- 1Institute of Animal Sciences, Chinese Academy of Agricultural Sciences, Beijing, China
- 2College of Food Science, Southwest University, Chongqing, China
- 3College of Agriculture and Animal Husbandry, Qinghai University, Xining, China
Current information on the differences between rumen bacteria and metabolites of the grass-fed and grain-fed yaks is limited. Understanding the composition and alterations of rumen microbial metabolites is important to clarify its potential role in grass-fed and grain-fed systems. The aim of this research was to explore the influence of different production systems on the functional attributes and metabolites in the rumen microbiota of yak using genomics (Illumina MiSeq sequencing of the 16S rRNA gene) and untargeted metabolomics (UHPLC-QTOF-MS). Rumen samples were obtained from grass-fed (C), grain-fed for 3-month (G3), and grain-fed for 6-month yaks (G6). Results showed that the grain-fed yaks presented a lower rumen bacterial richness and diversity when compared to grass-fed yaks. Bacteroidota, Firmicutes, and Fibrobacterota were the main bacterial phyla. At the phylum and genus level, the grass-fed yaks significantly increased the abundance of Fibrobacterota and Fibrobacter (p < 0.05), respectively. The metabolomics analysis revealed that the metabolite profiles differed among the three groups. Compared with the grass-fed group, grain feeding significantly increased azelaic acid, hypoxanthine, uridine, L-phenylalanine, anserine, and decreased alpha-linolenic acid, adenine. Pathway enrichment analysis showed significant differences in metabolic pathways among all comparison groups, but the glycerophospholipid metabolism and alpha-linolenic acid metabolism pathway were common key metabolic pathways. This study showed that the combined analysis of microbiota and metabolites could distinguish different production systems and the fattening time of yaks, providing novel insights for us to understand the function of the rumen bacteria.
Introduction
The yak (Bos grunniens) is the most valuable herbivorous livestock in the Qinghai-Tibetan Plateau, providing necessities like milk, meat, and transportation for local people (An et al., 2005). However, yaks are mainly grazing, and their living environment is challenged by extreme environments such as low temperature, hypoxia, high altitude, and long-term shortage of grain feed, which limits the quality and supply of diet, especially in winter (Ren et al., 2020). To ensure the sustainability of grassland utilization and the growth performance of yak, it is necessary to use management strategies, for instance, supplementary feeding and indoor feeding. In the course of evolution, grass-fed yaks have developed unique genetic traits that determine their ability to adapt to the environment (Qiu et al., 2012).
The rumen is a stable and incredibly complicated micro-ecosystem with a complex microbial community, including countless bacteria, archaea, fungi, and protozoa (Russell and Rychlik, 2001; Qiu et al., 2020). It is well known that the composition of the rumen bacteria is affected by feed diet or feeding methods, which is also the main reason for different feed conversion efficiency for ruminants (Henderson et al., 2015; Zhang et al., 2017; Zhou J. W. et al., 2017). Although previous studies have shown that grain-based feeding cattle had lower bacterial diversity compared with pasture-rich diets (Fernando et al., 2010; Plaizier et al., 2017; Liu et al., 2019), little is known about the interaction of microbial flora and feeding time, in the case of different feed types.
The application of high-throughput sequencing (via 16S rRNA sequencing technology) and metabolomics to analyze the changes of rumen microbiota under different diets is worthy of in-depth exploration. Zhou Z. et al. (2017) investigated the microbial composition of the rumen through high-throughput sequencing. Liu et al. (2019) used high-throughput sequencing and metabolomics to investigate the impacts of different feeding methods on the microbiota and metabolites in the rumen, but the rumen adaptation period was only 15 days. However, the stabilization of the bacterial community in the rumen takes at least 3 months (Qiu et al., 2019). Currently, there is limited information on the differences in microbial diversity and metabolic mechanism between grass-fed and grain-fed yak rumen, after a stable period to adapt to dietary changes.
Therefore, in this study, we combined ruminal microbiome and microbial metabolome to compare the bacterial community diversity and metabolite composition in the rumen of yak fed with grass, grain for 3 months, and grain for 6 months, and determine the potential biomarkers in the rumen fluid under different production systems.
Materials and Methods
Animals, Diets, and Trial Design
All animal-specific procedures were approved and authorized by the Animal Ethics Committee of the Chinese Academy of Agricultural Sciences. In this study, the animals were born, raised, and maintained in the Xiahua Beef Cattle Specialized Cooperative Base in Haiyan County, Haibei Tibetan Autonomous Prefecture, Qinghai Province, China. A total of eighteen healthy male yaks (3 years old, bodyweight 150 ± 10.5 kg) were selected and randomly divided into three groups, all of which came from a mixed pasture dominated by carex qinghaiensis and kobresia pygmaea. Grass-fed yaks continued to be reared for 6 months as a control (C, n = 6) on a natural pasture. Grain-fed yaks were divided into two groups: grain-fed for 3 months (G3, n = 6) and grain-fed for 6 months (G6, n = 6) with 40% concentrate and 60% forage. Among them, the yaks in the G3 group lived on pastures for 3 months before being kept in feedlot pens for 3 months. After being selected from the pasture, the yaks of the G6 group were housed in the feedlot pens. The proportions and chemical compositions of the basal diet is shown in Supplementary Table 1.
Rumen Fluid Sampling
After being weighed (190.7 ± 20.6 kg for the C group, 197.7 ± 17.2 kg for the G3 group, and 228.3 ± 23.1 kg for the G6 group), the yaks were transported to the commercial slaughterhouse. Then, the yaks were stunned by electricity before being slaughtered. Approximately 50 mL of rumen content was collected, and quickly frozen in liquid nitrogen, before processing (sequencing and metabolomics).
DNA Extraction and High-Throughput Sequencing
The DNA was extracted from ruminal fluid samples using the FastDNA SPIN kit (MP Biomedical, Solon, OH, United States) as instructed by the manufacturer. TBS-380 and NanoDrop2000 were used to determine the concentration and purity of extracted DNA, respectively. The quality of the DNA extract was detected through a 1% agarose gel. Covaris M220 (Gene Company Limited, China) was used to fragment the DNA extract to an average size of about 400 bp for the construction of a paired-end library. The following primers were used to amplify the V3–V4 region of the 16S rRNA gene: 338F, 5′-ACTCCTACGGGAGGCAGCAG-3′ and 806R, 5′-GGACTACHVGGGTWTCTAAT-3′. PCR was conducted using an S1000 thermal cycler (Bio-Rad, Hercules, CA, United States). The extracted PCR amplification products were purified and quantified using AxyPrep DNA Gel Extraction Kit (Axygen Biosciences, Union City, CA, United States) and QuantiFluor-ST (Promega, WI, United States). The sequencing library was constructed with the TruSeq DNA Sample Preparation Kit (Bioo Scientific, Austin, TX, United States), which was completed by Majorbio Bio-Pharm Technology Co., Ltd. (Shanghai, China). The purified amplicons were pooled in equimolar and sequenced on a MiSeq Platform (Illumina, San Diego, CA, United States) using sequencing 2 × 300 bp paired end with the MiSeq® Reagent Kit v3 reagent kit at Majorbio Bio-Pharm Technology Co., Ltd. (Shanghai, China).
