- 1Extreme Environments Biotechnology Lab, Center of Excellence in Translational Medicine, Universidad de La Frontera, Temuco, Chile
- 2Biotechnology Research Center, Department of Biology, Instituto Tecnológico de Costa Rica, Cartago, Costa Rica
- 3Scientific and Technological Bioresource Nucleus (BIOREN), Universidad de La Frontera, Temuco, Chile
Introduction: The antibiotic crisis is a major human health problem. Bioprospecting screenings suggest that proteobacteria and other extremophile microorganisms have biosynthetic potential for the production novel antimicrobial compounds. An Antarctic Sphingomonas strain (So64.6b) previously showed interesting antibiotic activity and elicitation response, then a relationship between environmental adaptations and its biosynthetic potential was hypothesized. We aimed to determine the genomic characteristics in So64.6b strain related to evolutive traits for the adaptation to the Antarctic environment that could lead to its diversity of potentially novel antibiotic metabolites.
Methods: The complete genome sequence of the Antarctic strain was obtained and mined for Biosynthetic Gene Clusters (BGCs) and other unique genes related to adaptation to extreme environments. Comparative genome analysis based on multi-locus phylogenomics, BGC phylogeny, and pangenomics were conducted within the closest genus, aiming to determine the taxonomic affiliation and differential characteristics of the Antarctic strain.
Results and discussion: The Antarctic strain So64.6b showed a closest identity with Sphingomonas alpina, however containing a significant genomic difference of ortholog cluster related to degradation multiple pollutants. Strain So64.6b had a total of six BGC, which were predicted with low to no similarity with other reported clusters; three were associated with potential novel antibiotic compounds using ARTS tool. Phylogenetic and synteny analysis of a common BGC showed great diversity between Sphingomonas genus but grouping in clades according to similar isolation environments, suggesting an evolution of BGCs that could be linked to the specific ecosystems. Comparative genomic analysis also showed that Sphingomonas species isolated from extreme environments had the greatest number of predicted BGCs and a higher percentage of genetic content devoted to BGCs than the isolates from mesophilic environments. In addition, some extreme-exclusive clusters were found related to oxidative and thermal stress adaptations, while pangenome analysis showed unique resistance genes on the Antarctic strain included in genetic islands. Altogether, our results showed the unique genetic content on Antarctic strain Sphingomonas sp. So64.6, −a probable new species of this genetically divergent genus–, which could have potentially novel antibiotic compounds acquired to cope with Antarctic poly-extreme conditions.
Introduction
From 2000 to 2015, antibiotic consumption increased by 65% (Klein et al., 2018). In addition, the proportion of the non-prescription supply of antibiotics was 62% from 2000 to 2017 (Auta et al., 2019). The misuse and overuse of antibiotics increased the mortality of common infections due to resistant strains of microorganisms, resulting in 1.27 million deaths globally attributed to antibiotic resistance in 2019 (Murray et al., 2022). Hence, the antibiotic resistance crisis is a major human health problem and is growing every year.
In this sense, developing new compounds with antimicrobial activity and novel mechanism of action is a main concern in the scientific community. Many studies have focused on using metals nanoparticles (e.g., silver) or a combination of nanomaterials and antibiotics to enhance their antimicrobial activity (Liao et al., 2019; Hochvaldová et al., 2022). Another recent approach is the use of predatory bacteria to kill pathogen bacteria, a technique that demands further investigations (Atterbury and Tyson, 2021). However, exploring secondary metabolites from microbes with antibiotic activities remains the primary source of research (Newman and Cragg, 2016).
Actinobacteria have been extensively explored for antibiotic compounds, with great variety and biotechnological potential (Genilloud, 2017; Jagannathan et al., 2021). Regardless of the promising results of antimicrobial compounds, the chances of discovering new metabolites from actinobacteria are low, forcing to explore new sources for bioprospecting (Bredholt et al., 2008). Some studies have suggested that proteobacteria might be a better target than actinobacteria for screening antimicrobial compounds (Al-Amoudi et al., 2016).
Proteobacteria is a diverse phylum of Gram-negative bacteria. Many natural products with medical relevance are produced by proteobacterial strains, such as the previously reported didemnins and thalassospiramides, showing medical relevant activities (Timmermans et al., 2017). Also, species of that phylum have been highlighted for its biotechnological potential for bioremediation and pollutants degradation, including Alcanivorax and Marinobacter strains (Chernikova et al., 2020). Moreover, the proteobacterial genus, Pseudoalteromonas, can produce a bioactive compound called thiomarinols, which is used against the multidrug-resistant pathogen Staphylococcus (Fukuda et al., 2011).
Bacteria from extreme environments (extremophiles) have recently been recommended for its predicted untapped variety of antimicrobial compounds, representing another focus of research for new antibiotics (Danilovich et al., 2018; Babich et al., 2021). Accordingly, extremophile proteobacteria have also been described to produce a great variety of specialized metabolites (Puri, 2019). Antarctic proteobacteria have shown antimicrobial activity against Candida albicans and S. aureus, both pathogenic, including bacteria from the Sphingomonas genus (Lee et al., 2012). This genus is best known for its ability to degrade organic pollutants, such as pharmaceutical and industrial residues (Aulestia et al., 2021; Zhang et al., 2022; Zhou et al., 2022). Nonetheless, antimicrobial activity has also been reported from species of the Sphingomonas genus, such as S. molluscorum (Romanenko et al., 2007; Lee et al., 2012).
In previous work, we reported an Antarctic Sphingomonas strain So64.6b with antibiotic activity in response to elicitation treatments (Núñez-Montero et al., 2020). Moreover, during elicitation treatments, it exceeded the number of metabolites capable of producing under regular conditions (Núñez-Montero et al., 2020). It was hypothesized that adaptation to the Antarctic might drive an untapped diversity of specialized metabolites; nonetheless, there is no evidence of a correlation between the bioactivity or production of new compounds with the adaptation to the extreme environments. Therefore, in this work we aimed to determine the genetic evolutive traits of Sphingomonas sp. So64.6b that might be associated with adaptation to the Antarctic environment and that could lead to its diversity of antibiotic metabolites.
Materials and methods
Antarctic bacterial strain and culture
An Antarctic bacteria strain (ID So64.6b) previously isolated from soil on Agar M1 (peptone 2.0 g/l, yeast extract 4.0 g/L, starch 10.0 g/L and agar 18 g/L; Lamilla et al., 2017) was used in this study. For storage, the bacterium was cultured in 3 ml of International Streptomyces Project-2 medium (ISP-2; yeast extract 4.0 g/L, malt extract 10.0 g/L, glucose 4.0 g/L, and agar 18 g/L) and cryopreserved at -80°C with 20% glycerol. Culture for DNA extraction was obtained by inoculating the isolated bacterial strain on the ISP-2 agar at 15°C for 7 days.
DNA extraction and genome sequencing
Genomic DNA extraction was performed using the UltraClean Microbial DNA Extraction Kit (MoBio Laboratories, Carlsbad, USA). DNA quality was assessed by fluorescence quantification using the QuantiFluor® ONE dsDNA System kit (Promega) on a Quantus device (Promega). Also, integrity was verified by 1% agarose gel and purity according to 260/280 and 260/230 absorption ratios. The sample was divided into two and sequencing using Illumina and Oxford Nanopore Technologies (ONT) technologies. The Illumina library was prepared with 2x150bp fragments using the Nextera XT DNA Sample Prep kit (Illumina, San Diego, CA) and sequenced on an Illumina MiSeq platform. The ONT library was prepared using the Rapid Sequencing kit SQK-RBK004 and sequenced on the MinION platform of the Extreme Environments Biotechnology Lab (Universidad de La Frontera, Chile), according to the manufacturer’s recommendations and using the MinKNOW software v.4.0.20. The basecalling of the ONT reads was performed with Guppy v3.1.5 software.
Whole genome assembly
Assembly was performed using the two data libraries (i.e., Illumina and ONT). The quality of Illumina reads was verified by FastQC (Andrews, 2010), and fastp (Chen et al., 2018) was used to filter poor-quality reads using default parameters. The quality of ONT reads was verified with nanoPLOT (De Coster et al., 2018). Adapter and low quality reads (phred >10, size >5,000 bp) were removed with Porechop and NanoFilt (De Coster et al., 2018), respectively. De novo assembly was performed using the Unicycler software v.0.4.8 (Wick et al., 2017) with default parameters for hybrid assembly with short + long reads. The whole genome results of this study were deposited in the DDBJ/ENA/GenBank database under the accession CP048817.
Genome-based taxonomic and phylogenetic analysis
Whole genome taxonomic identity was confirmed using Average Nucleotide Identity (ANI) values. The ANI value was calculated among the available Sphingomonas representative complete genomes on NCBI database (×49) using Pyani v0.2.10 software in ANIb mode (Pritchard et al., 2016). The reference genome with highest ANI value was used for DNA–DNA hybridization (DDH) estimation values, which was computed using the genome-to-genome distance calculator (GGDC v3; Meier-Kolthoff et al., 2022). In addition, phylogenetic distances between the closest taxonomic species were also determined by constructing the core proteome of the available representative complete genomes on the genus Sphingomonas (×49). First, core proteins were obtained with the web server M1CR0B1AL1Z3R1 (Avram et al., 2019) using an 80.0% cutoff value for the minimum identity of proteins found in all compared genomes. Next, an unrooted phylogenetic construction of the core proteome was performed with a maximum likelihood algorithm using RAxML (Stamatakis, 2014) based on the inferred proteome alignment. Phylogenetic tree visualization was performed with the iTOL v3 tool (Letunic and Bork, 2016).
Finally, for same-species strains, the single enriched functions for each strain were studied based on Gene Ontology using the OrthoVenn2 server with default parameters (accessed on 12/15/2020; Xu et al., 2019). Protein sequences obtained from Prokka annotation (Seemann, 2014) were used as input. Briefly, similar proteins are obtained by all-against-all alignment with DIAMOND v0.9.24 (Buchfink et al., 2014) with a threshold of e-value = 0.05. Then, clusters are generated with MCL (inflation value = 1.5) and gene ontology (GO), and enrichment is assigned by similarity with the UniProt protein database (Apweiler et al., 2004). GO enrichment analysis computed the p-values for GO terms in a clusters overlapping using a hypergeometric distribution with the variables N = total count of all GO terms, M = count of GO terms in the overlap, n = total count of a GO term and x = count of the GO term that overlap on the following formula:
Genome mining of biosynthetic gene clusters
Genetic elements associated with the production of secondary metabolites (BGCs) on the So64.6b genome were identified using the antiSMASH v.5.1.0 tool (Blin et al., 2019) with default parameters and selecting all prediction features. Additionally, structure prediction of BGCs was performed on PRISM 3 (Skinnider et al., 2017). The closest known BGCs were assigned when matches were found compared to the MiBiG Cluster Repository of Known Biosynthetic Gene Clusters.
Clusters associated with the production of potentially novel antibiotic secondary metabolites were predicted with ARTS v2.0 (Mungan et al., 2020) with default parameters. A search for known resistance elements is performed by comparing with the curated databases: ResFams, The Comprehensive Antibiotic Resistance Database (CARD), The LACtamase Engineering Database (LACED), and The Jacobi and Bush Collection. In addition, essential genes are identified with TIGRfam Equivologs as the core genes from a reference set of genomes and classified according to: (a) rare duplication (possible resistant target genes), defined as all essential genes that exceed the median and standard deviation thresholds of the sum of essential genes identified in the bacterial family; (b) acquisition by horizontal transfer when comparing the topology of core gene phylogeny against species tree; and (c) their proximity to the BGCs identified with ANTISMASH 3 software (Mungan et al., 2020). This allowed the prediction of the mode of action of encoded compounds of an uncharacterized BGC based on the identification of resistant target genes (Mungan et al., 2020), improving the selection of the strains with the highest potential for antibiotic metabolites discovery.
