- 1Department of Anesthesiology, The Second Affiliated Hospital of Guangzhou University of Chinese Medicine, Guangzhou, China
- 2School of Human and Social Sciences, University of West London, London, United Kingdom
- 3Department of Anesthesiology, Hubei Key Laboratory of Geriatric Anesthesia and Perioperative Brain Health, Wuhan Clinical Research Center for Geriatric Anesthesia, Tongji Hospital, Tongji Medical College, Huazhong University of Science and Technology, Wuhan, China
Introduction: Owing to advancements in non-invasive magnetic resonance imaging, many studies have repeatedly showed that diabetes affects the central nervous system in the presence of peripheral neuropathy, suggesting a common or interacting pathological mechanism for both complications.
Methods: We aimed to investigate the role of abnormal gut microbiota in rats with diabetic peripheral neuropathy (DPN) combined with cognitive dysfunction. Glucose-compliant rats with nerve conduction deficits were screened as a successful group of DPN rats. The DPN group was then divided into rats with combined cognitive impairment (CD) and rats with normal cognitive function (NCD) based on the results of the Novel object recognition test. Rat feces were then collected for 16S rRNA gene sequencing of the intestinal flora.
Results and Discussion: The results revealed that abnormalities in Firmicutes, Ruminococcaceae, Bacteroidia, and Actinobacteria-like microorganisms may induce DPN complicated by cognitive dysfunction.
Introduction
Cognitive impairments have been linked to poor glycemic control, long-term duration of Diabetes mellitus (DM), and microvascular complications such as diabetic retinopathy and diabetic peripheral neuropathy (DPN) (Groeneveld et al., 2018). Of these, DPN is the only peripheral nervous system (PNS) disease. With advancements in non-invasive magnetic resonance imaging, studies have indicated that the central nervous system (CNS) is also involved in DPN development (Selvarajah et al., 2011; Lee and Tracey, 2013; Wilkinson et al., 2013). There may be shared pathogenic mechanisms between cognitive impairment and DPN, and several risk factors such as HbA1c levels and chronic hyperglycemic states have been identified for both conditions (Zilliox et al., 2016). However, few studies have examined the co-occurrence of diabetic peripheral nerve complications and cognitive dysfunction.
The intestinal microecosystem, is a significant component of human flora and the largest and most complex microecosystem in the human body. The gut microbiota has been shown to influence disease pathogenesis, including neuroimmune and neurodegenerative diseases, obesity, diabetes, liver disease, and cancer, through the microbiota-gut-brain axis (Iannone et al., 2019; Cryan et al., 2020). In addition, many studies have demonstrated a complex interaction between the gut microbiota and the CNS.
Gut microbiota plays an important role in cognitive function through the gut-brain axis (Gareau, 2014; Rogers et al., 2016). Dysbiosis of the gut microbiota contributes to neuroinflammation, leading to cognitive dysfunction in mice with Parkinson’s disease (PD) (Sampson et al., 2016). Amyloid protein deposition in the intestine changes the level of short-chain fatty acids in the intestinal microflora, which exacerbates cognitive impairment in mice with Alzheimer’s disease (AD) (Zhang et al., 2017). The gut microbiota also impacts cognitive function in patients with AD by affecting the release and metabolism of bile acids (MahmoudianDehkordi et al., 2019) and is associated with other neurological diseases, such as depression (Zheng et al., 2016), seizures (Olson et al., 2018), schizophrenia (Zheng et al., 2019), and Huntington disease especially symptomized with chorea (Radulescu et al., 2019). Similarly, the occurrence and development of DPN is related to intestinal flora imbalance (Gao et al., 2018; Salguero et al., 2019); however, there is little information about the relationship between intestinal flora and cognitive impairment in DPN.
Therefore, we screened DPN rats with concomitant cognitive dysfunction for the first time using a new object recognition assay to compare and analyze the differences between their intestinal flora and those of rats with DPN alone.