Bioinformatic Analysis
The paired-end reads were trimmed and merged using trimmomatic version 0.32. After removing low-quality sequences and chimeras, the sequences were clustered into Operational Taxonomic Units (OTUs) with 97% sequence similarity. The classification of each 16S rRNA gene sequence database (Silva version 138) was analyzed with a 70% reliability threshold (Quast et al., 2012). The rarefaction curves and bar graphs used to analyze the abundance of bacteria in all samples were made by the vegan package in R (Hsieh et al., 2016). Alpha diversity indices were performed with Mothur (version v.1.30.2). The results of principal coordinate analysis (PCoA) were performed with GUnifrac and ape packages in R (Lozupone and Knight, 2005; Mao et al., 2016). Venn diagrams illustrating the overlap of OTUs between groups were generated in R (1.6.20) (Chen, 2018). The difference in the abundance of microbial communities between samples obtained by statistical methods was used to assess the significance of the difference using FDR (false discovery rate). The p-value obtained by adjusting the false discovery rate (FDR) by the Benjamini–Hochberg method. p < 0.05 was shown as statistically significant in the relative abundance of microbiota among the three feeding methods.
Metabolomics Analysis
The thawed rumen fluid samples were filtered through four layers of gauze, and then centrifuged at 11,200 × g for 10 min. The supernatant (100 μL) of each sample was mixed with 10 μL of internal standard (3.0 mg/mL, L-2-chlorophenylalanine), and methanol-acetonitrile (300 μL, 2:1, v/v), and then vortexed for 1 min. The LC-MS/MS analyses were carried out with an Agilent 1290 Infinity LC system (Agilent Technologies, Santa Clara, CA, United States) with an Acquity BEH C18 column (100 mm × 2.1 mm i.d., 1.7 μm, Waters, Milford, MA, United States) preheated to 45°C. The mobile phase was composed of solvent A (aqueous 0.1% (v/v) formic acid) and solvent B (acetonitrile) delivered at 0.40 mL/min with the following gradient: 0–1 min, 1% B, 1–5.5 min, 1% B–20% B, 5.5–6 min, 20% B–30% B, 6–8.5 min, 30% B–35% B, 8.5–10.5 min, 35% B–70% B, 10.5–11 min, 70% B–100% B, 11–13 min, 100% B, 13–13.1 min, 100% B–1% B, and 13.1–15 min, 1% B. The injection volume was 3 μL. The LTQ Orbitrap mass spectrometer (XL, Thermo Fisher Scientific, Waltham, MA, United States) was used to obtain sample mass spectrum data using positive ion or negative ion scan mode. The electron spray ionization source conditions were set as follows: sample voltage, 40 V, capillary voltage, 1.0 kV, ion source temperature 120°C, desolvation gas rate and temperature, 900 L/h and 500°C, the mass range, 50–1000 m/z, scan time and interscan delay, 0.15 and 0.02 s, respectively. The normalized collision energy was 6 eV.
Data Analysis
The preprocessing included the integration of peak intensities, normalization and peak alignment, and produced a data matrix composed of retention time (RT), the mass-to-charge ratio (m/z) values, and peak intensity finally. The processed data were input to the SIMCA-P software (V14.0, Umetric, Umeå, Sweden) using principal component analysis (PCA) and orthogonal partial least-squares discriminate analysis (OPLS-DA) to detect metabolic changes. Each metabolite received a value called variable importance for the projection (VIP). Differentially expressed metabolites (DEMs) were screened using Student’s t-test. If p < 0.05 and VIP > 1, the variables were considered to be significantly different in metabolites between the two groups. Enrichment analysis and pathway topology analysis were acquired by using MetaboAnalyst 4.01 and Kyoto Encyclopedia of Genes and Genomes (KEGG).2 The functional correlation between metabolites and rumen bacterial changes was conducted using Spearman’s correlation.
Results
16S rRNA Gene Sequencing Results
A total of 906,639 sequences (307,480 reads from C group, 291,459 reads from G3 group, and 307,700 reads from G6 group) with an average length of 441 bp were obtained through the 16S rRNA gene sequencing. Our study also obtained 5,285 OTUs at a sequence-similarity level of 97%. In Supplementary Figure 1, the Venn figure demonstrated that there were 1,178 common OTUs among the three groups. The rarefaction curves of each sample reached the plateau level (Supplementary Figure 2), indicating that the sequencing depth was sufficient.
Bacterial Diversity Analysis
Alpha diversity indices, including Chao1 and Shannon, are shown in Figures 1A,B. The Chao1 and Shannon indices in the grass-fed group (C) had higher values than the grain-fed group (G3 and G6) (p < 0.05), suggesting that the microbial diversity of the grass-fed group is higher than that of the grain-fed group. The results of beta diversity did show a significant difference among the three groups. PCoA showed the difference of microbiota in the samples of grass-fed, grain-fed for 3 months, and grain-fed for 6 months yak rumen (Figure 1C). The plot demonstrated that the microbial differentiation of the three groups had a clear separation, indicating that the microbial communities had obvious differences.
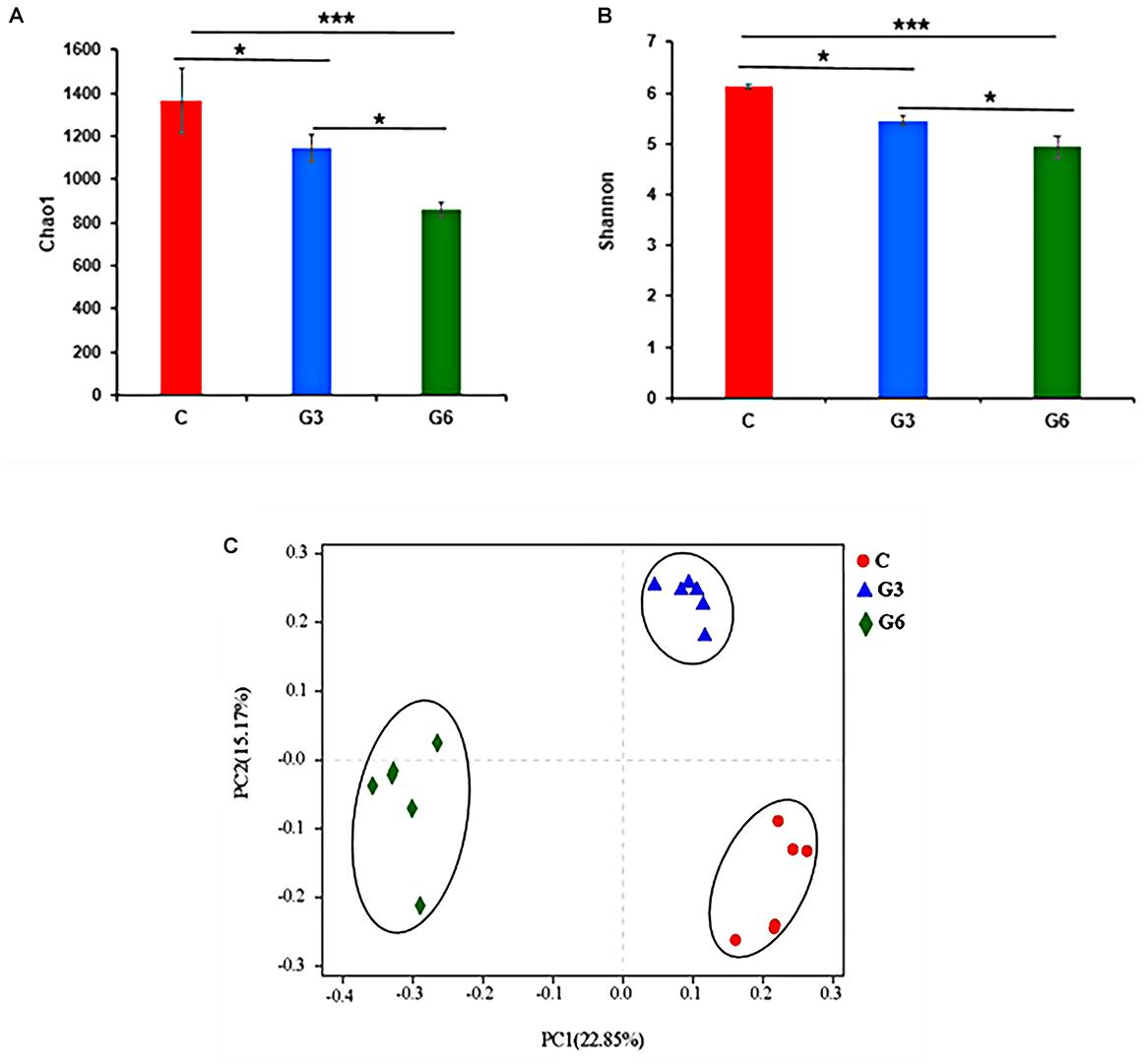
Figure 1. Bacterial diversity. (A,B) Alpha diversity metrics (A) Chao 1 and (B) Shannon for the rumen microbiota of grass-fed (C), grain-fed for 3 months (G3), and grain-fed for 6 months (G6) yaks. (C) The plot of Principal coordinate analysis (PCoA). The closer distance between the sample points means the higher similarity of the bacteria. *p < 0.05; ***p < 0.001.
