- 1Transversal Activities in Applied Genomics, Sciensano, Brussels, Belgium
- 2IDLab, Department of Information Technology, Ghent University - IMEC, Ghent, Belgium
- 3Department of Plant Biotechnology and Bioinformatics, Ghent University, Ghent, Belgium
- 4National Reference Laboratory for Foodborne Outbreaks (NRL-FBO) and for Coagulase Positive Staphylococci (NRL-CPS), Foodborne Pathogens, Sciensano, Brussels, Belgium
- 5Organic Contaminants and Additives, Sciensano, Brussels, Belgium
- 6Department of Genetics, University of Pretoria, Pretoria, South Africa
Through staphylococcal enterotoxin (SE) production, Staphylococcus aureus is a common cause of food poisoning. Detection of staphylococcal food poisoning (SFP) is mostly performed using immunoassays, which, however, only detect five of 27 SEs described to date. Polymerase chain reactions are, therefore, frequently used in complement to identify a bigger arsenal of SE at the gene level (se) but are labor-intensive. Complete se profiling of isolates from different sources, i.e., food and human cases, is, however, important to provide an indication of their potential link within foodborne outbreak investigation. In addition to complete se gene profiling, relatedness between isolates is determined with more certainty using pulsed-field gel electrophoresis, Staphylococcus protein A gene typing and other methods, but these are shown to lack resolution. We evaluated how whole genome sequencing (WGS) can offer a solution to these shortcomings. By WGS analysis of a selection of S. aureus isolates, including some belonging to a confirmed foodborne outbreak, its added value as the ultimate multiplexing method was demonstrated. In contrast to PCR-based se gene detection for which primers are sometimes shown to be non-specific, WGS enabled complete se gene profiling with high performance, provided that a database containing reference sequences for all se genes was constructed and employed. The custom compiled database and applied parameters were made publicly available in an online user-friendly interface. As an all-in-one approach with high resolution, WGS additionally allowed inferring correct isolate relationships. The different DNA extraction kits that were tested affected neither se gene profiling nor relatedness determination, which is interesting for data sharing during SFP outbreak investigation. Although confirming the production of enterotoxins remains important for SFP investigation, we delivered a proof-of-concept that WGS is a valid alternative and/or complementary tool for outbreak investigation.
Introduction
Through the production of staphylococcal enterotoxins (SEs), Staphylococcus aureus is globally one of the most common causative agents responsible for food poisoning outbreaks [European Food Safety Authority (EFSA) and European Centre for Disease Prevention and Control (ECDC), 2021]. Detection of staphylococcal food poisoning (SFP) is, therefore, mainly based on the detection of SEs in food leftovers, the enumeration of >105 S. aureus CFU/g food, and the isolation of S. aureus from the poisoned food source and affected human cases (Hennekinne et al., 2010).
For SE detection, commercial kits using immunological methods have been developed, such as the automated VIDAS® immunoanalyzer or the Staphylococcal Enterotoxin Reversed Passive Latex Agglutination (SET-RPLA) kit, and are widely used by National Reference Laboratories (NRLs) and Centers, often under standardized ISO 19020:2017 environments (Hennekinne et al., 2010). However, these kits only allow detection of at most five SEs (the classical five SE: SEA to SEE), whereas an arsenal of 27 different enterotoxins is described to date (Merda et al., 2020). Moreover, due to insertion, deletion, duplication, and recombination events in their encoding genes, new SEs [such as SELV and SELU arising from fusion between sem and sei (Thomas et al., 2006) and Ψent1 and Ψent2 pseudogenes (Letertre et al., 2003b; Thomas et al., 2006), respectively] and SE variants (such as SEC1–4, SECbovine, SECovine, SELU2, and others) are continuously generated. Many of the described SEs are determined to exhibit emetic properties [in alphabetical order: SEA to SEE, SEG to SEI, SEK to SET, and SEY (Spaulding et al., 2013; Johler et al., 2015; Wilson et al., 2018; Zhang et al., 2018; Aung et al., 2020)]. Moreover, it is most likely that multiple of these toxins are produced during SFP and play a (contributing) role in the provoked illness (Umeda et al., 2017; Fisher et al., 2018). Therefore, it is important to detect all toxins involved, not only during SFP outbreak investigation, but also during SFP surveillance, to assess the possible risk of strains isolated from food matrices in view of illness prevention. Because the development of new immunological assays remains difficult (Fisher et al., 2018), molecular methods, such as polymerase chain reactions (PCRs) are frequently used to detect SE encoding genes (se) (Fisher et al., 2018). Although no information on the eventual production of the toxin is obtained, detection at the genotypic level demonstrates the potential for toxin production. In response, many PCR primer pairs have been developed detecting the different se genes (for references, see Supplementary Table 1). However, the fact that multiple primer sets per se gene are described across the literature and not all are exhaustively investigated for their specificity impedes the selection of the most appropriate primers for routine applications. Indeed, the high sequence similarity between se (pseudo)genes and mutations in the primer binding sites of se variants increases the risk of false positives and negatives, respectively. Moreover, despite efforts in developing multiplex PCR assays targeting multiple se genes per assay (Becker et al., 1998; Schmitz et al., 1998; Monday and Bohach, 1999; Mehrotra et al., 2000; Sharma et al., 2000; Letertre et al., 2003a; Shylaja et al., 2010; Nagaraj et al., 2014), detecting the full arsenal of se genes currently described (from now on referred to as “complete se gene profiling”) remains a labor-intensive and time-consuming process.
Complete se gene profiling can, however, also provide an initial indication on the potential link between the contaminated food source and the human case(s) (Denayer et al., 2017). Yet, because food sources are sometimes contaminated with several S. aureus strains, the level of isolate discrimination based on the se gene profile might not be sufficient. Indeed, accurate epidemiological typing methods are a prerequisite to distinguish between isolated strains and aiding in identifying the causal strain for SFP outbreaks. For this purpose, pulsed-field gel electrophoresis (PFGE), multiple-locus variable number of tandem repeats analysis (MLVA), and phage typing are still regarded as the gold standard to study isolate relatedness (Blair and Williams, 1961; Wildemauwe et al., 2004; Roussel et al., 2015). However, these methods have shown some issues with interpretation and/or interlaboratory comparison of obtained profiles (Cookson et al., 1996; Murchan et al., 2003; Price et al., 2013). Therefore, DNA sequence-based methods, such as Staphylococcus protein A gene (spa) typing and multilocus sequence typing (MLST) are frequently applied as well because their analysis is less ambiguous. Also for MLVA, DNA sequencing of alleles has been used to increase the initially limited transferability of obtained data by confirming the deduced number of repeats (Schouls et al., 2009). However, all these conventional methods are recently shown to struggle in distinguishing closely related S. aureus isolates (Moore et al., 2015; Roussel et al., 2015; Cunningham et al., 2017; Cremers et al., 2020).
Because whole genome sequencing (WGS) analyzes the entire genome of bacteria, it enables complete genotypic characterization of isolates. Thanks to its unparalleled resolution standing out compared with conventional methods (Anderson et al., 2014; Moore et al., 2015; Cunningham et al., 2017; Cremers et al., 2020), it has moreover become the ultimate tool for inferring phylogenetic relationships between bacterial isolates, using core genome MLST (cgMLST) or single nucleotide polymorphism (SNP) analysis. Although WGS was already used to analyze Methicillin-resistant S. aureus strains responsible for nosocomial infections (Anderson et al., 2014; Moore et al., 2015; Peacock and Paterson, 2015; Cunningham et al., 2017; Sabat et al., 2017), it has been scarcely applied for SFP investigation despite the advantages highlighted above (Merda et al., 2020; Schwendimann et al., 2021).
For WGS to be applied in the analysis of S. aureus isolates for se gene profiling, it is important to have a DNA sample representative for the isolate so that WGS data are comparable between (inter)national laboratories. Data sharing is indeed indispensable in the scope of outbreak investigations. For S. aureus, this means that the extracted genome must contain DNA of mobile genetic elements, such as plasmids, as they often harbor se genes (Argudín et al., 2010). Some frequently used, commercial DNA extraction kits have, however, been described as having decreased plasmid extraction performances (Becker et al., 2016; Nouws et al., 2020a), which can potentially impact complete se gene profiling. The successful integration of WGS-based se gene profiling is, additionally, hindered by the lack of comprehensive and/or freely and easily accessible databases. Indeed, commonly used databases for virulence gene detection in S. aureus do not contain or correctly annotate the full arsenal of se genes currently described, are not publicly available through an easily accessible online resource, and/or are not always linked to a user-friendly interface to query WGS data for se genes (Joensen et al., 2015; Liu et al., 2019; Sayers et al., 2019; Merda et al., 2020).
This study aimed to assess whether WGS would have an added value for application in the investigation of SFP outbreaks. For this purpose, WGS was used for se gene detection with a custom database [based on the frequently used Virulence Factor Database (VFDB)] of in-house sequenced isolates, complemented with publicly available WGS data. The determined se profiles were compared with those previously obtained with conventional methods supplemented with in silico PCR. Different DNA extraction kits were moreover compared for their appropriateness to use for WGS-based se gene profiling. The performance of WGS in S. aureus isolate relatedness determination and the potential influence of using different DNA extraction kits on this analysis was evaluated by comparison with a priori known relationships.
Materials and Methods
Selected Isolates and Their Characteristics
Criteria for Isolate Selection
To evaluate the performance of WGS in determining se gene profiles, isolates (Table 1) were selected to cover a repertory as extensive as possible of different se genes. For each of the enterotoxins (genes) whose presence was assessed previously, an in-house available isolate was included, i.e., sea to see, seg to selj, sep, and ser as determined by the multiplex PCR of the European Union Reference Laboratory for Coagulase Positive Staphylococci (EURL-CPS) (Roussel et al., 2015) at genotypic level and/or SEA to SEE as determined by the immunoassays SET-RPLA (detection and separate typing of SEA to SED) and VIDAS (detection of SEA to SEE) analyses at the phenotypic level. Isolates that were part of an SFP outbreak [(Denayer et al., 2017), i.e., outbreak A], previously confirmed based on PFGE profiles, were also included to allow investigating if WGS has an added value in resolving the SFP outbreak as proof of concept. All isolates were provided by the Belgian NRL for Foodborne Outbreaks (NRL-FBO) and Coagulase Positive Staphylococci (NRL-CPS), from which two isolates were initially received from the EURL-CPS as reference strains, i.e., TIAC3971 (S-6) and TIAC3972 (FRI-362).
