- 1MOE Key Lab of Environment and Health, Department of Epidemiology and Biostatistics, School of Public Health, Tongji Medical College, Huazhong University of Science and Technology, Wuhan, China
- 2Department of Cancer Prevention and Control, Hunan Cancer Hospital/The Affiliated Cancer Hospital of Xiangya School of Medicine, Central South University, Changsha, China
- 3Department of Nuclear Medicine, Union Hospital, Tongji Medical College, Huazhong University of Science and Technology, Wuhan, China
- 4Department of Nutrition and Food Hygiene, School of Public Health, Tongji Medical College, Huazhong University of Science and Technology, Wuhan, China
- 5Department of Rehabilitation Medicine, Union Hospital, Tongji Medical College, Huazhong University of Science and Technology, Wuhan, China
Objective: This study aimed to explore the relationships between the common variants of R-spondin/Wnt signaling genes, gut microbiota composition, and osteoporosis (OP) risk in elderly Chinese Han population.
Design: Dual-energy X-ray absorptiometry was used to obtain the OP-associated measurements at multiple skeleton sites among all 1,168 participants. Genotyping data was obtained by using the next-generation sequencing in the discovery stage (n = 400, 228 OP patients) and SNPscan technology in the replication stage (n = 768, 356 OP patients). Bioinformatic analysis was performed to provide more evidence for the genotype-OP associations. The 16S ribosomal RNA gene high-throughput sequencing technology was adopted to explore OP-associated gut microbiota variations.
Results: The genetic variants of rs10920362 in the LGR6 gene (P-FDR = 1.19 × 10–6) and rs11178860 in the LGR5 gene (P-FDR = 1.51 × 10–4) were found to associate with OP risk significantly. Several microbial taxa were associated with the BMDs and T-scores at multiple skeleton sites. The associations between rs10920362 and BMD-associated microbiota maintained significance after adjusting confounders. The rs10920362 CT/TT genotype associated with a decreased relative abundance of Actinobacteria (β = −1.32, P < 0.001), Bifidobacteriaceae (β = −1.70, P < 0.001), and Bifidobacterium (β = −1.70, P < 0.001) compared to the CC genotype.
Conclusion: Our findings suggested that the variants loci of LGR6 may be associate with OP pathogenesis via gut microbiota modifications. The relationship between host genetics and gut microbiome provides new perspectives about OP prevention and treatment.
Introduction
Osteoporosis (OP) is a metabolic bone disease characterized by low bone mineral density (BMD) and microarchitectural deterioration of bony tissue, symptoms of which occur to one in five women over 70 years and two-thirds over 90 years (Kamiński et al., 2018). In the human body, bone metabolism is a dynamic process under strict and complex regulation affected by host genetic factors, environmental factors and lifestyle (Agas et al., 2013). A growing number of genome-wide association studies (GWASs) and SNPs studies demonstrated that genes in the R-spondin/wingless-related integration site (Wnt) signaling pathway associated with the risks of BMD reduction, OP and fracture (Baron and Gori, 2018; Correa-Rodríguez, 2018; Li et al., 2020). The leucine-rich repeat containing G protein-coupled receptor 5/6 (LGR5/6) are the most thoroughly studied members of the OP susceptibility genes in R-spondin/Wnt signaling pathway. LGR5/6 function as receptors of the R-spondin family to regulate bone metabolism by potentiating Wnt/b-catenin signaling (Liu et al., 2019). LGR6-deficient mice showed nail and bone regeneration defect (Liu et al., 2019). In addition to genetic influences on OP, various data from human and animal studies have suggested that gut microbiota plays an indispensable role in regulating bone metabolism (Parvaneh et al., 2015; Yan et al., 2016; Schwarzer et al., 2018). Several taxa with altered abundance and specific functional pathways were found in low-BMD individuals from our previous study (Li et al., 2019). Similarly, another study showed that Bifidobacterium longum supplementation could increase bone formation, decrease bone resorption parameters (Parvaneh et al., 2015). Anyhow, these risk factors for OP have been thoroughly studied but are still far from being comprehensively understood.
Recently, many converging lines of evidence suggested that host genetics contribute to gut microbiota composition (Rawls et al., 2006; Parvaneh et al., 2015; Lim et al., 2017; Shukla et al., 2017; Kurilshikov et al., 2021), even through this way to affect the disease susceptibility. For instance, Rawls et al. (2006) discovered that the diversities between zebrafish and mice microbiota were caused by the differences of the underlying host genetics. Besides, one microbiome GWAS (mGWAS) study conducted by Blekhman et al. (2015) showed that the host genetic variation in immunity-related pathways was significantly associated with microbiome composition. Similarly, host SNPs were identified to have a great impact on the microbial abundance in another mGWAS (Davenport et al., 2015). It has been reported that specific host genetic variation may contribute to the development of metabolic syndrome by mediating gut microbial abundance and diversity (Lim et al., 2017). Interestingly, data from several studies suggested that R-spondin/Wnt signaling networks played a vital role in intestinal development and maintenance of intestinal homeostasis, as well as the development and differentiation of immune cells (Staal et al., 2008; Clevers and Nusse, 2012; Hayase et al., 2017). And beyond that, several functional studies have reported the importance of Wnt signaling in the gut microbiota–bone axis (Tyagi et al., 2018; Chen et al., 2020). Significantly, LGR5/6 can promote R-spondin-mediated Wnt/PCP and Wnt/β-catenin signaling (Liu et al., 2019). Considering that both host genes and the microbiome influence the development of the OP, we hypothesized that host genetic variants in R-spondin/Wnt signaling pathway, especially the LGR5/6 genes, might partly regulate bone metabolism by mediating some microbe abundance. However, no information has yet been available on the interrelationships among host genetics, gut microbiota, and OP risk. Thus, what becomes particularly important is the identification of associations between specific genetic variants and microbes involving this process.
Herein, to some degree, the present study attempted to fill the gaps of this knowledge that R-spondin/Wnt signaling networks genetic variants contribute to OP risk via controlling gut microbiota composition. Therefore, we adopted the next-generation sequencing (NGS), SNPscan and 16S ribosomal RNA (rRNA) gene high-throughput sequencing technology to conduct an integrative analysis of LGR5/6 gene variants, gut microbiota composition, and OP risk based on a Han population of central China.
Materials and Methods
Study Design
The detailed analyses process was shown in Figure 1. In the present research, we first performed the targeted NGS to the R-spondin/Wnt pathway genes in 400 Chinese participants (228 OP patients) and replicated the findings using SNPsacn technology among another independent cohort of 768 individuals (356 OP patients) in the same area. Bioinformatic analysis was then performed referring to the HaploReg, SNPinfo, 3DSNP, and Genotype-Tissue Expression (GTEx) data to provide more evidence for the OP-genotype associations. Third, the 16S rRNA gene high-throughput sequencing technology was adopted to explore OP-associated gut microbiota variations. Finally, integrative analysis was conducted combining the genotyping and microbiome data to clarify potential genome-microbiome associations in OP pathogenesis.
Participants and Baseline Data Collection
The study involved a total of 1,168 Han Chinese participants aged ≥60 years recruited at two communities in Wuhan city and the Wuhan Union Hospital during 2016–2018. The exclusion criteria were as follows: (1) with other endocrine diseases (e.g., hyperthyroidism, hypothyroidism, etc.) that influences bone metabolism; (2) of surgical menopause (i.e., hysterectomy and/or ovariectomy); and (3) taking medicines affecting bone health such as hormones. Moreover, individuals were further excluded before stool collection if they met the following criterion: (1) use of antibiotics within 1 month before fecal sample collection; and (2) with prevalent diseases of diabetes and gastrointestinal diseases.
The covariates have been described in our previous study (Li et al., 2020). Briefly, body mass index (BMI) and data of self-reported menstrual history, lifestyle factors, prevalent diseases, and medication history were collected. This study was approved by the Ethics Committee of Tongji Medical College of Huazhong University of Science and Technology. Informed consent was obtained from all participants before enrollment.