Rumen Microbial Composition at the Phylum and Genus Levels
Figure 2 shows the differences in relative abundances of the top 10 most abundant microbial species in three groups. The results demonstrated that Bacteroidota, Firmicutes, and Fibrobacterota were the main bacterial phyla (Figure 2A). Bacteroidota and Firmicutes accounted for 53.22 and 21.46% in the C group, and 56.15 and 27.43% in the G3 group, and 62.51 and 23.46% in the G6 groups, respectively. Compared with the C group, Bacteroidota increased (p < 0.05) in the G6 group. In grass-fed yaks, the relative abundance of Fibrobacterota (15.88%) was significantly higher (p < 0.05) than that in grain-fed yaks (G3, 3.05% and G6, 3.60%). However, the relative abundances of the Patescibacteria in the G3 group was higher (p < 0.05) than that in C and G6 groups (Figure 2C). At the genus level, Prevotella, Rikenellaceae_RC9_gut_group, and norank_f_F082 were the main genera in these three groups (Figure 2B). Compared with the G6, the Rikenellaceae_RC9_gut_group of the G3 was significantly reduced (p < 0.05). While the relative abundance of Fibrobacter showed the highest value (p < 0.01) in C group, and norank_f_Bacteroidales_UCG-001 showed the lowest value (p < 0.01) in G6 group (p < 0.05), respectively. No significant differences were found in Prevotella, norank_f_Bacteroidales_BS11_gut_group, norank_f_F082, Treponema, norank_f_Muribaculaceae, Prevotellaceae_UCG-003, and Prevotellaceae_UCG-001 (p > 0.05) among three groups (Figure 2D).
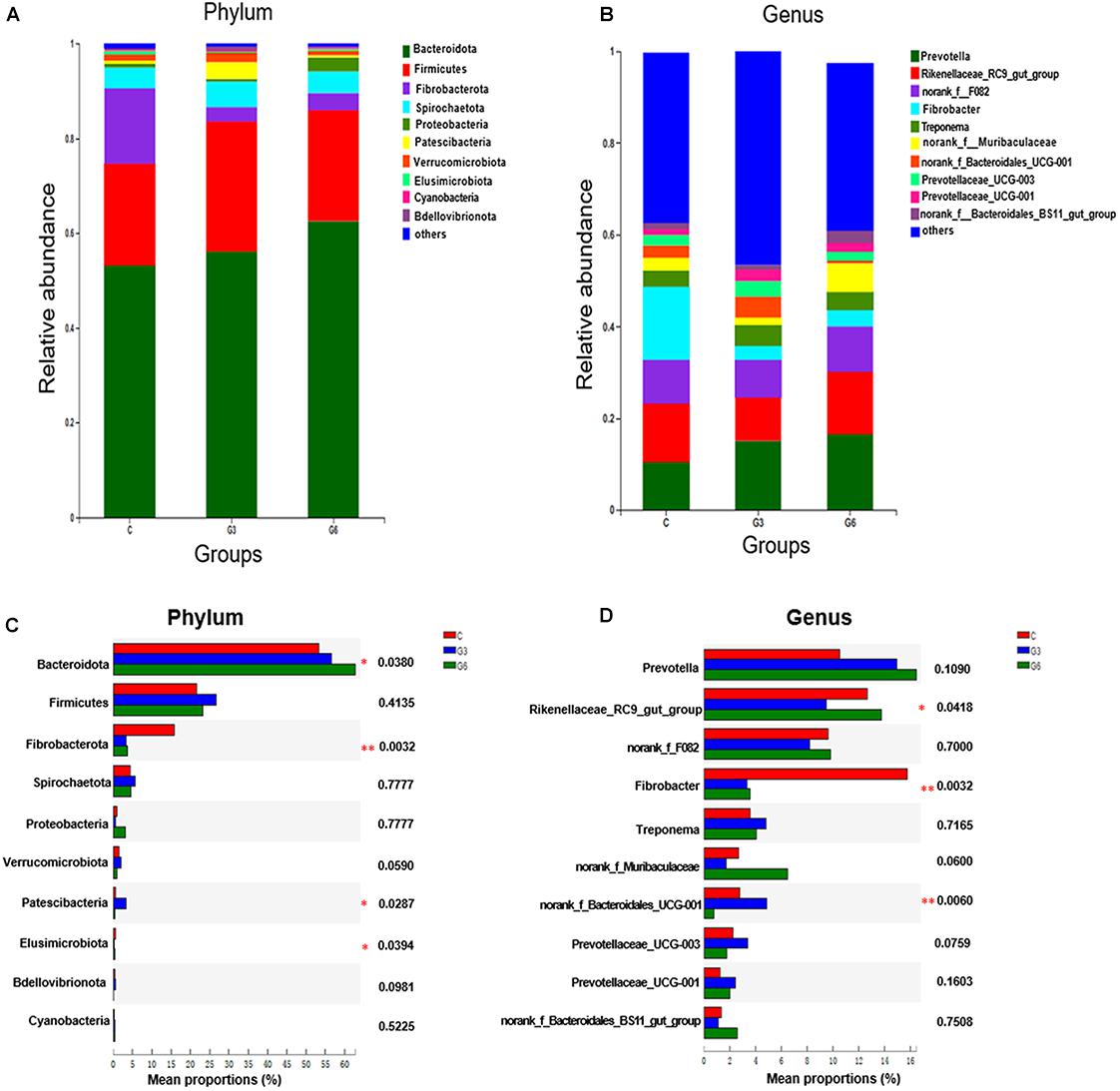
Figure 2. Relative rumen microbiota abundance at (A,C) phylum and (B,D) genus levels in the grass-fed (C), grain-fed for 3 months (G3), and grain-fed for 6 months (G6) yaks experimental groups. *p < 0.05; **p < 0.01.