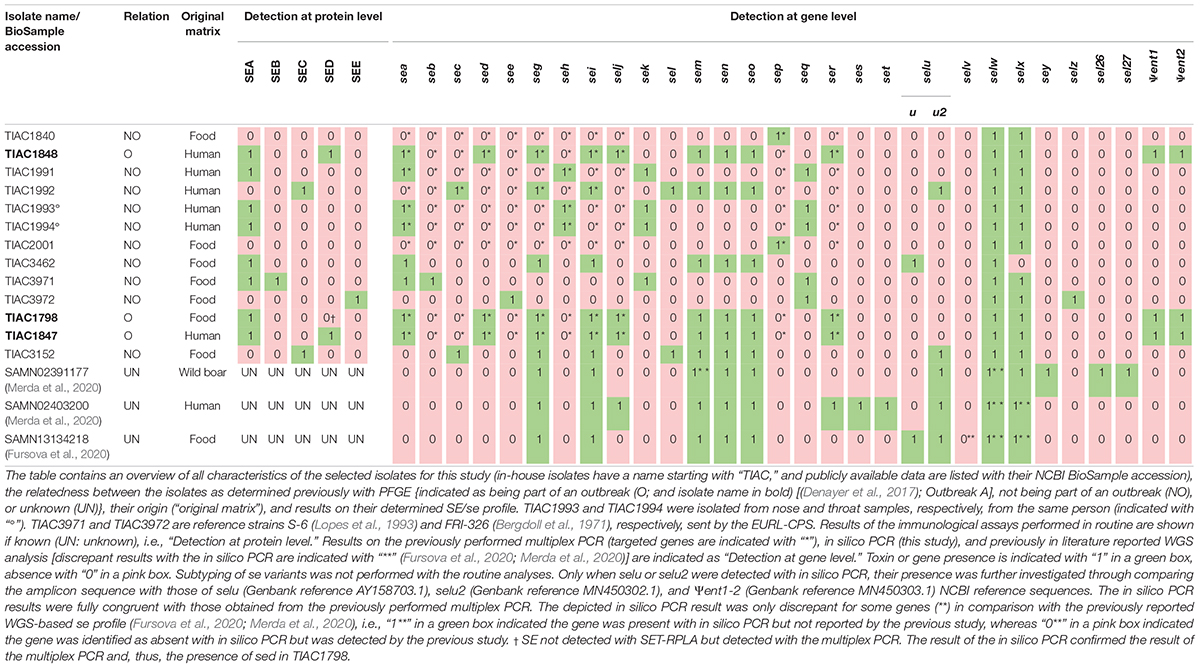
Table 1. Characteristics of the selected in-house isolates and isolates for which WGS data were publicly available.
For se genes not covered within the selected isolates, public WGS raw reads and/or assemblies of S. aureus isolates were incorporated (Table 1) to cover the complete arsenal of se genes. Accession numbers of the pubic WGS data employed in this study are provided in Supplementary Table 2.
Ethics Approval
This study includes S. aureus strains isolated from human feces or swabs from human cases and food matrices. Besides the origin of the strain, no human data were applied in this study, and thus, no ethical approval or written informed consent was required.
Determining the Complete se Gene Profile
Because no information concerning the presence/absence of se/SE other than those tested with the EURL-CPS multiplex PCR and/or SET-RPLA/VIDAS commercial kits was initially available (i.e., limited to a maximum of 11 out of the 27 se/SE), in silico PCR was performed to obtain the complete se gene profile of each isolate. The same was done for the isolates for which publicly available WGS data were used as a confirmation of the reported se genes in the corresponding publications [(Fursova et al., 2020; Merda et al., 2020); see Table 1 and Supplementary Table 2]. For this in silico PCR, the literature was searched to collect all reported conventional PCR primer sets (n: 155) for detection of 27 se genes and two pseudogenes (Supplementary Table 1).
Each primer pair described in the literature was checked for its specificity to the target gene (i.e., no aspecific detection of other staphylococcal genes with similar amplicon size) and, if not yet known, its amplicon size, by aligning the primer pairs against the NCBI nucleotide collection (nt) for S. aureus (taxid: 1280) using the Primer-BLAST tool of NCBI (Ye et al., 2012) through its online resource1 (see Supplementary Data). Besides the criteria previously described (Vanneste et al., 2018), default parameters were applied that assessed whether a primer pair allowed the formation of an in silico amplicon.
Based on the investigated specificity of all primer sets, 89.7% of the complete list of collected primers were used for the in silico PCR. For this purpose, these 139 primer pairs were aligned against the assemblies of the 13 in-house sequenced S. aureus isolates (see Supplementary Data) processed with the GenElute Bacterial gDNA kit and the downloaded assemblies of the three isolates for which WGS data were publicly available, using Primer-BLAST. A gene was considered present when at least one of the gene-specific primer pairs yielded an in silico product with correct amplicon size according to the preset criteria. Only when a gene was not detected by any of the primer sets was it considered absent.
Because conventional PCR does not enable discriminating between selu, its variant selu2, and the pseudogenes Ψent1 and Ψent2 due to high-sequence similarity (Heymans et al., 2010; Liang et al., 2016), their detection is generally followed by DNA Sanger sequencing for confirmation (Collery and Smyth, 2007). Therefore, when the primer pairs indicated the presence of selu, selu2, or Ψent1-2, in silico PCR was repeated using a primer pair [forward: 5′– TGA TAA TTA GTT TTA ACA CTA AAA TGC G-3′; reverse: 5′– CGT CTA ATT GCC ACG TTA TAT CAG T-3′; (Letertre et al., 2003b)] targeting the complete gene length. The amplicon sequence was extracted and aligned against those of NCBI sequences (see Supplementary Figure 1) harboring selu (Genbank reference AY158703.1), selu2 (Genbank reference MN450302.1), and Ψent1-2 (Genbank reference MN450303.1) using CLC Sequence Viewer 8.0 to type the specific gene based on similarity with the reference sequences.
DNA Preparation and Quality Control
All 13 in-house sequenced isolates were preserved in a glycerol-brain heart infusion (BHI) broth stock (40.0%) at –80°C until analysis. A loopful of each stock was grown overnight (16 h at 37°C) on nutrient agar plates, and a single colony was then inoculated in 10 ml of BHI and grown overnight with shaking at 37°C and 200 rpm.
DNA extraction was performed on all 13 cultures and a blank sample (BHI incubated overnight) using the GenElute Bacterial Genomic DNA (gDNA) kit (Sigma-Aldrich, Missouri, United States) according to the manufacturer’s protocol for Gram positive bacteria. Three of the 13 isolates (TIAC1798, TIAC1847, and TIAC3152) selected based on previously determined presence of se/SE exclusively located/encoded on plasmids [i.e., sed, selj, ser, ses, and set (Bayles and Iandolo, 1989; Zhang et al., 1998; Omoe et al., 2003; Ono et al., 2008)] and on mutual relationships (i.e., two outbreak isolates and one non-outbreak isolate) were also prepared using the DNeasy Blood & Tissue kit (Qiagen, Hilden, Germany) and the Wizard gDNA Purification kit (Promega, Wisconsin, United States) (Table 2). These kits were selected based on their frequent use for WGS of S. aureus isolates (Gordon et al., 2014; Lee et al., 2015; Ekkelenkamp et al., 2018) and recommendations by leading institutes [EURL-CPS; (Merda et al., 2020)] in the field, respectively. The kits were used according to the manufacturer’s instructions and proposed protocols (Merda et al., 2020), respectively. Moreover, the GenElute Bacterial gDNA kit was tested on the same three isolates according to the specific protocol for Staphylococcal species, which requires the use of the expensive lysostaphin (Sigma-Aldrich, Missouri, United States) during bacterial cell lysis.
DNA concentration, purity, and integrity were determined with, respectively, the dsDNA HS and BR assay kits for the Qubit 4 fluorometer (Thermo Fisher Scientific, Schwerte, Germany), NanoDrop 2000 spectrophotometer (Thermo Fisher Scientific, Schwerte, Germany), and the Genomic DNA ScreenTape and Reagent kits for TapeStation 4200 electrophoresis (Agilent Technologies, Santa Clara, CA), according to the manufacturer’s recommendations.
Library Preparation and Sequencing
One nanogram (in 5 μl) of each DNA extract was used for Nextera XT library preparation (Illumina, San Diego, CA). All libraries were sequenced on a MiSeq instrument (Illumina, San Diego, CA) using the MiSeq V3 chemistry to produce 2 × 250 bp paired-end reads, aiming at a theoretical sequencing depth of 60-fold.
Whole Genome Sequencing Data Analysis
All bioinformatic analyses were performed using the respective tools on an in-house instance of Galaxy (Afgan et al., 2018). An online user-friendly Galaxy interface to perform raw read trimming, assembly and se gene detection (among other functionalities) using the bioinformatic methods and criteria as described in this manuscript is publicly available upon registration.2
Read Trimming and Assembly
The raw reads were trimmed using Trimmomatic 0.38 (Bolger et al., 2014) by removing Nextera XT adaptors and other Illumina-specific sequences (“Illuminaclip” set to value “NexteraPE-PE.fa:2:30:10”), removing low-quality residues at the start and end of the reads (“leading:10” and “trailing:10”), clipping reads when average Q-scores dropped below 20 over a sliding window of four residues (“slidingwindow:4:20”), and dropping reads shorter than 40 bases after processing (“minlen:40”). Trimmed reads were de novo assembled using SPAdes 3.13.0 (Bankevich et al., 2012) setting the “–careful” and “–cov-cutoff 10” options to reduce mismatches and short indels and remove low coverage contigs, respectively. Based on advice from a technical report by the ECDC, contigs below 1000 bp in length were removed using Seqtk seq 1.23 using the “-L” option to improve assembly quality (ECDC, 2019). Relevant assembly statistics (N50, number of contigs, and median coverage against assembly) were calculated with Quast 4.4 (Gurevich et al., 2013) and are shown in Supplementary Table 3.
Database for se Gene Detection
The different publicly available databases for virulence gene detection in S. aureus, i.e., the Victors database (Sayers et al., 2019), VirulenceFinder database (Joensen et al., 2014), and VFDB_Full (Liu et al., 2019), were checked for the presence of se gene reference sequences. The VFDB_Full was selected because it was the most complete in containing reference sequences for the 27 se genes and was retrieved from its respective source4 on November 16, 2020. Some sequences were manually removed from and/or (re)added to the extended VFDB_Full database (i.e., sep, ses, set, selw, selx, sey, selz, sel26, and sel27; see Supplementary Data). Variants of sec (not separately annotated in the original VFDB_Full database) were also added so that variant subtyping of sec and selu (separately annotated in the original VFDB_Full database) was enabled. More information on sequences and accession numbers kept within (from the original VFDB_Full) and newly added to the extended VFDB_Full database is provided in Supplementary Table 4. The Supplementary Data also contains a FASTA file with sequences for all se genes present in the extended VFDB_Full database. Database sequences were clustered with an 85.0% sequence identity cutoff using the “cd-hit-est” function from CD-HIT 4.6.8 (Li and Godzik, 2006) to limit the detection of genes to one per cluster. This clustered database is also integrated in the online user-friendly interface for se gene detection.
Detection of se Genes Using the Extended Virulence Factor Database_Full Database
All samples were genotypically characterized for the presence of se genes, using two methods: (i) Aligning assemblies with BLAST + 2.6.0 (Camacho et al., 2009) and (ii) mapping trimmed reads with SRST2 0.2.0 (Inouye et al., 2014) with the options “–max-divergence 10,” “–min-coverage 60,” “–gene-max-mismatch 10,” and “–max-unaligned-overlap 150,” both against the extended VFDB_Full database. For the isolate with BioSample accession SAMN13134218, only the assembly was publicly available, limiting se gene detection to the assembly based approach using BLAST+ (i.e., no gene detection with SRST2 could be performed). Hits identified with <60.0% query coverage and/or >10.0% sequence divergence for SRST2 or with <60.0% query coverage and/or <90.0% sequence identity for BLAST+ were omitted. The best hit for each detected database cluster for BLAST+ was determined based on a previously described allele scoring method (Larsen et al., 2012). In the case of unexpected results, manual contig alignment against the corresponding reference gene using BLAST (Altschul et al., 1990) was performed to identify assembly artifacts. A gene was considered present with WGS when it could be detected with SRST2 and/or BLAST+.
