- 1Industrial Crop Research Institute, Heilongjiang Academy of Agricultural Sciences, Harbin, China
- 2College of Agronomy, Northeast Agricultural University, Harbin, China
Alfalfa mosaic virus (AMV) is an important plant virus causing considerable economic loss to alfalfa production. Knowledge of the evolutionary and demographic history of the pathogen is limited but essential to the development of effective and sustainable pathogen management schemes. In this study, we performed worldwide phylodynamic analyses of AMV based on 154 nucleotide sequences of the coat protein gene, sampled from 1985 to 2020, to understand the epidemiology of this pathogen. Bayesian phylogenetic reconstruction estimates that the crown group of AMV dates back to 1840 (95% credibility interval, 1687–1955). We revealed that AMV continuously evolves at a rate of 4.14 × 10−4 substitutions/site/year (95% credibility interval, 1.04 × 10−4 − 6.68 × 10−4). Our phylogeographic analyses identified multiple migration links between Europe and other regions, implying that Europe played a key role in spreading the virus worldwide. Further analyses showed that the clustering pattern of AMV isolates is significantly correlated to geographic regions, indicating that geography-driven adaptation may be a factor that affects the evolution of AMV. Our findings may be potentially used in the development of effective control strategies for AMV.
Introduction
Bayesian phylodynamic inference is recognized as one of the most extensively used methods in estimating how epidemics occur and in tracking their geographic spread, particularly RNA viruses, which rapidly accumulate genetic variation because of the lack of proofreading abilities by their replicases. Comprehensive analyses of the evolutionary dynamics of important pathogens can provide a view of the epidemiology and human-mediated spread of pathogens through time and space. To date, most phylodynamic studies have focused on key human RNA viruses, including influenza virus (Lam et al., 2008), dengue virus (Wei and Li, 2017), and SARS-CoV-2 (Lemey et al., 2020). However, the range of pathogens to which phylodynamic inference are applied is expanding. In recent decades, it has been applied to many important plant viruses such as tobacco mosaic virus (Gao et al., 2019), potato virus Y (Gao et al., 2020), and turnip mosaic potyvirus (Kawakubo et al., 2021). Understanding the evolution of emerging plant viruses can be of great importance for devising strategies to control the virus.
Alfalfa (Medicago sativa L.) or lucerne is a major forage crop that is cultivated worldwide. Alfalfa acreage in the world was maintained at a relatively stable level from the 1960s to 1980s. The cultivation area of alfalfa worldwide was 33 million ha in this period (Pan et al., 2017). However, this was followed by a sharp increase, reaching a peak in 1990.1 After the mid-2010s, the cultivation area of alfalfa decreased (Xie et al., 2021). However, North America remains the largest producer of alfalfa in the world, accounting for nearly 50% of the global production of alfalfa. Currently, the leading alfalfa producing countries are the United States, European Union, Argentina, Russia, Canada, and Australia. Comparatively, the leading alfalfa importing countries in descending order are the UAE, Saudi Arabia, Germany, Japan, and Jordan in 2020.2 However, the global production of alfalfa declined in recent years due to the SARS-CoV-2 pandemic.
Alfalfa mosaic virus, a species of the genus Alfamovirus within the family Bromoviridae, is an economically important pathogen in alfalfa worldwide (Hull, 1969; Lefkowitz et al., 2018). This virus has a wide host range, although its natural hosts mainly include the Fabaceae and Solanaceae families (Bol, 2003). AMV causes various mosaic, mottled, and calico blotching malformations, and necrosis (Hull, 1969; Jones et al., 2012), leading to crop losses of 14.8–22.8% losses in fresh weight and 15.0–18.1% in dry weight (Bailiss and Ollennu, 1986). It is transmitted by aphids in a non-persistent manner (Bol, 2003; Leur et al., 2019). Furthermore, AMV is transmitted mechanically and by plant seeds (Zhang and Guo, 1983; Jones and Pathipanawat, 1989; Valkonen et al., 1992; Jones and Coutts, 1996; He et al., 2010; Fidan et al., 2012). Moreover, weeds and cultivated plants also play an important role in AMV epidemiology because they serve as virus reservoirs and over-summer hosts for aphids, and thus enhanced the persistence and prevalence of AMV (Ormeño et al., 2006; Freeman and Aftab, 2011; Abdalla et al., 2020).
The genome of AMV consists of tripartite single-stranded positive-sense genomic RNA (RNA1, RNA2, and RNA3) and a subgenomic RNA 4 (Lefkowitz et al., 2018). RNA1 and RNA2 encode replicase subunits, P1 and P2, respectively. RNA 3 encodes the movement protein and the viral coat protein (CP) which is translated from the subgenomic RNA 4. The CP is thought to play an important role in the translational efficiency of RNAs, nucleolar and cytoplasmic shuttling, RNA-binding activity, virion formation, and systemic movement (Herranz et al., 2012). In addition, CP is commonly used as a molecular marker in the phylogenetic reconstruction of viruses belonging to the family Bromoviridae as well as other plant viruses (Codoñer et al., 2005; Duan et al., 2018; Guan et al., 2018; Xu et al., 2021).
Phylogenetically, all AMV isolates can be clustered into monophyletic groups. The separation seemed to correlate with differences in their geographic origins but not with differences in their host range or pathogenicity (Parrella et al., 2000). Based on restriction fragment length polymorphism analysis, Bergua et al. (2014) found that the AMV population was structured according to their geographic origin. Although there are many previous studies that focused on the genetic diversity and molecular evolution of AMV, most of the studies focused on the phylogenetic, recombination, and selection analyses at a national scale or on a specific host (Komorowska et al., 2021; Guo et al., 2022; Trucco et al., 2022). There are few studies on the evolutionary history and global spatio-temporal dynamics, yet may be valuable to the development of effective and sustainable pathogen management schemes.
In the present study, we conducted temporal dynamic, phylogeographic, and demographic history analysis on the 154 nucleotide sequences of the CP gene of AMV during the years from 1985 to 2020, to provide insights into the evolution and global spatiotemporal pattern of this pathogen.
Materials and methods
Alfalfa mosaic virus sequence dataset
One hundred and fifty-four nucleotide sequences of the CP gene of AMV isolates with known sampling dates and country of origins were downloaded from GenBank (Supplementary Table S1). The sampling regions and dates of these AMV isolates are presented in Figure 1. The viral isolates from 17 countries between 1985 and 2020 were geocoded and grouped into six populations according to their geographical origins: Asia (AS, n = 41), Europe (EU, n = 20), Middle East (ME, n = 45), North America (NAm, n = 30), Oceania (OC, n = 16), and South America (SAm, n = 2). We performed a codon-based alignment of the CP sequences with the MUSCLE algorithm (Edgar, 2004) implemented in MEGA X (Kumar et al., 2018).