Metabolomic Profiles of the Rumen Fluid
In this research, the PCA score plot confirmed the validity of the data (Supplementary Figure 3). Figure 3 showed the contribution of different variables to the discriminating ability of each OPLS-DA model. As shown in Figure 3A, the permutation test (C versus G3, R2Y = 0.827, Q2Y = −0.37 < 0, C versus G6, R2Y = 0.795, Q2Y = −0.598 < 0, G3 versus G6, R2Y = 0.895, Q2Y = −0.319 < 0) suggested that the model was adequate for its efficacy (Figures 3A,C,E). Except for 1 rumen fluid sample outside the ellipse in the score plots, the other samples were all inside the 95% Hotelling T2 ellipse (Figures 3B,D,F). All data in the three models in the C versus G3 groups, C versus G6 groups, and G3 versus G6 groups showed significant separation and differentiation, illustrating the effectiveness of the OPLS-DA model in a further screening of different metabolites.
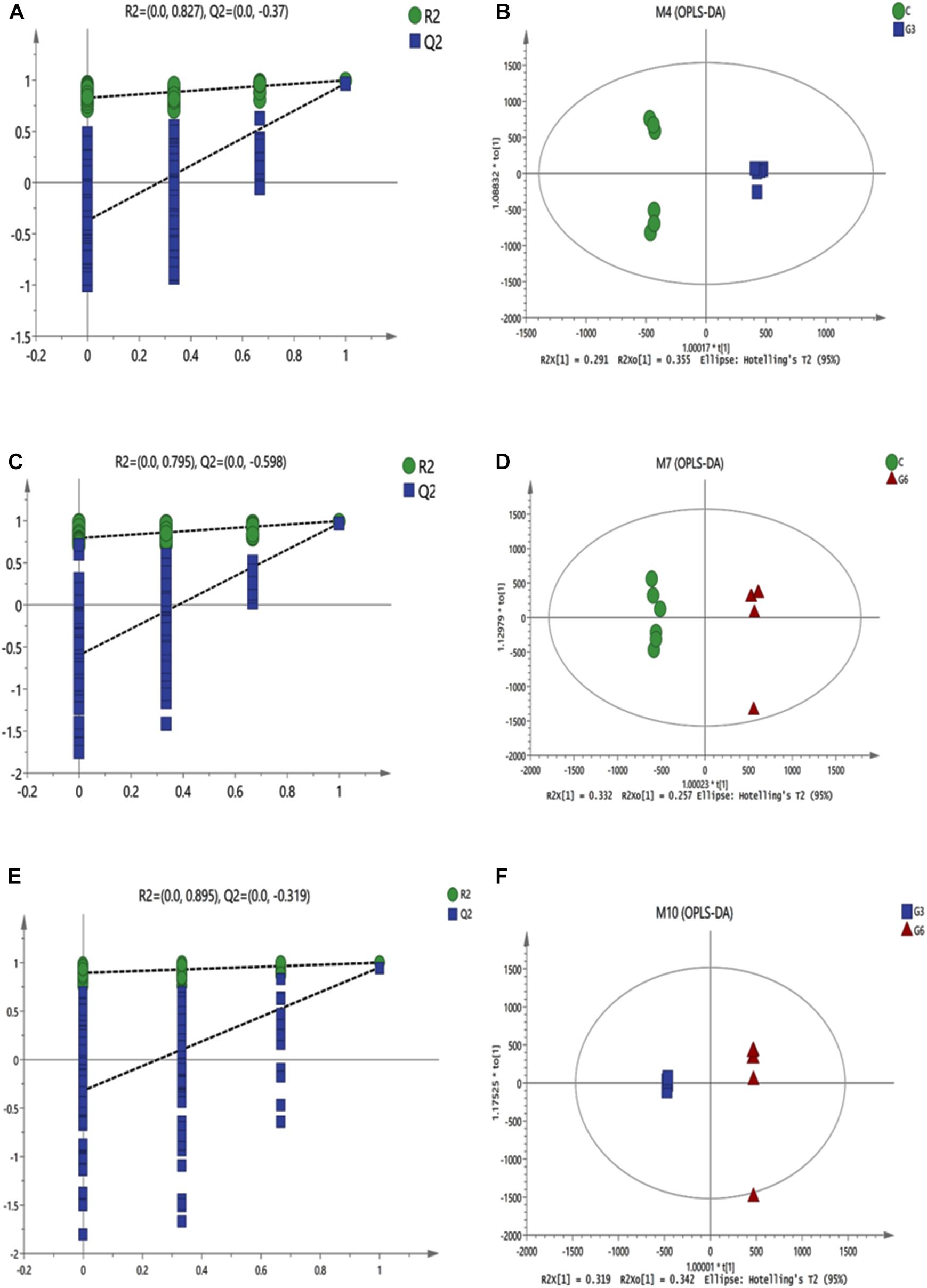
Figure 3. The permutation test (n = 200) of the OPLS-DA model (A,C,E) and OPLS-DA model (B,D,F) were derived from the liquid chromatography/mass spectrometry metabolomics profiles of yak rumen fluid samples from grass-fed (C), grain-fed for 3 months (G3), and grain-fed for 6 months (G6) groups. Green and blue respectively represent R2 value and Q2 value from the permutation test (A,C,E). The green circles represent the C group, blue squares represent the G3 group, and the red triangles represent the G6 group (B,D,F).
Identification of Metabolites
Overall 1,950 metabolites were identified. Using the criteria VIP > 1 and p < 0.05, 264 DEMs were detected in Group C versus Group G3, 261 DEMs were detected in Group C versus Group G6, and 271 DEMs were detected in Group G3 versus Group G6 (Supplementary Table 2). These different metabolites are mainly lipids, carbohydrates, organic acids, nucleic acids, peptides, steroids, hormones and transmitters, and vitamins and cofactors. Compared with the C group, azelaic acid, hypoxanthine, uridine, L-phenylalanine, anserine in the G3 group and G6 group were increased, and alpha-linolenic acid, and adenine were reduced in G3 and G6 groups (Table 1). Compared with the yak fed with grain for 3 months, the metabolites of organic acids, lipids, and peptides in the rumen fluid were upregulated after feeding grain for 6 months. Inversely, the concentrations of some nucleic acid metabolites such as thymidine, hypoxanthine, and uridine 5’-monophosphate, showed a significant decrease.
KEGG Annotation and Metabolic Pathway Analysis of Differential Metabolites
Pathway topology analysis was carried out according to the metabolites identified and the concentrations. The six enriched major metabolic pathways between the C and G3 have been shown in Figure 4A, including linoleic acid metabolism, purine metabolism, glycerophospholipid metabolism, sphingolipid metabolism, alpha-linolenic acid metabolism, and pyrimidine metabolism. Figure 4B displayed the five enriched metabolic pathways of C versus G6, including phenylalanine, tyrosine, and tryptophan biosynthesis metabolism, phenylalanine metabolism, glycerophospholipid metabolism, sphingolipid metabolism, and alpha-linolenic acid metabolism.