Comparative genomic analysis
Selection of genomes data Set
The maximum number of representative complete genomes of the taxonomically closest species were obtained from the NCBI database (accessed December 20, 2021) and subsequently used for genomic and phylogenetic comparison of the selected strains. Only representative genomes from the closest species were included for pangenome comparative analysis (×49). The chosen reference genomes were simultaneously re-annotated using Prokka 1.12 (Seemann, 2014).
BGC evolutionary comparison across the closest taxon
To understand the evolutionary relationships between secondary metabolites related to the production of potentially novel antibiotic compounds, a phylogenetic analysis of the main synthases present in most of the genomes of the dataset was performed. To do this, the BGC of interest with an analogous antimicrobial production cluster (using a BGC from MiBIG as reference) was selected. The 10 clusters with the highest similarity belonging to taxonomically close species (same genus) were chosen for this evolutionary analysis. The BGCs were predicted from each genome using antiSMASH 5.0 as previously described. The output.gbk files were used to determine genomic variations in major biosynthetic enzyme contiguity with CORASON platform of the BiG-SCAPE/CORASON tool2. CORASON employs a phylogenomic approach to elucidate evolutionary relationships between gene clusters by calculating high-resolution multi-locus phylogenies of BGCs within and across gene cluster families (GCFs); moreover, it allows to comprehensively identify all genomic contexts in which gene clusters of interest (‘subclusters’ within larger BGCs) can be found (Navarro-Muñoz et al., 2020).
Briefly, a conserved core of the reference BGC (from MiBIG) within the genomic database is identified using an all-versus-all algorithm between each gene set to remove the paralogs and retain only the true orthologs. As a primary biosynthetic enzyme, a reference gene is selected to ensure that at least one element will be present in the conserved core and is also used to align the BGC variations in the graphical output visually. Core sequences are identified by the longest common subcluster between the PFAM of a pair of BGCs, in this case in comparison with the cluster from the Antarctic Sphingomonas. For each BGC, the core sequences are concatenated and then aligned using MUSCLE v.3.8.31. The alignments are curated using Gblocks with a minimum block length of five positions, a maximum of 10 contiguous non-conserved positions, and considering only positions with a gap in less than 50% of the sequences in the final alignment. If the curation results are empty, the non-conserved alignment will be used for the tree. Finally, a maximum likelihood phylogenetic tree was inferred using FastTree43 v.2.1.10 from the curated amino acid alignment (Navarro-Muñoz et al., 2020).
BGC characterization of Sphingomonas genus
Name, source of isolation, country of isolation, genome size, and accessions were obtained from each representative complete genome of Sphingomonas species on the GenBank database. The genome in fasta format was analyzed by the antiSMASH v.5.1.0 bacterial version database to predict BGCs. AntiSMASH results were organized in a 49 × 19 matrix with the number of clusters and the corresponding type of the predicted metabolite. The genetic content for BGC on each genome was also calculated by the percentage of the base pair of the total BGCs divided by the genome size. Data were classified according to the source of the sample of each strain (water, soil, plant, animal, or unknown); and by extreme, mesophilic, or unknown environment, depending on the environmental conditions on the region of isolation.
Pangenome analysis
To unique genomic profile of the Antarctic strain and putative gene fission/fusion/duplication events were identified by pangenome analysis against representative genomes of Sphingomonas. Sequences annotated in Prokka were used as input on PIRATE v1.0.4 package (Bayliss et al., 2019). Default parameters were used with 60%, 70%, 80%, 90%, 90%, and 95% cutoff for amino acid identity. Briefly, PIRATE filters and reduces the dataset by iterative clustering using CD-HIT (Li and Godzik, 2006). The longest sequence from each CD-HIT cluster is used as a proxy for sequence similarity search with BLAST (Camacho et al., 2009). The normalized bit scores of the resulting all-vs-all comparisons are clustered using Markov Clustering Algorithm (MCL; Dongen, 2000) after eliminating matches below the percentage identity threshold. Unique clusters at higher thresholds are identified as “unique alleles.” In addition, a genomic island prediction was performed with IslandViewer v4 (Bertelli et al., 2017) and IslandCompare v1.0 (Bertelli et al., 2022) was used to identify the unique alleles associated with mobile elements among the closer strains.
Results and discussion
Genome-based taxonomic and phylogenetic analysis revealed that the Antarctic strain So64.6b might be a new species closely related to Sphingomonas alpina
The genome of the Antarctic strain So64.6b consists of 5,570,175 pb. Phylogeny based on core proteome showed that strain So64.6b is genetically close to S. alpina, followed by Sphinogmonas sp. strains AAP5 (isolated from alpine lake), HMP6 (isolated from ultra-oligotrophic lake in East Antarctica), and the species S. aliaeris DH-S5 (obtained from pork steak) and S. panacis (obtained from rusty ginseng root in South Korea based on Genbank repository records; (Singh et al., 2015; Sanangelantoni et al., 2018; Kim et al., 2020). The phylogeny shows a closer genetic relation of the Antarctic strain with Sphingomonas alpina DSM 22537 (S8-3T strain), a species isolated from the Alpes (Margesin et al., 2012; Figure 1). Both strains also share a 99.6% identity of 16S rRNA, assuming they belong to the same species. However, ANI values have no coincidence over the threshold that defines species (>95–96%) since they share 86% identity. Most similarities are between 76% and 78% with other species of Sphingomonas. (Supplementary Figure S1). The result confirms that S. alpina is the closest species, but in silico DDH calculates a 38% hybridization between S. alpina and our Antarctic isolate genome. Hence, our work suggests that S. alpina is a close relative of Sphingomonas sp. So64.6b, a potentially novel species of the genus Sphingomonas. Given the phylogenic results and to further explore the relation and differences that define each strain, we conducted an ortholog clusters analysis.
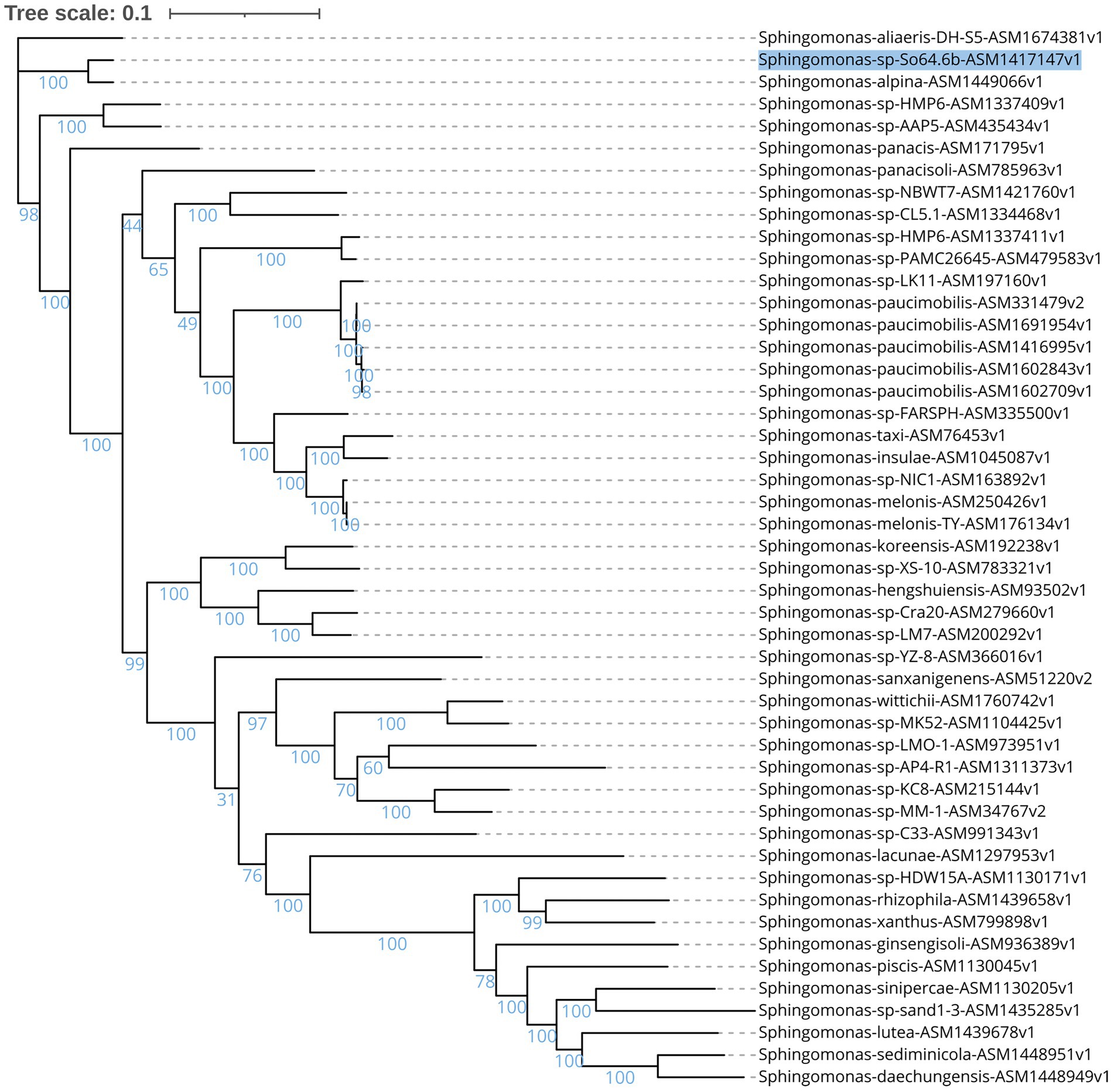
Figure 1. Maximun likelihood unrooted phylogenetic tree of proteome, based on sequences of concatenated amino acids from 126 ortholog genes present in 48 reference genomes from the Sphingomonas genus using RAxML. The accession number for the NCBI of each genome is present in the name. The branch lengths represent the number of substitutions per site (scale: 0.1 substitutions per site) and the percentage of the 1,000 replications bootstraps is also shown. Antarctic strain So64.6b is marked in blue.
Both Sphingomonas strains shared 3,385 ortholog clusters, while 98 clusters were unique for the So46.6b strain and only 52 for the Alpes strain (Supplementary Figure S2). The Antarctic strain presented an enrichment of the transposition functions (value of p = 0.00076) and catabolism of aromatic compounds (value of p = 0.00019), while the Alpes strains have the greatest function in the oxidoreductase activity (value of p = 0.00061). Clusters on the So46.6b strain showed 10 transposases–grouped in two ortholog clusters– and four insertion elements related to genetic mobility. In addition, it contains multiple proteins for the catabolism of polluting agents such as benzoate, gallate, lignin, xylene, and tetrahydrofolate (Supplementary Table S1). That could be related to the presence of these phenolic pollutants in the Antarctic environments, where a higher rate of redox reactions on pollutants/heavy metals at a cold temperature might increase their harmful potential (Ju et al., 2017; Zangrando et al., 2019). Alpine strain does not have any of these genes and showed only proteins related to the catabolism of xylene and lignin, commonly found in the Sphingomonas genus (Menon et al., 2019). Therefore, we suggest genomic differences among the strains might be in part caused by a large accessory genome related with adaptation to the harsh Antarctic environment.
