- 1Center for Evidence-Based and Translational Medicine, Zhongnan Hospital of Wuhan University, Wuhan, China
- 2Department of Stomatology, Zhongnan Hospital of Wuhan University, Wuhan, China
- 3Department of Gastroenterology, Zhongnan Hospital of Wuhan University, Wuhan, China
- 4Department of Stomatology, Kaifeng University Health Science Center, Kaifeng, China
Objectives: Periodontitis affects the progression of many diseases, while its detailed mechanism remains unclear. This study hopes to provide new ideas for exploring its mechanism by analyzing the gut microbiota and fecal metabolic characteristics of experimental periodontitis rats.
Methods: A total of 10 rats were randomly divided into ligature-induced experimental periodontitis (EP) group and healthy control group. After 4 weeks of the experiment, the feces of all rats were collected for sequencing through 16S ribosomal DNA (rDNA) sequencing technology and liquid chromatography–mass spectrometry (LC–MS).
Results: 16S rDNA sequencing results showed that the β-diversity of gut microbiota was significantly different between the EP and control group, and the levels of dominant genera were different. Compared with the control group, Ruminococcus, Escherichia, and Roseburia were significantly enriched in EP, and Coprococcus, Turicibacter, Lachnospira were significantly decreased. Correlation analysis showed that Roseburia exhibited the highest correlation within the genus. Of 3,488 qualitative metabolites, 164 metabolites were upregulated and 362 metabolites were downregulated in EP. Enrichment analysis showed that periodontitis significantly changed 45 positive/negative ion metabolic pathways. Five KEGG pathways, protein digestion and absorption, tyrosine metabolism, glycolysis/gluconeogenesis, niacin and nicotinamide metabolism, and oxidative phosphorylation, are enriched in both the microbiome and metabolome. Correlation analysis showed that the genera with significant differences in periodontitis were usually significantly correlated with more metabolites, such as Roseburia, Lachnospira, Escherichia, Turicibacter, and Ruminococcus. The genera with the same changing trend tended to have a similar correlation with some certain metabolites. In addition, vitamin D2 and protoporphyrin IX have the most significant correlations with microorganisms.
Conclusion: Our study reveals that periodontitis alters gut microbiota and fecal metabolites. The correlation analysis of microbiota and metabolome provides a deeper understanding of periodontitis, and also provides a direction for the study of periodontitis affecting other diseases.
Introduction
Periodontitis is an inflammatory disease caused by pathogenic microorganisms in the oral cavity, and the culprit is the dental plaque that grows on the surface of the teeth (Darveau, 2010). Patients with severe periodontitis may swallow 1012–1013 bacteria in saliva every day (Sender et al., 2016). A growing number of studies have shown that periodontitis is involved in the progression of various diseases, including metabolic syndrome, type 2 diabetes mellitus (T2D), benign prostatic hyperplasia, chronic kidney disease (CKD), coronary heart disease, and cancer (Tonetti and Van Dyke, 2013; Genco and Sanz, 2020; Fang et al., 2021b; Yuan et al., 2021). Studies suggest that its possible mechanisms include distant spread of periodontopathogens, ectopic infection, distal end migration of oral inflammatory–activated Th17 cells, and metabolic and immune disorders (Kitamoto et al., 2020; Fang et al., 2021b; Hajishengallis and Chavakis, 2021), but the specific mechanism is still not clear. Gut microbiota disorder is increasingly considered as the pathogenesis of many diseases. More and more studies have reported that periodontal pathogens can affect gut microbiota dysbiosis through ectopic colonization, pathological factors, or virulence factors (Yamazaki et al., 2020; Hajishengallis and Chavakis, 2021). Gut microbiota disturbance may be one of the mechanisms of periodontitis affecting distal diseases (Huang et al., 2020), but the detailed mechanism remains to be fully elucidated.
Metabolites are usually measured in clinical medicine as biomarkers for diagnosis, prognosis, or therapeutic response (Wishart, 2019), providing a new entry point for exploring the pathogenesis of the disease. Li et al. (2020) suggest that gut microbial alterations caused by periodontitis can lead to systemic inflammation and metabolic dysfunction, which will accelerate the progression of T2DM. Sakanaka et al. (2021) explored the potential link between periodontitis and cardiometabolic diseases by studying metabolites. Previous studies have pointed out that the use of metabolomics to study the relationship between periodontitis and obesity gets significantly more outputs than the pure oral microbiome (Chen et al., 2021). At present, 16S rDNA sequencing technology is mostly used in the analysis of the gut microbiome, but this technology has the limitation of lacking quantitative functional annotation. Fecal metabolomics can better interpret the metabolic interactions among host, diet, and gut microbiota, and provide microbiome function data to supplement the deficiency of sequencing (Marcobal et al., 2013). The combination of metabolomics and microbiome is increasingly used to study the pathogenesis of diseases (Zierer et al., 2018; Shi et al., 2020; Na et al., 2021). Understanding the mechanism of the link between periodontitis and comorbidities is the focus of future translational research, and combined microbial and metabolic multi-omics analysis may provide a better understanding of the underlying mechanisms by which periodontitis affects other diseases.
Liquid chromatography–mass spectrometry (LC–MS) is a widely used metabolomics research technique with high resolution, high throughput, high sensitivity, and high specificity. In this study, we constructed an experimental periodontitis model and performed multi-omics analysis of all rats’ feces, that is, a combination of microbiome analysis using 16S rDNA V3-V4 region sequencing and metabonomic analysis using LC–MS. We first analyzed the impact of periodontitis on the gut microbiota and fecal metabolites, and then analyze their correlations and participating pathways in detail. The combination of microbiome and metabolomics can effectively provide sufficient and accurate data to reveal the influence of periodontitis. It could provide ideas for studying the potential mechanism of periodontitis affecting other diseases.
Materials and Methods
Experimental Periodontitis Rat Model and Sample Collection
Ten 7-week-old male Sprague–Dawley (SD) rats (purchased from Beijing Vital River Laboratory Animal Technology Co. Ltd., Beijing, China) were randomly divided into two groups (n = 5 each group). We conducted the adaptability of feeding for 1 week before the experiment. One group did not take additional treatments (Control), the other group was ligated with sterile nylon thread around the cervical of bilateral maxillary first and second molars to induce experimental periodontitis (EP). The construction of the EP model and animal experiments were consistent with our previous study (Fang et al., 2021a). After 4 weeks of the experiment, the feces of each rat were collected using sterile cryopreservation tubes with a unique label, and frozen at −80°C within 3 h after sampling (Sato et al., 2021). Bilateral maxillary alveolar bone–contained molars were harvested and fixed with 4% paraformaldehyde. Rats were cared for in accordance with China’s “Animal Care and Use Guide.” This study conformed to the ARRIVE guidelines and was approved by the Animal Ethics Committee of Wuhan University (IACUC 2018119). Details are further described in the Supplementary Material.
Microcomputed Tomographic and Histopathological Analyses of Alveolar Bone
Microcomputed tomographic (Skyscan 1176, Bruker, Kontich, Belgium) was used to evaluate the alveolar bone loss in each group. Three-dimensional (3D) reconstruction was performed by the software NReCon (Bruker, Kontich, Belgium) after scanning. And then the 3D images were obtained by the software CTvox (Bruker, Kontich, Belgium). The periodontal attachment loss was measured by DataViewer 1.5.2.4 (Bruker, Kontich, Belgium) for the maxillary second molar of four sites (the proximal buccal, proximal palatal, distal buccal, and distal palatal), and then the average value of periodontal attachment loss was calculated for statistical analysis.
The residual side of the maxillae was decalcified with 10% ethylenediaminetetraacetic acid (EDTA) disodium salt for 1 month and then embedded in paraffin. And then, from the occlusal surface of the crown to the alveolar bone, 4 μm sections were prepared along a plane parallel to the long axis of the tooth. The sections were standardly stained with hematoxylin and eosin (H and E), and images were captured with a light microscope (Leica DFC295, Wetzlar, Germany).
DNA Extraction
According to the manufacturer’s instructions, we used MagPure Stool DNA KF kit B (Magen, Guangdong, China) to extract microbial genomic DNA from feces. The quantity and quality of DNA were checked by Qubit dsDNA BR Assay kit (Invitrogen, Carlsbad, CA, United States) with a Qubit Fluorometer and 1% agarose gel, respectively.