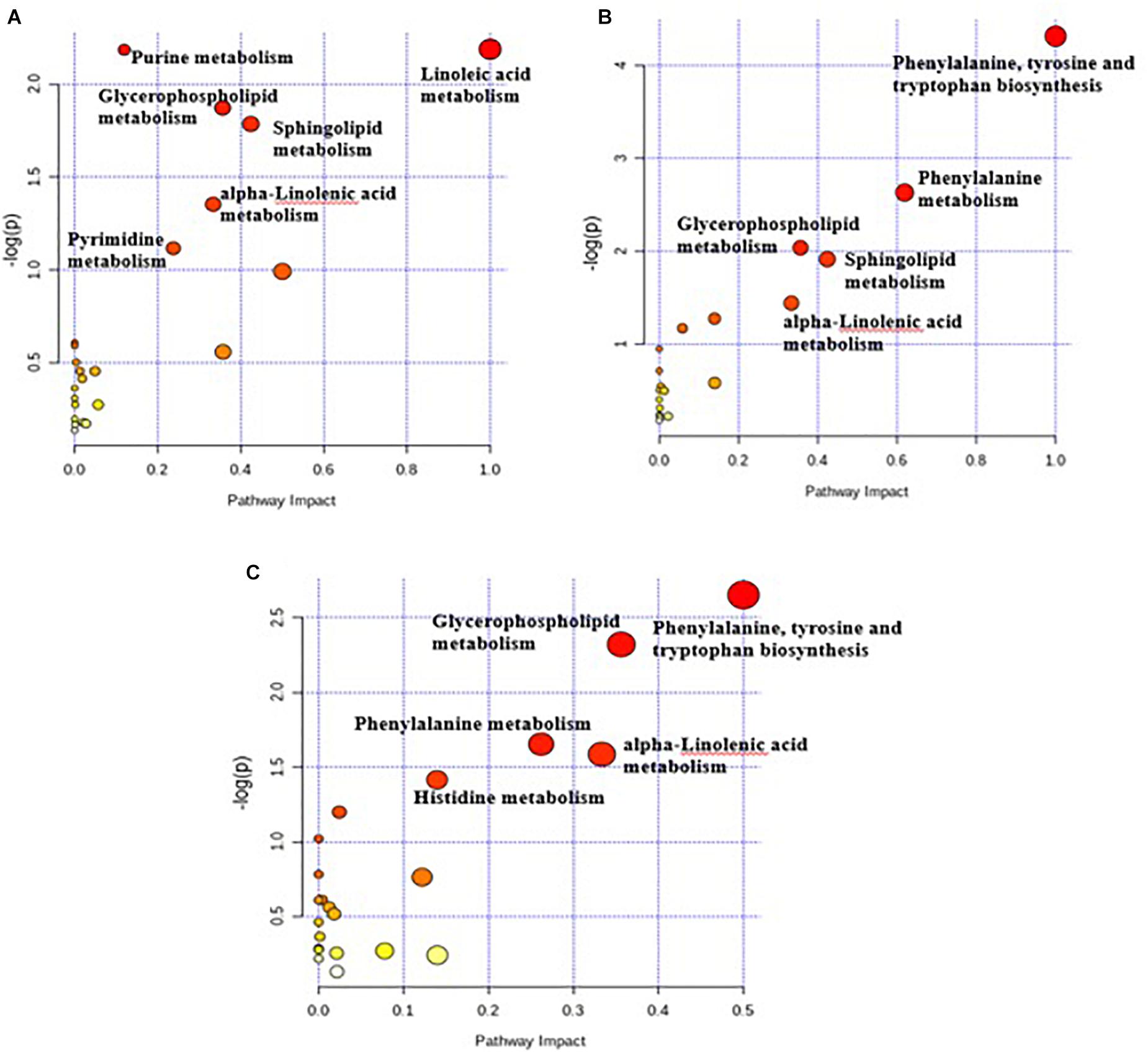
Figure 4. Pathway impact resulting from the differential ruminal metabolites based on Bos taurus KEGG database using MetaboAnalyst 4.0. The X-axis represents pathway impact and the Y-axis represents the pathway enrichment. The larger size of the circle indicates greater pathway enrichment and the darker color indicates higher pathway impact values. The closer the color is to red, the smaller the p-value is. (A) Metabolome view map of metabolites between C and G3 yaks; (B) metabolome view map of metabolites between C and G6 yaks; (C) metabolome view map of metabolites between G3 and G6 yaks.
The five important key metabolic pathways of G3 versus G6 included phenylalanine, tyrosine and tryptophan biosynthesis metabolism, glycerophospholipid metabolism, phenylalanine metabolism, alpha-linolenic acid metabolism, and histidine metabolism (Figure 4C). The pathway impact values of these metabolic pathways were higher than 0.1, which is the critical value for relevance. Although the highly important enrichment metabolic pathways (p < 0.01) of the three comparison groups were different, the highly important enrichment metabolic pathways of all comparison groups include glycerophospholipid metabolism and alpha-linolenic acid metabolism.
The Relationship Between the Metabolites and Rumen Bacteria
The results of this study showed that the genus Fibrobacter was positively associated with homocysteine and adenine, while negatively correlated with anserine, undecanedioic acid, guanosine monophosphate, eicosadienoic acid, hypoxanthine, uridine 5′-monophosphate, and L-phenylalanine (Figure 5). The genus Prevotella, having a higher abundance in the grain-fed groups (G3 and G6), was positively associated with eicosadienoic acid, melatonin and L-phenylalanine, and negatively correlated with adenine, and alpha-linolenic acid. The genus Prevotellaceae_UCG-001 was positively correlated with melatonin and L-phenylalanine. Rikenellaceae_RC9_gut_group was positively correlated with L-proline, and negatively correlated with eicosadienoic acid. There was a higher level of norank_f_Bacteroidales_UCG-001 in the G3 group, which was positively correlated with thymidine and alpha-linolenic acid.
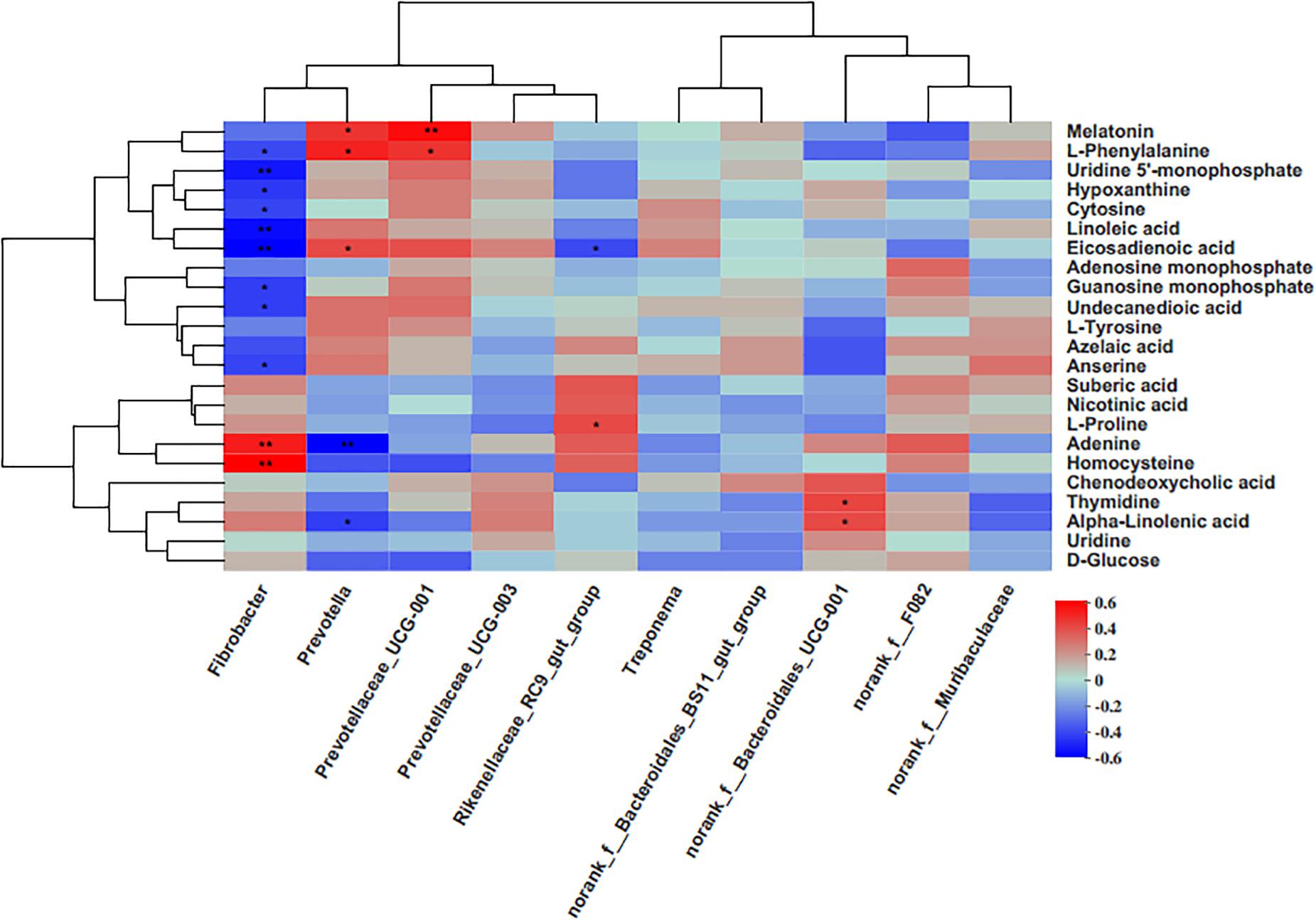
Figure 5. Correlation matrix between the genera level and the ruminal differential metabolites (used in Table 1). Each column in the graph represents a genus, each row represents a metabolite, and each lattice represents a Pearson correlation coefficient between a metabolite and a component. Red represents a positive correlation, while blue represents a negative correlation. *p < 0.05; **p < 0.01.
