- 1School of Biological Sciences, University of Auckland, Auckland, New Zealand
- 2Child Health Division, Menzies School of Health Research, Charles Darwin University, Darwin, NT, Australia
- 3Department of Emergency Medicine, Massachusetts General Hospital, Boston, MA, United States
- 4Department of Epidemiology, Harvard T.H. Chan School of Public Health, Boston, MA, United States
- 5Harvard Medical School, Boston, MA, United States
- 6Colegio de Ciencias Biológicas y Ambientales, Instituto de Microbiología, Universidad San Francisco de Quito, Quito, Ecuador
- 7Department of Respiratory and Sleep Medicine, Queensland Children’s Hospital, Brisbane, QLD, Australia
- 8Australian Centre for Health Services Innovation, Queensland University of Technology, Brisbane, QLD, Australia
- 9National Heart and Lung Institute, Imperial College London, London, United Kingdom
- 10Royal Brompton and Harefield NHS Foundation Trust, London, United Kingdom
- 11Department of Obstetrics and Gynecology, Peking University Shenzhen Hospital, Shenzhen, China
- 12School of Medicine, University of Western Australia, Perth, WA, Australia
- 13Department of Pediatrics, Gynecology and Obstetrics, Faculty of Medicine, University Hospitals of Geneva, Geneva, Switzerland
- 14Department of Pediatrics, University of Colorado School of Medicine, Aurora, CO, United States
- 15Seattle Children’s Hospital, Seattle, WA, United States
- 16Department of Pediatrics and Microbiology, University of Washington, Seattle, WA, United States
- 17Department of Pediatrics, Childhood Asthma Atopy Center, Humidifier Disinfectant Health Center, Asan Medical Center, University of Ulsan College of Medicine, Seoul, South Korea
- 18Hospices Civils de Lyon, Lyon, France
- 19Division of Pediatric Infectious Diseases, Duke University, Durham, NC, United States
- 20Department of Life Science, Multidisciplinary Genome Institute, Hallym University, Chuncheon, South Korea
- 21Department of Biostatistics, Yale School of Public Health, Yale University, New Haven, CT, United States
- 22Department of Computer Science, City University of Hong Kong, Kowloon, Hong Kong SAR, China
- 23Department of Biomedical Engineering, City University of Hong Kong, Kowloon, Hong Kong SAR, China
- 24Department of Pediatrics, Boston Children’s Hospital, Boston, MA, United States
- 25Division of Pediatric Infectious Diseases, Department of Pediatrics, Center for Vaccines and Immunity, Abigail Wexner Research Institute at Nationwide Children’s Hospital, The Ohio State University College of Medicine, Columbus, OH, United States
- 26Department of Microbiology and Immunology, Geisel School of Medicine at Dartmouth, Hanover, NH, United States
- 27Finnish Institute for Health and Welfare (THL), Helsinki, Finland
- 28Department of Biostatistics and Bioinformatics, Computational Biology Institute, Milken Institute School of Public Health, George Washington University, Washington, DC, United States
- 29CIBIO-InBIO, Centro de Investigação em Biodiversidade e Recursos Genéticos, Universidade do Porto, Campus Agrário de Vairão, Vairão, Portugal
- 30Department of Epidemiology of Microbial Diseases, Yale School of Public Health, New Haven, CT, United States
- 31CHU Poitiers, Infectious Agents Department, Poitiers, France
- 32University of Poitiers, INSERM U1070, Poitiers, France
- 33Department of Biosciences, University of Helsinki, Helsinki, Finland
- 34Nestlé Research, Lausanne, Switzerland
- 35Department of Pediatrics, Feinberg School of Medicine, Northwestern University, Chicago, IL, United States
- 36Department of Life Sciences, Manchester Metropolitan University, Manchester, United Kingdom
- 37Department of Biostatistics and Informatics, Colorado School of Public Health, University of Colorado, Aurora, Aurora, CO, United States
- 38School of Biosystem and Biomedical Science, Korea University, Seoul, South Korea
- 39Shenzhen Children’s Hospital, Shenzhen, China
- 40Starship Children’s Hospital, Auckland, New Zealand
Introduction: The airway microbiota has been linked to specific paediatric respiratory diseases, but studies are often small. It remains unclear whether particular bacteria are associated with a given disease, or if a more general, non-specific microbiota association with disease exists, as suggested for the gut. We investigated overarching patterns of bacterial association with acute and chronic paediatric respiratory disease in an individual participant data (IPD) meta-analysis of 16S rRNA gene sequences from published respiratory microbiota studies.
Methods: We obtained raw microbiota data from public repositories or via communication with corresponding authors. Cross-sectional analyses of the paediatric (<18 years) microbiota in acute and chronic respiratory conditions, with >10 case subjects were included. Sequence data were processed using a uniform bioinformatics pipeline, removing a potentially substantial source of variation. Microbiota differences across diagnoses were assessed using alpha- and beta-diversity approaches, machine learning, and biomarker analyses.
Results: We ultimately included 20 studies containing individual data from 2624 children. Disease was associated with lower bacterial diversity in nasal and lower airway samples and higher relative abundances of specific nasal taxa including Streptococcus and Haemophilus. Machine learning success in assigning samples to diagnostic groupings varied with anatomical site, with positive predictive value and sensitivity ranging from 43 to 100 and 8 to 99%, respectively.
Conclusion: IPD meta-analysis of the respiratory microbiota across multiple diseases allowed identification of a non-specific disease association which cannot be recognised by studying a single disease. Whilst imperfect, machine learning offers promise as a potential additional tool to aid clinical diagnosis.
Introduction
The human respiratory tract has long been of interest to clinicians and microbiologists, with a traditional focus on single, putatively pathogenic microorganisms (Huang and Lynch, 2011). More recent research, enabled by advances in DNA sequencing, has revealed the existence of complex bacterial communities (microbiota) throughout the airways of even healthy individuals (Charlson et al., 2011; Man et al., 2017; Cox et al., 2019). While a protective function of the respiratory microbiota has been proposed (Man et al., 2017), it is increasingly apparent that a microbiota imbalance, or dysbiosis, is frequently associated with disease. Understanding how, or even if, patterns within the microbiota correspond to different respiratory diagnoses is a key challenge.
Respiratory disease in children is a known risk factor for chronic disease in adulthood (Grimwood and Chang, 2015; Bui et al., 2018; Zhang, 2020). As such, any reduction in childhood disease burden could also improve the outlook for adult respiratory health. In particular, respiratory infections in children are associated with future impaired lung function as adults (Zhang, 2020). While connections between paediatric and adult respiratory disease remain enigmatic, it is likely that the airway microbiota plays a role. Indeed, atypical development of the infant upper airway microbiota has been linked to unfavourable respiratory outcomes in older children (Biesbroek et al., 2014; Teo et al., 2015), a pattern which may continue into adulthood. These findings, together with the role of microbes in early life immune education and evidence for a shared core microbiota across different respiratory diseases in children but not adults (van der Gast et al., 2014), suggest a potential therapeutic window aiming at a beneficial microbiota (e.g., via probiotics or targetted antibiotics). Although the microbiota of the upper airway may not contribute directly to pathogenesis of lower airway disease, it nonetheless demands consideration both as a reservoir for lower airway microorganisms as well as being a more clinically accessible site.
Studying the respiratory microbiota in children brings challenges including logistical and ethical considerations with sampling the paediatric lower airway and inherent difficulties with low-microbial-biomass samples (Marsh et al., 2018). Consequently, understanding of the paediatric respiratory microbiota is not as developed as for other organs with more accessible specimens, such as the bowel. However, with an ever-increasing body of studies, and a field that is already starting to look beyond the typical sequencing of amplified bacterial 16S rRNA genes, it is both feasible and timely to examine what these data as a whole reveal about the microbiota and paediatric respiratory illness. Indeed, rigorous examination of existing data should serve to guide future application of approaches based on microbial function, such as metagenomics and metatranscriptomics (Ritchie and Singanayagam, 2020).
Variation within the human microbiota is considerable, with differences among individuals sometimes swamping signals from clinical factors such as disease (Biswas et al., 2015). Further challenges arise due to a lack of standardisation among studies, such as anatomical site sampled, collection technique, DNA extraction method, 16S rRNA gene region sequenced, and bioinformatics approach. This signals the need for large sample numbers which are not always feasible in paediatric respiratory studies. By combining individual participant data (IPD) from multiple studies, IPD meta-analyses help identify biologically and/or clinically relevant patterns that may not otherwise be detected in small, unstratified studies. Standardised bioinformatics pipelines for re-processing microbiota data from multiple studies have been successfully applied to the human gut (Duvallet et al., 2017), sinus (Wagner Mackenzie et al., 2017), and cystic fibrosis (CF) lung microbiotas (Li et al., 2016).
The value of contextualising results across multiple diseases was eloquently demonstrated by Duvallet et al. (2017) in a recent IPD meta-analysis of the gut microbiota. As this approach has never been done for respiratory microbiota across a range of acute and chronic childhood diseases, we collated and re-analysed published 16S rRNA gene-based microbiota data from 20 studies encompassing such diagnoses. We aimed to test several hypotheses, namely that: (a) the airway microbiota differs between health and disease; (b) there is a consistent microbiota signature in children with lower airway disease, irrespective of specific diagnosis; and (c) airway microbiota profiles can be used to detect lower airway disease.
