- 1Department of Microbiology and Biotechnology, Institute of Plant Science and Microbiology, University of Hamburg, Hamburg, Germany
- 2Bioinformatics Core, University Medical Center Hamburg-Eppendorf, Hamburg, Germany
- 3Virus Genomics, Heinrich-Pette-Institute, Leibniz Institute for Experimental Virology, Hamburg, Germany
- 4Institute of General Microbiology, Molecular Microbiology, Kiel University, Kiel, Germany
- 5Faculty of Medicine, Core Unit Systems Medicine, University of Würzburg, Würzburg, Germany
- 6T/TQ-MN, Lufthansa Technik AG HAM, Hamburg, Germany
Microbial contamination of fuels, associated with a wide variety of bacteria and fungi, leads to decreased product quality and can compromise equipment performance by biofouling or microbiologically influenced corrosion. Detection and quantification of microorganisms are critical in monitoring fuel systems for an early detection of microbial contaminations. To address these challenges, we have analyzed six metagenomes, one transcriptome, and more than 1,200 fluid and swab samples taken from fuel tanks or kerosene. Our deep metagenome sequencing and binning approaches in combination with RNA-seq data and qPCR methods implied a metabolic symbiosis between fungi and bacteria. The most abundant bacteria were affiliated with α-, β-, and γ-Proteobacteria and the filamentous fungi Amorphotheca. We identified a high number of genes, which are related to kerosene degradation and biofilm formation. Surprisingly, a large number of genes coded enzymes involved in polymer degradation and potential bio-corrosion processes. Thereby, the transcriptionally most active microorganisms were affiliated with the genera Methylobacteria, Pseudomonas, Kocuria, Amorpotheka, Aspergillus, Fusarium, and Penicillium. Many not yet cultured bacteria and fungi appeared to contribute to the biofilm transcriptional activities. The largest numbers of transcripts were observed for dehydrogenase, oxygenase, and exopolysaccharide production, attachment and pili/flagella-associated proteins, efflux pumps, and secretion systems as well as lipase and esterase activity.
Introduction
Microbial contamination of fuels is a phenomenon widely reported in the literature. Microbiological analyses showed that fuel systems like aviation fuel and storage tanks are often contaminated by a variety of microorganisms with a rather low diversity (Gaylarde et al., 1999; Rauch et al., 2006; Yemashova et al., 2007). In fact, detailed knowledge of the physiology and metabolism of complex bacterial–fungal communities in fuel-associated environments is limited. Previous studies implied that the bacteria–fungi microbial communities can form tightly attached biofilms on various surfaces. These studies identified mainly microorganisms associated with the bacterial genera Methylobacterium, Bacillus, Pseudomonas, Serratia, Sphingomonas, Variovorax, Flavobacterium, Arthrobacter, and Alcaligenes, as well as a limited number of fungal isolates linked to Amorphotheca (also known as Cladosporium and Hormoconis), Aspergillus, and Fusarium; no archaea were reported (Beech and Sunner, 2004; Beale et al., 2014; Khan et al., 2015).
Crude oil and derived products consist of hydrocarbons of various molecular weights and other organic compounds. Fuel products are refined alkanes, alkenes, and cycloalkanes of different chain lengths as well as aromatic compounds, especially benzene, toluene, and xylene. In general, fuel products are subject to microbial degradation (i.e., biofouling), which affects mostly kerosene (aviation fuel) and diesel fuels. Thereby, microbial alkane degradation is important for the bioremediation of petroleum-contaminated environments as well as for microbial enhanced oil recovery (Gaylarde et al., 1999; Brown et al., 2010).
Bacterial and fungal biocatalytic oxidation and degradation reactions of alkanes, alkenes, and aromatics are mediated by different enzymes such as dehydrogenases, laccases, and peroxidases (Clemente et al., 2001; Saratale et al., 2007; Khan et al., 2015). By utilizing fuels as a C-source and by metabolite excretion, microorganisms cause fuel alteration and deterioration. Other effects of microbial contamination are biofouling/biodegradation and corrosion of fuel system components such as pipelines, filters, storage tanks, and effects of the protective layer of paint on the tank surfaces (Rauch et al., 2006; Passman, 2013; Li et al., 2017; Martin-Sanchez et al., 2018). Under aerobic conditions, the utilization of the hydrocarbons of diesel fuel and aviation kerosene by microorganisms results in the production of organic acids, which are corrosive to metals (Hendey, 1964; Parbery, 1969; Morton and Surman, 1994). Under anaerobic conditions, sulfate-reducing bacteria and the expression of the dissimilatory sulfate reductase (DsrA, DsrB) are a major cause of corrosion in low-oxygen or oxygen-free environments (King and Miller, 1971; Gaylarde and Beech, 1989; Morton and Surman, 1994).
Initial studies suggests that polymeric coatings, which are designed to prevent contact of the underlying materials with corrosive media and microorganisms, were found to be susceptible to microbial degradation after formation of microbial biofilms on the surfaces of the coating materials, including epoxy and polyamide primers and aliphatic polyurethanes, exposing the underlying metals to biofilm-enhanced bio-corrosion (Thorp et al., 1997; Gu et al., 1998; Ii et al., 1998; Stern and Howard, 2000). Notably, in general, the overall degradation pathways and microbial interactions have yet to be fully researched. Within this framework, we addressed the following questions in the current study: Can we identify specific bacterial communities associated with fungi in different aircraft habitats and fuel-containing biofilms? What are the most active genes in these communities, and do they give any hints on biofouling of kerosene and bio-corrosion processes? To answer these questions, we first systematically characterized the microbiomes of six biofilms grown directly in aircraft tanks. We used these data to map transcriptomes of one fresh kerosene biofilm. In addition, we have analyzed more than 1,200 fluid and swab samples to evaluate the diversity of bacteria and fungi. This comprehensive data set gives a detailed insight into the community structure and the most strongly expressed genes. Further, it offers first clues on biofouling and the initial corrosion of the tank and protective paint.
Materials and Methods
Sampling
Metagenome/Metatranscriptome Samples
Fuel biofilm and liquid samples were taken from different suppliers and were provided via the Lufthansa Basis in Hamburg, Germany. Samples were collected from right-hand, left-hand, main, center, and reserve tanks mainly (Supplementary Table 1). Samples 1–3 for the metagenomic studies represent biofilm samples of planes mostly based in the equatorial climate zone. Samples 4–6 are derived from planes based in the central European climate zone. Samples for quantitative PCRs were obtained from fuel samples and only few biofilm samples or water samples (Supplementary Table 1). For total RNA isolation, the samples were taken by the use of the “DNA/RNA Shield Collection Tube w/Swab” (R1107-E, Zymo Research, Freiburg, Germany) and stored at –80°C for further analysis.
Microbial Community Analyses
In addition, we analyzed > 1,200 kerosene, water/kerosene, and biofilm samples (Supplementary Table 1). The samples were all collected from airplanes made by global manufacturers and were obtained from the Lufthansa Basis in Hamburg, Germany. Liquid samples were first sonicated by the use of a Handheld Ultrasonic Homogenizer UP200Ht (200 W, 26 kHz) (Hielscher Ultrasonics GmbH, Teltow, Germany) following the manufacturer’s description. Sonification was done for a maximum of 30 s using an amplitude of 60% and a cycle condition of 0.5. One hundred milliliters was filtrated using a 0.22-μm cellulose-acetate filter (Typ 11107, Sartorius Stedim Biotech, Göttingen, Germany).
Scanning Electron Microscopy
Scanning electron microscopy (SEM) was performed as previously published (Krohn-Molt et al., 2013, 2017). Therefore, samples were fixed in paraformaldehyde (1%) (30525-89-4, Merck, Taufkirchen Deutschland) and glutaraldehyde (0.25%), (G4004, Merck, Taufkirchen Deutschland), dehydrated by ascending alcohol series and dried at the critical point with Balzers CPD 030 Critical Point Dryer (BAL-TEC, Schalksmühle, Germany). After coating samples with gold/carbon using a sputter coater SCD 050 (BAL-TEC, Schalksmühle, Germany), scanning electron micrographs were taken with a LEO 1525 (Zeiss, Oberkochen, Germany).
Total DNA Extraction From Biofilm and Liquid Samples
To obtain highly purified DNA, different isolation kits were tested. Finally, we settled that, for the metagenome analyses, high-quality DNA was extracted from the filters and cotton swabs by using the “NucleoBond High Molecular Weight Genomic DNA-Kit” with minor modifications (740160.20, MACHEREY-NAGEL, Düren, Germany). For the analyses via qPCR, the DNA was extracted via the “Quick DNA Fungal/Bacterial Microprep-Kit” (D6007, Zymo Research, Freiburg, Germany) with the addition of 5 mg lysozyme and 0.5 mg proteinase K (89833 and EO0491, Thermo ScientificTM, Darmstadt, Germany). Extracted DNA was stored at 4°C overnight. The concentration and purity of DNA were analyzed using a NanoPhotometer® NP80 (IMPLEN, München, Germany).
Metagenome Sequencing, de novo Assembly, and Binning
DNA Libraries were constructed applying the NEBNext® UltraTM DNA Library Prep Kit for Illumina (E7370L, New England Biolabs, Frankfurt am Main, Germany), according to the manufacturer’s protocol, which is based on ligation of Illumina adapters. Depending on sample concentration, 3–60 ng DNA was used. The initial fragmentation of DNA was performed on the Bioruptor® NGS [Diagenode, Seraing (Ougrée), Belgium] with 30 s on/30 s off for 16 cycles. Sequencing of metagenomic DNA libraries was performed on the NextSeq 500 platform (Illumina, San Diego, CA, United States) as paired-end run (2 × 150 cycles) with approximately 33–168 mio reads per sample.
The quality of raw sequencing data was assessed using FastQC (v0.11.8, Andrews, 2010). The sequences of sequencing adapters and low-quality bases (Q-score < 20) were removed using Trimmomatic (v0.39, Bolger et al., 2014). Kraken2 (v2.0.7-beta, Wood and Salzberg, 2014) was used in combination with the NCBI nt database (obtained on October 27, 2019) for the k-mer-based taxonomic classification. Relative abundances were then estimated with Bracken (v2.2, Lu and Salzberg, 2020). Complementarily, an assembly based analysis was carried out. Trimmed sequence reads were assembled with metaSPAdes (v3.14.0, Nurk et al., 2017). Contigs shorter than 1,000 bps were not used in subsequent analysis steps. Sequence reads were aligned to contigs using BWA mem (v0.7.17, Li, 2013) for abundance estimation and subsequent binning with metaBAT2 (v2.12.1, Kang et al., 2019). The binned sequences were aligned to the nt database using blastn of the BLAST + suite (v2.7.1) in megablast mode (Camacho et al., 2009). The alignment results were used to manually curate the binning results.
For the sequences’ functional characterization and additional 16S rRNA phylogenetic profiling, the Integrated Microbial Genomes (IMG) pipeline and homology searches was used (Chen et al., 2019; Mukherjee et al., 2019).
RNA Extraction and Sequencing
Triplicates of the biofilm sample were used for total RNA extraction. Therefore, we used a hot phenol method with minor modifications previously published (Krohn-Molt et al., 2017). The concentration and quality of the total RNA were measured by a NanoDrop ND 2000 instrument (PEQLAB Biotechnologie GmbH, Erlangen, Germany) and verified on a 1.2% formaldehyde-agarose gel. For the preparation of DNA libraries suitable for sequencing, ∼300 ng of total RNA was subjected to rRNA depletion using Illumina’s Ribo-Zero Gold Epidemiology kit (MRZE724, Illumina, San Diego, CA, United States). After rRNA depletion, RNA samples were fragmented with Mg2 + at 94°C for 3 min using the NEBNext Magnesium RNA Fragmentation Module (E6150S, New England Biolabs, Frankfurt am Main, Germany) followed by RNA purification with the Zymo RNA Clean & Concentrator kit (R1019, Zymo Research, Freiburg, Germany). Fragmented RNA was dephosphorylated at the 3′ end, phosphorylated at the 5′ end, and decapped using 10 U T4-PNK ± 40 nmol ATP and 5 U RppH, respectively (M0356S, New England Biolabs, Frankfurt am Main, Germany). After each enzymatic treatment RNA was purified with the Zymo RNA Clean & Concentrator kit. The small RNA fragments were ligated for cDNA synthesis to the 3′ SR and 5′ SR adapter using the NEBNext Multiplex Small RNA Library Prep kit for Illumina (E7560S, New England Biolabs, Frankfurt am Main, Germany). PCR amplification to add Illumina adaptors and indices to the cDNA was performed for 16 cycles. Barcoded DNA Libraries were purified using magnetic MagSi-NGSPREP Plus beads (MDKT00010500, AMS Biotechnology, Abingdon, United Kingdom). Libraries were quantified with the Qubit 3.0 Fluometer (Thermo ScientificTM, Darmstadt, Germany), and library quality and size distribution were checked on a 2100 Bioanalyzer with the high-sensitivity DNA kit (Agilent, Waldbronn, Germany). Sequencing was performed with 5% PhiX Control Library as spike-in on the NextSeq 500 platform (Illumina, San Diego, United States) in 2 × 150 nt paired-end mode with the High Output Kit v2.5 (300 cycles). Demultiplexed FASTQ files were generated with bcl2fastq2.