BGCs of Sphingomonas sp. So64.6b might be related to the production of novel antibiotic molecules and showed evolutive divergences related to the isolation environment
The antiSMASH results are summarized in Table 1. Sphingomonas sp. So46.6b has a total of 6 BGCs related to polyketides synthases (PKS), terpene, two RiPP Recognition Elements-containing (RRE), lassopeptide, and a hybrid cluster lassopeptide-homoserine lactone. None of the BGCs have similarities to previously reported clusters from neither Sphingomonas nor other bacteria genres, excluding the terpene, which presented a low similarity (30%) to the known carotenoid BGC. This result indicates the potential of new untapped natural products from the Antarctic strain. Furthermore, BGCs prediction with the ARTS tool demonstrates that three of those clusters were also associated with the production of potentially novel antibiotic compounds due to their proximity with duplicated essential genes (×13), known resistant genes (×36), and recent horizontal gene transfer events (×189).
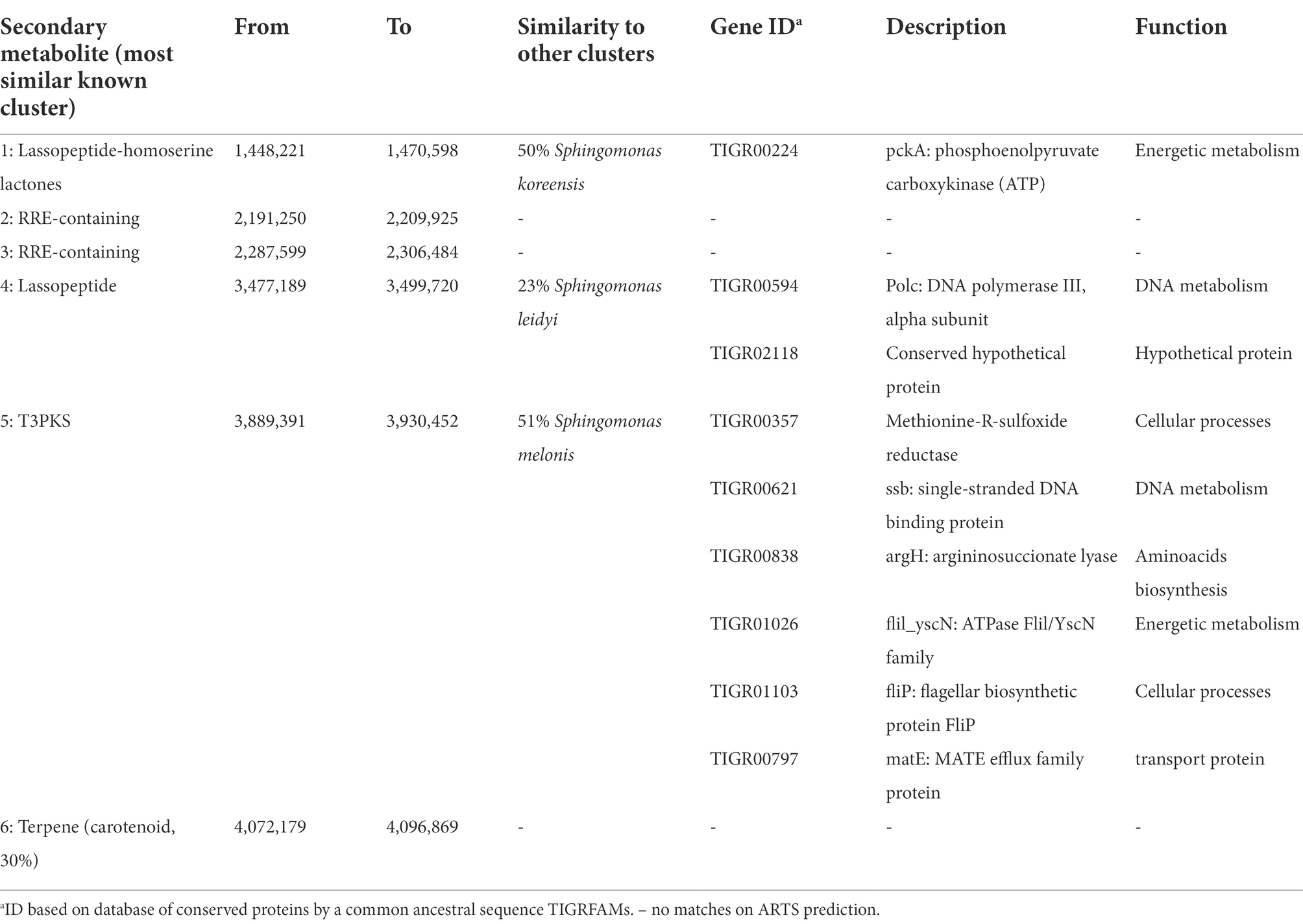
Table 1. Summary of Biosynthetic Gene Cluster (BGC) identified in the genome of Antarctic Sphingomonas sp. So64.6b and their genes related with auto-resistance to antibiotics when present on ARTS prediction.
Particularly we highlight the lassopeptide BGC-4 of Sphingomonas spSo64.6b as a potential novel antibiotic since it does not match with any other known BGC and has a low similarity with any other genetic cluster (Table 1, 23% shared genes with S. leidyi). Moreover, this cluster contains an essential gene related to resistance prediction due to duplication and Horizontal Gene Transfer (HGT) events, identified as a hypothetical protein (TIGR02118). A gene with this characteristic could be a resistant copy of the antibiotic target acquired by horizontal transfer along with the identified BGC (Alanjary et al., 2017). Most BGCs showed resistance genes related to typical functions of antibiotic activity, mainly in amino acid biosynthesis, protein production and transport, DNA synthesis, and central metabolism (Table 1). Although those BGCs might also produce unknown antibiotic compounds with usual targets, the BGC-4 cluster could be involved in more specific unknown activities; then, its lassopeptidic products could represent a new class of antibiotics with an unknown target molecule. The study of unknown targets for discovering new kinds of broad-spectrum antibiotics is considered one of the main strategies to fight against the antibiotic crisis (Matos De Opitz and Sass, 2020).
In addition, two molecular structures were predicted on PRIMS from the BGCs of So64.6b (Figure 2), one belonging to BGC-4. Both structures corresponded with lassopeptides types and showed high molecular weights (over 2,000 g/mol), which escapes from the detection spectrum of the antibiotic metabolites previously reported for this strain (Núñez-Montero et al., 2020). Such high molecular weight and predicted structure stand out the high molecular complexity of the compounds eventually produced by the BGC-4. This complexity in the lassopeptides structure appeals to their engineering regarding thermal stability, proteolytic protection, and chemical properties, promoting bioactive activities (Maksimov and Link, 2014).
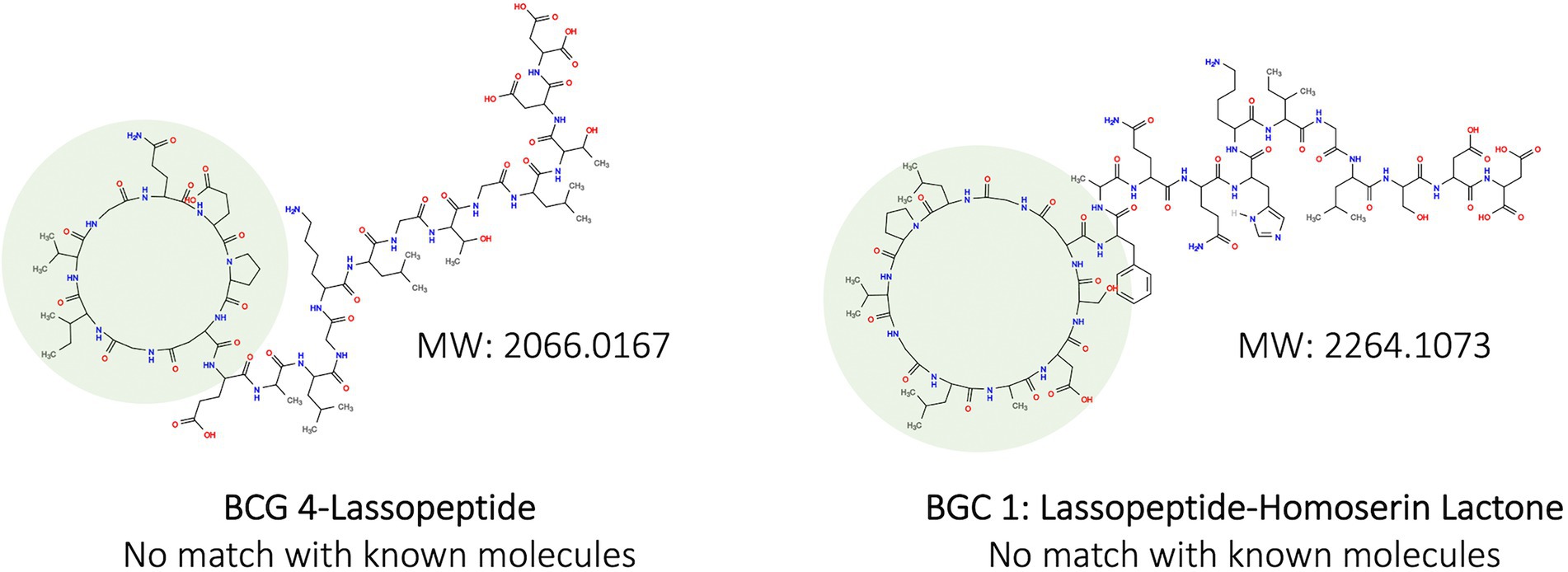
Figure 2. Predicted chemical structures produced by biosynthetic clusters in the genome of the Antarctic strain So64.6b based on PRISM detection. Macrocyclic ring formed by multiple N-terminal amino acid residues is highlighted in green. Remaining structure is the C-terminal tail of a lassopeptide. MW: molecular weight.
Since we hypothesize that the Antarctic environment might be partially responsible for the unknown diversity of BGCs found on the strain So64.6b, a phylogenetic analysis using CORASON was performed to identify genetic divergences of a common BGC between the Antarctic bacteria and other closely related Sphingomonas strains. This analysis is an alternative for prioritizing potentially novel BGCs based on comparative phylogenomics. Instead of comparing between full and partial merged BGCs as done with other tools, CORASON avoids artifact errors by a glocal-type comparison, which first finds the longest common subcluster between the PFAM of a pair of BGCs (including our Antarctic query strain) and continues with an extension of this alignment applying match/mismatch penalties (Navarro-Muñoz et al., 2020). The analysis was performed using the BGC-6 of strain So64.6b since this was the only cluster shared with other Sphingomonas species, including Antarctic strains, and matching a known BGC on MiBIG database. The synteny of genes was based on the carotenoid cluster, specifically by comparing the gene ctrl, coding for the phytoene desaturase enzyme. This enzyme converts phytoene or squalene to lycopene by desaturation in four places.
