611
Heir, E., Møretrø, T., Simensen, A., and Langsrud, S. (2018). Listeria monocytogenes strains show large variations in competitive growth in mixed culture biofilms and suspensions with bacteria from food processing environments. Int. J. Food Microbiol. 275, 46–55. doi: 10.1016/j.ijfoodmicro.2018.03.026
Hultman, J., Rahkila, R., Ali, J., Rousu, J., and Björkroth, J. K. (2015). Meat processing plant microbiome and contamination patterns of cold-tolerant bacteria causing food safety and spoilage risks in the manufacture of vacuum-packaged cooked sausages. Appl. Environ. Microbiol. 81, 7088–7097. doi: 10.1128/AEM.02228-15
Jackson, B. R., Tarr, C., Strain, E., Jackson, K. A., Conrad, A., Carleton, H., et al. (2016). Implementation of nationwide real-time whole-genome sequencing to enhance listeriosis outbreak detection and investigation. Clin. Infect. Dis. 63, 380–386. doi: 10.1093/cid/ciw242
Kaptchouang Tchatchouang, C. D., Fri, J., De Santi, M., Brandi, G., Schiavano, G. F., Amagliani, G., et al. (2020). Listeriosis outbreak in South Africa: a comparative analysis with previously reported cases worldwide. Microorganisms 8:135. doi: 10.3390/microorganisms8010135
Kaszoni-Rückerl, I., Mustedanagic, A., Muri-Klinger, S., Brugger, K., Wagner, K., Wagner, M., et al. (2020). Predominance of distinct Listeria Innocua and Listeria monocytogenes in recurrent contamination events at dairy processing facilities. Microorganisms 8:234. doi: 10.3390/microorganisms8020234
Kimura, M. (1980). A simple method for estimating evolutionary rates of base substitutions through comparative studies of nucleotide sequences. J. Mol. Evol. 16, 111–120. doi: 10.1007/BF01731581
Kumar, S., Stecher, G., Li, M., Knyaz, C., and Tamura, K. (2018). MEGA X: molecular evolutionary genetics analysis across computing platforms. Mol. Biol. Evol. 35, 1547–1549. doi: 10.1093/molbev/msy096
Lahti, L., and Shetty, S. (2017). “Tools for microbiome analysis in R.” Available at: http://microbiome.github.com/microbiome (Accessed January 28, 2021).
Leclercq, A., Chenal-Francisque, F., Dieye, H., Cantinelli, T., Drali, R., Brisse, S., et al. (2011). Characterization of the novel Listeria monocytogenes PCR serogrouping profile IVb-V1. Int. J. Food Microbiol. 147, 74–77. doi: 10.1016/j.ijfoodmicro.2011.03.010
Love, M. I., Huber, W., and Anders, S. (2014). Moderated estimation of fold change and dispersion for RNA-seq data with DESeq2. Genome Biol. 15:550. doi: 10.1186/s13059-014-0550-8
McMurdie, P. J., and Holmes, S. P. (2013). Phyloseq: an R package for reproducible interactive analysis and graphics of microbiome census data. PLoS One 8:e61217. doi: 10.1371/journal.pone.0061217
Mendez, E., Walker, D. K., Vipham, J., and Trinetta, V. (2020). The use of a CDC biofilm reactor to grow multi-strain Listeria monocytogenes biofilm. Food Microbiol. 92:103592. doi: 10.1016/j.fm.2020.103592
Muhterem-Uyar, M., Dalmasso, M., Bolocan, A. S., Hernandez, M., Kapetanakou, A. E., Kuchta, T., et al. (2015). Environmental sampling for Listeria monocytogenes control in food processing facilities reveals three contamination scenarios. Food Control 51, 94–107. doi: 10.1016/j.foodcont.2014.10.042
Nyarko, E., Kniel, K. E., Zhou, B., Millner, P. D., Luo, Y., Handy, E. T., et al. (2018). Listeria monocytogenes persistence and transfer to cantaloupes in the packing environment is affected by surface type and cleanliness. Food Control 85, 177–185. doi: 10.1016/j.foodcont.2017.09.033
Odeyemi, O. A., Alegbeleye, O. O., Strateva, M., and Stratev, D. (2020). Understanding spoilage microbial community and spoilage mechanisms in foods of animal origin. Compr. Rev. Food Sci. Food Saf. 19, 311–331. doi: 10.1111/1541-4337.12526
Oksanen, J., Blanchet, F. G., Kindt, R., Legendre, P., O’Hara, R. B., Simpson, G. L., et al. (2011). vegan: Community Ecology Package. R package version 2.5–6.