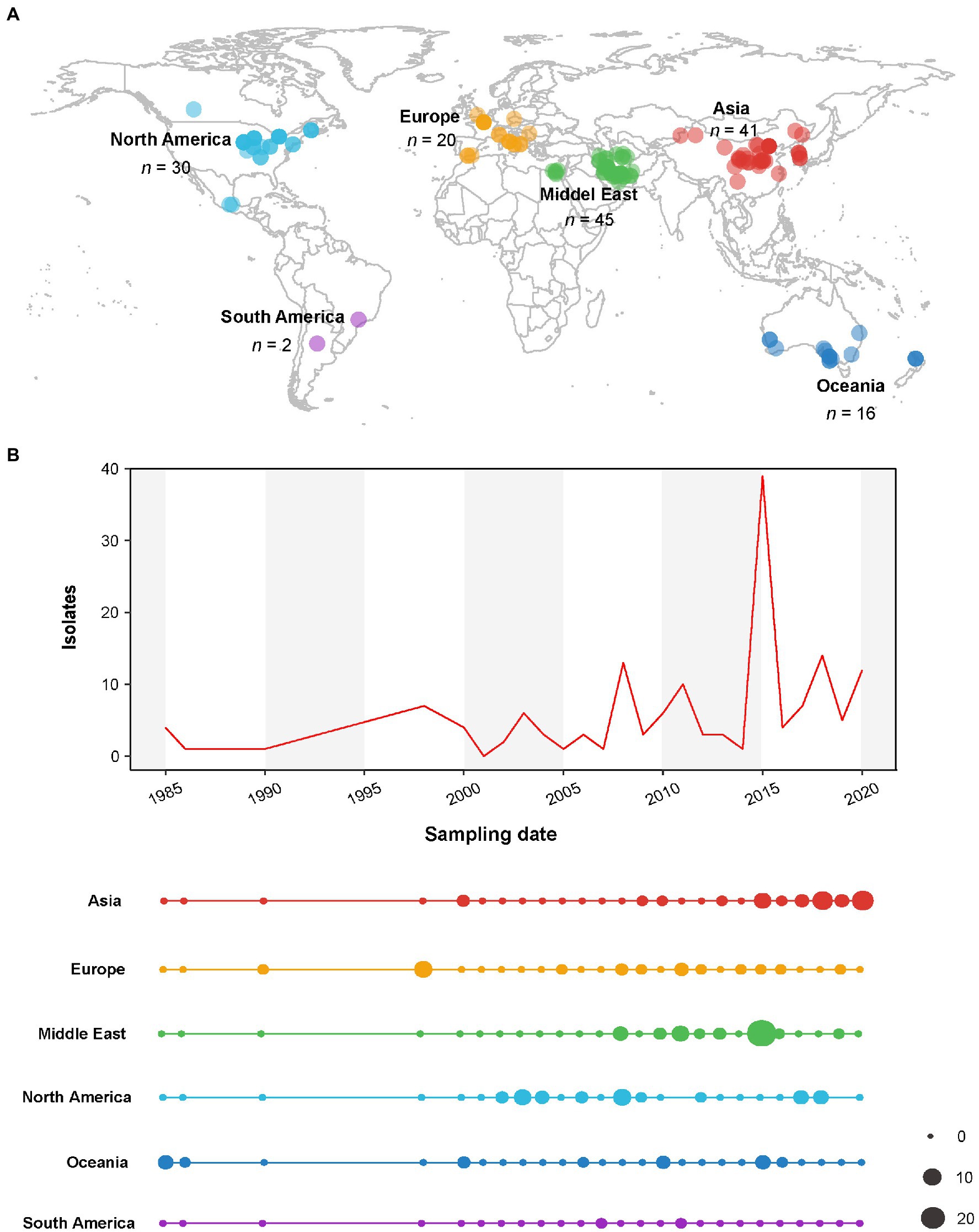
Figure 1. Spatial and temporal distribution of alfalfa mosaic virus (AMV) isolates used in this study. (A) Map illustrating geographic locations of AMV. (B) The total sample size and the distribution across the geographic locations over time (years). The dot sizes are proportional to the sample sizes.
Recombination and likelihood-mapping analysis
To identify recombination signals within the CP sequences, we initially calculated the pairwise homoplasy index (PHI) using SplitsTree 4.13.1 (Huson, 1998) and subsequently screened for signals of recombination using the RDP4 suite (Martin et al., 2015), which provides formal testing for recombination using up to seven different algorithms: RDP, GENECONV, BOOTSCAN, MAXCHI, CHIMAERA, SISCAN, and 3SEQ. To minimize false identification, only the events detected by at least four algorithms were accepted, with an associated p-value of 10−6. No statistically significant evidence of recombination was observed in the data by either of the two methods; hence, the complete data set was used in subsequent analyses.
To examine the phylogenetic signal contained in the data set, a likelihood-mapping analysis (Strimmer and von Haeseler, 1997) based on the maximum likelihood was performed using the program IQ-TREE 2.13 (Nguyen et al., 2015) with 4,000 quartets randomly drawn. We found a fair amount of star-likeness, with 23.2% of all quartet points in region A (<33.0%, Supplementary Figure S1), indicating a stronger tree-like phylogenetic signal in our data set, which allowed for a reliable phylogeny inference.
Assessing the temporal signal
To assess the clock-like behavior of our data set, we first calculated the correlation coefficients (r) of a regression of the root-to-tip genetic distance against the sampling time using TempEst (Rambaut et al., 2016). For this analysis, we estimated the tree topology and branch lengths using maximum likelihood analysis in IQ-TREE (Nguyen et al., 2015) with the SYM + I + G4 substitution model, which was selected based on the Bayesian information criterion calculated using ModelFinder (Kalyaanamoorthy et al., 2017) implemented in PhyloSuite 1.2.1 (Zhang et al., 2020). We then assessed the extent of the temporal structure using the recently developed BETS (Duchene et al., 2020). This approach compared the fit to data of two competing models, a heterochronous model (Mhet) and an isochronous model (Miso), using the marginal likelihood estimated by generalized stepping-stone sampling (Baele et al., 2016). For the Mhet, the data were accompanied by the heterochronous sampling time (with tip dates), and for the Miso, the actual sampling time was constrained to be contemporaneous (without tip dates). The best fit model to the data set was selected using Bayes factors (BF; Kass and Raftery, 1995). The results yielding a (log) BF log[P(Y|Mhet)]−log[P(Y|Miso)], and a value of at least five was considered as positive evidence for Mhet over Miso, suggesting the presence of a sufficient temporal signal in the data.
Bayesian phylogenetic analysis
To infer the evolutionary rate and timescale of AMV, we employed Bayesian coalescent approaches implemented in BEAST 1.10.4 (Suchard et al., 2018) under the best fit substitution model, as described above. To compare the fit of the constant size, exponential growth, and Bayesian skyline coalescent tree priors, we calculated marginal likelihoods using path and stepping-stone sampling (Baele et al., 2012). Therefore, this model combination was used for Markov chain Monte Carlo (MCMC) runs. The MCMC analysis was run for 200 million generations, with sampling every 20,000 generations. The sampling time of the sequences was used to calibrate the molecular clock during each run. Posterior distribution of the model parameters was estimated by sampling from the three independent Markov chains. Convergence of the chains was assessed using effective sample size values that were higher than 200 with Tracer 1.7 (Rambaut et al., 2018) and 10% of the sampling was discarded as burn-in.
Discrete phylogeographic analysis
To infer the spatial–temporal spread of AMV across the world, we used a Bayesian stochastic search variable selection (BSSVS) model (Lemey et al., 2009) as implemented in BEAST to determine the asymmetric diffusion rates among localities designated as discrete geographical locations, which included Asia, Europe, Middle East, North America, and Oceania. The AMV population of South America was excluded from the BSSVS analysis due to an inadequate sample size (n = 2). The diffusion rates used to estimate rates of viral migration across the five geographic regions were calculated from the resulting log files using SpreaD3 (Bielejec et al., 2016). Significant migration pathways were determined based on the criteria of a BF > 3 (Kass and Raftery, 1995) and mean indicator >0.5 with the following categories: decisively supported diffusion, BF > 1,000; very strongly supported diffusion, 150 < BF < 1,000; strongly supported diffusion, 20 ≤ BF < 150; and supported diffusion, 3 ≤ BF < 20. The expected number of transitions at location state during ancestral history, relative to the data observed at tree distinct tips, was also calculated using Markov jump counts (Minin and Suchard, 2008).
To further analyze the inferred load and direction of migration through time, the maximum clade credibility tree from the MCMC analyses was read using Python scripts by Brynildsrud et al. (2018; https://github.com/admiralenola/globall4scripts). Here, migration events were presumed to occur at the nodes. This presumption may have resulted in a slight bias for inflated ages of AMV migration events.