16S rDNA Microbiome Sequencing
The 16S rDNA amplicon (V3–V4 region) sequencing was performed on the Illumina HiSeq 2500 (BGI, Shenzhen, China) platform. The primers were all processed with Illumina standard method, and the sequence used was 341F (5′-ACTCCTACGGGAGGCAGCAG-3′) and 806R (5′-GGACTACHVGGGTWTCTAAT-3′). A 30 ng of qualified genomic DNA samples and the corresponding fusion primers were taken to configure the PCR reaction system. The PCR products were purified and eluted with AmpureXP beads to construct the library. We obtained 2 × 300 bp paired-end reads and the raw data have been deposited under NCBI BioProject accession numbers PRJNA778630 (EP) and PRJNA762590 (Control).
Untargeted Metabolomics
Waters 2D UPLC (Waters, Milford, CT, United States) tandem Q Exactive HF high-resolution mass spectrometer (Thermo Fisher Scientific, Waltham, MA, United States) was used to separate and detect metabolites (see Supplementary Material for mobile phase and chromatographic separation). Samples (5 μl) were detected on a BEH C18 column (1.7 μm 2.1*100 mm, Waters, United States) at 45°C at a flow rate of 0.35 ml/min. Q Exactive HF mass spectrometer (Thermo Fisher Scientific, United States) was used for primary and secondary mass spectrometry data acquisition. Primary and secondary resolutions are 120,000 and 30,000, respectively. The samples were analyzed in positive ion (Spray voltage was 3.8 kV) and negative ion (Spray voltage was 3.2 kV) modes. The mass scanning range was 70–1050 m/z, and the ion transfer tube temperature was 320°C. The flow rates of sheath gas and auxiliary gas were set to 40 L/min and 10 L/min, respectively.
Bioinformatic Analysis and Visualization
The total number of raw reads obtained by sequencing was 1,411,750, filtered to remove adaptors, low-quality, and ambiguous bases, and finally, 1,361,514 clean reads were obtained, with an average of 68,075.7*2 reads per sample. The detailed analysis steps of OTU clustering, chimera removal, α-diversity, β-diversity, difference comparison, and functional prediction are shown in the Supplementary Material.
Compound Discoverer 3.1 (Thermo Fisher Scientific, United States) was used to process the mass spectrum raw data and identify metabolites. R software package metaX (Wen et al., 2017) was used for data preprocessing, statistical analysis, metabolite classification annotation, and functional annotation. Principal component analysis (PCA) was used to analyze the overall distribution and stability between and within groups. Then, Partial Least-Squares Method–Discriminant Analysis (PLS–DA) was used to analyze the differences between groups and calculate Variable importance for the projection (VIP) of metabolites. To ensure model quality, sevenfold cross-validation was performed during modeling and validated by 200 response permutation testing. The obtained VIP values, combined with Fold Change (FC) obtained by univariate analysis, and p obtained by Student’s t-test were used to identify differential metabolites. Kyoto Encyclopedia of Genes and Genomes (KEGG) is a database for analyzing higher-order functions based on genomic information. KEGG pathway enrichment analysis was obtained by using hypergeometric tests to compare the significant difference metabolites with all identified metabolites as a background.
Spearman’s rank correlation coefficient was used to analyze the correlation between microbiota and metabolites, and visualization was made in the form of a heat map.
Statistical Analyses
Data were evaluated using the Wilcoxon rank-sum test for two-group comparisons using the Wilcox-test package in R (v3.4.1). Correlations were identified by Spearman’s rank correlation coefficient (significance thresholds were p < 0.05) using the corrplot package in R (v3.4.1). GraphPad Prism V.8.0 (GraphPad Software Inc., San Diego, CA, United States) and R are used for various analyses and chart preparation. *p < 0.05, **p < 0.01 and ***p < 0.001 were considered significant.
Results
Establishment of Experimental Periodontitis and Detection of Gut Microbiota
Microcomputed tomographic was used to obtain 3D reconstruction images of alveolar bone, and the maxillary alveolar bone morphology was displayed (Figure 1A). Compared with the control group, obvious absorption of alveolar bone and exposure of roots were observed in the EP group. In addition, statistical analysis showed that the periodontal attachment loss in the EP group was significantly higher than that in the control group (Figure 1B). And the results of the H and E staining section (Figure 1C) focused on the periodontal tissue of the second molar. The images from EP groups presented gingival epithelium erosion with infiltrated inflammatory cell, collagen fiber breakdown, and resorption of alveolar bone. While no obvious pathological changes were observed in the control group. Taken together, these results confirmed that the experimental periodontitis was successfully induced.
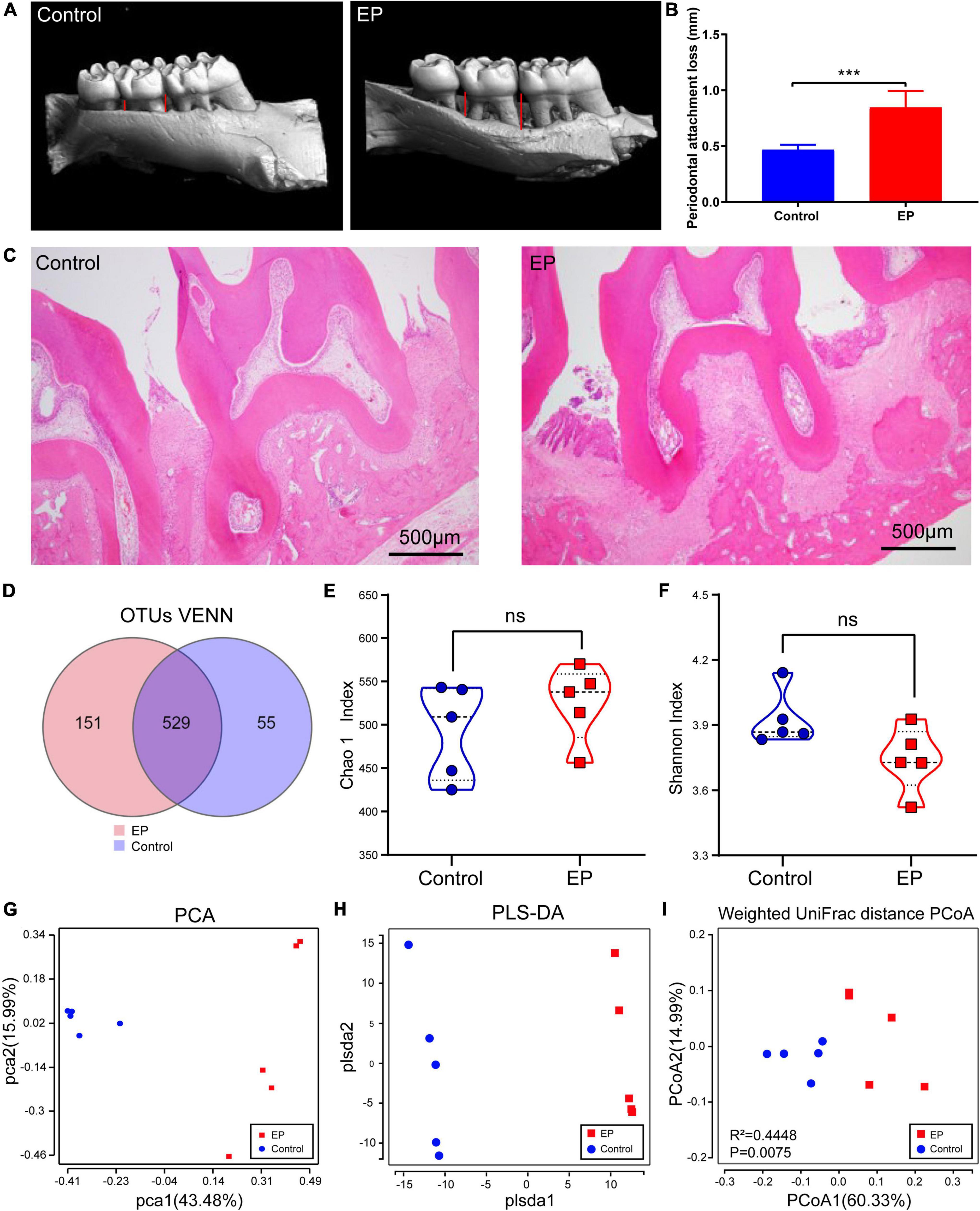
Figure 1. Detection of periodontal tissue in rats and diversity analysis of gut microbiota. (A) Representative 3D reconstruction images of alveolar bone by micro-CT. (B) Histogram of statistical analysis of periodontal attachment. (C) Representative figures from H and E staining for the second molar. (D) Shared and unique OTUs in the gut microbiome in EP and control group. Chao 1 index (E) and Shannon index (F) of the two groups. Principal component analysis (PCA) analysis (G), partial least-squares method–discriminant analysis (PLS–DA) (H), and Principal Coordinate Analysis (PCoA) based on Weighted Unifrac distance matrix (I) of EP and Control group. EP, experimental periodontitis group; Control, healthy control group. ***p < 0.001; ns, no significance.