Processing and Analysis of RNA-seq Reads
Quality assessment was performed with FastQC as described above. Quality control was performed with fastp (v0.20.0, Chen et al., 2018); paired-end reads were corrected and merged in this step (with parameters –merge –correction). The merged reads were then processed with CoMW for functional annotation (v1.0.0, Anwar et al., 2019). During this step, reads were assembled and aligned to integrated databases M5nr and eggNOG (v3.0) (with parameter -d 1) (Powell et al., 2012; Wilke et al., 2012). Contigs assembled by CoMW were aligned to the NCBI nt database used as previously described (Gallardo-Becerra et al., 2020). Mapping of RNA reads to the reference genomes of Methylobacterium brachiatum (GCF_003697185.1), Amorphotheca resinae (GCA_003019875.1), and the de novo-assembled transcriptomes was performed with Bowtie2 v2.3.5.1 (Langmead et al., 2019) and TopHat2 v2.1.1 (Kim et al., 2013). Htseq-count v0.13.5 (Anders et al., 2015) was used to calculate the number of reads mapping to each feature in the genomes. Subsequently, RPKM values were calculated for each feature and circular representations of both genomes in Figure 6 were constructed with DNAPlotter (Carver et al., 2009), included in Artemis v17.0.1 (Carver et al., 2012). Log2-normalized values were calculated against the ‘‘housekeeping’’ sigma factor 70-coding rpoD (EBB05_11765) for M. brachiatum and transcription factor TFIIB (M430DRAFT_41748) for A. resinae. In order to overcome the lack of annotation for the genome of A. resinae, all encoded protein sequences were searched locally against NCBI’s non-redundant database1 with DIAMOND blastp v0.9.30.131 (Buchfink et al., 2015). All data are found in Supplementary Table 6.
qPCR Procedures and Quantification
The number of 16S rRNA and ITS-region gene copies was determined for all samples by real-time PCR. The analysis was performed by the QuantStudio 3 detection system by the use of SYBR® Select Master Mix (4472919, Thermo ScientificTM, Darmstadt, Germany) and the primer bact_338f/518r (5′-ACT CCT ACG GGA GGC AGC AG-3′, 5′-ATT ACC GCG GCT GCT GG-3′; Ovreås et al., 1997; Ishii and Fukui, 2001) and fungi_NL1f/LS2r (5′-ATA TCA ATA AGC GGA GGA AAAG-3′, 5′-ATT CCC AAA CAA CTC GAC TC-3′; O’Donnell, 1993; Cocolin et al., 2000). Each reaction contained 0.15 ng/μl of template DNA, 1.25 μM of each primer (Eurofins, Hamburg, Germany), and one-time SYBR® Select Master Mix. The protocol for the real-time PCR was as follows: an initial denaturation step for 3 min at 95°C followed by 40 cycles of 15 s at 95°C, 20 s at 58°C, and 20 s at 72°C. Finally, a melting curve was plotted after each run to verify the product specificity. We used a standard curve for real-time PCR of known copy numbers of total DNA of Burkholderia glumae for bacteria and Amorphotheca resinae for fungi. This standard curve with serial dilutions was generated to show the proportion between gene copy number and Ct value.
Sequences Obtained and GenBank Submissions
Raw sequence data have been submitted to the European Nucleotide Archive. They are publicly available under accession PRJEB40662. Assemblies of the six fungi–bacteria metagenomes are available via IMG/MER2 using the IMG IDs 3300039030, 3300039916, 3300038809, 3300039918, 3300038808, and 3300039917.
Results
Macro- and Microscopic Investigations of Fuel Biofilms
Biofouling is a result of microbial growth in fuels. Growth occurs at the interface of the fuel and water phase and on the tank surface. Today, only a limited number of detailed studies are available. Therefore, to better understand the 3D structures and makeup of kerosene-grown microbial biofilms, we used microscopical techniques. Scanning electron microscope (SEM) images showed a multilayer and complex structure of the formed biofilms. Further SEM inspections identified various bacterial and fungi-like structures (Figure 1). The morphological differences of the bacteria ranged from spherical to cylindrical forms (rods) with more or less rounded ends. In addition, various extensions and attachment “organs” were visible. In addition, we observed various fungal filamentous structures. Furthermore, we noticed a tight adherence of bacteria to few fungal species. The SEM images also indicated that the cells produced exopolymeric substance EPS and in part net-like or filamentous structures to from lump-like aggregates (Figures 1, 2). Surprisingly, not only in the biofilm sample but also in the liquid samples were we able to detect three-dimensional microbial aggregates (Figure 2). The sizes of these liquid phase multispecies aggregates ranged from 7.69 to 61.26 μm in length and width. Interestingly, the use of sonification allowed the disruption of these aggregates. After a sonification step of up to 30 s, we were able to dissolve particles and separate the microorganisms from their containing EPS matrix.
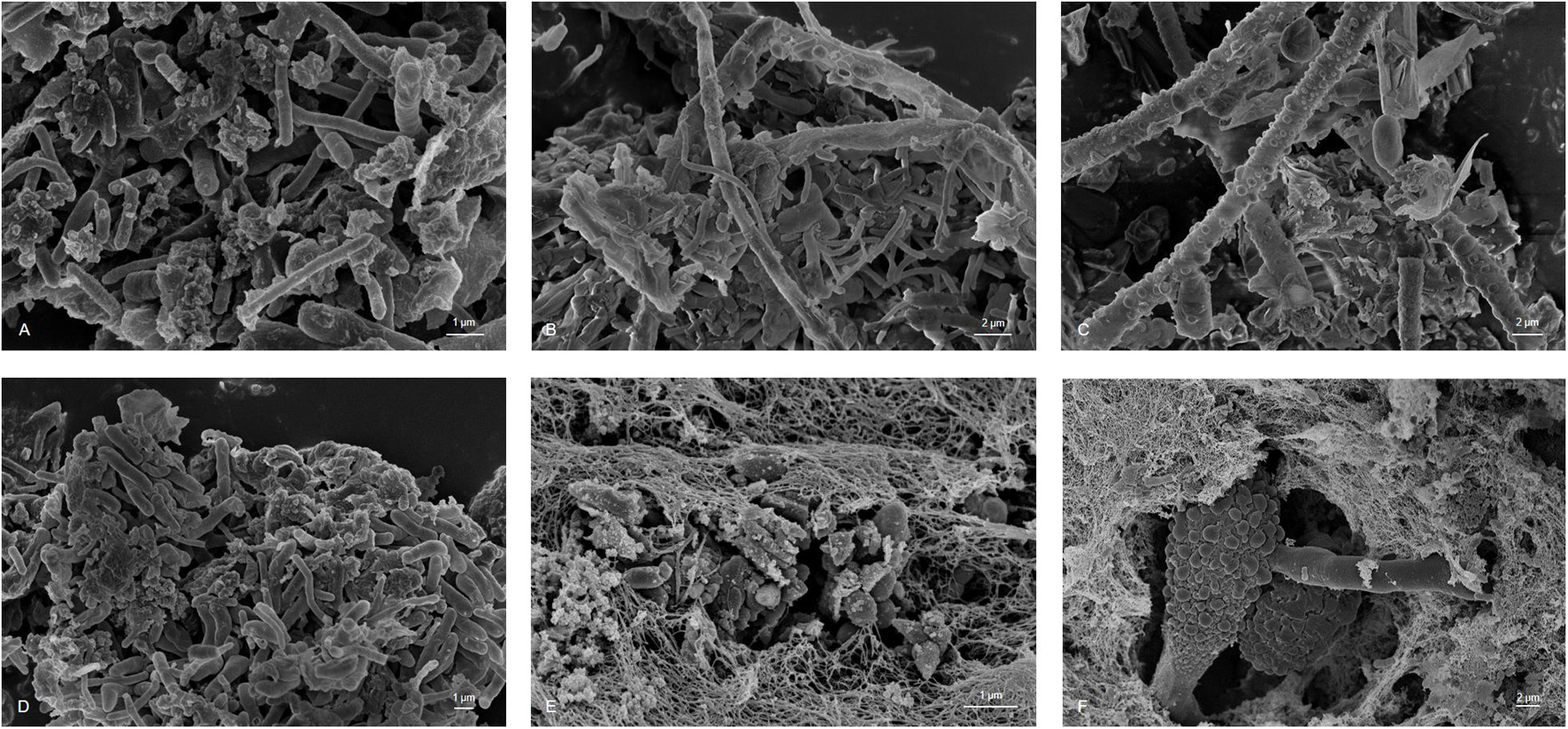
Figure 1. Scanning Electron Microscopy microphotography of sampled material showing hypha and conidia of fungi species and bacteria integrated in an EPS matrix (A) sample 1, (B) sample 2, (C) sample 3, (D) sample 4, (E) sample 5, and (F) sample 6; scale bars of 1–2 μm are indicated in the images (REM LEO 1525, 5.00 kV).
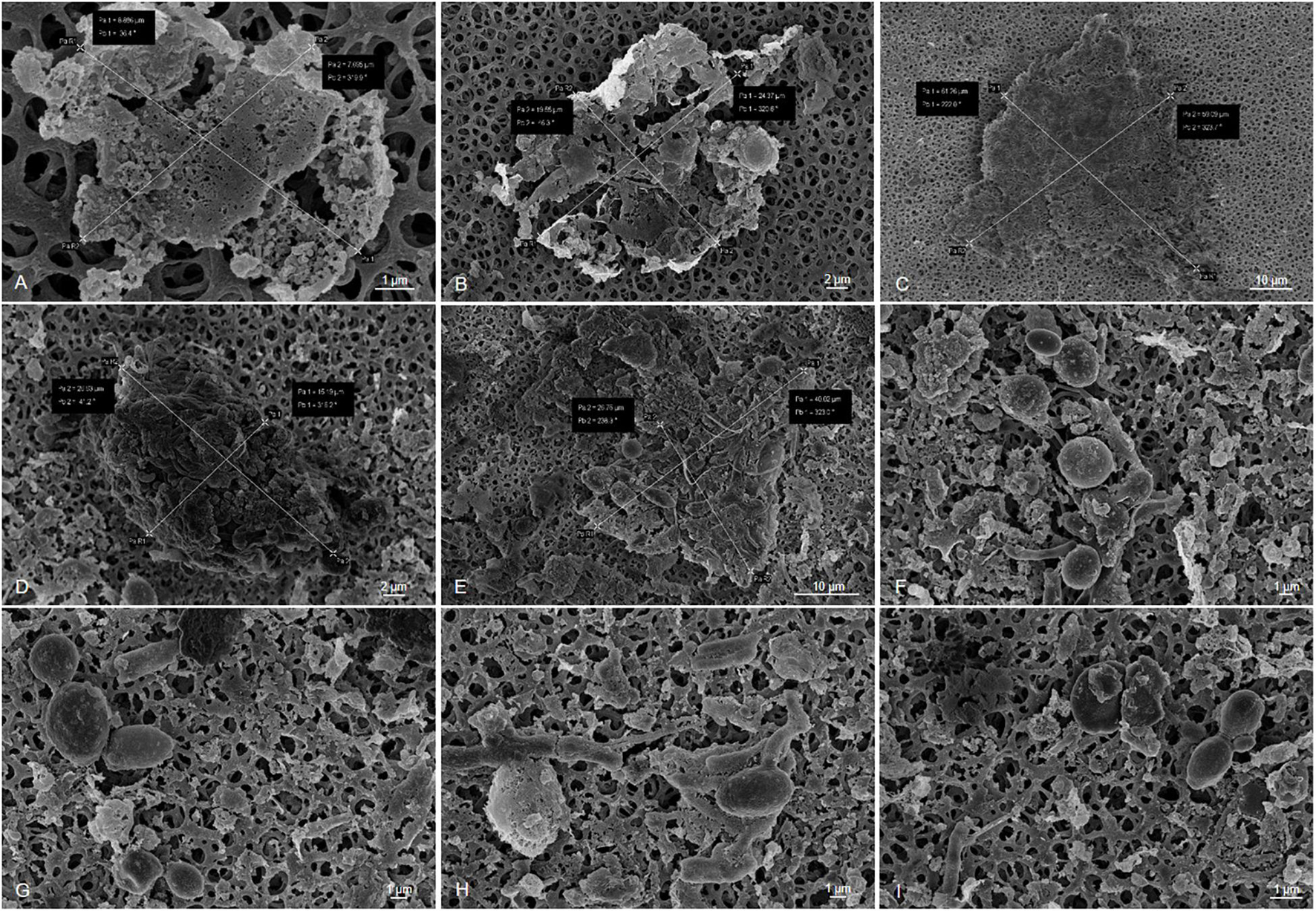
Figure 2. Sonification experiments of filtered kerosene on cellulose acetate membranes (F and W sampling). Sonification conditions: amplitude 60% of 200 W, cycle: 50%, volume: 500 ml, (A–C) sonification time: 0 s, (D–F) Sonification time: 15 s, (G–I) sonification time: 30 s. Scale bars of 1–10 μm are indicated in the images (REM LEO 1525, 5.00 kV).