Phylogenetic Analysis of the S. aureus Isolates Using Core Genome Multilocus Sequence Typing-Typing
In silico cgMLST-typing was performed as previously described (Bogaerts et al., 2021) by aligning assemblies using BLAST+ against the S. aureus cgMLST scheme of PubMLST (Jolley et al., 2018) containing 2208 loci (downloaded on January 3, 2021). Only exact allele calls (i.e., requiring a full-length, 100.0% identical match) were accepted. For tree construction, loci called in <80.0% of samples were stripped from the allele call matrix. A minimum spanning tree based on the allele call matrix was created using GrapeTree 1.5.0 (Zhou et al., 2018) with the “method” option set to “MSTreeV2′,” and afterward visualized using FigTree 1.4.4 (Rambaut, 2007).
Identifying the Completeness of se Gene Profiling and Evaluating the Performance of Whole Genome Sequencing in Inferring Phylogenetic Relationships
To evaluate if WGS can identify the complete se gene profile of S. aureus isolates, the se gene profiles determined with SRST2 and BLAST+ against the extended VFDB_Full database were compared with the expected profiles obtained using in silico PCR. From this comparison, WGS-based se gene detection results were classified as either true positives (TPs), false positives (FPs), true negatives (TNs), or false negatives (FNs), for which definitions are shown in Supplementary Table 5.
Moreover, phylogenetic relationships inferred from the cgMLST profiles determined with WGS were compared to the a priori known relationships to evaluate the additional benefit of WGS in SFP investigation as a proof of concept.
The potential influences of the tested DNA extraction kits and protocols on both se gene detection and inferring phylogenetic relationships were assessed similarly.
Results
Selected Isolates and Their Characteristics
To identify the benefits of WGS in SFP investigation, 13 in-house sequenced isolates (Table 1) were selected based on a priori known relationships to include outbreak (n: 3) and non-outbreak (n: 10) isolates and on the previously assessed presence of se and/or SE (i.e., sea to see, seg to selj, sep, and ser, and/or SEA to SEE). The selection of isolates included isolates of food (n: 7) and human (n: 6) origin, including two (TIAC1993 and TIAC1994) that were isolated from nose and throat of one human case. Because the previously identified se/SE profiles of the in-house sequenced isolates was limited to a maximum 11 out of 27 described se genes, the complete se gene profile per isolate was extended with in silico PCR (Supplementary Table 6). To cover the complete repertoire of currently described se genes (ses, set, selv, sey, sel26, and sel27 that were missing in the in-house sequenced isolates), a publicly available WGS data of three isolates were added (Table 1). The in silico PCR was similarly applied for the publicly available WGS data to confirm the previously reported se genes (Fursova et al., 2020; Merda et al., 2020). Based on the investigated specificity of all primer sets published across the literature (see Supplementary Data and Supplementary Table 1), 139 out of 155 primer pairs (i.e., 89.7%) were selected for the in silico PCR. From the in silico PCR results, seven sets (i.e., 5.0% of the 139 used sets) were, moreover, identified to not consistently detect their target genes even when present (see Supplementary Data and Supplementary Table 6). This is most likely because these primers were not designed to anneal in sufficiently conserved gene regions. More information on the in silico PCR can be found in the Supplementary Data and Supplementary Table 6. For se genes or SEs whose presence was identified with conventional methods (EURL-CPS multiplex PCR assays and/or VIDAS/SET-RPLA), results of the in silico PCR were fully congruent (Table 1). For all se genes that were previously reported to be present in the publicly available WGS data [see Table 1 and Supplementary Table 2 (Fursova et al., 2020; Merda et al., 2020)], their presence was accordingly identified with in silico PCR except for selv that was not detected in SAMN13134218 based on the in silico PCR (see Supplementary Table 6 and Table 1) although it was previously reported to be present (Fursova et al., 2020). Moreover, across these three isolates analyzed with public data, in silico PCR enabled the detection of three additional se genes (i.e., sem in SAMN02391177, selx in SAMN02403200 and SAMN13134218, and selw in the three isolates; Supplementary Table 6 and Table 1), previously not identified to be present (Fursova et al., 2020; Merda et al., 2020).
Identifying the Completeness of Whole Genome Sequencing-Based se Gene Profiling
By comparison with the expected se gene profiles (Table 1), it was assessed whether WGS could identify the complete se gene repertoire (reportable range) of S. aureus isolates using the extended VFDB_Full database (Table 3). The presence or absence of, in total, 27 different se genes, among which are sec and selu variants, and of two pseudogenes was determined. Compared with solely using the VFDB_Full database, the presence or absence of eight extra genes (ses, set, selw to selz, sel26, and sel27) and sec variants could, hence, be determined.
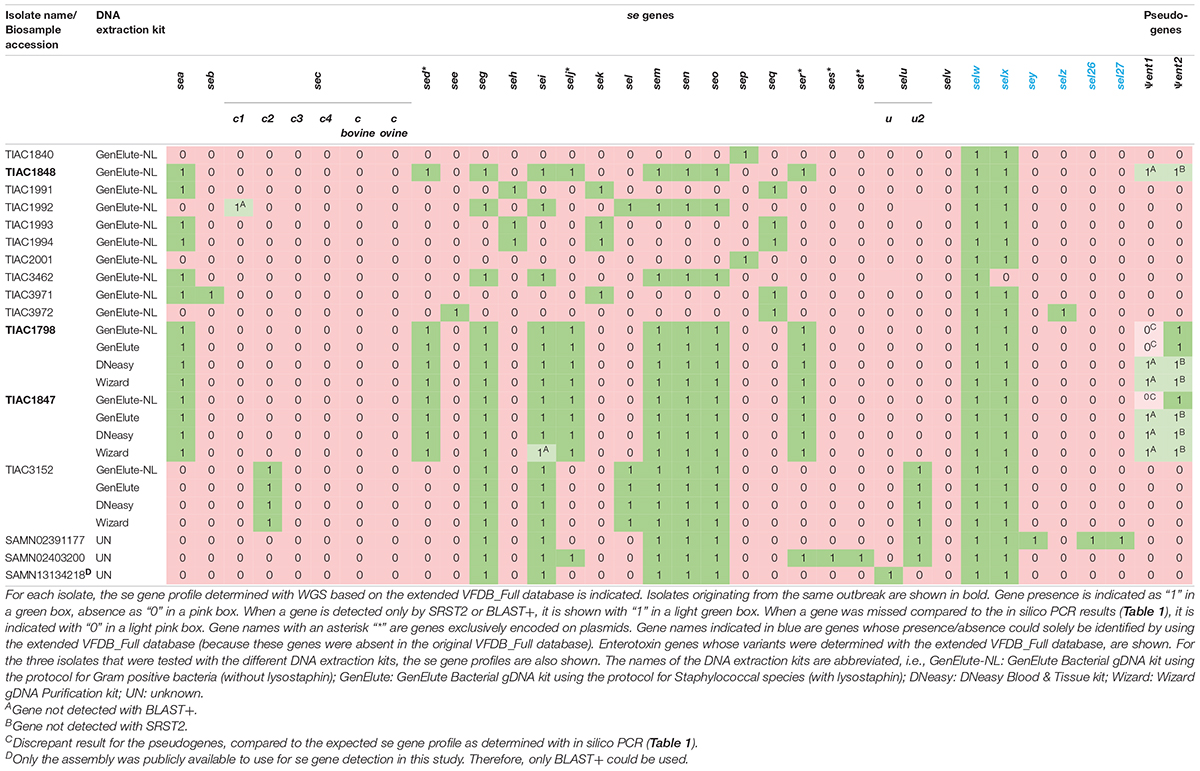
Table 3. WGS-based se gene profile of the selected in-house isolates and influence of the DNA extraction kits on se gene profiling.
For se gene detection, the WGS-determined profile per isolate (Table 3) was identical to what was expected (i.e., based on the conventional methods and in silico PCR as indicated in Table 1). Indeed, across the, in total, 432 observations (27 se genes for each of the 16 isolates), i.e., 127 positive and 305 negative observations, there were no FPs or FNs. In comparison with the analyses performed during the initial SFP outbreak investigation [Table 1; (Denayer et al., 2017)], WGS provided information on the presence/absence of 17 extra se genes and additionally allowed subtyping of sec and selu variants (Table 3). For the isolates for which public WGS data were available (Fursova et al., 2020; Merda et al., 2020), the WGS-based se gene detection methods applied in this study using the extended VFDB_Full database enabled the detection of three extra se genes (i.e., sem in SAMN02391177, selx in SAMN02403200 and SAMN13134218, and selw in the three isolates) in line with the expected characteristics within Table 1. The selv gene that was previously detected in SAMN13134218 (Fursova et al., 2020) was missed with the WGS method using the extended VFDB_Full database in this study. However, manual alignment of the contigs with the NCBI reference sequence of selv (Genbank reference EF030427.1) with BLAST confirmed its absence as was similarly found with the in silico PCR and, hence, the results of this study. Between the se gene profiles obtained with SRST2 and with BLAST+, only one difference was observed for se gene detection, related to a fragmentation of the assembly in the sec gene for TIAC1992 that led to its missed detection only with BLAST+. By using the extended VFDB_Full database, WGS, thus, enabled complete se gene profiling using both the read mapping-based (SRST2) and assembly based (BLAST+) approach.
For the WGS-based detection of the Ψent pseudogenes, there were some discrepancies in isolates TIAC1798, TIAC1847, and TIAC1848 compared with what was expected (cfr. Table 1). One of both pseudogenes was systematically missed with BLAST+ and SRST2, which could be explained by the clustering of both genes in the database that limits their detection to only one of them. Differences in the detected Ψent pseudogene in TIAC1848 by BLAST+ and SRST2 can be explained by the fact that both methods use different allele scoring methods. However, manual alignment of the respective contigs with a Ψent1-2 reference sequence (Genbank reference MN450303.1) confirmed the presence of both pseudogenes in the TIAC1798, TIAC1847, and TIAC1848 isolates with 100.0% sequence identity and query coverage (data not shown). Different DNA extraction kits and protocols (i.e., including lysostaphin in the bacterial lysis step) were tested for their influence on detecting se genes. Although lysostaphin increased the DNA yield, sufficient amounts and concentrations were obtained for Nextera XT library preparation without its usage [Table 2; (Illumina, 2018)]. For the detection of the 27 se genes, identical profiles were obtained with WGS for all samples (i.e., WGS data of an isolate processed with different DNA extraction kits) per isolate, irrespective of the DNA extraction kit, when compared with the expected se gene profiles. No problems in detecting any of the exclusively plasmid-encoded se genes (i.e., sed, selj, ser, ses, and set) were observed. When comparing se gene profiles obtained with SRST2 and BLAST+ separately for the samples per isolate, only one difference was obtained, related to the missed assembly-based detection of sei due to assembly fragmentation in TIAC1847 processed by the Wizard gDNA Purification kit. Therefore, no influence of the kit on the detection of se genes, whether or not plasmid-encoded, could be identified. Difficulties with the detection of both Ψent pseudogenes could again be observed across all samples of TIAC1798 and TIAC1847 with both detection methods, explained by the clustering of the pseudogenes in the database in combination with differences in the allele scoring methods of BLAST+ and SRST2. Manual alignment of the contigs against the Ψent1-2 reference (Genbank reference MN450303.1), confirmed the presence of both pseudogenes with 100.0% sequence identity and query coverage in both isolates for the different extraction kits (data not shown).