The rarefaction curve of gut microbial 16S rDNA sequencing shows that the sample’s coverage is almost approached 100% (>99.8%), indicating that the sequencing depth of this study is sufficient to reflect most bacterial characteristics of the samples (Supplementary Figure 1A). A total of 735 OTUs have been detected by clustering the clean reads with a threshold of 97% (Figure 1D). EP and control groups had 529 OTUs in common, 151 OTUs were unique in the EP group and 55 OTUs were unique in the control group. α-Diversity analysis showed that compared with the control group, slightly more OTUs (Supplementary Figure 1B), decreased abundance (Chao1), and increased diversity (Shannon) were observed in the EP group, but the differences were not statistically significant (Wilcoxon signed-rank test, p > 0.05) (Figures 1E,F).
β-Diversity considers the differences in the composition of bacterial communities in different groups. PCA and PLS–DA analysis scatter plot showed that gut microbiota in the EP group was significantly different from that in the control group (Figures 1G–I). In the principal coordinate analysis (PCoA) of the weighted UniFrac distance (Figure 1I; p = 0.0075) and unweighted UniFrac distance (Supplementary Figure 1C; p = 0.0089), both the EP and control groups were well-separated, suggesting that periodontitis alters the structure of gut microbiota.
Periodontitis Alters Gut Microbiota Composition
To further understand the differences of microbial communities between EP and control group, we classified OTUs by comparing them with the Greengene database, and obtained the species abundance of each sample at phylum and genus levels. Species with abundance below 0.2% in all samples were merged as others, and the species without taxonomic-level annotations were merged as unclassified. At the phylum level, Actinobacteria, Bacteroidetes, Cyanobacteria, Firmicutes, and Proteobacteria account for more than 99% of the bacteria (Figure 2A). Among them, Firmicutes and Bacteroidetes were dominant. Compared with the control group, Firmicutes was significantly decreased in the EP group (57.3% vs. 47.13%, p < 0.05; Supplementary Figure 2).
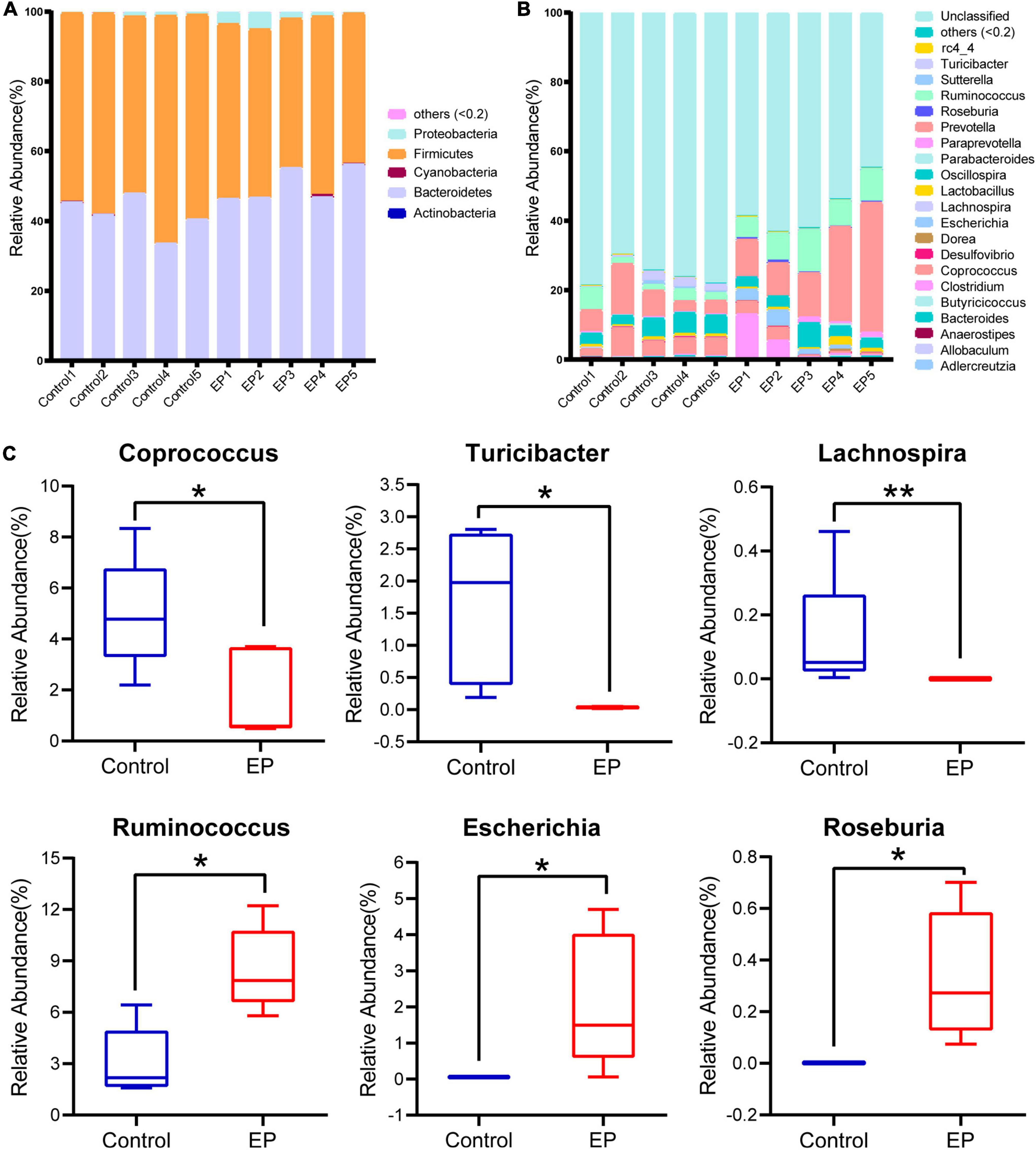
Figure 2. Differences in gut microbiota profile between EP and Control. Relative abundance of fecal microbiota composition at the level of phylum (A) and genus (B) of fecal samples in EP and Control. (C) Significantly different gut microbiota at genus level in EP compared with Control. *p < 0.05 and **p < 0.01. EP, experimental periodontitis group; Control, healthy control group.
A total of 21 genera had a relative abundance of more than 0.2% in at least one sample. The five dominant genera in the EP group were Prevotella (19.60%), Ruminococcus (8.51%), Oscillospira (3.93%), Clostridium (3.87%), and Escherichia (2.14%), but the ranking of dominant bacteria in the control group was different (Figure 2B). Compared to the control group, there were six significantly different genera in the EP group (p < 0.05; Figure 2C), in which three genera (Coprococcus, Turicibacter, Lachnospira) decreased, and three genera (Ruminococcus, Escherichia, Roseburia) increased. The LEfSe results further confirmed the difference in bacteria between EP and the control group (Supplementary Figure 3). These results suggest that gut microbiota is altered during the development of periodontitis.
The ecological connection and interaction among different genera were further investigated through Spearman’s correlation analysis. As shown in Figure 3A, Roseburia, enriched in the EP group, was associated with the highest number of microorganisms. It was significantly positively correlated with Clostridium, Escherichia, and Ruminococcus, and was co-excluded with Turicibacter and Lachnospira. Turicibacter, which was enriched in the control group, showed the strongest correlation. It was significantly positively correlated with Prevotella, Ruminococcus, and Roseburia, and significantly negatively correlated with Coprococcus, Allobaculum, and Lachnospira. The above results suggest that periodontitis induces tremendous changes and complex connections in the gut microbiota.