Materials and methods
Animals
SPF Sprague–Dawley rats weighing 200–220 g were purchased from the Laboratory Animal Management Center of Southern Medical University. Animal production license number: SYXK (Guangdong) 2018–0094, animal qualification number: SCXK (Guangdong) 22,016–0041. The experimental animals were reared according to the feeding and management regulations of SPF Experimental Animal Center, Guangdong Academy of Chinese Medicine. Animal use license number: No. 44002100027214. All operations in the experiment followed the relevant regulations of Animal Ethics Committee of Laboratory Animal Center, Guangdong Academy of Chinese Medicine.
Modeling
DPN model
Rats were randomly divided into the normal control group and DPN groups. After 1 week of adaptive feeding with an ordinary diet, rats in the model group were fed a high-fat and high-sugar diet for 8 weeks and then intraperitoneally injected with 0.1% STZ solution (35 mg/kg). They were then banned from consuming food for 60 h, albeit drinking water. Blood from the tail vein was collected after 72 h, and fasting blood glucose was measured using a blood glucose meter and test strips. When the rat’s blood glucose level was ≥16.7 mmol/L and the blood glucose level was stable for 3 consecutive days, the DM model was judged successful and included in the experiment. Two weeks after the STZ injection, the sciatic nerve conduction velocity of the rats in the DM group was measured. The DPN model was successfully constructed if a nerve conduction disorder occurred in rats that reached the target blood glucose level.
Novel object recognition test
The NORTs conducted in our study were based on relevant literature (Tarragon et al., 2014; Li et al., 2022; Liu et al., 2022; Sun et al., 2022; Yang et al., 2022; He et al., 2023). Twenty-four hours before testing or training, the animals were placed in the testing room to acclimatize to the testing environment. The test was divided into training and detection phases. Two identical objects, A and B, were placed symmetrically in the experimental box, and the rat was placed in the field with its back facing the two objects. The distance from the rat’s nose to the two objects was the same. The rat was placed in the box for 10 min, and the video recording device was turned on immediately. The experimenter left the test room, then record the number of times the nose or mouth touched the object and the exploration within a range of 2–3 cm from the object (fore paws resting on an object, sniffing an object, and licking an object were recorded as exploration, and posing or climbing on an object did not count as exploration of a new novel object). One hour after the end of the training period, the experiment in the test period was conducted, in which an object was replaced by a novel object(C) with different shapes and colors but the same size. In different experimental stages and between each task of the two rats, the box and objects were wiped with 70% alcohol to avoid disturbance of the free exploration behavior caused by the smell left by the animals. The exploration time of the rats was calculated by two people watching the video at the same time and finally the average was taken for calculation.
The design process of the novel object recognition experiment is illustrated in the figure below.
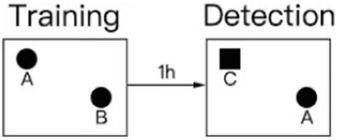
Finally, the recognition index ratio of the time spent exploring object C in the total exploration time was calculated, and SPSS statistical software (IBM, SPSS Inc., NY, United States) was used for cluster analysis to count the cognitive impairment and non-cognitive impairment of novel object recognition in the model group rats. The rats in the modeling group were divided into a CD group with cognitive dysfunction and an NCD group without cognitive dysfunction.
Open-field test
Prior to the novel object recognition experiment, an open-field experiment was performed to measure the movement time and distance of the rats, which confirmed that the movement ability of the rats was normal. To eliminate fear, the rats were acclimated to the laboratory for 1 day before the experiment. The rat open-field reaction box was 40 cm high and 100 cm long at the bottom, and the inner walls were painted black. Observation indicators included the number of times the subjects entered the central area, walking distance, time spent in the area, maximum running speed, number of hindlimb stands, and number of times they entered the peripheral area.