Materials and Methods
Study Selection Strategy
To identify publications with 16S rRNA gene sequence data from cross-sectional analyses containing paediatric respiratory samples, Scopus and PubMed databases were searched on January 2, 2018 using 25 search terms (Supplementary File A). To be included in the analysis studies could only have a single timepoint per individual within that publication and investigate one of the following illnesses: bronchiolitis, bronchiectasis, CF, asthma, wheeze, acute respiratory infections, chronic suppurative lung disease, and protracted or persistent bacterial bronchitis. Studies which focused on lung transplants, individuals undergoing mechanical ventilation, contained 10 or fewer disease samples, or where age, sex, or diagnosis were unclear, were excluded. We also contributed new, at the time unpublished, data of our own (Pillarisetti et al., 2019). Overall, we obtained data from 4884 samples across 21 studies. Separate ethics approvals had been obtained for each original study and the University of Auckland Human Participants Ethics Committee deemed that re-use of these published data did not require additional approval.
Sequence Data Processing
We used a uniform bioinformatics pipeline to analyze all of the included studies (code is supplied in Supplementary Material). The analysis pipeline utilised USEARCH (v.11.0667) (Edgar, 2010) for quality filtering and, where applicable, merging of paired-end reads, after which reference gene alignment and chimaera removal (chimera.uchime) (Edgar et al., 2011) were performed using mothur (v1.38.1) (Schloss et al., 2009). Taxonomic classification (classify.otu) was performed in mothur using the SILVA SSU database (v132) as reference (Quast et al., 2012). Sequences assigned to non-bacterial lineages or that could not be identified to genus level were removed. Reprocessing of the data led to removal of 557 samples not containing any reads which could be identified at genus level through our uniform pipeline. The wide range of sequenced 16S rRNA regions (Supplementary File A and Supplementary Figure E1) necessitated a genus-level phylotype approach (Waite and Taylor, 2014; Callahan et al., 2017). Following data reprocessing, our uniformly applied bioinformatics approach was able to successfully recapitulate most testable claims identified in the original papers, across multiple normalisation approaches and rarefaction depths (Supplementary File C). Removal of samples with <1000 sequences (348 total) resulted in removal of a single study (Langevin et al., 2017), as retention of only 8% of their samples meant this study could not be fairly represented. Hence, only 20 studies were ultimately included. Following normalisation, pseudoreplication bias (i.e., over-representation of a particular bacterial community) was avoided by splitting data into four broad anatomical sites with distinct physicochemical features [nasal, oral, sputum, lower airways (bronchoalveolar lavage or bronchial brushings)] (Marsh et al., 2016; Man et al., 2017; Ronchetti et al., 2018), and avoiding cross-site comparisons. Ultimately, 2789 samples (from 2624 individuals) were retained.
Defining Diagnostic Groupings
Given variations in how clinical diagnoses are reported across studies, we grouped reported diagnoses into separate groups on two levels. The first, broad level grouped diagnoses into (a) controls, comprising individuals deemed healthy or suitable to use as disease controls in the original study; and (b) disease, comprising individuals with diagnosed respiratory disease. For the second, more nuanced level we defined seven mutually exclusive diagnostic groupings: acute infections, asthma, CF, disease control, healthy, suppurative lung diseases (e.g., bronchiectasis, protracted bacterial bronchitis), and wheezing illness.
Data Analysis
Statistical analyses were performed in R (v3.6.2) (R Core Team, 2019), using vegan (Jari et al., 2015) for diversity calculations, and ggplot2 (Wickham, 2016) for generating plots. Genus-level phylotypes differing significantly between diagnostic groupings were identified using linear discriminant analysis effect size (LEfSe) (Segata et al., 2011). We identified the core microbiota for specific anatomical sites and diagnostic groupings by applying a prevalence threshold of ≥75% in the relevant samples, and an abundance filter whereby a genus must represent ≥10% in at least one sample.
Sensitivity Analyses
In addition to anatomical site and clinical condition, we attempted to account for other factors affecting the respiratory microbiota. In sensitivity analyses we examined effect of participant age, data normalisation approach and influence of two specific studies on our overall findings. While results shown here are based on rarefaction to 1000 sequence reads/sample, we also considered a size factor-based normalisation approach (GMPR) (Chen et al., 2018). This allowed for an accounting of the flaws in each method, namely data compositionality in rarefaction and bias caused by differing sequencing depths in GMPR. The data from one study (Wang et al., 2016) formed a distinctly separate cluster in ordination (principle coordinates analysis, multidimensional scaling) analyses (Supplementary Figure E6). The second study (Luna et al., 2018) represented such a large proportion of the overall dataset that we had concerns our findings could simply reflect that study’s original findings. To test for any such effects, we: (a) eliminated all samples from a particular category (e.g., a specific age group/study) then repeated the analyses; and (b) retained only samples pertaining to the single category in question and re-analysed these independently. Both approaches aimed to determine whether our initial overall findings could be recapitulated.
Machine Learning
To determine whether diagnostic groups could be identified based only on microbiota composition, machine learning trials were performed on rarefied data in python (Pedregosa et al., 2011). For this, 60% of samples from a given anatomical site were selected at random to use as training data, with the remaining 40% used for validation. Of five different initial approaches [random forest; neural network; support vector machine (SVM): linear; SVM: radial bias function; SVM: polynomial], random forest was most successful across the majority of trials. Success in this context was determined by the accuracy value (the fraction of correct calls over all calls) (Sokolova et al., 2006). The random forest approach was then applied independently to both the rarefied and GMPR-normalised datasets using the training and validation strategy outlined above. In addition, for both normalisation methods we generated a sample dataset in which the contribution of different diagnostic groupings was set as equivalent to account for differences in sample numbers. These final machine learning approaches were assessed for both their positive predictive value (defined as the fraction of calls of a diagnostic grouping which are correct) and sensitivity (defined as the fraction of samples within a diagnostic grouping which are correctly identified) for all specific diagnostic groupings.
For more details see Supplementary File A.
Results
In total, 1806 potentially eligible studies were identified by literature database searches, of which 347 required screening and evaluation against specific criteria (Supplementary File A). Thirty eligible datasets were ultimately interrogated, and we were able to access 16S rRNA gene sequences and essential metadata for 20 of these, in addition to our own new data (Pillarisetti et al., 2019; Figure 1). After removal of one study (Langevin et al., 2017) due to low sample retention, ultimately 20 datasets were included.
Bioinformatic processing using a uniform analysis pipeline resulted in a final dataset comprising 2789 samples, from 2624 individuals. Following sequence data reprocessing, the assembled dataset encompassed 20 unique studies (Table 1) spanning chronic and acute diagnoses, multiple anatomical sites, and 11 countries. Technical, clinical, and demographic heterogeneity were considerable (Table 2). While use of a uniform bioinformatics pipeline is expected to reduce some underlying data variation, other technical factors such as sequencing technology used, 16S rRNA gene region sequenced, and DNA extraction method could not be readily controlled. This was evident in that the variables “Study,” “Extraction method,” “16S rRNA gene region,” and “sequencing platform” accounted for 12.4, 6.75, 4.76, and 0.438% of underlying data variation, respectively, according to PERMANOVA analysis (Table 3). The most substantial of these technical factors was DNA extraction method, as accounting for this factor could reduce the explanatory power of “Study” to less than 1% (Table 3). Clinical and demographic heterogeneity reflected the broad range of diagnoses included, ages spanning infancy to near-adulthood (<18 years), and diverse strategies regarding anatomical site sampled and sample collection technique. For many clinically relevant factors, e.g., antibiotics and other medication usage, data were incomplete or inconsistently reported.
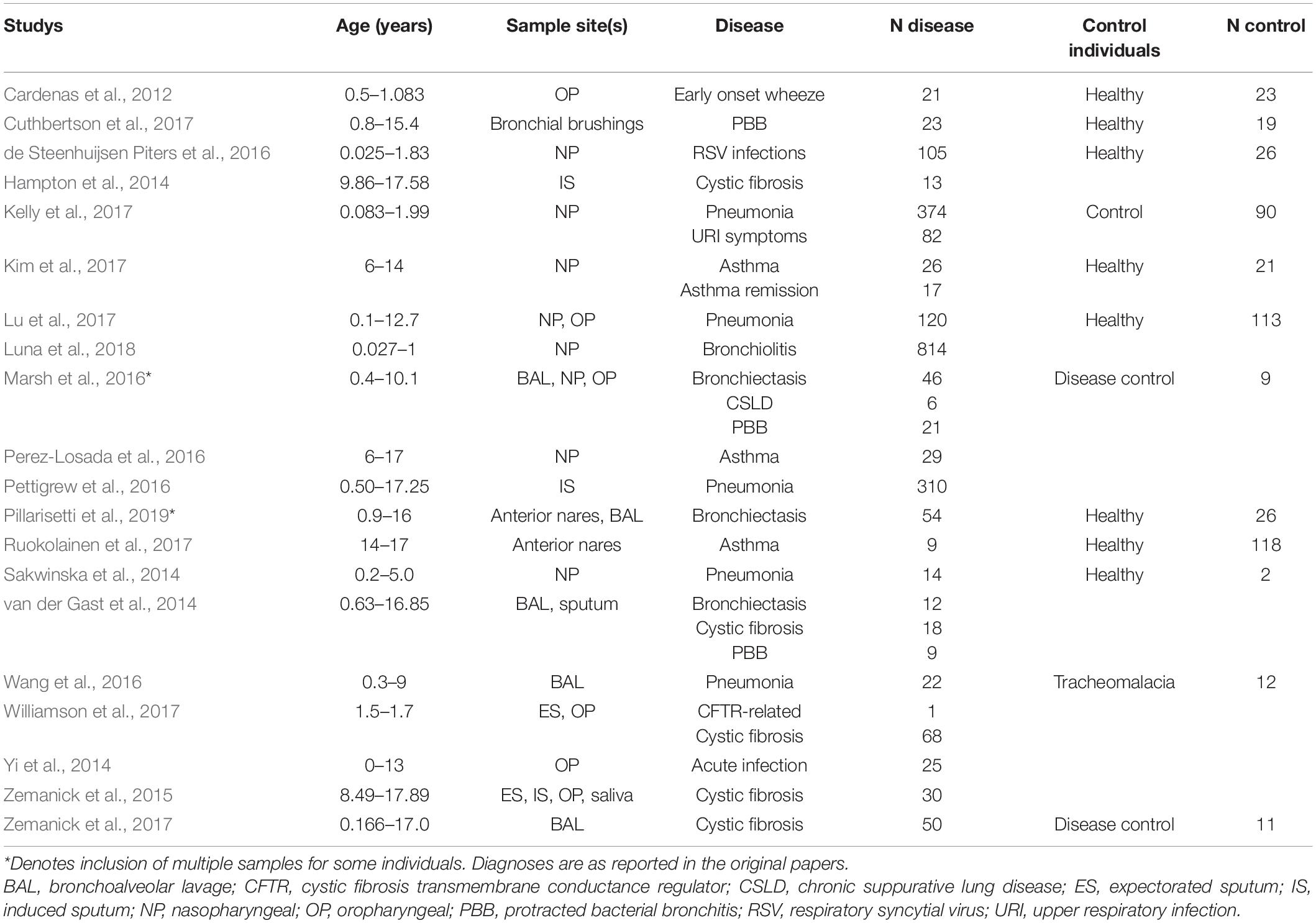
Table 1. Summary of studies which contributed to the final dataset; for more detailed summaries see Supplementary File A (Supplementary Tables E2–E5).