Demographic dynamics of alfalfa mosaic virus population
To reconstruct the demographic changes over time of AMV, a coalescent Bayesian skyline plot (BSP) was analyzed for the AMV populations of the five geographic regions, as well the combined population. For the separated populations, BSP analyses were implemented in BEAST 1.10.4 using an uncorrelated lognormal relaxed clock model with a uniform distribution for the prior of substitution rate of the CP gene, based on the previous estimate from the Bayesian phylogenetic analysis as described above, running 100 million steps sampled every 10,000 steps, with the first 10% discarded as burn-in.
Phylogeny-trait association analysis
To identify the potential effects of geographical origin and host species on AMV evolution, we calculated three summary statistics (association index AI, parsimony score PS, and maximum monophyletic clade size MC) from the posterior tree samples using BaTS 2.0 (Parker et al., 2008). The AI and PS assess the association between traits (geographic origin and host species) and tree topology. The MC index assesses the association of traits to phylogeny. For BaTS analyses, we used phylogenetic uncertainty to evaluate phylogeny-trait correlations, using 1,000 random permutations of tip locations to calculate the null distribution for the AI, PS, and MC statistics.
Episodic adaptive evolutionary selection
To examine specific sites for episodic adaptive evolutionary selection in the AMV, we employed an algorithm known as mixed effects model of evolution (MEME; Murrell et al., 2012) implemented in the Datamonkey server (Delport et al., 2010). In this analysis, two ω rate classes (a single dS value α and two separate dN values β− and β+) were calculated using MEME per site with corresponding weights (the probability that the site evolved under each rate class at a specified branch). In the null model, β− and β+ were constrained to be less than or equal to α, whereas in the alternative model β+ was not constrained. The fit of the models was compared using a likelihood ratio test (LRT). Episodic positive selection was inferred for the site when β+ > α at a site, and the alternative model provided a better fit than the null model.
Results
Temporal signal of alfalfa mosaic virus
For tip-dated analyses, data sets with a temporal structure are informative (Rieux and Balloux, 2016). Using TempEst, we found a weak correlation between tip dates and genetic distances (r2 = 7.07 × 10−3), suggesting the presence of various clock rates among lineages in our data set, and that a relaxed molecular clock might be most appropriate. Further BETS analysis results showed that the Mhet yielded a higher log marginal likelihood (−5777.49) than the Miso (−5782.85), implying that the Mhet provided the best fit to our data set, and confirmed the data was considered to have an excellent temporal signal for subsequent dating analysis.
Temporal dynamics of alfalfa mosaic virus
Using path and stepping-stone sampling, we confirmed a strong preference for a Bayesian skyline coalescent tree prior and uncorrelated lognormal relaxed clock model for the sequence data analysis (Table 1). Estimation using Bayesian phylogenetic analysis revealed that the mean substitution rate of the CP gene of AMV was 4.14 × 10−4 (95% credibility interval: 1.04 × 10−4 − 6.68 × 10−4) subs/site/year. The time scaled MCC tree revealed that AMV isolates comprised two lineages (Figure 2). Lineage 1 included viral isolates exhibiting considerable diversity among sampling regions, while lineage 2 contained 15 isolates, mainly from Europe. The results of our Bayesian phylogenetic analysis indicated that the crown group dates back to the 1840 CE (Common Era; 95% credibility interval, 1,687–1955) and the most recent common ancestors of AMV isolates in lineage 1 and lineage 2 were placed in 1880 (95% credibility interval, 1774–1956) and 1907 (95% credibility interval, 1806–1976), respectively. However, our Bayesian analysis did not provide support for a specific geographic location as a root node, with the Middle East (posterior probability (pp) = 0.32), Europe (pp = 0.28), and Asia (pp = 0.23) having similar posterior probabilities (Figure 2).
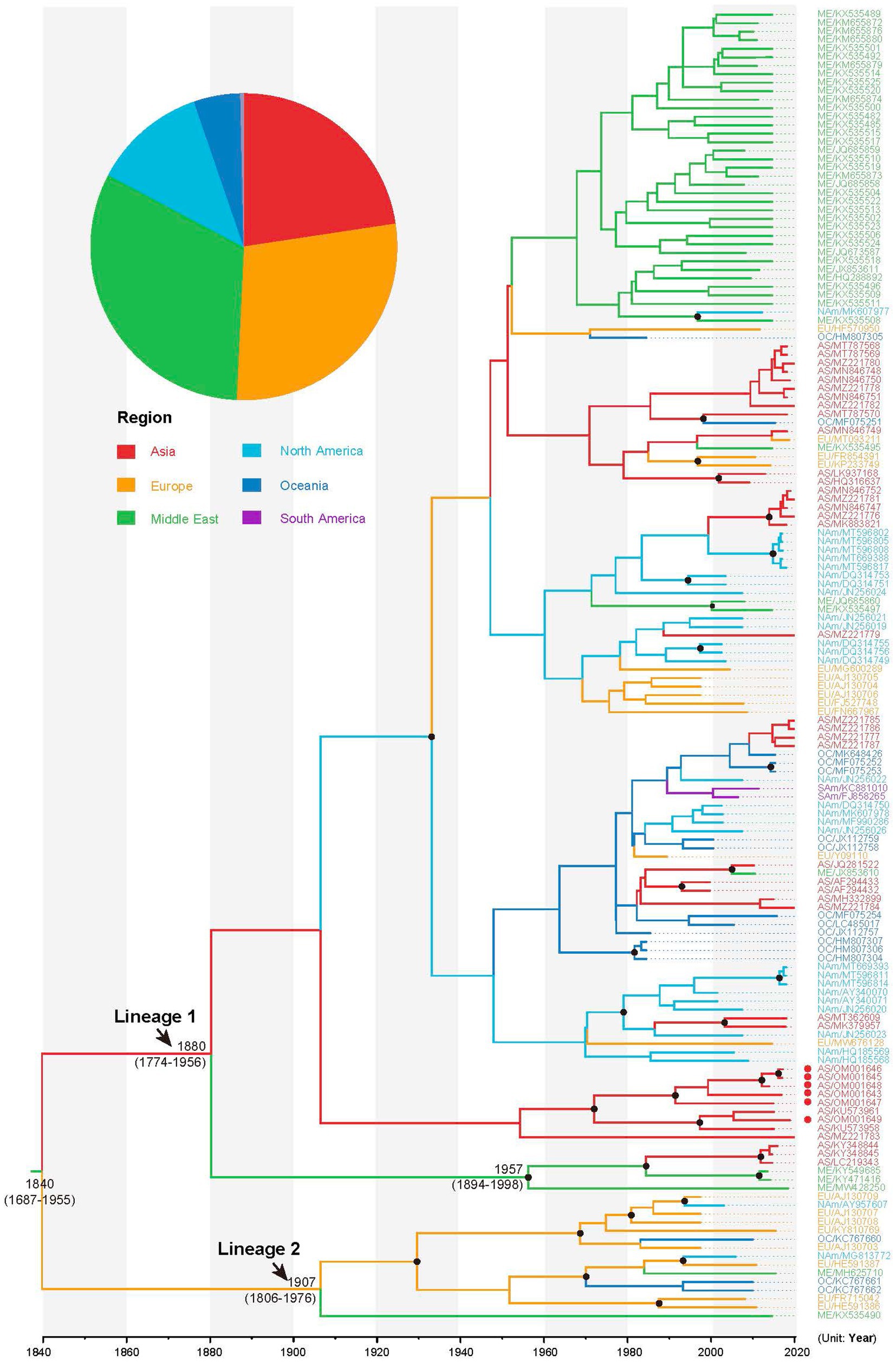
Figure 2. Maximum clade credibility tree inferred from the CP sequences of alfalfa mosaic virus (AMV). The tree topology has been chosen to maximize the product of node posterior probabilities and the tree branches have been color-coded according to their geographic origins. The inferred probability of the root for each geographic region is shown in the pie charts. Black circles indicate strong node support with posterior probability >0.95. AMV isolates sequenced in this study are indicated in red circles.