The result shows a unique and shared syntenic organization of the BGC across nine strains of the genus Sphingomonas, where 54–68% of the genes were found in all the strains (Figure 3A). Furthermore, the phylogenetic result showed a clear grouping of the cluster in three clades, with the Antarctic strain cluster being the most distant one from the rest of the sequences (Figure 3B). In this context, it is interesting to notice that the Antarctic So64.6b strain shares a clade with strains from similar extreme environments, including the PAMC strain obtained from the Arctic (Shin et al., 2012) –and the AAP strain, isolated from an Alpine lake (Kopejtka et al., 2020). Such environments shared extreme conditions such as high altitude, high UV radiations and exposure to freeze/thaw cycles. Besides, strains related to different environments, such as those isolated from plant microbiome or soil, are grouped apart from those of extreme environments (Figure 3B). The production of pigments has been reported in other Antarctic organisms to cope with high UV radiation (Rodrigues et al., 2021; Singh et al., 2022). Then, this carotenoid variations might play a role on the resistance to UV radiation. The result supports our hypothesis showing a genetic divergence on an Antarctic strain’s BGC; while such genetic differences might drive the variations observed in the biosynthetic modules, highlighting its potential for finding new molecules. In addition, that genetic divergence could be associated with the adaptation of this bacteria to an extreme environment. Specialized metabolites have evolved to mediate important ecological functions, explaining their diversity of chemical products (van Bergeijk et al., 2020). Our hypothesis established that some of those metabolites might be driven by the Antarctic environmental harsh conditions. Other authors have reports findings that are consistent with this theory. Among then, chromomycin, an antibiotic produced by Streptomyces flaviscleroticus showed antioxidant effect that confers resistance oxidative stress (Prajapati et al., 2019); the lipid-based esters, and Cys-GSH isomers were related to antioxidant response, significantly shifted by Cadmium concentrations in Scenedesmus obliquus (Mangal et al., 2020); secondary metabolites production and additional genes for specific peptides were found to potentially enhance the response to environmental changes on members of the order Candidatus Thermoprofundales, enabling them inhabit marine hydrothermal vents, deep subsurface oil reservoirs and hot springs (Liu et al., 2022); and the products of shikimate pathway including mycosporines and mycosporines-like-amino-acids which are well-known radiation resistant substances (Sarkar et al., 2018; Gacem et al., 2021).
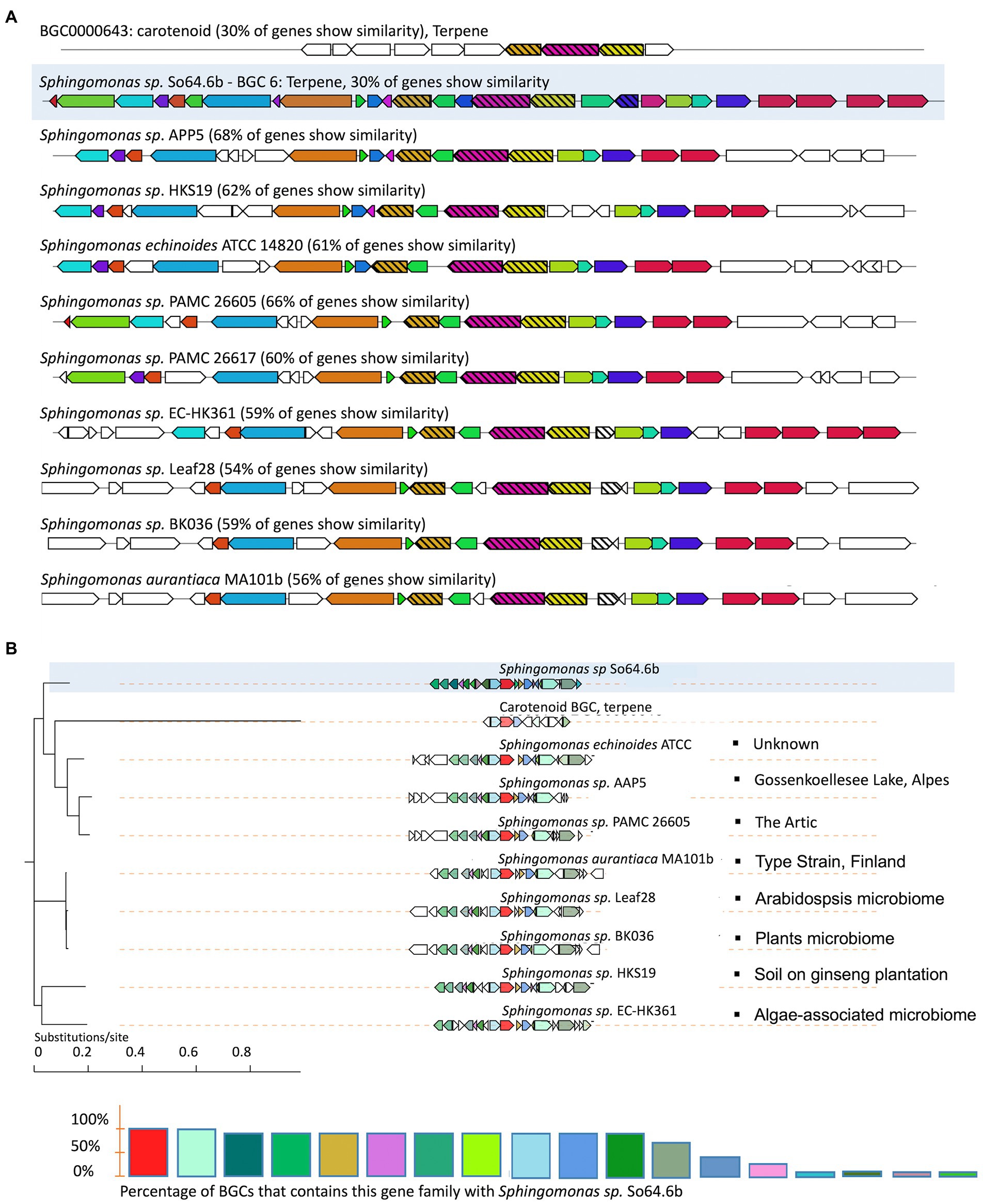
Figure 3. Variation in the genomic proximity of the terpene-like BGC-6 of the Antarctic strain So64.6b with similarity to the MiBIG reference BGC0000643 from Brevundimonas vesicularis (Tao et al., 2006). (A) Similarity plots of terpene BGCs from species of the genus Sphingomonas, including the Antarctic Sphingomonas sp. So64.6 (highlighted) and its closest species, mapped to the known carotenoid cluster BGC0000643 (on top). The colors match the shared genes, and those related with biosynthesis are shown in strip-pattern. (B) Terpene cluster phylogeny inferred by CORASON showing the frequency of occurrence of each gene family in comparison with the cluster from Antarctic strain So64.6b. Differences in sequence similarity are also indicated by a color gradient in the depicted gene blocks. The bullets at the end of each branch indicate the bacterial origin according to the metadata reported for the sample (Biosample data from NCBI database).
To further support this idea we checked whether the environmental conditions influences the overall composition and diversity of BGCs on Sphingomonas strains by comparing the predicted BGCs within this genus and between the representative genomes of Sphingomonas species isolated from different environments available on the Genbank database. An average of four clusters were found on 49 genomes analyzed, ranging from one in Sphingomonas sp. LM7, to eight in Sphingomonas sp. AP4-R1 (Figure 4). Previous studies regarding genome-mining of proteobacteria species also showed that the most common types of BGC were nonribosomal peptide synthetases (NRPS), PKS, and hybrids; however, lanthipeptides and terpenes are also present (Brotherton et al., 2018). NRPS and PKS are peptide biosynthesis machinery of natural products with multiple bioactivities (Giordano et al., 2015). Likewise, lanthipeptides and terpenes have been related to antimicrobial activity compounds (Giordano et al., 2015; Oladipo et al., 2022).
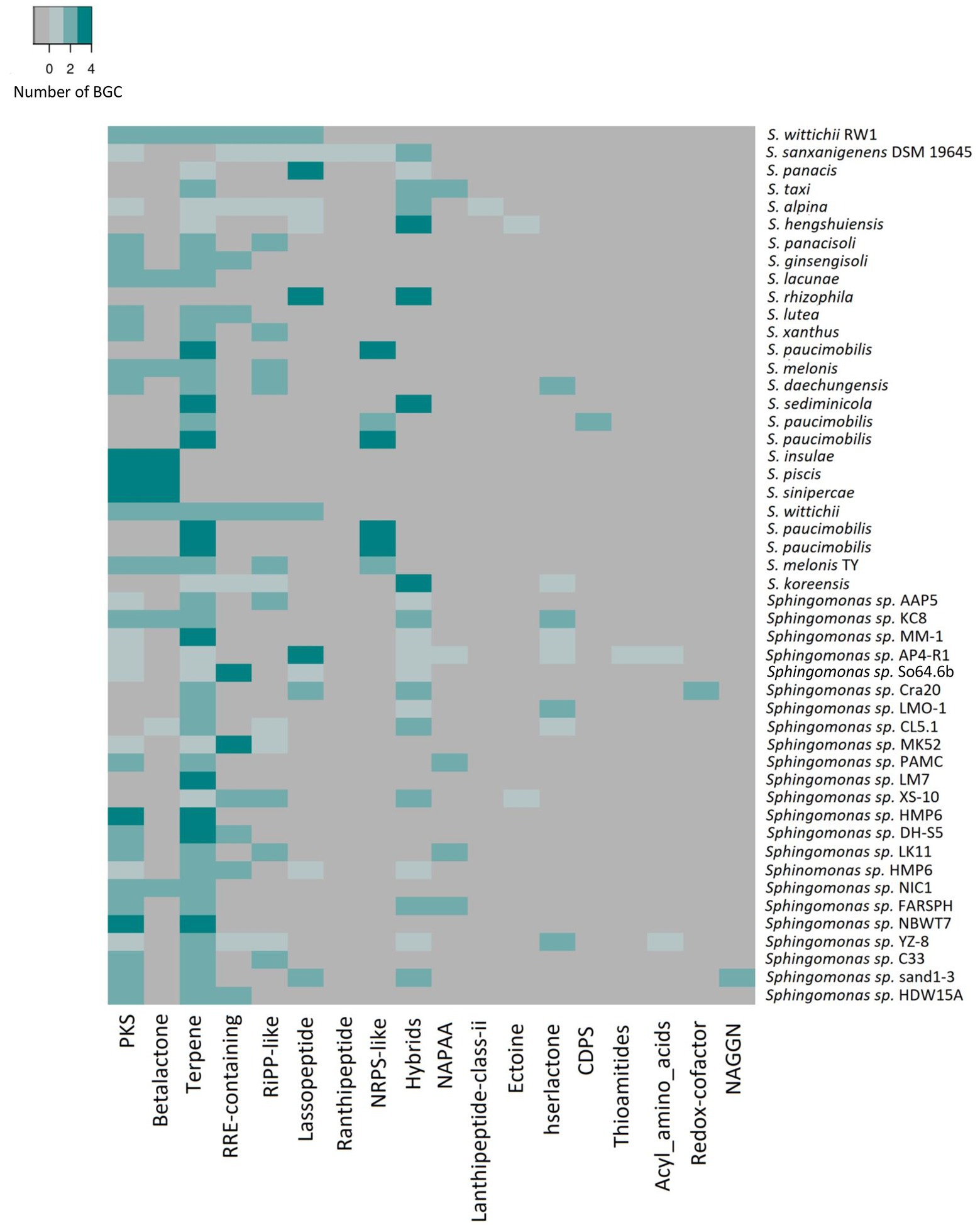
Figure 4. Heatmap of number and types of BGCs predicted by AntiSMASH analysis for 49 representative genomes of Sphingomonas genus, including the Antarctic strain So64.6b. The color scale represents the number of counts per type of BGC found on the genome.