Bone Mineral Density Measurement
Dual-energy X-ray absorptiometry (Lunar Prodigy, GE, United States) was used to obtain BMD measurements, including values of BMD (expressed in g/cm2), T-score, and Z-score, at skeleton sites of the lumbar spine (LS) and total hip among all participants. A T-score ≤ −2.5 indicated prevalent osteoporosis referring to the World Health Organization criteria (Chen et al., 2020). Subjects with T-score ≤ −2.5 were grouped into OP patients group, and those with T-score > -2.5 were classified into the controls.
Genotype Data
Details of venous blood sample collection, genome DNA extraction and gene sequencing methods as NGS and SNPscan were available in one of our studies (Li et al., 2020). Briefly speaking, the targeted NGS was used in the discovery stage (N = 400 and 228 OP patients) to reveal the OP-associated genetic variants located in the target regions of R-spondin/Wnt pathway genes including RSPO1, RSPO2, RSPO3, RSPO4, LRP5, LRP6, LGR4, LGR5, and LGR6. Then in the replication stage, the SNPscanTM Kit (Genesky Biotechnologies Inc., Suzhou, China) was used for sequencing of the observed genetic variants in association with OP risk in an independent cohort (N = 768 and 356 OP patients). Since limited numbers of sample sizes in our study, we only involved common genetic variants (Minor Allele Frequency > 0.05) for analysis in the present study.
Bioinformatic Analysis of Single Nucleotide Polymorphisms Function
The identified OP-associated SNPs and their LD proxies (r2 ≥ 0.8) were annotated for potential regulatory function by HaploReg v4.1 (Ward and Kellis, 2016). The FuncPred tool was used for functional SNPs prediction in the SNPinfo web server (Xu and Taylor, 2009). Three-dimensional (3D) chromatin looping data (Lu et al., 2017) were used to link promising SNPs to their three-dimensional interacting genes. Expression quantitative trait loci (eQTL) and splicing quantitative trait loci (sQTL) analysis were conducted on the SNPs using the GTEx project (Carithers and Moore, 2015).
Intestinal Flora Data
The high-throughput 16S rRNA gene sequencing method was used to detect the microbiota of fecal samples collected from eligible participants in this study. Details of fecal sample collection, DNA extraction, and PCR amplification were described in one previous study (Li et al., 2019). Briefly, fecal samples were collected and stored at −80°C until further processing. The fecal microbial DNA was extracted using the QIAamp DNA Stool Mini Kit (Qiagen, Hilden, Germany). PCR amplification was carried out to the V3–V4 hypervariable region of the 16S rRNA gene with bacterial genomic DNA as template. Then, the PCR products were pooled and purified with Agencourt AMPure XP magnetic beads (Beckman Coulter, United States) using the TopTaq DNA Polymerase kit (Transgen, China). PCR products were sequenced using the Illumina Miseq platform with the 2 × 250 bp paired-end method after the library was quantified, mixed, and quality checked. To obtain clean reads, we firstly used TrimGalore1 to filter raw reads at Q20 and adapter sequence and removed the reads with length <100 bp. Then, FLASH2 (Magoč and Salzberg, 2011) was used to merge pairs of reads from the original DNA fragments. We further removed the low-quality sequences after merging. After that, Mothur (Schloss et al., 2009) was used to remove primers in sequence, and sequences including N-base/homopolymer > 6 bp. Reads with an error rate of >2 and reads with length <100 bp were removed using USEARCH2 to obtain clean reads for further analyses. Operational taxonomic units (OTUs) were assigned by clustering the sequences with a threshold of 97% pairwise identity and chimeras were removed using UPARSE (Edgar, 2013). OTUs were taxonomically assigned at a confidence threshold of 80% based on the Ribosomal Database Project database by Mothur.
Statistical Analyses
The Pearson χ2 test was used to analyze the between-group differences of categorical variables (e.g., sex, smoking, and drinking), which were expressed as percentages. Two independent-sample t-test was used to analyze the between-group differences of variables of a normal distribution, which were expressed as mean ± standard deviation (SD). Mann–Whitney U test was used to analyze the between-group differences of continuous variables not conforming to a normal distribution, which were presented as median [interquartile range (IQR)]. To assess the beta diversity, a principal coordinate analysis (PCoA) based on the weighted UniFrac was performed for the visualization of the microbiome structure separation across groups. Statistical significance was confirmed using Permutational MANOVA (PERMANOVA). The linear discriminant analysis (LDA) effect size (LEfSe) was performed to identify the species accounting for the differences between groups, where the species with LDA values > 2.5 at a P-value < 0.05 were considered significantly enriched. Logistic regression modeling was performed to assess the associations of sequenced genetic variants with OP risk. Spearman’s correlation analysis was used to investigate the correlation between SNPs and gut microbiota. A generalized linear model based on negative binomial distribution was conducted to explore the relationship between SNPs and gut microbiota by taking the attributes of the microbiome (like the relative abundance of the microbiome) as the dependent variables, the genotypes in the host loci dominant model as the independent variables, and the gender, age, BMI, smoking, and drinking as covariates. The R software and SPSS version 23.0 were used for statistical analyses. A two-sided P < 0.05 was considered to achieve statistical significance.
Results
General Characteristics of the Subjects
The demographic characteristics of all participants were presented in Table 1. In the discovery and replication stages, the mean age of individuals in the cases with prevalent osteoporosis (OP) was slightly younger than the controls with normal BMD values. Taking the idea that women have high risk of OP prevalence into account, we involved all 400 females (228 cases and 172 controls) in the discovery stage of genotyping. And in the replication stage among 768 individuals, we also included males. It seems that the OP patients were likely to have larger BMI compared to controls. For the women studied, the cases and controls had similar mean menarche age (about 14 years old), as well as menopause age (about 49 years old). Generally speaking, the cases had higher proportions of smoking, and alcohol drinking, while lower proportions of self-reporting history of fracture and osteoarthritis compared to the controls though the differences achieved significance or not.
Associations Between Two Common Variants in LGR5/6 and Osteoporosis Risk
Four genetic models (i.e., the additive, dominant, co-dominant, and recessive models) were employed to analyze the association between the studied SNPs and OP risk, results of which were presented in Table 2. Combing the data from both stages, it was observed in the additive model that the rs10920362 T allele carriers had a significant increased risk of OP prevalence compared to the C allele carriers (OR = 1.78, 95% CI: 1.44–2.22, P-FDR = 1.19 × 10–6). The dominant model revealed that the CT/TT genotypes were associated with larger OP risk than the CC genotypes (OR = 1.86, 95% CI: 1.44–2.41, P-FDR = 2.50 × 10–5). And compared to the CT/CC genotypes, the TT genotype was significantly associated with an increased risk of OP prevalence (OR = 3.06, 95% CI: 1.71–5.49, P-FDR = 8.43 × 10–4). As to the rs11178860, an increased risk of OP was observed in individuals with the A allele compared with the individuals carrying the G allele (OR = 1.48, 95% CI: 1.23–1.78, P-FDR = 1.51 × 10–4). Importantly, the dominant and recessive models also showed results achieve significance. Moreover, the two common SNPs were also associated with the values of BMD measurements at skeleton sites of LS and total hip (Supplementary Table 1).
Annotation and Function Assessment of the Two Osteoporosis-Associated Genetic Variants
Referring to the predictions by HaploReg v4.1, the variant rs11178860 was a proxy (r2 > 0.8) of genetic variants locating in a variety of regulatory elements of “Promoter histone marks,” “Enhancer histone marks,” “Motifs changed,” “GRASP QTL hits,” and “Selected eQTL hits” (Supplementary Table 2). Besides, the SNP rs10920362 was predicted to function in regulatory elements of “DNAse,” “Enhancer histone mark,” “Proteins bound,” “Motifs changed,” “GRASP QTL hits,” and “Selected eQTL hits” (Supplementary Table 2). According to the functional analysis by SNPinfo, the rs10920362 was identified as “possibly damaging” with features of an exonic splicing enhancer or exonic splicing silencer (Supplementary Table 3). Transcription factor binding sites (TFBSs) were identified for the rs2304269 of strong LD with the rs11178860 (r2 = 0.814 in CHB) (Supplementary Table 3). The 3D chromatin looping data in bone demonstrated that the interacting genes of rs10920362 are LGR6 and SYT2, and rs10920362 was linked with 14 TFBSs (Supplementary Figure 1).