Performance Evaluation of Whole Genome Sequencing-Based Isolate Relatedness Determination
The performance of WGS in isolate relatedness determination was evaluated through comparison of inferred phylogenetic relationships by cgMLST-typing with the a priori known relationships of the in-house sequenced isolates. Of all 2208 core gene loci, on average, 91.2 ± 5.7% could be detected with 100.0% query coverage and sequence identity across all isolates. Figure 1 visualizes the relatedness between the S. aureus isolates, based on cgMLST. The tree demonstrates that the outbreak strain isolated from the food matrix (TIAC1798) clustered together with those of the human cases (TIAC1847 and TIAC1848) in a single clade carried by one branch, being separated from all other non-outbreak isolates. Similarly, both strains isolated from an identical human case at different locations (i.e., TIAC1993 and TIAC1994) also clustered together on one branch. No cgMLST allele differences were identified between any of the outbreak isolates within the outbreak clade or between the two isolates from the same human case. WGS-based cgMLST analyses, therefore, provided inferred phylogenetic relationships between the S. aureus isolates that were in accordance with the a priori known relationships.
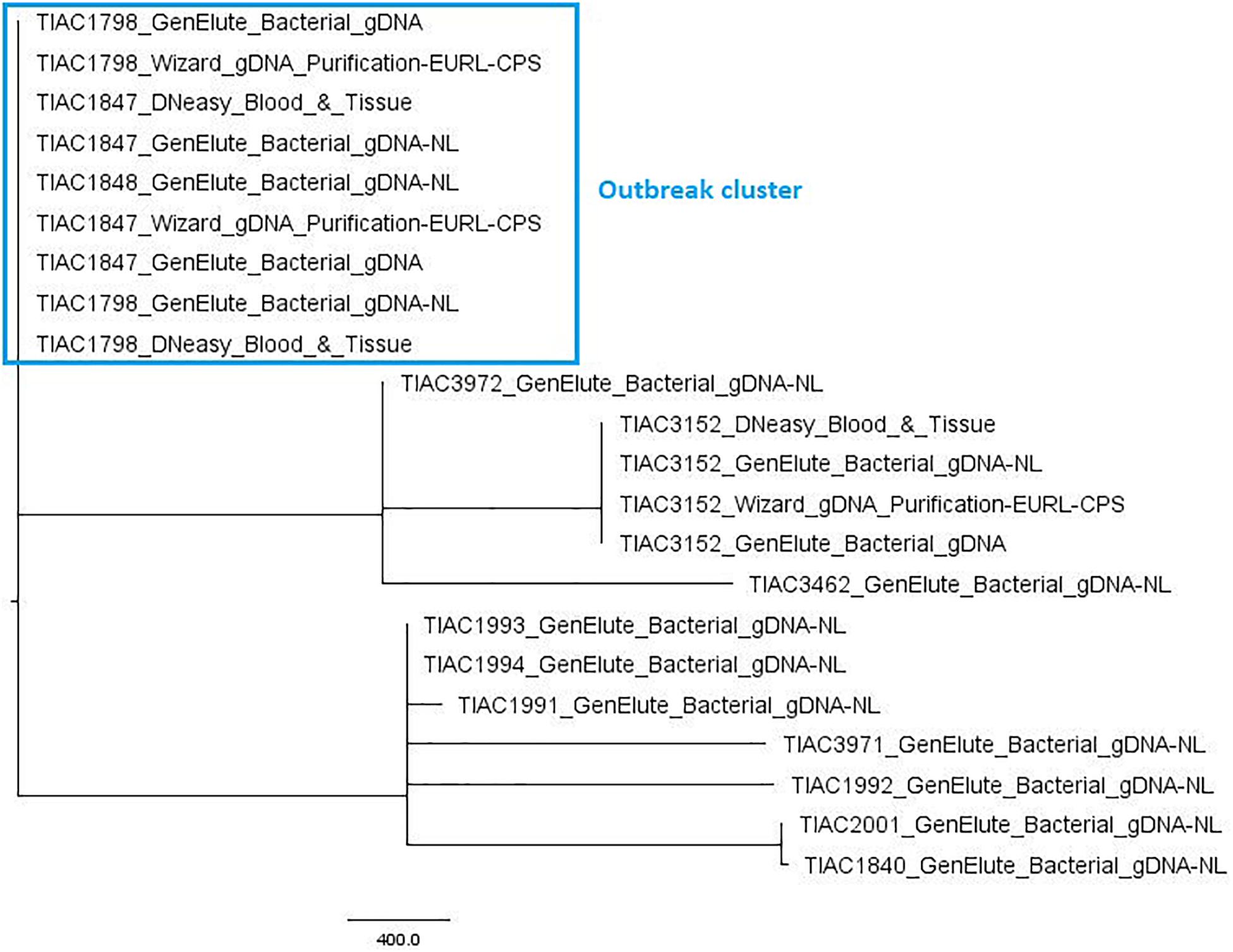
Figure 1. Minimum spanning tree based on cgMLST for S. aureus isolates. A minimum spanning tree was created with GrapeTree using the MSTreeV2 method on all in-house sequenced outbreak and non-outbreak samples, i.e., all isolates, some of which processed with different DNA extraction kits. The sample names in the figure consist of the name of the respective isolate and an abbreviation of the applied DNA extraction kit, i.e., GenElute_Bacterial_gDNA-NL: GenElute Bacterial gDNA kit using the protocol for Gram-positive bacteria [without (i.e., no) lysostaphin, NL]; GenElute_Bacterial_gDNA: GenElute Bacterial gDNA kit using the protocol for Staphylococcal species (with lysostaphin); DNeasy_Blood_&_Tissue: DNeasy Blood & Tissue kit; Wizard_gDNA_Purification: Wizard gDNA Purification kit. The outbreak samples (outlined in the blue box) from food origin (TIAC1798) consistently cluster together with those of human cases (TIAC1847 and TIAC1848) while non-outbreak samples (TIAC1840, TIAC1991, TIAC1992, TIAC1993, TIAC1994, TIAC2001, TIAC3152, TIAC3462, TIAC3971, and TIAC3972) are separated from the outbreak cluster and delineated per isolate (except for TIAC1993 and TIAC1994 sampled from the same person that also cluster together because of their identical cgMLST profiles). The scale bar represents the number of cgMLST allele differences between samples. No cgMLST allele differences were identified between the outbreak isolates, nor isolates processed with different DNA extraction kits.
Moreover, the used DNA extraction kit and workflow had no influence on the obtained cgMLST profiles or on the retrieved relationships between isolates. Indeed, no cgMLST allele differences were obtained between samples from the same isolate and, thus, clustered together per isolate in one single clade carried by a single branch, irrespective of the applied DNA extraction kit.
Discussion
The goal of this study was to assess the potential benefits of WGS compared with conventional molecular methods currently used in the investigation of SFP outbreaks. For this purpose, WGS was employed for se gene detection applying a custom database, and its profiles were compared with those previously obtained by routine methods (the EURL-CPS multiplex PCR and/or SET-RPLA/VIDAS analyses) and extended by in silico PCR. To cover the complete arsenal of currently described se genes, we included publicly available WGS data (Fursova et al., 2020; Merda et al., 2020) that were also verified with in silico PCR. Moreover, the performance of WGS-based isolate relatedness determination was evaluated using cgMLST on a data set with a priori known phylogenetic relationships. Within the scope of SFP investigation, different DNA extraction kits were tested for their influence on the comparability of WGS data.
This study demonstrates that WGS presents a valid alternative to molecular methods, serving as the ultimate multiplexing approach for se gene detection in S. aureus isolates. WGS enabled complete se gene profiling within one single assay. In contrast to WGS, PCR-based methods for the analysis of se gene profiles tend to miss the detection of genes that were not targeted by the approach, or when using primer pairs not targeting sufficiently conserved gene regions (see Supplementary Table 1). As a result, SFP outbreaks caused by non-classical enterotoxins that cannot be detected with existing commercial kits (i.e., SEs other than SEA to SEE) risk being left unresolved when also not targeted with PCR. However, complete PCR-based se gene profiling is very time-consuming and labor-intensive. Besides giving a first indication on the possible causality of a strain toward SFP outbreaks, knowledge on the complete se gene profile of isolates is also important in SFP surveillance. Its combination with clinical data of human cases can help in risk assessment of S. aureus strains to predict the potential pathogenicity of isolates. By analyzing isolated strains of SFP outbreaks for their complete se gene profile, more knowledge can moreover be acquired on se genes most commonly involved in SFP outbreaks.
Both SRST2 and BLAST+ showed highly similar WGS-based se gene detection outputs. Across all isolates, there was only one se gene left undetected because of assembly fragmentation when using BLAST+. The application of SRST2 read mapping is, hence, preferred for WGS-based se gene profiling. Although not encountered in this study, assembly issues might not only result in BLAST+ being more prone to the missed detection of se genes, but also the false positive detection of (pseudo)genes (Mason et al., 2018) that have recombined to new se genes. Indeed, selv and selu genes have been described to be formed from recombination events in sem and sei genes, or Ψent1 and Ψent2 pseudogenes, respectively (Thomas et al., 2006). More extensive performance evaluation of applying BLAST+ and SRST2 for se gene detection, which was, however, not the main goal of this study, would require a higher number of S. aureus isolates to be analyzed. Although previous research shows performance differences to be limited for gene detection in other bacterial species when using high-coverage data sets (Bogaerts et al., 2021), it is an interesting future research topic to also verify for S. aureus enterotoxin gene detection.
Whole genome sequencing also allows subtyping of se variants. Recently, an increasing number of SE variants are being described (Blaiotta et al., 2004; Fernández et al., 2006; Kohler et al., 2012; Johler et al., 2016; Aziz et al., 2020; Etter et al., 2020; Merda et al., 2020). Multiple variants (such as for SEC) are already shown to exhibit different structural and superantigenic features (Etter et al., 2020), but the influence on their emetic activity has not yet been investigated. However, in the future, based on this knowledge, subtyping of variants can become increasingly important in predicting the emetic potential of S. aureus strains. In this study, subtyping of sec and selu was done as proof of concept, but the applied se gene detection thresholds (i.e., >60.0% query coverage and >90.0% sequence identity for BLAST+ and >60.0% query coverage and <10.0% sequence divergence for SRST2) allow also detecting other se variants, including novel ones, without subtyping. However, WGS offers the potential to expand se subtyping to all variants when annotated as such in the applied gene detection database. This is an additional added value of WGS compared with conventional PCR-based methods, in which extra Sanger sequencing analyses of the obtained amplicons are often required for further subtype identification (Collery and Smyth, 2007).