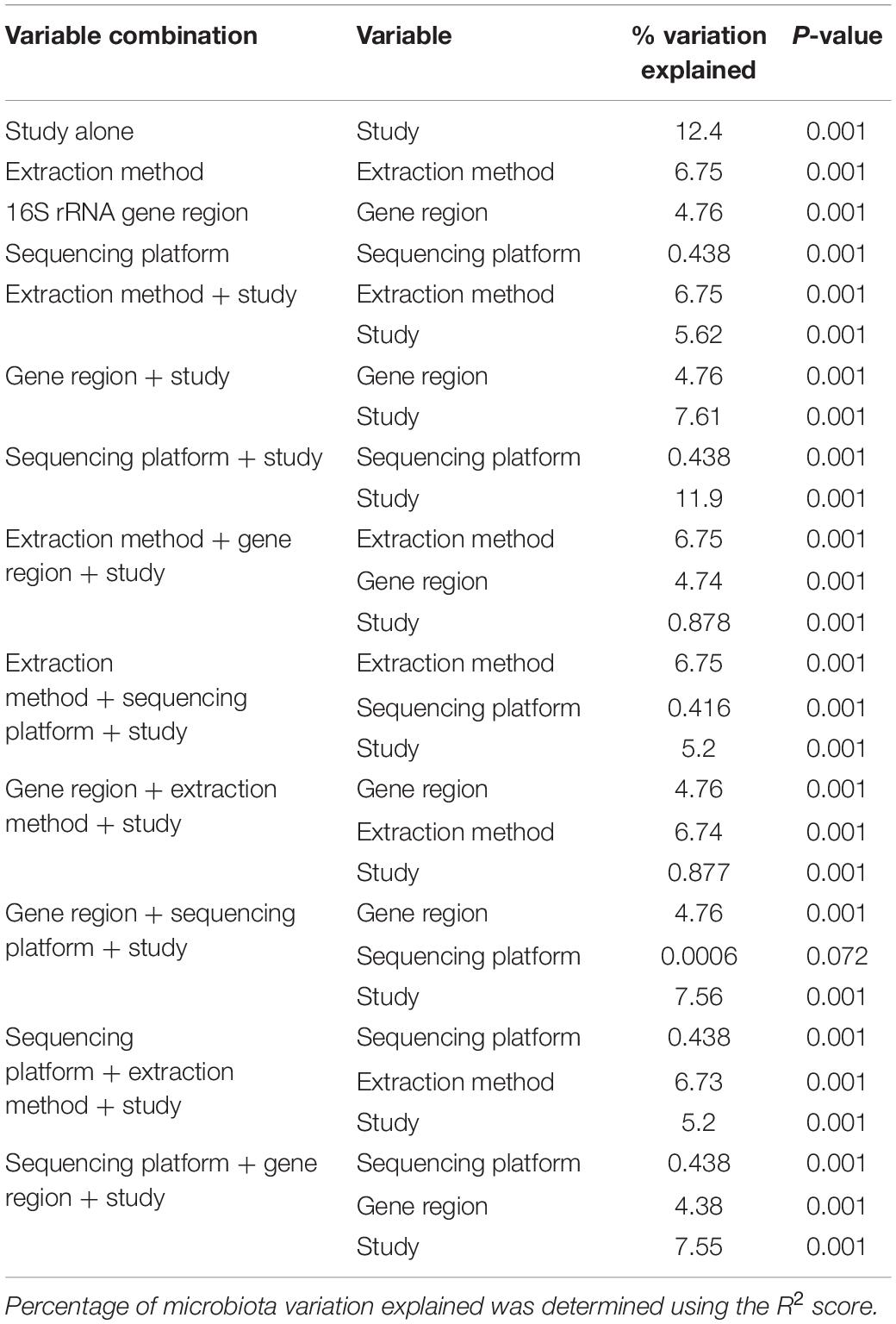
Table 3. PERMANOVA analysis of technical variables amongst microbiota studies included in this meta-analysis.
Bacterial Diversity in Health and Disease
Reduced bacterial diversity, reflecting a lower number and/or uneven distribution of bacterial taxa, is sometimes considered a marker of human disease. We therefore calculated bacterial alpha-diversity on aggregated disease vs. controls data, as well as at a more granular level in which disease and control diagnoses were separated into a total of seven groups (Figure 2). For the aggregated data, alpha-diversity (described by multiple metrics) was significantly higher in controls than disease for nasal and lower airway sites, with the opposite trend for oral samples (Figure 2A). Bacterial richness (observed phylotypes, ACE) differed little between different anatomical sites, though nasal samples did have lower evenness (Shannon, Gini–Simpson), implying dominance by specific taxa. At diagnostic group level (Figure 2B), findings varied depending on anatomical site and whether richness or evenness were considered. One notable finding was that of decreased bacterial diversity in the lower airways of CF patients, although as these data were derived from a single study – and reflect varied pulmonary statuses including both clinical stability and exacerbations at the time of sampling (Zemanick et al., 2017) – one must be circumspect if attempting to infer a wider trend.
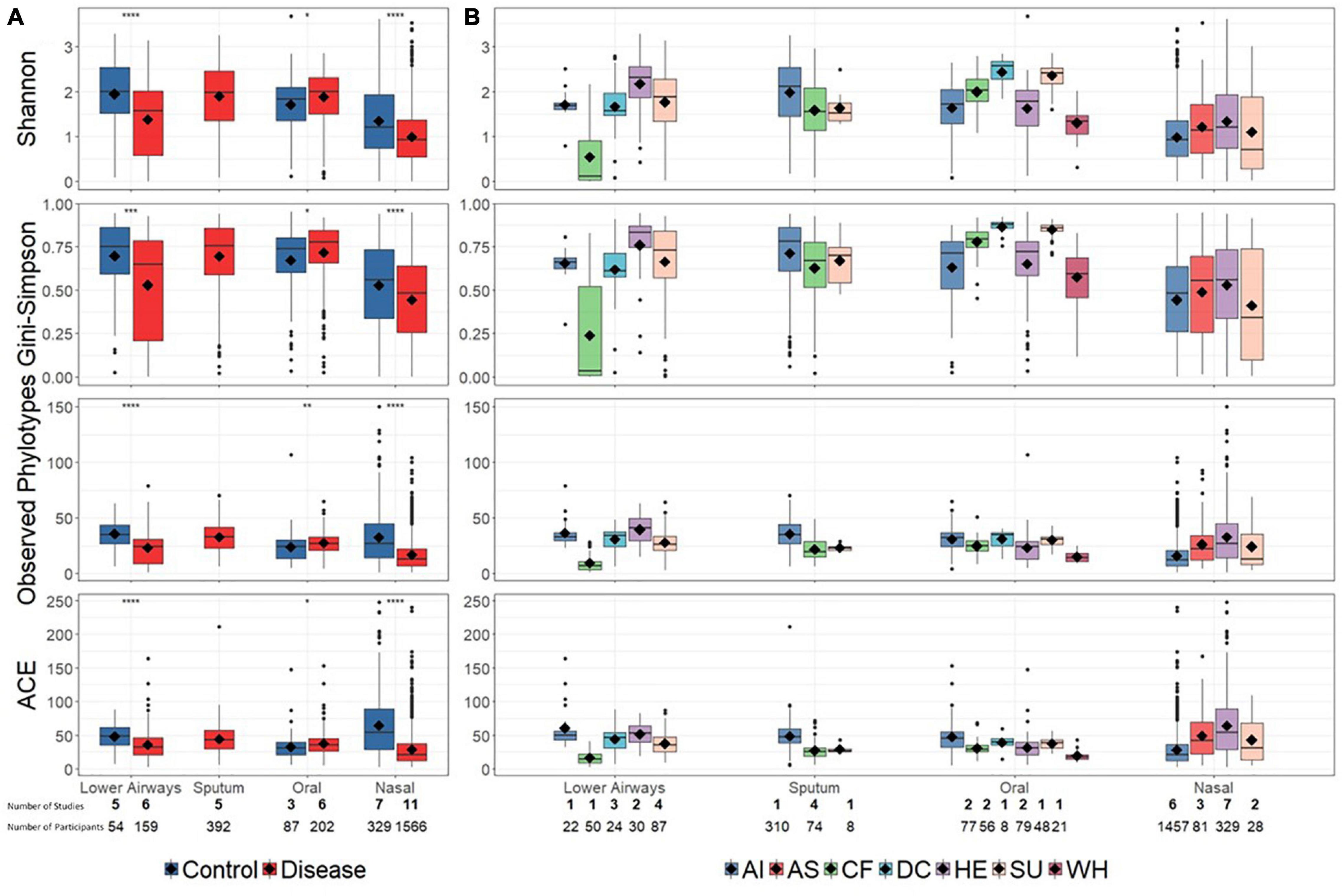
Figure 2. Bacterial alpha-diversity at broad disease (A) and specific diagnostic grouping levels (B). Significant differences in (A), as assessed by a Bonferroni corrected t-test, are denoted by asterisks (p < 0.05). For clarity, statistical significance for (B) is presented in Supplementary Tables E6–9. Diamonds on each box represent the mean value. Alpha-diversity across different anatomical sites (lower airways, sputum, oral, and nasal) was not explicitly compared. Below the plot the number of studies (bold) and number of contributing samples is reported. AI, acute infections; AS, asthma; CF, cystic fibrosis; DC, disease control; HE, healthy; SU, suppurative; WH, wheezing illness.
