- State Key Laboratory of Crop Stress Biology in Arid Areas, College of Life Sciences, Northwest A&F University, Xianyang, China
Root diameter and rooting depth lead to morphological and architectural heterogeneity of plant roots; however, little is known about their effects on root-associated microbial communities. Bacterial community assembly was explored across 156 samples from three rhizocompartments (the rhizosphere, rhizoplane, and endosphere) for different diameters (0.0–0.5 mm, 0.5–1.0 mm, 1.0–2.0 mm, and>2.0 mm) and depths (0–5 cm, 5–10 cm, 10–15 cm, and 15–20 cm) of soybean [Glycine max (L.) Merrill] root systems. The microbial communities of all samples were analyzed using amplicon sequencing of bacterial 16S rRNA genes. The results showed that root diameter significantly affected the rhizosphere and endosphere bacterial communities, while rooting depth significantly influenced the rhizosphere and rhizoplane bacterial communities. The bacterial alpha diversity decreased with increasing root diameter in all three rhizocompartments, and the diversity increased with increasing rooting depth only in the rhizoplane. Clearly, the hierarchical enrichment process of the bacterial community showed a change from the rhizosphere to the rhizoplane to the endosphere, and the bacterial enrichment was higher in thinner or deeper roots (except for the roots at a depth of 15–20 cm). Network analysis indicated that thinner or deeper roots led to higher bacterial network complexity. The core and keystone taxa associated with the specific root diameter class and rooting depth class harbored specific adaptation or selection strategies. Root diameter and rooting depth together affected the root-associated bacterial assembly and network complexity in the root system. Linking root traits to microbiota may enhance our understanding of plant root-microbe interactions and their role in developing environmentally resilient root ecosystems.
Introduction
Plant roots harbor a high diversity of microbiota in various root habitats, including the rhizosphere, rhizoplane, and endosphere (Edwards et al., 2015). The interactions between the root-associated microbiota and plants range from parasitism (detrimental to the host) to mutualism (mutually beneficial), and their outcome can be pivotal for plant performance (Berendsen et al., 2012; Bulgarelli et al., 2012). Studies have reported that plant root-associated microbes provide a plethora of functional capacities and influence plants by modulating root architecture, nutrient acquisition, or resistance to biotic and/or abiotic stressors (Panke-Buisse et al., 2014; Reinhold-Hurek et al., 2015; Vandenkoornhuyse et al., 2015; Verbon and Liberman, 2016). Recent studies further indicated that root phenotypic traits could lead to variations in microbial communities (Saleem et al., 2018; Pervaiz et al., 2020). Investigating the relationships between root traits and root-associated microbiota could improve the fundamental understanding of root-associated microbial assembly processes and associations between microorganisms and plants.
Root diameter and rooting depth are important root morphological and architectural traits that determine the spatial configuration of the root system, and roots of different diameters or depths could potentially differ in their physical, biochemical, and functional traits (Bardgett et al., 2014; Ma et al., 2018b; Liu et al., 2019; Germon et al., 2020; Pervaiz et al., 2020). Fine roots (root diameter<2 mm) enable plants to efficiently leverage photosynthetic carbon for exploring resource-rich soil patches (Hoeppner and Dukes, 2012), while coarse roots (root diameter>2 mm) mainly support the fine root network and plant structure to deliver and store nutrients (Ma et al., 2018a). Deeper roots enable the acquisition of water under drought (Debinski et al., 2010; Hoeppner and Dukes, 2012). In general, plant roots distribute more roots in superficial soil layers than in subsoil layers, so root length densities and surface area densities decrease with increasing soil depth (Hati et al., 2006). Microbes can affect plant growth by modifying root development and traits (Verbon and Liberman, 2016). Linking microbial communities to the root categories may increase our understanding of the microbial communities and their functions and variations in the context of soil and plant health.
To date, a few studies have investigated the obvious impact of root heterogeneities on microbial communities (Perez-Jaramillo et al., 2017; Yahara et al., 2019; Gray and Kernaghan, 2020). The fine, secondary, and primary roots of tobacco plants harbor distinct microbial communities (Saleem et al., 2016, 2018). Wang et al. (2017a) indicated that low root orders of poplar trees harbored higher bacterial diversity in the rhizosphere than high root orders. Yang et al. (2016) also reported a significant difference in microbial communities between shallow and deep roots of Jerusalem artichokes. However, the variation in the microbial communities along the root diameter and rooting depth gradients was not well profiled together.
The plant root microbiome is predominantly assembled from the surrounding soil. The assembly patterns of root-associated microbial communities have been shown with a three-step enrichment model from the rhizosphere soil to the root rhizoplane and to the plant endosphere (Lundberg et al., 2012; Edwards et al., 2015; Zhang et al., 2017). Briefly, a specialized community in the bulk soil is enriched toward the roots due to general nutrient gradients imposed by the roots and forms the rhizosphere microbiome. Then, a more specialized microbial community is further enriched on the rhizoplane following close microbe-host interactions. Finally, certain microbiota enter and inhabit the root interior, forming the endosphere microbiome. The successful colonization of microbes requires the capability to overcome the host immune system and abiotic stresses (Vorholt, 2012; Bulgarelli et al., 2013). The assembly patterns have been mainly studied among plant species or genotypes (Bulgarelli et al., 2012; Mendes et al., 2014; Xiong et al., 2021). Dissecting the enrichment and filtration patterns from rhizosphere to rhizoplane to endosphere can give us a more comprehensive insight into the microbial assembly process along the root diameter and root depth.
Soybean [Glycine max (L.) Merrill] is one of the most widely grown legume crops. Soybean roots with a taproot system are mainly distributed in the topsoil layer (0–20 cm depth) and have strong plasticity under different nutrient stresses and agricultural practices (Benjamin and Nielsen, 2006; Meister et al., 2014; Li et al., 2017). Although the root-associated microbiomes of soybean have been well studied (Madhaiyan et al., 2014; Sugiyama et al., 2014; Zhang et al., 2018), little is currently known about the relationships between the microbiomes and root diameter or rooting depth. In the present study, we conducted a detailed examination of the rhizosphere, rhizoplane, and endosphere bacterial communities along root diameter and rooting depth gradients using Illumina sequencing of the 16S rRNA gene. The objectives were to answer the following questions. (i) Do the root-associated bacterial communities exhibit different diversities along the root diameter or rooting depth gradients?, (ii) do root diameter and rooting depth together affect the enrichment of bacterial communities from the root rhizosphere to the endosphere?, and (iii) what associations exist among bacterial taxa in roots with different diameters or depths? Addressing these questions is essential for understanding the interactions between the microbial community and plant root systems, and we expect to provide a root diameter-dependent and rooting depth-dependent model for analyzing the microbial community structure in root systems.
Materials and Methods
Experimental Site and Sampling Methods
Sampling was conducted in September 2018 at the podding stage of soybean in an agricultural experimental field (34°18' N, 108°6' E) on the Guanzhong Plain of northwestern China, with an average yearly temperature of 14.6°C and a mean annual precipitation of 590.3 mm. Soil in the experimental area is classified as a Fimic Anthrosol, and the topsoil texture classification is loam (Harmonized World Soil Database, FAO Soil Taxonomic system). The experimental field was previously planted with soybeans. The soybean [Glycine max (L.) Merrill, Zhonghuang 13] studied in this experiment is a widely grown soybean cultivar in China. The intrarow and interrow plant spacing used was 40 cm. Conventional management practices using hand hoe with no fertilizer and pesticide inputs represented farmers’ normal practice for soybean production in the area. The plots were ploughed in June before cultivation. Weeds were removed with hand hoes as needed during soybean growth development. Appropriate irrigation was carried out at the branching stage and flowering stage of soybean. The amount of irrigation was set based on the accumulated experience of the local farmers during agricultural production and the precipitation in the study area.
Three random subplots (approximately 5×5 m each) were chosen, and the subplots were spaced 5 m apart to preclude interactions. Each soybean root was collected within a 20-cm distance from the main stem and within a 20-cm vertical profile depth according to the main distribution area of a soybean root system in our study. For each plot, a group of twenty fresh roots was collected from four soil layers (Layer I, 0–5 cm; Layer II, 5–10 cm; Layer III, 10–15 cm; and Layer IV, 15–20 cm). Meanwhile, a group of five bulk soil samples away from plant roots was also collected from four soil layers. The roots and soil from the same soil layer were mixed and then sealed in zip-lock bags for transport to the laboratory in an ice box. Subsequently, the roots of the same soil layer were divided into four classes based on root diameter (Root I, 0.0–0.5 mm; Root II, 0.5–1.0 mm; Root III, 1.0–2.0 mm; and Root IV, >2.0 mm) using a graduated ruler (0.1mm resolution).
Furthermore, the roots of each diameter class were used for the sample collection of three rhizocompartment fractions (rhizosphere, rhizoplane, and endosphere) according to a previously described method (Edwards et al., 2015). Briefly, the roots were placed in a sterile tube with sterile phosphate buffer and then vortexed to collect the soil adhering to roots as the rhizosphere compartment. The roots in the tube were sonicated for 30 s each (time interval 30 s) at 50 Hz to strip the rhizoplane microbes from the root surface. The roots were then removed, and the liquid PBS (phosphate buffer solution) fraction was centrifuged and retained as the rhizoplane compartment. The sonicated roots were then placed in a new tube and sonicated twice more, and these roots were retained as the endosphere compartment. All rhizocompartment samples were stored at −80°C for DNA extraction. Bulk soils were divided into two subsamples. One bulk soil subsample was stored at −80°C for subsequent DNA extraction. The remaining bulk soil subsample was used to analyze the soil physicochemical properties. Finally, a total of 156 samples (four root diameters × four rooting depths × three rhizocompartments × three replicates + bulk soil × four soil layers × three replicates) were used for DNA extraction and PCR amplification of the V4-V5 regions of the 16S rRNA gene and subsequent high-throughput sequencing.