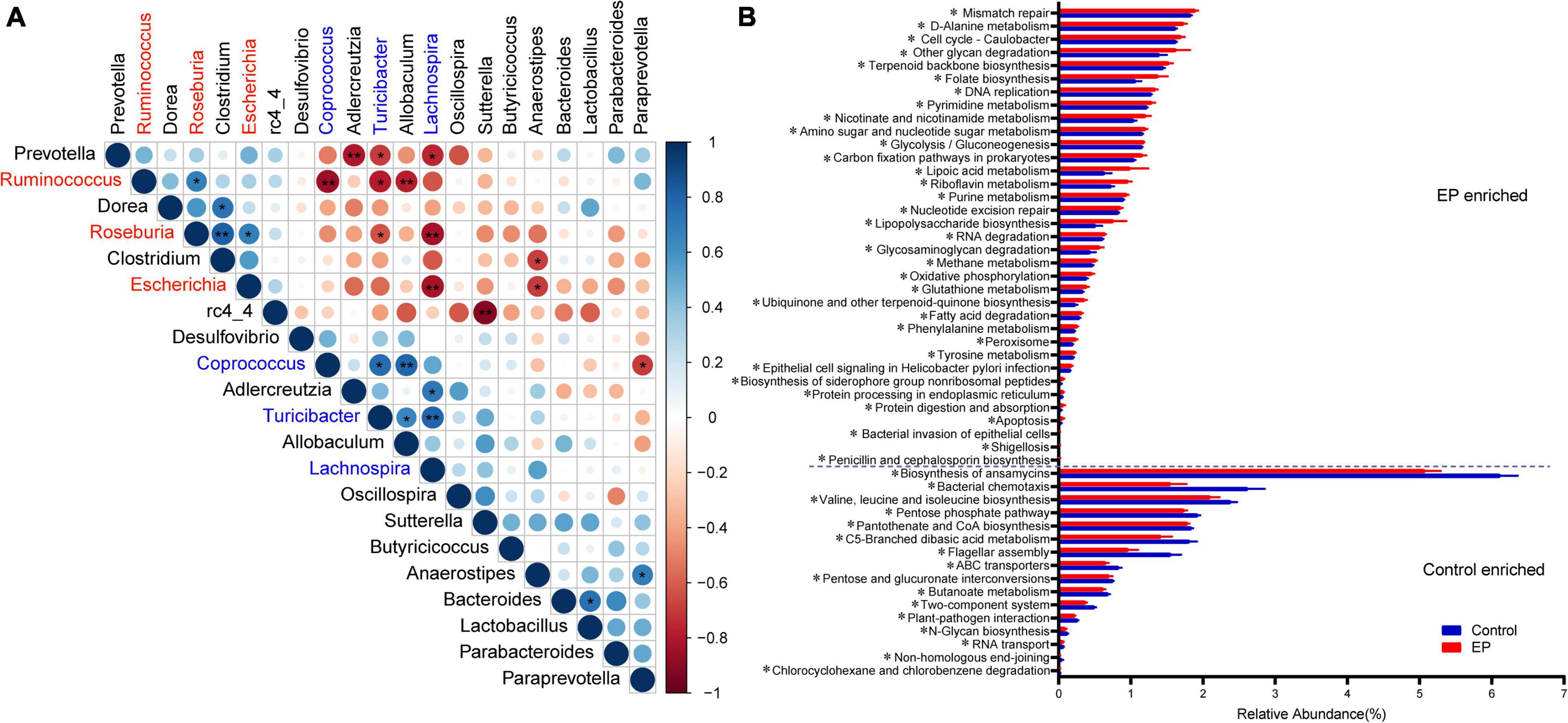
Figure 3. Relationships and predicted functions of gut microbiota. (A) Spearman’s rank correlation matrix of bacterial genera with >0.2% abundance in at least one sample. Circle size increases with absolute correlation and circle color denotes the nature of the correlation. On the scale, 1 indicates a complete positive correlation (dark blue) and −1 indicates a complete negative correlation (dark red) between the two microbial genera. Significant correlation between genera (*p < 0.05 and **p < 0.01). (B) Differentially enriched KEGG functions pathways (level 3) between EP and Control by PICRUST2 analysis. EP, experimental periodontitis group; Control, healthy control group.
Effect of Periodontitis on Functional Pathways
PICRUSt2 is a species-based bacterial community function prediction tool (Douglas et al., 2020), and we used it to predict the function of gut microbes. Figure 3B and Supplementary Figure 4 depict the significantly different functions of the two groups and focus on differences in metabolic functions. There were several pathways significantly enriched in the experimental periodontitis, including the metabolism of cofactors and vitamins (ubiquinone and another terpenoid–quinone biosynthesis, riboflavin metabolism, lipoic acid metabolism, nicotinate and nicotinamide metabolism, folate biosynthesis) and energy metabolism (oxidative phosphorylation, methane metabolism, carbon fixation pathways in prokaryotes). However, carbohydrate metabolism (butanoate metabolism, pentose, and glucuronate interconversions, C5-branched dibasic acid metabolism, pentose phosphate pathway) was significantly reduced in the experimental periodontitis. We found that periodontitis significantly affected the KEGG functional pathways by affecting the gut microbiota.
Periodontitis Disrupts the Fecal Metabolome
We further used LC–MS to analyze metabolites in feces to assess whether periodontitis disrupts the metabolome of rats. The base peak chromatogram of LC–MS experiments in positive ion modes and negative ion modes are shown in Supplementary Figures 5, 6. A total of 2,632 qualitative positive-ion metabolites and 856 qualitative negative-ion metabolites were identified. PCA analysis was used to reveal global metabolic changes in EP and control rats, and the results showed that the two groups were distinguishable but not very significant (Figures 4A,E). To capture unique metabolic model and to maximize the distinction between the EP and control group metabolites, we applied the PLS–DA model. Results showed good modeling and prediction capabilities, and the metabolite clusters of EP and control group were well-separated (Figures 4B,C,F,G). VIP value, FC, and two-tailed Student’s t-test were used to distinguish differential metabolites, and the volcano plot of metabolites is shown in Figures 4D,H. Compared with the control group, 122 positive-ion and 42 negative-ion metabolites were significantly increased in the EP group (p < 0.05, VIP > 1, and FC ≥ 1.5), and 301 positive-ion and 61 negative-ion metabolites were significantly decreased in the EP group (p < 0.05, VIP > 1, and FC ≤ 0.67). KEGG database was used to annotate all the differential metabolites, among which 27 positive-ion and 15 negative-ion metabolites participated in the signaling pathway and were taken as key metabolites (Supplementary Table 1).