Discussion
Considering that diet is directly related to the microbiome, this study explored the influence of diet type and diet time on the rumen microbiome and metabolome, which could further provide a reference for selective breeding of yak by manipulating the relationship between diet and rumen microbiome.
The species diversity and abundance of rumen microbiota play a vital role in maintaining the normal physiology of the host. According to previous studies, 16S rRNA gene sequencing was sufficient to illustrate the bacterial diversity in the foregut of herbivores (Shanks et al., 2011; Jami and Mizrahi, 2012; Wang et al., 2018). In this study, the sequencing results revealed most of the microbiota in the sample. The Chao1 value reflected the bacterial species richness, and the Shannon value reflected the bacterial diversity. Our findings proved that the rumen samples of grass-fed yaks showed both higher richness and diversity when compared to grain-fed yaks, consistently with other reports (Plaizier et al., 2017; Liu et al., 2019; Qiu et al., 2020), indicating that the grain-based diet reduced the richness and diversity of rumen bacteria. The results also clarified that the abundance and diversity of rumen bacteria decreased significantly with the extension of the grain diet. The PCoA analyses displayed obvious clusters, and significant differences were observed in the rumen microbial communities among the three groups.
In the current study, the phyla Bacteroidota and Firmicutes were dominant bacteria in the yak rumen in three groups, in agreement with previous findings in yaks (Zhou Z. et al., 2017); Xue et al., 2018; Hu et al., 2019). The abilities of Bacteroidota and Firmicutes to degrade structural polysaccharides have been previously reported (Zened et al., 2013). The main role of Bacteroides is to improve the nutrient utilization of the host by helping the host degrade carbohydrates and to enhance the host’s immunity (Bäckhed et al., 2005; Sears, 2005). We also found that the abundance of Bacteroidota of the grain-fed yak rumen was significantly higher than that in the grass-fed yak. This finding indicated that Bacteroidetes in the rumen show adaptive changes under long-term grain feeding, mainly involved in the degradation of carbohydrates. Fibrobacterota, as well as Fibrobacter, is known for being an important bacterial phylum for ruminants to degrade and digest plant cellulose (Ransom-Jones et al., 2012), and they are usually detected in fiber-rich diets (Fernando et al., 2010). In this study, the abundance of Fibrobacterota in the C group was much higher than that in the G3 and G6 groups, which indicated that Fibrobacterota might be actively involved in the digestion of fiber. Our data showed that the G3 group harbored greater proportions of Patescibacteria, that is, higher abundances than in the C and G6 groups, which meant that the feed type and the grain-fed time affected the changes in microbia population structure. Differences in microbiota may explain their changes in more detail (Qiu et al., 2019). The relative abundance of Rikenellaceae_RC9_gut_group increased with the increase of grain-feeding time, which was similar to the result of a previously reported study (Wang et al., 2019), indicating that this genus may be involved in the carbohydrate degradation.
Principal component analysis and OPLS-DA showed a significant difference in the metabolic components of rumen fluid between the grass-fed and grain-fed groups, and proved the obvious effect of grain-feeding time on rumen metabolites. Many studies have shown that the grain diet could result in the accumulation of organic acids in the rumen (Kleen et al., 2003; Yang et al., 2018a). We observed the same result in our study, as grain feeding increased the concentration of azelaic acid in the rumen when compared to grass feeding. Moreover, compared with the G3 group, the azelaic acid and suberic acid were increased in the G6 group. The superposition of these changes led to a decrease of the rumen pH, which also verified that the richness and diversity of rumen bacteria in the G6 group in this study were lower than the other two groups.
From the perspective of ruminant health, grain diet is prone to cause an acidic intestinal environment, which affects the rumen. The grass-fed method may be a better choice to avoid this phenomenon (Liu et al., 2020). Nucleic acid metabolites such as cytosine, hypoxanthine, uridine, adenosine monophosphate, uridine 5’-monophosphate, and guanosine monophosphate in the rumen were higher in the G3 rumen when compared to C group. This reflected on the degradation of certain bacterial nucleic acids in the rumen due to grain diets, which was supported by the research from McAllan and Smith (1973). They found that bacterial nucleic acid (RNA or DNA) incubated with rumen fluid was quickly converted into xanthine, hypoxanthine, and uracil. However, with the extension of grain-feeding time, the concentration of thymidine, hypoxanthine, and uridine 5′-monophosphate decreased. A possible explanation for this result is that the lower bacterial diversity and abundance in the rumen led to a decrease of bacterial nucleic acid that could be degraded. The study of the pathway topology determined that the main metabolic pathways were purine metabolism and pyrimidine metabolism. In addition, according to the correlation analysis in the present result, the hypoxanthine was negatively associated with Fibrobacter. Therefore, it may be possible to use hypoxanthine as a biomarker to identify grass-fed or grain-fed production systems. The amino acids in the rumen were mainly derived from dietary proteins degraded by the rumen microbiota (Zhang et al., 2019). L-phenylalanine in the G3 and G6 groups was significantly higher than the C group. Moreover, pathway analyses indicated that the most abundant pathways after 6 months of grain feeding include phenylalanine, tyrosine and tryptophan biosynthesis, and phenylalanine metabolism. These two metabolic pathways were related to phenylalanine, illustrating the important role of phenylalanine in the metabolic pathways of grain-fed diets. The production of tyrosine by the catalysis of phenylalanine hydroxylase was the main metabolic pathway of phenylalanine (Yang et al., 2018b). This could also explain that the concentration of tyrosine in the rumen fluid increased after 6 months of grain feeding. Biohydrogenation, active lipolysis, and microbial fatty acid synthesis are indicated by the concentration of long-chain fatty acids in the rumen (Kim et al., 2009). Compared with the C group, alpha-linolenic acid decreased in the G3 and G6 groups. In addition, with the increase of grain-feeding time, the metabolites involved in lipid metabolism decreased. The enriched pathways observed in this study, including linoleic acid metabolism, and alpha-linolenic acid metabolism. Rumen microbiota would rapidly hydrogenate unsaturated fatty acids ingested by the rumen through diet. Before being hydrogenated into a saturated end product, galactosidase, lipase, and phospholipase produced by rumen microbes remove unesterified fatty acids (Jenkins, 1993). This process can produce different intermediate fatty acids, especially odd-chain fatty acids (Jenkins et al., 2015). Therefore, linoleic acid and alpha-linolenic acid may be rumen potential biomarkers.