Animal sample materials
The rats were placed in sterilized cages with Ultraviolet light (UV)-sterilized filter paper, and immediately after natural defecation, feces were collected in sterile Eppendorf (EP) tubes with sterilized tweezers to avoid urine contamination. The collected samples were immediately transferred to a −80°C refrigerator for storage, waiting for analysis. The filter paper was changed every time a rat’s feces were collected to ensure sterility. The collected stool samples were subjected to 16 s rRNA high-throughput sequencing.
DNA extraction and amplification
Total genomic DNA was extracted using MagPure Soil DNA LQ Kit (Magan) following the manufacturer’s instructions. DNA concentration and integrity were measured with NanoDrop 2000 (Thermo Fisher Scientific, United States) and agarose gel electrophoresis. Extracted DNA was stored at −20°C until further processing. The extracted DNA was used as template for PCR amplification of bacterial 16S rRNA genes with the barcoded primers and Takara Ex Taq (Takara). For bacterial diversity analysis, V3-V4 (or V4-V5) variable regions of 16S rRNA genes was amplified with universal primers 343F (5′-TACGGRAGGCAGCAG-3′) and 798R (5′-AGGGTATCTAATCCT-3′) (Nossa et al., 2010) for V3-V4 regions.
Library construction and high-throughput sequencing
Libraries were constructed using a library construction kit and quantified using a Qubit instrument and qPCR. Then, after the MiSeq libraries passed quality checks, 16S rRNA gene V3-V4 sequencing was performed on the Illumina MiSeq platform.
Sequence analysis
The library sequencing and data processing were conducted by OE biotech Co., Ltd. (Shanghai, China). Raw sequencing data were in FASTQ format. Paired-end reads were then preprocessed using Trimmomatic software to detect and cut off ambiguous bases (N). It also cut off low-quality sequences with average quality score below 20 using sliding window trimming approach. After trimming, paired-end reads were assembled using FLASH software. Parameters of assembly were: 10 bp of minimal overlapping, 200 bp of maximum overlapping, and 20% of maximum mismatch rate. Sequences were performed further denoising as follows: reads with ambiguous, homologous sequences or below 200 bp were abandoned. Reads with 75% of bases above Q20 were retained. Then, reads with chimera were detected and removed. These two steps were achieved using QIIME software (version 1.8.0).
Clean reads were subjected to primer sequences removal and clustering to generate operational taxonomic units (OTUs) using Vsearch software with 97% similarity cutoff. The representative read of each OTU was selected using QIIME package. All representative reads were annotated and blasted against Silva database Version 138 using RDP classifier (confidence threshold was 70%).
QIIME software was used for alpha- and beta-diversity analysis. The microbial diversity in samples was estimated using the alpha-diversity that include Chao1 index and Shannon index. The unweighted UniFrac distance matrix performed by R package was used for unweighted UniFrac Principal coordinates analysis (PCoA) to estimate the beta diversity. Then the R package was used to analyze the significant differences between different groups using ANOVA/Kruskal–Wallis statistical test. The linear discriminant analysis effect size (LEfSe) method was used to compare the taxonomy abundance spectrum. LEfSe analysis was calculated using LEfSe software, version V 1.0.8 (Segata et al., 2011).
Statistical analysis of data
Statistical analysis of all data was performed using SPSS 17 software, and the results were expressed as mean plus or minus standard deviation (MEAN ± SEM). In our analysis of the experimental results, body weight, blood glucose and nerve conduction velocity of control rats and model rats were analyzed by t-test under the precondition that the normal distribution was met. The data regarding the new object recognition test in the model group rats were analyzed using hierarchical cluster analysis, dividing the rats in the model group into the CD group and the NCD group. The data regarding the analysis of the locomotor ability of the rats in the CD group and the rats in the NCD group, i.e., the data in the open-field part of the experiment, also conformed to a normal distribution, so the t-test was used. p < 0.05 was considered to be statistically significant.