Soil Physical and Chemical Property Analyses
Soil pH, soil water content (SWC), total organic carbon (TOC), total nitrogen (TN), total phosphorus (TP), total potassium (TK), nitrate nitrogen (NO3−-N), ammonium nitrogen (NH4+-N), available phosphorus (AP), and available potassium (AK) according to routine methods (Bao, 2000). Soil pH was determined with a fresh soil to water ratio of 1:5 using a pH meter (Thermo Fisher Scientific, MA, United States). SWC was measured by the oven-drying method. TOC was determined by applying a traditional dichromate oxidation titration. TN was quantified following the Kjeldahl method. The NH4+-N and NO3−-N were determined by extraction with 1M KCl, steam distillation, and titration following the alkaline diffusion method. TP was extracted using 1 M HCl after ignition, and AP were extracted using 0.5 M NaHCO3, and then, they were measured following the Mo-Sb colorimetric method. AK extracted by 1 M CH3COONH4 and TK were measured using the flame photometry method.
DNA Extraction and High-Throughput Sequencing
Genomic DNA was extracted from bulk soil (0.5 g) and rhizosphere soil (0.5 g) using a FastDNA SPIN Kit for soil (MP Biomedicals, Solon, OH, United States) according to the manufacturer’s protocols. Due to the lower amount of rhizoplane samples, they were resuspended in 100 μl of PBS. Then, the genomic DNA of the root rhizoplane liquid samples was also extracted using the FastDNA SPIN Kit for soil. The extraction of the root samples (0.5 g) was performed using an Invisorb Spin Plant Mini Kit (Stratec Biomedical AG, Birkenfeld, Germany). The V4-V5 region of the 16S rRNA gene was amplified using the primer pair F515 (5'-GTGCCAGCMGCCGCGGTAA-3') and R907 (5'-CCGTCAATTCCTTTGAGTTT-3'; Edwards et al., 2015). Single-end high-throughput sequencing of the purified PCR products (400–450 bp) was performed on an IonS5TMXL platform (Thermo Fisher) at Novogene Bioinformatics Technology Co., Ltd. (Beijing, China). The acquired reads were quality-filtered using Cutadapt (Martin, 2011) and Vsearch (Rognes et al., 2016). Then, the sequences were clustered into operational taxonomic units (OTUs) based on >97% similarity using Uparse (Edgar, 2013). The representative sequences were further annotated against the SSU rRNA database of the SILVA 132 Database (http://www.arbsilva.de/) using Mothur (Schloss et al. 2009). Abundance data of sequences matching chloroplasts and mitochondria were removed from the datasets. To minimize the impact of sequencing artifacts, singletons were removed from the datasets.
Statistical Analysis
The statistical analyses were performed using R software 3.4.4 (http://www.r-project.org). Alpha diversity indices were calculated using the package “vegan” (Dixon, 2003). Principal coordinates analysis (PCoA) based on Bray-Curtis distances and constrained analysis of principal coordinates (CAP) based on Bray-Curtis distances were performed to visualize the relationships between samples. Permutational multivariate analysis of variance (PERMANOVA) based on Bray-Curtis distances was performed to determine whether sample classifications contained significant differences in species diversity using the package “vegan.” The “edgeR” package (Robinson et al., 2009) was used to identify the significantly altered OTUs by fitting a negative binomial distribution model to the 6,908 OTUs. The OTU counts from the bulk soil served as a control, and the adjusted p value cutoff was 0.05. The ratio of the enriched to depleted index (EDI) was defined to evaluate the bacterial enrichment effect in each rhizocompartment (Xiong et al., 2021). The core taxa associated with each root diameter or rooting depth were identified by canonical discriminant analysis (CDA) using the “candisc” package (Friendly and Fox, 2015).
OTUs with relative abundances higher than 0.1% were selected to construct co-occurrence networks. Spearman’s correlation between two OTUs was considered statistically robust if Spearman’s correlation coefficient (ρ) was >0.6 and the value of p was <0.01. To describe the network topology features, a set of measures (number of total nodes, total links, negative links, positive links, average path length, average degree, average clustering coefficient and modularity) were calculated using the “igraph” package (Csardi and Nepusz, 2006). The natural connectivity and average degree after the removal of nodes in the static network were used to estimate the network stability (Peng and Wu, 2016). The networks were visualized using the interactive platform Gephi (Bastian et al., 2009) or Cytoscape (Shannon, 2003). Keystone species were identified according to the betweenness centrality value, which indicates the relevance of a node as capable of holding together communicating nodes (Lupatini et al., 2014). In this study, the OTU with the highest betweenness centrality value was considered to be the keystone taxon.
Results
Alpha Diversity and Beta Diversity of Bacterial Community
In our study, there was no significant difference in the soil physical and chemical properties among the different soil layers, suggesting that the soil environment was homogeneous across the 0–20 cm layer (Supplementary Table S1). A total of 9,637,423 high-quality sequences from all 156 samples were clustered into 6,908 OTUs and classified into 54 phyla and 651 genera. In general, the bacterial taxon numbers at all levels (from genus to phylum) and the diversity indices were different among the four root diameters and four rooting depths (Supplementary Table S2). Alpha diversities, expressed as the OTU richness (the observed OTUs) and the Shannon diversity (Shannon-Wiener index), were significantly influenced by both root diameter and rooting depth. The bacterial alpha diversity in all three rhizocompartments showed a significantly increasing trend with decreasing root diameter (Figure 1A). The bacterial alpha diversity in the rhizoplane significantly increased with increasing rooting depth (Figure 1B).
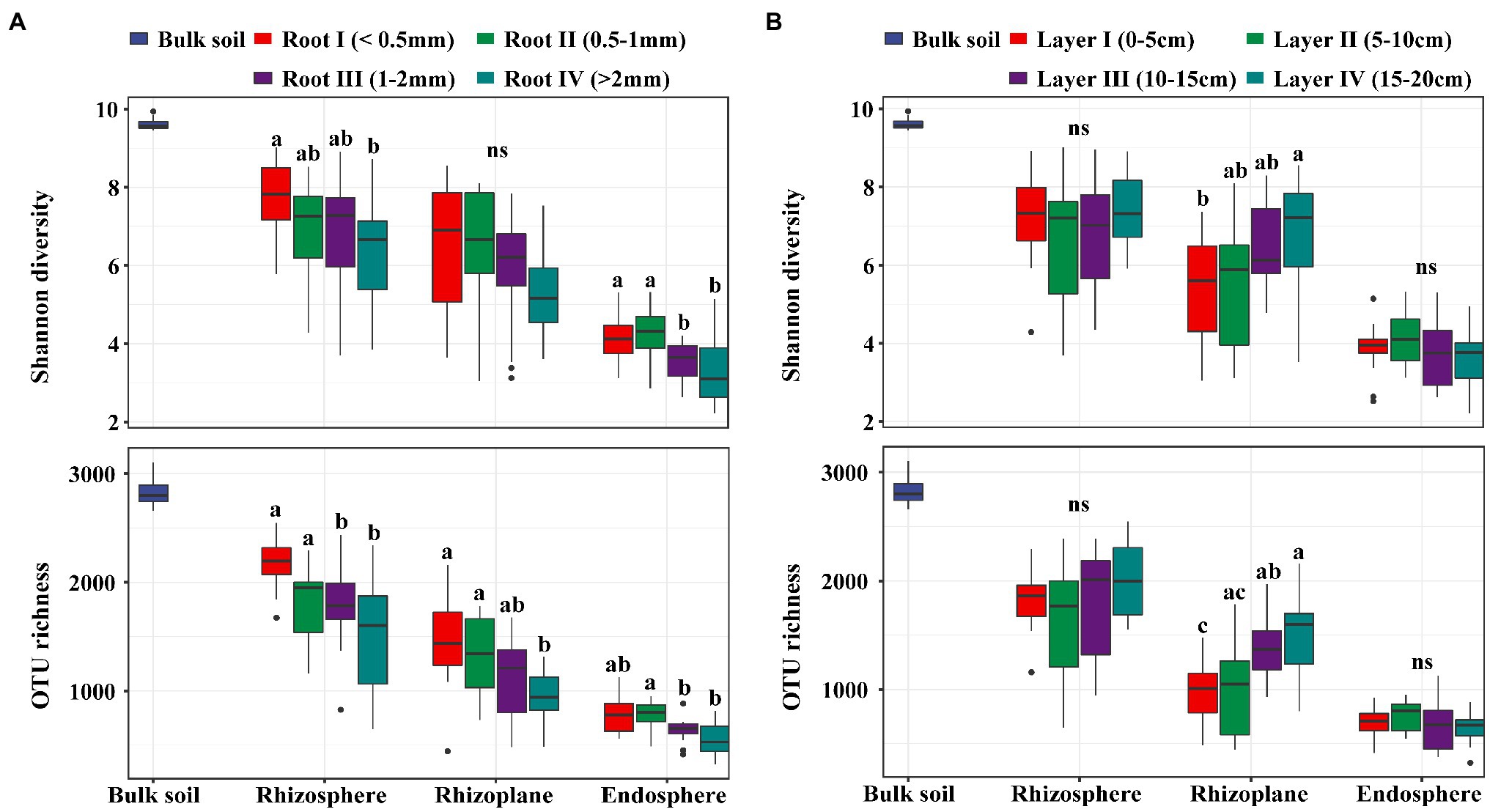
Figure 1. The boxplot of Shannon diversity and OTU richness (number of observed OTUs) of bacterial communities in rhizosphere, rhizoplane and endosphere among different root diameters (A; n=12) or different rooting depths (B; n=12). Different letters above the box indicate significant differences (p<0.05, “ns” to indicate non-significance, multiple comparison with Kruskal-Wallis tests).
To further explore the influence of root diameter and rooting depth on the entire root-associated microbiota structure, we performed PCoA, CAP, and PERMANOVA. The PERMANOVA results showed that the largest source of variation in the microbial communities was the rhizocompartment based on a Bray-Curtis distance metric (R2=0.462, p=0.001). Small but significant effects were also explained by the root diameter (R2=0.035, p=0.001) and rooting depth (R2=0.048. p=0.001). The PCoA plot (Figure 2A) confirmed that the microbiomes in different rhizocompartments clearly separated from each other; however, the clustering patterns of the microbiomes among different root diameters or rooting depths were not evident. The CAP results further showed that the Root I group, Root IV group, and the Root II and Root III groups were clearly separated (Supplementary Figure S1). Similar clustering patterns also occurred in the rhizosphere (R2=0.095, p=0.018) and endosphere (R2=0.121, p=0.021; Figure 2B). Among rooting depths, the Layer I group, Layer II group, and the Layer II and Layer IV groups were separated. Clustering patterns occurred in the rhizosphere (R2=0.132, p=0.001) and rhizoplane (R2=0.164, p=0.001; Figure 2C). Pairwise comparisons of the bacterial community between root diameters or rooting depths based on PERMANOVA further confirmed these results (Supplementary Table S4). In summary, the root diameter and rooting depth significantly influenced the composition of the root-associated bacterial community.