Microbiota Taxonomic Differences Between Health and Disease
Having established that bacterial diversity differs between respiratory diseases and controls, we sought to determine which bacterial taxa drive these differences. While for oral and lower airway sites the most abundant genera in samples from diseased individuals largely mirrored those from controls (Figure 3), there was far less concordance within the nasal data: disease was associated with clear decreases in relative sequence abundances of Corynebacterium_1, Staphylococcus, and Dolosigranulum but substantial increases in Streptococcus and, to a lesser extent, Haemophilus. Biomarker analysis of nasal samples via LEfSe largely supported these findings, with Haemophilus and Streptococcus identified as potential markers of disease, while Corynebacterium_1, Staphylococcus, and Dolosigranulum were associated with controls (Supplementary File B and Supplementary Tables E10–16). Observed minor changes in rank-abundance for oral samples were also supported by LEfSe, with Veillonella identified as a marker for disease and Prevotella_7 a marker for controls. In addition to the abundant taxa, rare taxa – phylotypes present in ≤10% of samples for a given anatomical site which never comprise ≥1% of 16S rRNA gene sequences within a single sample – were more commonly identified by LEfSe as potential markers for controls, though some could represent potential contaminants (Marsh et al., 2018).
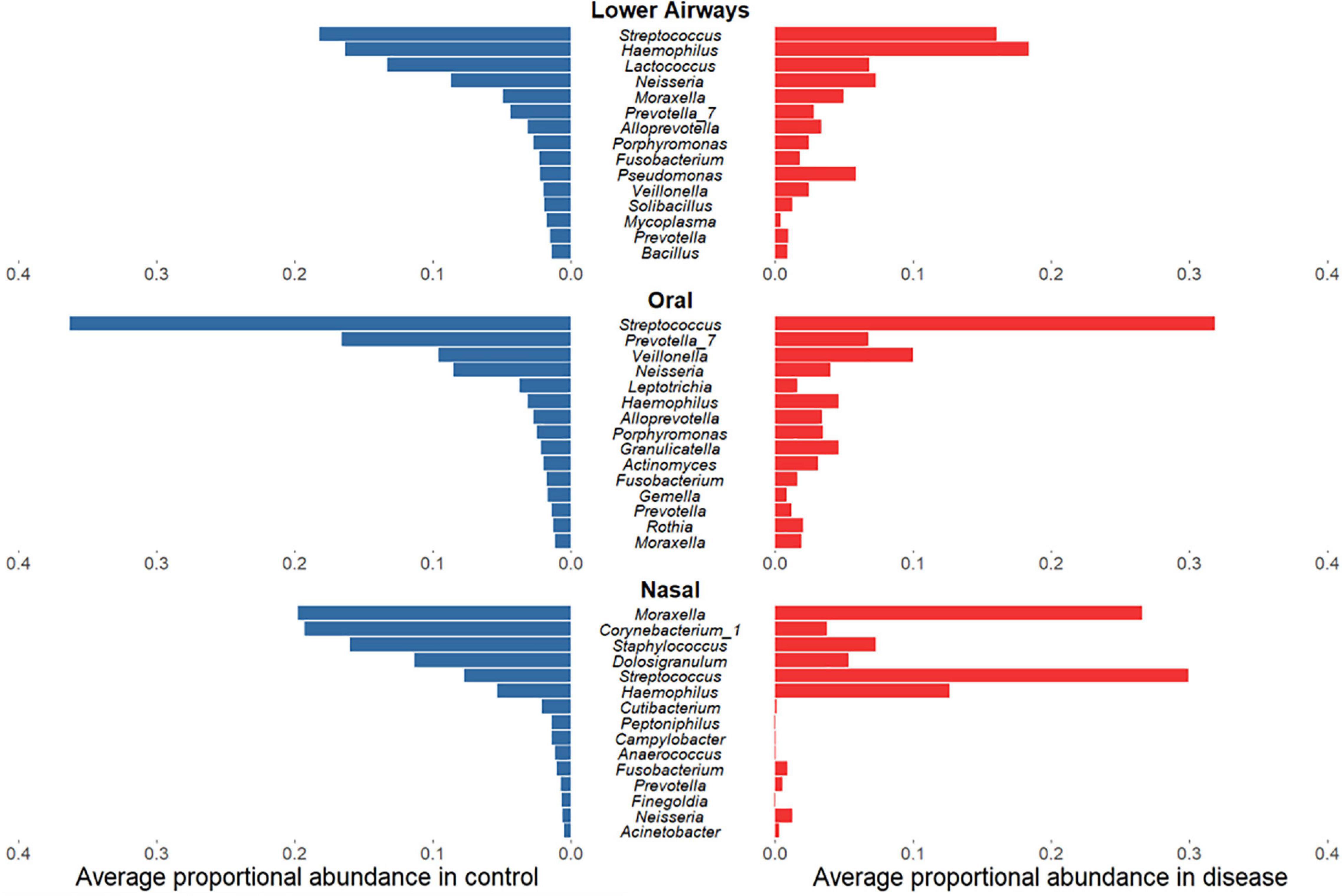
Figure 3. Rank-abundance plots showing the 15 most abundant bacterial phylotypes in control samples (blue) and their respective proportional relative abundance in disease samples (red), for lower airway, oral, and nasal samples. Taxa are ranked based on their relative sequence abundance in controls.
Core Microbiota
Clinical samples often contain a wide variety of microbial taxa and identifying which, if any, of these could be relevant to disease pathology is not straightforward. Core microbiota approaches reduce complexity of microbiota analyses by focusing on only the most prevalent (and in some cases abundant) members of a bacterial community (Astudillo-García et al., 2017). Filtering data to retain only genera present in ≥75% of samples with a relative abundance of ≥10% in at least one sample for a given anatomical site revealed that members of the genus Streptococcus were present in the core microbiota of almost all diagnostic groups, irrespective of anatomical site (Figure 4). The genera Prevotella, Haemophilus, and Granulicatella were also widespread. Notably, there was no 75%-core microbiota for lower airway samples from CF patients, although LEfSe did identify Pseudomonas as a marker of this group. Intuitively, cores for specific diagnostic groups were typically larger than those for overall disease or control cores, potentially due to the lower number of samples required to meet the prevalence threshold and/or other factors common to samples within a given diagnostic group.
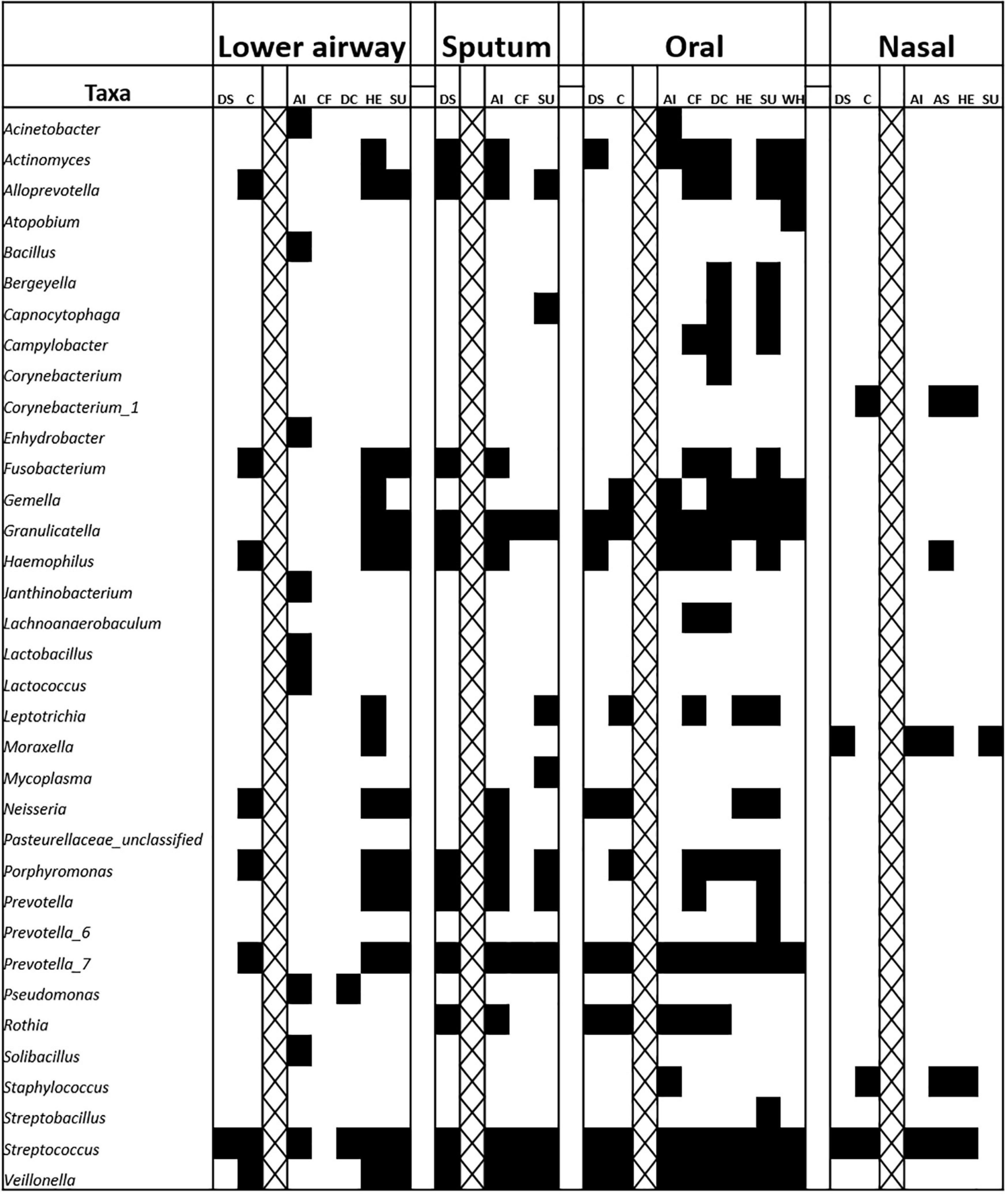
Figure 4. Representation of bacterial genus-level phylotypes in the core microbiota from nasal, oral, sputum, and lower airway samples. A core was defined as presence in at least 75% of samples, based on the rarefied data. An abundance filter was also applied, whereby a genus must represent ≥10% in at least one sample. Cross-hatching separates broad-level comparisons from those involving specific diagnostic groupings, within a given anatomical site. AI, acute infections; AS, asthma; C, control; CF, cystic fibrosis; DC, disease control; DS, disease (any respiratory diagnosis); HE, healthy; SU, suppurative; WH, wheezing illness.