Results
Changes in animal body weight, blood glucose levels, and nerve conduction velocity
After 8 weeks of high-fat and high-sugar feeding, 35 mg/kg streptozotocin (STZ) was injected intraperitoneally for 3 consecutive days to establish type 2 DM (Sharma et al., 2023). We continued the high-fat and high-sugar feeding for 2 weeks; then, the rats were tested for sciatic nerve conduction velocity to establish a DPN model (Figure 1A). There was no significant difference in fasting blood glucose and body weight between control rats (9 rats) and model rats (24 rats) before starting diabetic diet feeding (Figures 1B,C). The blood glucose values of the model rats were significantly higher than those of control rats on days 3 and 6 after the STZ injection (Figure 1B). However, body weight of the rats in the model group was significantly lower than that of the control rats only on day 6 after the STZ injection (Figure 1C). There was no difference in nerve conduction velocity between both groups before the start of the experiment. Two weeks after completion of the intraperitoneal injection of STZ, the sciatic nerve conduction velocity of rats in the model group was significantly lower than that in the control group (Figure 1D).
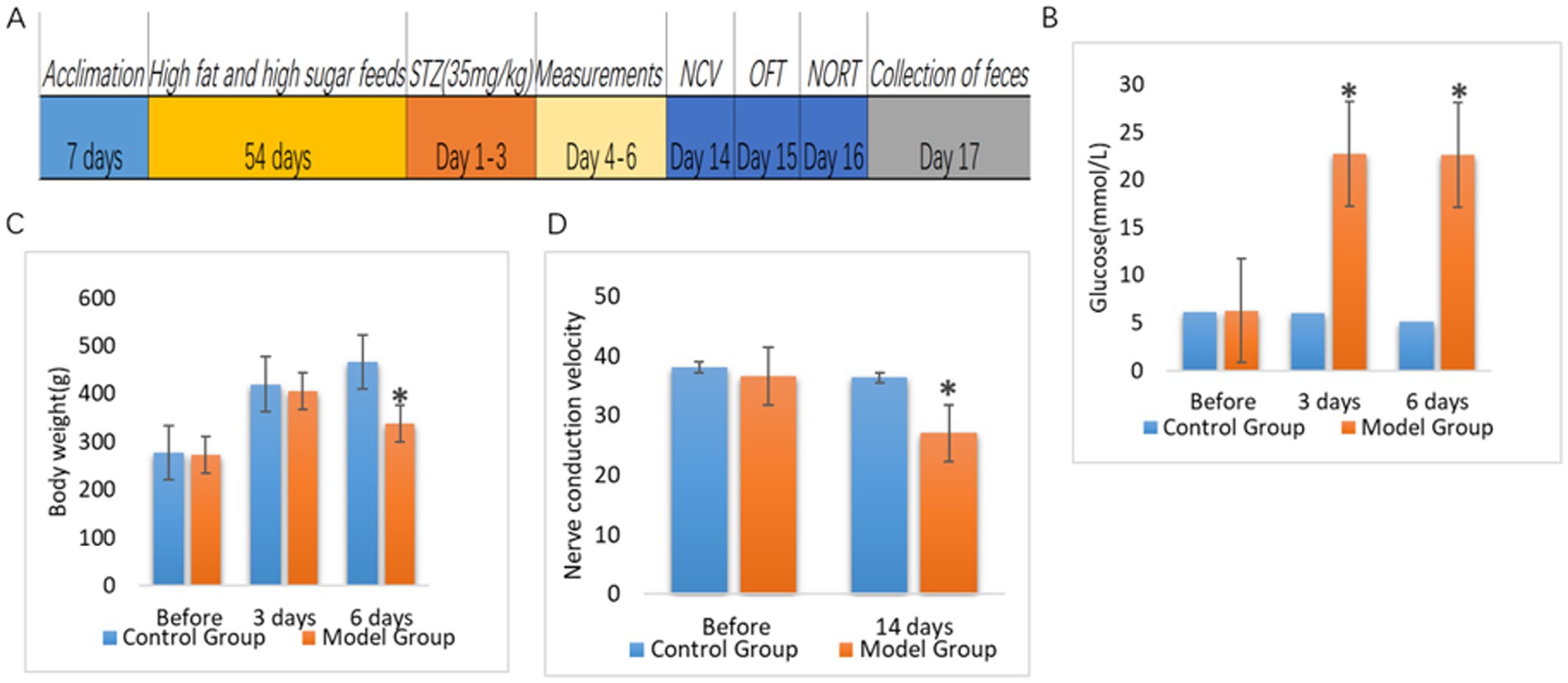