Based on the GTEx datasets, we analyzed the correlation between the two genetic variants (rs10920362 and rs11178860) and gene expressions in various tissues, results of which are shown in Supplementary Table 4. We observed potential associations between rs10920362 and the LGR6 gene expression in tissues of thyroid (P = 7.20 × 10–39), brain hypothalamus (P = 1.10 × 10–11), minor salivary gland (P = 2.10 × 10–9), artery tibial (P = 1.00 × 10–8), brain substantia nigra (P = 3.00 × 10–7), artery aorta (P = 4.20 × 10–7), and brain cortex (P = 4.30 × 10–5). We failed to find the rs11178860 and LGR5 gene expression association, but the sQTL data showed a potential association between rs10879301 (of large LD with rs11178860: r2 = 1.00 in CHB) and the splicing changes of the LGR5 gene in the muscle-skeletal tissue (Supplementary Figure 2).
Osteoporosis Risk-Associated Variations in Gut Microbiota
A total of 180 fecal samples from 77 OP patients and 103 controls were included in the gut microbiota abundance analysis (Supplementary Table 5). The OP group had comparatively smaller numbers of bacterial taxa at species, genus, family, order, class, and phylum levels than the control group (Supplementary Table 6). A total of 1,699 OTUs were quantified in this study, specifically, 1,288 in the OP group and 1,556 in the control group with 1,145 shared in both groups.
The PCoA of weighted UniFrac distance showed a significant difference between the OP and control groups (Supplementary Figure 3). The PC1 explained 46.64% of variation and the PC2 explained 10.53%. The PERMANOVA analysis also revealed that the two groups had a significant difference in beta diversity (F = 3.413, P = 1.0 × 10–4). LEfSe analysis revealed that the Bacteroidetes (phylum), Porphyromonadaceae (family), Bacteroidaceae (family), Parabacteroides (genus), and Bacteroides (genus) were enriched in the OP individuals; and the Firmicutes (phylum), Proteobacteria (phylum), Actinobacteria (phylum), Lachnospiraceae (family), Bifidobacteriaceae (family), Butyricicoccus (genus) and Bifidobacterium (genus) were enriched in the controls (Supplementary Figure 4). The Firmicutes, Bacteroidetes, Proteobacteria, and Actinobacteria mainly dominated at phylum level in gut microbiota of all samples. The proportion of Bacteroidetes was significantly larger in the OP group than that in the control group (P < 0.05), while those of the Firmicutes, Proteobacteria, and Actinobacteria were smaller in the former than the latter (all P < 0.05) (Table 3). At family level, the OP patients had a higher proportion of Bacteroidaceae and Porphyromonadaceae than the controls (both P < 0.05). At genus level, the Bacteroides and Parabacteroides accounted for a larger proportion in the case group than the controls (both P < 0.05). Spearman’s correlation analysis observed that levels of the Firmicutes, Proteobacteria, and Actinobacteria phylum, the Bifidobacteriaceae, Lactobacillaceae, Ruminococcaceae, and Ruminococcaceae families, and the Bifidobacterium, Lactobacillus, and Gemmiger genus correlated positively with the BMDs and T-scores among all subjects (Supplementary Table 7). Of note, the ridge regression model still showed significant associations between gut microbiota and skeleton site-specific BMD measurements after adjusting for age, sex, smoking, and BMI (Supplementary Table 8).
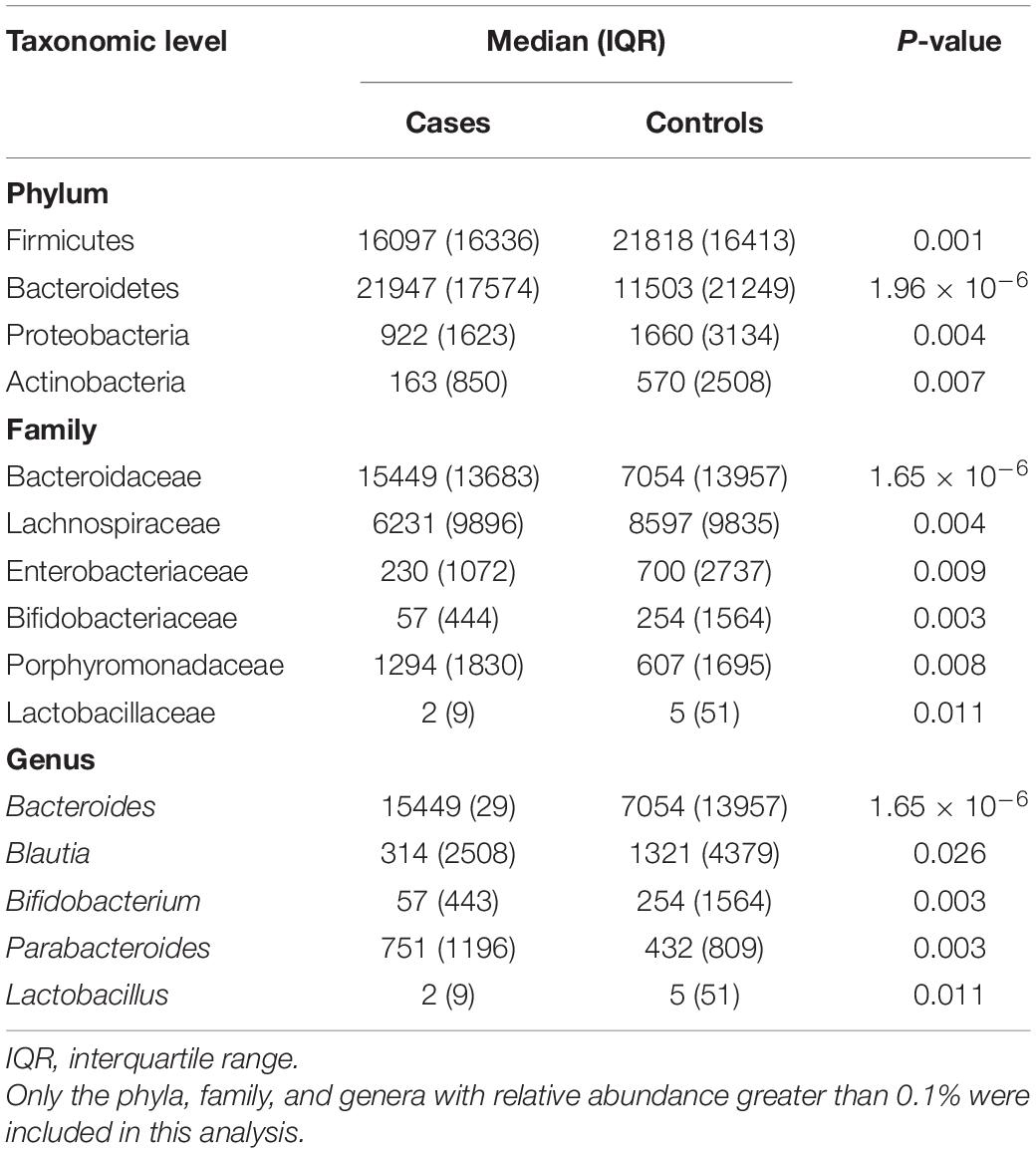
Table 3. Comparisons of gut microbiota composition at several taxonomic levels between the osteoporosis patients (cases) and controls.