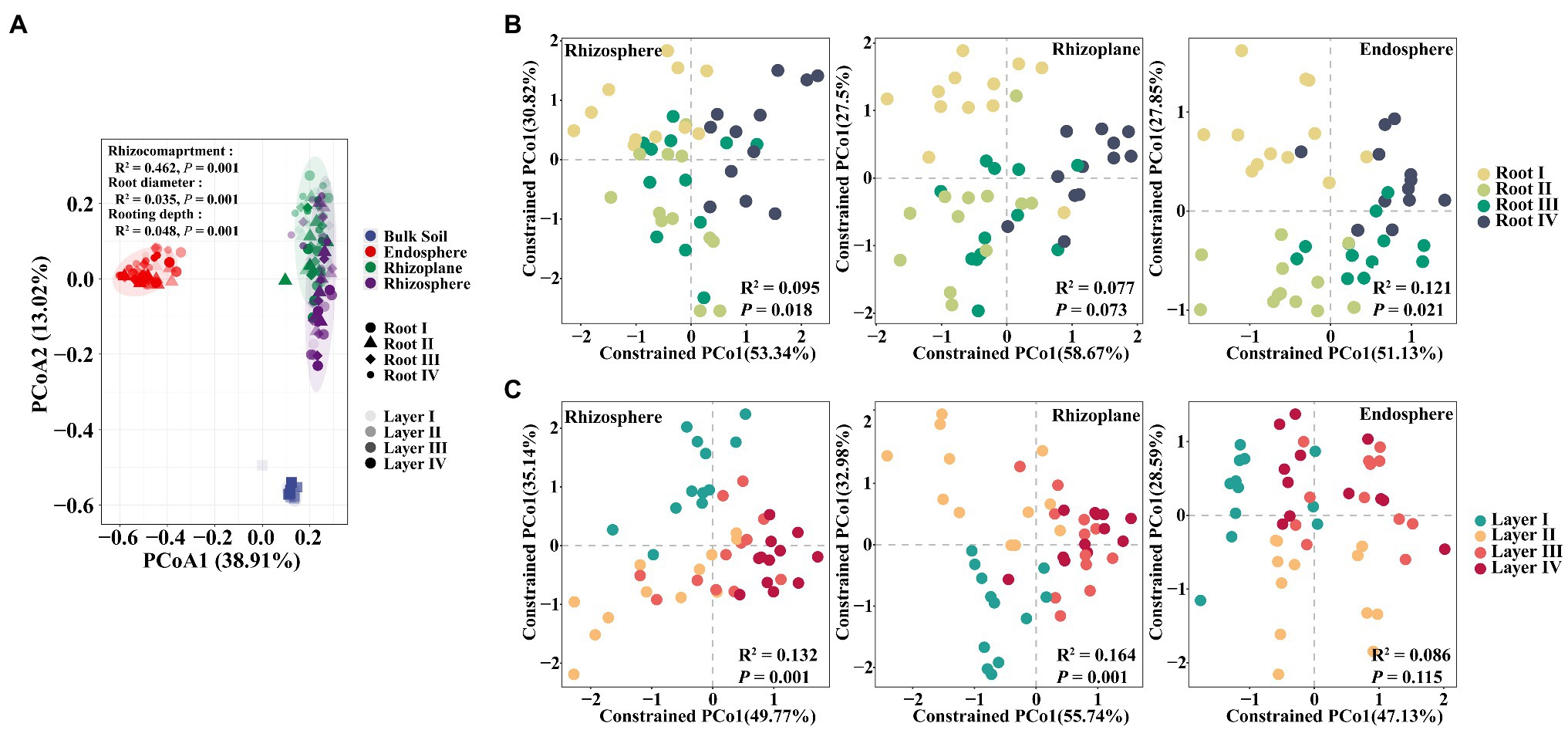
Figure 2. Principal coordinates analysis (PCoA) based on Bray-Curtis distances and constrained analysis of principal coordinates (CAP) based on Bray-Curtis distances of soybean root-associated microbiome. (A) PCoA plot showing differences in the beta diversity of all samples. (B) CAP plot showing bacterial compositional variation in rhizosphere, rhizoplane or endosphere explained by root diameter when controlling for rooting depth. (C) CAP plot showing bacterial compositional variation in rhizosphere, rhizoplane or endosphere explained by rooting depth when controlling for root diameter. Variance values were examined via PERMANOVA test, which are shown in each plot.
Distribution and Enrichment Process of the Bacterial Community
Taxonomic differences between the root classes based on the dominant phyla or subphyla (top 10 abundance) and genera (top 15 abundance) are displayed in bar plots and pheatmaps (Figure 3). The phyla Deltaproteobacteria, Bacteroidetes, Acidobacteria, and Chloroflexi significantly varied among root diameters (Supplementary Table S5). Meanwhile, the genus Neorhizobium was more abundant in the rhizoplane of Root III and Root IV, and the genus Streptomyces was more abundant in the endosphere of Root I and Root II (Figure 3B and Supplementary Table S6). The phyla Alphaproteobacteria, Deltaproteobacteria, Gammaproteobacteria, Oxyphotobacteria, Actinobacteria, Acidobacteria, and Planctomycetes in both the rhizosphere and rhizoplane significantly varied among rooting depths, while Oxyphotobacteria and Gammaproteobacteria significantly varied in the endosphere (Supplementary Table S5). The genus Klebsiella was more abundant in the rhizosphere of Layer I, Layer II, and Layer III, Novosphingobium, Variovorax, and Delftia were more abundant in the rhizoplane of Layer III and Layer IV, and Pseudomonas and Pseudochrobactrum were more abundant in the endosphere of Layer I (Figure 3D and Supplementary Table S6). In addition, the heatmap plot showed a strong clustering of different root diameters or rooting depths (Figures 3B,D), which was similar to the CAP results. These results indicated the different distribution patterns of dominant species abundance among root diameters or rooting depths.
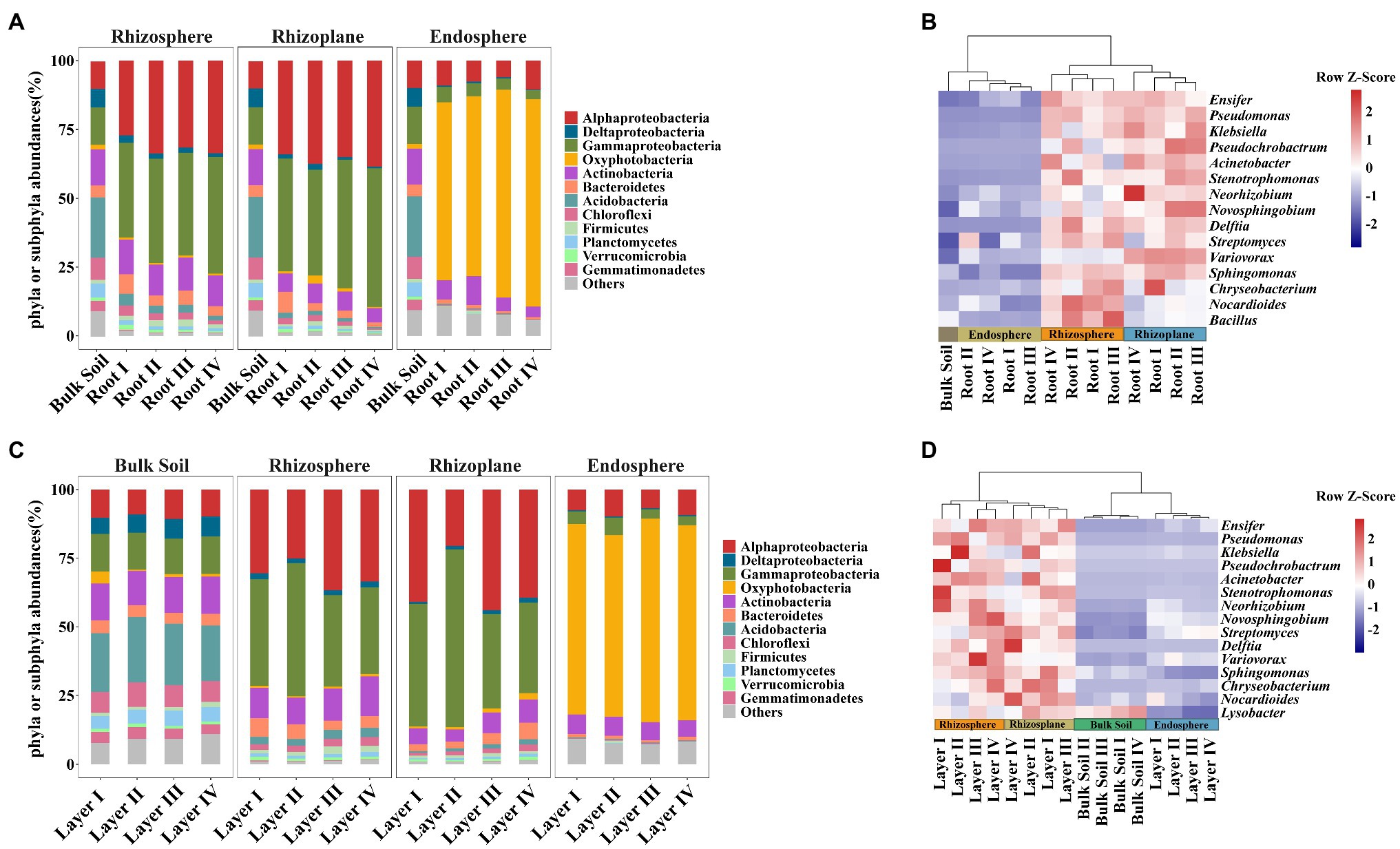
Figure 3. Bacterial community composition plots in different root diameters and rooting depths. (A,C) Bar chart showing the relative abundance of dominant bacterial phyla or subphyla (top 10). (B,D) Heatmap showing the distribution of bacterial genus level (top 15). Heatmap color (blue to dark red) displays the row scaled relative abundance of each taxon across all samples.