Figure 1. Comparison of blood glucose, body weight and nerve conduction velocity between control rats (Control Group) and rats with diabetic peripheral neuropathy (Model Group). (A) Timeline of this study. After 7 days of acclimatization feeding, rats in the experimental group were started on high-fat, high-sugar feeding for 8 weeks. After 8 weeks, rats in the experimental group were injected intraperitoneally with STZ (35 mg/kg) for 3 consecutive days to induce diabetes, and rats in the control group were injected intraperitoneally with saline (35 mg/kg). Body weight and blood glucose levels were measured from day 4 to day 6 after the start of injection, and nerve conduction velocity was measured in the rats on day 14. (B) Blood glucose levels (p < 0.05). (C) Body weight (p < 0.05). (D) Sciatic nerve conduction velocity (p < 0.05). *p<0.05.
Cognitive performance of rats in the DPN group
Based on the results of the Novel object recognition test (NORT) behavioral experiment, the model group rats were analyzed by hierarchical clustering into the DPN group with cognitive dysfunction (CD) and DPN group without cognitive dysfunction (NCD) (Figure 2A). After the NORT, an open-field experiment was conducted to compare the total distance and mean locomotor speed of rats in both groups. The results revealed no difference in the locomotor ability of the two groups (Figures 2B,C).
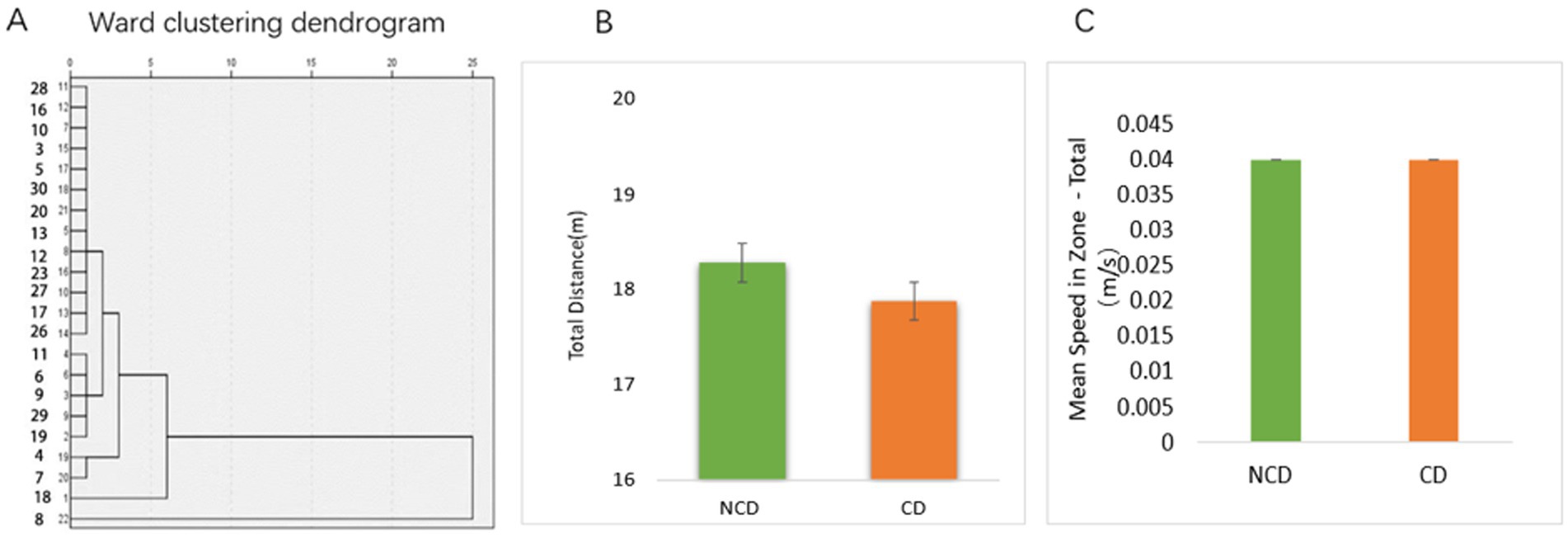
Figure 2. New object recognition in diabetic peripheral neuropathy combined with cognitive impairment (CD) and diabetic peripheral neuropathy non-CD rats. (A) Hierarchical cluster analysis of the dendrograms. Results of the