Altogether, these data implied that the analyzed kerosene-grown biofilms built rather complex 3D structures and that they are found not only on the interphase but also in form of aggregates (organic matter particles, OMPs) in the liquid phase.
Population Structure, Metabolic Potential, and Most Active Genes of Microorganisms in Fuel-Containing Biofilms
Metagenome-Based Microbial Community Analyses of Fuel Biofilms
To further advance the phylogenetic and genome-wide analyses, we established the metagenomes of six fuel samples derived from kerosene tanks of different commercial airplanes. The Illumina-based analyses established DNA contig sequences ranging from 53.9 to 116.2 Mbp, for each of the six metagenomes relying on 19,529 to 53,653 assembled contigs. The largest contigs ranged from 0.3 to 1.5 Mbp, respectively (Supplementary Table 2). Assuming that the genome size of the different bacteria ranges from 3 to 8 Mbp and the main fungi’s genome size is about 28 Mbp, it is reasonable to speculate that the established DNA sequences represent the microbial community’s metagenome significantly.
A Kraken2 analysis (Wood and Salzberg, 2014) calculated, under a cutoff of 1% of abundance, a presence of up to 14 microbial genera per metagenome (Figure 3A). Our data imply that the gene sequences were mainly affiliated with members of α-, β-, and γ-Proteobacteria and the Ascomycota. On the genus level, the bacteria Pseudomonas, Burkholderia, Sphingomonas, and Methylobacterium and the filamentous fungus Amorphotheca were the most abundant organisms, while it is important to note that the datasets implied a highly diverse distribution of various genera inside the respective kerosene tanks (Figure 3A).
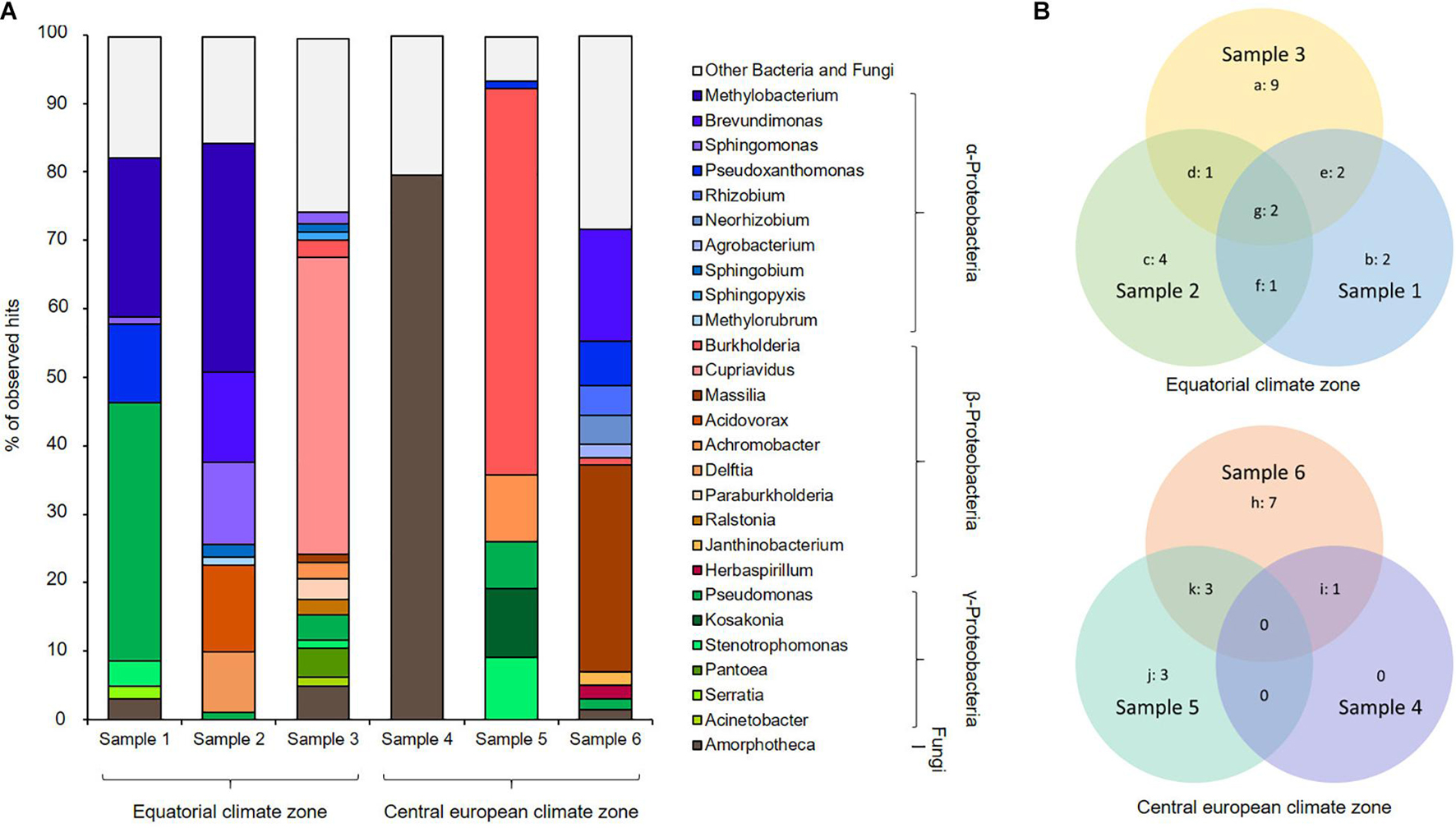
Figure 3. Phylogenetic profiling of fuel biofilm-derived gene sequences based on Kraken2 analysis. (A) Metagenome stacked bar chart Kraken2, cutoff 1%, (B) Venn diagram showing the number of bins affiliated with assumed organisms uniquely, and the overlap of organisms within particular relationships using the Kraken2 dataset; digits indicate number of organisms a: Cupriavidus, Acinetobacter, Burkholderia, Paraburkholderia, Sphingopyxis, Pantoea, Massilia, Ralstonia, Achromobacter, b: Serratia, Pseudoxanthomonas, c: Acidovorax, Delftia, Methylorubrum, Brevundimonas, d: Sphingobium, e: Amorphotheca, Stenotrophomonas, f: Methylobacterium, g: Pseudomonas, Sphingomonas, h: Agrobacterium, Herbaspirillum, Rhizobium, Janthinobacterium, Massilia, Neorhizobium, Brevundimonas, i: Amorphotheca, j: Kosakonia, Stenotrophomonas, Achromobacter, k: Pseudomonas, Burkholderia, Pseudoxanthomonas.
At least one of the samples, sample 4, appeared to be dominated by fungal sequences affiliated with Amorphoteca. For sample 1, we mainly identified Methylobacterium, Pseudoxanthomonas, and Pseudomonas; sample 2 was mainly composed of Methylobacterium, Brevundimonas, Sphingomonas, and Acidovorax. In fact, sample 3 contained primarily Cupriavidus but harbored the highest number of different microorganisms, namely, 14 various organisms, whereas the other metagenomes were composed of 1 to 11 different organisms. Sample 5 was dominated by Burkholderia but also showed proportions of Achromobacter, Kosakonia, and Stenotrophomonas, while we identified mainly Brevundimonas and Massilia for sample 6. Apart from sample 5, all metagenomes contained proportions of the filamentous fungus Amorphotheca.
A Venn diagram analysis of the Kraken2 dataset was performed to visualize the commonly harbored and individually distributed genera between the six assembled metagenomes (Figure 3B). Sample 1, sample 2, and sample 3 were collected in the equatorial climate zone and showed a core genome of the genera of Pseudomonas and Sphingomonas. The genera Amorphoteca and Stenotrophomonas were shared by sample 1 and sample 3 whereas Methylobacterium was detectable in sample 1 and sample 2 and Sphingobium in sample 2 and sample 3. The individual distribution of the microbial communities appeared even higher within the three metagenomes collected in the central European climate zone, which are sample 4, sample 5, and sample 6. No core microbiome could be described for the three samples from the central European climate zone. Sample 5 and sample 6 uniquely harbored the genera Pseudomonas, Burkholderia, and Pseudoxanthomonas whereas sample 4 and sample 6 shared DNA sequences from the fungal genus Amorphoteca.
Taken together, the Venn diagrams implied that the fuel based-pangenomes harbor a highly diverse gene set based on DNA similarity searches (Figure 3B). However, based on a functional analysis, the functional pangenome was relatively similar. The metabolic properties of the respective habitats did not differ on a larger scale (Tables 1, 2).
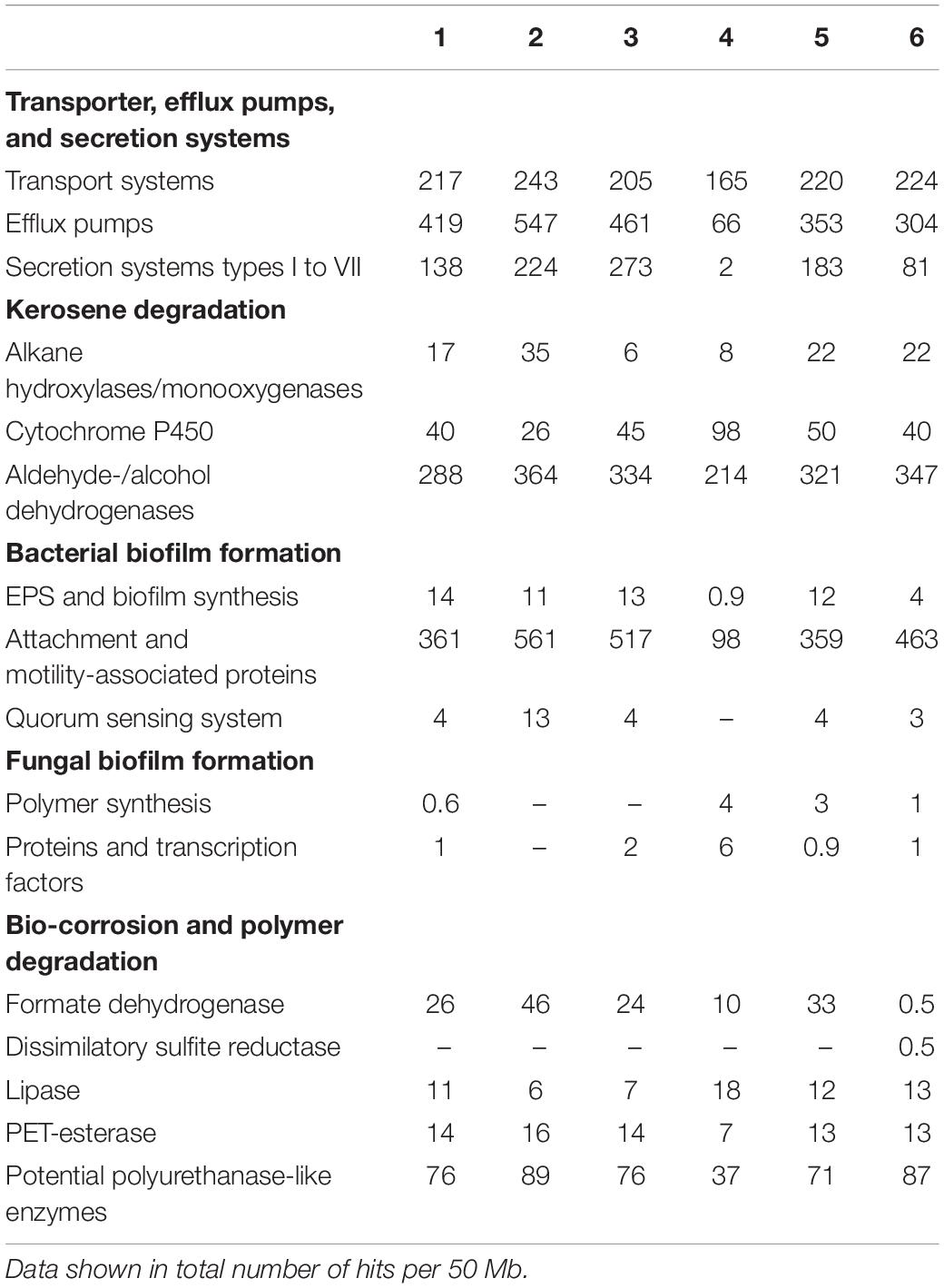
Table 1. Key features of microbial biofilm formation and bio-corrosion in kerosene environments of metagenome samples 1–6 using IMG function search.