Integrative Analysis of Osteoporosis-Associated Genetic Variants and Gut Microbiota
A total of 113 participants (53 OP patients) simultaneously with genetic and gut microbiota data were included in this part. A total of 1,521 OTUs were identified. The Venn diagram showed that the rs10920362 CC and CT/TT carriers shared 973 (64.0%) OTUs with 348 found only in the CC carriers and 200 found only in the CT/TT carriers (Figure 2). The CC carriers had more OTUs (1,321) than the CT/TT carriers (1,173). A total of 864 (56.8%) OTUs were shared across the rs11178860 GG and GA/AA genotypes with 569 OTUs only found in the GG genotype individuals and 88 found only in the GA/AA genotype individuals. The number of OTUs was larger in the GG genotypes (1,433) than the GA/AA genotypes (952).
LEfSe analysis revealed that the bacterial species belonging to phylum Actinobacteria, family Bifidobacteriaceae, and family Streptococcaceae were enriched in the LGR6 rs10920362 CC genotype. Compared to the LGR6 rs10920362 CT/TT genotype individuals, CC genotype individuals had a higher level of Bifidobacterium and Streptococcus (Figure 3). As for rs11178860, no significant differences were found.
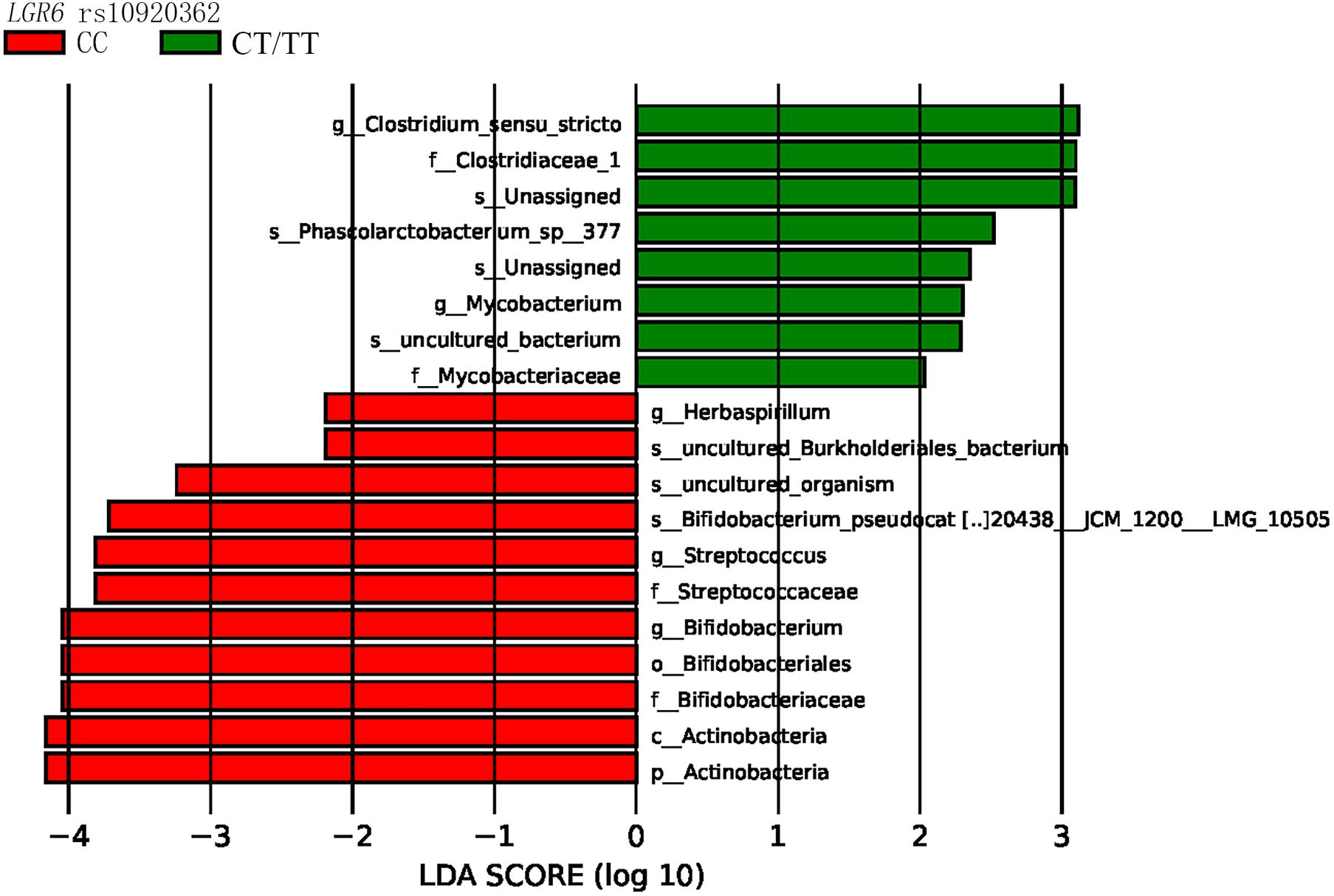
Figure 3. LEfSe indicating differences in the bacterial taxa in different rs10920362 genotypes (p, phylum; c, class; o, order; f, family; g, genus; and s, species), only the taxes having a P < 0.05, and LDA value > 2 are shown in the figure.
As data shown in further analyses, the proportion of Actinobacteria in the rs10920362 CT/TT genotype was significantly lower than that in the CC genotype at the phylum level (P = 0.005) (Table 4). At the family level, the individuals carrying CT/TT genotype had lower abundances of Bifidobacteriaceae compared to those with CC genotype (P = 0.002). At the genus level, individuals with CT/TT genotype had lower abundances of Bifidobacterium than individuals with CC genotype (P = 0.002). However, no significant differences in gut microbial community abundance were found between rs11178860 GG and GA/AA genotypes. Of note, the abundances of Actinobacteria, Bifidobacteriaceae, and Bifidobacterium were also positively correlated with BMD and T-score (Li et al., 2019).
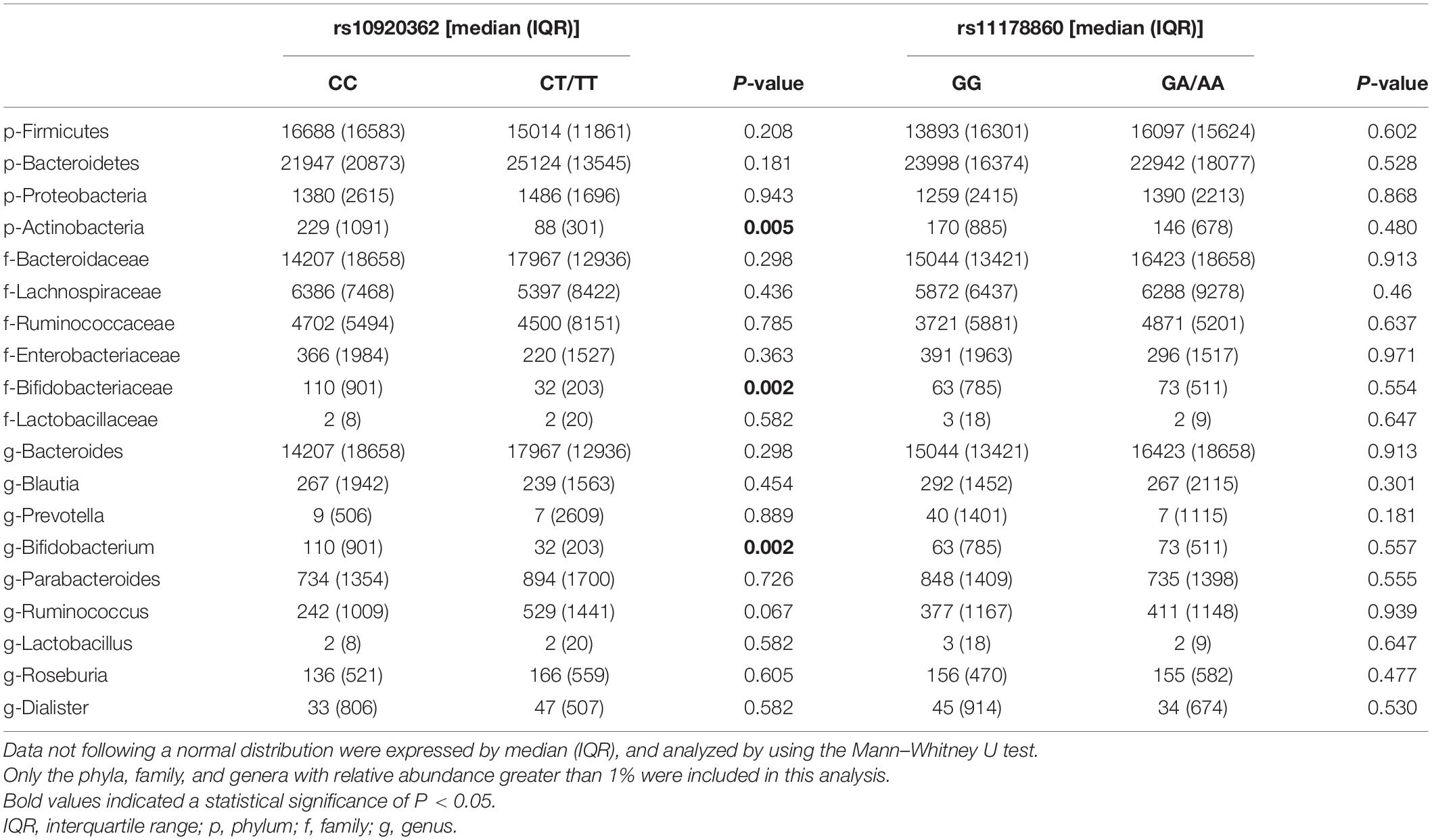
Table 4. Comparisons of gut microbiota composition stratified by the rs10920362 and rs11178860 genotypes.