The rumen microbial composition of grass-fed and grain-fed yak was significantly different. Grain feeding reduced the richness and diversity of microbiota. Long-term feeding of grains could reduce the richness and diversity of microbiota further. These changes in microbiota were related to lipid metabolism, amino acid metabolism, and nucleotide metabolism. The rumen metabolites azelaic acid, suberic acid, hypoxanthine, phenylalanine, linoleic acid, and alpha-linolenic acid had sufficient sensitivity and specificity to distinguish grass-fed and grain-fed yaks. The metabolites may be used as potential biomarkers to predict rumen function in different production systems. Our study contributes to a comprehensive understanding of the dynamic and complex relationships between diet and rumen microbiota function, which is essential for formulating appropriate feeding strategies to improve yak production performance.
Data Availability Statement
Publicly available datasets were analyzed in this study. This data can be found here: https://www.ncbi.nlm.nih.gov/bioproject/PRJNA657445.
Ethics Statement
The animal study was reviewed and approved by Animal Ethics Committee of the Chinese Academy of Agricultural Sciences.
Author Contributions
WL, HS, and SZ designed the study. YY, YL, SZ, and LC carried out the sample processing. CX performed the experimental data analysis and wrote the manuscript. PX and BS modified the manuscript. All authors have read and agreed to the published version of the manuscript.
Funding
This research was supported by the National Key R&D Program-Application and Demonstration of High-Efficiency and Safe Yak Breeding Technology in Qinghai-Tibet Plateau (2018YFD0502300), Research and Demonstration on Key Technologies of Qaidam Beef Cattle Industry Development (2018-NK-107), the Modern Agricultural Industry Technology System and Beef Industry Innovation Construction Project of Hebei Province (HBCT2018130204), and Key R&D Projects in Tibet: Preservation of Characteristic Biological Germplasm Resources and Utilization of Gene Technology in Tibet (XZ202001ZY0016N).
Conflict of Interest
The authors declare that the research was conducted in the absence of any commercial or financial relationships that could be construed as a potential conflict of interest.
Publisher’s Note
All claims expressed in this article are solely those of the authors and do not necessarily represent those of their affiliated organizations, or those of the publisher, the editors and the reviewers. Any product that may be evaluated in this article, or claim that may be made by its manufacturer, is not guaranteed or endorsed by the publisher.
Supplementary Material
The Supplementary Material for this article can be found online at: https://www.frontiersin.org/articles/10.3389/fmicb.2021.642959/full#supplementary-material
Supplementary Figure 1 | Venn diagram of Operational Taxonomic Units (OTUs) in three groups.
Supplementary Figure 2 | Rarefaction curves for each sample in three groups.
Supplementary Figure 3 | Principal compon ent analysis (PCA) score plots of metabolite profile. Ellipses represent the 95% confidence in the score plots. The green circles represent grass-fed yaks (C), the blue squares represent grain-fed for 3 months yaks (G3), the red triangles represent grain-fed for 6 months yaks (G6), and the yellow inverted triangles represent the quality control (QC) samples.
Supplementary Table 1 | Ingredients and chemical composition of the formulation.
Supplementary Table 2 | List of identified metabolites.
Footnotes
References
An, D., Dong, X., and Dong, Z. (2005). Prokaryote diversity in the rumen of yak (Bos grunniens) and Jinnan cattle (Bos taurus) estimated by 16S rDNA homology analyses. Anaerobe 11, 207–215. doi: 10.1016/j.anaerobe.2005.02.001
Bäckhed, F., Ley, R. E., Sonnenburg, J. L., Peterson, D. A., and Gordon, J. I. (2005). Host-bacterial mutualism in the human intestine. Science 307, 1915–1920. doi: 10.1126/science.1104816
Chen, H. (2018). VennBiagram: Generating High-Resolution Venn and Euler Plots. R package version 1.6.20.
Fernando, S. C., Purvis, H. T., Najar, F. Z., Sukharnikov, L. O., Krehbiel, C. R., Nagaraja, T. G., et al. (2010). Rumen microbial population dynamics during adaptation to a high-grain diet. Appl. Environ. Microbiol. 76, 7482–7490. doi: 10.1128/AEM.00388-10
Henderson, G., Cox, F., Ganesh, S., Jonker, A., Young, W., Abecia, L., et al. (2015). Rumen microbial community composition varies with diet and host, but a core microbiome is found across a wide geographical range. Sci. Rep. 5:14567. doi: 10.1038/srep14567
Hsieh, T. C., Ma, K. H., and Chao, A. (2016). iNEXT: an R package for rarefaction and extrapolation of species diversity (H ill numbers). Methods Ecol. Evol. 7, 1451–1456. doi: 10.1111/2041-210X.12613
Hu, R., Zou, H., Wang, Z., Cao, B., Peng, Q., Jing, X., et al. (2019). Nutritional interventions improved rumen functions and promoted compensatory growth of growth-retarded yaks as revealed by integrated transcripts and microbiome analyses. Front. Microbiol. 10:318. doi: 10.3389/fmicb.2019.00318
Jami, E., and Mizrahi, I. (2012). Composition and similarity of bovine rumen microbiota across individual animals. PLoS One 7:e33306. doi: 10.1371/journal.pone.0033306
Jenkins, B., West, J. A., and Koulman, A. (2015). A review of odd-chain fatty acid metabolism and the role of entadecanoic acid (C15: 0) and heptadecanoic acid (C17: 0) in health and disease. Molecules 20, 2425–2444. doi: 10.3390/molecules20022425
Jenkins, T. C. (1993). Lipid metabolism in the rumen. J. Dairy Sci. 76, 3851–3863. doi: 10.3168/jds.S0022-0302(93)77727-9
Kim, E. J., Huws, S. A., Lee, M. R., and Scollan, N. D. (2009). Dietary transformation of lipid in the rumen microbial ecosystem. Asian Australas. J. Anim. Sci. 22, 1341–1350. doi: 10.1038/ismej.2016.150
Kleen, J. L., Hooijer, G. A., Rehage, J., and Noordhuizen, J. P. (2003). Subacute ruminal acidosis (SARA): a review. J. Vet. Med. A Physiol. Pathol. Clin. Med. 50, 406–414. doi: 10.1046/j.1439-0442.2003.00569.x
Liu, C., Wu, H., Liu, S., Chai, S., Meng, Q., and Zhou, Z. (2019). Dynamic alterations in yak rumen bacteria community and metabolome characteristics in response to feed type. Front. Microbiol. 10:1116. doi: 10.3389/fmicb.2019.01116
Liu, J., Liu, F., Cai, W., Jia, C., Bai, Y., He, Y., et al. (2020). Diet-induced changes in bacterial communities in the jejunum and their associations with bile acids in Angus beef cattle. Anim. Microbiome 2:33. doi: 10.1186/s42523-020-00051-7