Microbiota Homogeneity Within and Between Diagnostic Groupings
To determine the extent to which different clinical diagnostic groupings overlap or differ in terms of their microbiota, we analysed bacterial beta-diversity. Bray–Curtis dissimilarity (a common measure of beta-diversity) was similar within and between broad control vs. disease groupings regardless of anatomical site (Supplementary File A and Supplementary Figure E7). By contrast, in the finer diagnostic groupings Bray–Curtis dissimilarity was highly dependent on both anatomical site and diagnostic grouping. For instance, in nasal samples values within and between groups were similar (indicating equivalent levels of dissimilarity and overall general lack of microbiota distinctiveness for a given diagnosis). In contrast, for some oral (e.g., suppurative, wheezing illness) and lower airway (e.g., acute infections, suppurative) groupings there was greater microbiota homogeneity within compared to between groups, suggesting a more distinct microbiota associated with these diagnostic groupings. According to PERMANOVA analysis, variables contributing most to variability in the microbiota data were individual study (encompassing multiple technical factors; 12.4% of variation explained), anatomical site (2.5%) and diagnostic group (2.4%) (Supplementary File B and Supplementary Table E17).
Detection of Lower Airway Disease Based on Microbiota Profiles
A key aim was to determine whether lower airway disease could be detected based on composition of upper- and/or lower-airway microbiota. We thus developed a machine learning (random forest) model to predict disease state based upon bacterial distinctiveness of different clinical diagnoses, i.e., is there a distinct microbiota “signature” that allows us to detect different diseases? Machine learning predictions were assessed according to positive predictive value (fraction of calls of a diagnostic grouping which are correct) and sensitivity (fraction of samples within a diagnostic grouping which are correctly identified) for all specific diagnostic groupings. When attempting to use microbiota data alone to predict from which diagnostic group a sample came, success varied with both diagnostic group and anatomical site (Figure 5). For example, sputum samples were particularly effective at distinguishing among diagnostic groupings (with both positive predictive value and sensitivity scoring close to 1), while oral samples also performed well. Lower airway samples were poorest overall at identifying lower airway disease, while detection success of nasal samples varied considerably.
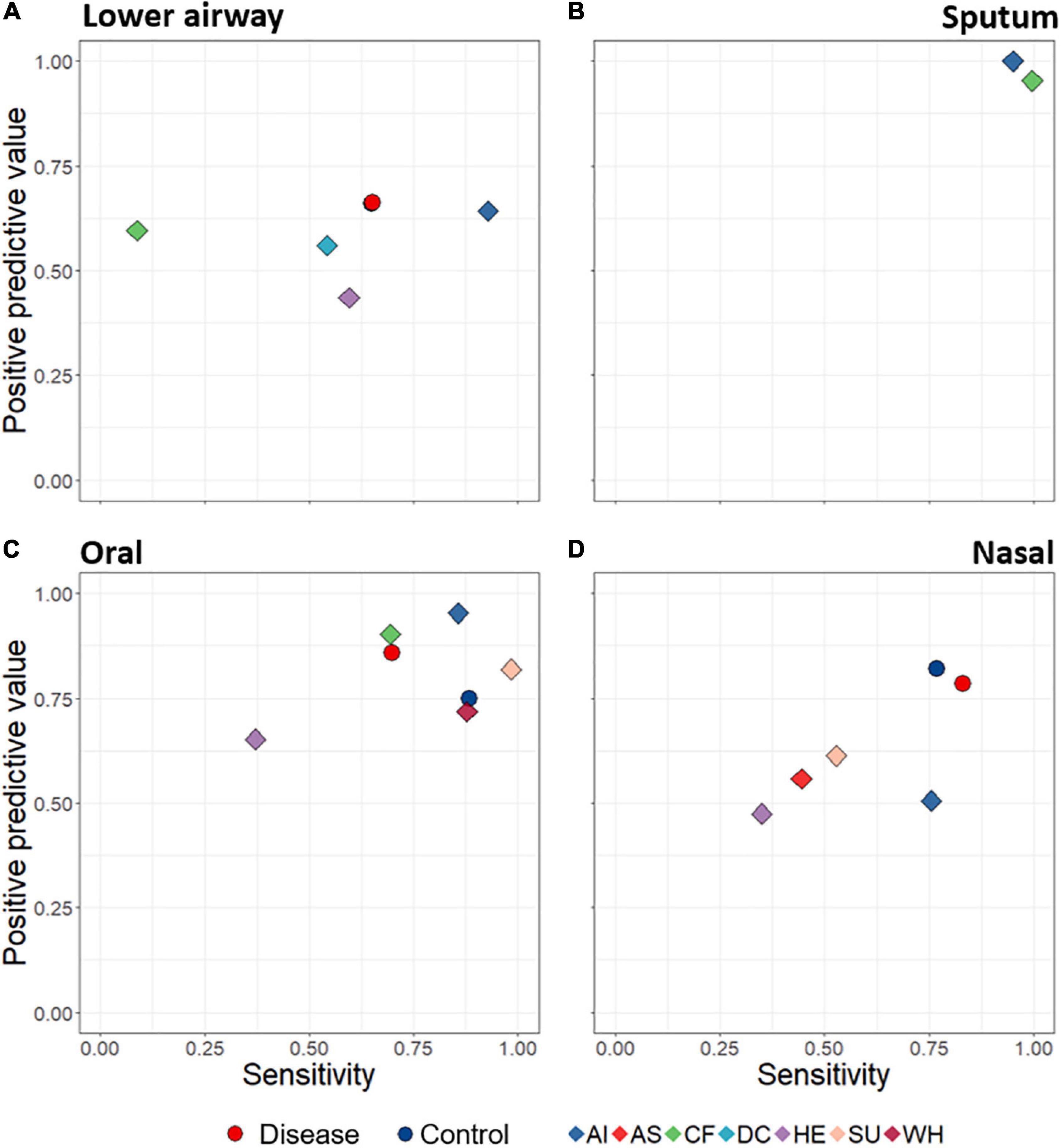
Figure 5. Average positive predictive value (fraction of calls of a diagnostic grouping which are correct) and sensitivity (fraction of samples within a diagnostic grouping which are correctly identified) rates of sample assignments to both broad disease level (circles) and specific diagnostic groupings (diamonds), through use of random forest machine learning. Data are displayed according to anatomical category: lower airways (A), sputum (B), oral (C), and nasal (D). Predictions were made based on rarefied data in which the numbers of samples for each diagnostic grouping were made equal. The Control symbol (blue circles) in (D) is hidden behind the red (Disease) circle. AI, acute infections; AS, asthma; CF, cystic fibrosis; DC, disease control; HE, healthy; SU, suppurative; WH, wheezing illness.
Sensitivity Analyses
Sensitivity analyses investigating effects of participant age, sequence data normalisation approach (rarefaction vs. GMPR), and the Wang et al. (2016) and Luna et al. (2018) studies, yielded results largely consistent with those presented above (for details see Supplementary File A).
Discussion
Our compilation and re-analysis of 16S rRNA gene data using a uniform bioinformatic pipeline of specimens from >2500 individuals obtained from 20 distinct studies enabled testing of three important hypotheses in childhood respiratory diseases. Inclusion of multiple diseases in the same IPD meta-analysis facilitated the search for overarching microbiota patterns of health and disease. The importance of such an approach was highlighted recently for the gut, where half of all genera linked to specific diseases in a single study were in fact associated with more than one disease (Duvallet et al., 2017). Application of this approach to the respiratory microbiota yielded several novel findings with potential clinical implications.