Spearman’s correlation analysis showed that the abundances of Actinobacteria, Bifidobacteriaceae, and Bifidobacterium correlated negatively with the rs10920362 CT/TT genotype (all P < 0.05) (Table 5). No significant correlation was found between SNP rs11178860 and gut microbiota abundance.
Based on above results, we took the relative abundance of Actinobacteria, Bifidobacteriaceae, and Bifidobacterium as dependent variables, and the rs10920362 and rs11178860 genotypes as independent variables in the generalized linear modeling. Compared with the rs10920362 CC genotype, the CT/TT genotype associated with decreased relative abundance of Actinobacteria (β = −1.32, P < 0.001), Bifidobacteriaceae (β = −1.70, P < 0.001), and Bifidobacterium (β = −1.70, P < 0.001) controlling for sex, age, BMI, cigarette smoking, alcohol drinking, and self-reporting history of osteoarthritis (Table 6). No significant associations were found between the rs11178860 genotype and the relative abundance of above-mentioned gut microbiota.
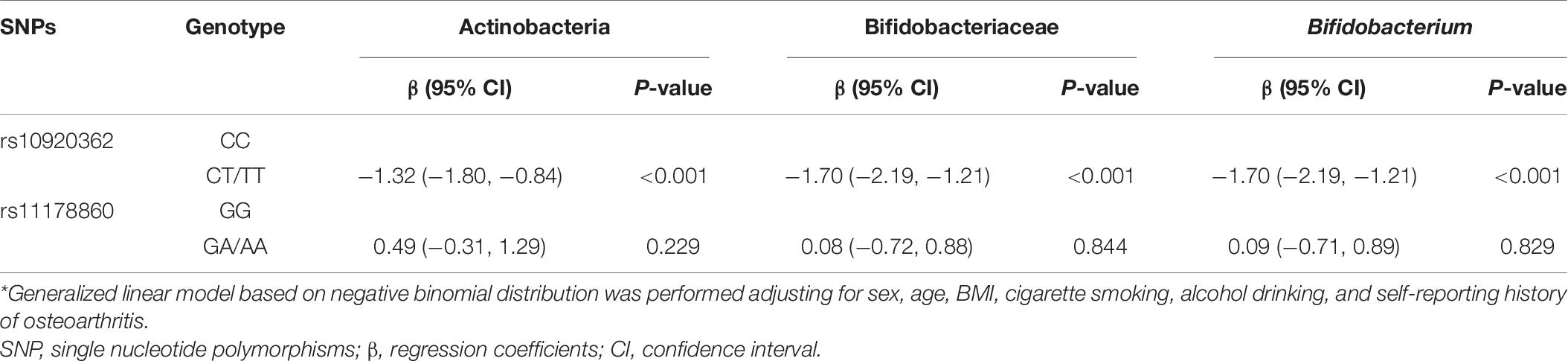
Table 6. Results of generalized linear model analysis of the dependency of gut microbiota abundance on the two genetic variants*.
Discussion
In this study, we conducted an integrative analysis of R-spondin/Wnt signaling network gene polymorphisms and gut microbiota composition in relation to the OP risk among a Han Chinese population in central China. As we knew, it was firstly reported that the genetic variants of rs11178860 in LGR5 gene and rs10920362 in LGR6 gene associated with OP prevalence risk among Chinese elderly individuals. Besides, the abundance of several bacterial taxa was revealed to be associated with OP prevalence risk and/or relevant disease traits as BMDs and T-scores at the studied skeleton sites. Importantly, we showed for the first time that the rs10920362 polymorphism was significantly associated with several BMD-associated microbial taxa including Actinobacteria, Bifidobacteriaceae, and Bifidobacterium. In short, we provided epidemiological evidence that two genetic variants in two genes of R-spondin/Wnt signaling networks contribute to OP risk possibly via biological influence on gut microbiota compositions.
The associations between the two common SNPs and OP risk were genuinely supported by evidence from previous functional studies (Cui et al., 2018; Lin et al., 2019) and bioinformatics analysis in our study. Specifically, the Haploreg predicted that mutations in the two gene loci (or those in high LD with them) can cause disrupted transcription factor binding sites (Ward and Kellis, 2016). That was also evidenced by predictions of the SNPinfo (Xu and Taylor, 2009) and 3DSNP (Lu et al., 2017) web servers. Consistently, the GTEx data suggested that rs10920362 act as an eQTL for the LGR6 gene across various tissues. Besides, the rs11178860 was a proxy of a genetic variant (the rs10879301 in LD with rs11178860) predicted to associate with the splicing changes of LGR5 gene in the muscle-skeletal tissue. Other functional studies also provided consistent findings. It was demonstrated that inhibition of LGR6 promoted the osteogenic differentiation of bone marrow stromal cells in vitro (Cui et al., 2018). Moreover, transplantation of LGR6-knockout bone marrow stromal cells in rat models contributed to better recovery after the fracture (Cui et al., 2018). Lin et al. (2019) showed that Lgr5 was implicated in the cellular processes of osteogenic differentiation of mesenchymal stem cells by regulating Wnt and ERK signaling pathways and mitochondrial dynamics in fusion and fission. Inhibition of Lgr5 expression induced elevations in mitochondrial fragmentation and suppression of osteogenesis (Lin et al., 2019). Taken together, the functional links of rs10920362 and rs11178860 with OP pathogenesis are biologically convincing. Furthermore, the consistent results of LGR6 rs10920362 and LGR5 rs11178860 in the discovery and replication stages supported the credibility of the findings. Genotype-phenotype associations between risk alleles and disease subtypes may provide insight into disease etiology and mechanisms. Intriguingly, the significant associations between the two common variants and BMDs at diverse skeleton sites suggested that our findings are tenable from other perspectives. Overall, multiple lines of evidence strengthened the effectiveness and credibility of the association between LGR6 rs10920362 and LGR5 rs11178860 and OP risk.