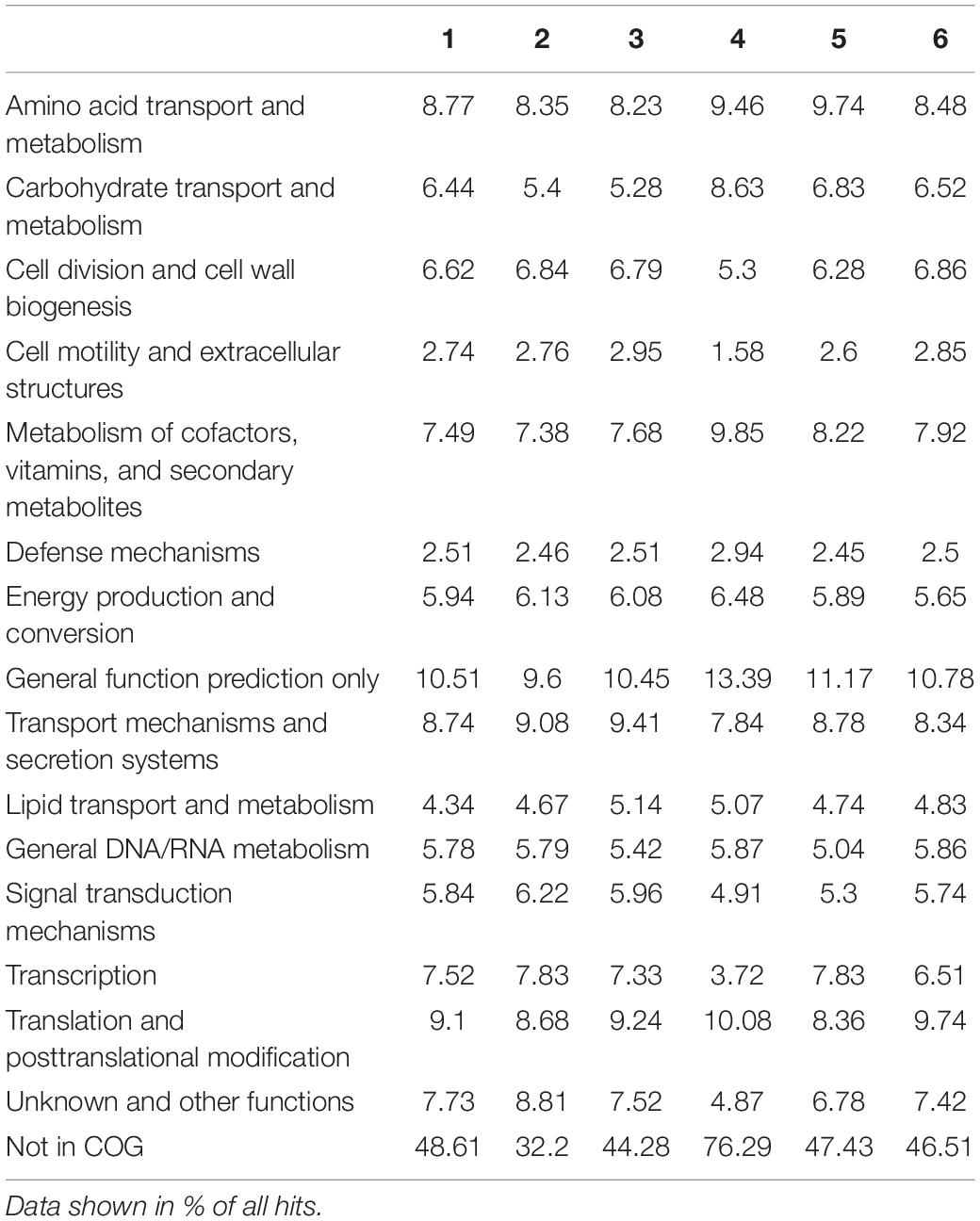
Table 2. Key features observed in the microbial metagenomes of samples 1–6 using COG-based analysis.
To validate the Kraken2-based phylogeny, an IMG phylogeny was generated based on the phylogenetic distribution of genes using gene count. Furthermore, a 16S rRNA analysis based on the IMG 16S rRNA genes from the metagenomic dataset was generated (Supplementary Table 3). Both additional phylogenies support the Kraken2 analysis. The IMG phylogenetic and IMG 16S rRNA analyses cover the most relevant genera found by Kraken2 up to the respective cutoffs.
16S rDNA Gene and 18S ITS-Spacer Amplicon Analyses of 1,258 Aircraft Swabs
Notably, the relative abundance of the bacteria and fungi cannot be estimated based on the above-described metagenome data sets. For this purpose, quantitative PCR (qPCR) is a better-suited and well-known technology (Sørensen et al., 2011; Leuchtle et al., 2015; Martin-Sanchez et al., 2016; de Azambuja et al., 2017; Radwan et al., 2018). Therefore, we analyzed the abundance of the overall copy number of bacteria and fungi by using specific primers for either the 16S rRNA genes or the 18 S ITS spacer regions. In detail, we used the primers bact_338f/518r and fungi_NL1f/LS2r, which amplify regions of the 16S rRNA and the ITS gene, respectively (O’Donnell, 1993; Ovreås et al., 1997; Cocolin et al., 2000; Ishii and Fukui, 2001). Thereby, it was possible to estimate and validate the bacterial and fungal abundance in various fuel (F), water (W), and biofilm samples (B). In total 1,258 samples (1,008 F-, 110 W-, 140 B-samples) were tested for the general presence of bacteria and fungi. Our analyses implied a thoroughly variable distribution of bacteria and fungi within the different sample types (Supplementary Figure 1). Recurring patterns are observable in bacterial gene copy numbers scaling up to 8,000 in F-samples, up to 80,000 in W-samples, and up to 140,000 in B-samples. Fungi are limited in F- and W-samples, with up to 100 and 22,000 gene copy numbers, respectively, but may be distinctly present in B-samples with up to 100,000 copy numbers in particular samples.
Altogether, these data confirm the relatively high microbial diversity in the different fuel samples. These analyses also confirmed that mainly bacteria affiliated with the genera of Methylobacterium, Pseudomonas, Sphingomonas, Pseudoxanthomonas, Brevundimonas, Achromobacter, Kosakonia, and Burkholderia were present and that fungi mostly belonged to Amorphotheca and members of the genera Aspergillus, Fusarium, and Penicillium.
Metabolic Potential of Microbial Communities
Based on the above made observations, we set out to analyze the metabolic potential of selected kerosene biofilms. Thereby, we analyzed the metagenomes of the above sequenced biofilms.
For the functional analyses of the metagenomes, we used the Pfam, COG, and KEGG databases. The general COG-based analysis highlighted the metabolism of amino acids, carbohydrates, and lipids (Table 2) as well as key features affiliated to transport of ions, cell motility, and extracellular structures, crucial functions for alkane degradation, biofilm formation, and most probably bio-corrosion (Table 1). Searching for genetically coded properties for microbial fuel degradation, biofilm formation, and bio-corrosion, the IMG function search pipeline identified a multitude of genes for alkane hydroxylases, as well as aldehyde- and alcohol dehydrogenases possibly involved in fuel degradation. For possible microbial biofilm formation, we identified a large number of Quorum sensing systems involving proteins, attachment pili and flagella-associated proteins, and genes, which are involved in the synthesis of EPS components. Mechanisms and genes linked to biofouling and corrosion are secreted hydrolases, mono- and di-oxygenases, lipases and various esterases, produced acids (propionate, succinate, acetate, formate, and butyrate), and cation and electron transporters (Table 1).
RNA-seq Identifies Active Genes
While metagenome-based studies only describe the genetic potential, no transcriptional studies are available on fuel-affiliated biofilms.
Therefore, and in the light of the above made analyses and observed results, we asked which genes were the most strongly transcribed ones in fuel biofilms. Because of technical reasons and the rather limited access to aircraft fuel tank access, it was, however, not possible to analyze a larger number of fuel biofilm transcriptomes. To obtain a comprehensive overview of all genes that could possibly play an important role in fuel biofilms, we analyzed the transcriptome of a single biofilm sample (Supplementary Table 4 and Figure 4). From this single sample, triplicates were analyzed by RNA-seq. The generated sequence data were mapped to the available six fuel metagenomes. In total, there are 112,137,168 reads mapped to bacteria and 130,184,802 mapped to fungi corresponding to the NCBI datasets. After the filtering of a mapping quality score ≥ 10, there are 36,214,506 reads affiliated with bacterial contigs, and 20,180,691 reads were affiliated with fungal contigs (Supplementary Table 4). The ribosomal proteins of bacteria and fungi are, of course, distinct from the ribosomal rRNAs depleted from (meta)-transcriptomic assays.
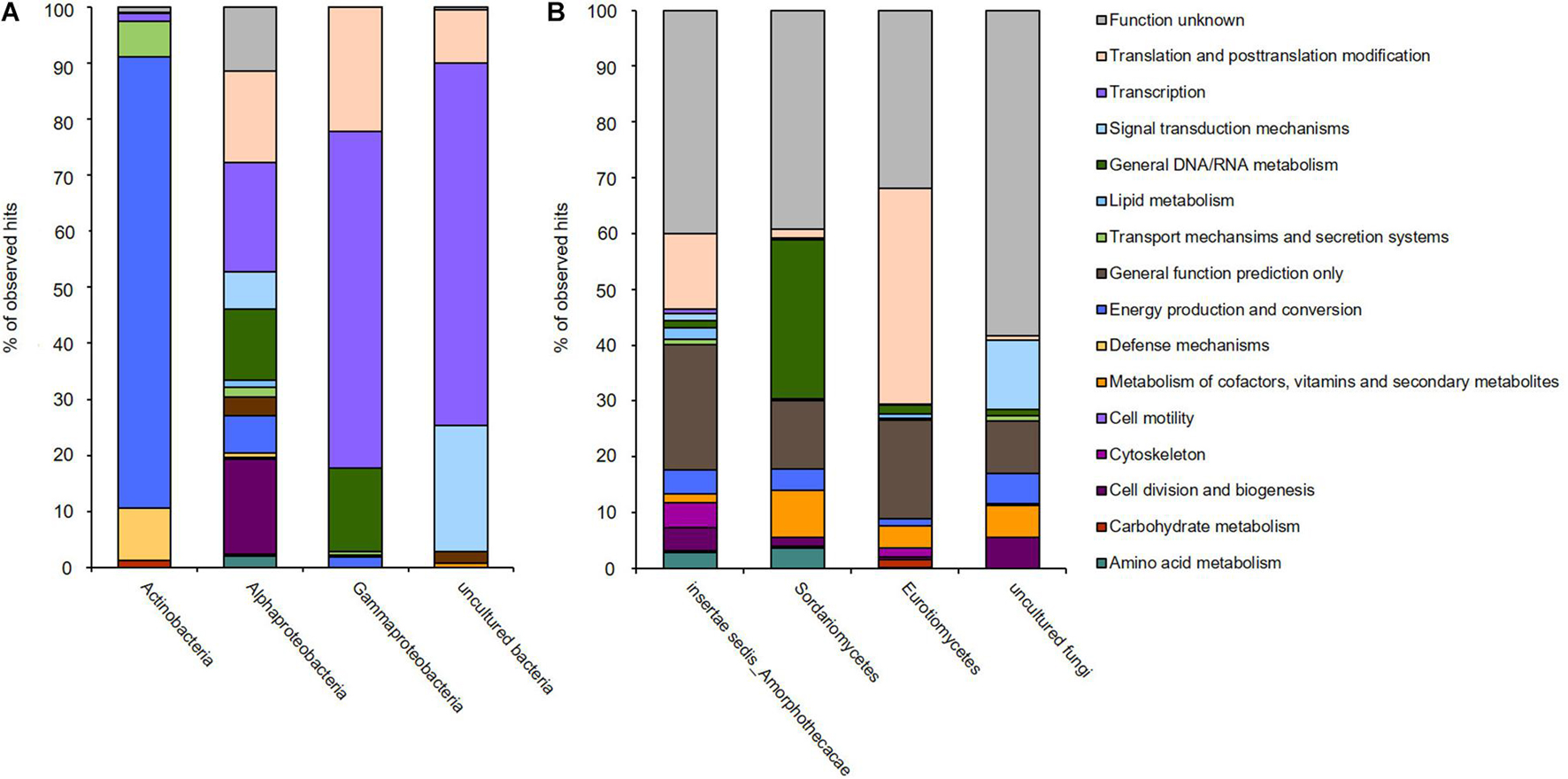
Figure 4. Transcribed microbiome and expressed genes of (A) bacterial and (B) fungal classes of one biofilm sample from a fuel-containing habitat. The generated sequence data (approximately 132 mio reads) were mapped to the available six metagenomes.