Differential abundance analysis was used to explore the changes in the significantly enriched and filtered OTUs among the different root diameters or rooting depths. The EDI value (the ratio of significantly enriched OTUs to depleted OTUs) was used to evaluate the species enrichment and filtration processes from the bulk soil to other root-associated niches. The EDI value decreased in the order of rhizosphere > rhizoplane > endosphere for all roots (Figure 4A; Supplementary Figure S4), suggesting that the root-associated bacterial communities were mainly derived from bulk soils and were gradually enriched and filtered in different rhizocompartments by a hierarchical process. Within each rhizocompartment, the EDI values were clearly different among root diameters or rooting depths (Figure 4A; Supplementary Figure S4). Generally, the EDI value had an increasing trend with decreasing root diameter and increasing rooting depth (except Layer IV). The roots of Root I and Layer III showed the highest EDI values across the three rhizocompartments, suggesting the largest enrichment effect of root-associated bacterial communities. These results suggested that root diameter and rooting depth significantly influenced the hierarchical process of bacterial enrichment and filtration in the root system.
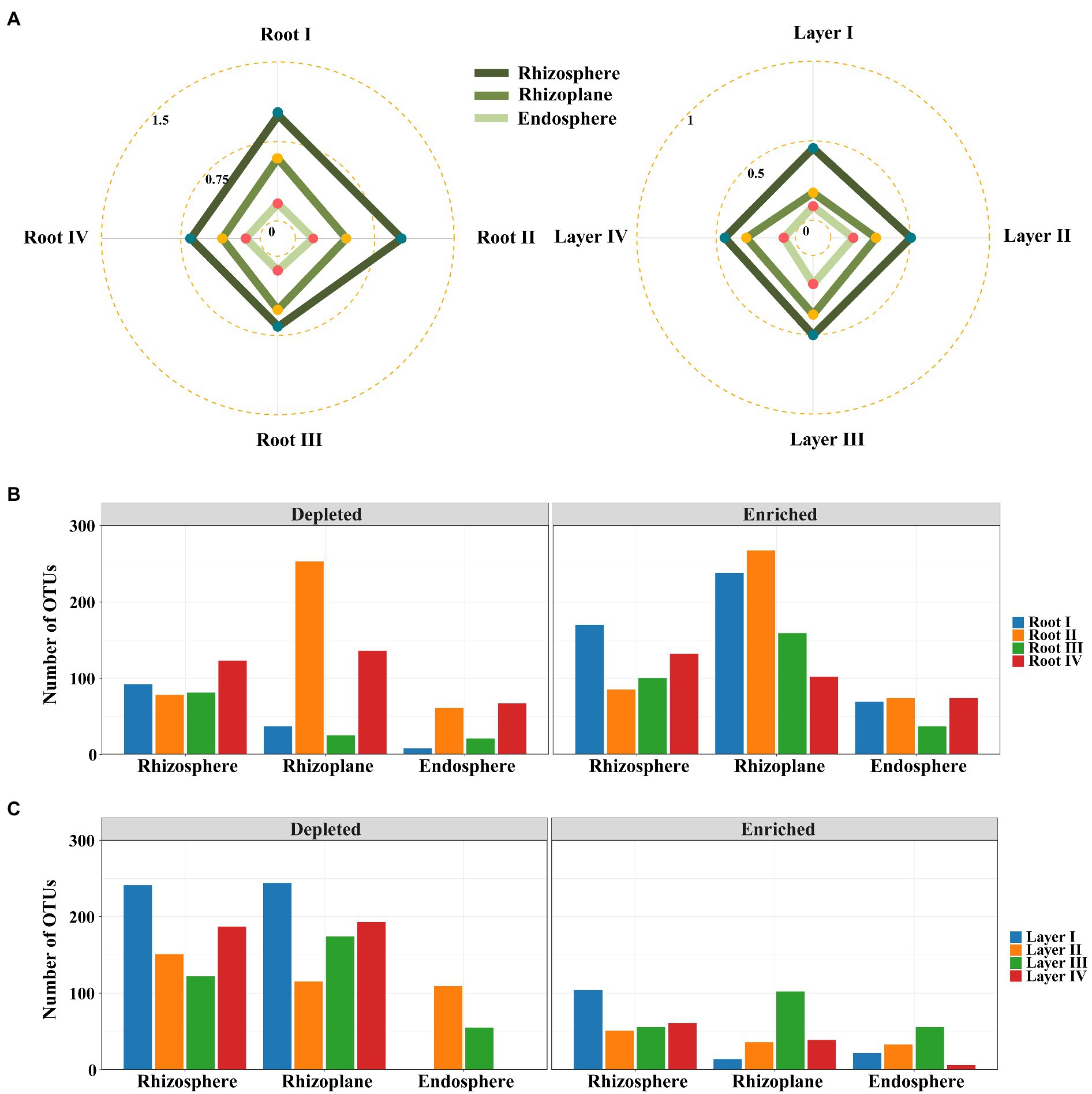
Figure 4. Enrichment and filtration of bacterial communities within different root diameters and rooting depths. (A) Radar plots of the ratios of statistically significantly enriched OTUs to the depleted OTUs with root diameter and rooting depth. Bar plots of the specifically enriched and depleted OTUs of the microbiota colonized in rhizocompartments of shared among root diameters (B) or rooting depths (C).
Irrespective of root diameters or rooting depths, the overlaps of enriched OTUs were mainly from Proteobacteria, Actinobacteria, and Bacteroidetes in the three rhizocompartments. In the rhizosphere and rhizoplane, the enriched genera mainly belonged to Ensifer, Pseudomonas, Pseudochrobactrum, Klebsiella, Acinetobacter, Stenotrophomonas, and Delftia, while the enriched genera mainly belonged to Ensifer, Streptomyces, Novosphingobium, Neorhizobium, Variovorax, Phytohabitans, and Actinoplanes in the endosphere (Supplementary Figures S2, S3). Among them, Ensifer was strongly enriched in all rhizocompartments of all root classes. Almost all of these genera were members of the dominant genera (Figures 3B,D). In addition, Root I had the highest number of specifically enriched OTUs in the rhizosphere, and Root IV had the highest number of specifically enriched OTUs in the rhizoplane and endosphere (Figure 4B; Supplementary Figure S4). Among rooting depths, Layer I had the highest number of specifically enriched OTUs in the rhizosphere, and Layer III had the highest number of specifically enriched OTUs in the rhizoplane and endosphere (Figure 4C; Supplementary Figure S4). The five specifically enriched and dominant genera in each root diameter and rooting depth (Supplementary Figures S5, S6) further showed distinct enrichment functions in different root classes.
Variation of the Root-Associated Core Bacterial Community
The root core microbiome (the broadly distributed microbial taxa in the root system) was identified as the bacterial taxa present in at least 95% of the samples (n=144). The 130 core taxa identified represented only 13.49% of the total number of OTUs, but these taxa accounted for the majority of the reads (57.11%). The relative abundance of the core taxa significantly varied among root diameters in the endosphere and among rooting depths in the rhizoplane and rhizosphere (Supplementary Figure S7). The significant effects were further confirmed in the endosphere (root diameter, R2=0.15, p=0.031), rhizoplane (rooting depth, R2=0.16, p=0.001), and rhizosphere (rooting depth, R2=0.21, p=0.001) using PERMANOVA.
The core taxa were mainly members of the phyla Proteobacteria, Actinobacteria, Acidobacteria, and Gemmatimonadetes. The CDA results of the 15 most abundant core genera revealed differences in their associations with different root diameters and rooting depths (Figure 5; Supplementary Figure S8). For example, Novosphingobium and Bradyrhizobium were associated with the Root II group, and Neorhizobium was associated with the Root IV group. Stenotrophomonas, Klebsiella, Variovorax, and Bradyrhizobium were associated with the Layer I, Layer II, Layer III, and Layer IV groups, respectively.
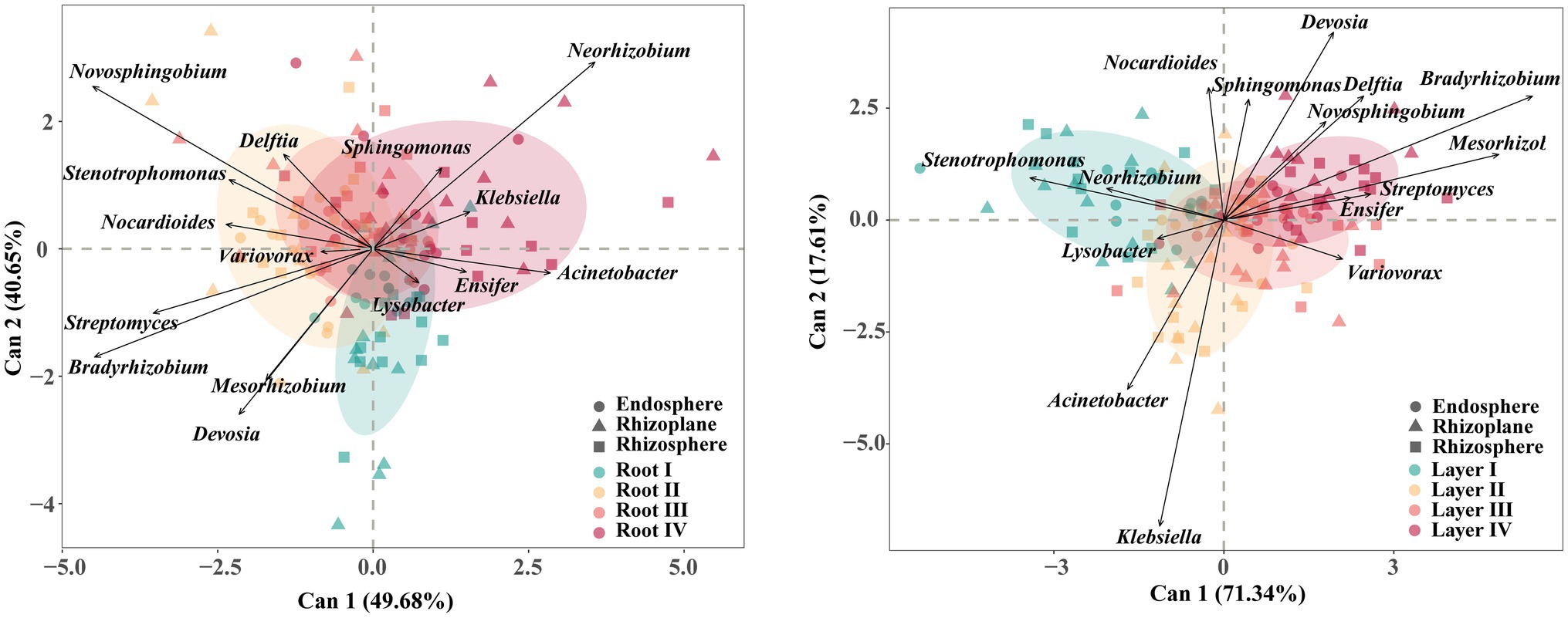
Figure 5. Canonical discriminant analysis (CDA) plot based on the predominant (top 15 abundance) core bacterial taxa at genus level. Arrows represent the degree of correlation between each taxon and each root diameter or rooting depth. Circles represent the canonical group means and 85% confidence interval for each root class.