Worldwide migration of alfalfa mosaic virus
Bayesian phylogeographic analysis suggested that nine migration links contributed to the diffusion of AMV throughout the world (Figure 3A). All the routes originating in Europe toward other regions showed significant support based on high BF values, implying that Europe plays a key role in AMV seeding across the world. Virus migration rates that we inferred were highest between Europe and North America with a mean rate of 1.44 (that is, migration events per lineage per year), followed by Oceania to Asia (a mean rate of 1.42). We observed the lowest mean migration rates from the Middle East to North America (Figure 3B), with a mean rate of 0.49. In addition, the total mean rate per Markov jumps for all regions supported the role of Europe as a seeding population. In contrast, it was shown that the in-migration of AMV was the greatest in Asia (Figure 3C).
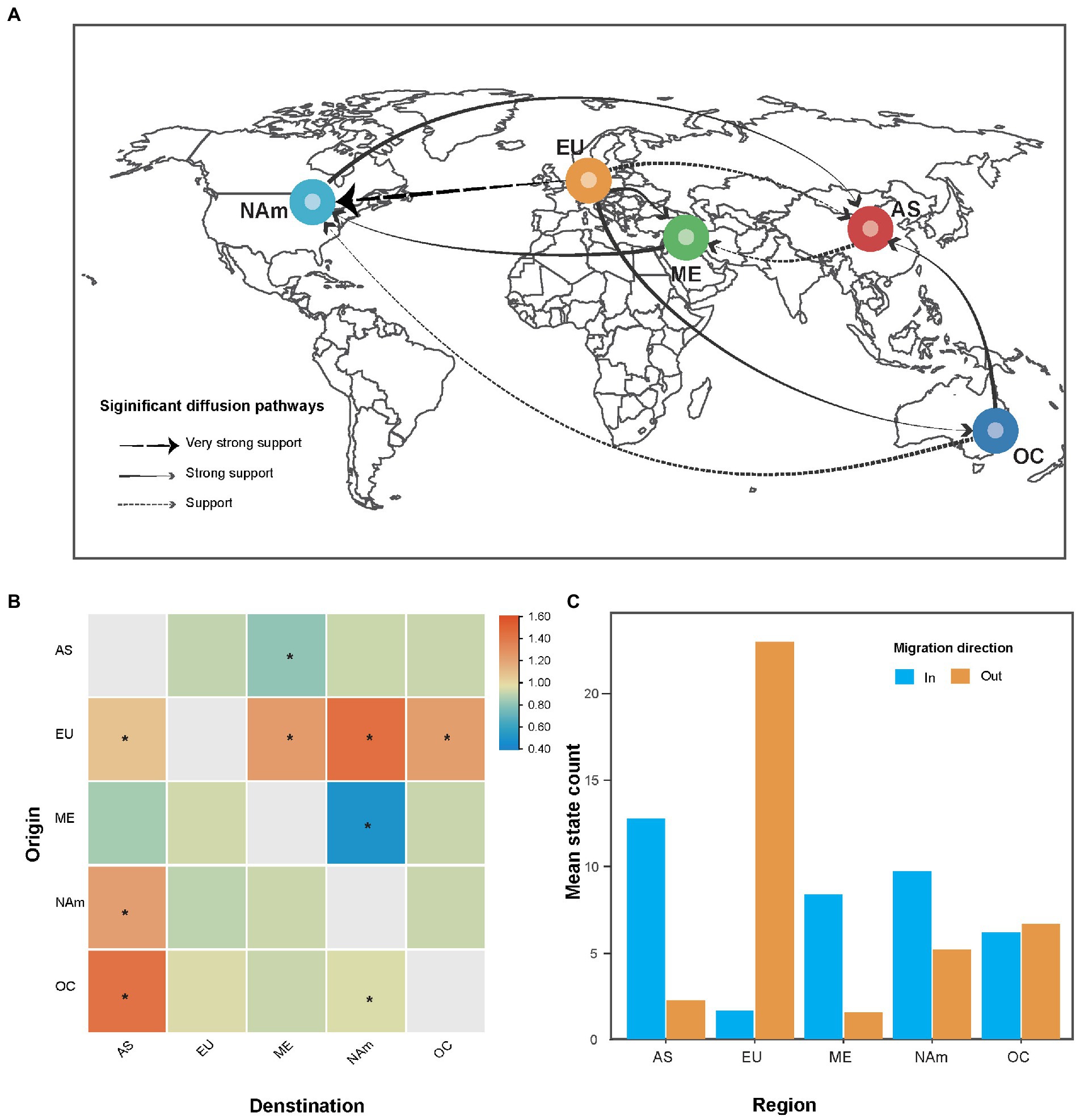
Figure 3. The spatial dynamics analysis of alfalfa mosaic virus (AMV). (A) Spatial diffusion pathway, (B) asymmetric migration rate matrix of AMV between each pair of regions, and (C) histograms of the total number of location-state transitions. Bayes factor (BF) > 3 and mean indicator of >0.5. Dashed black arrows, very strong support with 150 < BF < 1,000; solid grey arrows, strong support with 20 < BF < 150; and dashed grey arrows with BF < 20. The asterisks at the center of the cell indicate supported rates with 3 < BF < 20. AS, Asia; EU, Europe; ME, Middle East; NAm, North America; OC, Oceania.
Spatial dynamics of alfalfa mosaic virus over time
AMV migration load and direction across time are summarized in Figure 4. Although a small wave of AMV migration from Europe to Asia had started before the early 1900s, the migrations from Europe to the Middle East, North America, and Oceania were not started until the early 1960s. Comparatively, a strong increase in prevalence of internal migration had been observed, particularly in Asia and the Middle East (Figures 4A,C) since the early 1940s. Despite the internal migration within Europe experiencing an increase before the 1980s, there was a sudden decline from the 1980s to the last sampling year (Figure 4B).
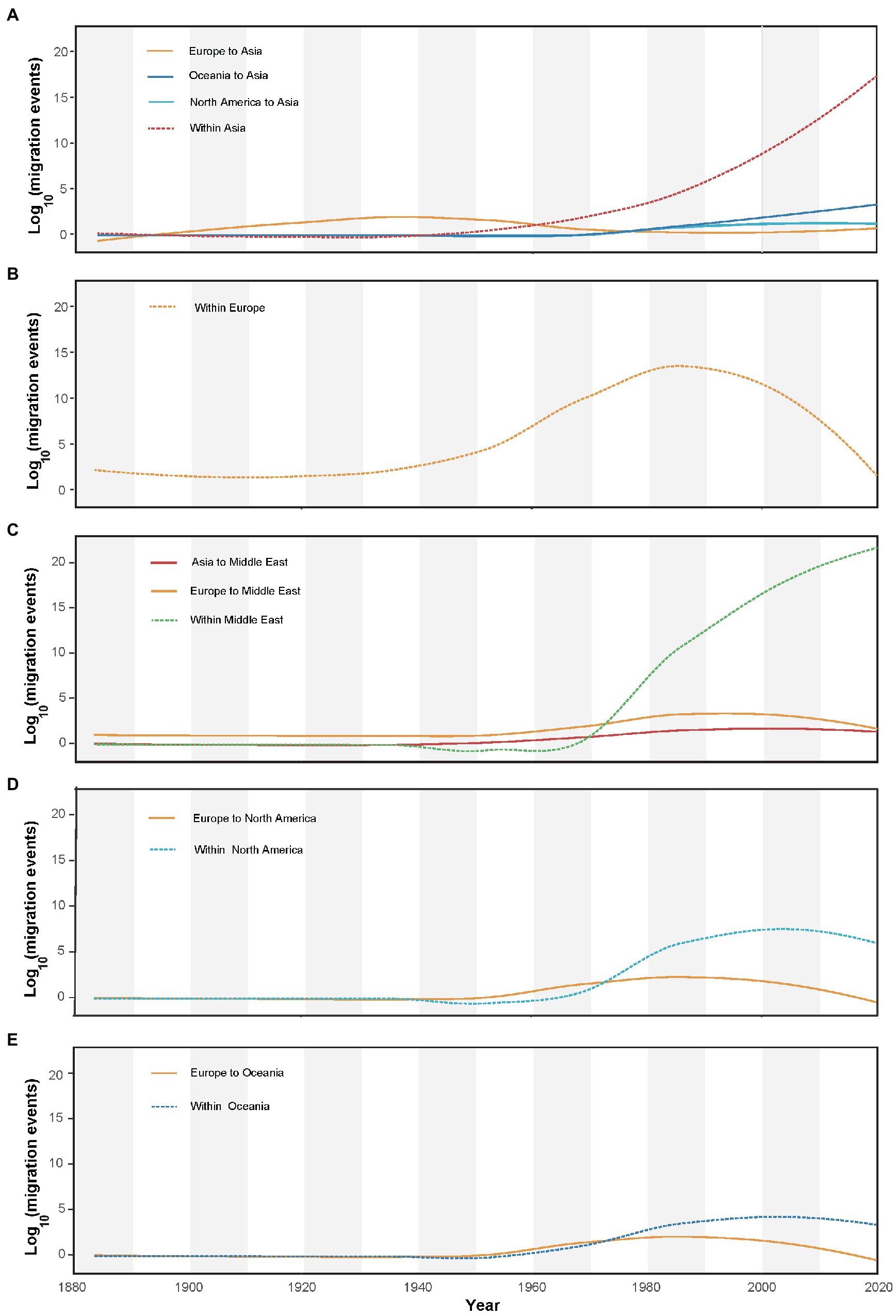
Figure 4. Inferred migration events (on log10 scale) of alfalfa mosaic virus through time (year). The plots also show within-region migration over time for (A) Asia, (B) Europe, (C) Middle East, (D) North America, and (E) Oceania.