Airway Microbiota Diversity Differs Between Health and Disease
Observed relationships between bacterial diversity and respiratory disease are complex and vary among different conditions, clinical states and sample types (Man et al., 2017). The primary literature is inconsistent, with reports of both higher (Sakwinska et al., 2014; Cuthbertson et al., 2017) and lower (Marsh et al., 2016; Kim et al., 2017) bacterial diversity in controls compared to disease states. Our IPD meta-analysis revealed significantly lower bacterial diversity with disease in samples of nasal and lower airway origin, but the opposite trend for the oral microbiota. In contrast to diversity, overall microbiota profiles were similar for a given anatomical site, with major bacterial taxa such as Moraxella, Streptococcus, Haemophilus, and Neisseria prominent in both health and disease. What did sometimes differ was relative abundances of specific genera, with the ubiquitous Streptococcus notable for its greatly increased abundance in nasal samples from individuals with disease. Rather than a wholesale shift in microbiota composition, disease may instead manifest more as a decrease in bacterial community evenness, with one or more “bloom” taxa increasing their abundance relative to others. Antibiotic usage, while not explicitly tested here due to a lack of comparable data, may contribute to observed reductions in diversity. The most dramatic example of reduced diversity was in the lower airways of children with CF. Although the exacerbation status of these patients may contribute to this low alpha-diversity, the dataset included a mixture of clinically stable and exacerbating individuals with the original study noting no differences in diversity with exacerbation (Zemanick et al., 2017). Whilst speculative, arresting disease progression via microbiota preservation and/or restoration, particularly in long-term or permanent conditions such as CF in which diversity loss may be most marked, may be feasible with wider use of complementary, antibiotic-free approaches such as physiotherapy, anti-inflammatory drugs, probiotics, and vaccines as the first line of defence against disease.
Specific and Non-specific (Overarching) Microbiota Signatures Across Diagnostic Groups
Our analyses revealed that some findings, such as Pseudomonas as a potential biomarker of CF in lower airway samples, were specific to an individual diagnostic group and consistent with previous literature (Emerson et al., 2002). We also saw evidence for non-specific signatures of respiratory disease. LEfSe biomarker analysis identified more putative markers for disease overall than for any single diagnostic grouping. This highlights the need for caution when comparing disease to controls for a single condition, in that one may identify apparent markers of that disease which are in fact more general markers of multiple respiratory diseases. Moreover, shared phylotypes in the cores of multiple diagnostic groupings, but not in control cores, provided further evidence for a non-specific disease signature. Moraxella within nasal samples is a standout example, being present in all disease group cores but not corresponding control cores. These findings are supported by previous research suggesting a shared core microbiota among different diseases (van der Gast et al., 2014). Caution should therefore be exercised when considering specific bacterial taxa identified via microbiota analyses as potentially diagnostic of particular diseases.
Microbiota-Based Detection of Clinical Diagnoses
We used machine learning to evaluate the ability of microbiota profiles to determine clinical diagnostic categories. Sputum and to a lesser extent oral samples yielded the most promising results. Whether this reflects true biological signal or technical biases influencing the random forest model remains unclear. For example, sputum was derived from only two distinct diseases with underlying cohort differences, likely enhancing assignment capabilities beyond that attributable to the microbiota. Indeed, the fact that diagnostic groupings in this meta-analysis were largely reflective of research groups, all of which had distinct methodological characteristics, creates a largely unavoidable bias within the data and presents a challenge for the machine learning model. Such biases could explain the counter-intuitive finding that the microbiota of lower airway specimens appeared to be least effective for detecting lower airway disease, implying the lack of a strong disease signal in the lower airway. However, this result should be interpreted with caution and there is a need for further studies using standardised analytic methods to support or refute these preliminary findings. The previously mentioned technical differences among studies may also explain some of our findings, including that related to the lower airways, yet there was still a detectable signal of diagnostic grouping. Parallel PERMANOVA analyses identified a significant albeit minor contribution of diagnostic grouping to underlying variability within the data, even after accounting for technical factors. Ongoing validation of machine learning (using larger datasets and greater standardisation of approaches) may ultimately lead to a complementary diagnostic strategy for diagnosing paediatric lower airway disease via relatively non-invasive sampling and analysis of the upper airway microbiota.
Methodological Considerations
While the meta-analysis approach is a powerful one, it does have constraints. Some combinations of diagnostic grouping and anatomical site were represented by a single study, limiting more general conclusions. Moreover, even with such a large dataset (∼2800 samples from >2600 individuals) power is quickly lost when attempting to split the data into specific categories. Applying a uniform bioinformatics pipeline removes a potentially substantial source of variation, but clinical (e.g., exacerbation vs. clinical stability) and technical factors (e.g., sampling, DNA extraction) will still contribute to variability within the microbiota data where there is methodological heterogeneity between studies. Indeed, the high explanatory power assigned to individual study by PERMANOVA corroborates the pervasive influence of laboratory-specific approaches and highlights the benefits of a more standardised, cross-laboratory approach. The application of batch correlation tools, particularly where both case and control data are available (Gibbons et al., 2018), also warrants further investigation in an attempt to account for some of these factors. Another useful aspect would be the routine inclusion of both negative technical controls (to detect contaminants) and quantitative approaches such as real-time PCR or droplet digital PCR (to estimate bacterial load). The limited taxonomic resolution of genus-level phylotypes is also noteworthy. The phylotype approach was necessary due to the different 16S rRNA gene regions sequenced, preventing application of operational taxonomic unit or amplicon sequence variant approaches (Callahan et al., 2017). Species- or strain-level differences are likely to be important clinically, and a future focus on deciphering such interactions is warranted. Streptococcus provides a salient example: this genus was prevalent and abundant throughout the assembled dataset, but our analyses based on short-read 16S rRNA gene sequences cannot determine whether this was a single species (e.g., pneumococcus) or, more likely, many different species. This is an inherent limitation of 16S rRNA approaches more generally, due to the conserved nature of this gene and the short-read sequences generated by amplicon sequencing techniques. Additionally, while we relied on LEfSe for detecting differential abundance between controls, disease and different diagnostic groups, this is but one of many techniques for identifying differential taxa. Recent comparative studies of various differential abundance approaches have highlighted both considerable variation in outcomes when different techniques are applied and the lack of a clear-cut candidate for the best available tool at present (Nearing et al., 2021; Wallen, 2021). Finally, in our analysis we only considered bacterial members of the respiratory microbiota, whereas viruses and fungi also likely play key roles within the respiratory tract (Wylie, 2017; Cox et al., 2019; Cuthbertson et al., 2020).
Conclusion
Despite some limitations, our IPD meta-analysis offered key advantages not available through other approaches. Ethical and logistical considerations associated with sampling the airway microbiota contribute to a paucity of case-control studies (only 60% of included studies contained both cases and controls), constraining the ability of individual studies to explicitly compare health and disease. While this complicates interpretation of broader patterns within the airway microbiota, especially compared with the more accessible microbial communities sampled from human faeces (Duvallet et al., 2017), this meta-analysis enabled disease samples from studies lacking controls to be compared to controls from other studies. Additionally, using sensitivity analyses and the twofold (rarefaction and GMPR) normalisation approach, we were able to evaluate the association of various factors presumed to affect microbiota profiles. Re-analysis of multiple studies also enabled comparison of many more diseases than would be feasible in a single study. While airway bacterial diversity differed between health and disease, other differences were more subtle with a combination of non-specific and anatomical site-dependent contributions to microbiota signatures of any specific diagnostic grouping. Identifying such factors via meta-analyses is a further step toward development of novel treatments aimed at rebalancing the airway microbiota, in a manner analogous to faecal transplants and other interventions focused on the gut (Ali and Sweeney, 2020). Moreover, our analysis provides a platform to build future prospective studies where diagnostic categories are uniform.
Data Availability Statement
The data analysed in this study is subject to the following licences/restrictions: we have not made any new datasets available through this study, as data availability is subject to the original studies and their particular ethics agreements. Requests to access these datasets should be directed to the corresponding authors of the original studies.
Ethics Statement
Ethical approval was not provided for this study on human participants because separate ethics approvals had been obtained for each original study and the University of Auckland Human Participants Ethics Committee deemed that re-use of these published data did not require additional approval. Written informed consent for participation was not provided by the participants’ legal guardians/next of kin because separate ethics approvals had been obtained for each original study and the University of Auckland Human Participants Ethics Committee deemed that re-use of these published data did not require additional approval.
Author Contributions
DB: research design, data collation, bioinformatic analysis, and co-wrote manuscript. DW: bioinformatic pipeline development, data interpretation, and contributed to manuscript writing and editing. MT: conceived study, research design, data interpretation, and co-wrote manuscript. RM, CC, AC, and NP: research design, facilitated access to original data, data interpretation, and contributed to manuscript writing and editing. JH, KH, JM, GO’T, and CvdG: facilitated access to original data, data interpretation, and contributed to manuscript writing and editing. PC, WC, LC, WD, ME, AG, LH, S-JH, LJ, MK, B-SK, YK, SL, AM, LP, MP-L, MPe, MPi, OR, LR, OS, PS, BW, HY, EZ, and YZ: facilitated access to original data, data interpretation, and contributed to manuscript editing. All authors contributed to the article and approved the submitted version.
Conflict of Interest
MPi reports personal fees from Mérieux Université, grants from Abacus Diagnostica, outside the submitted work. AM reports grants and personal fees from Janssen, personal fees from Merck, personal fees from Sanofi-Pasteur, personal fees from Roche, outside the submitted work. EZ reports grants and personal fees from Cystic Fibrosis Foundation, outside the submitted work. OS is an employee of Nestlé Research – Societé des Produits Nestlé S.A.
The remaining authors declare that the research was conducted in the absence of any commercial or financial relationships that could be construed as a potential conflict of interest.