Co-occurrence Network of the Root-Associated Bacterial Communities
To investigate the variation in interrelationships between root-associated bacterial taxa along root diameter and rooting depth, network analysis was performed (Figure 6). The topological features of the networks were calculated at the unique node (OTU) level (Table 1). The average clustering coefficient and average path length in these empirical networks were greater than those in random networks, suggesting that the empirical networks had the properties of “small worlds” and nonrandom co-occurrence patterns. The topological structure of bacterial networks of different root diameters and rooting depths was different from those of the whole root system and bulk soil networks (Table 1; Supplementary Figure S9). In addition, significant variations in the topological structure of networks occurred along the root diameter and rooting depth gradients (Figures 6B,D; Table 1). The network complexity (a higher average degree representing a greater network complexity) decreased in the order of Root I>Root II/Root III>Root IV and of Layer IV>Layer III>Layer II>Layer I (Table 1). The ratio of negative links to positive links (N/P) and modularity values (MD) exhibited similar trends in the bacterial networks. Natural connectivity represents the stability of network topology against random destruction or intentional attacks. The values of natural connectivity in all root networks decreased sharply when more network nodes were randomly removed. The natural connectivity value decreased in the order of Root I>Root II/Root III>Root IV and the order of Layer IV>Layer III/Layer II>Layer I (Supplementary Figure S10).
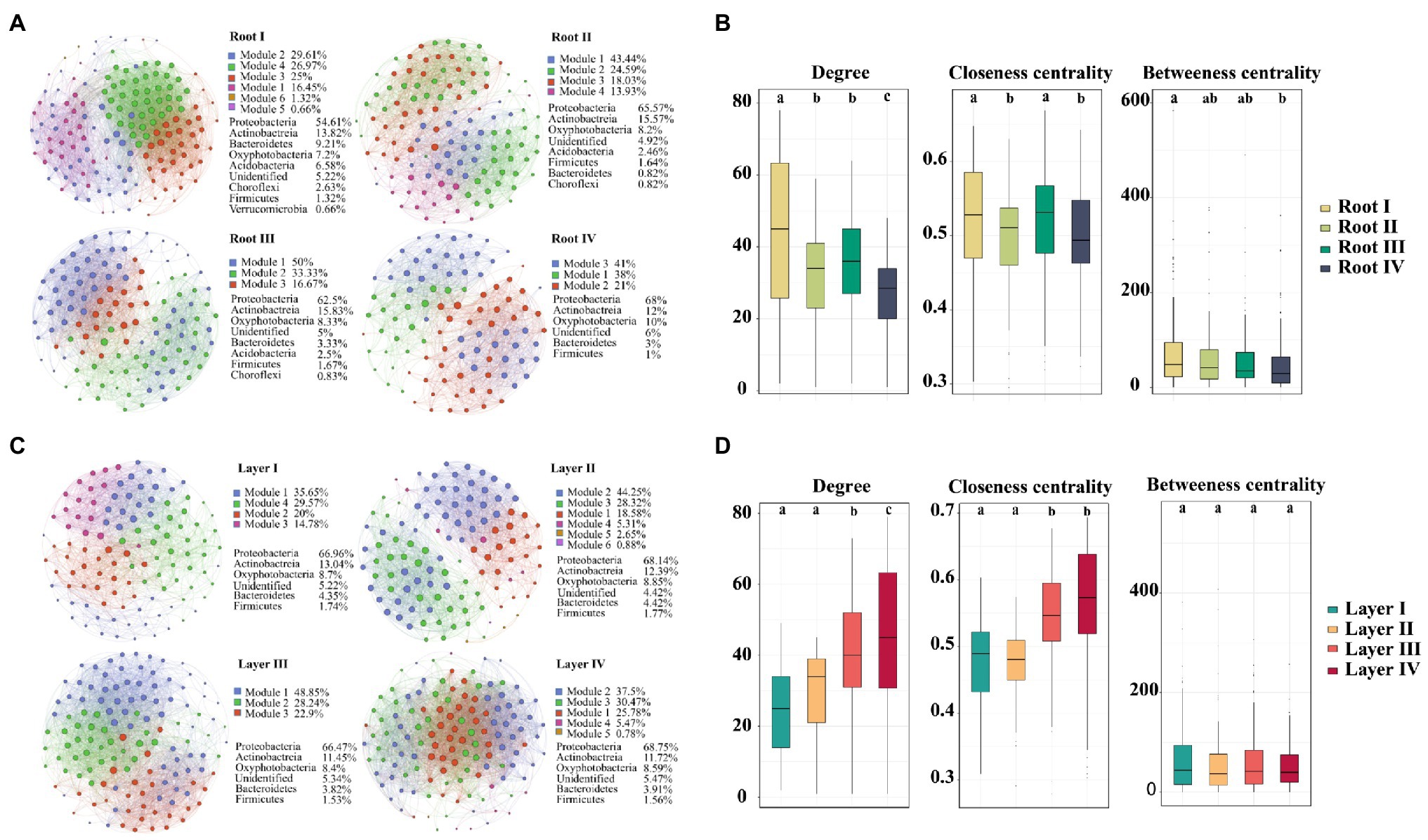
Figure 6. An operational taxonomic unit (OTU) network map showing the composition and correlation of the OTUs in different root diameters (A) and rooting depths (C). Network connections indicate a strong (Spearman’s ρ>0.6) and significant (p<0.01) correlation. The size and color shade of each node is proportional to the number of connections (degree), and the thickness of each connection between two nodes (edge) is proportional to the value of Spearman’s correlation coefficients. Unique node-level topological features of bacteria of different root diameters (B) and rooting depths (D), specifically the degree, betweenness, closeness. Different letters above the box indicate significant differences (p<0.05, multiple comparison with Kruskal-Wallis test).
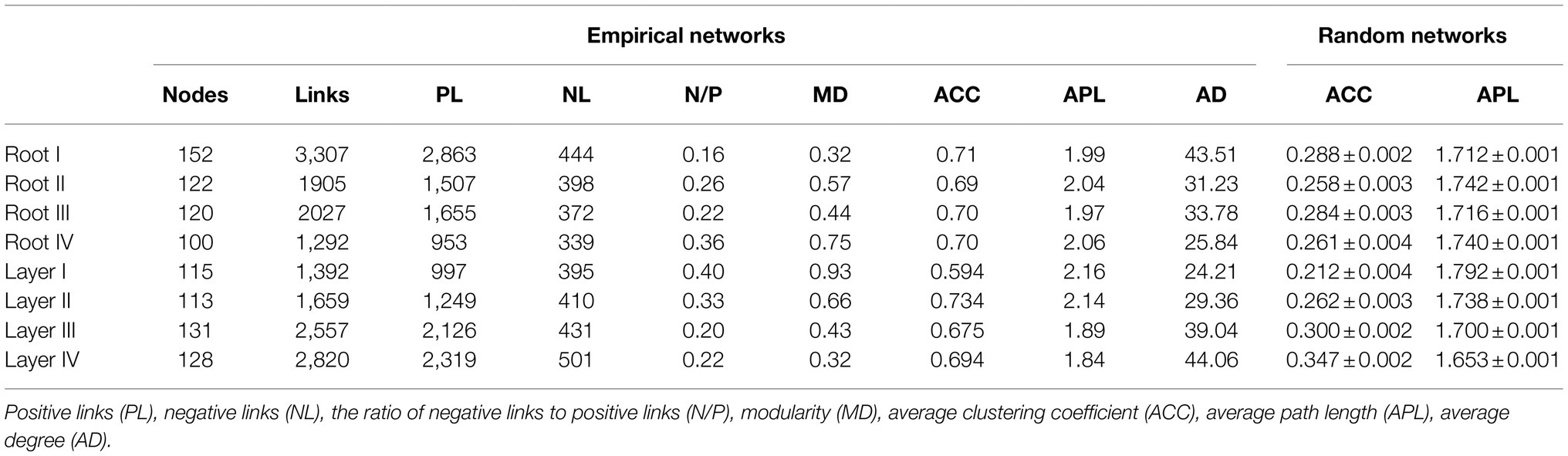
Table 1. Topological features the bacterial networks of different root diameters and rooting depths.
The taxa composition of the networks clearly varied among root diameters or rooting depths. Of the 184 and 178 distinct OTUs in the networks of different root diameters and rooting depths, only 71 and 73 nodes were shared, respectively (Supplementary FigureS11). Proteobacteria was the primary member of networks of different root classes (root diameters, 54.61–68% of the total nodes; rooting depths, 66.47–68.75%; Figures 6A,B). When the distribution of nodes was modularized, the networks of the root diameters or rooting depths were mainly grouped into three to six modules (Figures 6A,C), and the composition of the modules varied greatly among the root diameters or rooting depths (Supplementary Figure S12). The keystone taxa with the highest betweenness centrality value were members of Bacillus, Ramlibacter, Ensifer, and Sphingomonas in the Root I, Root II, Root III, and Root IV groups, respectively. The keystone taxa in the rooting depth networks were members of Actinoplanes, Arthrobacter, Pseudomonas, and Ensifer in the Layer I, Layer II, Layer III, and Layer IV groups, respectively.