For WGS to provide full se gene profiling, the application of a database containing reference sequences for all se genes and, if subtyping is of interest, also all variants is indispensable. However, publicly available reference databases for virulence gene detection in S. aureus isolates (Joensen et al., 2014; Liu et al., 2019; Sayers et al., 2019; Merda et al., 2020) do not enable complete se gene profiling (e.g., ses, set, selw to selz, sel26 and sel27 are missing in the VFDB_Full database, and ses, set, selv to selz, sel26 and sel27 in the VirulenceFinder DataBase) or subtyping of se variants (because they are not or ambiguously annotated in the databases). Studies using these databases as such (Huang et al., 2017; Petit and Read, 2018; Fursova et al., 2020; Karki et al., 2020; Merda et al., 2020; Schwendimann et al., 2021), thus risk underestimating the prevalence of se genes, potentially affecting obtained results. Indeed, more se genes were reported to be present in the isolates with publicly available WGS data after reanalysis with our methods compared with their previously WGS-determined se gene profiles, mainly because of the lack of complete databases in the other studies (Fursova et al., 2020; Merda et al., 2020). Recently, the EURL-CPS developed a workflow for S. aureus analysis in the scope of SFP investigation, i.e., NAuRA, using a database that allows almost complete (selw is missing and no variant subtyping) se gene detection (Merda et al., 2020). NAuRA is available on github, and the Uniprot accession numbers of SE protein sequences used as reference in their genomic analyses are available in the supplementary data of the corresponding publication (Merda et al., 2020). NAuRA can be locally installed by an experienced bioinformatician, who might not always be available in each NRL. Nevertheless, the implementation of a publicly available database that is easily accessible and queryable is favorable in a routine setting because it supports the comparability of WGS data results, especially important during SFP investigation. To make WGS more approachable, it is, thus, important that reference databases enabling full se gene profiling are made publicly available through online open repositories. Moreover, these databases should be continuously updated with the state-of-the-art knowledge so that full se gene profiling and variant subtyping is possible. A FASTA file containing the se gene sequences and their corresponding accession numbers used for the database constructed in this manuscript was added to the Supplementary Data. This database was also integrated in the open access online interface5 (registration required), using the parameters as described in this manuscript, to moreover allow user-friendly se gene detection.
Although present in the extended VFDB_Full database, in our study, discrepant observations were identified with the detection of Ψent pseudogenes when using WGS. This was related to (i) database sequence clustering, which limits gene detection to one per cluster, and (ii) differences in the allele scoring methods of SRST2 and BLAST+ that decide upon the detected gene (allele) per cluster. Because both Ψent pseudogenes coexist on the enterotoxin gene cluster (egc) (Jarraud et al., 2001), one of them risks being left undetected when using BLAST+ or SRST2. However, because pseudogenes are non-functional (Letertre et al., 2003b; Liang et al., 2016), the impact of these discrepancies can be regarded as negligible. Nevertheless, if their detection would be of special interest, we suggest performing manual alignment of the assembly with a Ψent1-2 reference sequence to identify their potential joint presence as performed in this study. This extra alignment step still remains much less time-consuming compared with the Sanger sequencing analyses that would be required when using conventional PCR methods to distinguish pseudogene presence from those of the selu gene (Collery and Smyth, 2007). Sequence clustering of gene variants is, however, important when applying large databases, such as the VFDB_Full, to aid in identifying the most appropriate gene (allele) within the cluster. Without clustering of gene variants in combination with default gene detection thresholds, a hit to all of those variants might be picked up if the gene is present, whereas manual inspection would reveal that it concerns only one gene (variant) that is present. Based on sequence similarity between all se genes and variants (Merda et al., 2020), we decided to apply a sequence identity threshold of 85.0% to cluster the extended VFDB_Full database, combined with default gene detection thresholds (i.e., >60.0% query coverage and >90.0% sequence identity for BLAST+ and >60.0% query coverage and <10.0% sequence divergence for SRST2). Most se genes and variants are consequently clustered together per gene except for selu and the pseudogenes or sem and selv that are present in the same cluster together. With the combination of both clustering and gene detection thresholds, a sufficient level of sensitivity and specificity is acquired to detect novel se variants and avoid FP and FN gene detection, respectively. The importance of appropriate gene detection (and database sequence clustering) thresholds is also shown by analyzing publicly available WGS data. Based on in silico PCR and manual inspection of the contigs, the selv gene was found to have been previously falsely detected within these public WGS data [SAMN13134218; (Fursova et al., 2020)]. This could likely be explained by the application of default SRST2 gene detection thresholds (i.e., >90.0% query coverage and <10.0% sequence divergence), which are not stringent enough to distinguish between the present sem and sei, and the absent selv, in the absence of a database clustering step. Indeed, with these detection criteria, sem, sei, and selv were detected in the previous study (Fursova et al., 2020) although only sem and sei were determined to be present here. Although the gene detection criteria in our study were looser, the presence and absence of these genes could be correctly identified because they were combined with a database whose sequences were clustered. Of all 27 enterotoxins described to date, selv was, thus, determined not to be present in any of the selected strains/public data. Expanding the number of isolates in this study would likely also increase the potential of covering selv. However, because this gene is not targeted by the VIDAS/SET-RPLA immunoassays or EURL-CPS multiplex PCR applied routinely in-house and is found to be only scarcely prevalent (Merda et al., 2020), including an isolate containing selv would not be straightforward. Moreover, because sem and selv are not yet found to co-occur within the same strain to date (Thomas et al., 2006; Argudín et al., 2010), detection issues related to their clustering are not expected if an isolate containing selv would have been added to the study (as was attempted with the public WGS data). Nor are selu and pseudogene detection issues expected related to their clustering in the database. When adding more variant sequences to the database, as elaborated above to allow subtyping, it is necessary that applied gene detection thresholds and database sequence identity thresholds for clustering are further reviewed to ensure accurate and specific se variant detection.
To examine the completeness of WGS-based se gene profiling in S. aureus isolates, previously assessed se gene profiles were extended with in silico PCR. Using in silico PCR to validate the detection of se genes in all in-house sequenced isolates and isolates for which WGS data were publicly available might seem conflicting because both approaches are dependent on the same WGS data. However, although BLAST+ and SRST2 approaches detect complete se gene coding sequences, in silico PCR only evaluates primer binding sites and obtained amplicon sizes. Sixteen out of 155 primer pairs (i.e., 10.3%) described in literature did not result in a specific in silico product. Moreover, from the 139 primer sets used for the in silico PCR, another seven pairs (i.e., 5.0%) were identified to anneal in non-conserved gene regions leading to the missed detection of its corresponding target gene in some isolates tested within this study (see Supplementary Data). Increasing the number of S. aureus isolates, likely also supplements the gene sequence variability and potentially leads to the identification of extra primers that are not developed in sufficiently conserved regions. Although this remains an interesting research question for further research, with the limited number of isolates present, we highlight the added value of incorporating genomic data for more in-depth primer optimization (Vanneste et al., 2018) and show the importance of profound sensitivity and specificity studies for developed primer sets before publication. Indeed, if these primer pairs were used in a conventional PCR, a FN result might have been obtained, impeding a full correct characterization of the se profile. By applying the in silico PCR approach with 139 PCR primer pairs (i.e., 89.7%) selected based on their specificity, something that would be less feasible in a conventional approach, this risk was circumvented and even showed an added value. For the 11 conventionally PCR tested se genes, all results were completely congruent with the in silico PCR results, thereby validating our approach. Moreover, the in silico PCR indicated an inconsistency with the reported se profiles in a previous study, which was further confirmed using manual alignments and explained by the applied computational methods. Our review of published primer pairs and in silico investigation for their specificity can, therefore, also be valuable information for laboratories not having the resources to perform WGS.
Besides the fact that complete se gene profiling can provide an initial insight into the potential causality of strains toward outbreaks, WGS also allows inferring more refined phylogenetic relationships between S. aureus isolates based on cgMLST-typing. Because 91.2 ± 5.7% of the 2208 core genome loci could be typed, a relatively high number of genomic markers was available to reliably resolve isolate relationships in this study. cgMLST-typing has gained interest with regard to standardization and harmonization because of the transferability of the applied scheme, enabling the use of the same database (Sabat et al., 2017), which allows SFP outbreaks to be investigated across laboratories. Although other cgMLST schemes have been developed by multiple instances (Leopold et al., 2014), we adopted the PubMLST scheme to infer S. aureus isolate relationships in this study because it is the most frequently used scheme, that is, moreover, also applied by the EURL-CPS (Merda et al., 2020). The ultimate level of discriminative power is provided by SNP analysis. However, this can only be used to further fine-tune relationships between closely related strains. Because the overall distance based on cgMLST loci differences between the isolates in this study is large, except for the outbreak strains and two identical strains TIAC1993 and TIAC1994 isolated from one human case, picking a suitable reference genome for SNP analysis would be impossible. Increasing the number of outbreak and non-outbreak isolates can be used to illustrate the discriminative power of WGS even more than in this study. However, the rapid course of disease and the complexity of S. aureus isolation because of potentially affected strain viability, often complicate the collection of strains during outbreak investigation. Moreover, not many studies were found to analyze S. aureus isolates with WGS in the scope of SFP outbreak investigation. Our study, by demonstrating its added value, might contribute in stimulating the use of WGS in SFP outbreak investigations so that its benefits can be fully exploited.
Data sharing between laboratories is crucial in the investigation of SFP outbreaks. However, the comparability of WGS data can potentially be affected by impaired plasmid extraction performances of commercial DNA preparation kits (Becker et al., 2016; Nouws et al., 2020b), especially when harboring important virulence genes, such as se genes. Therefore, we tested a frequently used kit (DNeasy Blood & Tissue kit), the kit recommended by the EURL-CPS for DNA preparation of S. aureus isolates (Wizard gDNA Purification kit) and the kit used at the Belgian NRL-FBO/NRL-CPS (GenElute Bacterial gDNA kit) for their influence on WGS data analyses of S. aureus. Although the DNeasy Blood & Tissue and Wizard gDNA Purification kits were previously described to have impaired plasmid extraction performances in Gram-negative species, possibly leading to missed WGS-based detection of plasmid-encoded genes (Becker et al., 2016; Pasquali et al., 2019; Nouws et al., 2020b), this assumption could not be extrapolated to S. aureus isolates based on the results in our study. Indeed, identical profiles of detected se genes, whether or not plasmid-encoded, were obtained between the samples per isolate, irrespective of the applied DNA extraction kit. Moreover, the use of different kits had no influence on inferred cgMLST-based isolate relationships. Kit choice to prepare DNA of S. aureus for WGS can, thus, be based on other factors. Because of its earlier communication to be appropriate for WGS data analysis of Gram-negative and -positive bacteria (Nouws et al., 2020b), the GenElute Bacterial gDNA kit can be of interest for usage in laboratories, such as NRLs, investigating multiple foodborne pathogens. We moreover examined the necessity of employing the expensive lysostaphin enzyme within its protocol as specified for Staphylococcal species (Zhao et al., 2012). Because sufficient amounts of DNA were obtained without using lysostaphin, and its use did not influence the outcome of WGS analyses, the GenElute Bacterial gDNA protocol for Gram-positive bacteria not using lysostaphin is especially beneficial for routine application because discarding lysostaphin nearly halves the cost per sample (Table 2).