Our results showed that the rs10920362 polymorphism were also associated with the abundance of several BMD-associated microbial taxa like the Actinobacteria, Bifidobacteriaceae, and Bifidobacterium. Genetic variants associated with the quantitative traits of microbiome are defined as microbiome QTLs (mQTLs) (Bloomgarden, 2018; Cavadas et al., 2020). Several studies also reported the role of genetic variants affecting gut microbiota composition (Benson et al., 2010; McKnite et al., 2012; Goodrich et al., 2014). Animal studies revealed numerous loci associated with gut microbial community composition by using QTL-mapping approaches, some of which overlapped genes involved in immune response (Benson et al., 2010; McKnite et al., 2012). A metagenomic sequencing research to 1,514 individuals also evidenced associations between genetic variants and gut microbiome composition and function, indicating potential interactions between host genome and microbiome in human (Bonder et al., 2016). Several studies have identified mQTLs in relation to human diseases, including IBD, cancer, heart disease, and meningitis (Hillhouse et al., 2011; Snijders et al., 2016). These mQTLs can be regulated by genes involved in microbiome-related pathways, including the immune system, food metabolism, and drug-related systems. Interestingly, a functional study of Chen et al. (2020) found that the Clostridium butyricum, a butyrate-producing probiotics, can modulate Wnt signaling and gut microbiota. Similarly, another study of Tyagi et al. (2018) showed that the butyrate indirectly increased CD8+ T cell expression of Wnt10b via Treg cells, suggesting the importance of Wnt signaling in the gut microbiota–bone axis. Furthermore, LGR6 functioned as a receptor of RSPOs to potentiate Wnt/β-catenin signaling (Gong et al., 2012). Therefore, we believe it is reasonable to consider that the LGR6 gene is capable of regulating gut microbiota composition through some underlying mechanisms possibly relevant to particular mQTLs. However, regarding the identified association between rs10920362 and OP-associated gut microbiota, functional studies are still required to confirm a QTL effect of the LGR6 rs10920362 mutation and elucidate the molecular mechanism through which this QTL acts.
So far, the underlying mechanisms of gut microbiota variations in association with BMD decline still remain unclear. One reasonable explanation is that the overproduction of lipopolysaccharide from the gut microbiota possibly contributes to bone mass loss via inflammation-relevant pathways (Itoh et al., 2003). What is more, the high heritability of Bifidobacterium, Bifidobacteriaceae, and Actinobacteria has been demonstrated by several studies (Goodrich et al., 2014; Lim et al., 2017). Moreover, the functional study shows that a decreased number of Actinobacteria, especially the Bifidobacteria family (Duca et al., 2013), is associated with an enhancement of gut permeability, leading to the translocation of lipopolysaccharide into the serum. In addition, administration of Bifidobacterium pseudocatenulatum CECT 7765 along with a high-fat diet in mice can down-regulate the inflammation by reducing the production of inflammatory cytokines and chemokines (Cano et al., 2013), especially the TNF-α and IL-6. Of note, TNF-α and IL-6 contribute to reduced BMD (Zhao, 2018). Based on above evidence, it is safe to infer that the rs10920362 CT/TT genotype may increase the OP risk by reducing the abundance of Actinobacteria, Bifidobacteriaceae, and Bifidobacterium. It is also worth noting that the rs10920362 may be just a surrogate for some actually functional mQTLs in high LD with it. Overall, the result is of great encouragement and motivates future studies in this field to clarify the underlying biological mechanisms.
Our study is characterized by several strengths. First, it was first observed that two genetic variants as LGR5 rs11178860 and LGR6 rs10920362 were significantly associated with OP risk and/or BMDs and T-scores among Chinese elderly individuals. These findings provided new insights into R-Spondin/Wnt signaling pathway genes associated with the OP development. Second, to explore associations between gut microbiota composition and OP risk, our research took one step forward by analyzing more subjects. Third, we presented a systematic view of the associations among genetic variants, gut microbiota, and OP risk via integrative analysis of genome and microbiome data. We showed for the first time that the OP risk-related SNP (LGR6 rs10920362) also associated with the abundance of disease-associated microbial taxa (Actinobacteria, Bifidobacteriaceae, and Bifidobacterium), providing potential directions for individualized OP prevention and treatment. Fourth, considering that the composition of gut microbiota is dynamic, complicated, and affected by immutable and modifiable factors (like dietary, prevalent diseases, and medications) (von Martels et al., 2017), we adjusted for potential confounders including sex, age, BMI, smoking and alcohol drinking. Last but not least, all participants were of Han ethnicity recruited from nearby communities in Wuhan, minimizing the heterogeneity of microbiota composition and host genetic architecture due to geographical factors (Mancabelli et al., 2017; Timpson et al., 2018).
However, several limitations should also be concerned. First, our study is of a cross-sectional design, failing to confirm a causal relationship. We did not conduct in vitro laboratory or in vivo animal studies to clarify underlying mechanisms for our findings. Moreover, large-scale prospective studies and functional experiments are warranted to elucidate the causality among the genetic variants, alterations of gut microbiota, and OP pathogenesis. As well, comprehensive researches of high quality are still needed to explore OP-related microbiome QTLs. Second, the participants recruited in this study were Chinese residents living in Wuhan, limiting the generalization of the study findings to other regions and other ethnic populations. Third, we adopted relative abundance indices to quantify the microbial composition (without absolute abundance measures), which was possibly inadequate enough to clarify disease-related microbiome alterations.
Conclusion
Our data firstly supported that the LGR5 rs11178860 and LGR6 rs10920362 correlates with susceptibility of OP. Additionally, our comprehensive study of associations among R-spondin/Wnt signaling network gene polymorphisms, gut microbiota composition, and OP risk showed for the first time that the host genetic variant of rs10920362 in the LGR6 gene may contribute to OP pathogenesis by reducing the relative abundance of Actinobacteria, Bifidobacteriaceae, and Bifidobacterium. The exploration of the interaction between host genes and gut microbiota provides new perspectives for the individualized and precise prevention and treatment of OP. To achieve better efficiency of OP prevention and treatment, the prospective microbiome-targeted therapeutics should take host genetic factors into consideration.
Data Availability Statement
The data presented in the study are deposited in the National Center for Biotechnology Information (NCBI) Bioproject database with accession number PRJNA772039 (https://www.ncbi.nlm.nih.gov/bioproject/PRJNA772039).
Ethics Statement
The studies involving human participants were reviewed and approved by the Ethics Committee of Tongji Medical College of Huazhong University of Science and Technology. The patients/participants provided their written informed consent to participate in this study.
Author Contributions
QW, D-SD, CL, and YD had full access to the data in the study and take responsibility for the integrity of the data and the accuracy of the data analysis. QW, D-SD, and CL conceived the study. QW, D-SD, CL, and YD were responsible for conception of the study and drafted the manuscript. QW was responsible for design of the study. M-HW, S-SW, W-JS, H-LZ, YC, and R-YZ contributed to preparation and data analysis. QW, QH, D-SD, CL, and YD contributed to revision of the manuscript. All authors contributed to the interpretation of the data and critically reviewed the manuscript for publication. All authors read and approved the final manuscript.
Funding
This work was supported by the National Natural Science Foundation of China (Grant Numbers 72061137006 and 81573235), the Natural Science Foundation of Hubei Province (Grant Number 2019CFB647), and the Fundamental Research Funds for the Central Universities (Grant Number 2019kfyXKJC003).
Conflict of Interest
The authors declare that the research was conducted in the absence of any commercial or financial relationships that could be construed as a potential conflict of interest.
Publisher’s Note
All claims expressed in this article are solely those of the authors and do not necessarily represent those of their affiliated organizations, or those of the publisher, the editors and the reviewers. Any product that may be evaluated in this article, or claim that may be made by its manufacturer, is not guaranteed or endorsed by the publisher.
Supplementary Material
The Supplementary Material for this article can be found online at: https://www.frontiersin.org/articles/10.3389/fmicb.2021.765008/full#supplementary-material
Supplementary Figure 1 | Chromosome interactions and epigenetic signatures in bone related to the rs10920362 in 3DSNP database.