Discussion
Effect of Root Diameter and Rooting Depth on the Composition of Root-Associated Bacterial Communities
Our results based on the complete dataset showed that the rhizocompartment niche considerably influenced the bacterial community assembly (Figure 2). Some studies on the root microbiome of rice, poplar trees, and soybean also indicated that different root niches harbored distinct microbial communities (Edwards et al., 2015; Beckers et al., 2017; Xiao et al., 2017). Notably, root diameter and rooting depth had significant impacts on the root-associated bacterial community, although the effects were smaller in comparison with the rhizocompartment (Figure 2). Together, these results suggest that root diameter and rooting depth can influence the roots, selecting niche-compliant microbes to occupy various rhizocompartments. Previous studies also indicated differentiation of rhizosphere or endosphere bacterial communities between fine and coarse roots (Saleem et al., 2016; Perez-Jaramillo et al., 2017; Wang et al., 2017a) or shallow and deep roots (Yang et al., 2016). Our study further showed that the rhizosphere microbiome was affected by both root diameter and rooting depth, while the rhizoplane and endosphere microbiomes were mainly affected by rooting depth and root diameter, respectively (Figure 2). These results indicated that root diameter and rooting depth jointly determined the selection of the root-associated microbiome.
Differences in the Bacterial Enrichment Processes Along the Root Diameter and Rooting Depth Gradients
Our results showed that regardless of how the root diameter and rooting depth changed, there was a hierarchical enrichment effect for microbes in the roots from the rhizosphere to the rhizoplane to the endosphere (Figure 4). Such an enrichment effect has been previously reported in the roots of soybean, rice, and Arabidopsis (Lundberg et al., 2012; Edwards et al., 2015; Xiao et al., 2017). We further found that the hierarchical enrichment of bacterial communities varied along the root diameter and rooting depth gradients. The thinner roots (smaller root diameter) and deeper roots displayed higher enrichment effects for the bacterial communities than other root classes. This might be due to a possible functional specialization of these roots to absorb nutrients and water by a large microbial community (Germon et al., 2020). Previous studies indicated that thinner plant roots have higher nutrient concentrations, colonization space, and root metabolic activity, such as the root C/N ratio, specific root surface area, turnover rate, and respiration rate (Eissenstat et al., 2000; Pregitzer et al., 2002; Guo et al., 2004; Wang et al., 2017b), and deeper roots are oriented toward the acquisition and transport of nutrients and water (Germon et al., 2020). The enrichment effects of root diameters and rooting depths were similar to our bacterial diversity results in which higher bacterial diversity was found in thinner and deeper roots (Figure 1). However, it should be noted that the enrichment effect of the deepest roots decreased. This is similar to our previous study on the deep-rooted plant Robinia pseudoacacia L (Zai et al., 2021). The changes can be explained by the fact that the root density and soil nutrients decreased rapidly.
The core microbiota generally provides broad ecological functions (Shade and Handelsman, 2012). Not surprisingly, the genus Ensifer, which can establish highly effective nitrogen-fixing symbiosis with soybean plants (Albareda et al., 2009), was widely enriched in all root classes based on the results of differential enrichment analysis. Our study further showed that root diameter and rooting depth together significantly influenced the selection of root-associated core microbial taxa (Supplementary Figure S7). Root diameter mainly affected the core bacterial group in the endosphere; however, rooting depth mainly affected the core group in the rhizoplane and rhizosphere. The differential effects of root diameter and rooting depth on conserved core taxa in the whole system are worthy of attention, which suggests that root diameter and rooting depth are tightly linked to the potential vital functions of the root-associated microbial community in soybean. In our study, the core taxa Novosphingobium, Bradyrhizobium, Neorhizobium, Stenotrophomonas, Klebsiella, and Variovorax were associated with specific root diameter or rooting depth. These taxa have been reported to be related to soybean secretion of soyasaponins, nodulation, and resistance to biotic and abiotic stresses (Molla et al., 2001; Chen et al., 2016; Egamberdieva et al., 2016; Bianca et al., 2018; Liu et al., 2018; Fujimatsu et al., 2020). Therefore, root diameter and rooting depth play important roles in affecting the relationships between core beneficial microbes and specific root classes.
Changes in Bacterial Co-occurrence Patterns Across Root Diameters and Rooting Depths
In natural ecosystems, microorganisms live together within complex networks that are linked through positive (commensalism and mutualism) and negative (amensalism and competition) interactions (Deng et al., 2012). In our study, the bacterial subnetworks of thinner and deeper roots were more complex (Figure 6 and Table 1), indicating that thinner and deeper roots promoted interactive processes in the community, playing positive roles in microbial coexistence (Goldford et al., 2018). The networks of thinner and deeper roots became more robust with higher network stability (Supplementary Figure S10), supporting the ecological belief that complexity begets stability (Montesinos-Navarro et al., 2017; Landi et al., 2018). In addition, higher positive correlations emerged in the networks of thinner and deeper roots, indicating that they included more cooperative or syntrophic associations (Karimi et al., 2017). These roots may rely on the associations between bacterial taxa to find and absorb nutrients and water.
Large variations in the bacterial module composition (functional ecological cluster) among different root diameters or rooting depths (Supplementary Figure S12) indicated that there was potential functional heterogeneity of the bacterial community among the different root classes (Newman, 2006). The keystone taxa belonging to Bacillus, Ramlibacter and Ensifer within the thinner root networks (Root I, Root II and Root III) are obviously beneficial for nutrient uptake and root development in an oligotrophic environment (Calvo et al., 2019; Props et al., 2019). Sphingomonas in the thicker root network (Root IV) can influence the water status and transport in root tissues (Asaf et al., 2017). The keystone taxa associated with the different root diameters indicate that thin roots more efficiently obtain nutrients and thick roots are more likely to transport nutrients. In terms of the bacterial networks of different rooting depths, the keystone taxa belonging to Actinoplanes and Arthrobacter in the shallower root networks (Layer I and Layer II) are associated with root stress tolerance (Sharma et al., 2016; Safdarian et al., 2019; El-Tarabily et al., 2020). Pseudomonas and Ensifer in the deeper root networks (Layer III and Layer IV) have mainly been shown to be related to the development and nutrient uptake of the root system (Cherni et al., 2019; Gan et al., 2020; Merino-Martín et al., 2020). These results indicated that the keystone taxa in deeper roots were more inclined to promote the nutrient uptake of roots. Overall, the complex associations of root-associated bacterial communities among root diameters and rooting depths appeared to be nonrandom and function driven.
Conclusion
In summary, root diameter and rooting depth jointly affected the root-associated (rhizosphere, rhizoplane, and endosphere) bacterial communities of soybean plants. The structure, enrichment process, and network interactions of the bacterial community varied along the root diameter and rooting depth gradients. The thinner roots and deeper roots were inclined to enrich more diverse bacteria due to a higher hierarchical enrichment effect and to increase interspecific interactions of the root-associated bacterial community. Our results not only confirmed the bacterial niche differentiation from the rhizosphere soil to the root rhizoplane to the endosphere but also showed that there is a tuned adaptation or selective effect of the bacterial community among root diameters or rooting depths. These findings expand our understanding of relationships between bacterial communities and root traits and the complex host–microbe interactions in a root system.
Data Availability Statement
The datasets presented in this study can be found in online repositories. The names of the repository/repositories and accession number(s) can be found in the article/Supplementary Material.
Author Contributions
WC conceived and designed the study. GL helped with the experiment design. XZ, JS, YL, and DL helped with the collection of the samples. WL analyzed the data and wrote the manuscript. WC and GW helped with the improvement of the manuscript. All authors contributed to the article and approved the submitted version.
Funding
This study was supported by the National Natural Science Foundation of China (31870476 and 41830755).
Conflict of Interest
The authors declare that the research was conducted in the absence of any commercial or financial relationships that could be construed as a potential conflict of interest.
Publisher’s Note
All claims expressed in this article are solely those of the authors and do not necessarily represent those of their affiliated organizations, or those of the publisher, the editors and the reviewers. Any product that may be evaluated in this article, or claim that may be made by its manufacturer, is not guaranteed or endorsed by the publisher.