Since the above-analyzed microbial fuel biofilms contained a rather small number of different bacteria, the RNA-seq data covered a significant portion of overall metagenomes. Using these comprehensive data sets, we asked three main questions: (i) first, which are the most strongly expressed genes in fuel biofilms in general? (ii) second, what are the main metabolic routes in these biofilms? and (ii) third, which are the genes and enzymes possibly involved biofouling? (Supplementary Table 5 and Figure 5).
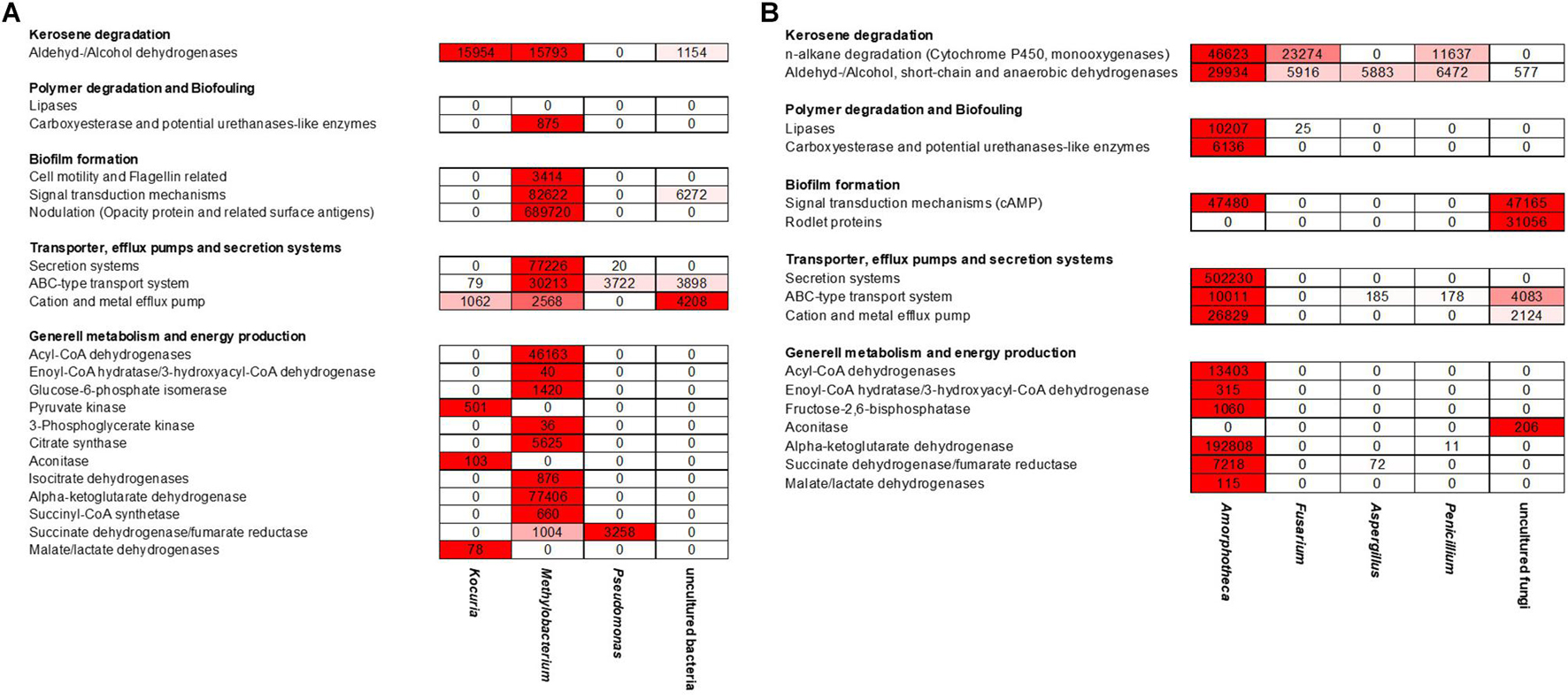
Figure 5. Heat map reflecting the expression of genes affiliated with kerosene and polymer degradation, biofilm formation, transport and secretion systems, and general metabolism and energy production mechanisms of the individual (A) bacterial and (B) fungi-related reads; color key: high expression level,
low expression level.
The transcriptionally most active bacterial species were Methylobacterium sp. (5,332,547 reads mapped), Pseudomonas sp. (176,762 reads mapped), and Kocuria sp. (37,444 reads mapped). For fungi, Amorphoteca sp. (11,826,916 reads mapped), Fusarium sp. (2,521,069 reads mapped), Penicillium sp. (962,343 reads mapped), and Aspergillus sp. (480,511 reads mapped) were most active on the level of transcription and a high number of reads for other and unknown bacteria and fungi were identified (2,067,530 reads mapped). To identify the most relevant and strongly expressed genes, we decided to focus on the top 250 expressed genes (excluding ribosomal proteins) in our analyses (Supplementary Table 5).
Most notably, however, was that the transcriptome dataset hinted toward a metabolic symbiosis between fungi and bacteria (Figure 5). Thus, in our analyses we identified various genes possibly affiliated with the degradation of C10–C18 carbons of kerosene (e.g., hydrolases and oxygenases) mainly by the fungi up to short-chain carbons, which can then be used in the metabolism of the bacteria. We observed a relatively high number of genes, which related to esterase/lipase activity and which are potentially relevant for long-chain fatty acid degradation. Our datasets determined the metabolic activity of the bacteria up to the citrate cycle. In addition, we identified many bacterial transporters and efflux pumps, which are important for cation/multidrug and metal metabolism. Furthermore, we are able to detect a high number of strongly transcribed genes, which encoded for various secretion systems and cation/multidrug and metal efflux pumps. In addition, we identified genes which are related to cell motility, flagellin synthesis, and adhesion to host structures.
A more detailed analysis of the Methylobacterium and Amorphotheca-affiliated transcriptome data identified a number of genes with potential relevance in the degradation of fuel components. For this, transcripts were mapped to the reference genomes of both organisms (Figure 6 and Supplementary Table 6). Expression levels (RPKM) were normalized against rpoD for bacteria and TFIIB genes for fungi, where a log2 fold value above 2.0 was understood as highly expressed (Supplementary Table 6) (Knutson and Hahn, 2013; Gelev et al., 2014; McMillan and Pereg, 2014; Krzyżanowska et al., 2019). In Figure 6, highly expressed genes are marked. Highlighted areas show gene clusters which are highly significant for the analyzed biofilm and habitat.
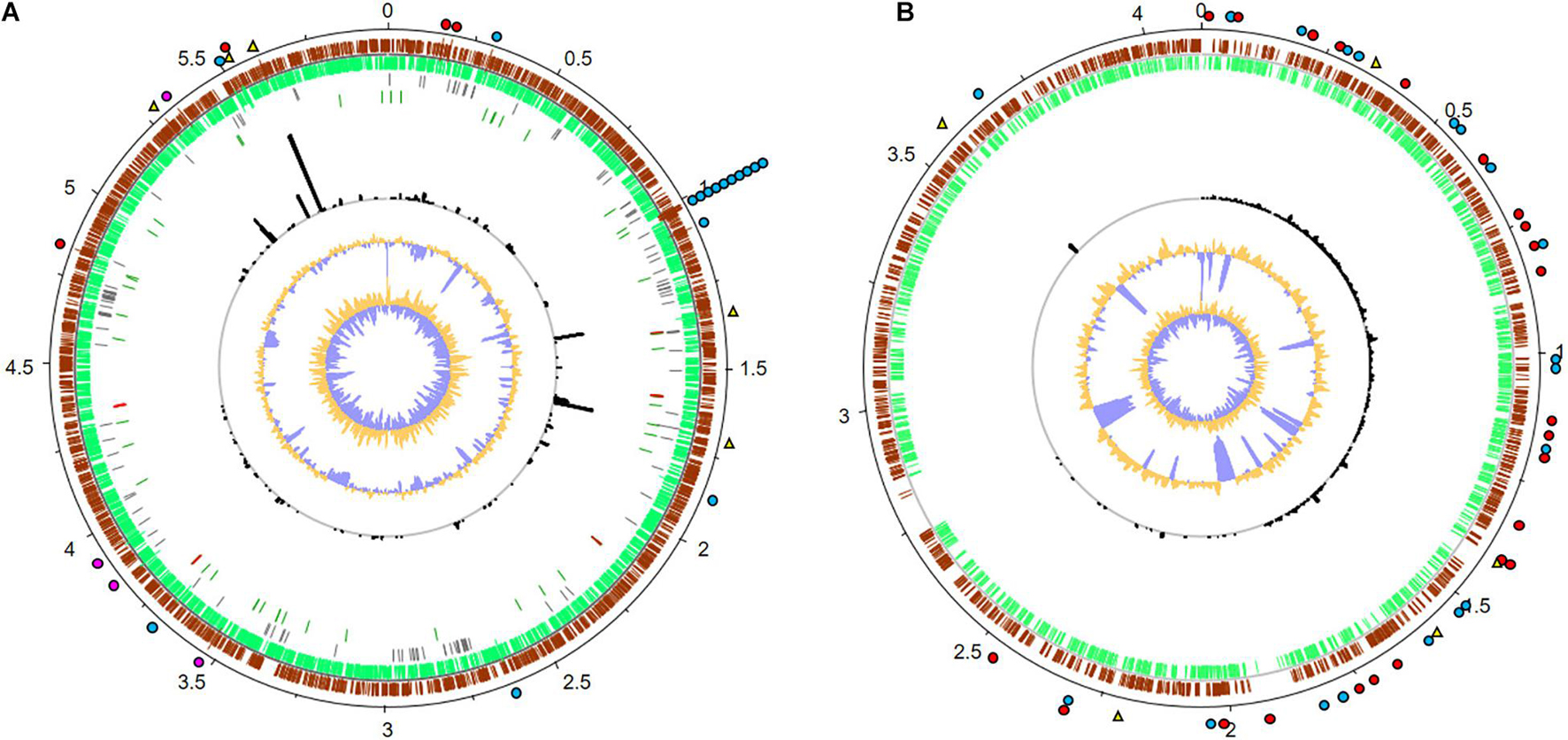
Figure 6. Metatranscriptome mapping to the reference genomes of (A) Methylobacterium brachiatum (GCF_003697185.1) and (B) Amorphotheca resinae (GCA_003019875.1). Numbers outside the circles show the genome coordinates in Mbp. Moving inward, the subsequent two rings show CDSs in forward (brown) and reverse (green) strands. Pseudogenes are displayed in gray; genes coding for tRNAs and rRNAs are marked in dark green and red, respectively (M. brachiatum only). Black bars indicate RPKM values for each CDS and are displayed in the same scale for (A,B). The last two inner plots indicate GC content and a GC skew [(GC)/(G + C)], where dark yellow and purple indicate values above and below average, respectively. The top 5 most expressed genes are denoted with a yellow triangle. Genes associated with kerosene degradation are marked with a red dot, potential mechanisms involved in polymer degradation with a blue dot, and genes for biofilm formation with a pink dot. For real RPKM values and further details, see Supplementary Table 6.
For Methylobacterium, the five most strongly expressed genes are genes which were 5-formyltetrahydrofolate cyclo-ligase, efflux transporter permeases, and hypothetical proteins. Further, we identified many transcriptionally active gene clusters (3,574 total mapped reads) coding for a multitude of oxidoreductases, dehydrogenases, and proteases (Figure 6). In addition, a remarkable number of genes are involved in hypothetical proteins. Notably, a large number of reads (5,947) mapped to genes code for aldehyde dehydrogenases and alkene reductases. Furthermore, a total number of 875 reads are involved in alginate biosynthesis and flagella-related proteins, which are relevant for biofilm formation (Supplementary Table 6).