Although a limited number of isolates was used in this study, it is clear that WGS has benefits in the investigation of SFP outbreaks, yielding information on the complete se profile and relatedness between strains, all within one single test. Although WGS can provide, besides se gene detection, complete isolate characterization by detecting other virulence and antimicrobial resistance (AMR) genes, such as, among others, the virulence tsst gene (Kulhankova et al., 2014) responsible for, e.g., menstrual toxic shock syndrome, or the AMR mecA gene causing methicillin resistance (Peacock and Paterson, 2015), these characteristics were not addressed in this study because of their irrelevance in SFP or its treatment (Fisher et al., 2018), and because no conventional metadata concerning these characteristics were available with which to compare the performance of WGS-based detection. Performing in silico PCR to obtain information on the presence/absence of these genes would be virtually impossible because of the large numbers of AMR and virulence genes that exist. However, other studies have already demonstrated that WGS scores very well in predicting the AMR phenotype within S. aureus (Gordon et al., 2014; Cunningham et al., 2020). For WGS to yield all benefits in SFP investigation, it is important that S. aureus strain isolation is successful. Because the viability of S. aureus can be affected by food processing through, e.g., heating, while integrally preserving the emetic properties of the produced enterotoxins (Fisher et al., 2018), a proportion of the suspected foods might be left unable to be investigated with the proposed method. Metagenomics sequencing of the complete sample without strain isolation might offer a solution relevant for further research (Boers et al., 2019). However, both WGS and metagenomics sequencing only deliver information at the genotypic level. Ideally, sequencing-based methods should be used in first line to screen for se gene presence and can then be combined with a method that allows detection of all produced SEs at the protein level. Nevertheless, through complete se gene detection, WGS enables broadening the insight in S. aureus and SFP and examines the potential of isolates to produce SEs and to play a role in SFP. Moreover, thanks to its ultimate discriminatory power, WGS can simultaneously more accurately pinpoint strains as the cause for a SFP outbreak and, thus, accelerate its management. Conclusively, this study shows the added value of using WGS in SFP outbreak investigation and encourages its use in a routine setting.
Data Availability Statement
The datasets generated for this study can be found in the NCBI SRA repository under the accession number PRJNA750393 (http://www.ncbi.nlm.nih.gov/bioproject/750393). Corresponding ac-cession numbers are listed in Supplementary Table 7.
Author Contributions
SN, NR, and SDK conceived and designed the study. SDK supervised the project. SN performed the wet lab experiments. BB and KV provided the bioinformatic tools in Galaxy. SN and BB performed the bioinformatic analysis. SD and BV were responsible for the former generation of all results obtained with the conventional methods. SN and SDK participated in the interpretation of the results and wrote the manuscript. KV, BB, LL, NR, and KM provided specialized feedback on the obtained results. All co-authors commented and approved the submitted version.
Funding
This research that yielded these results was funded by Sciensano (contract RP.Be READY).
Conflict of Interest
The authors declare that the research was conducted in the absence of any commercial or financial relationships that could be construed as a potential conflict of interest.
Publisher’s Note
All claims expressed in this article are solely those of the authors and do not necessarily represent those of their affiliated organizations, or those of the publisher, the editors and the reviewers. Any product that may be evaluated in this article, or claim that may be made by its manufacturer, is not guaranteed or endorsed by the publisher.
Acknowledgments
We thank the technicians of the service transversal activities in Applied Genomics at Sciensano, Belgium for conducting all Next Generation Sequencing runs. We also express our gratitude to the NRL-FBO/NRL-CPS and the EURL-CPS for sharing S. aureus isolates and their metadata concerning the SE/se profiles (determined with VIDAS/SET-RPLA and the multiplex PCR) and mutual relationships between isolates (based on PFGE) using conventional methods.
Supplementary Material
The Supplementary Material for this article can be found online at: https://www.frontiersin.org/articles/10.3389/fmicb.2021.750278/full#supplementary-material
Footnotes
- ^ https://www.ncbi.nlm.nih.gov/tools/primer-blast/index.cgi
- ^ https://galaxy.sciensano.be/tool_runner?tool_id=pipeline_staphylococcus
- ^ https://github.com/lh3/seqtk
- ^ http://www.mgc.ac.cn/VFs/download.htm
- ^ https://galaxy.sciensano.be/tool_runner?tool_id=pipeline_staphylococcus
References
Afgan, E., Baker, D., Batut, B., van den Beek, M., Bouvier, D., Čech, M., et al. (2018). The Galaxy platform for accessible, reproducible and collaborative biomedical analyses: 2018 update. Nucleic Acids Res. 46, W537–W544. doi: 10.1093/nar/gky379
Altschul, S. F., Gish, W., Miller, W., Myers, E. W., and Lipman, D. J. (1990). Basic local alignment search tool. J. Mol. Biol. 215, 403–410. doi: 10.1016/S0022-2836(05)80360-2
Anderson, D. J., Harris, S. R., Godofsky, E., Toriscelli, T., Rude, T. H., Elder, K., et al. (2014). Whole Genome Sequencing of a Methicillin-Resistant Staphylococcus aureus Pseudo-Outbreak in a Professional Football Team. Open Forum Infect. Dis. 1:2633851. doi: 10.1093/ofid/ofu096
Argudín, M. A., Mendoza, M. C., and Rodicio, M. R. (2010). Food Poisoning and Staphylococcus aureus Enterotoxins. Toxins 2, 1751–1773. doi: 10.3390/toxins2071751
Aung, M. S., Urushibara, N., Kawaguchiya, M., Ito, M., Habadera, S., and Kobayashi, N. (2020). Prevalence and genetic diversity of staphylococcal enterotoxin (-Like) genes sey, selw, selx, selz, sel26 and sel27 in community-acquired methicillin-resistant Staphylococcus aureus. Toxins 12, 1–13. doi: 10.3390/toxins12050347
Aziz, F., Hisatsune, J., Yu, L., Kajimura, J., Sato’o, Y., Ono, H. K., et al. (2020). Staphylococcus aureus Isolated from Skin from Atopic-Dermatitis Patients Produces Staphylococcal Enterotoxin Y, Which Predominantly Induces T-Cell Receptor Vα-Specific Expansion of T Cells. Infect. Immun. 88:19. doi: 10.1128/IAI.00360-19
Bankevich, A., Nurk, S., Antipov, D., Gurevich, A. A., Dvorkin, M., Kulikov, A. S., et al. (2012). SPAdes: A New Genome Assembly Algorithm and Its Applications to Single-Cell Sequencing. J. Comput. Biol. 19, 455–477. doi: 10.1089/cmb.2012.0021
Bayles, K. W., and Iandolo, J. J. (1989). Genetic and molecular analyses of the gene encoding staphylococcal enterotoxin D. J. Bacteriol. 171, 4799–4806. doi: 10.1128/jb.171.9.4799-4806.1989
Becker, K., Roth, R., and Peters, G. (1998). Rapid and Specific Detection of Toxigenic Staphylococcus aureus: Use of Two Multiplex PCR Enzyme Immunoassays for Amplification and Hybridization of Staphylococcal Enterotoxin Genes, Exfoliative Toxin Genes, and Toxic Shock Syndrome Toxin 1 Gene. J. Clin. Microbiol. 36, 2548–2553. doi: 10.1128/JCM.36.9.2548-2553.1998
Becker, L., Steglich, M., Fuchs, S., Werner, G., and Nübel, U. (2016). Comparison of six commercial kits to extract bacterial chromosome and plasmid DNA for MiSeq sequencing. Sci. Rep. 6:28063. doi: 10.1038/srep28063
Bergdoll, M. S., Borja, C. R., Robbins, R. N., and Weiss, K. F. (1971). Identification of enterotoxin E. Infect. Immun. 4, 593–595. doi: 10.1128/iai.4.5.593-595.1971
Blaiotta, G., Ercolini, D., Pennacchia, C., Fusco, V., Casaburi, A., Pepe, O., et al. (2004). PCR detection of staphylococcal enterotoxin genes in Staphylococcus spp. strains isolated from meat and dairy products. Evidence for new variants of seG and seI in S. aureus AB-8802. J. Appl. Microbiol. 97, 719–730. doi: 10.1111/j.1365-2672.2004.02349.x
Blair, J. E., and Williams, R. E. (1961). Phage typing of staphylococci. Bull. World Health Organ. 24, 771–784.
Boers, S. A., Jansen, R., and Hays, J. P. (2019). Understanding and overcoming the pitfalls and biases of next-generation sequencing (NGS) methods for use in the routine clinical microbiological diagnostic laboratory. Eur. J. Clin. Microbiol. Infect. Dis. 38, 1059–1070. doi: 10.1007/s10096-019-03520-3
Bogaerts, B., Nouws, S., Verhaegen, B., Denayer, S., Van Braekel, J., Winand, R., et al. (2021). Validation strategy of a bioinformatics whole genome sequencing workflow for Shiga toxin-producing Escherichia coli using a reference collection extensively characterized with conventional methods. Microb. Genomics 7:531. doi: 10.1099/mgen.0.000531
Bolger, A. M., Lohse, M., and Usadel, B. (2014). Trimmomatic: a flexible trimmer for Illumina sequence data. Bioinformatics 30, 2114–2120. doi: 10.1093/bioinformatics/btu170
Camacho, C., Coulouris, G., Avagyan, V., Ma, N., Papadopoulos, J., Bealer, K., et al. (2009). BLAST+: Architecture and applications. BMC Bioinformatics 10, 1–9. doi: 10.1186/1471-2105-10-421
Collery, M. M., and Smyth, C. J. (2007). Rapid differentiation of Staphylococcus aureus isolates harbouring egc loci with pseudogenes ψent1 and ψent2 and the selu or selu v gene using PCR-RFLP. J. Med. Microbiol. 56, 208–216. doi: 10.1099/jmm.0.46948-0
Cookson, B. D., Aparicio, P., Deplano, A., Struelens, M., Goering, R., and Marples, R. (1996). Inter-centre comparison of pulsed-field gel electrophoresis for the typing of methicillin-resistant Staphylococcus aureus. J. Med. Microbiol. 44, 179–184. doi: 10.1099/00222615-44-3-179