Pietzka, A., Allerberger, F., Murer, A., Lennkh, A., Stüger, A., Cabal Rosel, A., et al. (2019). Whole genome sequencing based surveillance of L. monocytogenes for early detection and investigations of listeriosis outbreaks. Front. Public Health 7:139. doi: 10.3389/fpubh.2019.00139
Pruesse, E., Peplies, J., and Glöckner, F. O. (2012). SINA: accurate high-throughput multiple sequence alignment of ribosomal RNA genes. Bioinformatics 28, 1823–1829. doi: 10.1093/bioinformatics/bts252
Puga, C. H., Dahdouh, E., SanJose, C., and Orgaz, B. (2018). Listeria monocytogenes colonizes Pseudomonas fluorescens biofilms and induces matrix over-production. Front. Microbiol. 9:1706. doi: 10.3389/fmicb.2018.01706
Quast, C., Pruesse, E., Yilmaz, P., Gerken, J., Schweer, T., Yarza, P., et al. (2013). The SILVA ribosomal RNA gene database project: improved data processing and web-based tools. Nucleic Acids Res. 41, 590–596. doi: 10.1093/nar/gks1219
Quijada, N. M., Mann, E., Wagner, M., Rodríguez-Lázaro, D., Hernández, M., and Schmitz-Esser, S. (2018). Autochthonous facility-specific microbiota dominates washed-rind Austrian hard cheese surfaces and its production environment. Int. J. Food Microbiol. 267, 54–61. doi: 10.1016/j.ijfoodmicro.2017.12.025
R Core Team (2019). R: A Language and Environment for Statistical Computing. Vienna, Austria: R Foundation for Statistical Computing. Available at: https://www.r-project.org (Accessed January 28, 2021).
Ripolles-Avila, C., Hascoët, A. S., Martínez-Suárez, J. V., Capita, R., and Rodríguez-Jerez, J. J. (2019). Evaluation of the microbiological contamination of food processing environments through implementing surface sensors in an Iberian pork processing plant: an approach towards the control of Listeria monocytogenes. Food Control 99, 40–47. doi: 10.1016/j.foodcont.2018.12.013
Rodríguez-López, P., Bernárdez, M., Rodríguez-Herrera, J. J., Comesaña, A. S., and Cabo, M. L. (2019). Identification and metagenetic characterisation of Listeria monocytogenes-harbouring communities present in food-related industrial environments. Food Control 95, 6–17. doi: 10.1016/j.foodcont.2018.07.023
Rodríguez-López, P., Rodríguez-Herrera, J. J., and Cabo, M. L. (2020). Tracking bacteriome variation over time in Listeria monocytogenes-positive foci in food industry. Int. J. Food Microbiol. 315:108439. doi: 10.1016/j.ijfoodmicro.2019.108439
Rodríguez-López, P., Rodríguez-Herrera, J. J., Vázquez-Sánchez, D., and Cabo, M. L. (2018). Current knowledge on Listeria monocytogenes biofilms in food-related environments: incidence, resistance to biocides ecology and biocontrol. Foods 7:85. doi: 10.3390/foods7060085
Rodríguez-Melcón, C., Riesco-Peláez, F., García-Fernández, C., Alonso-Calleja, C., and Capita, R. (2019). Susceptibility of Listeria monocytogenes planktonic cultures and biofilms to sodium hypochlorite and benzalkonium chloride. Food Microbiol. 82, 533–540. doi: 10.1016/j.fm.2019.03.020
Shannon, C. E. (1948). A mathematical theory of communication. Bell Syst. Tech. J. 27, 379–423. doi: 10.1002/j.1538-7305.1948.tb01338.x
Spor, A., Camargo, A. R. O., Bru, D., Gaba, S., Garmyn, D., Gal, L., et al. (2020). Habitat disturbances modulate the barrier effect of resident soil microbiota on Listeria monocytogenes invasion success. Front. Microbiol. 11:927. doi: 10.3389/fmicb.2020.00927
Stellato, G., De Filippis, F., La Storia, A., and Ercolini, D. (2015). Coexistence of lactic acid bacteria and potential spoilage microbiota in a dairy processing environment. Appl. Environ. Microbiol. 81, 7893–7904. doi: 10.1128/AEM.02294-15