Supplementary Material
The Supplementary Material for this article can be found online at: https://www.frontiersin.org/articles/10.3389/fmicb.2021.783563/full#supplementary-material
References
Albareda, M., Rodríguez-Navarro, D. N., and Temprano, F. J. (2009). Use of Sinorhizobium (Ensifer) fredii for soybean inoculants in South Spain. Eur. J. Agron. 30, 205–211. doi: 10.1016/j.eja.2008.10.002
Asaf, S., Khan, A. L., Khan, M. A., Imran, Q. M., Yun, B., and Lee, I. (2017). Osmoprotective functions conferred to soybean plants via inoculation with Sphingomonas sp. LK11 and exogenous trehalose. Microbiol. Res. 205, 135–145. doi: 10.1016/j.micres.2017.08.009
Bardgett, R. D., Mommer, L., and De Vries, F. T. (2014). Going underground: root traits as drivers of ecosystem processes. Trends Ecol. Evol. 29, 692–699. doi: 10.1016/j.tree.2014.10.006
Bastian, M., Heymann, S., and Jacomy, M. (2009). Gephi: An open source software for exploring and manipulating networks. ICWSM 69, 1613–1639. doi: 10.1590/2175-7860201869411
Beckers, B., Op De Beeck, M., Weyens, N., Boerjan, W., and Vangronsveld, J. (2017). Structural variability and niche differentiation in the rhizosphere and endosphere bacterial microbiome of field-grown poplar trees. Microbiome 5:25. doi: 10.1186/s40168-017-0241-2
Benjamin, J. G., and Nielsen, D. C. (2006). Water deficit effects on root distribution of soybean, field pea and chickpea. Field Crop Res. 97, 248–253. doi: 10.1016/j.fcr.2005.10.005
Berendsen, R. L., Pieterse, C. M. J., and Bakker, P. A. H. M. (2012). The rhizosphere microbiome and plant health. Trends Plant Sci. 17, 478–486. doi: 10.1016/j.tplants.2012.04.001
Bianca, D., Carpentieri-Pipolo, V., Djordje, F., Alberto, B. P., Yanil, L., Henrique, O. T., et al. (2018). Screening of bacterial endophytes as potential biocontrol agents against soybean diseases. J. Appl. Microbiol. 125, 1466–1481. doi: 10.1111/jam.14041
Bulgarelli, D., Rott, M., Schlaeppi, K., van Themaat, E. L., Ahmadinejad, N., Assenza, F., et al. (2012). Revealing structure and assembly cues for Arabidopsis root-inhabiting bacterial microbiota. Nature 488, 91–95. doi: 10.1038/nature11336
Bulgarelli, D., Schlaeppi, K., Spaepen, S., van Themaat, E. V. L., and Schulze-Lefert, P. (2013). Structure and functions of the bacterial microbiota of plants. Annu. Rev. Plant Biol. 64, 807–838. doi: 10.1146/annurev-arplant-050312-120106
Calvo, P., Zebelo, S., McNear, D., Kloepper, J., and Fadamiro, H. (2019). Plant growth-promoting rhizobacteria induce changes in Arabidopsis thaliana gene expression of nitrate and ammonium uptake genes. J. Plant Interact. 14, 224–231. doi: 10.1080/17429145.2019.1602887
Chen, L., He, L. Y., Wang, Q., and Sheng, X. F. (2016). Synergistic effects of plant growth-promoting Neorhizobium huautlense T1-17 and immobilizers on the growth and heavy metal accumulation of edible tissues of hot pepper. J. Hazard. Mater. 312, 123–131. doi: 10.1016/j.jhazmat.2016.03.042
Cherni, M., Ferjani, R., Mapelli, F., Boudabous, A., Borin, S., and Ouzari, H. (2019). Soil parameters drive the diversity of Citrus sinensis rhizosphere microbiota which exhibits a potential in plant drought stress alleviation. Appl. Soil Ecol. 135, 182–193. doi: 10.1016/j.apsoil.2018.12.006
Csardi, G., and Nepusz, T. (2006). The igraph software package for complex network research. Int. J. Complex Syst. 1695, 1–9.
Debinski, D. M., Wickham, H., Kindscher, K., Caruthers, J. C., and Germino, M. (2010). Montane meadow change during drought varies with background hydrologic regime and plant functional group. Ecology 91, 1672–1681. doi: 10.1890/09-0567.1
Deng, Y., Jiang, Y., Yang, Y., He, Z., Luo, F., and Zhou, J. (2012). Molecular ecological network analyses. Bmc. Bioinformatics 13:113. doi: 10.1186/1471-2105-13-113
Dixon, P. (2003). VEGAN, a package of R functions for community ecology. J. Veg. Sci. 14, 927–930. doi: 10.1658/1100-9233
Edgar, R. C. (2013). UPARSE: Highly accurate OTU sequences from microbial amplicon reads. Nat. Methods 10, 996–998. doi: 10.1038/nmeth.2604
Edwards, J., Johnson, C., Santos-Medellín, C., Lurie, E., Podishetty, N. K., Bhatnagar, S., et al. (2015). Structure, variation, and assembly of the root-associated microbiomes of rice. Proc. Natl. Acad. Sci. U. S. A. 112, e911–e920. doi: 10.1073/pnas.1414592112
Egamberdieva, D., Jabborova, D., Berg, G., Saikkonen, K., Mercado-Blanco, J., Mitter, B., et al. (2016). Synergistic interactions between Bradyrhizobium japonicum and the endophyte Stenotrophomonas rhizophila and their effects on growth, and nodulation of soybean under salt stress. Plant Soil 405, 35–45. doi: 10.1007/s11104-015-2661-8
Eissenstat, D. M., Wells, C. E., Yanai, R. D., and Whitbeck, J. L. (2000). Building roots in a changing environment: implications for root longevity. New Phytol. 147, 33–42. doi: 10.1046/j.1469-8137.2000.00686.x
El-Tarabily, K. A., ElBaghdady, K. Z., AlKhajeh, A. S., Ayyash, M. M., Aljneibi, R. S., El-Keblawy, A., et al. (2020). Polyamine-producing actinobacteria enhance biomass production and seed yield in Salicornia bigelovii. Biol. Fert. Soils 56, 499–519. doi: 10.1007/s00374-020-01450-3
Friendly, M., and Fox, J. (2015). Candisc: visualizing generalized canonical discriminant and canonical correlation analysis. Clin. Chem. 46, 373–378.
Fujimatsu, T., Endo, K., Yazaki, K., and Sugiyama, A. (2020). Secretion dynamics of soyasaponins in soybean roots and effects to modify the bacterial composition. Plant Direct 4:e00259. doi: 10.1002/pld3.259
Gan, L., Zhang, W., Fang, F., and Yang, L. (2020). Evidence of a trade-off between root aeration and architecture in Vallisneria natans in the presence of Pseudomonas putida KT2440. Aquat. Bot. 162:103189. doi: 10.1016/j.aquabot.2019.103189
Germon, A., Laclau, J., Robin, A., and Jourdan, C. (2020). Tamm review: deep fine roots in forest ecosystems: why dig deeper? Forest Ecol. Manag. 466:118135. doi: 10.1016/j.foreco.2020.118135
Goldford, J. E., Lu, N., Bajic, D., Estrela, S., Tikhonov, M., Sanchez-Gorostiaga, A., et al. (2018). Emergent simplicity in microbial community assembly. Science 361, 469–474. doi: 10.1126/science.aat1168
Gray, L., and Kernaghan, G. (2020). Fungal succession during the decomposition of ectomycorrhizal fine roots. Microb. Ecol. 79, 271–284. doi: 10.1007/s00248-019-01418-3
Guo, D. L., Mitchell, R. J., and Hendricks, J. J. (2004). Fine root branch orders respond differentially to carbon source-sink manipulations in a longleaf pine forest. Oecologia 140, 450–457. doi: 10.1007/s00442-004-1596-1
Hati, K. M., Mandal, K. G., Misra, A. K., Ghosh, P. K., and Bandyopadhyay, K. K. (2006). Effect of inorganic fertilizer and farmyard manure on soil physical properties, root distribution, and water-use efficiency of soybean in Vertisols of Central India. Bioresour. Technol. 97, 2182–2188. doi: 10.1016/j.biortech.2005.09.033
Hoeppner, S. S., and Dukes, J. S. (2012). Interactive responses of old-field plant growth and composition to warming and precipitation. Glob. Chang. Biol. 18, 1754–1768. doi: 10.1111/j.1365-2486.2011.02626.x
Karimi, B., Maron, P. A., Chemidlin-Prevost Boure, N., Bernard, N., Gilbert, D., and Ranjard, L. (2017). Microbial diversity and ecological networks as indicators of environmental quality. Environ. Chem. Lett. 15, 265–281. doi: 10.1007/s10311-017-0614-6
Landi, P., Minoarivelo, H. O., Brännström, Å., Hui, C., and Dieckmann, U. (2018). Complexity and stability of ecological networks: a review of the theory. Popul. Ecol. 60, 319–345. doi: 10.1007/s10144-018-0628-3
Li, H., Mollier, A., Ziadi, N., Shi, Y., Parent, L., and Morel, C. (2017). Soybean root traits after 24 years of different soil tillage and mineral phosphorus fertilization management. Soil Till. Res. 165, 258–267. doi: 10.1016/j.still.2016.09.002
Liu, D., Chen, L., Zhu, X., Wang, Y., Xuan, Y., Liu, X., et al. (2018). Klebsiella pneumoniae SnebYK mediates resistance against Heterodera glycines and promotes soybean growth. Front. Microbiol. 9:1134. doi: 10.3389/fmicb.2018.01134
Liu, C., Xiang, W., Zou, L., Lei, P., Zeng, Y., Ouyang, S., et al. (2019). Variation in the functional traits of fine roots is linked to phylogenetics in the common tree species of Chinese subtropical forests. Plant Soil 436, 347–364. doi: 10.1007/s11104-019-03934-0
Lundberg, D. S., Lebeis, S. L., Paredes, S. H., Yourstone, S., Gehring, J., Malfatti, S., et al. (2012). Defining the core Arabidopsis thaliana root microbiome. Nature 488, 86–90. doi: 10.1038/nature11237
Lupatini, M., Suleiman, A. K. A., Jacques, R. J. S., Antoniolli, Z. I., de Siqueira Ferreira, A. O., Kuramae, E. E., et al. (2014). Network topology reveals high connectance levels and few key microbial genera within soils. Front. Environ. Sci. 2:10. doi: 10.3389/fenvs.2014.00010
Ma, Z., Guo, D., Xu, X., Lu, M., Bardgett, R. D., Eissenstat, D. M., et al. (2018a). Evolutionary history resolves global organization of root functional traits. Nature 555, 94–97. doi: 10.1038/nature25783
Ma, X., Zarebanadkouki, M., Kuzyakov, Y., Blagodatskaya, E., Pausch, J., and Razavi, B. S. (2018b). Spatial patterns of enzyme activities in the rhizosphere: effects of root hairs and root radius. Soil Biol. Biochem. 118, 69–78. doi: 10.1016/j.soilbio.2017.12.009