Despite the poor level of annotation of the reference genome of Amorphotheca, a protein homolog with a proper annotation could be found for more than 80% of all the CDS. In the available dataset, the five most strongly transcribed expressed genes were marked as hypothetical proteins. In addition, a total of 15,441 RNA reads are mapped to potential aldehyde dehydrogenases and cytochrome P450-like enzymes. These are potentially involved in oxidative processes that could be linked to kerosene degradation. In addition, more than 4,322 reads are mapped to proteins annotated as carboxylesterase type II and putative lipases or esterases that could potentially be involved in hydrolysis of various ester bonds (Figure 6). In addition, and because of the relatively high number of nodulation and surface antigen-regulated genes (3,450 total reads mapped, Supplementary Table 6) which are linked to biosynthesis of biofilms and general metabolism, one can speculate that the biofilm lifestyle is of strong importance for growth and survival in kerosene- and fuel-containing habitats. This is a significant point for additional effects of polymer degradation and biofouling processes. These enzymes are significant for the analyzed habitat and for implementing surviving strategies of the organisms and a possible symbiotic interaction between fungi and bacteria.
In summary, these data give forth a hint on the overall metabolism of a fuel biofilm on a metagenome and transcriptome level.
Discussion
A comprehensive understanding of the composition of the microbial community, the grade of microbial contamination, and its functional properties is a requirement for controlling and reducing microbial proliferation in fuel-containing habitats.
In this study, we mainly used next-generation sequencing and qPCR strategies to analyze the fungi–bacteria interaction and physiological properties in more detail. With respect to our phylogenetic analysis and in addition to previous studies, our data imply that the main microbial contaminants were mainly affiliated with members of α-, β-, and γ-Proteobacteria and Ascomycota. In general, several microorganisms, including bacteria, filamentous fungi, and yeasts are able to degrade n-alkanes. Among these microorganisms, medium-length-chain n-alkane degraders have dehydrogenases, cytochrome P450, and monooxygenases (Whyte et al., 2002; Rojo, 2009; Das and Chandran, 2011; Jurelevicius et al., 2013). Many of the generated contigs of the metagenome datasets code for known mechanisms involved in the degradation of kerosene.
Our metagenome and transcriptome datasets support in part this hypothesis and indicated that in general very common processes are expressed. The most active bacteria observed within the fuel biofilms were Methylobacterium, Kocuria, and Pseudomonas as well as uncultured and unknown bacteria. Methylobacterium sp. are a strictly aerobic, facultatively methylotrophic, fastidious, slow-growing bacteria isolated from various environments (i.e., leaf surfaces, soil, dust, and fresh water) (Patt et al., 1976; Rice et al., 2000; Sanders et al., 2000; Kovaleva et al., 2014). Methylobacterium is a strong biofilm producer and exhibits tolerance to cleaning and disinfecting agents and to high temperatures (Simões et al., 2010; Yano et al., 2013; Beale et al., 2014). Due to its slow growth, the bacterium can be easily missed during culture-dependent screening. Kocuria is a genus of Gram-positive bacteria in the phylum Actinobacteria and belongs to the family Micrococcaceae. It grows in a wide range of ecological niches (Stackebrandt et al., 1995; Park et al., 2010). SEM images of biofilms formed by K. rhizophila are composed of amassed cocci and fibriform extracellular matrix of proteins and carbohydrate (Kavitha and Raghavan, 2018). Pseudomonas sp. is a ubiquitous Gram-negative bacterium, capable of adapting to versatile environments such as human tissues and environmental surfaces (Mesaros et al., 2007; Winsor et al., 2011; Percival et al., 2015). Members of the genus Pseudomonas are well-known biofilm-forming bacteria and naturally resistant to a wide range of antibiotics, making antibiotic and disinfection treatments ineffective (Drenkard and Ausubel, 2002; Sillankorva et al., 2008; Chang, 2018; Maurice et al., 2018).
With respect to the assumed bio-corrosion potential, we observed a rather low number of dissimilatory sulfite reductase (DsrA, DsrB) genes, which participate in the last step of dissimilatory sulfate reduction to generate sulfides, a mechanism that was shown to induce corrosion of iron and steel (King and Miller, 1971). Based on the above results, we hypothesize that microbia-influenced corrosion (MIC) by sulfate-reducing bacteria (SRB) are potentially not of high relevance in the analyzed samples.
Instead, we were able to detect a significant number of bacterial and fungal genes and transcripts, which are related to biofilm formation, cation and metal efflux pump transport, and acid production. Notably, heterogeneous microbial structures, primarily formed by aerobic and facultative anaerobic microorganisms, lead to creation of oxygen gradients due to oxygen/nutrient consumption and pH gradient formation (Picioreanu and van Loosdrecht, 2002). The different concentrations of dissolved oxygen lead to cathodic and anodic areas where, e.g., metal is oxidized to ions in anodic areas and dissolved oxygen is reduced in cathodic areas (Vargas et al., 2017). Thereby, EPS binds free metal ions, which further stimulates the anodic dissolution of metal (Beech and Sunner, 2004). Overall, an increasing production of EPS in biofilms leads to destruction of protective oxide films in technical systems, thereby promoting corrosion (Chen and Zhang, 2018). Within this framework, it is notable that formate dehydrogenase further contributes to corrosion. The transmembrane domain of the beta subunit consists of a single-transmembrane helix. This domain acts as a transmembrane anchor, allowing the conduction of electrons within the protein. In combination with biofilm formation, an induction of corrosion via direct metal–microbe electron transfer could be a major point for MIC (Jormakka et al., 2002; Beech and Sunner, 2004; Deutzmann et al., 2015; Tang et al., 2019).
While current corrosion research has mainly focused on the tank or fouling of the fuel, scarce information is yet available on the corrosion of the coating used to protect the tank material. These protective paintings are many polyurethane (PUR)-based polymers (Seymour and Kauffman, 1992). Our data imply that many enzymes especially lipases and other hydrolases may act on these protective paintings and contribute to the corrosion. Thereby, it is well known that lipase/esterase-like (including carboxylesterase) and polyurethanase-like enzymes are able to attack and decompose various polyester compounds including coatings and sealant material (Russell et al., 2011; Danso et al., 2018, 2019; Kawai et al., 2019).
The most prominent fungal representative in these selected fuel samples was Amorphotheca. Members of the genera Aspergillus, Fusarium, and Penicillium as well as other and unknown fungi were also confirmed to be active fungal contaminants (Darby et al., 2001). Amorphotheca, widely known by the anamorph name Hormoconis resinae (Lindau) Arx & G.A. de Vries or its obligate synonym Cladosporium resinae (Lindau) G.A. de Vries, grows in hydrocarbon-rich substrates such as jet fuel, cosmetics, and wood preserved with creosote or coal tar (Parbery, 1969; Seifert et al., 2007).
Many metabolites produced by fungi, which include formic, citric, and acetic acids, are damaging to metals, glass, masonry, and other materials and therefore contribute to corrosion (Little et al., 2001; Gu, 2003; de Leo et al., 2013; Flemming, 2020). The organic acids produced by fungal species may contribute significantly to microbiologically influenced corrosion. Bio-corrosion of aluminum and its alloys has been attributed to contamination of jet fuels caused by the fungi Amorphotheca resinae, Aspergillus fumigatus, Penicillium corylophilum, and Fusarium oxysporum (Teh and Lee, 1973). Amorphotheca resinae utilizes the hydrocarbons of fuel to produce organic acids; the large quantities of organic compounds excreted by this fungus are capable of causing bio-corrosion of storage tanks and transporting pipelines (Flemming and Geesey, 1995). Other fungi detected that may contribute to bio-corrosion of oil pipelines, blockage of pipes, valves, and filters, and incorrect reading of fuel probes include A. fumigatus which is involved in iron reduction from ferric state (Fe3+) to ferrous (Fe2+) state. Aspergillus terreus produces aspartic acid, a product of general metabolism, which at high concentrations will contribute to bio-corrosion of oil pipelines (Haas, 2003). Each fungus presents a different pattern of biofilm development, spore adhesion, and monolayer and EPS production (de Siqueira, 2015), which may be involved in several functions, including adhesion to host proteins and cells, dispersion by air currents, and protection against chemicals, enzymes, and phagocytic cells (Beauvais et al., 2007; Mowat et al., 2009; Siqueira and Lima, 2012).
Within this framework, our findings imply that at least some of the triggers and signals involved in the microbial interaction with fungi and bacteria are already of relevance in industrially and biotechnologically used systems. In summary, the current study gives a detailed insight into the metagenomes and partial transcriptome of fuel microbial communities. Future work will now have to unravel the detailed interaction between the bacteria and eukaryotes in different aspects of biofouling.
Data Availability Statement
Raw sequence data have been submitted to the European Nucleotide Archive. They are publicly available under accession PRJEB40662. Assemblies of the six fungi bacteria metagenomes are available via IMG/MER (https://img.jgi.doe.gov) using the IMG IDs: 3300039030, 3300039916, 3300038809, 3300039918, 3300038808, and 3300039917.
Author Contributions
IK, LB, and YH contributed to experimental design; lab work of phylogenetic, metagenomic, and transcriptomic approaches; and writing of the research article. MQ, MA, and PP-G contributed to assembly of metagenomic and transcriptomic datasets and bioinformatic approaches. DI contributed to lab work on metagenomic and phylogenetic approaches. EK contributed to lab work of transcriptomic approaches. BH, TL, CS and RR contributed to the delivery of sample material for analysis and lab work for sonification processes. WS contributed to general experimental design and writing of the research article. All authors contributed to manuscript revision, read, and approved the submitted version.
Funding
This work was in part supported by the Universit t Hamburg (project AimM; https://www.biologie.unihamburg.de/forschung/mikrobiologie-undinfektionsbiologie/mikrobiobiotech/2images/aimmvs9-final-web.pdf) and the Bundesministerium f r Wirtschaft (project 20Y1514; https://www.bmbf.de/bmbf/de/home/home_node.html).
Conflict of Interest
BH, TL, CS, and RR were employed by company Lufthansa Technik AG HAM.
The remaining authors declare that the research was conducted in the absence of any commercial or financial relationships that could be construed as a potential conflict of interest.
Publisher’s Note
All claims expressed in this article are solely those of the authors and do not necessarily represent those of their affiliated organizations, or those of the publisher, the editors and the reviewers. Any product that may be evaluated in this article, or claim that may be made by its manufacturer, is not guaranteed or endorsed by the publisher.
Acknowledgments
The authors would like to thank the CAFT initiative Contamination of Aircraft Fuel Tanks coordinated by Lufthansa Technik AG, Hamburg, Germany for cooperation and intense discussions from suitable sampling variants and influencing factors via the analysis steps up to technical issues in aviation systems and materials. This enables also the involvement and consideration of the very broad range of liquid and biofilm sample sets sourced from a global operational profile.
Supplementary Material
The Supplementary Material for this article can be found online at: https://www.frontiersin.org/articles/10.3389/fmicb.2021.722259/full#supplementary-material
Supplementary Figure 1 | Gene copy-number quantification, primer 338/518 for bacteria and NL1/LS2 for fungi, minimum cutoff 1000 copies. color key: copy number/ng DNA for bacteria,
copy number/ng DNA for fungi, (A) for selected fuel samples (F_01-F_14), (B) for different water samples (W_01-W_22), (C) for various biofilm samples (B_01-B_28).
Supplementary Table 1 | Sample collection of the six samples for the metagenome analysis, the transcriptome analysis and the used 1258 samples for the qPCR analysis. Samples were collected and processed as described in section “Materials and Methods.” Samples were collected and transported to the laboratory and kept at 4° until processing.
Supplementary Table 2 | Overall numbers of sequences and contigs generated for microbial communities of sample 1–6 and IMG metagenome statistic.
Supplementary Table 3 | Phylogenetic profiling_Comparison between Kranken2, IMG Phylogeny and 16S rRNA Hits analysis.
Supplementary Table 4 | Overall numbers of sequences and contigs generated for the transcriptome.
Supplementary Table 5 | Top 250 expressed genes in the biofilm sample.
Supplementary Table 6 | Transcriptome map representing the genome-wide RNA-seq data for Methylobacterium (GCF_003697185.1) and Amorphotheca (GCA_003019875.1).
Footnotes
References
Anders, S., Pyl, P. T., and Huber, W. (2015). HTSeq–a Python framework to work with high-throughput sequencing data. Bioinformatics 31, 166–169. doi: 10.1093/bioinformatics/btu638
Andrews, S. (2010). FASTQC. A quality control tool for high throughput sequence data. Available online at: https://www.bioinformatics.babraham.ac.uk/projects/fastqc/ (accessed October 21, 2019).
Anwar, M. Z., Lanzen, A., Bang-Andreasen, T., and Jacobsen, C. S. (2019). To assemble or not to resemble—a validated comparative metatranscriptomics workflow (CoMW). GigaScience 8:giz096.