The total amount of clusters in the strains was variable; then, to understand whether it can be affected by the origin of each sample, they were grouped by source of isolation from animals (×6), plants (×5), soil (×19), water (×12) or from an unknown source (×7) when it was not reported on Biosample Genbank information. The samples were also classified into extreme or mesophilic environments regarding the expected conditions of the region of the isolation source. Strains from water showed the greatest average of the total amount of predicted clusters (×4), while animal-isolated bacteria were the lowest. Nonetheless, soil isolated bacteria have the maximum number of clusters (×8), while plant isolated bacteria showed the lowest maximum (×4). In addition, animal-isolated bacteria had the greatest genetic content devoted to BGC in their genome (6.02%) compared to strains isolated from plants (2.66%), soil (2.60%), and water (4.20%; Figure 5C). This result suggests that the total amount of BGCs in the Sphingomonas might be related to the conditions they face in their specific samples. Also, strains from animals seem to have fewer BGCs, but with more genetic content on each cluster, which could be associated with a stronger regulation due to the interspecies communication or additional biosynthetic genes for modifications on the scaffold molecule to produce a larger number of derived products. Besides, soil and water samples were related to a larger amount of BGCs, mainly NRPS, PKS, and ribosomally synthesized and post-translationally modified peptides (RiPPs). These results are similar to other studies on Streptomyces mining for specialized metabolism and marine Pseudoalteromonas pangenome analysis (Cordero and Polz, 2014; Maansson et al., 2016; Nicault et al., 2020)
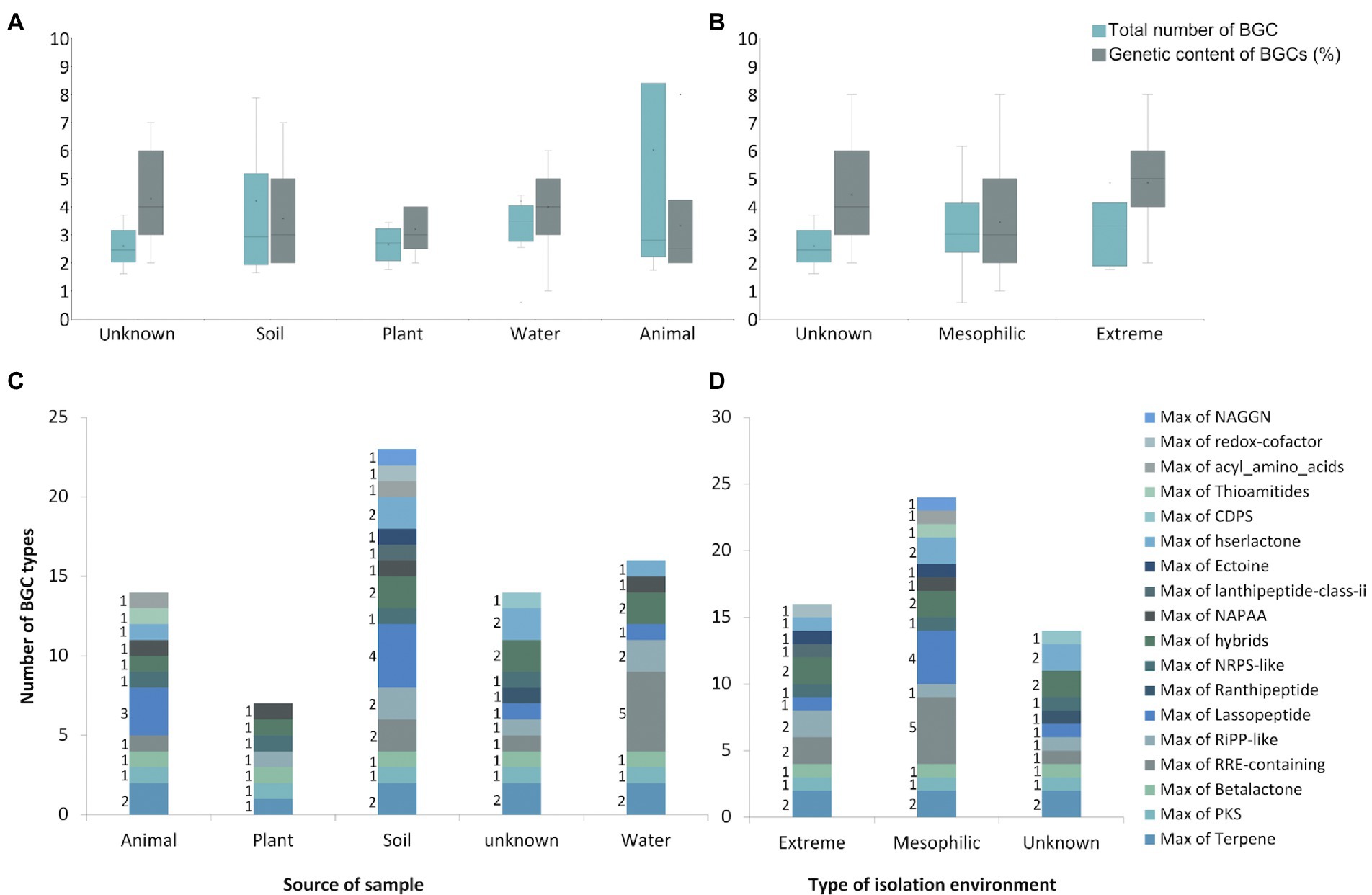
Figure 5. BGCs characterization of the Sphingomonas genus. (A) The Maximum number of BGC metabolites by the source of samples. (B) The Maximum number of BGC metabolites by environmental conditions. (C) Genetic content for BGCs and number of clusters by the source of the sample. (D) Genetic content for BGCs and number of clusters by environmental conditions. In C and D box plot are shown, where central lines of the box represent the median derived using the lower and upper quartile values. The maximum and minimum values are displayed with vertical lines connecting the points to the center box. NAGGN: N-acetylglutaminylglutamine amide dipeptide. CDPS: cyclodipeptides. NAPAA: Non-Alpha Poly-Amino group Acids. NRPS: Non-Ribosomal Peptide Synthetase. RiPP: Ribosomally synthesized and Post-translationally modified Peptides. RRE: RiPP precursor peptide Recognition Element. PKS: Polyketide Synthases.
In the environment-type context, seven bacteria were classified as isolated from extreme environments, 35 strains were isolated from mesophilic environments, and seven could not be classified. Bacteria from extreme environments had the greatest average of BGC (five), while mesophilic environments had the lowest (three). However, the same maximum number of clusters (eight) were found on strains from all environments. Interestingly, bacteria isolated from extreme environments also presented the greatest average of genetic content devoted to BGC (4.84% vs. 4.17% in mesophilic environment, Figure 5D). The results also indicate a major capacity for production of secondary metabolites in Sphingomonas isolated extreme environments, compared to the average percentage of 3.7% (± 3.1%) reported for proteobacteria phyla (Cimermancic et al., 2014).
To explore whether those differences were also found for particular types of BGCs –because of their different functionalities– the amount of each type of BGCs across the genomes was compared and related to the isolation origin. More than 16 types of BGCs were found across the Sphingomonas genomes. Interestingly, a maximum number of five clusters associated with the type of RRE-containing metabolites were found on species isolated from water. Conversely, other BGC types were less commonly found in the sum of similar samples, and, particularly, the RRE-containing type was present in less than two isolated species in other types of samples. In contrast, the plant isolated species did not have this type of BGC (Figure 5A). Besides, a higher number of lassopeptides-related clusters were found in soil and animal isolated species (four and three, respectively); one was identified in water and an unknown source, while none was present for plant isolated species (Figure 5A). In addition, the greatest amount of lassopeptides and RRE-containing metabolites were found in mesophilic environments (five and four, respectively). On the other hand, strains from extreme environments exclusively present clusters related to lanthipeptide class II and redox cofactor (Figure 5B). These can be associated with the adaption to the oxidative stress and low-temperature conditions of the Antarctic environment (Simpson and Codd, 2011; Grimalt-Alemany et al., 2021). The overall results showed that the number and some types of BGCs found on Sphingomonas genus seems to be associated with the environment they inhabit. Then BGC content in thus genus might be drive as evolutionary response to the requirements of the specific environment. Particularly, the identified BGCs in our soil-extreme environment bacteria might be related to an ecological advantage instead of taxonomic characteristics. This is expected since in those environments, bacteria deal with multiple physico-chemical complex changes, requiring a plethora of physiological and metabolic response (Cordero and Polz, 2014). Here we highlight the relevance of elucidating new natural products from strain So64.6b –isolated from polyextreme soil–, as others of biosynthetic interest in the Sphinogmonas species.
Pangenome analysis showed mobile genetic elements and resistance genes associated with the adaptation of Sphingomonas sp. So64.6b to the Antarctic environment
Since the Antarctic strain So64.6b had characteristics related to the metabolite production potential, we continue to study if this strain has other genetic elements particularly related to its adaptability to the Antarctic environment explaining its divergent genome content. From the pangenome analysis of the Sphingomonas genus, 34,242 genetic families were obtained, 1,546 were unique for the Antarctic strain, including metabolic pathways for the degradation of aromatic compounds previously mentioned. The result evidenced a significant genetic diversity of the genus with a vast number of unique genes belonging to one species. This is consistent with similar studies on comparative genomics of Sphingomonas, where it has been suggested that the presence of this group in a wide diversity of environments has led to a large genetic difference and a small core genome for the genus (Kim et al., 2020).
We identify multiple ribonucleases, duplicated genes for the synthesis of asparagine, kinases, and aminotransferases of serine exclusively present on the genome of the Antarctic So46.6b strain. Considering that a great genetic variability might be associated with the adaptation mechanisms of Sphingomonas species to specific conditions, this can be part of adaptation to extreme temperatures. For example, it has been described that highly catalytic enzymes at low temperatures have a greater amount of asparagine and serine than their mesophilic homologs, with more arginine and lysine (Qin et al., 2014).
In addition, the Antarctic strain shows multiple unique genes related to antibiotic and heavy metal resistance, such as CzcC protein for cobalt, zinc, and cadmium resistance; CorA for cobalt and magnesium transportations; copper resistance and transportation proteins; receptors and iron transporters (FepC and FieF); regulators for the resistance and reduction of mercury; metalo-beta-lactamases; multidrug excretion pumps (EmrA, EmrB, AcrB, MedtA-C-G, MexA, NorM, Stp, OqxB13-17); SugE for quaternary ammonium resistance, and penicillin-binding proteins. These genes might also have an important role in the Antarctic environment. Studies have reported the presence of pollutants in the Antarctic continent, including heavy metals (cadmium, zinc, vanadium, arsenic, and gold), antibiotics, and pesticides transported by natural processes through the air and water (Corsolini, 2009; Lo Giudice et al., 2013). Also, in this place, active volcanoes contribute a significant amount of heavy metals to the soil (Singh et al., 2007). Regarding antibiotic resistance, it has been described that the introduction of foreign microorganisms due to tourism and scientific activities increased the acquisition of these genes by HGT (Cowan et al., 2011).