Madhaiyan, M., Poonguzhali, S., Saravanan, V. S., and Kwon, S. (2014). Rhodanobacter glycinis sp. Nov., A yellow-pigmented gammaproteobacterium isolated from the rhizoplane of field-grown soybean. Int. J. Syst. Evol. Micr. 64, 2023–2028. doi: 10.1099/ijs.0.055525-0
Martin, M. (2011). Cutadapt removes adapter sequences from high-throughput sequencing reads. EMBnet J. 17, 10–12. doi: 10.14806/ej.17.1.200
Meister, R., Rajani, M. S., Ruzicka, D., and Schachtman, D. P. (2014). Challenges of modifying root traits in crops for agriculture. Trends Plant Sci. 19, 779–788. doi: 10.1016/j.tplants.2014.08.005
Mendes, L. W., Kuramae, E. E., Navarrete, A. A., van Veen, J. A., and Tsai, S. M. (2014). Taxonomical and functional microbial community selection in soybean rhizosphere. ISME J. 8, 1577–1587. doi: 10.1038/ismej.2014.17
Merino-Martín, L., Griffiths, R. I., Gweon, H. S., Furget-Bretagnon, C., Oliver, A., Mao, Z., et al. (2020). Rhizosphere bacteria are more strongly related to plant root traits than fungi in temperate montane forests: insights from closed and open forest patches along an elevational gradient. Plant Soil 450, 183–200. doi: 10.1007/s11104-020-04479-3
Molla, A. H., Shamsuddin, Z. H., Halimi, M. S., Morziah, M., and Puteh, A. B. (2001). Potential for enhancement of root growth and nodulation of soybean co-inoculated with Azospirillum and Bradyrhizobium in laboratory systems. Soil Biol. Biochem. 33, 457–463. doi: 10.1016/S0038-0717(00)00186-3
Montesinos-Navarro, A., Hiraldo, F., Tella, J. L., and Blanco, G. (2017). Network structure embracing mutualism-antagonism continuums increases community robustness. Nat. Ecol. Evol. 1, 1661–1669. doi: 10.1038/s41559-017-0320-6
Newman, M. E. J. (2006). Modularity and community structure in networks. Proc. Natl. Acad. Sci. U. S. A. 103, 8577–8582. doi: 10.1073/pnas.0601602103
Panke-Buisse, K., Poole, A. C., Goodrich, J. K., Ley, R. E., and Kao-Kniffin, J. (2014). Selection on soil microbiomes reveals reproducible impacts on plant function. ISME J. 9, 980–989. doi: 10.1038/ismej.2014.196
Peng, G. S., and Wu, J. (2016). Optimal network topology for structural robustness based on natural connectivity. Physica A 443, 212–220. doi: 10.1016/j.physa.2015.09.023
Perez-Jaramillo, J. E., Carrion, V. J., Bosse, M., Ferrao, L. F. V., de Hollander, M., Garcia, A. A. F., et al. (2017). Linking rhizosphere microbiome composition of wild and domesticated Phaseolus vulgaris to genotypic and root phenotypic traits. ISME J. 11, 2244–2257. doi: 10.1038/ismej.2017.85
Pervaiz, Z. H., Contreras, J., Hupp, B. M., Lindenberger, J. H., Chen, D., Zhang, Q., et al. (2020). Root microbiome changes with root branching order and root chemistry in peach rhizosphere soil. Rhizosphere 16:100249. doi: 10.1016/j.rhisph.2020.100249
Pregitzer, K. S., DeForest, J. L., Burton, A. J., Allen, M. F., Ruess, R. W., and Hendrick, R. L. (2002). Fine root architecture of nine north american trees. Ecol. Monogr. 72, 293–309. doi: 10.1890/0012-9615
Props, R., Monsieurs, P., Vandamme, P., Leys, N., Denef, V. J., and Boon, N. (2019). Gene expansion and positive selection as bacterial adaptations to oligotrophic conditions. Msphere 4, e11–e19. doi: 10.1128/mSphereDirect.00011-19
Reinhold-Hurek, B., Bünger, W., Burbano, C. S., Sabale, M., and Hurek, T. (2015). Roots shaping their microbiome: global hotspots for microbial activity. Annu. Rev. Phytopathol. 53, 403–424. doi: 10.1146/annurev-phyto-082712-102342
Robinson, M. D., McCarthy, D. J., and Smyth, G. K. (2009). EdgeR: A bioconductor package for differential expression analysis of digital gene expression data. Bioinformatics 26, 139–140. doi: 10.1093/bioinformatics/btp616
Rognes, T., Flouri, T., Nichols, B., Quince, C., and Mahé, F. (2016). VSEARCH: A versatile open source tool for metagenomics. Peerj 4:e2584. doi: 10.7717/peerj.2584
Safdarian, M., Askari, H., Shariati, J., and V., and Nematzadeh, G., (2019). Transcriptional responses of wheat roots inoculated with Arthrobacter nitroguajacolicus to salt stress. Sci. Rep 9:1792. doi: 10.1038/s41598-018-38398-2
Saleem, M., Law, A. D., and Moe, L. A. (2016). Nicotiana roots recruit rare rhizosphere taxa as major root-inhabiting microbes. Microb. Ecol. 71, 469–472. doi: 10.1007/s00248-015-0672-x
Saleem, M., Law, A. D., Sahib, M. R., Pervaiz, Z. H., and Zhang, Q. (2018). Impact of root system architecture on rhizosphere and root microbiome. Rhizosphere 6, 47–51. doi: 10.1016/j.rhisph.2018.02.003
Shade, A., and Handelsman, J. (2012). Beyond the Venn diagram: The hunt for a core microbiome. Environ. Microbiol. 14, 4–12. doi: 10.1111/j.1462-2920.2011.02585.x
Shannon, P. (2003). Cytoscape: A software environment for integrated models of biomolecular interaction networks. Genome Res. 13, 2498–2504. doi: 10.1101/gr.1239303
Sharma, M., Mishra, V., Rau, N., and Sharma, R. S. (2016). Increased iron-stress resilience of maize through inoculation of siderophore-producing Arthrobacter globiformis from mine. J. Basic Microb. 56, 719–735. doi: 10.1002/jobm.201500450
Schloss, P. D., Westcott, S. L., Ryabin, T., Hall, J. R., Hartmann, M., Hollister, E. B., et al. (2009). Introducing mothur: Open-Source, Platform-Independent, Community-Supported software for describing and comparing microbial communities. Appl. Environ. Microb. 75, 7537–7541. doi: 10.1128/AEM.01541-09
Sugiyama, A., Ueda, Y., Zushi, T., Takase, H., and Yazaki, K. (2014). Changes in the bacterial community of soybean rhizospheres during growth in the field. PLoS One 9:e100709. doi: 10.1371/journal.pone.0100709
Vandenkoornhuyse, P., Quaiser, A., Duhamel, M., Van, A. L., and Dufresne, A. (2015). The importance of the microbiome of the plant holobiont. New Phytol. 206, 1196–1206. doi: 10.1111/nph.13312
Verbon, E. H., and Liberman, L. M. (2016). Beneficial microbes affect endogenous mechanisms controlling root development. Trends Plant Sci. 21, 218–229. doi: 10.1016/j.tplants.2016.01.013
Vorholt, J. A. (2012). Microbial life in the phyllosphere. Nat. Rev. Microbiol. 10, 828–840. doi: 10.1038/nrmicro2910
Wang, Q., Wang, N., Wang, Y., Wang, Q., and Duan, B. (2017a). Differences in root-associated bacterial communities among fine root branching orders of poplar (Populus × euramericana (Dode) Guinier.). Plant Soil 421, 123–135. doi: 10.1007/s11104-017-3449-9
Wang, R., Wang, Q., Zhao, N., Xu, Z., Zhu, X., Jiao, C., et al. (2017b). Different phylogenetic and environmental controls of first-order root morphological and nutrient traits: evidence of multidimensional root traits. Funct. Ecol. 32, 29–39. doi: 10.1111/1365-2435.12983
Xiao, X., Chen, W., Zong, L., Yang, J., Jiao, S., Lin, Y., et al. (2017). Two cultivated legume plants reveal the enrichment process of the microbiome in the rhizocompartments. Mol. Ecol. 26, 1641–1651. doi: 10.1111/mec.14027
Xiong, C., Zhu, Y. G., Wang, J. T., Singh, B., Han, L. L., Shen, J. P., et al. (2021). Host selection shapes crop microbiome assembly and network complexity. New Phytol. 229, 1091–1104. doi: 10.1111/nph.16890
Yahara, H., Tanikawa, N., Okamoto, M., and Makita, N. (2019). Characterizing fine-root traits by species phylogeny and microbial symbiosis in 11 co-existing woody species. Oecologia 191, 983–993. doi: 10.1007/s00442-019-04546-2
Yang, H., Hu, J., Long, X., Liu, Z., and Rengel, Z. (2016). Salinity altered root distribution and increased diversity of bacterial communities in the rhizosphere soil of Jerusalem artichoke. Sci. Rep. 6:20687. doi: 10.1038/srep20687
Zai, X., Luo, W., Bai, W., Li, Y., Xiao, X., Gao, X., et al. (2021). Effect of root diameter on the selection and network interactions of root-associated bacterial microbiomes in Robinia pseudoacacia L. Microb. Ecol. 82, 391–402. doi: 10.1007/s00248-020-01678-4
Zhang, Y., Xu, J., Riera, N., Jin, T., Li, J., and Wang, N. (2017). Huanglongbing impairs the rhizosphere-to-rhizoplane enrichment process of the citrus root-associated microbiome. Microbiome 5:97. doi: 10.1186/s40168-017-0304-4
Keywords: rhizocompartment, rhizosphere microbiota, legume plant, shallow root system, root traits
Citation: Luo W, Zai X, Sun J, Li D, Li Y, Li G, Wei G and Chen W (2021) Coupling Root Diameter With Rooting Depth to Reveal the Heterogeneous Assembly of Root-Associated Bacterial Communities in Soybean. Front. Microbiol. 12:783563. doi: 10.3389/fmicb.2021.783563
Edited by:
Milko Alberto Jorquera, University of La Frontera, ChileReviewed by:
Xingang Zhou, Northeast Agricultural University, ChinaZongzhuan Shen, Nanjing Agricultural University, China
Copyright © 2021 Luo, Zai, Sun, Li, Li, Li, Wei and Chen. This is an open-access article distributed under the terms of the Creative Commons Attribution License (CC BY). The use, distribution or reproduction in other forums is permitted, provided the original author(s) and the copyright owner(s) are credited and that the original publication in this journal is cited, in accordance with accepted academic practice. No use, distribution or reproduction is permitted which does not comply with these terms.
*Correspondence: Weimin Chen, Y2hlbndtMDI5QG53c3VhZi5lZHUuY24=