Supplementary Figure 2 | Association between the rs10879301 and the splicing changes of LGR5 gene in the muscle skeletal tissue.
Supplementary Figure 3 | Principal coordinate analysis (PCoA) based on weighted UniFrac distances comparing the bacterial community among the osteoporosis patients and controls groups.
Supplementary Figure 4 | LEfSe indicating differences in the bacterial taxa at different levels (p, phylum; c, class; o, order; f, family; g, genus; and s, species). Only the taxes having a P < 0.05, and LDA value > 2 are shown in the figure.
Supplementary Table 1 | Results of multiple linear regression analysis of the effects of the two top common variants on BMD measurements.
Supplementary Table 2 | The HaploReg v4.1 predictions for the proxies of genetic variants rs11178860 and rs10920362.
Supplementary Table 3 | Results of SNPinfo predictions for the proxies of genetic variants rs11178860 and rs10920362.
Supplementary Table 4 | Results of the GTEx predictions for the genetic variant of rs10920362 with respect to gene expressions in various tissues.
Supplementary Table 5 | Characteristics of included subjects for gut microbiota abundance analysis.
Supplementary Table 6 | Bacterial compositions between the case and control groups at different levels.
Supplementary Table 7 | Spearman correlation analysis between relative abundance of gut microbiota (at several taxonomic levels) and BMD measurements.
Supplementary Table 8 | Ridge regression analysis of the effects of relative abundance of gut microbiota on BMD measurements.
Footnotes
References
Agas, D., Sabbieti, M. G., and Marchetti, L. (2013). Endocrine disruptors and bone metabolism. Arch. Toxicol. 87, 735–751. doi: 10.1007/s00204-012-0988-y
Baron, R., and Gori, F. (2018). Targeting WNT signaling in the treatment of osteoporosis. Curr. Opin. Pharmacol. 40, 134–141. doi: 10.1016/j.coph.2018.04.011
Benson, A. K., Kelly, S. A., Legge, R., Ma, F., Low, S. J., Kim, J., et al. (2010). Individuality in gut microbiota composition is a complex polygenic trait shaped by multiple environmental and host genetic factors. Proc. Natl. Acad. Sci. U. S. A. 107, 18933–18938. doi: 10.1073/pnas.1007028107
Blekhman, R., Goodrich, J. K., Huang, K., Sun, Q., Bukowski, R., Bell, J. T., et al. (2015). Host genetic variation impacts microbiome composition across human body sites. Genome Biol. 16:191. doi: 10.1186/s13059-015-0759-1
Bloomgarden, Z. (2018). Diabetes and branched-chain amino acids: what is the link? J. Diabetes. 10, 350–352. doi: 10.1111/1753-0407.12645
Bonder, M. J., Kurilshikov, A., Tigchelaar, E. F., Mujagic, Z., Imhann, F., Vila, A. V., et al. (2016). The effect of host genetics on the gut microbiome. Nat. Genet. 48, 1407–1412. doi: 10.1038/ng.3663
Cano, P. G., Santacruz, A., Trejo, F. M., and Sanz, Y. (2013). Bifidobacterium CECT 7765 improves metabolic and immunological alterations associated with obesity in high-fat diet-fed mice. Obesity 21, 2310–2321. doi: 10.1002/oby.20330
Carithers, L. J., and Moore, H. M. (2015). The Genotype-Tissue Expression (GTEx) Project. Biopreserv. Biobank 13, 307–308. doi: 10.1089/bio.2015.29031.hmm
Cavadas, B., Camacho, R., Ferreira, J. C., Ferreira, R. M., Figueiredo, C., Brazma, A., et al. (2020). Gastric Microbiome Diversities in Gastric Cancer Patients from Europe and Asia Mimic the Human Population Structure and Are Partly Driven by Microbiome Quantitative Trait Loci. Microorganisms 8:1196. doi: 10.3390/microorganisms8081196
Chen, D., Jin, D., Huang, S., Wu, J., Xu, M., Liu, T., et al. (2020). Clostridium butyricum, a butyrate-producing probiotic, inhibits intestinal tumor development through modulating Wnt signaling and gut microbiota. Cancer Lett. 469, 456–467. doi: 10.1016/j.canlet.2019.11.019
Clevers, H., and Nusse, R. (2012). Wnt/β-catenin signaling and disease. Cell 149, 1192–1205. doi: 10.1016/j.cell.2012.05.012
Correa-Rodríguez, M. (2018). Schmidt Rio-Valle J, Rueda-Medina B. The RSPO3 gene as genetic markers for bone mass assessed by quantitative ultrasound in a population of young adults. Ann. Hum. Genet. 82, 143–149. doi: 10.1111/ahg.12235
Cui, Y., Huang, R., Wang, Y., Zhu, L., and Zhang, X. (2018). Down-regulation of LGR6 promotes bone fracture recovery using bone marrow stromal cells. Biomed. Pharmacother. 99, 629–637. doi: 10.1016/j.biopha.2017.12.109
Davenport, E. R., Cusanovich, D. A., Michelini, K., Barreiro, L. B., Ober, C., and Gilad, Y. (2015). Genome-Wide Association Studies of the Human Gut Microbiota. PLoS One 10:e0140301. doi: 10.1371/journal.pone.0140301
Duca, F. A., Sakar, Y., and Covasa, M. (2013). The modulatory role of high fat feeding on gastrointestinal signals in obesity. J. Nutr. Biochem. 24, 1663–1677. doi: 10.1016/j.jnutbio.2013.05.005
Edgar, R. C. (2013). UPARSE: highly accurate OTU sequences from microbial amplicon reads. Nat. Methods 10, 996–998. doi: 10.1038/nmeth.2604
Gong, X., Carmon, K. S., Lin, Q., Thomas, A., Yi, J., and Liu, Q. (2012). LGR6 is a high affinity receptor of R-spondins and potentially functions as a tumor suppressor. PLoS One 7:e37137. doi: 10.1371/journal.pone.0037137
Goodrich, J. K., Waters, J. L., Poole, A. C., Sutter, J. L., Koren, O., Blekhman, R., et al. (2014). Human genetics shape the gut microbiome. Cell 159, 789–799. doi: 10.1016/j.cell.2014.09.053
Hayase, E., Hashimoto, D., Nakamura, K., Noizat, C., Ogasawara, R., Takahashi, S., et al. (2017). R-Spondin1 expands Paneth cells and prevents dysbiosis induced by graft-versus-host disease. J. Exp. Med. 214, 3507–3518. doi: 10.1084/jem.20170418
Hillhouse, A. E., Myles, M. H., Taylor, J. F., Bryda, E. C., and Franklin, C. L. (2011). Quantitative trait loci in a bacterially induced model of inflammatory bowel disease. Mamm. Genome 22, 544–555. doi: 10.1007/s00335-011-9343-5
Itoh, K., Udagawa, N., Kobayashi, K., Suda, K., Li, X., Takami, M., et al. (2003). Lipopolysaccharide promotes the survival of osteoclasts via Toll-like receptor 4, but cytokine production of osteoclasts in response to lipopolysaccharide is different from that of macrophages. J. Immunol. 170, 3688–3695. doi: 10.4049/jimmunol.170.7.3688
Kamiński, A., Bogacz, A., Uzar, I., and Czerny, B. (2018). Association betweenGREM2 gene polymorphism with osteoporosis and osteopenia in postmenopausal women. Eur. J. Obstet. Gynecol. Reprod. Biol. 228, 238–242. doi: 10.1016/j.ejogrb.2018.07.009
Kurilshikov, A., Medina-Gomez, C., Bacigalupe, R., Radjabzadeh, D., Wang, J., Demirkan, A., et al. (2021). Large-scale association analyses identify host factors influencing human gut microbiome composition. Nat. Genet. 53, 156–165. doi: 10.1038/s41588-020-00763-1