Our results highlight the genetic dynamism of the Sphingomonas genus. Specifically for the Antarctic strain, we reported enrichment of ortholog genes related to transposons and resistance genes, usually transferable by plasmids, and pointed out multiple resistance genes. To confirm whether those unique genes of the Antarctic strain could have been acquired by mobile elements, driving the genetic differences among the Antarctic strain and the Alpine strain (closer relative), we studied the genomic islands in its genome. More genomic islands were identified on the genome of the Antarctic strain (Figure 6A) and most of them were unique for this genome (Figure 6B). Within those regions, we identified the unique genes of this strain related to degradation of benzoate, hydrolysis of ferulic acids, resistance to copper, nickel, and cobalt, as well as multiple transposases and insertion elements. Meanwhile, genomic island on Alpine strain were mostly composed of phage genes, transposases or hypothetical proteins. Some were already present in the Antarctic strain and only one gene of antibiotic resistance were found (erythromycin esterase) as evidence of adaptability genetic material. Hence these results suggest that the differential genetic elements of the Antarctic strain So64.6b could be recently acquired by HGT environments to cope with the Antarctic poly-extreme conditions. Previous studies regarding genetic diversity showed a correlation between taxonomic distance and the production of secondary metabolites featuring unique bioactive natural products (Hoffmann et al., 2018). Also, similar results were observed in Pseudoalteromonas sp., a model of adaptation to extreme environments. A notable genomic diversity on this group has been attributed to recent acquisition a large set of mobile elements, mainly by HGT (Parrilli et al., 2021). The results support our hypothesis that some of the BGCs detected in this strain might be linked to the adaptation to harsh conditions of the Antarctic; for example, those without similarity to any other clusters within its genus or phylogenetically closer to strains from similar environments. Therefore, the Sphingomonas sp. So64.6b strain should be considered a new source for mining and bioprospecting antimicrobials compounds.
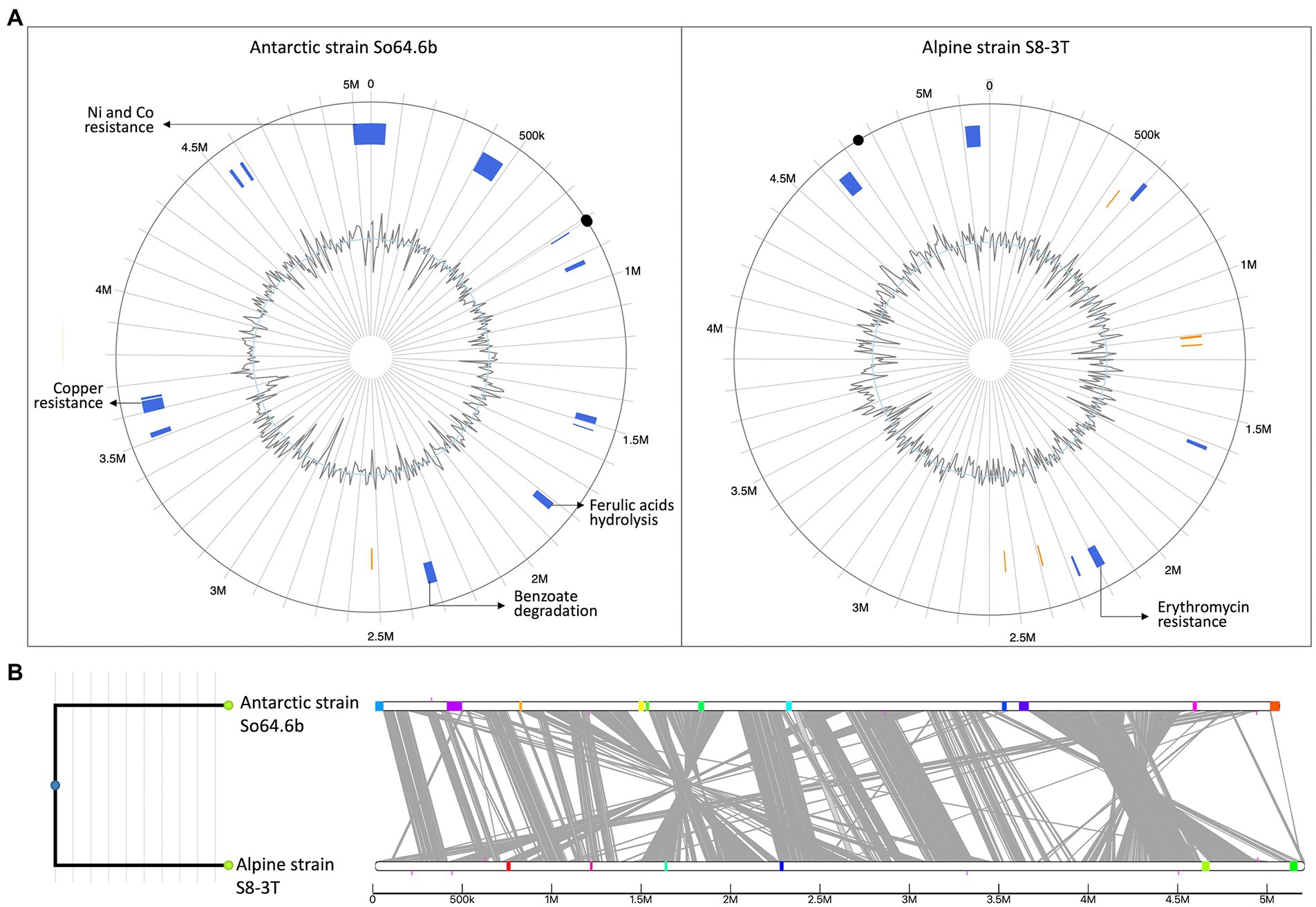
Figure 6. Genomic island (GIs) predictions and locations in two Sphingomonas genomes. (A) GI predictions using Island Viewer 4 and the two circular genomes of Antarctic Sphingomonas strain So64.6b (left) and Alpine strains S. alpina S8-3T (right). The circle represents a single chromosome, within the circle, GIs predictions are marked as colored blocks according to the prediction method; IslandPath-DIMOB (blue) and SIGI-HMM (orange). Evidence of genes related with adaptability, metabolism or resistances are described on pointing arrows. Inner line shows the change in GC skew. Black dots mark the OriC, predicted by proximity with recA, rnpA and dnaA and verified with Ori-Finder tool (Dong et al., 2022). (B) Comparative genomics visualization the Sphingomonas genomes. GIs are represented as coloured blocks placed on a linear representation of the genome (linear white bars indicate genomes, with alignments between genomes shown in grey). Same color represents matching GIs.
Strains belonging to the family Sphingomonadaceae have been explored due to their biotechnological applications in bioremediation and degradation of refractory pollutants and in the production of valuable biopolymers called sphinganes (Huang et al., 2009), which have an important role as growth promoters in plants. Particularly the genus Sphingomonas has been characterized as a degrader of multiple environmental pollutants, so, in addition to its role as a soil conditioner, it has a relevant ecological role in the recycling of complex molecules (Bhatt et al., 2020; Zhang et al., 2020). Also, due to its capacity to store heavy metals, it has improved plant resistance in environments with high zinc, cadmium, and copper contents, in addition to collaborating in stress conditions such as drought (Asaf et al., 2020). This background agrees with the genomic results observed in the Antarctic strain, showing a high capacity for resistance to metals and degradation of pollutants, which appear to be unique to the strain and absent even its closest relative. This highlights the influence that the selective pressure of the Antarctic environment may have exerted on the evolution, shaping a likely new species; and the genetic conformation of the Antarctic strain under study related with an essential role it probably plays in the Antarctic soil by protecting other organisms from harmful compounds. Moreover, the high genetic variation observed in this work, which has been previously reported for the genus Sphingomonas, together with the unique BGCs, make this Antarctic strain an important target in the searching for new metabolic pathways and the discovery of natural products.
Data availability statement
The datasets presented in this study can be found in online repositories. The names of the repository/repositories and accession number (s) can be found in the article/Supplementary material.
Author contributions
KN-M: conceptualization, methodology, validation, formal analysis, data curation, writing—original draft preparation, writing—review and editing, and visualization. DR-V: formal analysis, data curation, writing—original draft preparation, writing—review and editing, and visualization. LB: conceptualization, supervision, project administration, and funding acquisition. All authors contributed to the article and approved the submitted version.
Funding
This research was funded by grants from the Agencia Nacional de Investigacion y Desarrollo de Chile (ANID) - FONDECYT-1210563, the Instituto Antártico Chileno (INACH), INACH DG_01–19; Network for Extreme Environments Research (NEXER), NXR17-0003; INSTITUTO TECNOLÓGICO DE COSTA RICA project 5402–1510-1035, and CONICYT–PFCHA/Doctorado Nacional/2017–21170263 for KN-M.
Conflict of interest
The authors declare that the research was conducted in the absence of any commercial or financial relationships that could be construed as a potential conflict of interest.
Publisher’s note
All claims expressed in this article are solely those of the authors and do not necessarily represent those of their affiliated organizations, or those of the publisher, the editors and the reviewers. Any product that may be evaluated in this article, or claim that may be made by its manufacturer, is not guaranteed or endorsed by the publisher.
Supplementary material
The Supplementary material for this article can be found online at: https://www.frontiersin.org/articles/10.3389/fmicb.2022.1007225/full#supplementary-material
Footnotes
References
Al-Amoudi, S., Razali, R., Essack, M., Amini, M. S., Bougouffa, S., Archer, J. A. C., et al. (2016). Metagenomics as a preliminary screen for antimicrobial bioprospecting. Gene 594, 248–258. doi: 10.1016/j.gene.2016.09.021
Alanjary, M., Kronmiller, B., Adamek, M., Blin, K., Weber, T., Huson, D., et al. (2017). The antibiotic resistant target seeker (ARTS), an exploration engine for antibiotic cluster prioritization and novel drug target discovery. Nucleic Acids Res. 45, W42–W48. doi: 10.1093/nar/gkx360
Andrews, S. (2010). Fast QC: a quality control tool for high throughput sequence data. Bioinformatics Babraham.
Apweiler, R., Bairoch, A., Wu, C. H., Barker, W. C., Boeckmann, B., Ferro, S., et al. (2004). UniProt: the universal protein knowledgebase. Nucleic Acids Res. :32. doi: 10.1093/nar/gkh131
Asaf, S., Numan, M., Khan, A. L., and Al-Harrasi, A. (2020). Sphingomonas: from diversity and genomics to functional role in environmental remediation and plant growth. Crit. Rev. Biotechnol. 40, 138–152. doi: 10.1080/07388551.2019.1709793
Atterbury, R. J., and Tyson, J. (2021). Predatory bacteria as living antibiotics – where are we now? Microbiology (United Kingdom) 167, 1–8. doi: 10.1099/mic.0.001025
Aulestia, M., Flores, A., Mangas, E. L., Pérez-Pulido, A. J., Santero, E., and Camacho, E. M. (2021). Isolation and genomic characterization of the ibuprofen-degrading bacterium Sphingomonas strain MPO218. Environ. Microbiol. 23, 267–280. doi: 10.1111/1462-2920.15309
Auta, A., Hadi, M. A., Oga, E., Adewuyi, E. O., Abdu-Aguye, S. N., Adeloye, D., et al. (2019). Global access to antibiotics without prescription in community global access to antibiotics without prescription in community pharmacies: a systematic review and meta-analysis. J. Infect. 78, 8–18. doi: 10.1016/j.jinf.2018.07.001
Avram, O., Rapoport, D., Portugez, S., and Pupko, T. (2019). M1CR0B1AL1Z3R - a user-friendly web server for the analysis of large-scale microbial genomics data. Nucleic Acids Res. 47, W88–W92.