hierarchical cluster analysis based on NORT results. DPN rats were divided into cognitive dysfunction group (Group_CD) and no cognitive dysfunction group (Group_NCD). (B,C) The NORT test was followed by an absentee field experiment to test the locomotor ability of the rats (p > 0.05).
Analysis of the structure and diversity of the intestinal flora of various groups of rats
The structure and diversity of the intestinal flora was analyzed comparatively between the CONTROL, CD, and NCD groups by means of Circos plots, alpha-diversity, and beta-diversity. Circos plots showed that the structure of the flora was very different between the three groups, with a significant increase in thick-walled bacteria observed in DPN rats (Figure 3A). For alpha-diversity, the Shannon and Simpson indices indicated no significant variation between the groups (Figures 3B,C). Beta-diversity of PCA showed the gut microbiota to be far apart from each other (Figures 3D,E).
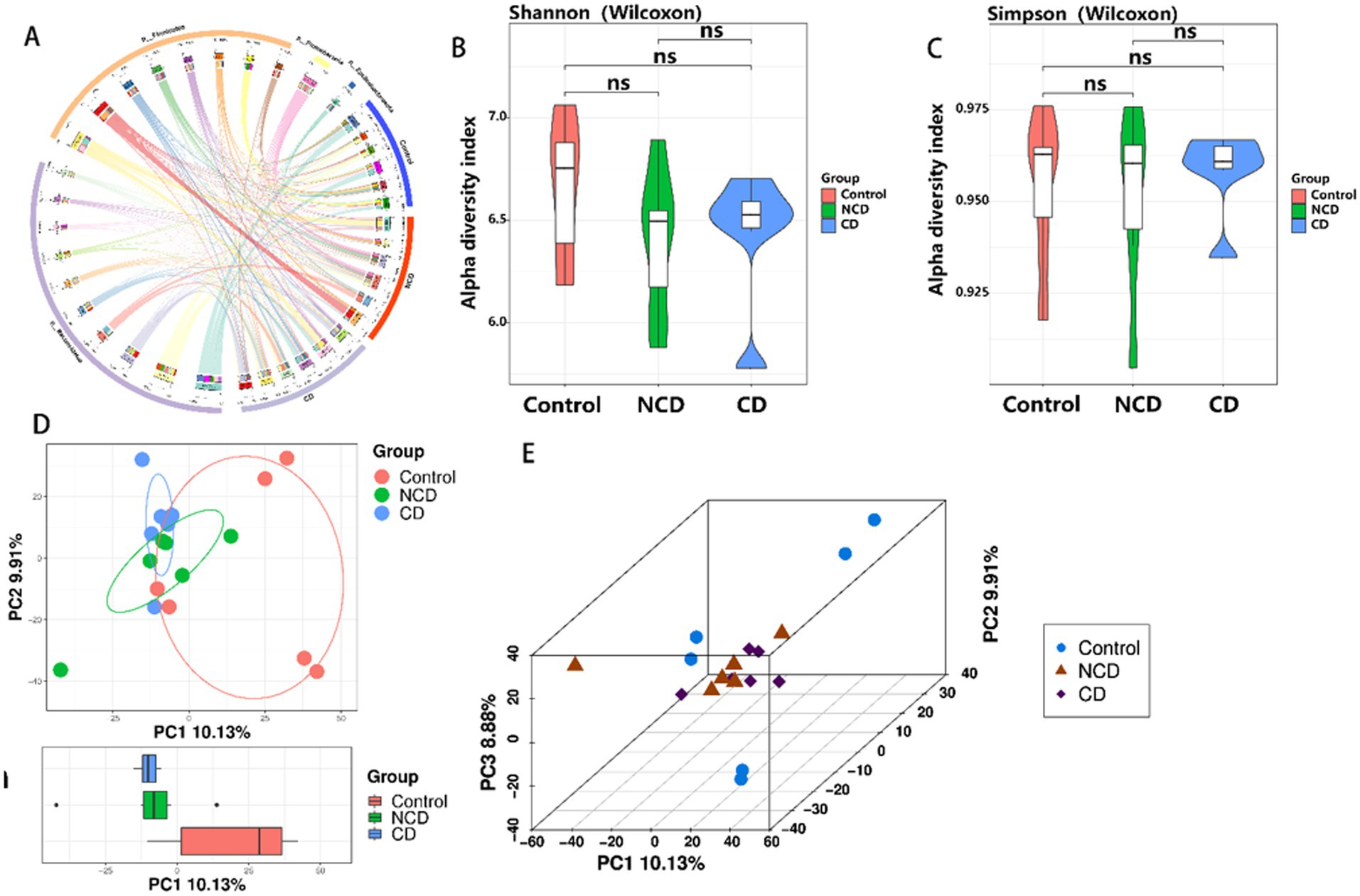
Figure 3. Altered community structure and diversity of the 3 groups of intestinal flora. (A) Circos plot shows the different community structures between the three groups. (B,C) Alpha-diversity Shannon and Simpson tree indices (p > 0.05). (D) Boxplot of PCA. (E) 3D plot of PCA. Data are presented as mean ± SEM. p < 0.05; ns, not significant.
Changes in intestinal flora richness in DPN rats