Cremers, A. J. H., Coolen, J. P. M., Bleeker-Rovers, C. P., van der Geest-Blankert, A. D. J., Haverkate, D., Hendriks, H., et al. (2020). Surveillance-embedded genomic outbreak resolution of methicillin-susceptible Staphylococcus aureus in a neonatal intensive care unit. Sci. Rep. 10:2619. doi: 10.1038/s41598-020-59015-1
Cunningham, S. A., Chia, N., Jeraldo, P. R., Quest, D. J., Johnson, J. A., Boxrud, D. J., et al. (2017). Comparison of Whole-Genome Sequencing Methods for Analysis of Three Methicillin-Resistant Staphylococcus aureus Outbreaks. J. Clin. Microbiol. 55, 1946–1953. doi: 10.1128/JCM.00029-17
Cunningham, S. A., Jeraldo, P. R., Schuetz, A. N., Heitman, A. A., and Patel, R. (2020). Staphylococcus aureus whole genome sequence–based susceptibility and resistance prediction using a clinically amenable workflow. Diagn. Microbiol. Infect. Dis. 97:115060. doi: 10.1016/j.diagmicrobio.2020.115060
Denayer, S., Delbrassinne, L., Nia, Y., and Botteldoorn, N. (2017). Food-Borne Outbreak Investigation and Molecular Typing: High Diversity of Staphylococcus aureus Strains and Importance of Toxin Detection. Toxins 9:407. doi: 10.3390/toxins9120407
ECDC (2019). Proficiency test for Listeria monocytogenes whole genome assembly – 2018. Europe: European Centre for Disease Prevention and Control. Available online at: https://www.ecdc.europa.eu/en/publications-data/proficiency-test-listeria-monocytogenes-whole-genome-assembly-2018
Ekkelenkamp, M. B., Vervoorn, M. T., Bayjanov, J. R., Fluit, A. C., Benaissa-Trouw, B. J., and Ramjankhan, F. Z. (2018). Therapy and Outcome of Staphylococcus aureus Infections of Intracorporeal Ventricular Assist Devices. Artif. Organs 42, 983–991. doi: 10.1111/aor.13159
Etter, D., Schelin, J., Schuppler, M., and Johler, S. (2020). Staphylococcal Enterotoxin C—An Update on SEC Variants, Their Structure and Properties, and Their Role in Foodborne Intoxications. Toxins 12:584. doi: 10.3390/toxins12090584
European Food Safety Authority (EFSA) and European Centre for Disease Prevention and Control (ECDC) (2021). The European Union One Health 2019 Zoonoses Report. EFSA J. 19:6406. doi: 10.2903/j.efsa.2021.6406
Fernández, M. M., De Marzi, M. C., Berguer, P., Burzyn, D., Langley, R. J., Piazzon, I., et al. (2006). Binding of natural variants of staphylococcal superantigens SEG and SEI to TCR and MHC class II molecule. Mol. Immunol. 43, 927–938. doi: 10.1016/j.molimm.2005.06.029
Fisher, E. L., Otto, M., and Cheung, G. Y. C. (2018). Basis of Virulence in Enterotoxin-Mediated Staphylococcal Food Poisoning. Front. Microbiol. 9, 1–18. doi: 10.3389/fmicb.2018.00436
Fursova, K., Sorokin, A., Sokolov, S., Dzhelyadin, T., Shulcheva, I., Shchannikova, M., et al. (2020). Virulence Factors and Phylogeny of Staphylococcus aureus Associated With Bovine Mastitis in Russia Based on Genome Sequences. Front. Vet. Sci. 7, 1–10. doi: 10.3389/fvets.2020.00135
Gordon, N. C., Price, J. R., Cole, K., Everitt, R., Morgan, M., Finney, J., et al. (2014). Prediction of Staphylococcus aureus Antimicrobial Resistance by Whole-Genome Sequencing. J. Clin. Microbiol. 52, 1182–1191. doi: 10.1128/JCM.03117-13
Gurevich, A., Saveliev, V., Vyahhi, N., and Tesler, G. (2013). QUAST: quality assessment tool for genome assemblies. Bioinformatics 29, 1072–1075. doi: 10.1093/bioinformatics/btt086
Hennekinne, J.-A., Ostyn, A., Guillier, F., Herbin, S., Prufer, A.-L., and Dragacci, S. (2010). How Should Staphylococcal Food Poisoning Outbreaks Be Characterized? Toxins 2, 2106–2116. doi: 10.3390/toxins2082106
Heymans, F., Fischer, A., Stow, N. W., Girard, M., Vourexakis, Z., Courtis, A., et al. (2010). Screening for Staphylococcal Superantigen Genes Shows No Correlation with the Presence or the Severity of Chronic Rhinosinusitis and Nasal Polyposis. PLoS One 5:e9525. doi: 10.1371/journal.pone.0009525
Huang, A. D., Luo, C., Pena-Gonzalez, A., Weigand, M. R., Tarr, C. L., and Konstantinidis, K. T. (2017). Metagenomics of Two Severe Foodborne Outbreaks Provides Diagnostic Signatures and Signs of Coinfection Not Attainable by Traditional Methods. Appl. Environ. Microbiol. 83, 1–14. doi: 10.1128/AEM.02577-16
Illumina (2018). Nextera XT DNA library prep kit reference guide #15031942. Available online at: https://support.illumina.com/content/dam/illumina-support/documents/documentation/chemistry_documentation/samplepreps_nextera/nextera-xt/nextera-xt-library-prep-reference-guide-15031942-05.pdf (accessed date May 2019).
Inouye, M., Dashnow, H., Raven, L.-A., Schultz, M. B., Pope, B. J., Tomita, T., et al. (2014). SRST2: Rapid genomic surveillance for public health and hospital microbiology labs. Genome Med. 6:90. doi: 10.1186/s13073-014-0090-6
Jarraud, S., Peyrat, M. A., Lim, A., Tristan, A., Bes, M., Mougel, C., et al. (2001). A Highly Prevalent Operon of Enterotoxin Gene, Forms a Putative Nursery of Superantigens in Staphylococcus aureus. J. Immunol. 166, 669–677. doi: 10.4049/jimmunol.166.1.669
Joensen, K. G., Scheutz, F., Lund, O., Hasman, H., Kaas, R. S., Nielsen, E. M., et al. (2014). Real-Time Whole-Genome Sequencing for Routine Typing, Surveillance, and Outbreak Detection of Verotoxigenic Escherichia coli. J. Clin. Microbiol. 52, 1501–1510. doi: 10.1128/JCM.03617-13
Joensen, K. G., Tetzschner, A. M. M., Iguchi, A., Aarestrup, F. M., and Scheutz, F. (2015). Rapid and Easy In Silico Serotyping of Escherichia coli Isolates by Use of Whole-Genome Sequencing Data. J. Clin. Microbiol. 53, 2410–2426. doi: 10.1128/JCM.00008-15
Johler, S., Giannini, P., Jermini, M., Hummerjohann, J., Baumgartner, A., and Stephan, R. (2015). Further Evidence for Staphylococcal Food Poisoning Outbreaks Caused by egc-Encoded Enterotoxins. Toxins 7, 997–1004. doi: 10.3390/toxins7030997
Johler, S., Sihto, H.-M., Macori, G., and Stephan, R. (2016). Sequence Variability in Staphylococcal Enterotoxin Genes seb, sec, and sed. Toxins 8:169. doi: 10.3390/toxins8060169
Jolley, K. A., Bray, J. E., and Maiden, M. C. J. (2018). Open-access bacterial population genomics: BIGSdb software, the PubMLST.org website and their applications. Wellcome Open Res. 3:124. doi: 10.12688/wellcomeopenres.14826.1
Karki, A. B., Neyaz, L., and Fakhr, M. K. (2020). Comparative Genomics of Plasmid-Bearing Staphylococcus aureus Strains Isolated From Various Retail Meats. Front. Microbiol. 11:574923. doi: 10.3389/fmicb.2020.574923
Kohler, P. L., Greenwood, S. D., Nookala, S., Kotb, M., Kranz, D. M., and Schlievert, P. M. (2012). Staphylococcus aureus Isolates Encode Variant Staphylococcal Enterotoxin B Proteins That Are Diverse in Superantigenicity and Lethality. PLoS One 7:e41157. doi: 10.1371/journal.pone.0041157
Kulhankova, K., King, J., and Salgado-Pabón, W. (2014). Staphylococcal toxic shock syndrome: superantigen-mediated enhancement of endotoxin shock and adaptive immune suppression. Immunol. Res. 59, 182–187. doi: 10.1007/s12026-014-8538-8
Larsen, M. V., Cosentino, S., Rasmussen, S., Friis, C., Hasman, H., Marvig, R. L., et al. (2012). Multilocus Sequence Typing of Total-Genome-Sequenced Bacteria. J. Clin. Microbiol. 50, 1355–1361. doi: 10.1128/JCM.06094-11
Lee, G. C., Long, S. W., Musser, J. M., Beres, S. B., Olsen, R. J., Dallas, S. D., et al. (2015). Comparative Whole Genome Sequencing of Community-Associated Methicillin-Resistant Staphylococcus aureus Sequence Type 8 from Primary Care Clinics in a Texas Community. Pharmacother. J. Hum. Pharmacol. Drug Ther. 35, 220–228. doi: 10.1002/phar.1536
Leopold, S. R., Goering, R. V., Witten, A., Harmsen, D., and Mellmann, A. (2014). Bacterial Whole-Genome Sequencing Revisited: Portable, Scalable, and Standardized Analysis for Typing and Detection of Virulence and Antibiotic Resistance Genes. J. Clin. Microbiol. 52, 2365–2370. doi: 10.1128/JCM.00262-14
Letertre, C., Perelle, S., Dilasser, F., and Fach, P. (2003b). Identification of a new putative enterotoxin SEU encoded by the egc cluster of Staphylococcus aureus. J. Appl. Microbiol. 95, 38–43. doi: 10.1046/j.1365-2672.2003.01957.x
Letertre, C., Perelle, S., Dilasser, F., and Fach, P. (2003a). A strategy based on 5′ nuclease multiplex PCR to detect enterotoxin genes sea to sej of Staphylococcus aureus. Mol. Cell. Probes 17, 227–235. doi: 10.1016/S0890-8508(03)00058-6
Li, W., and Godzik, A. (2006). Cd-hit: a fast program for clustering and comparing large sets of protein or nucleotide sequences. Bioinformatics 22, 1658–1659. doi: 10.1093/bioinformatics/btl158
Liang, C., Schaack, D., Srivastava, M., Gupta, S., Sarukhanyan, E., Giese, A., et al. (2016). A Staphylococcus aureus Proteome Overview: Shared and Specific Proteins and Protein Complexes from Representative Strains of All Three Clades. Proteomes 4:8. doi: 10.3390/proteomes4010008
Liu, B., Zheng, D., Jin, Q., Chen, L., and Yang, J. (2019). VFDB 2019: a comparative pathogenomic platform with an interactive web interface. Nucleic Acids Res. 47, D687–D692. doi: 10.1093/nar/gky1080
Lopes, H. R., Noleto, A. L. S., de Las Heras, M. D., and Bergdoll, M. S. (1993). Selective Enterotoxin Production in Foods by Staphylococcus aureus Strains That Produce More Than One Enterotoxin. J. Food Prot. 56, 538–540. doi: 10.4315/0362-028x-56.6.538
Mason, A., Foster, D., Bradley, P., Golubchik, T., Doumith, M., Gordon, N. C., et al. (2018). Accuracy of Different Bioinformatics Methods in Detecting Antibiotic Resistance and Virulence Factors from Staphylococcus aureus Whole-Genome Sequences. J. Clin. Microbiol. 56, 1–12. doi: 10.1128/JCM.01815-17
Mehrotra, M., Wang, G., and Johnson, W. M. (2000). Multiplex PCR for Detection of Genes for Staphylococcus aureus Enterotoxins, Exfoliative Toxins, Toxic Shock Syndrome Toxin 1, and Methicillin Resistance. J. Clin. Microbiol. 38, 1032–1035. doi: 10.1128/JCM.38.3.1032-1035.2000
Merda, D., Felten, A., Vingadassalon, N., Denayer, S., Titouche, Y., Decastelli, L., et al. (2020). NAuRA: Genomic Tool to Identify Staphylococcal Enterotoxins in Staphylococcus aureus Strains Responsible for FoodBorne Outbreaks. Front. Microbiol. 11, 1–12. doi: 10.3389/fmicb.2020.01483