Publisher’s Note
All claims expressed in this article are solely those of the authors and do not necessarily represent those of their affiliated organizations, or those of the publisher, the editors and the reviewers. Any product that may be evaluated in this article, or claim that may be made by its manufacturer, is not guaranteed or endorsed by the publisher.
Acknowledgments
We gratefully acknowledge the many funders, supporters, and participants of the original studies on which this meta-analysis is based. We also thank the researchers who conducted those studies for their invaluable contributions. Specific funders for each original study are listed in Supplementary Material.
Supplementary Material
The Supplementary Material for this article can be found online at: https://www.frontiersin.org/articles/10.3389/fmicb.2021.711134/full#supplementary-material
References
Ali, F., and Sweeney, D. A. (2020). In pursuit of microbiome-based therapies for acute respiratory failure. Am. J. Respir. Crit. Care Med. 12, 1616–1618. doi: 10.1164/rccm.202008-3146ed
Astudillo-García, C., Bell, J. J., Webster, N. S., Glasl, B., Jompa, J., Montoya, J. M., et al. (2017). Evaluating the core microbiota in complex communities: a systematic investigation. Environ. Microbiol. 19, 1450–1462. doi: 10.1111/1462-2920.13647
Biesbroek, G., Tsivtsivadze, E., Sanders, E. A., Montijn, R., Veenhoven, R. H., Keijser, B. J., et al. (2014). Early respiratory microbiota composition determines bacterial succession patterns and respiratory health in children. Am. J. Respir. Crit. Care Med. 190, 1283–1292. doi: 10.1164/rccm.201407-1240oc
PubMed Abstract | PubMed Abstract | CrossRef Full Text | Google Scholar
Biswas, K., Hoggard, M., Jain, R., Taylor, M. W., and Douglas, R. G. (2015). The nasal microbiota in health and disease: variation within and between subjects. Front. Microbiol. 6:134. doi: 10.3389/fmicb.2015.00134
Bui, D. S., Lodge, C. J., Burgess, J. A., Lowe, A. J., Perret, J., Bui, M. Q., et al. (2018). Childhood predictors of lung function trajectories and future COPD risk: a prospective cohort study from the first to the sixth decade of life. Lancet Respir. Med. 6, 535–544. doi: 10.1016/S2213-2600(18)30100-0
Callahan, B. J., McMurdie, P. J., and Holmes, S. P. (2017). Exact sequence variants should replace operational taxonomic units in marker-gene data analysis. ISME J. 11:2639. doi: 10.1038/ismej.2017.119
Cardenas, P. A., Cooper, P. J., Cox, M. J., Chico, M., Arias, C., Moffatt, M. F., et al. (2012). Upper airways microbiota in antibiotic-naive wheezing and healthy infants from the tropics of rural Ecuador. PLoS One 7:e46803. doi: 10.1371/journal.pone.0046803
PubMed Abstract | PubMed Abstract | CrossRef Full Text | Google Scholar
Charlson, E. S., Bittinger, K., Haas, A. R., Fitzgerald, A. S., Frank, I., Yadav, A., et al. (2011). Topographical continuity of bacterial populations in the healthy human respiratory tract. Am. J. Respir. Crit. Care Med. 184, 957–963. doi: 10.1164/rccm.201104-0655OC
Chen, L., Reeve, J., Zhang, L., Huang, S., Wang, X., and Chen, J. G. M. P. R. (2018). A robust normalization method for zero-inflated count data with application to microbiome sequencing data. PeerJ 6:e4600. doi: 10.7717/peerj.4600
Cox, M. J., Ege, M. J., and von Mutius, E. (2019). “The lung microbiome,” in European Respiratory Society eds M. J. Cox, M. J. Ege, and E. von Mutius (Lausanne: European Respiratory Society).
Cuthbertson, L., Craven, V., Bingle, L., Cookson, W. O. C. M., Everard, M. L., and Moffatt, M. F. (2017). The impact of persistent bacterial bronchitis on the pulmonary microbiome of children. PLoS One 12:e0190075. doi: 10.1371/journal.pone.0190075
Cuthbertson, L., Felton, I., James, P., Cox, M. J., Bilton, D., Schelenz, S., et al. (2020). The fungal airway microbiome in cystic fibrosis and non-cystic fibrosis bronchiectasis. J. Cystic Fibros 20, 295–302. doi: 10.1016/j.jcf.2020.05.013
de Steenhuijsen Piters, W. A., Heinonen, S., Hasrat, R., Bunsow, E., Smith, B., Suarez-Arrabal, M., et al. (2016). Nasopharyngeal microbiota, host transcriptome, and disease severity in children with respiratory syncytial virus infection. Am. J. Respir. Crit. Care Med. 194, 1104–1115. doi: 10.1164/rccm.201602-0220OC
PubMed Abstract | PubMed Abstract | CrossRef Full Text | Google Scholar
Duvallet, C., Gibbons, S. M., Gurry, T., Irizarry, R. A., and Alm, E. J. (2017). Meta-analysis of gut microbiome studies identifies disease-specific and shared responses. Nat. Commun. 8:1784. doi: 10.1038/s41467-017-01973-8
Edgar, R. C. (2010). Search and clustering orders of magnitude faster than BLAST. Bioinformtaics 26, 2460–2461.
Edgar, R. C., Haas, B. J., Clemente, J. C., Qunice, C., and Knight, R. (2011). UCHIME improves sensitivity and speed of chimera detection. Bioinformatics 27, 2194–2200.
Emerson, J., Rosenfeld, M., McNamara, S., Ramsey, B., and Gibson, R. L. (2002). Pseudomonas aeruginosa and other predictors of mortality and morbidity in young children with cystic fibrosis. Pediatr. Pulmonol. 34, 91–100. doi: 10.1002/ppul.10127
Gibbons, S. M., Duvallet, C., and Alm, E. J. (2018). Correcting for batch effects in case-control microbiome studies. PLoS Comput. Biol. 14:e1006102. doi: 10.1371/journal.pcbi.1006102
PubMed Abstract | PubMed Abstract | CrossRef Full Text | Google Scholar
Grimwood, K., and Chang, A. B. (2015). Long-term effects of pneumonia in young children. Pneumonia 6, 101–114. doi: 10.15172/pneu.2015.6/671
Hampton, T. H., Green, D. M., Cutting, G. R., Morrison, H. G., Sogin, M. L., Gifford, A. H., et al. (2014). The microbiome in pediatric cystic fibrosis patients: the role of shared environment suggests a window of intervention. Microbiome 2:14. doi: 10.1186/2049-2618-2-14
PubMed Abstract | PubMed Abstract | CrossRef Full Text | Google Scholar
Huang, Y. J., and Lynch, S. V. (2011). The emerging relationship between the airway microbiota and chronic respiratory disease: clinical implications. Expert. Rev. Respir. Med. 5, 809–821. doi: 10.1586/ers.11.76
Jari, O., Blanchet, F. G., Friendly, M., Kindt, R., Legendre, P., McGlinn, D., et al. (2015). Vegan: Community Ecology Package. R package version 2.2-1.
Kelly, M. S., Surette, M. G., Smieja, M., Pernica, J. M., Rossi, L., Luinstra, K., et al. (2017). The nasopharyngeal microbiota of children with respiratory infections in Botswana. Pediatr. Infect. Dis. J. 36, e211–e218. doi: 10.1097/INF.0000000000001607
Kim, B., Lee, E., Lee, M., Kang, M., Yoon, J., Cho, H., et al. (2017). Different functional genes of upper airway microbiome associated with natural course of childhood asthma. Allergy 73, 644–652. doi: 10.1111/all.13331
PubMed Abstract | PubMed Abstract | CrossRef Full Text | Google Scholar
Langevin, S., Pichon, M., Smith, E., Morrison, J., Bent, Z., Green, R., et al. (2017). Early nasopharyngeal microbial signature associated with severe influenza in children: a retrospective pilot study. J. Gen. Virol. 98, 2425–2437. doi: 10.1099/jgv.0.000920
Li, J., Hao, C., Ren, L., Xiao, Y., Wang, J., and Qin, X. (2016). Data mining of lung microbiota in cystic fibrosis patients. PLoS One:11:e0164510 doi: 10.1371/journal.pone.0164510
Lu, Z., Dai, W., Liu, Y., Zhou, Q., Wang, H., Li, D., et al. (2017). The alteration of nasopharyngeal and oropharyngeal microbiota in children with MPP and non-MPP. Genes 8:380. doi: 10.3390/genes8120380
Luna, P. N., Hasegawa, K., Ajami, N. J., Espinola, J. A., Henke, D. M., Petrosino, J. F., et al. (2018). The association between anterior nares and nasopharyngeal microbiota in infants hospitalized for bronchiolitis. Microbiome 6:2. doi: 10.1186/s40168-017-0385-0
Man, W. H., de Steenhuijsen Piters, W. A., Wouter, A. A., and Bogaert, D. (2017). The microbiota of the respiratory tract: gatekeeper to respiratory health. Nat. Rev. Microbiol. 15:259. doi: 10.1038/nrmicro.2017.14
PubMed Abstract | PubMed Abstract | CrossRef Full Text | Google Scholar
Marsh, R. L., Kaestli, M., Chang, A. B., Binks, M. J., Pope, C. E., Hoffman, L. R., et al. (2016). The microbiota in bronchoalveolar lavage from young children with chronic lung disease includes taxa present in both the oropharynx and nasopharynx. Microbiome 4:37. doi: 10.1186/s40168-016-0182-1
Marsh, R., Nelson, M. T., Pope, C. E., Leach, A. J., Hoffman, L. R., Chang, A. B., et al. (2018). How low can we go? The implications of low bacterial load in respiratory microbiota studies. Pneumonia 10:7. doi: 10.1186/s41479-018-0051-8
Nearing, J. T., Douglas, G. M., Hayes, M., MacDonald, J., Desai, D. K., Allward, N. E., et al. (2021). Microbiome differential abundance methods produce disturbingly different results across 38 datasets. bioRxiv [Preprint]. doi: 10.1101/2021.05.10.443486
Pedregosa, F., Varoquaux, G., Gramfort, A., Michel, V., Thirion, B., Grisel, O., et al. (2011). Scikit-learn: machine learning in Python. J. Mach. Learn. Res. 12, 2825–2830.