Babich, O., Shevchenko, M., Ivanova, S., Pavsky, V., Zimina, M., Noskova, S., et al. (2021). Antimicrobial potential of microorganisms isolated from the bottom sediments of lake baikal. Antibiotics 10:927. doi: 10.3390/antibiotics10080927
Bayliss, S, Thorpe, H, Coyle, N, Sheppard, S, and Feil, E. PIRATE: a fast and scalable pangenomics toolbox for clustering diverged orthologues in bacteria. bio Rxiv. [Preprint].
Bertelli, C., Gray, K. L., Woods, N., Lim, A. C., Tilley, K. E., Winsor, G. L., et al. (2022). Enabling genomic island prediction and comparison in multiple genomes to investigate bacterial evolution and outbreaks. Microb. Genom. 8. doi: 10.1099/mgen.0.000818
Bertelli, C., Laird, M. R., Williams, K. P., Lau, B. Y., Hoad, G., Winsor, G. L., et al. (2017). Island viewer 4: expanded prediction of genomic islands for larger-scale datasets. Nucleic Acids Res. 45, W30–W35. doi: 10.1093/nar/gkx343
Bhatt, P., Huang, Y., Rene, E. R., Kumar, A. J., and Chen, S. (2020). Mechanism of allethrin biodegradation by a newly isolated Sphingomonas trueperi strain CW3 from wastewater sludge. Bioresour. Technol. 305:123074. doi: 10.1016/j.biortech.2020.123074
Blin, K., Shaw, S., Steinke, K., Villebro, R., Ziemert, N., Lee, S. Y., et al. (2019). antiSMASH 5.0: updates to the secondary metabolite genome mining pipeline. Nucleic Acids Res. 47, 81–87. doi: 10.1093/nar/gkz310
Bredholt, H., Fjærvik, E., Johnsen, G., and Zotchev, S. B. (2008). Actinomycetes from sediments in the Trondheim Fjord, Norway: diversity and biological activity. Mar. Drugs 6, 12–24. doi: 10.3390/md0002
Brotherton, C. A., Medema, M. H., and Greenberg, E. P. (2018). lux R homolog-linked biosynthetic gene clusters in proteobacteria. mSystems 3:e00045-18. doi: 10.1128/mSystems.00208-17
Buchfink, B., Xie, C., and Huson, D. H. (2014). Fast and sensitive protein alignment using DIAMOND. Nat. Methods 12, 59–60. doi: 10.1038/nmeth.3176
Camacho, C., Coulouris, G., Avagyan, V., Ma, N., Papadopoulos, J., Bealer, K., et al. (2009). BLAST+: architecture and applications. BMC Bioinform. 10, 1–9. doi: 10.1186/1471-2105-10-421
Chen, S., Zhou, Y., Chen, Y., and Gu, J. (2018). Fastp: an ultra-fast all-in-one FASTQ preprocessor. Bioinformatics 34, 884–890. doi: 10.1093/bioinformatics/bty560
Chernikova, T. N., Bargiela, R., Toshchakov, S. V., Shivaraman, V., Lunev, E. A., Yakimov, M. M., et al. (2020). Hydrocarbon-degrading bacteria Alcanivorax and Marinobacter associated with microalgae Pavlova lutheri and Nannochloropsis oculata. Front. Microbiol. 11:2650.
Cimermancic, P., Medema, M. H., Claesen, J., Kurita, K., Wieland Brown, L. C., Mavrommatis, K., et al. (2014). Insights into secondary metabolism from a global analysis of prokaryotic biosynthetic gene clusters. Cells 158, 412–421. doi: 10.1016/j.cell.2014.06.034
Cordero, O. X., and Polz, M. F. (2014). Explaining microbial genomic diversity in light of evolutionary ecology. Nat. Rev. Microbiol. 12, 263–273. doi: 10.1038/nrmicro3218
Corsolini, S. (2009). Industrial contaminants in Antarctic biota. J. Chromatogr. A 1216, 598–612. doi: 10.1016/j.chroma.2008.08.012
Cowan, D. A., Chown, S. L., Convey, P., Tuffin, M., Hughes, K., Pointing, S., et al. (2011). Non-indigenous microorganisms in the Antarctic: assessing the risks. Trends Microbiol. 19, 540–548. doi: 10.1016/j.tim.2011.07.008
Danilovich, M. E., Sánchez, L. A., Acosta, F., and Delgado, O. D. (2018). Antarctic bioprospecting: in pursuit of microorganisms producing new antimicrobials and enzymes. Polar Biol. 41, 1417–1433. doi: 10.1007/s00300-018-2295-4
De Coster, W., D’Hert, S., Schultz, D. T., Cruts, M., and Van Broeckhoven, C. (2018). NanoPack: visualizing and processing long-read sequencing data. Bioinformatics 34, 2666–2669. doi: 10.1093/bioinformatics/bty149
Dong, M, Luo, H, and Gao, F. O. F. (2022). A comprehensive web server for prediction and analysis of bacterial replication origins. bio Rxiv [Preprint].
Fukuda, D., Haines, A. S., Song, Z., Murphy, A. C., Hothersall, J., Stephens, E. R., et al. (2011). A natural plasmid uniquely encodes two biosynthetic pathways creating a potent anti-MRSA antibiotic. PLoS One 6:e18031. doi: 10.1371/journal.pone.0018031
Gacem, M. A., Ould-El-Hadj-Khelil, A., Abd-Elsalam, K. A., and Wink, J. (2021). Actinobacteria in the Algerian Sahara: diversity, adaptation mechanism and special unexploited biotopes for the isolation of novel rare taxa. Biologia (Bratisl) 76, 3787–3799. doi: 10.1007/s11756-021-00928-1
Genilloud, O. (2017). Actinomycetes: still a source of novel antibiotics. Nat. Prod. Rep. 34, 1203–1232. doi: 10.1039/C7NP00026J
Giordano, D., Coppola, D., Russo, R., Denaro, R., Giuliano, L., Lauro, F. M., et al. (2015). Marine microbial secondary metabolites: pathways, evolution and physiological roles. Adv. Microb. Physiol. 66, 357–428.
Grimalt-Alemany, A., Etler, C., Asimakopoulos, K., Skiadas, I. V., and Gavala, H. N. (2021). ORP control for boosting ethanol productivity in gas fermentation systems and dynamics of redox cofactor NADH/NAD+ under oxidative stress. J. CO2 Util. 50:101589. doi: 10.1016/j.jcou.2021.101589
Hochvaldová, L., Večeřová, R., Kolář, M., Prucek, R., Kvítek, L., Lapčík, L., et al. (2022). Antibacterial nanomaterials: upcoming hope to overcome antibiotic resistance crisis. Nanotechnol. Rev. 11, 1115–1142. doi: 10.1515/ntrev-2022-0059
Hoffmann, T., Krug, D., Bozkurt, N., Duddela, S., Jansen, R., Garcia, R., et al. (2018). Correlating chemical diversity with taxonomic distance for discovery of natural products in myxobacteria. Nat. Commun. 9:803. doi: 10.1038/s41467-018-03184-1
Huang, H. D., Wang, W., Ma, T., Li, G. Q., Liang, F. L., and Liu, R. L. (2009). Sphingomonas sanxanigenens sp. nov., isolated from soil. Int. J. Syst. Evol. Microbiol. 59, 719–723. doi: 10.1099/ijs.0.000257-0
Jagannathan, S. V., Manemann, E. M., Rowe, S. E., Callender, M. C., and Soto, W. (2021). Marine Actinomycetes, new sources of biotechnological products. Mar. Drugs 19:365. doi: 10.3390/md19070365
Ju, J., Kim, J., Vetráková, Ľ., Seo, J., Heger, D., Lee, C., et al. (2017). Accelerated redox reaction between chromate and phenolic pollutants during freezing. J. Hazard. Mater. 329, 330–338.
Kim, Y. J., Park, J. Y., Balusamy, S. R., Huo, Y., Nong, L. K., Le, H. T., et al. (2020). Comprehensive genome analysis on the novel species Sphingomonas panacis DCY99T reveals insights into iron tolerance of ginseng. Int. J. Mol. Sci. 21:2019.
Klein, E. Y., van Boeckel, T. P., Martinez, E. M., Pant, S., Gandra, S., Levin, S. A., et al. (2018). Global increase and geographic convergence in antibiotic consumption between 2000 and 2015. Proc. Natl. Acad. Sci. U. S. A. 115, E3463–E3470. doi: 10.1073/pnas.1717295115
Kopejtka, K., Tomasch, J., Zeng, Y., Selyanin, V., Dachev, M., Piwosz, K., et al. (2020). Simultaneous presence of bacteriochlorophyll and Xanthorhodopsin genes in a freshwater bacterium. mSystems 5:e01044-20
Lamilla, C., Pavez, M., Santos, A., Hermosilla, A., Llanquinao, V., and Barrientos, L. (2017). Bioprospecting for extracellular enzymes from culturable Actinobacteria from the South Shetland Islands, Antarctica. Polar Biol. 40, 719–726. doi: 10.1007/s00300-016-1977-z
Lee, L. H., Cheah, Y. K., Nurul Syakima, A., Shiran, M., Tang, Y. L., Lin, H. P., et al. (2012). Analysis of Antarctic proteobacteria by PCR fingerprinting and screening for antimicrobial secondary metabolites. Genet. Mol. Res. 11, 1627–1641. doi: 10.4238/2012.June.15.12
Letunic, I., and Bork, P. (2016). Interactive tree of life (iTOL) v3: an online tool for the display and annotation of phylogenetic and other trees. Nucleic Acids Res. 44, W242–W245. doi: 10.1093/nar/gkw290
Li, W., and Godzik, A. (2006). Cd-hit: a fast program for clustering and comparing large sets of protein or nucleotide sequences. Bioinformatics 22, 1658–1659. doi: 10.1093/bioinformatics/btl158
Liao, S., Zhang, Y., Pan, X., Zhu, F., Jiang, C., Liu, Q., et al. (2019). Antibacterial activity and mechanism of silver nanoparticles against multidrug-resistant Pseudomonas aeruginosa. Int J Nanomed. 14, 1469–1487. doi: 10.2147/IJN.S191340
Liu, Y. F., Yang, L., Liu, Z. L., Chen, J., Fang, B., Zhou, L., et al. (2022). Discovery of the non-cosmopolitan lineages in Candidatus Thermoprofundales. Environ. Microbiol. 24, 3063–3080.
Lo Giudice, A., Casella, P., Bruni, V., and Michaud, L. (2013). Response of bacterial isolates from Antarctic shallow sediments towards heavy metals, antibiotics and polychlorinated biphenyls. Ecotoxicology 22, 240–250. doi: 10.1007/s10646-012-1020-2
Maansson, M., Vynne, N. G., Klitgaard, A., Nybo, J. L., Melchiorsen, J., Nguyen, D. D., et al. (2016). An integrated Metabolomic and genomic mining workflow to uncover the biosynthetic potential of bacteria. mSystems 1:e00028-15. doi: 10.1128/mSystems.00028-15
Maksimov, M. O., and Link, A. J. (2014). Prospecting genomes for lasso peptides. J. Ind. Microbiol. Biotechnol. 41, 333–344.