Population dynamics of alfalfa mosaic virus
Reconstruction of the demographic history by a Bayesian skyline plot indicated that the size of the AMV population changed with time (Figure 5). The global AMV population underwent a slight expansion between 1960 and 1990 and subsequently remained relatively constant, followed by a recent decline. AMV isolates originating from Europe and the Middle East underwent population expansion before a period of stability, while those from North America showed steady population size with a slight increase until the latest sampling year. In contrast, AMV from Asia and Oceania have maintained constant population sizes throughout the study time period.
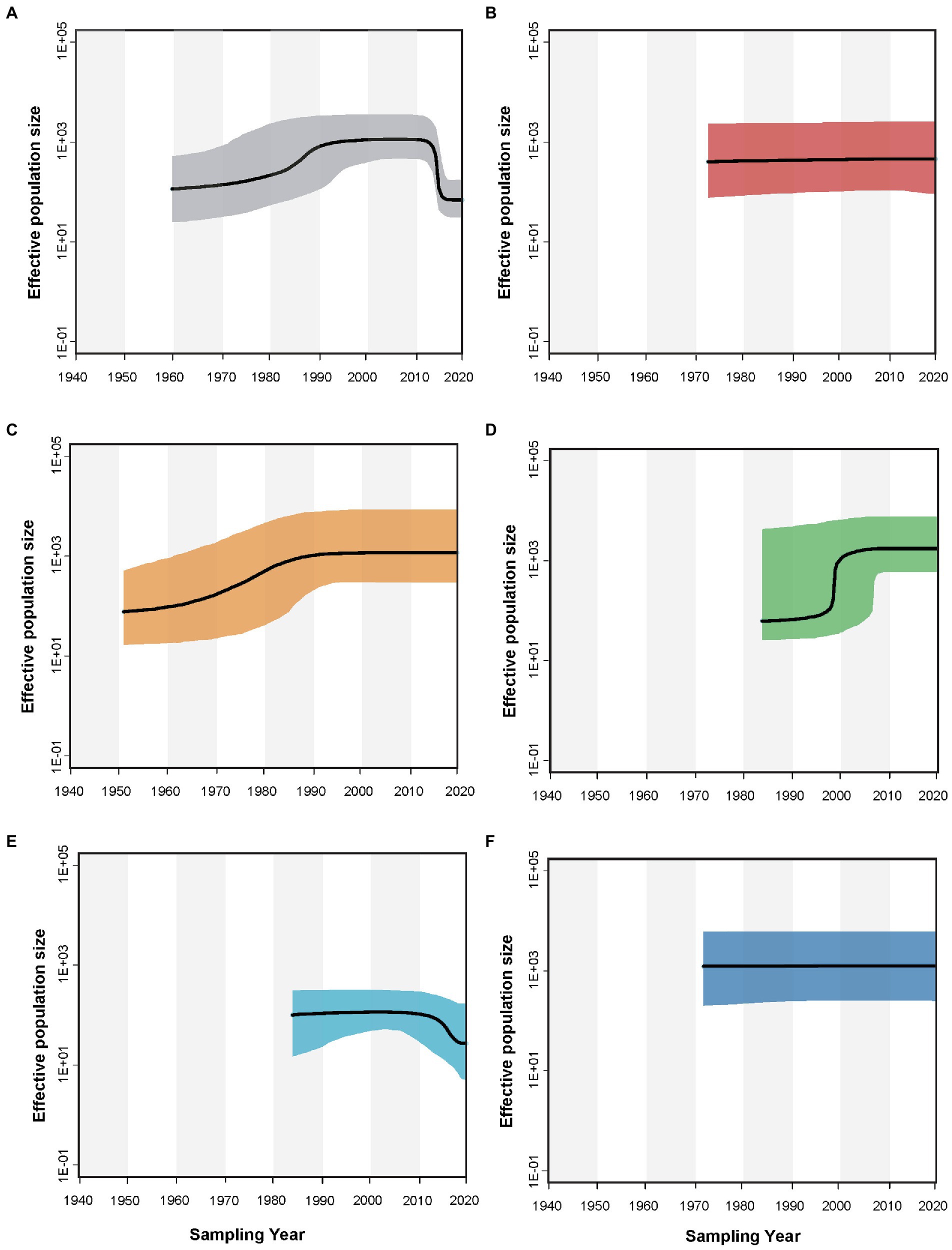
Figure 5. Bayesian skyline plot that describes the demographic history of alfalfa mosaic virus for (A) the global, (B) Asian, (C) European, (D) Middle Eastern, (E) North American, and (F) Oceanian AMV populations. The y-axis represents the population size (net) whereas the x-axis is given in years. The black lines show the median estimate of the population size, and the shaded areas show the 95% credibility interval.
Adaptative evolution of alfalfa mosaic virus
When geographic regions were used as grouping factors, phylogeny-trait association analysis revealed that significant signals were found for the association between sampling regions and phylogenetic relationships (Table 2).
However, with the exception of viral isolates from the host species of the Actinidiaceae, Solanaceae, and Umbelliferae families (pMC < 0.05), no signal was observed between host species and phylogenetic relationships when the AMV isolates were clustered based on their host origins (Table 2). The BaTS results indicated an extensive geographical spatial variability of the pathogen, implying that geography-driven adaptation could be an important determinant of a factor during the evolution of AMV. Further, the results from the analysis of episodic adaptive evolutionary selection indicated that 13 out of 331 (3.92%, Table 3) of codon sites were found under positive selection (p ≤ 0.05).
Discussion
We here investigated the molecular epidemiology of AMV based on the CP gene sequences of this virus and unveiled the comprehensive evolutionary history of the virus with a sampling window across 35 years.
AMV was first discovered in alfalfa (Medicago sativa) in America and was not officially named until 1931 (Weimer, 1931). Our phylogenetic analysis revealed that the most recent AMV common ancestor existed in 1840 (95% credibility interval 1687–1955; Figure 2), much earlier than that of the documented emergence of AMV. This can be explained by the fact that this disease has gone unnoticed for a long period.