Perez-Losada, M., Crandall, K. A., and Freishtat, R. J. (2016). Two sampling methods yield distinct microbial signatures in the nasopharynges of asthmatic children. Microbiome 4:25. doi: 10.1186/s40168-016-0170-5
Pettigrew, M. M., Gent, J. F., Kong, Y., Wade, M., Gansebom, S., Bramley, A. M., et al. (2016). Association of sputum microbiota profiles with severity of community-acquired pneumonia in children. BMC Infect. Dis. 16:317. doi: 10.1186/s12879-016-1670-4
Pillarisetti, N., Broderick, D., Ainsworth, A., Mulholland, A., Wagner Mackenzie, B., Middleton, D., et al. (2019). The airway microbiota in children newly diagnosed with bronchiectasis largely retains its diversity. Eur. Respir. J. 54:1900704. doi: 10.1183/13993003.00704-2019
Quast, C., Pruesse, E., Yilmaz, P., Gerken, J., Schweer, T., Yarza, P., et al. (2012). The SILVA ribosomal RNA gene database project: improved data processing and web-based tools. Nucleic Acids Res. 41, D590–D596. doi: 10.1093/nar/gks1219
PubMed Abstract | PubMed Abstract | CrossRef Full Text | Google Scholar
Ritchie, A. I., and Singanayagam, A. (2020). Metagenomic characterization of the respiratory microbiome: a pièce de résistance. Am. J. Respir. Crit. Care Med. 3, 321–322. doi: 10.1164/rccm.202005-1686ed
Ronchetti, K., Tame, J., Paisey, C., Thia, L. P., Doull, I., Howe, R., et al. (2018). The CF-Sputum Induction Trial (CF-SpIT) to assess lower airway bacterial sampling in young children with cystic fibrosis: a prospective internally controlled interventional trial. Lancet Respir. Med. 6, 461–471. doi: 10.1016/S2213-2600(18)30171-1
Ruokolainen, L., Paalanen, L., Karkman, A., Laatikainen, T., Hertzen, L., Vlasoff, T., et al. (2017). Significant disparities in allergy prevalence and microbiota between the young people in Finnish and Russian Karelia. Clin. Exp. Allergy 47, 665–674. doi: 10.1111/cea.12895
Sakwinska, O., Bastic Schmid, V., Berger, B., Bruttin, A., Keitel, K., Lepage, M., et al. (2014). Nasopharyngeal microbiota in healthy children and pneumonia patients. J. Clin. Microbiol. 52, 1590–1594. doi: 10.1128/JCM.03280-13
PubMed Abstract | PubMed Abstract | CrossRef Full Text | Google Scholar
Schloss, P. D., Westcott, S. L., Ryabin, T., Hall, J. R., Hartmann, M., Hollister, E. B., et al. (2009). Introducing mothur: open-source, platform-independent, community-supported software for describing and comparing microbial communities. Appl. Environ. Microbiol. 75, 7537–7541. doi: 10.1128/AEM.01541-09
Segata, N., Izard, J., Waldron, L., Gevers, D., Miropolsky, L., Garrett, W. S., et al. (2011). Metagenomic biomarker discovery and explanation. Genome Biol. 12:R60. doi: 10.1186/gb-2011-12-6-r60
Sokolova, M., Japkowicz, N., and Szpakowicz, S. (2006). “Beyond accuracy, F-score and ROC: a family of discriminant measures for performance evaluation”, in Proceedings of the Australasian Joint Conference On Artificial Intelligence. Vol 4304, (Berlin: Springer).
Teo, S. M., Mok, D., Pham, K., Kusel, M., Serralha, M., Troy, N., et al. (2015). The infant nasopharyngeal microbiome impacts severity of lower respiratory infection and risk of asthma development. Cell Host Microbe 17, 704–715. doi: 10.1016/j.chom.2015.03.008
van der Gast, C., Cuthbertson, L., Rogers, G. B., Pope, C., Marsh, R. L., Redding, G. J., et al. (2014). Three clinically distinct chronic pediatric airway infections share a common core microbiota. Ann. Am. Thorac. Soc. 11, 1039–1048. doi: 10.1513/AnnalsATS.201312-456OC
PubMed Abstract | PubMed Abstract | CrossRef Full Text | Google Scholar
Wagner Mackenzie, B., Waite, D. W., Hoggard, M., Douglas, R. G., Taylor, M. W., and Biswas, K. (2017). Bacterial community collapse: a meta-analysis of the sinonasal microbiota in chronic rhinosinusitis. Environ. Microbiol. 19, 381–392. doi: 10.1111/1462-2920.13632
Waite, D. W., and Taylor, M. W. (2014). Characterizing the avian gut microbiota: membership, driving influences, and potential function. Front. Microbiol. 5:223. doi: 10.3389/fmicb.2014.00223
PubMed Abstract | PubMed Abstract | CrossRef Full Text | Google Scholar
Wallen, Z. D. (2021). Comparison study of differential abundance testing methods using two large Parkinson disease gut microbiome datasets derived from 16S amplicon sequencing. BMC Bioinform. 22:265. doi: 10.1186/s12859-021-04193-6
Wang, H., Dai, W., Qiu, C., Li, S., Wang, W., Xu, J., et al. (2016). Mycoplasma pneumoniae and Streptococcus pneumoniae caused different microbial structure and correlation network in lung microbiota. J. Thorac. Dis. 8, 1316–1322. doi: 10.21037/jtd.2016.04.63
Williamson, K. M., Wagner, B. D., Robertson, C. E., Johnson, E. J., Zemanick, E. T., and Harris, J. K. (2017). Impact of enzymatic digestion on bacterial community composition in CF airway samples. PeerJ 5:e3362. doi: 10.7717/peerj.3362
Wylie, K. M. (2017). The virome of the human respiratory tract. Clin. Chest Med. 38, 11–19. doi: 10.1016/j.ccm.2016.11.001
Yi, H., Yong, D., Lee, K., Cho, Y., and Chun, J. (2014). Profiling bacterial community in upper respiratory tracts. BMC Infect. Dis. 14:583. doi: 10.1186/s12879-014-0583-3
PubMed Abstract | PubMed Abstract | CrossRef Full Text | Google Scholar
Zemanick, E. T., Wagner, B. D., Robertson, C. E., Ahrens, R., Chmiel, J. F., Clancy, J. P., et al. (2017). Airway microbiota across age and disease spectrum in cystic fibrosis. Eur. Respir. J. 50:1700832. doi: 10.1183/13993003.00832-2017
Zemanick, E. T., Wagner, B. D., Robertson, C. E., Stevens, M. J., Szefler, S. J., Accurso, F. J., et al. (2015). Assessment of airway microbiota and inflammation in cystic fibrosis using multiple sampling methods. Ann. Am. Thorac. Soc. 12, 221–229. doi: 10.1513/AnnalsATS.201407-310OC
Keywords: microbiota (16S), respiratory tract, respiratory infection, paediatrics, meta-analysis, individual participant data (IPD) meta-analysis
Citation: Broderick DTJ, Waite DW, Marsh RL, Camargo CA Jr, Cardenas P, Chang AB, Cookson WOC, Cuthbertson L, Dai W, Everard ML, Gervaix A, Harris JK, Hasegawa K, Hoffman LR, Hong S-J, Josset L, Kelly MS, Kim B-S, Kong Y, Li SC, Mansbach JM, Mejias A, O’Toole GA, Paalanen L, Pérez-Losada M, Pettigrew MM, Pichon M, Ramilo O, Ruokolainen L, Sakwinska O, Seed PC, van der Gast CJ, Wagner BD, Yi H, Zemanick ET, Zheng Y, Pillarisetti N and Taylor MW (2021) Bacterial Signatures of Paediatric Respiratory Disease: An Individual Participant Data Meta-Analysis. Front. Microbiol. 12:711134. doi: 10.3389/fmicb.2021.711134
Received: 18 May 2021; Accepted: 01 December 2021;
Published: 23 December 2021.
Edited by:
Leonard Peruski, Centers for Disease Control and Prevention (CDC), United StatesReviewed by:
Benjamin G. Wu, New York University, United StatesCeline Pattaroni, Monash University, Australia
Copyright © 2021 Broderick, Waite, Marsh, Camargo, Cardenas, Chang, Cookson, Cuthbertson, Dai, Everard, Gervaix, Harris, Hasegawa, Hoffman, Hong, Josset, Kelly, Kim, Kong, Li, Mansbach, Mejias, O’Toole, Paalanen, Pérez-Losada, Pettigrew, Pichon, Ramilo, Ruokolainen, Sakwinska, Seed, van der Gast, Wagner, Yi, Zemanick, Zheng, Pillarisetti and Taylor. This is an open-access article distributed under the terms of the Creative Commons Attribution License (CC BY). The use, distribution or reproduction in other forums is permitted, provided the original author(s) and the copyright owner(s) are credited and that the original publication in this journal is cited, in accordance with accepted academic practice. No use, distribution or reproduction is permitted which does not comply with these terms.
*Correspondence: Michael W. Taylor, bXcudGF5bG9yQGF1Y2tsYW5kLmFjLm56