Mangal, V., Nguyen, T. Q., Fiering, Q., and Guéguen, C. (2020). An untargeted metabolomic approach for the putative characterization of metabolites from Scenedesmus obliquus in response to cadmium stress. Environ. Pollut. 266:115123. doi: 10.1016/j.envpol.2020.115123
Margesin, R., Zhang, D. C., and Busse, H. J. (2012). Sphingomonas alpina sp. nov., a psychrophilic bacterium isolated from alpine soil. Int. J. Syst. Evol. Microbiol. 62, 1558–1563. doi: 10.1099/ijs.0.035964-0
Matos De Opitz, C. L., and Sass, P. (2020). Tackling antimicrobial resistance by exploring new mechanisms of antibiotic action. Fut. Microbiol. 15, 703–708. doi: 10.2217/fmb-2020-0048
Meier-Kolthoff, J. P., Carbasse, J. S., Peinado-Olarte, R. L., and Göker, M. (2022). TYGS and LPSN: a database tandem for fast and reliable genome-based classification and nomenclature of prokaryotes. Nucleic Acids Res. 50, D801–D807. doi: 10.1093/nar/gkab902
Menon, R. R., Kumari, S., Kumar, P., Verma, A., Krishnamurthi, S., and Rameshkumar, N. (2019). Sphingomonas pokkalii sp. nov., a novel plant associated rhizobacterium isolated from a saline tolerant pokkali rice and its draft genome analysis. Syst. Appl. Microbiol. 42, 334–342.
Mungan, M. D., Alanjary, M., Blin, K., Weber, T., Medema, M. H., and Ziemert, N. (2020). ARTS 2.0: feature updates and expansion of the antibiotic resistant target seeker for comparative genome mining. Nucleic Acids Res. 48, W546–W552. doi: 10.1093/nar/gkaa374
Murray, C. J., Ikuta, K. S., Sharara, F., Swetschinski, L., Robles Aguilar, G., Gray, A., et al. (2022). Global burden of bacterial antimicrobial resistance in 2019: a systematic analysis. Lancet 399, 629–655. doi: 10.1016/S0140-6736(21)02724-0
Navarro-Muñoz, J. C., Selem-Mojica, N., Mullowney, M. W., Kautsar, S. A., Tryon, J. H., Parkinson, E. I., et al. (2020). A computational framework to explore large-scale biosynthetic diversity. Nat. Chem. Biol. 16, 60–68. doi: 10.3390/biom10050673
Newman, D. J., and Cragg, G. M. (2016). Natural products as sources of new drugs from 1981 to 2014. J. Nat. Prod. 79, 629–661. doi: 10.1021/acs.jnatprod.5b01055
Nicault, M., Tidjani, A. R., Gauthier, A., Dumarcay, S., Gelhaye, E., Bontemps, C., et al. (2020). Mining the biosynthetic potential for specialized metabolism of a Streptomyces soil community. Antibiotics 9:271. doi: 10.3390/antibiotics9050271
Núñez-Montero, K., Quezada-Solís, D., Khalil, Z. G., Capon, R. J., Andreote, F. D., and Barrientos, L. (2020). Genomic and metabolomic analysis of Antarctic bacteria revealed culture and elicitation conditions for the production of antimicrobial compounds. Biomolecules 10:673.
Oladipo, A., Enwemiwe, V., Ejeromedoghene, O., Adebayo, A., Ogunyemi, O., and Fu, F. (2022). Production and functionalities of specialized metabolites from different organic sources. Meta 12:534. doi: 10.3390/metabo12060534
Parrilli, E., Tedesco, P., Fondi, M., Tutino, M. L., Lo Giudice, A., de Pascale, D., et al. (2021). The art of adapting to extreme environments: the model system Pseudoalteromonas. Phys. Life Rev. 36, 137–161. doi: 10.1016/j.plrev.2019.04.003
Prajapati, D., Kumari, N., Dave, K., Chatupale, V., and Pohnerkar, J. (2019). Chromomycin, an antibiotic produced by Streptomyces flaviscleroticus might play a role in the resistance to oxidative stress and is essential for viability in stationary phase. Environ. Microbiol. 21, 814–826. doi: 10.1111/1462-2920.14515
Pritchard, L., Glover, R. H., Humphris, S., Elphinstone, J. G., and Toth, I. K. (2016). “Genomics and taxonomy in diagnostics for food security: soft-rotting enterobacterial plant pathogens” in Analytical methods (London, United Kingdom: Royal Society of Chemistry), 12–24.
Puri, A. W. (2019). Specialized metabolites from methylotrophic proteobacteria. Curr. Issues Mol. Biol. 33, 211–223. doi: 10.21775/cimb.033.211
Qin, Q. L., Xie, B. B., Yu, Y., Shu, Y. L., Rong, J. C., Zhang, Y. J., et al. (2014). Comparative genomics of the marine bacterial genus G laciecola reveals the high degree of genomic diversity and genomic characteristic for cold adaptation. Environ. Microbiol. 16, 1642–1653. doi: 10.1111/1462-2920.12318
Rodrigues, E., Silva, T., Francelino Silva, L. C., Cavalcanti de Queiroz, A., Alexandre Moreira, M. S., de Carvalho Fraga, C. A., et al. (2021). Pigments from Antarctic bacteria and their biotechnological applications. Crit. Rev. Biotechnol. 41, 809–826. doi: 10.1080/07388551.2021.1888068
Romanenko, L. A., Uchino, M., Frolova, G. M., Tanaka, N., Kalinovskaya, N. I., Latyshev, N., et al. (2007). Sphingomonas molluscorum sp. nov., a novel marine isolate with antimicrobial activity. Int. J. Syst. Evol. Microbiol. 57, 358–363. doi: 10.1099/ijs.0.64441-0
Sanangelantoni, A. M., Malatrasi, M., Trivelloni, E., Visioli, G., and Agrimonti, C. (2018). A novel β-propeller p.hytase from the dioxin-degrading bacterium Sphingomonas wittichii RW-1. Appl. Microbiol. Biotechnol. 102, 8351–8358. doi: 10.1007/s00253-018-9248-2
Sarkar, I., Tisa, L. S., Gtari, M., and Sen, A. (2018). Biosynthetic energy cost of potentially highly expressed proteins vary with niche in selected actinobacteria. J. Basic Microbiol. 58, 154–161. doi: 10.1002/jobm.201700350
Seemann, T. (2014). Prokka: rapid prokaryotic genome annotation. Bioinformatics 30, 2068–2069. doi: 10.1093/bioinformatics/btu153
Shin, S. C., Ahn, D. H., Lee, J. K., Kim, S. J., Hong, S. G., Kim, E. H., et al. (2012). Genome sequence of Sphingomonas sp. strain PAMC 26605, isolated from arctic lichen (Ochrolechia sp.). J. Bacteriol. :1607. doi: 10.1128/JB.00004-12
Simpson, P. J. L., and Codd, R. (2011). Cold adaptation of the mononuclear molybdoenzyme periplasmic nitrate reductase from the Antarctic bacterium Shewanella gelidimarina. Biochem. Biophys. Res. Commun. 414, 783–788. doi: 10.1016/j.bbrc.2011.10.003
Singh, P., Kim, Y. J., Hoang, V. A., Farh, M. E. A., and Yang, D. C. (2015). Sphingomonas panacis sp. nov., isolated from rhizosphere of rusty ginseng. Antonie van Leeuwenhoek 108, 711–720. doi: 10.1007/s10482-015-0527-y
Singh, B. K., Munro, S., Potts, J. M., and Millard, P. (2007). Influence of grass species and soil type on rhizosphere microbial community structure in grassland soils. Appl. Soil Ecol. 36, 147–155. doi: 10.1016/j.apsoil.2007.01.004
Singh, J., Singh, R. P., and Khare, R. (2022). “Higher rate of pigment synthesis in Antarctic plants: a strategy of survival under UV radiations” in Assessing the Antarctic Environment from Climate Change Perspective (Cham, Switzerland: Springer), 255–275.
Skinnider, M. A., Merwin, N. J., Johnston, C. W., and Magarvey, N. A. (2017). PRISM 3: expanded prediction of natural product chemical structures from microbial genomes. Nucleic Acids Res. 45, W49–W54. doi: 10.1093/nar/gkx320
Stamatakis, A. (2014). RAxML version 8: a tool for phylogenetic analysis and post-analysis of large phylogenies. Bioinformatics 30, 1312–1313. doi: 10.1093/bioinformatics/btu033
Tao, L., Rouvière, P. E., and Cheng, Q. (2006). A carotenoid synthesis gene cluster from a non-marine Brevundimonas that synthesizes hydroxylated astaxanthin. Gene 379, 101–108. doi: 10.1016/j.gene.2006.04.017
Timmermans, M. L., Paudel, Y. P., and Ross, A. C. (2017). Investigating the biosynthesis of natural products from marine proteobacteria: a survey of molecules and strategies. Mar. Drugs 15:235. doi: 10.3390/md15080235
van Bergeijk, D. A., Terlouw, B. R., Medema, M. H., and van Wezel, G. P. (2020). Ecology and genomics of actinobacteria: new concepts for natural product discovery. Nat. Rev. Microbiol. 18, 546–558. doi: 10.1038/s41579-020-0379-y
Wick, R. R., Judd, L. M., Gorrie, C. L., and Holt, K. E. (2017). Unicycler: resolving bacterial genome assemblies from short and long sequencing reads. PLoS Comput. Biol. 13. doi: 10.1371/journal.pcbi.1005595
Xu, L., Dong, Z., Fang, L., Luo, Y., Wei, Z., Guo, H., et al. (2019). OrthoVenn2: a web server for whole-genome comparison and annotation of orthologous clusters across multiple species. Nucleic Acids Res. 47, W52–W58. doi: 10.1093/nar/gkz333
Zangrando, R., Corami, F., Barbaro, E., Grosso, A., Barbante, C., Turetta, C., et al. (2019). Free phenolic compounds in waters of the Ross Sea. Sci. Total Environ. 650, 2117–2128. doi: 10.1016/j.scitotenv.2018.09.360
Zhang, J., Gan, W., Zhao, R., Yu, K., Lei, H., Li, R., et al. (2020). Chloramphenicol biodegradation by enriched bacterial consortia and isolated strain Sphingomonas sp. CL5.1: the reconstruction of a novel biodegradation pathway. Water Res. 187:116397
Zhang, J., Li, X., Lei, H., Zhao, R., Gan, W., Zhou, K., et al. (2022). New insights into thiamphenicol biodegradation mechanism by Sphingomonas sp. CL5.1 deciphered through metabolic and proteomic analysis. J. Hazard. Mater. 426:128101. doi: 10.1016/j.jhazmat.2021.128101
Keywords: natural products, antibiotics discovery, extreme environments, Antarctic, genome mining
Citation: Núñez-Montero K, Rojas-Villalta D and Barrientos L (2022) Antarctic Sphingomonas sp. So64.6b showed evolutive divergence within its genus, including new biosynthetic gene clusters. Front. Microbiol. 13:1007225. doi: 10.3389/fmicb.2022.1007225
Edited by:
Birgit Sattler, University of Innsbruck, AustriaReviewed by:
Juan Antonio Ugalde, Andres Bello University, ChileAngelina Lo Giudice, Institute of Polar Sciences (CNR), Italy
Hindra Hindra, McMaster University, Canada
Copyright © 2022 Núñez-Montero, Rojas-Villalta and Barrientos. This is an open-access article distributed under the terms of the Creative Commons Attribution License (CC BY). The use, distribution or reproduction in other forums is permitted, provided the original author(s) and the copyright owner(s) are credited and that the original publication in this journal is cited, in accordance with accepted academic practice. No use, distribution or reproduction is permitted which does not comply with these terms.
*Correspondence: Leticia Barrientos, bGV0aWNpYS5iYXJyaWVudG9zQHVmcm9udGVyYS5jbA==