Our phylogenetic analysis was unable to place the root of the tree in any particular geographic location because the Middle East, Europe, and Asia had similar posterior probabilities (Figure 2). One explanation is that the earlier AMV isolates were not sampled. In this study, the oldest AMV isolate was collected in 1985 (Supplementary Table S1). The other explanation is the multiple introductions of AMV due to pandemics. It is documented that there are two different origins of alfalfa, one the Mediterranean basin and the other Asia minor (Iran or Afghanistan; Hanson, 1972). Our phylogeographic analysis identified four migration pathways between Europe and other regions in the diffusion processes of AMV, indicating Europe might have played a key role in seeding the AMV epidemics. This finding is concordant with the global alfalfa trade during the past few decades, implying that AMV migration is associated with human-mediated activities. After North America, European countries have the second largest production of alfalfa in the world, with a cultivation area of nearly 2.5 million ha (Annicchiarico et al., 2015).
It is reported that geographic factors and host species play contributory roles in the evolution of many RNA viruses (Cuevas et al., 2012; Yu et al., 2021). Here, we hypothesized that deterministic events contributed to the current spatial population genetic structure, which is supported by the results of phylogeny-trait association analysis (Table 2). However, the results from the phylogeny-trait association analysis did not provide evidence for host-specific grouping of AMV isolates, except for the plant families Umbelliferae, Actinidiaceae, and Solanaceae, and we propose that to a certain degree, host-driven adaptation resulted in AMV diversification.
Drastic changes in population size coupled with demographic events may influence the generation, distribution, and maintenance of genetic variation. These effects not only act directly through genetic drift and mutation, but also act indirectly through impacts on migration and recombination as well as on the efficiency of natural selection to eliminate or amplify mutations (Wang and Whitlock, 2003). Our demographic analyses showed that the global population of AMV has remained small but has undergone recent expansion that may be associated with global alfalfa overproduction. Historical records indicate an oversupply of alfalfa on the market, reaching a peak of more than 33 million ha in 1990.3 In 2014, however, the cultivation area of alfalfa declined to 24 million ha (Xie et al., 2021). This is also concordant with our estimate of changes in AMV global population size (Figure 5), which suggests a correlation between the alfalfa cultivation area and the population size. It will be interesting to understand how human activities affect the demographic expansion of the AMV population.
It is notable that there are several limitations to the current study. For instance, AMV is a multipartite virus with a wide host range. There is evidence that the evolutionary stable equilibrium for the three genomic RNAs of AMV is host-species dependent (Wu et al., 2017). This suggests that a multi-gene data set is needed for a full appreciation of the evolution of AMV. Nevertheless, this study represents the first attempt to understand the global phylogeography of AMV, one of the most common viral pathogens of forage crops in the world.
Conclusion
This study provides new insights into the evolutionary history of AMV based on Bayesian phylodynamic analysis of the CP gene data. We have identified multiple AMV migration pathways originating from Europe to other regions, suggesting that Europe is the major seeding region for the global spread of this pathogen. The dispersal patterns are likely to correlate with human activities. In addition, we found that geographically-driven adaptation may be an important determinant of the evolution of AMV. These results increase our knowledge about the evolution of AMV and may have potential implications for developing sustainable management strategies to control this pathogen.
Data availability statement
The datasets presented in this study can be found in online repositories. The names of the repository/repositories and accession number(s) can be found at: https://www.ncbi.nlm.nih.gov/genbank/, OM001643, https://www.ncbi.nlm.nih.gov/genbank/, OM001644, https://www.ncbi.nlm.nih.gov/genbank/, OM001645, https://www.ncbi.nlm.nih.gov/genbank/, OM001646, https://www.ncbi.nlm.nih.gov/genbank/, OM001647, https://www.ncbi.nlm.nih.gov/genbank/, OM001648, https://www.ncbi.nlm.nih.gov/genbank/, OM001649, https://zenodo.org/record/7255913#.Y1np9OzP2ZY, 10.5281/zenodo.7255913.
Author contributions
YB conceived the study. YG, GF, SC, and WZ performed the experiments. YG and YB analyzed the data and interpreted the results. YG and YB led the writing of the manuscript. All authors contributed to the article and approved the submitted version.
Funding
This work was supported by grants from the China Agriculture Research System of MOF and MARA.
Acknowledgments
We thank Fangluan Gao at the Fujian Agriculture and Forestry University (FAFU) for his generous help in analyzing the data.
Conflict of interest
The authors declare that the research was conducted in the absence of any commercial or financial relationships that could be construed as a potential conflict of interest.
Publisher’s note
All claims expressed in this article are solely those of the authors and do not necessarily represent those of their affiliated organizations, or those of the publisher, the editors and the reviewers. Any product that may be evaluated in this article, or claim that may be made by its manufacturer, is not guaranteed or endorsed by the publisher.
Supplementary material
The Supplementary material for this article can be found online at: https://www.frontiersin.org/articles/10.3389/fmicb.2022.1051834/full#supplementary-material
Footnotes
References
Abdalla, O. A., Al-Shahwan, I. M., Al-Saleh, M. A., and Amer, M. A. (2020). Molecular characterization of alfalfa mosaic virus (AMV) isolates in alfalfa and other plant species in different regions in Saudi Arabia. Eur. J. Plant Pathol. 156, 603–613. doi: 10.1007/s10658-019-01910-z
Annicchiarico, P., Barrett, B., Brummer, E. C., Julier, B., and Marshall, A. H. (2015). Achievements and challenges in improving temperate perennial forage legumes. Crit. Rev. Plant Sci. 34, 327–380. doi: 10.1080/07352689.2014.898462
Baele, G., Lemey, P., Bedford, T., Rambaut, A., Suchard, M. A., and Alekseyenko, A. V. (2012). Improving the accuracy of demographic and molecular clock model comparison while accommodating phylogenetic uncertainty. Mol. Biol. Evol. 29, 2157–2167. doi: 10.1093/molbev/mss084
Baele, G., Lemey, P., and Suchard, M. A. (2016). Genealogical working distributions for Bayesian model testing with phylogenetic uncertainty. Syst. Biol. 65, 250–264. doi: 10.1093/sysbio/syv083