Stellato, G., La Storia, A., De Filippis, F., Borriello, G., Villani, F., and Ercolini, D. (2016). Overlap of spoilage-associated microbiota between meat and the meat processing environment in small-scale and large-scale retail distributions. Appl. Environ. Microbiol. 82, 4045–4054. doi: 10.1128/AEM.00793-16
Stellato, G., Utter, D. R., Voorhis, A., De Angelis, M., Eren, A. M., and Ercolini, D. (2017). A few Pseudomonas oligotypes dominate in the meat and dairy processing environment. Front. Microbiol. 8:264. doi: 10.3389/fmicb.2017.00264
Stessl, B., Szakmary-Brändle, K., Vorberg, U., Schoder, D., and Wagner, M. (2020). Temporal analysis of the Listeria monocytogenes population structure in floor drains during reconstruction and expansion of a meat processing plant. Int. J. Food Microbiol. 314:108360. doi: 10.1016/j.ijfoodmicro.2019.108360
Tan, X., Chung, T., Chen, Y., Macarisin, D., LaBorde, L., and Kovac, J. (2019). The occurrence of Listeria monocytogenes is associated with built environment microbiota in three tree fruit processing facilities. Microbiome 7:115. doi: 10.1186/s40168-019-0726-2
Teixeira, P., Kolomeytseva, M., Silva, J., Castro, S. M. A., and Saraiva, J. A. (2014). High hydrostatic pressure applied to ready-to-eat meat products focus on Listeria monocytogenes inactivation. Front. Food Sci. Technol. 1, 14–23.
Van Reckem, E., De Vuyst, L., Weckx, S., and Leroy, F. (2020). Next-generation sequencing to enhance the taxonomic resolution of the microbiological analysis of meat and meat-derived products. Curr. Opin. Food Sci. 37, 58–65. doi: 10.1016/j.cofs.2020.09.004
Wagner, E. M., Pracser, N., Thalguter, S., Fischel, K., Rammer, N., Pospíšilová, L., et al. (2020). Identification of biofilm hotspots in a meat processing environment: detection of spoilage bacteria in multi-species biofilms. Int. J. Food Microbiol. 328:108668. doi: 10.1016/j.ijfoodmicro.2020.108668
Walsh, P. S., Metzger, D. A., and Higushi, R. (2013). Chelex 100 as a medium for simple extraction of DNA for PCR-based typing from forensic material. BioTechniques 10, 506–513. doi: 10.2144/000114018
Keywords: Listeria monocytogenes, meat processing, microbiome, spoilage, microbial communities
Citation: Zwirzitz B, Wetzels SU, Dixon ED, Fleischmann S, Selberherr E, Thalguter S, Quijada NM, Dzieciol M, Wagner M and Stessl B (2021) Co-Occurrence of Listeria spp. and Spoilage Associated Microbiota During Meat Processing Due to Cross-Contamination Events. Front. Microbiol. 12:632935. doi: 10.3389/fmicb.2021.632935
Edited by:
Marta López, Consejo Superior de Investigaciones Científicas (CSIC), SpainReviewed by:
Antonio Valero, University of Cordoba, SpainAnca Ioana Nicolau, Dunarea de Jos University, Romania
Copyright © 2021 Zwirzitz, Wetzels, Dixon, Fleischmann, Selberherr, Thalguter, Quijada, Dzieciol, Wagner and Stessl. This is an open-access article distributed under the terms of the Creative Commons Attribution License (CC BY). The use, distribution or reproduction in other forums is permitted, provided the original author(s) and the copyright owner(s) are credited and that the original publication in this journal is cited, in accordance with accepted academic practice. No use, distribution or reproduction is permitted which does not comply with these terms.
*Correspondence: Benjamin Zwirzitz, YmVuamFtaW4uendpcnppdHpAdmV0bWVkdW5pLmFjLmF0