Monday, S. R., and Bohach, G. A. (1999). Use of Multiplex PCR To Detect Classical and Newly Described Pyrogenic Toxin Genes in Staphylococcal Isolates. J. Clin. Microbiol. 37, 3411–3414. doi: 10.1128/JCM.37.10.3411-3414.1999
Moore, G., Cookson, B., Gordon, N. C., Jackson, R., Kearns, A., Singleton, J., et al. (2015). Whole-genome sequencing in hierarchy with pulsed-field gel electrophoresis: the utility of this approach to establish possible sources of MRSA cross-transmission. J. Hosp. Infect. 90, 38–45. doi: 10.1016/j.jhin.2014.12.014
Murchan, S., Kaufmann, M. E., Deplano, A., de Ryck, R., Struelens, M., Zinn, C. E., et al. (2003). Harmonization of Pulsed-Field Gel Electrophoresis Protocols for Epidemiological Typing of Strains of Methicillin-Resistant Staphylococcus aureus: a Single Approach Developed by Consensus in 10 European Laboratories and Its Application for Tracing the Spr. J. Clin. Microbiol. 41, 1574–1585. doi: 10.1128/JCM.41.4.1574-1585.2003
Nagaraj, S., Ramlal, S., Sripathy, M. H., and Batra, H. V. (2014). Development and evaluation of a novel combinatorial selective enrichment and multiplex PCR technique for molecular detection of major virulence-associated genes of enterotoxigenic Staphylococcus aureus in food samples. J. Appl. Microbiol. 116, 435–446. doi: 10.1111/jam.12364
Nouws, S., Bogaerts, B., Verhaegen, B., Denayer, S., Crombé, Fl, De Rauw, K., et al. (2020a). The Benefits of Whole Genome Sequencing for Foodborne Outbreak Investigation from the Perspective of a National Reference Laboratory in a Smaller Country. Foods 9:1030. doi: 10.3390/foods9081030
Nouws, S., Bogaerts, B., Verhaegen, B., Denayer, S., Piérard, D., Marchal, K., et al. (2020b). Impact of DNA extraction on whole genome sequencing analysis for characterization and relatedness of Shiga toxin-producing Escherichia coli isolates. Sci. Rep. 10:14649. doi: 10.1038/s41598-020-71207-3
Omoe, K., Hu, D.-L., Takahashi-Omoe, H., Nakane, A., and Shinagawa, K. (2003). Identification and Characterization of a New Staphylococcal Enterotoxin-Related Putative Toxin Encoded by Two Kinds of Plasmids. Infect. Immun. 71, 6088–6094. doi: 10.1128/IAI.71.10.6088-6094.2003
Ono, H. K., Omoe, K., Imanishi, K., Iwakabe, Y., Hu, D. L., Kato, H., et al. (2008). Identification and characterization of two novel staphylococcal enterotoxins, types S and T. Infect. Immun. 76, 4999–5005. doi: 10.1128/IAI.00045-08
Pasquali, F., Do Valle, I., Palma, F., Remondini, D., Manfreda, G., Castellani, G., et al. (2019). Application of different DNA extraction procedures, library preparation protocols and sequencing platforms: impact on sequencing results. Heliyon 5:e02745. doi: 10.1016/j.heliyon.2019.e02745
Peacock, S. J., and Paterson, G. K. (2015). Mechanisms of Methicillin Resistance in Staphylococcus aureus. Annu. Rev. Biochem. 84, 577–601. doi: 10.1146/annurev-biochem-060614-034516
Petit, R. A., and Read, T. D. (2018). Staphylococcus aureus viewed from the perspective of 40,000+ genomes. PeerJ 6:e5261. doi: 10.7717/peerj.5261
Price, J. R., Didelot, X., Crook, D. W., Llewelyn, M. J., and Paul, J. (2013). Whole genome sequencing in the prevention and control of Staphylococcus aureus infection. J. Hosp. Infect. 83, 14–21. doi: 10.1016/j.jhin.2012.10.003
Rambaut, A. (2007). FigTree. Available online at: http://tree.bio.ed.ac.uk/software/figtree/ (accessed date 2018-November-25)
Roussel, S., Felix, B., Vingadassalon, N., Grout, J., Hennekinne, J.-A., Guillier, L., et al. (2015). Staphylococcus aureus strains associated with food poisoning outbreaks in France: comparison of different molecular typing methods, including MLVA. Front. Microbiol. 6, 1–12. doi: 10.3389/fmicb.2015.00882
Sabat, A. J., Hermelijn, S. M., Akkerboom, V., Juliana, A., Degener, J. E., Grundmann, H., et al. (2017). Complete-genome sequencing elucidates outbreak dynamics of CA-MRSA USA300 (ST8-spa t008) in an academic hospital of Paramaribo, Republic of Suriname. Sci. Rep. 7:41050. doi: 10.1038/srep41050
Sayers, S., Li, L., Ong, E., Deng, S., Fu, G., Lin, Y., et al. (2019). Victors: a web-based knowledge base of virulence factors in human and animal pathogens. Nucleic Acids Res. 47, D693–D700. doi: 10.1093/nar/gky999
Schmitz, F.-J., Steiert, M., Hofmann, B., Verhoef, J., Hadding, U., Heinz, H.-P., et al. (1998). Development of a multiplex-PCR for direct detection of the genes for enterotoxin B and C, and toxic shock syndrome toxin-1 in Staphylococcus aureus isolates. J. Med. Microbiol. 47, 335–340. doi: 10.1099/00222615-47-4-335
Schouls, L. M., Spalburg, E. C., van Luit, M., Huijsdens, X. W., Pluister, G. N., van Santen-Verheuvel, M. G., et al. (2009). Multiple-Locus Variable Number Tandem Repeat Analysis of Staphylococcus Aureus: Comparison with Pulsed-Field Gel Electrophoresis and spa-Typing. PLoS One 4:e5082. doi: 10.1371/journal.pone.0005082
Schwendimann, L., Merda, D., Berger, T., Denayer, S., Feraudet-Tarisse, C., Kläui, A. J., et al. (2021). Staphylococcal Enterotoxin Gene Cluster: Prediction of Enterotoxin (SEG and SEI) Production and of the Source of Food Poisoning on the Basis of v Saβ Typing. Appl. Environ. Microbiol. 87, 2662–2620. doi: 10.1128/AEM.02662-20
Sharma, N. K., Rees, C. E. D., and Dodd, C. E. R. (2000). Development of a Single-Reaction Multiplex PCR Toxin Typing Assay for Staphylococcus aureus Strains. Appl. Environ. Microbiol. 66, 1347–1353. doi: 10.1128/AEM.66.4.1347-1353.2000
Shylaja, R., Murali, H. S., Batra, H. V., and Bawa, A. S. (2010). A novel multiplex PCR system for the detection of Staphylococcal enterotoxin B, tsst, nuc and fem genes of Staphylococcus aureus in food system. J. Food Saf. 30, 443–454. doi: 10.1111/j.1745-4565.2010.00218.x
Spaulding, A. R., Salgado-Pabón, W., Kohler, P. L., Horswill, A. R., Leung, D. Y. M., and Schlievert, P. M. (2013). Staphylococcal and Streptococcal Superantigen Exotoxins. Clin. Microbiol. Rev. 26, 422–447. doi: 10.1128/CMR.00104-12
Thomas, D. Y., Jarraud, S., Lemercier, B., Cozon, G., Echasserieau, K., Etienne, J., et al. (2006). Staphylococcal Enterotoxin-Like Toxins U2 and V, Two New Staphylococcal Superantigens Arising from Recombination within the Enterotoxin Gene Cluster. Infect. Immun. 74, 4724–4734. doi: 10.1128/IAI.00132-06
Umeda, K., Nakamura, H., Yamamoto, K., Nishina, N., Yasufuku, K., Hirai, Y., et al. (2017). Molecular and epidemiological characterization of staphylococcal foodborne outbreak of Staphylococcus aureus harboring seg, sei, sem, sen, seo, and selu genes without production of classical enterotoxins. Int. J. Food Microbiol. 256, 30–35. doi: 10.1016/j.ijfoodmicro.2017.05.023
Vanneste, K., Garlant, L., Broeders, S., Van Gucht, S., and Roosens, N. H. (2018). Application of whole genome data for in silico evaluation of primers and probes routinely employed for the detection of viral species by RT-qPCR using dengue virus as a case study. BMC Bioinformatics 19:312. doi: 10.1186/s12859-018-2313-0
Wildemauwe, C., Godard, C., Verschraegen, G., Claeys, G., Duyck, M.-C., De Beenhouwer, H., et al. (2004). Ten years phage-typing of Belgian clinical methicillin-resistant Staphylococcus aureus isolates (1992–2001). J. Hosp. Infect. 56, 16–21. doi: 10.1016/j.jhin.2003.09.014
Wilson, G. J., Tuffs, S. W., Wee, B. A., Seo, K. S., Park, N., Connelley, T., et al. (2018). Bovine Staphylococcus aureus Superantigens Stimulate the Entire T Cell Repertoire of Cattle. Infect. Immun. 86, 1–16. doi: 10.1128/IAI.00505-18
Ye, J., Coulouris, G., Zaretskaya, I., Cutcutache, I., Rozen, S., and Madden, T. L. (2012). Primer-BLAST: A tool to design target-specific primers for polymerase chain reaction. BMC Bioinformatics 13:134. doi: 10.1186/1471-2105-13-134
Zhang, D.-F., Yang, X.-Y., Zhang, J., Qin, X., Huang, X., Cui, Y., et al. (2018). Identification and characterization of two novel superantigens among Staphylococcus aureus complex. Int. J. Med. Microbiol. 308, 438–446. doi: 10.1016/j.ijmm.2018.03.002
Zhang, S., Iandolo, J. J., and Stewart, G. C. (1998). The enterotoxin D plasmid of Staphylococcus aureus encodes a second enterotoxin determinant (sej). FEMS Microbiol. Lett. 168, 227–233. doi: 10.1016/S0378-1097(98)00423-6
Zhao, J., Carmody, L. A., Kalikin, L. M., Li, J., Petrosino, J. F., Schloss, P. D., et al. (2012). Impact of Enhanced Staphylococcus DNA Extraction on Microbial Community Measures in Cystic Fibrosis Sputum. PLoS One 7:e33127. doi: 10.1371/journal.pone.0033127
Keywords: whole genome sequencing, Staphylococcus aureus, staphylococcal food poisoning (SFP), outbreak investigation, enterotoxin gene profiling, relatedness determination, DNA extraction kit
Citation: Nouws S, Bogaerts B, Verhaegen B, Denayer S, Laeremans L, Marchal K, Roosens NHC, Vanneste K and De Keersmaecker SCJ (2021) Whole Genome Sequencing Provides an Added Value to the Investigation of Staphylococcal Food Poisoning Outbreaks. Front. Microbiol. 12:750278. doi: 10.3389/fmicb.2021.750278
Received: 30 July 2021; Accepted: 04 October 2021;
Published: 02 November 2021.
Edited by:
Caroline Barretto, Nestle (Switzerland), SwitzerlandReviewed by:
Roger Stephan, University of Zurich, SwitzerlandValentine Usongo, Health Canada, Canada
Hisaya Ono, Kitasato University, Japan
Copyright © 2021 Nouws, Bogaerts, Verhaegen, Denayer, Laeremans, Marchal, Roosens, Vanneste and De Keersmaecker. This is an open-access article distributed under the terms of the Creative Commons Attribution License (CC BY). The use, distribution or reproduction in other forums is permitted, provided the original author(s) and the copyright owner(s) are credited and that the original publication in this journal is cited, in accordance with accepted academic practice. No use, distribution or reproduction is permitted which does not comply with these terms.
*Correspondence: Sigrid C. J. De Keersmaecker, U2lncmlkLkRlS2VlcnNtYWVja2VyQFNjaWVuc2Fuby5iZQ==