Li, C., Huang, Q., Yang, R., Dai, Y., Zeng, Y., Tao, L., et al. (2019). Gut microbiota composition and bone mineral loss-epidemiologic evidence from individuals in Wuhan. China. Osteoporos Int. 30, 1003–1013. doi: 10.1007/s00198-019-04855-5
Li, C., Huang, Q., Yang, R., Guo, X., Dai, Y., Zeng, J., et al. (2020). Targeted next generation sequencing of nine osteoporosis-related genes in the Wnt signaling pathway among Chinese postmenopausal women. Endocrine 68, 669–678. doi: 10.1007/s12020-020-02248-x
Lim, M. Y., You, H. J., Yoon, H. S., Kwon, B., Lee, J. Y., Lee, S., et al. (2017). The effect of heritability and host genetics on the gut microbiota and metabolic syndrome. Gut 66, 1031–1038. doi: 10.1136/gutjnl-2015-311326
Lin, W., Xu, L., Pan, Q., Lin, S., Feng, L., Wang, B., et al. (2019). Lgr5-overexpressing mesenchymal stem cells augment fracture healing through regulation of Wnt/ERK signaling pathways and mitochondrial dynamics. FASEB J. 33, 8565–8577. doi: 10.1096/fj.201900082RR
Liu, S. L., Zhou, Y. M., Tang, D. B., Zhou, N., Zheng, W. W., Tang, Z. H., et al. (2019). LGR6 promotes osteogenesis by activating the Wnt/β-catenin signaling pathway. Biochem. Biophys. Res. Commun. 519, 1–7. doi: 10.1016/j.bbrc.2019.08.122
Lu, Y., Quan, C., Chen, H., Bo, X., and Zhang, C. (2017). 3DSNP: a database for linking human noncoding SNPs to their three-dimensional interacting genes. Nucleic Acids Res. 45, D643–D649. doi: 10.1093/nar/gkw1022
Magoč, T., and Salzberg, S. L. (2011). FLASH: fast length adjustment of short reads to improve genome assemblies. Bioinformatics 27, 2957–2963. doi: 10.1093/bioinformatics/btr507
Mancabelli, L., Milani, C., Lugli, G. A., Turroni, F., Ferrario, C., van Sinderen, D., et al. (2017). Meta-analysis of the human gut microbiome from urbanized and pre-agricultural populations. Environ. Microbiol. 19, 1379–1390. doi: 10.1111/1462-2920.13692
McKnite, A. M., Perez-Munoz, M. E., Lu, L., Williams, E. G., Brewer, S., Andreux, P. A., et al. (2012). Murine gut microbiota is defined by host genetics and modulates variation of metabolic traits. PLoS One 7:e39191. doi: 10.1371/journal.pone.0039191
Parvaneh, K., Ebrahimi, M., Sabran, M. R., Karimi, G., Hwei, A. N., Abdul-Majeed, S., et al. (2015). Probiotics (Bifidobacterium longum) Increase Bone Mass Density and Upregulate Sparc and Bmp-2 Genes in Rats with Bone Loss Resulting from Ovariectomy. Biomed. Res. Int. 2015:897639. doi: 10.1155/2015/897639
Rawls, J. F., Mahowald, M. A., Ley, R. E., and Gordon, J. I. (2006). Reciprocal gut microbiota transplants from zebrafish and mice to germ-free recipients reveal host habitat selection. Cell 127, 423–433. doi: 10.1016/j.cell.2006.08.043
Schloss, P. D., Westcott, S. L., Ryabin, T., Hall, J. R., Hartmann, M., Hollister, E. B., et al. (2009). Introducing mothur: open-source, platform-independent, community-supported software for describing and comparing microbial communities. Appl. Environ. Microbiol. 75, 7537–7541. doi: 10.1128/AEM.01541-09
Schwarzer, M., Strigini, M., and Leulier, F. (2018). Gut Microbiota and Host Juvenile Growth. Calcif. Tissue Int. 102, 387–405. doi: 10.1007/s00223-017-0368-y
Shukla, S. D., Budden, K. F., Neal, R., and Hansbro, P. M. (2017). Microbiome effects on immunity, health and disease in the lung. Clin. Transl. Immunol. 6:e133. doi: 10.1038/cti.2017.6
Snijders, A. M., Langley, S. A., Kim, Y. M., Brislawn, C. J., Noecker, C., Zink, E. M., et al. (2016). Influence of early life exposure, host genetics and diet on the mouse gut microbiome and metabolome. Nat. Microbiol. 2:16221. doi: 10.1038/nmicrobiol.2016.221
Staal, F. J., Luis, T. C., and Tiemessen, M. M. (2008). WNT signalling in the immune system: WNT is spreading its wings. Nat. Rev. Immunol. 8, 581–593. doi: 10.1038/nri2360
Timpson, N. J., Greenwood, C. M. T., Soranzo, N., Lawson, D. J., and Richards, J. B. (2018). Genetic architecture: the shape of the genetic contribution to human traits and disease. Nat. Rev. Genet. 19, 110–124. doi: 10.1038/nrg.2017.101
Tyagi, A. M., Yu, M., Darby, T. M., Vaccaro, C., Li, J. Y., Owens, J. A., et al. (2018). The Microbial Metabolite Butyrate Stimulates Bone Formation via T Regulatory Cell-Mediated Regulation of WNT10B Expression. Immunity 49, 1116–1131.e7. doi: 10.1016/j.immuni.2018.10.013
von Martels, J. Z. H., Sadaghian Sadabad, M., Bourgonje, A. R., Blokzijl, T., Dijkstra, G., Faber, K. N., et al. (2017). The role of gut microbiota in health and disease: in vitro modeling of host-microbe interactions at the aerobe-anaerobe interphase of the human gut. Anaerobe 44, 3–12. doi: 10.1016/j.anaerobe.2017.01.001
Ward, L. D., and Kellis, M. (2016). HaploReg v4: systematic mining of putative causal variants, cell types, regulators and target genes for human complex traits and disease. Nucleic Acids Res. 44, D877–D881. doi: 10.1093/nar/gkv1340
Xu, Z., and Taylor, J. A. (2009). SNPinfo: integrating GWAS and candidate gene information into functional SNP selection for genetic association studies. Nucleic Acids Res. 37, W600–W605. doi: 10.1093/nar/gkp290
Yan, J., Herzog, J. W., Tsang, K., Brennan, C. A., Bower, M. A., Garrett, W. S., et al. (2016). Gut microbiota induce IGF-1 and promote bone formation and growth. Proc. Natl. Acad. Sci. U. S. A. 113, E7554–E7563. doi: 10.1073/pnas.1607235113
Keywords: R-spondin/Wnt pathway, gut microbiota, osteoporosis, host genetics, LGR5, LGR6
Citation: Di D-s, Li C, Dai Y, Wei M-h, Wang S-s, Song W-j, Zhou H-l, Cui Y, Zhang R-y, Huang Q and Wang Q (2021) Integrative Analysis of LGR5/6 Gene Variants, Gut Microbiota Composition and Osteoporosis Risk in Elderly Population. Front. Microbiol. 12:765008. doi: 10.3389/fmicb.2021.765008
Received: 02 September 2021; Accepted: 13 October 2021;
Published: 02 November 2021.
Edited by:
Baolei Jia, Chung-Ang University, South KoreaReviewed by:
Hu T. Huang, Syapse, Inc., United StatesTeruyuki Sano, University of Illinois at Chicago, United States
Copyright © 2021 Di, Li, Dai, Wei, Wang, Song, Zhou, Cui, Zhang, Huang and Wang. This is an open-access article distributed under the terms of the Creative Commons Attribution License (CC BY). The use, distribution or reproduction in other forums is permitted, provided the original author(s) and the copyright owner(s) are credited and that the original publication in this journal is cited, in accordance with accepted academic practice. No use, distribution or reproduction is permitted which does not comply with these terms.
*Correspondence: Qi Wang, bHdxOTVAMTI2LmNvbQ==; Qin Huang, anVkeTExMzBAMTI2LmNvbQ==
†These authors have contributed equally to this work