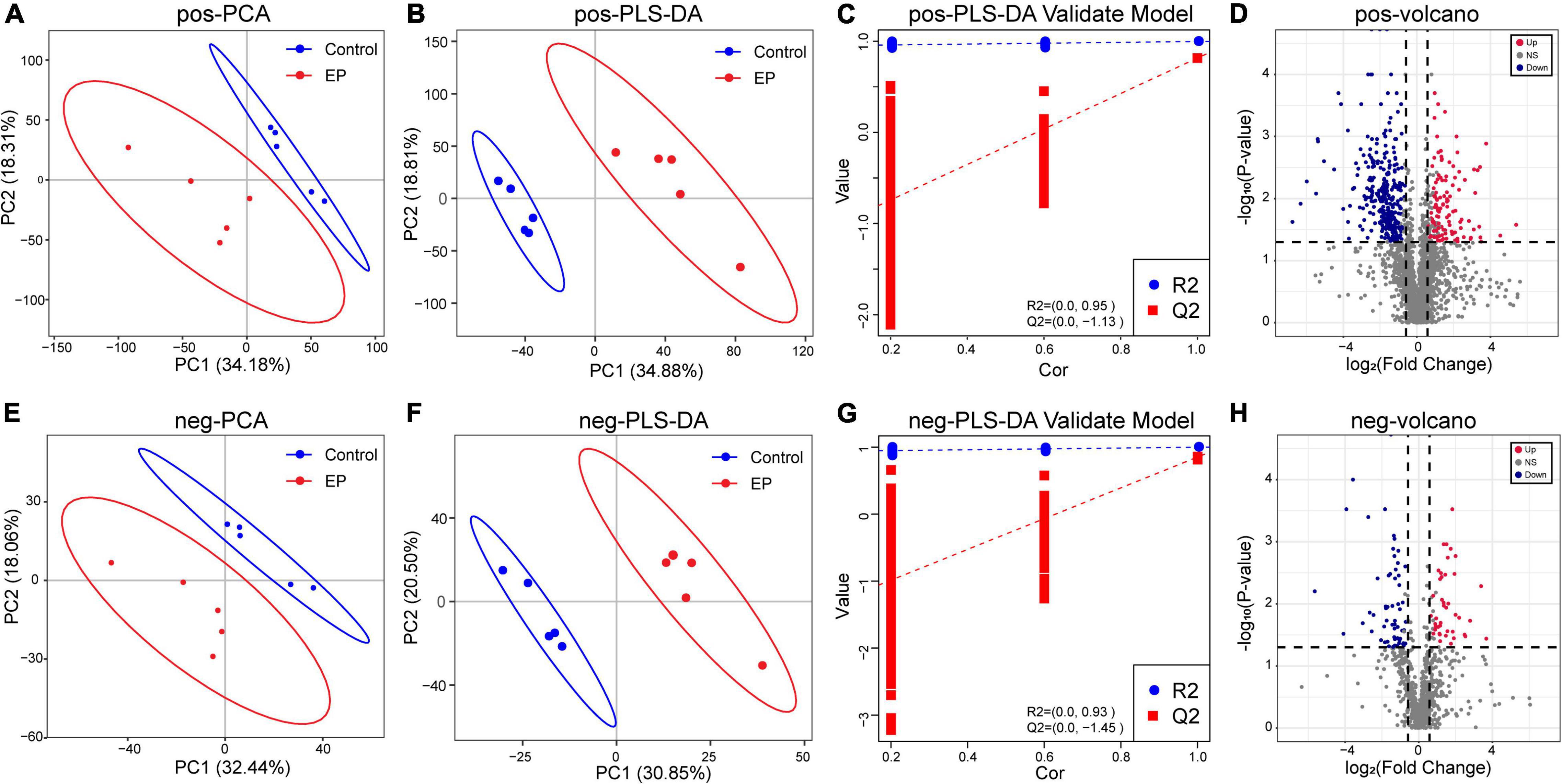
Figure 4. Differences of metabolic profiles between EP and Control groups based on LC–MS technology. Principal component analysis (PCA) analysis of positive ions (A) and negative ions (E). Partial least-squares method–discriminant analysis (PLS–DA) analysis of positive ions (B) and negative ions (F). The response permutation testing of PLS–DA for positive ions (C) and negative ions (G). The volcano chart shows the difference in positive ion metabolites (D) and the difference in negative ion metabolites (H) between EP and Control groups. The red point means upregulation, blue point means downregulation. EP, experimental periodontitis group; Control, healthy control group; pos, positive ions; neg, negative ions.
Kyoto Encyclopedia of Genes and Genomes Pathways Analysis and Enrichment
To further analyze the effects of periodontitis on metabolism, KEGG pathway annotation and enrichment analysis were performed on metabolites in both the groups. The metabolic annotation pathways and the number of corresponding upregulated and downregulated metabolites are shown in Supplementary Figures 7, 8. The results showed that these differential metabolites were mainly involved in metabolism, focusing on amino acid metabolism, lipid metabolism, and carbohydrate metabolism. The enrichment results showed that 11 positive-ion metabolic pathways (Figure 5A) and 34 negative-ion metabolic pathways (Figure 5B) were significantly enriched (Supplementary Table 2). The main positive-ion metabolic pathways involved were cysteine and methionine metabolism, tyrosine metabolism, and cAMP signaling pathway. The main negative-ion metabolic pathways involved were biosynthesis of unsaturated fatty acids, arginine biosynthesis, and biosynthesis of amino acids. The hub pathways of microbiota and metabolome were protein digestion and absorption, tyrosine metabolism, glycolysis/gluconeogenesis, nicotinate and nicotinamide metabolism, and oxidative phosphorylation, as shown in the Venn diagrams (Figure 5C).
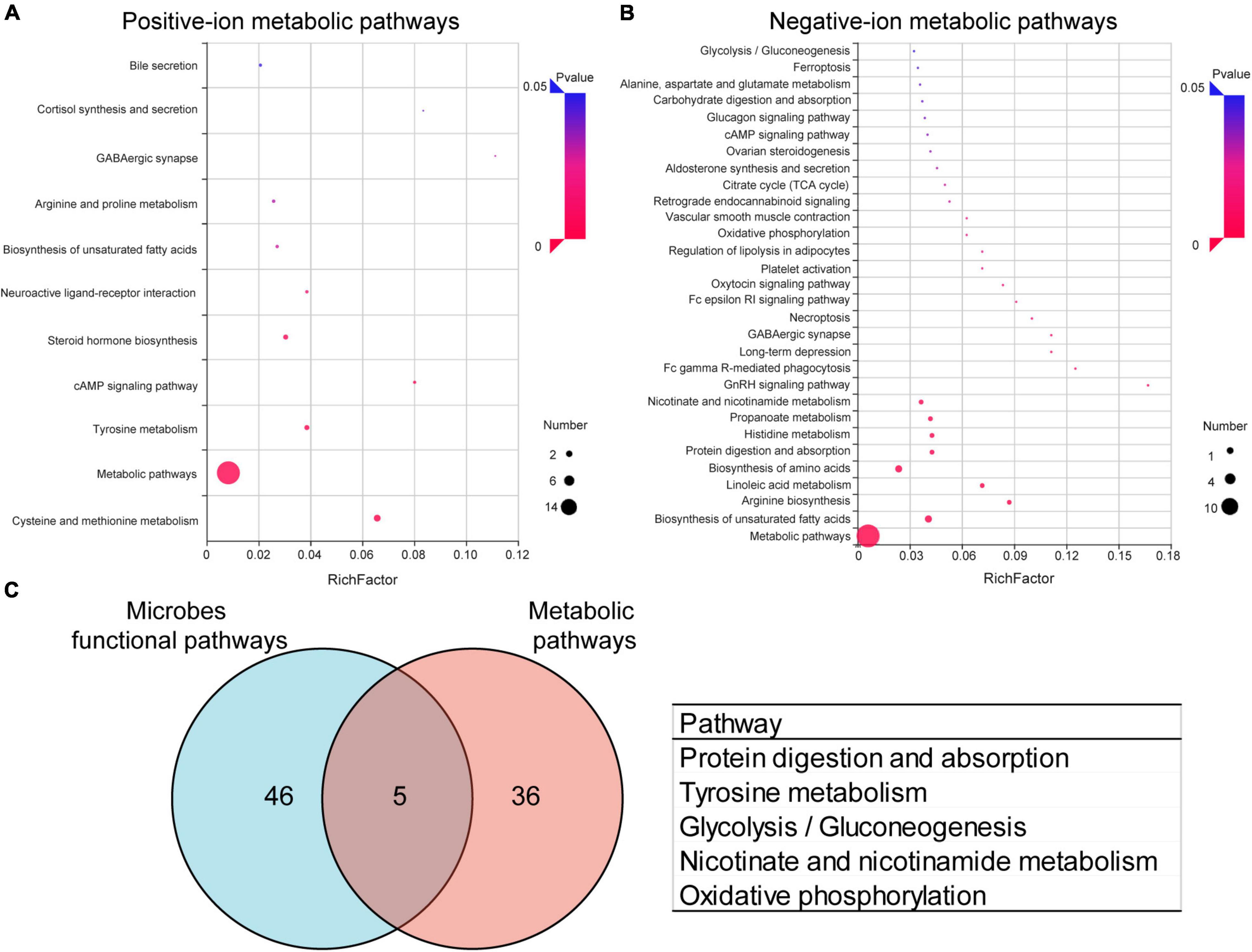
Figure 5. Effects of periodontitis on gut microbe and fecal metabolism. Enrichment of positive-ion metabolic pathways (A) and negative-ion metabolic pathways (B) in EP vs. Control. (C) Venn diagram of differential metabolic enrichment pathway and the predictive differential functional pathway of gut microbes, the table shows the KEGG pathway in common. EP, experimental periodontitis group; Control, healthy control group; pos, positive ions; neg, negative ions.
Correlation Between Gut Microbiota and Fecal Metabolites
There is a strong correlation (R = 0.94) between microbial groups and the first component of differential metabolites (Supplementary Figure 9). To better understand the relationship between gut microbiota and fecal metabolites, we conducted a correlation analysis between genus-level microorganisms and key differential metabolites (Figure 6). Roseburia, Lachnospira, Escherichia, Turicibacter, Ruminococcus, Clostridium, and Coprococcus were the genera with the greatest correlations with differential fecal metabolites, and they have significantly correlated with 35, 34, 20, 17, 15, 13, and 11 metabolites, respectively (Supplementary Table 3). On the other hand, vitamin D2 and protoporphyrin IX had the most significant correlations with gut microbiota, which were significantly related to 7 and 6 different bacterial genera, respectively (Supplementary Table 4). Second, N-acetyl-DL-glutamic acid, D-glucuronic acid 1-phosphate, Paracetamol, D-(-)-salicin, L-methionine sulfoxide, Valaciclovir, Hordenine, Hydroquinone, Kaempferol, Desoxycortone, and 17α-hydroxyprogesterone were significantly correlated with 5 different genera (Supplementary Table 4).