Beale, D. J., Morrison, P. D., Key, C., and Palombo, E. A. (2014). Metabolic profiling of biofilm bacteria known to cause microbial influenced corrosion. Water Sci. Technol. 69, 1–8. doi: 10.2166/wst.2013.425
Beauvais, A., Schmidt, C., Guadagnini, S., Roux, P., Perret, E., Henry, C., et al. (2007). An extracellular matrix glues together the aerial-grown hyphae of Aspergillus fumigatus. Cell Microbiol. 9, 1588–1600. doi: 10.1111/j.1462-5822.2007.00895.x
Beech, I. B., and Sunner, J. (2004). Biocorrosion: towards understanding interactions between biofilms and metals. Curr. Opin. Biotechnol. 15, 181–186. doi: 10.1016/j.copbio.2004.05.001
Bolger, A. M., Lohse, M., and Usadel, B. (2014). Trimmomatic: a flexible trimmer for Illumina sequence data. Bioinformatics 30, 2114–2120. doi: 10.1093/bioinformatics/btu170
Brown, L. M., McComb, J. P., Vangsness, M. D., Bowen, L. L., Mueller, S. S., Balster, L. M., et al. (2010). Community dynamics and phylogenetics of bacteria fouling Jet A and JP-8 aviation fuel. Int. Biodeterior. Biodegradation. 64, 253–261. doi: 10.1016/j.ibiod.2010.01.008
Buchfink, B., Xie, C., and Huson, D. H. (2015). Fast and sensitive protein alignment using DIAMOND. Nat. Methods 12, 59–60. doi: 10.1038/nmeth.3176
Camacho, C., Coulouris, G., Avagyan, V., Ma, N., Papadopoulos, J., Bealer, K., et al. (2009). BLAST+: architecture and applications. BMC Bioinformatics 10:421. doi: 10.1186/1471-2105-10-421
Carver, T., Harris, S. R., Berriman, M., Parkhill, J., and McQuillan, J. A. (2012). Artemis: an integrated platform for visualization and analysis of high-throughput sequence-based experimental data. Bioinformatics 28, 464–469. doi: 10.1093/bioinformatics/btr703
Carver, T., Thomson, N., Bleasby, A., Berriman, M., and Parkhill, J. (2009). DNAPlotter: circular and linear interactive genome visualization. Bioinformatics 25, 119–120. doi: 10.1093/bioinformatics/btn578
Chang, C. Y. (2018). Surface Sensing for Biofilm Formation in Pseudomonas aeruginosa. Front. Microbiol. 8:2671–2671.
Chen, I. A., Chu, K., Palaniappan, K., Pillay, M., Ratner, A., Huang, J., et al. (2019). IMG/M v.5.0: an integrated data management and comparative analysis system for microbial genomes and microbiomes. Nucleic Acids Res. 47, D666–D677.
Chen, S., and Zhang, D. (2018). Study of corrosion behavior of copper in 3.5 wt.% NaCl solution containing extracellular polymeric substances of an aerotolerant sulphate-reducing bacteria. Corros Sci. 136, 275–284. doi: 10.1016/j.corsci.2018.03.017
Chen, S., Zhou, Y., Chen, Y., and Gu, J. (2018). fastp: an ultra-fast all-in-one FASTQ preprocessor. Bioinformatics 34, i884–i890.
Clemente, A. R., Anazawa, T. A., and Durrant, L. R. (2001). Biodegradation of polycyclic aromatic hydrocarbons by soil fungi. Braz. J. Microbiol. 32, 255–261. doi: 10.1590/s1517-83822001000400001
Cocolin, L., Bisson, L. F., and Mills, D. A. (2000). Direct profiling of the yeast dynamics in wine fermentations. FEMS Microbiol. Lett. 189, 81–87. doi: 10.1111/j.1574-6968.2000.tb09210.x
Danso, D., Chow, J., and Streit, W. R. (2019). Plastics: environmental and biotechnological perspectives on microbial degradation. Appl. Environ. Microbiol. 85, e1095–e1019.
Danso, D., Schmeisser, C., Chow, J., Zimmermann, W., Wei, R., Leggewie, C., et al. (2018). New insights into the function and global distribution of polyethylene terephthalate (PET)-degrading bacteria and enzymes in marine and terrestrial metagenomes. Appl. Environ. Microbiol. 2018:84.
Darby, R., Simmons, E., and Wiley, B. (2001). A survey of fungi in a military aircraft fuel supply system (Reprinted). Int. Biodeterior. Biodegr. 48, 159–161.
Das, N., and Chandran, P. (2011). Microbial degradation of petroleum hydrocarbon contaminants: an overview. Biotechnol. Res. Int. 2011:941810.
de Azambuja, A. O., Bücker, F., de Quadros, P. D., Zhalnina, K., Dias, R., Vacaro, B. B., et al. (2017). Microbial community composition in Brazilian stored diesel fuel of varying sulfur content, using high-throughput sequencing. Fuel 189, 340–349. doi: 10.1016/j.fuel.2016.10.108
de Leo, F., Campanella, G., Proverbio, E., and Urzì, C. (2013). Laboratory tests of fungal biocorrosion of unbonded lubricated post-tensioned tendons. Constr. Build Mater. 49, 821–827. doi: 10.1016/j.conbuildmat.2013.08.071
de Siqueira, V. M. (2015). “Fungal biofilms: an overview,” in Fungal Biomolecules: Sources, Applications and Recent Developments, eds V. K. Gupta, R. L. Mach, and S. Sreenivasaprasad (Hoboken: Wiley-Blackwell), 1–10. doi: 10.1002/9781118958308.ch1
Deutzmann, J. S., Sahin, M., and Spormann, A. M. (2015). Extracellular Enzymes Facilitate Electron Uptake in Biocorrosion and Bioelectrosynthesis. mBio 6, e496–e415.
Drenkard, E., and Ausubel, F. M. (2002). Pseudomonas biofilm formation and antibiotic resistance are linked to phenotypic variation. Nature 416, 740–743. doi: 10.1038/416740a
Flemming, H. C. (2020). Biofouling and me: My Stockholm syndrome with biofilms. Water Res. 173:115576. doi: 10.1016/j.watres.2020.115576
Flemming, H., and Geesey, G. G. (1995). Biofouling and biocorrosion. Chem. Ing. Tech. 67, 1425–1430.
Gallardo-Becerra, L., Cornejo-Granados, F., García-López, R., Valdez-Lara, A., Bikel, S., Canizales-Quinteros, S., et al. (2020). Metatranscriptomic analysis to define the Secrebiome, and 16S rRNA profiling of the gut microbiome in obesity and metabolic syndrome of Mexican children. Microb. Cell Fact. 19, 1–18. doi: 10.1007/978-3-319-12125-3_26-1
Gaylarde, C. C., and Beech, I. B. (1989). “Bacterial polysaccharidesand corrosion. Biocorrosion,” eds. C. C. Gaylarde, L. H. G. Morton (Kew, Surrey: The Biodeterioration Society), 85–98.
Gaylarde, C. C., Bento, F. M., and Kelley, J. (1999). Microbial contamination of stored hydrocarbon fuels and its control. Rev. Microbiol. 30, 01–10. doi: 10.1590/s0001-37141999000100001
Gelev, V., Zabolotny, J. M., Lange, M., (2014). A new paradigm for transcription factor TFIIB functionality. Sci. Rep. 4:3664.
Gu, J. D. (2003). Microbiological deterioration and degradation of synthetic polymeric materials: recent research advances. Int. Biodeterior. Biodegradation 52, 69–91. doi: 10.1016/s0964-8305(02)00177-4
Gu, J. D., Roman, M., Esselman, T., and Mitchell, R. (1998). The role of microbial biofilms in deterioration of space station candidate materials. Int. Biodeterior. Biodegr. 41, 25–33. doi: 10.1016/s0964-8305(97)00070-x
Haas, H. (2003). Molecular genetics of fungal siderophore biosynthesis and uptake: the role of siderophores in iron uptake and storage. Appl. Microbiol. Biotechnol. 62, 316–330. doi: 10.1007/s00253-003-1335-2
Hendey, N. I. (1964). Some observations on Cladosporium resinae as a fuel contaminant and its possible role in the corrosion of aluminium alloy fuel tanks. Mycol. Res. 47, 467–IN461.
Ii, R. C. B., Norton, W. N., and Howard, G. T. (1998). Adherence and growth of a Bacillus species on an insoluble polyester polyurethane. Int. Biodeterior. Biodegr. 42, 63–73. doi: 10.1016/s0964-8305(98)00048-1
Ishii, K., and Fukui, M. (2001). Optimization of annealing temperature to reduce bias caused by a primer mismatch in multitemplate PCR. Appl. Environ. Microbiol. 67, 3753–3755. doi: 10.1128/aem.67.8.3753-3755.2001
Jormakka, M., Törnroth, S., Byrne, B., and Iwata, S. (2002). Molecular basis of proton motive force generation: structure of formate dehydrogenase-N. Science 295, 1863–1868. doi: 10.1126/science.1068186
Jurelevicius, D., Alvarez, V. M., Peixoto, R., Rosado, A. S., and Seldin, L. (2013). The use of a combination of alkB primers to better characterize the distribution of alkane-degrading bacteria. PLoS One 8:e66565. doi: 10.1371/journal.pone.0066565
Kang, D. D., Li, F., Kirton, E., Thomas, A., Egan, R., An, H., et al. (2019). MetaBAT 2: an adaptive binning algorithm for robust and efficient genome reconstruction from metagenome assemblies. PeerJ 7:e7359. doi: 10.7717/peerj.7359
Kavitha, S., and Raghavan, V. (2018). Isolation and characterization of marine biofilm forming bacteria from a ship’s hull. Front. Biol. 13:208–214. doi: 10.1007/s11515-018-1496-0
Kawai, F., Kawabata, T., and Oda, M. (2019). Current knowledge on enzymatic PET degradation and its possible application to waste stream management and other fields. Appl. Microbiol. Biotechnol. 103, 4253–4268. doi: 10.1007/s00253-019-09717-y
Khan, S. R., Nirmal Kumar, J. I., Nirmal Kumar, R., and Patel, J. (2015). Enzymatic evaluation during biodegradation of kerosene and diesel by locally isolated fungi from petroleum-contaminated soils of western india. Internat. J. 24, 514–525.
Kim, D., Pertea, G., Trapnell, C., Pimentel, H., Kelley, R., and Salzberg, S. L. (2013). TopHat2: accurate alignment of transcriptomes in the presence of insertions, deletions and gene fusions. Genome Biol. 14, R36.
King, R. A., and Miller, J. D. (1971). Corrosion by the sulphate-reducing bacteria. Nature 233, 491–492.
Knutson, B. A., and Hahn, S. (2013). TFIIB-related factors in RNA polymerase I transcription. Biochim. Biophys. Acta 1829, 265–273. doi: 10.1016/j.bbagrm.2012.08.003
Kovaleva, J., Degener, J. E., and van der Mei, H. C. (2014). Methylobacterium and its role in health care-associated infection. J. Clin. Microbiol. 52, 1317–1321. doi: 10.1128/jcm.03561-13
Krohn-Molt, I., Alawi, M., and Förstner, K. U. (2017). Insights into microalga and bacteria interactions of selected phycosphere biofilms using metagenomic, transcriptomic, and proteomic approaches. Front. Microbiol. 2017:8.
Krohn-Molt, I., Wemheuer, B., and Alawi, M. (2013). Metagenome survey of a multispecies and alga-associated biofilm revealed key elements of bacterial-algal interactions in photobioreactors. Appl. Environ. Microbiol. 79, 6196–6206. doi: 10.1128/aem.01641-13
Krzyżanowska, D. M., Supernat, A., Maciąg, T., Matuszewska, M., and Jafra, S. (2019). Selection of reference genes for measuring the expression of aiiO in Ochrobactrum quorumnocens A44 using RT-qPCR. Sci. Rep. 9:13129.
Langmead, B., Wilks, C., Antonescu, V., and Charles, R. (2019). Scaling read aligners to hundreds of threads on general-purpose processors. Bioinformatics 35, 421–432. doi: 10.1093/bioinformatics/bty648
Leuchtle, B., Xie, W., Zambanini, T., Eiden, S., Koch, W., Lucka, K., et al. (2015). Critical factors for microbial contamination of domestic heating oil. Energy Fuels 29, 6394–6403. doi: 10.1021/acs.energyfuels.5b01023
Li, H. (2013). Aligning sequence reads, clone sequences and assembly contigs with BWA-MEM. arXiv preprint arXiv 2013, 13033997.
Li, X. X., Yang, T., Mbadinga, S. M., Liu, J. F., Yang, S.-Z., Gu, J. D., et al. (2017). Responses of microbial community composition to temperature gradient and carbon steel corrosion in production water of petroleum reservoir. Front. Microbiol. 8:2379.