Bailiss, K. W., and Ollennu, L. A. A. (1986). Effect of alfalfa mosaic virus isolates on forage yield of lucerne (Medicago sativa) in Britain. Plant Pathol. 35, 162–168. doi: 10.1111/j.1365-3059.1986.tb02000.x
Bergua, M., Luis-Arteaga, M., and Escriu, F. (2014). Genetic diversity, reassortment, and recombination in alfalfa mosaic virus population in Spain. Phytopathology 104, 1241–1250. doi: 10.1094/PHYTO-11-13-0309-R
Bielejec, F., Baele, G., Vrancken, B., Suchard, M. A., Rambaut, A., and Lemey, P. (2016). SpreaD3: interactive visualization of spatiotemporal history and trait evolutionary processes. Mol. Biol. Evol. 33, 2167–2169. doi: 10.1093/molbev/msw082
Bol, J. F. (2003). Alfalfa mosaic virus: coat protein-dependent initiation of infection. Mol. Plant Pathol. 4, 1–8. doi: 10.1046/j.1364-3703.2003.00146.x
Bol, J. F., Vloten-Doting, L. V., and Jaspars, E. M. J. (1971). A functional equivalence of top component a RNA and coat protein in the initiation of infection by alfalfa mosaic virus. Virology 46, 73–85. doi: 10.1016/0042-6822(71)90007-9
Brynildsrud, O. B., Pepperell, C. S., Suffys, P., Grandjean, L., Monteserin, J., Debech, N., et al. (2018). Global expansion of mycobacterium tuberculosis lineage 4 shaped by colonial migration and local adaptation. Sci. Adv. 4:eaat5869. doi: 10.1126/sciadv.aat5869
Codoñer, F. M., Cuevas, J. M., Sánchez-Navarro, J. A., Pallás, V., and Elena, S. F. (2005). Molecular evolution of the plant virus family Bromoviridae based on RNA3-encoded proteins. J. Mol. Evol. 61, 697–705. doi: 10.1007/s00239-005-0021-7
Cuevas, J. M., Delaunay, A., Rupar, M., Jacquot, E., and Elena, S. F. (2012). Molecular evolution and phylogeography of potato virus Y based on the CP gene. J. Gen. Virol. 93, 2496–2501. doi: 10.1099/vir.0.044347-0
Delport, W., Poon, A. F. Y., Frost, S. D. W., and Kosakovsky Pond, S. L. (2010). Datamonkey 2010: a suite of phylogenetic analysis tools for evolutionary biology. Bioinformatics 26, 2455–2457. doi: 10.1093/bioinformatics/btq429
Duan, G., Zhan, F., Du, Z., Ho, S. Y. W., and Gao, F. (2018). Europe was a hub for the global spread of potato virus S in the 19th century. Virology 525, 200–204. doi: 10.1016/j.virol.2018.09.022
Duchene, S., Lemey, P., Stadler, T., Ho, S. Y. W., Duchene, D. A., Dhanasekaran, V., et al. (2020). Bayesian evaluation of temporal signal in measurably evolving populations. Mol. Biol. Evol. 37, 3363–3379. doi: 10.1093/molbev/msaa163
Edgar, R. C. (2004). MUSCLE: multiple sequence alignment with high accuracy and high throughput. Nucleic Acids Res. 32, 1792–1797. doi: 10.1093/nar/gkh340
Fidan, H., Adak, N. A., Konuksal, A., Akerzurumlu, E., and Yılmaz, M. A. (2012). Occurrence of alfalfa mosaic virus (AMV) diseases on potato crops in northern Cyprus. Acta Hortic. 960, 341–346. doi: 10.17660/ActaHortic.2012.960.49
Freeman, A. J., and Aftab, M. (2011). Effective management of viruses in pulse crops in south eastern Australia should include management of weeds. Australas. Plant Pathol. 40, 430–441. doi: 10.1007/s13313-011-0058-6
Gao, F., Kawakubo, S., Ho, S. Y. W., and Ohshima, K. (2020). The evolutionary history and global spatio-temporal dynamics of potato virus Y. Virus Evol. 6:veaa056. doi: 10.1093/ve/veaa056
Gao, F., Liu, X., Du, Z., Hou, H., Wang, X., Wang, F., et al. (2019). Bayesian phylodynamic analysis reveals the dispersal patterns of tobacco mosaic virus in China. Virology 528, 110–117. doi: 10.1016/j.virol.2018.12.001
Guan, X., Yang, C., Fu, J., Du, Z., Ho, S. Y. W., and Gao, F. (2018). Rapid evolutionary dynamics of pepper mild mottle virus. Virus Res. 256, 96–99. doi: 10.1016/j.virusres.2018.08.006
Guo, Z., Zhang, T., Chen, Z., Niu, J., Cui, X., Mao, Y., et al. (2022). Occurrence, distribution, and genetic diversity of alfalfa (Medicago sativa L.) viruses in four major alfalfa-producing provinces of China. Front. Microbiol. 12:771361. doi: 10.3389/fmicb.2021.771361
Hanson, H. (1972). Alfalfa science and technology. Madison: American Society of Agronomy Inc, Publisher
He, B., Fajolu, O. L., Wen, R. H., and Hajimorad, M. R. (2010). Seed transmissibility of alfalfa mosaic virus in soybean. Plant Health Prog. 11:41. doi: 10.1094/PHP-2010-1227-01-BR
Herranz, M. C., Pallas, V., and Aparicio, F. (2012). Multifunctional roles for the N-terminal basic motif of alfalfa mosaic virus coat protein: nucleolar/cytoplasmic shuttling, modulation of RNA-binding activity, and virion formation. Mol. Plant-Microbe Interact. 25, 1093–1103. doi: 10.1094/MPMI-04-12-0079-R
Hull, R. (1969). Alfalfa mosaic virus. Adv. Virus Res. 15, 365–433. doi: 10.1016/S0065-3527(08)60880-5
Huson, D. H. (1998). SplitsTree: analyzing and visualizing evolutionary data. Bioinformatics 14, 68–73. doi: 10.1093/bioinformatics/14.1.68
Jones, R. A. C., and Coutts, B. A. (1996). Alfalfa mosaic and cucumber mosaic virus infection in chickpea and lentil: incidence and seed transmission. Ann. Appl. Biol. 129, 491–506. doi: 10.1111/j.1744-7348.1996.tb05771.x
Jones, R. A. C., and Pathipanawat, W. (1989). Seed-borne alfalfa mosaic virus infecting annual medics (Medicago spp.) in Western Australia. Ann. Appl. Biol. 115, 263–277. doi: 10.1111/j.1744-7348.1989.tb03385.x
Jones, R. A. C., Real, D., Vincent, S. J., Gajda, B. E., and Coutts, B. A. (2012). First report of alfalfa mosaic virus infecting tedera (Bituminaria bituminosa (L.) C.H. stirton var. albomarginata and crassiuscula) in Australia. Plant Dis. 96:1384. doi: 10.1094/PDIS-04-12-0378-PDN
Kalyaanamoorthy, S., Minh, B. Q., Wong, T. K. F., von Haeseler, A., and Jermiin, L. S. (2017). ModelFinder: fast model selection for accurate phylogenetic estimates. Nat. Methods 14, 587–589. doi: 10.1038/nmeth.4285
Kass, R. E., and Raftery, A. E. (1995). Bayes factors. J. Am. Stat. Assoc. 90, 773–795. doi: 10.1080/01621459.1995.10476572
Kawakubo, S., Gao, F., Li, S., Tan, Z., Huang, Y.-K., Adkar-Purushothama, C. R., et al. (2021). Genomic analysis of the brassica pathogen turnip mosaic potyvirus reveals its spread along the former trade routes of the silk road. Proc. Natl. Acad. Sci. U. S. A. 118:e2021221118. doi: 10.1073/pnas.2021221118
Komorowska, B., Hasiów-Jaroszewska, B., and Budzyńska, D. (2021). Genetic variability and molecular evolution of arabis mosaic virus based on the coat protein gene sequence. Plant Pathol. 70, 2197–2206. doi: 10.1111/ppa.13447
Kumar, S., Stecher, G., Li, M., Knyaz, C., and Tamura, K. (2018). MEGA X: molecular evolutionary genetics analysis across computing platforms. Mol. Biol. Evol. 35, 1547–1549. doi: 10.1093/molbev/msy096
Lam, T. T.-Y., Hon, C.-C., Pybus, O. G., Kosakovsky Pond, S. L., Wong, R. T.-Y., Yip, C.-W., et al. (2008). Evolutionary and transmission dynamics of reassortant H5N1 influenza virus in Indonesia. PLoS Pathog. 4:e1000130. doi: 10.1371/journal.ppat.1000130
Lefkowitz, E. J., Dempsey, D. M., Hendrickson, R. C., Orton, R. J., Siddell, S. G., and Smith, D. B. (2018). Virus taxonomy: the database of the international committee on taxonomy of viruses (ICTV). Nucleic Acids Res. 46, D708–D717. doi: 10.1093/nar/gkx932