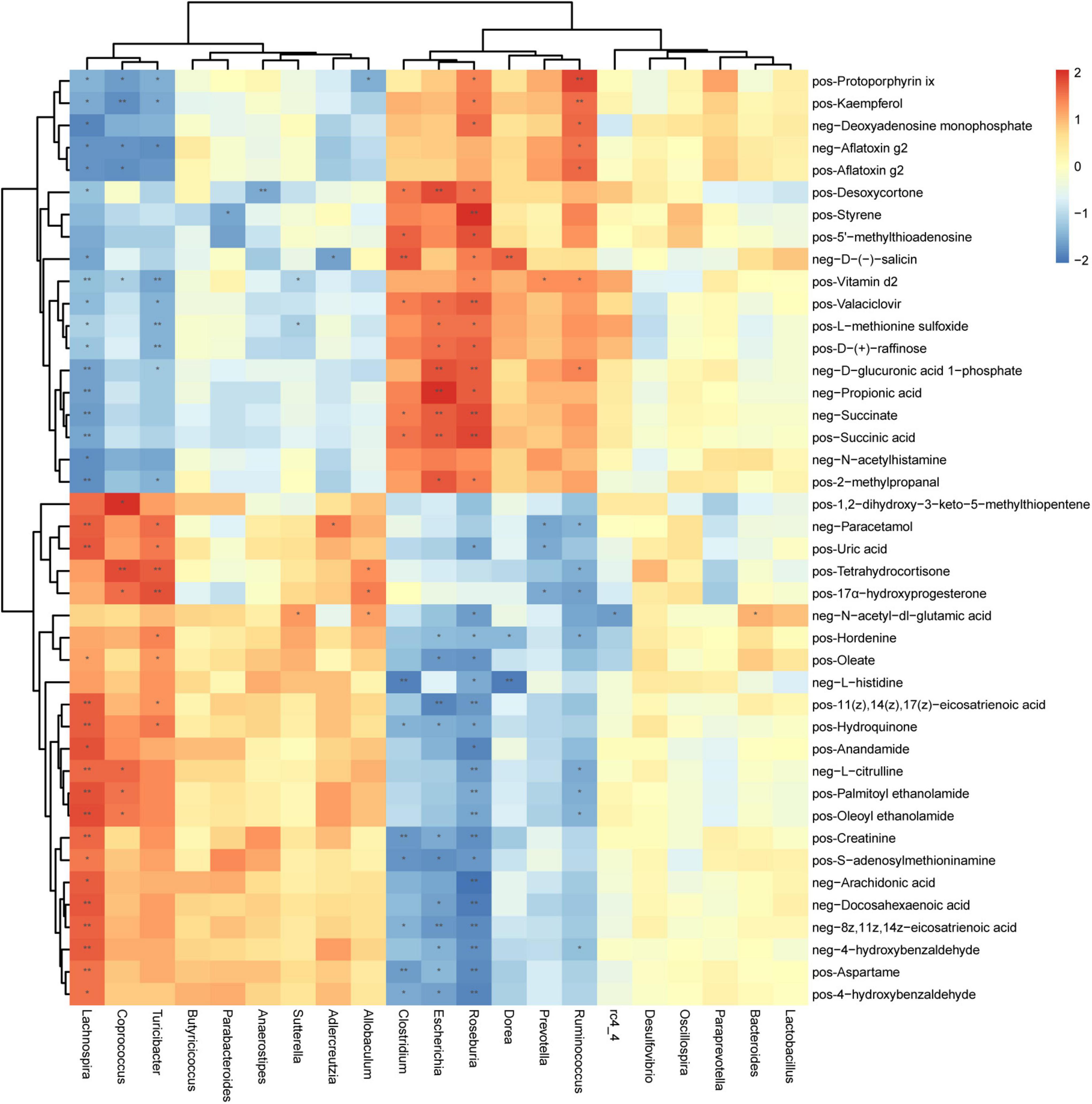
Figure 6. Correlation between microorganisms (genus level) and metabolites in feces samples of periodontitis. Each row represents a metabolite and each column represents a genus. Each grid represents the correlation coefficient between a metabolite and a genus. Red is positive correlation, and blue is negative correlation. *(p < 0.05), **(p < 0.01) Significant correlation between the genera and metabolites. pos, positive ions; neg, negative ions.
Discussion
Our multi-omics analysis results confirmed that periodontitis can change the composition of the gut microbiota and the fecal metabolism spectrum. Although species richness (i.e., the number of OTUs) was slightly higher in the EP group, there was no significant difference in α-diversity between the two groups, which is consistent with previous studies (Kirst et al., 2015; Rajan et al., 2020). However, the results of different researchers on periodontitis are not completely consistent (Wei et al., 2019). Our results are consistent with a previous study (Huang et al., 2020), there were significant differences in β-diversity between periodontitis and control based on the weighted and unweighted UniFrac distance.
This study found that periodontitis alters gut microbiota composition. Previous studies found that compared to healthy individuals, Firmicutes increased in EP groups (Lourenςo et al., 2018; Huang et al., 2020). Our results are contrary to this, which may partly be due to the high degree of variation between individuals in the microbiome (Costello et al., 2009). Porphyromonas gingivalis is a common pathogen of periodontitis. Nakajima et al. found that oral P. gingivalis significantly reduced Firmicutes in the intestinal flora (Arimatsu et al., 2014; Nakajima et al., 2015). The decrease in Firmicutes in this study may be due to P. gingivalis in the EP group. At the genus level, this study showed that Ruminococcus, Roseburia, and Escherichia were enriched in rats with periodontitis, while the proportion of Coprococcus, Turicibacter, and Lachnospira was significantly reduced. Our single microbiome analysis results are comparable to the results of previous studies (Lourenςo et al., 2018; Li et al., 2021; Watanabe et al., 2021) to a large extent.
Function analysis showed that there were more than 30 KEGG pathways significantly different in the gut microbiota of periodontitis. Including ABC transporters, valine, leucine, and isoleucine biosynthesis, fatty acid degradation, and cell cycle-Caulobacter. Other researchers have found similar functional pathways in the subgingival microbiota of periodontitis (LaMonte et al., 2018; Ebersole et al., 2020). Deng et al. (2017) have shown that the enriched functional pathways of the periodontal pocket in chronic periodontitis include bacterial chemotaxis, flagellar assembly, and two-component system. And these pathways are also detected in our study. The results of this study are comparable and unique to the results of previous studies. Through the correlation analysis, we found it interesting that the genera with significant differences in periodontitis are usually significantly correlated with more metabolites, such as Roseburia, Lachnospira, Escherichia, Turicibacter, and Ruminococcus. The genera with the same changing trend in EP tended to have a similar correlation with certain metabolites. Clostridium, which did not show any significant difference in the EP group, showed a significant correlation with fecal metabolites. It is suggested that there are complex connections between bacterial communities and metabolites.
More and more research evidence shows that the changes of gut microbiota affect the levels of metabolites, leading to the development of insulin resistance, atherosclerosis, and other diseases (Lin and Zhang, 2017; Canfora et al., 2019; Verhaar et al., 2020). Chen et al. (2021) showed that compared with healthy controls, periodontitis and high-fat diet had a significant impact on gingival metabolome, and experiments verified that high-fat diet had a superimposed effect on metabolome such as arginine metabolism in the presence of periodontitis. A recent animal study suggested that the periodontal pathogen P. gingivalis may increase insulin resistance and circulating branched-chain amino acids elevation through its metabolic activity (Tian et al., 2020). Komazaki et al. (2017) investigated the possible mechanism of periodontitis and non-alcoholic fatty liver by administering periodontal pathogens bacteria (Aggregatibacteractino mycetemcomitans) to rats, and found that it was mainly through fatty acid biosynthesis and fatty acid degradation pathways.