Little, B., Staehle, R., and Davis, R. (2001). Fungal influenced corrosion of post-tensioned cables. Int. Biodeterior. Biodegr. 47, 71–77. doi: 10.1016/s0964-8305(01)00039-7
Lu, J., and Salzberg, S. L. (2020). Ultrafast and accurate 16S rRNA microbial community analysis using Kraken 2. Microbiome 8:124.
Martin-Sanchez, P. M., Gorbushina, A. A., and Toepel, J. (2018). Quantification of microbial load in diesel storage tanks using culture- and qPCR-based approaches. Int. Biodeterior. Biodegr. 126, 216–223. doi: 10.1016/j.ibiod.2016.04.009
Martin-Sanchez, P. M., Gorbushina, A. A., Kunte, H. J., and Toepel, J. (2016). A novel qPCR protocol for the specific detection and quantification of the fuel-deteriorating fungus Hormoconis resinae. Biofouling 32, 635–644. doi: 10.1080/08927014.2016.1177515
Maurice, N. M., Bedi, B., and Sadikot, R. T. (2018). Pseudomonas aeruginosa Biofilms: Host response and clinical implications in lung infections. Am. J. Respir. Cell Mol. Bio 58, 428–439.
McMillan, M., and Pereg, L. (2014). Evaluation of reference genes for gene expression analysis using quantitative RT-PCR in Azospirillum brasilense. PLoS One 9:e98162. doi: 10.1371/journal.pone.0098162
Mesaros, N., Nordmann, P., Plésiat, P., et al. (2007). Pseudomonas aeruginosa: resistance and therapeutic options at the turn of the new millennium. Clin. Microbiol. Infect. 13, 560–578. doi: 10.1111/j.1469-0691.2007.01681.x
Morton, L., and Surman, S. (1994). Biofilms in biodeterioration—a review. Int. Biodeterior. Biodegr. 34, 203–221. doi: 10.1016/0964-8305(94)90083-3
Mowat, E., Williams, C., Jones, B., McChlery, S., and Ramage, G. (2009). The characteristics of Aspergillus fumigatus mycetoma development: is this a biofilm? Med. Mycol. 47(Suppl. 1), S120–S126.
Mukherjee, S., Stamatis, D., Bertsch, J., Ovchinnikova, G., Katta, H. Y., Mojica, A., et al. (2019). Genomes OnLine database (GOLD) v.7: updates and new features. Nucleic Acids Res. 47, D649–D659.
Nurk, S., Meleshko, D., Korobeynikov, A., and Pevzner, P. A. (2017). metaSPAdes: a new versatile metagenomic assembler. Genome Res. 27, 824–834. doi: 10.1101/gr.213959.116
Ovreås, L., Forney, L., Daae, F. L., and Torsvik, V. (1997). Distribution of bacterioplankton in meromictic Lake Saelenvannet, as determined by denaturing gradient gel electrophoresis of PCR-amplified gene fragments coding for 16S rRNA. Appl. Environ. Microbiol. 63, 3367–3373. doi: 10.1128/aem.63.9.3367-3373.1997
Parbery, D. (1969). Amorphotheca resinae, gen. nov., sp. nov.: The perfect state of Cladosporium resinae. Austr. J. Bot. 17, 331–357. doi: 10.1071/bt9690331
Park, E. J., Kim, M. S., Roh, S. W., Jung, M. J., and Bae, J. W. (2010). Kocuria atrinae sp. nov., isolated from traditional Korean fermented seafood. Int. J. Syst. Evol. Microbiol. 60, 914–918. doi: 10.1099/ijs.0.014506-0
Passman, F. J. (2013). Microbial contamination and its control in fuels and fuel systems since 1980 – a review. Int. Biodeterior. Biodegr. 81, 88–104. doi: 10.1016/j.ibiod.2012.08.002
Patt, T. E., Cole, G. C., and Hanson, R. S. (1976). Methylobacterium, a new genus of facultatively methylotrophic bacteria. Int. J. Syst. Evol. Microbiol. 26, 226–229. doi: 10.1099/00207713-26-2-226
Percival, S. L., Suleman, L., Vuotto, C., and Donelli, G. (2015). Healthcare-associated infections, medical devices and biofilms: risk, tolerance and control. J. Med. Microbiol. 64, 323–334. doi: 10.1099/jmm.0.000032
Picioreanu, C., and van Loosdrecht, M. C. (2002). A mathematical model for initiation of microbiologically influenced corrosion by differential aeration. J. Electrochem. Soc. 149:B211.
Powell, S., Szklarczyk, D., Trachana, K., Roth, A., Kuhn, M., Muller, J., et al. (2012). eggNOG v3. 0: orthologous groups covering 1133 organisms at 41 different taxonomic ranges. Nucleic Acids Res. 40, D284–D289.
Radwan, O., Gunasekera, T. S., and Ruiz, O. N. (2018). Robust multiplex quantitative polymerase chain reaction assay for universal detection of microorganisms in fuel. Energy Fuels 32, 10530–10539. doi: 10.1021/acs.energyfuels.8b02292
Rauch, M. E., Graef, H. W., Rozenzhak, S. M., Jones, S. E., Bleckmann, C. A., Kruger, R. L., et al. (2006). Characterization of microbial contamination in United States Air Force aviation fuel tanks. J Ind Microbiol. Biotechnol. 33, 29–36. doi: 10.1007/s10295-005-0023-x
Rice, E. W., Reasoner, D. J., Johnson, C. H., and DeMaria, L. A. (2000). Monitoring for methylobacteria in water systems. J. Clin. Microbiol. 38, 4296–4297. doi: 10.1128/jcm.38.11.4296-4297.2000
Rojo, F. (2009). Degradation of alkanes by bacteria. Environ. Microbiol. 11, 2477–2490. doi: 10.1111/j.1462-2920.2009.01948.x
Russell, J. R., Huang, J., Anand, P., Kucera, K., Sandoval, A. G., Dantzler, K. W., et al. (2011). Biodegradation of polyester polyurethane by endophytic fungi. Appl. Environ. Microbiol. 77, 6076–6084. doi: 10.1128/aem.00521-11
Sanders, J. W., Martin, J. W., Hooke, M., and Hooke, J. (2000). Methylobacterium mesophilicum Infection: Case Report and Literature Review of an Unusual Opportunistic Pathogen. Clin. Infect. Dis. 30, 936–938. doi: 10.1086/313815
Saratale, G., Kalme, S., Bhosale, S., and Govindwar, S. (2007). Biodegradation of kerosene by Aspergillus ochraceus NCIM-1146. J. Basic Microbiol. 47, 400–405. doi: 10.1002/jobm.200710337
Seifert, K. A., Hughes, S. J., Boulay, H., and Louis-Seize, G. (2007). Taxonomy, nomenclature and phylogeny of three cladosporium-like hyphomycetes, Sorocybe resinae, Seifertia azaleae and the Hormoconis anamorph of Amorphotheca resinae. Stud. Mycol. 58, 235–245. doi: 10.3114/sim.2007.58.09
Seymour, R. B., and Kauffman, G. B. (1992). Polyurethanes: A class of modern versatile materials. J. Chem. Educ. 69:909.
Sillankorva, S., Neubauer, P., and Azeredo, J. (2008). Pseudomonas fluorescens biofilms subjected to phage phiIBB-PF7A. BMC Biotechnol. 8, 79–79. doi: 10.1186/1472-6750-8-79
Simões, L. C., Simões, M., and Vieira, M. J. (2010). Adhesion and biofilm formation on polystyrene by drinking water-isolated bacteria. Antonie van Leeuwenhoek 98, 317–329. doi: 10.1007/s10482-010-9444-2
Siqueira, V., and Lima, N. (2012). Surface Hydrophobicity of Culture and Water Biofilm of Penicillium spp. Curr. Microbiol. 64, 93–99. doi: 10.1007/s00284-011-0037-8
Sørensen, G., Pedersen, D. V., Nørgaard, A. K., Sørensen, K. B., and Nygaard, S. D. (2011). Microbial growth studies in biodiesel blends. Bioresour. Technol. 102, 5259–5264. doi: 10.1016/j.biortech.2011.02.017
Stackebrandt, E., Koch, C., Gvozdiak, O., and Schumann, P. (1995). Taxonomic dissection of the genus Micrococcus: Kocuria gen. nov., Nesterenkonia gen. nov., Kytococcus gen. nov., Dermacoccus gen. nov., and Micrococcus Cohn 1872 gen. emend. Int. J. Syst. Bacteriol. 45, 682–692. doi: 10.1099/00207713-45-4-682
Stern, R. V., and Howard, G. T. (2000). The polyester polyurethanase gene (pueA) from Pseudomonas chlororaphis encodes a lipase. FEMS Microbiol. Lett. 185, 163–168. doi: 10.1111/j.1574-6968.2000.tb09056.x
Tang, H. Y., Holmes, D. E., Ueki, T., Palacios, P. A., and Lovley, D. R. (2019). Iron corrosion via direct metal-microbe electron transfer. mBio 10, e00303–e00319.
Teh, J., and Lee, K. (1973). Utilization of n-alkanes by Cladosporium resinae. Appl. Microbiol. 25, 454–457. doi: 10.1128/aem.25.3.454-457.1973
Thorp, K. E., Crasto, A. S., Gu, J.-D., and Mitchell, R. (1997). Contribution of microorganisms to corrosion. Richardson, TX: OnePetro.
Vargas, I. T., Fischer, D. A., Alsina, M. A., Pavissich, J. P., Pastén, P. A., and Pizarro, G. E. (2017). Copper corrosion and biocorrosion events in premise plumbing. Materials 10:1036. doi: 10.3390/ma10091036
Whyte, L. G., Schultz, A., Beilen, J. B., Luz, A. P., Pellizari, V., Labbé, D., et al. (2002). Prevalence of alkane monooxygenase genes in Arctic and Antarctic hydrocarbon-contaminated and pristine soils. FEMS Microbiol. Ecol. 41, 141–150. doi: 10.1016/s0168-6496(02)00282-9
Wilke, A., Harrison, T., Wilkening, J., Field, D., Glass, E. M., Kyrpides, N., et al. (2012). The M5nr: a novel non-redundant database containing protein sequences and annotations from multiple sources and associated tools. BMC bioinformatics 13:141. doi: 10.1186/1471-2105-13-141
Winsor, G. L., Lam, D. K., Fleming, L., Lo, R., Whiteside, M. D., Yu, N. Y., et al. (2011). Pseudomonas Genome Database: improved comparative analysis and population genomics capability for Pseudomonas genomes. Nucleic Acids Res. 39, D596–D600.
Wood, D. E., and Salzberg, S. L. (2014). Kraken: ultrafast metagenomic sequence classification using exact alignments. Genome Biol. 15:R46.
Yano, T., Kubota, H., Hanai, J., Hitomi, J., and Tokuda, H. (2013). Stress tolerance of Methylobacterium biofilms in bathrooms. Microbes Environ. 28, 87–95. doi: 10.1264/jsme2.me12146
Keywords: fuel contamination, biofilm formation, biocorrosion, biofouling, bacteria and fungi, omics analysis, kerosene and aircraft
Citation: Krohn I, Bergmann L, Qi M, Indenbirken D, Han Y, Perez-Garcia P, Katzowitsch E, Hägele B, Lübcke T, Siry C, Riemann R, Alawi M and Streit WR (2021) Deep (Meta)genomics and (Meta)transcriptome Analyses of Fungal and Bacteria Consortia From Aircraft Tanks and Kerosene Identify Key Genes in Fuel and Tank Corrosion. Front. Microbiol. 12:722259. doi: 10.3389/fmicb.2021.722259
Received: 08 June 2021; Accepted: 19 August 2021;
Published: 04 October 2021.
Edited by:
Dimitris G. Hatzinikolaou, National and Kapodistrian University of Athens, GreeceReviewed by:
Ashraf Mohammad Fahmi Al Ashhab, Dead Sea and Arava Science Center, IsraelHesham Ali El Enshasy, University of Technology Malaysia, Malaysia
Copyright © 2021 Krohn, Bergmann, Qi, Indenbirken, Han, Perez-Garcia, Katzowitsch, Hägele, Lübcke, Siry, Riemann, Alawi and Streit. This is an open-access article distributed under the terms of the Creative Commons Attribution License (CC BY). The use, distribution or reproduction in other forums is permitted, provided the original author(s) and the copyright owner(s) are credited and that the original publication in this journal is cited, in accordance with accepted academic practice. No use, distribution or reproduction is permitted which does not comply with these terms.
*Correspondence: Ines Krohn, ines.krohn@uni-hamburg.de