Lemey, P., Hong, S. L., Hill, V., Baele, G., Poletto, C., Colizza, V., et al. (2020). Accommodating individual travel history and unsampled diversity in Bayesian phylogeographic inference of SARS-CoV-2. Nat. Commun. 11:5110. doi: 10.1038/s41467-020-18877-9
Lemey, P., Rambaut, A., Drummond, A. J., and Suchard, M. A. (2009). Bayesian phylogeography finds its roots. PLoS Comput. Biol. 5:e1000520. doi: 10.1371/journal.pcbi.1000520
Leur, J., Duric, Z., George, J., and Boschma, S. (2019). Alfalfa mosaic virus infects the tropical legume Desmanthus virgatus in Australia and the potential role of the cowpea aphid (Aphis craccivora) as the virus vector. Aust Plant Dis Notes 14, 1–4. doi: 10.1007/s13314-019-0335-8
Martin, D. P., Murrell, B., Golden, M., Khoosal, A., and Muhire, B. (2015). RDP4: detection and analysis of recombination patterns in virus genomes. Virus Evol. 1:vev003. doi: 10.1093/ve/vev003
Minin, V. N., and Suchard, M. A. (2008). Counting labeled transitions in continuous-time Markov models of evolution. J. Math. Biol. 56, 391–412. doi: 10.1007/s00285-007-0120-8
Murrell, B., Wertheim, J. O., Moola, S., Weighill, T., Scheffler, K., and Kosakovsky Pond, S. L. (2012). Detecting individual sites subject to episodic diversifying selection. PLoS Genet. 8:e1002764. doi: 10.1371/journal.pgen.1002764
Nguyen, L.-T., Schmidt, H. A., von Haeseler, A., and Minh, B. Q. (2015). IQ-TREE: a fast and effective stochastic algorithm for estimating maximum likelihood phylogenies. Mol. Biol. Evol. 32, 268–274. doi: 10.1093/molbev/msu300
Olsthoorn, R. C. L., Haasnoot, P. C., and Bol, J. F. (2004). Similarities and differences between the subgenomic and minus-strand promoters of an RNA plant virus. J. Virol. 78, 4048–4053. doi: 10.1128/JVI.78.8.4048-4053.2004
Ormeño, J., Sepúlveda, P., Rojas, R., and Araya, J. E. (2006). Datura genus weeds as an epidemiologcal factor of alfalfa mosaic virus (AMV), cucumber mosaic virus (CMV), and potato virus Y (PVY) on solanaceus crops Chilean. J. Agric. Res. 66, 333–341. doi: 10.4067/S0365-28072006000400001
Pan, X., Gao, Y., Liu, B., and Ren, Y. (2017). Current situation and prospect of alfalfa industry. J. Green Sci. Technol. 4, 104–107. doi: 10.16663/j.cnki.lskj.2017.13.044
Parker, J., Rambaut, A., and Pybus, O. G. (2008). Correlating viral phenotypes with phylogeny: accounting for phylogenetic uncertainty. Infect. Genet. Evol. 8, 239–246. doi: 10.1016/j.meegid.2007.08.001
Parrella, G., Lanave, C., Marchoux, G., Finetti Sialer, M. M., Di Franco, A., and Gallitelli, D. (2000). Evidence for two distinct subgroups of alfalfa mosaic virus (AMV) from France and Italy and their relationships with other AMV strains brief report. Arch. Virol. 145, 2659–2667. doi: 10.1007/s007050070014
Rambaut, A., Drummond, A. J., Xie, D., Baele, G., and Suchard, M. A. (2018). Posterior summarisation in Bayesian phylogenetics using tracer 1.7. Syst. Biol. 67, 901–904. doi: 10.1093/sysbio/syy032
Rambaut, A., Lam, T. T., Max Carvalho, L., and Pybus, O. G. (2016). Exploring the temporal structure of heterochronous sequences using TempEst (formerly path-O-gen). Virus Evol. 2:vew007. doi: 10.1093/ve/vew007
Rieux, A., and Balloux, F. (2016). Inferences from tip-calibrated phylogenies: a review and a practical guide. Mol. Ecol. 25, 1911–1924. doi: 10.1111/mec.13586
Ronquist, F., Teslenko, M., van der Mark, P., Ayres, D. L., Darling, A., Hohna, S., et al. (2012). MrBayes 3.2: efficient bayesian phylogenetic inference and model choice across a large model space. Syst. Biol. 61, 539–542. doi: 10.1093/sysbio/sys029
Strimmer, K., and von Haeseler, A. (1997). Likelihood-mapping: a simple method to visualize phylogenetic content of a sequence alignment. Proc. Natl. Acad. Sci. U. S. A. 94, 6815–6819. doi: 10.1073/pnas.94.13.6815
Suchard, M. A., Lemey, P., Baele, G., Ayres, D. L., Drummond, A. J., and Rambaut, A. (2018). Bayesian phylogenetic and phylodynamic data integration using BEAST 1.10. Virus Evol. 4:vey016. doi: 10.1093/ve/vey016
Trucco, V., Castellanos Collazo, O., Vaghi Medina, C. G., Cabrera Mederos, D., Lenardon, S., and Giolitti, F. (2022). Alfalfa mosaic virus (AMV): genetic diversity and a new natural host. J. Plant Pathol. 104, 349–356. doi: 10.1007/s42161-021-00961-8
Valkonen, J. P. T., Pehu, E., and Watanabe, K. (1992). Symptom expression and seed transmission of Afalfa mosaic virus and potato yellowing virus (SB22) in Solanum brevidens and S. etuberosum. Potato Res. 35, 403–410. doi: 10.1007/BF02357596
Wang, J., and Whitlock, M. C. (2003). Estimating effective population size and migration rates from genetic samples over space and time. Genetics 163, 429–446. doi: 10.1093/genetics/163.1.429
Wei, K., and Li, Y. (2017). Global evolutionary history and spatio-temporal dynamics of dengue virus type 2. Sci. Rep. 7:45505. doi: 10.1038/srep45505
Wu, B., Zwart, M. P., Sánchez-Navarro, J. A., and Elena, S. F. (2017). Within-host evolution of segments ratio for the tripartite genome of alfalfa mosaic virus. Sci. Rep. 7:5004. doi: 10.1038/s41598-017-05335-8
Xie, H., Yang, Y., Dong, Y., and Wang, T. (2021). Analysis on international development trends of alfalfa. Chin. Bull. Bot. 56, 740–750. doi: 10.11983/CBB21121
Xu, Y., Zhang, S., Shen, J., Wu, Z., Du, Z., and Gao, F. (2021). The phylogeographic history of tomato mosaic virus in Eurasia. Virology 554, 42–47. doi: 10.1016/j.virol.2020.12.009
Yu, C., Lian, Q., Lin, H., Chen, L., Lu, Y., Zhai, Y., et al. (2021). A clade of telosma mosaic virus from Thailand is undergoing geographical expansion and genetic differentiation in passionfruit of Vietnam and China. Phytopathol. Res. 3:24. doi: 10.1186/s42483-021-00101-1
Zhang, D., Gao, F., Jakovlić, I., Zou, H., Zhang, J., Li, W. X., et al. (2020). PhyloSuite: an integrated and scalable desktop platform for streamlined molecular sequence data management and evolutionary phylogenetics studies. Mol. Ecol. Resour. 20, 348–355. doi: 10.1111/1755-0998.13096
Keywords: alfalfa mosaic virus, Bayesian phylodynamics, spatiotemporal transmission, geography-driven adaptation, positive selection, population dynamics
Citation: Gao Y, Fan G, Cheng S, Zhang W and Bai Y (2022) Evolutionary history and global spatiotemporal pattern of alfalfa mosaic virus. Front. Microbiol. 13:1051834. doi: 10.3389/fmicb.2022.1051834
Edited by:
Francesco Dal Grande, University of Padua, ItalyReviewed by:
James M. Wainaina, The Ohio State University, United StatesAlexander N. Ignatov, Peoples’ Friendship University of Russia, Russia
Copyright © 2022 Gao, Fan, Cheng, Zhang and Bai. This is an open-access article distributed under the terms of the Creative Commons Attribution License (CC BY). The use, distribution or reproduction in other forums is permitted, provided the original author(s) and the copyright owner(s) are credited and that the original publication in this journal is cited, in accordance with accepted academic practice. No use, distribution or reproduction is permitted which does not comply with these terms.
*Correspondence: Yanju Bai, YmFpeWFuanVoYXJiaW5AMTI2LmNvbQ==