Growing research suggests that periodontitis may influence the progression of other diseases by altering gut microbiota and metabolism. It is reported that the gut microbiota affects the host’s metabolome (Visconti et al., 2019). We found that periodontitis increased gut bacteria Ruminococcus. Pernigoni et al. (2021) found that Ruminococcus gnavus converts the metabolites pregnenolone and hydroxypregnenolone to dehydroepiandrosterone and testosterone, regulates the steroid hormone biosynthesis pathway, thereby promoting the growth of prostate cancer. Studies have shown that benign prostatic hyperplasia has a significant impact on gut microbes and metabolism, and differential metabolites are mainly enriched in the steroid hormone biosynthesis pathways (Li et al., 2022). Periodontitis is a risk factor for prostate diseases, and animal experiments have found that periodontitis can promote the progression of benign prostatic hyperplasia (Fang et al., 2021a). However, the mechanism of interaction between periodontitis and prostate diseases has not been fully elucidated so far. We speculate that gut microbiota disturbances affecting metabolism may be one reason for periodontitis affecting prostate disease. The results of this study may provide some directions for future research, while the speculation still needed further study to confirm. If the hypothesis that periodontitis may influence other diseases by “oral-gut axis” be confirmed in the future, it can strengthen the maintenance of periodontal health to decrease the risk of systemic diseases.
In conclusion, this study not only provides the catalog of fecal metabolites that may reflect the metabolic changes in periodontitis but also illustrates the correlation analysis and related pathways between gut microbiota and fecal metabolites. The advantage of our study is that, compared to single-omics studies, the use of multi-omics methods provides a rich and complementary understanding of periodontitis and helps researchers better understand the disease. This study does have some limitations at present. First, the sample size of the study is small, and more samples need to be used for further verification research. Second, there are large differences in the gut microbiota between individuals. Overall, this multi-omics study systematically analyzes the effects of periodontitis on gut microbiota, fecal metabolites, and their enrichment pathways, which has great potential to help understand and provides a direction for further analysis of the potential mechanisms of periodontitis involved in other diseases.
Data Availability Statement
The datasets presented in this study can be found in online repositories. The names of the repository/repositories and accession number(s) can be found in the article/Supplementary Material.
Ethics Statement
The animal study was reviewed and approved by the Animal Ethics Committee of Wuhan University (IACUC 2018119).
Author Contributions
LW contributed to design, data acquisition, and analysis and drafted and critically revised the manuscript. J-YN and JH contributed to design and data interpretation and critically revised the manuscript. TD and CL contributed to data acquisition and analysis and critically revised the manuscript. CF and W-ZX contributed to conception, design, and data interpretation, and critically revised the manuscript. S-YW contributed to data analysis and interpretation and drafted and critically revised the manuscript. X-TZ contributed to conception and design and critically revised the manuscript. All authors gave final approval and agreed to be accountable for all aspects of the work.
Funding
This work was supported by the Fundamental Research Funds for the Central Universities (Nos. 2042021kf1041 and 2042020kf0130), the Key Scientific Research Project of Education Department of Henan Province (No. 22A320038), and the Nature Science Foundation of Hubei Province (No. 2019CFB760).
Conflict of Interest
The authors declare that the research was conducted in the absence of any commercial or financial relationships that could be construed as a potential conflict of interest.
Publisher’s Note
All claims expressed in this article are solely those of the authors and do not necessarily represent those of their affiliated organizations, or those of the publisher, the editors and the reviewers. Any product that may be evaluated in this article, or claim that may be made by its manufacturer, is not guaranteed or endorsed by the publisher.
Supplementary Material
The Supplementary Material for this article can be found online at: https://www.frontiersin.org/articles/10.3389/fmicb.2022.865191/full#supplementary-material
References
Arimatsu, K., Yamada, H., Miyazawa, H., Minagawa, T., Nakajima, M., Ryder, M. I., et al. (2014). Oral pathobiont induces systemic inflammation and metabolic changes associated with alteration of gut microbiota. Sci. Rep. 4:4828. doi: 10.1038/srep04828
Canfora, E. E., Meex, R. C. R., Venema, K., and Blaak, E. E. (2019). Gut microbial metabolites in obesity, NAFLD and T2DM. Nat. Rev. Endocrinol. 15, 261–273. doi: 10.1038/s41574-019-0156-z
Chen, Z. Y., Xu, T. T., Liang, Z. J., Zhao, L., Xiong, X. Q., Xie, K. K., et al. (2021). Untargeted and targeted gingival metabolome in rodents reveal metabolic links between high-fat diet-induced obesity and periodontitis. J. Clin. Periodontol. 48, 1137–1148. doi: 10.1111/jcpe.13486
Costello, E. K., Lauber, C. L., Hamady, M., Fierer, N., Gordon, J. I., and Knight, R. (2009). Bacterial community variation in human body habitats across space and time. Science 326, 1694–1697. doi: 10.1126/science.1177486
Darveau, R. P. (2010). Periodontitis: a polymicrobial disruption of host homeostasis. Nat. Rev. Microbiol. 8, 481–490. doi: 10.1038/nrmicro2337
Deng, Z. L., Szafrañski, S. P., Jarek, M., Bhuju, S., and Wagner-Döbler, I. (2017). Dysbiosis in chronic periodontitis: Key microbial players and interactions with the human host. Sci. Rep. 7:3703. doi: 10.1038/s41598-017-03804-8
Douglas, G. M., Maffei, V. J., Zaneveld, J. R., Yurgel, S. N., Brown, J. R., Taylor, C. M., et al. (2020). PICRUSt2 for prediction of metagenome functions. Nat. Biotechnol. 38, 685–688. doi: 10.1038/s41587-020-0548-6
Ebersole, J. L., Kirakodu, S. S., Neumann, E., Orraca, L., Gonzalez Martinez, J., and Gonzalez, O. A. (2020). Oral microbiome and gingival tissue apoptosis and autophagy transcriptomics. Front. Immunol. 11:585414. doi: 10.3389/fimmu.2020.585414
Fang, C., Wu, L., Zhu, C., Xie, W. Z., Hu, H., and Zeng, X. T. (2021b). A potential therapeutic strategy for prostatic disease by targeting the oral microbiome. Med. Res. Rev. 41, 1812–1834. doi: 10.1002/med.21778
Fang, C., Wu, L., Zhao, M. J., Deng, T., Gu, J. M., Guo, X. P., et al. (2021a). Periodontitis exacerbates benign prostatic hyperplasia through regulation of oxidative stress and inflammation. Oxid. Med. Cell. Longev. 2021:2094665. doi: 10.1155/2021/2094665
Genco, R. J., and Sanz, M. (2020). Clinical and public health implications of periodontal and systemic diseases: An overview. Periodontol. 2000 83, 7–13. doi: 10.1111/prd.12344
Hajishengallis, G., and Chavakis, T. (2021). Local and systemic mechanisms linking periodontal disease and inflammatory comorbidities. Nat. Rev. Immunol. 21, 426–440. doi: 10.1038/s41577-020-00488-6
Huang, Y., Liao, Y., Luo, B., Li, L., Zhang, Y., and Yan, F. (2020). Non-surgical periodontal treatment restored the gut microbiota and intestinal barrier in apolipoprotein E(-/-) mice with periodontitis. Front. Cell. Infect. Microbiol. 10:498. doi: 10.3389/fcimb.2020.00498
Kirst, M. E., Li, E. C., Alfant, B., Chi, Y. Y., Walker, C., Magnusson, I., et al. (2015). Dysbiosis and alterations in predicted functions of the subgingival microbiome in chronic periodontitis. Appl. Environ. Microbiol. 81, 783–793. doi: 10.1128/aem.02712-14
Kitamoto, S., Nagao-Kitamoto, H., Jiao, Y., Gillilland, M. G. III, Hayashi, A., Imai, J., et al. (2020). The intermucosal connection between the mouth and gut in commensal pathobiont-driven colitis. Cell 182, 447–462.e414. doi: 10.1016/j.cell.2020.05.048
Komazaki, R., Katagiri, S., Takahashi, H., Maekawa, S., Shiba, T., Takeuchi, Y., et al. (2017). Periodontal pathogenic bacteria, Aggregatibacter actinomycetemcomitans affect non-alcoholic fatty liver disease by altering gut microbiota and glucose metabolism. Sci. Rep. 7:13950.