Lozupone, C., and Knight, R. (2005). UniFrac: a new phylogenetic method for comparing microbial communities. Appl. Environ. Microbiol. 71, 8228–8235. doi: 10.1128/AEM.71.12.8228-8235.2005
Mao, S. Y., Huo, W. J., and Zhu, W. Y. (2016). Microbiome–metabolome analysis reveals unhealthy alterations in the composition and metabolism of ruminal microbiota with increasing dietary grain in a goat model. Environ. Microbiol. 18, 525–541. doi: 10.1111/1462-2920.12724
McAllan, A., and Smith, R. (1973). Degradation of nucleic acids in the rumen. Br. J. Nutr. 29, 331–345. doi: 10.1079/bjn19730107
Plaizier, J. C., Li, S., Danscher, A. M., Derakshani, H., Andersen, P. H., and Khafipour, E. (2017). Changes in microbiota in rumen digesta and feces due to a grain-based subacute ruminal acidosis (SARA) challenge. Microb. Ecol. 74, 485–495. doi: 10.1007/s00248-017-0940-z
Qiu, Q., Gao, C., Aziz ur Rahman, M., Cao, B., and Su, H. (2020). Digestive ability, physiological characteristics, and rumen bacterial community of holstein finishing steers in response to three nutrient density diets as fattening phases advanced. Microorganisms 8:335. doi: 10.3390/microorganisms8030335
Qiu, Q., Gao, C., Gao, Z., He, Y., Cao, B., and Su, H. (2019). Temporal dynamics in rumen bacterial community composition of finishing steers during an adaptation period of three months. Microorganisms 7:410. doi: 10.3390/microorganisms7100410
Qiu, Q., Zhang, G., Ma, T., Qian, W., Wang, J., Ye, Z., et al. (2012). The yak genome and adaptation to life at high altitude. Nat Genet. 44, 946–949. doi: 10.1038/ng.2343
Quast, C., Pruesse, E., Yilmaz, P., Gerken, J., Schweer, T., Yarza, P., et al. (2012). The SILVA ribosomal RNA gene database project: improved data processing and web-based tools. Nucleic Acids Res. 41, D590–D596. doi: 10.1093/nar/gks1219
Ransom-Jones, E., Jones, D. L., McCarthy, A. J., and McDonald, J. E. (2012). The fibrobacteres: an important phylum of cellulose-degrading bacteria. Microb. Ecol. 63, 267–281. doi: 10.1007/s00248-011-9998-1
Ren, Q., Si, H., Yan, X., Liu, C., Ding, L., Long, R., et al. (2020). Bacterial communities in the solid, liquid, dorsal, and ventral epithelium fractions of yak (Bos grunniens) rumen. Microbiologyopen 9:e963. doi: 10.1002/mbo3.963
Russell, J. B., and Rychlik, J. L. (2001). Factors that alter rumen microbial ecology. Science 292, 1119–1122. doi: 10.1126/science.1058830
Sears, C. L. (2005). A dynamic partnership: celebrating our gut flora. Anaerobe 11, 247–251. doi: 10.1016/j.anaerobe.2005.05.001
Shanks, O. C., Kelty, C. A., Archibeque, S., Jenkins, M., Newton, R. J., McLellan, S. L., et al. (2011). Community structures of fecal bacteria in cattle from different animal feeding operations. Appl. Environ. Microb. 77, 2992–3001. doi: 10.1128/aem.02988-10
Wang, H., He, Y., Li, H., Wu, F., Qiu, Q., Niu, W., et al. (2019). Rumen fermentation, intramuscular fat fatty acid profiles and related rumen bacterial populations of Holstein bulls fed diets with different energy levels. Appl. Microbiol. Biotechnol. 103, 4931–4942. doi: 10.1007/s00253-019-09839-3
Wang, L., Wu, D., Yan, T., and Wang, L. (2018). The impact of rumen cannulation on the microbial community of goat rumens as measured using 16S rRNA high-throughput sequencing. J. Anim. Physiol. Anim. Nutr. 102, 175–183. doi: 10.1111/jpn.12676
Xue, D., Chen, H., Luo, X., Guan, J., He, Y., and Zhao, X. (2018). Microbial diversity in the rumen, reticulum, omasum, and abomasum of yak on a rapid fattening regime in an agro−pastoral transition zone. J. Microbiol. 56, 734–743. doi: 10.1007/s12275-018-8133-0
Yang, Y., Dong, G., Wang, Z., Liu, J., Chen, J., and Zhang, Z. (2018a). Treatment of corn with lactic acid or hydrochloric acid modulates the rumen and plasma metabolic profiles as well as inflammatory responses in beef steers. BMC Vet. Res. 14:408. doi: 10.1186/s12917-018-1734-3
Yang, Y., Dong, G., Wang, Z., Wang, J., Zhang, Z., and Liu, J. (2018b). Rumen and plasma metabolomics profiling by UHPLC-QTOF/MS revealed metabolic alterations associated with a high-corn diet in beef steers. PLoS One 13:e0208031. doi: 10.1371/journal.pone.0208031
Zened, A., Combes, S., Cauquil, L., Mariette, J., Klopp, C., Bouchez, O., et al. (2013). Microbial ecology of the rumen evaluated by 454 GS FLX pyrosequencing is affected by starch and oil supplementation of diets. FEMS Microbiol. Ecol. 83, 504–514. doi: 10.1111/1574-6941.12011
Zhang, R., Zhu, W., Jiang, L., and Mao, S. (2017). Comparative metabolome analysis of ruminal changes in Holstein dairy cows fed low-or high-concentrate diets. Metabolomics 13:74. doi: 10.1007/s11306-017-1204-0
Zhang, R. Y., Liu, Y. J., Yin, Y. Y., Jin, W., Mao, S. Y., and Liu, J. H. (2019). Response of rumen microbiota, and metabolic profiles of rumen fluid, liver and serum of goats to high-grain diets. Animal 13, 1855–1864. doi: 10.1017/s1751731118003671
Zhou, J. W., Zhong, C. L., Liu, H., Degen, A. A., Titgemeyer, E. C., Ding, L. M., et al. (2017). Comparison of nitrogen utilization and urea kinetics between yaks (Bos grunniens) and indigenous cattle (Bos taurus). J. Anim. Sci. 95, 4600–4612. doi: 10.2527/jas2017.1428
Keywords: yak, rumen, 16S rRNA, metabolomics, bacteria, bacterial diversity
Citation: Xu C, Liu W, Sun B, Zhang S, Zhang S, Yang Y, Lei Y, Chang L, Xie P and Suo H (2021) Multi-Omics Analysis Reveals a Dependent Relationship Between Rumen Bacteria and Diet of Grass- and Grain-Fed Yaks. Front. Microbiol. 12:642959. doi: 10.3389/fmicb.2021.642959
Received: 17 December 2020; Accepted: 20 July 2021;
Published: 06 August 2021.
Edited by:
Hauke Smidt, Wageningen University and Research, NetherlandsReviewed by:
Marcello Abbondio, University of Sassari, ItalyYanghua He, University of Hawai‘i at Mānoa, United States
Copyright © 2021 Xu, Liu, Sun, Zhang, Zhang, Yang, Lei, Chang, Xie and Suo. This is an open-access article distributed under the terms of the Creative Commons Attribution License (CC BY). The use, distribution or reproduction in other forums is permitted, provided the original author(s) and the copyright owner(s) are credited and that the original publication in this journal is cited, in accordance with accepted academic practice. No use, distribution or reproduction is permitted which does not comply with these terms.
*Correspondence: Peng Xie, xiepeng@caas.cn; Huayi Suo, birget@swu.edu.cn