LaMonte, M. J., Genco, R. J., Zheng, W., McSkimming, D. I., Andrews, C. A., Hovey, K. M., et al. (2018). Substantial differences in the subgingival microbiome measured by 16S metagenomics according to periodontitis status in older women. Dent. J. (Basel) 6:58. doi: 10.3390/dj6040058
Li, J., Lu, H., Wu, H., Huang, S., Chen, L., Gui, Q., et al. (2020). Periodontitis in elderly patients with type 2 diabetes mellitus: impact on gut microbiota and systemic inflammation. Aging (Albany NY) 12, 25956–25980. doi: 10.18632/aging.202174
Li, L. L., Bao, J., Chen, B., and Yan, F. H. (2021). [High-fat diet promotes the impact of periodontitis on gut microbiota and glucose metabolism]. Zhonghua Kou Qiang Yi Xue Za Zhi 56, 539–548. doi: 10.3760/cma.j.cn112144-20210123-00037
Li, L. Y., Han, J., Wu, L., Fang, C., Li, W. G., Gu, J. M., et al. (2022). Alterations of gut microbiota diversity, composition and metabonomics in testosterone-induced benign prostatic hyperplasia rats. Mil. Med. Res. 9. doi: 10.1186/s40779-022-00373-4
Lin, L., and Zhang, J. (2017). Role of intestinal microbiota and metabolites on gut homeostasis and human diseases. BMC Immunol. 18:2. doi: 10.1186/s12865-016-0187-3
Lourenςo, T. G. B., Spencer, S. J., Alm, E. J., and Colombo, A. P. V. (2018). Defining the gut microbiota in individuals with periodontal diseases: an exploratory study. J. Oral Microbiol. 10, 1487741. doi: 10.1080/20002297.2018.1487741
Marcobal, A., Kashyap, P. C., Nelson, T. A., Aronov, P. A., Donia, M. S., Spormann, A., et al. (2013). A metabolomic view of how the human gut microbiota impacts the host metabolome using humanized and gnotobiotic mice. ISME J. 7, 1933–1943. doi: 10.1038/ismej.2013.89
Na, H. S., Kim, S., Kim, S., Yu, Y., Kim, S. Y., Kim, H. J., et al. (2021). Molecular subgroup of periodontitis revealed by integrated analysis of the microbiome and metabolome in a cross-sectional observational study. J. Oral Microbiol. 13:1902707. doi: 10.1080/20002297.2021.1902707
Nakajima, M., Arimatsu, K., Kato, T., Matsuda, Y., Minagawa, T., Takahashi, N., et al. (2015). Oral administration of P. gingivalis induces dysbiosis of gut microbiota and impaired barrier function leading to dissemination of enterobacteria to the liver. PLoS One 10:e0134234. doi: 10.1371/journal.pone.0134234
Pernigoni, N., Zagato, E., Calcinotto, A., Troiani, M., Mestre, R. P., Calì, B., et al. (2021). Commensal bacteria promote endocrine resistance in prostate cancer through androgen biosynthesis. Science 374, 216–224. doi: 10.1126/science.abf8403
Rajan, K., Zielesny, A., and Steinbeck, C. (2020). DECIMER: towards deep learning for chemical image recognition. J. Cheminform. 12:65. doi: 10.1186/s13321-020-00469-w
Sakanaka, A., Kuboniwa, M., Katakami, N., Furuno, M., Nishizawa, H., Omori, K., et al. (2021). Saliva and plasma reflect metabolism altered by diabetes and periodontitis. Front. Mol. Biosci. 8:742002. doi: 10.3389/fmolb.2021.742002
Sato, K., Yamazaki, K., Kato, T., Nakanishi, Y., Tsuzuno, T., Yokoji-Takeuchi, M., et al. (2021). Obesity-related gut microbiota aggravates alveolar bone destruction in experimental periodontitis through elevation of uric acid. mBio 12:e0077121. doi: 10.1128/mBio.00771-21
Sender, R., Fuchs, S., and Milo, R. (2016). Revised estimates for the number of human and bacteria cells in the body. PLoS Biol. 14:e1002533. doi: 10.1371/journal.pbio.1002533
Shi, M., Wei, Y., Nie, Y., Wang, C., Sun, F., Jiang, W., et al. (2020). Alterations and correlations in microbial community and metabolome characteristics in generalized aggressive periodontitis. Front. Microbiol. 11:573196. doi: 10.3389/fmicb.2020.573196
Tian, J., Liu, C., Zheng, X., Jia, X., Peng, X., Yang, R., et al. (2020). Porphyromonas gingivalis induces insulin resistance by increasing BCAA levels in mice. J. Dent. Res. 99, 839–846. doi: 10.1177/0022034520911037
Tonetti, M. S., and Van Dyke, T. E. (2013). Periodontitis and atherosclerotic cardiovascular disease: consensus report of the Joint EFP/AAPWorkshop on Periodontitis and Systemic Diseases. J. Periodontol. 84(Suppl. 4S), S24–S29. doi: 10.1902/jop.2013.1340019
Verhaar, B. J. H., Prodan, A., Nieuwdorp, M., and Muller, M. (2020). Gut microbiota in hypertension and atherosclerosis: a review. Nutrients 12:2982. doi: 10.3390/nu12102982
Visconti, A., Le Roy, C. I., Rosa, F., Rossi, N., Martin, T. C., Mohney, R. P., et al. (2019). Interplay between the human gut microbiome and host metabolism. Nat. Commun. 10:4505. doi: 10.1038/s41467-019-12476-z
Watanabe, K., Katagiri, S., Takahashi, H., Sasaki, N., Maekawa, S., Komazaki, R., et al. (2021). Porphyromonas gingivalis impairs glucose uptake in skeletal muscle associated with altering gut microbiota. FASEB J. 35:e21171. doi: 10.1096/fj.202001158R
Wei, Y., Shi, M., Zhen, M., Wang, C., Hu, W., Nie, Y., et al. (2019). Comparison of subgingival and buccal mucosa microbiome in chronic and aggressive periodontitis: a pilot study. Front. Cell. Infect. Microbiol. 9:53. doi: 10.3389/fcimb.2019.00053
Wen, B., Mei, Z., Zeng, C., and Liu, S. (2017). metaX: a flexible and comprehensive software for processing metabolomics data. BMC Bioinformatics 18:183. doi: 10.1186/s12859-017-1579-y
Wishart, D. S. (2019). Metabolomics for investigating physiological and pathophysiological processes. Physiol. Rev. 99, 1819–1875. doi: 10.1152/physrev.00035.2018
Yamazaki, K., Sato, K., Tsuzuno, T., Sulijaya, B., Mashima, I., Kawamura, Y., et al. (2020). Orally administered pathobionts and commensals have comparable and innocuous systemic effects on germ-free mice. Microb. Pathog. 140:103962. doi: 10.1016/j.micpath.2020.103962
Yuan, S., Fang, C., Leng, W. D., Wu, L., Li, B. H., Wang, X. H., et al. (2021). Oral microbiota in the oral-genitourinary axis: identifying periodontitis as a potential risk of genitourinary cancers. Mil. Med. Res. 8:54. doi: 10.1186/s40779-021-00344-1
Keywords: periodontitis, fecal metabolites, KEGG functional pathway, periodontal disease, gut microbiota, multi-omics analysis
Citation: Wu L, Han J, Nie J-Y, Deng T, Li C, Fang C, Xie W-Z, Wang S-Y and Zeng X-T (2022) Alterations and Correlations of Gut Microbiota and Fecal Metabolome Characteristics in Experimental Periodontitis Rats. Front. Microbiol. 13:865191. doi: 10.3389/fmicb.2022.865191
Received: 29 January 2022; Accepted: 16 March 2022;
Published: 14 April 2022.
Edited by:
Jozsef Soki, University of Szeged, HungaryReviewed by:
Tianfan Cheng, The University of Hong Kong, Hong Kong SAR, ChinaRosario Serpico, Università della Campania Luigi Vanvitelli, Italy
Copyright © 2022 Wu, Han, Nie, Deng, Li, Fang, Xie, Wang and Zeng. This is an open-access article distributed under the terms of the Creative Commons Attribution License (CC BY). The use, distribution or reproduction in other forums is permitted, provided the original author(s) and the copyright owner(s) are credited and that the original publication in this journal is cited, in accordance with accepted academic practice. No use, distribution or reproduction is permitted which does not comply with these terms.
*Correspondence: Shuang-Ying Wang, wsy1592738@163.com; Xian-Tao Zeng, zengxiantao1128@163.com, zengxiantao1128@whu.edu.cn