Adonis analysis was used to test whether there were significant differences between groups, and the results revealed significant differences in the intestinal flora species (p < 0.01) (Supplementary Table 1).
Identification of different gut flora types in the three groups by linear discriminant analysis effect size (LEfSe) analysis (Figure 4A) showed 55 distinguishing characteristics of relative abundance that varied considerably among the three groups. Next, we conducted statistical analyses at each phylum, order, family, genus, and species level. The analysis of fecal samples indicated that 70 bacteria were significantly altered in the three groups (phylum, 5; class, 6; order, 11; family, 14; genus, 23; species, 11). Finally, we selected the relative abundances of the top 10 genera that differed significantly to obtain the abundance of the dominant species within the group for comparison. Compared to the control, DPN rats illustrated significantly increased relative abundance of Escherichia-Shigella, Ruminococcaceae_UCG-010, Bifidobacterium, Parasutterella, Enterococcus, Anaeroplasma, Allobaculum, Turicibacter, Coprococcus_3, and Dubosiella (Figures 4B–D and Supplementary Figure 1); conversely, there was significantly lower relative abundance of Ruminiclostridium_6, Parabacteroides, Lachnospiraceae_MK4B4_group, Anaerotruncus, and Rikenella in DPN rats (Supplementary Figure 2). However, the abundance of Anaeroplasma, Turicibacter, and Coprococcus_3 was significantly higher in CD group than in NCD group (Supplementary Figure 3).
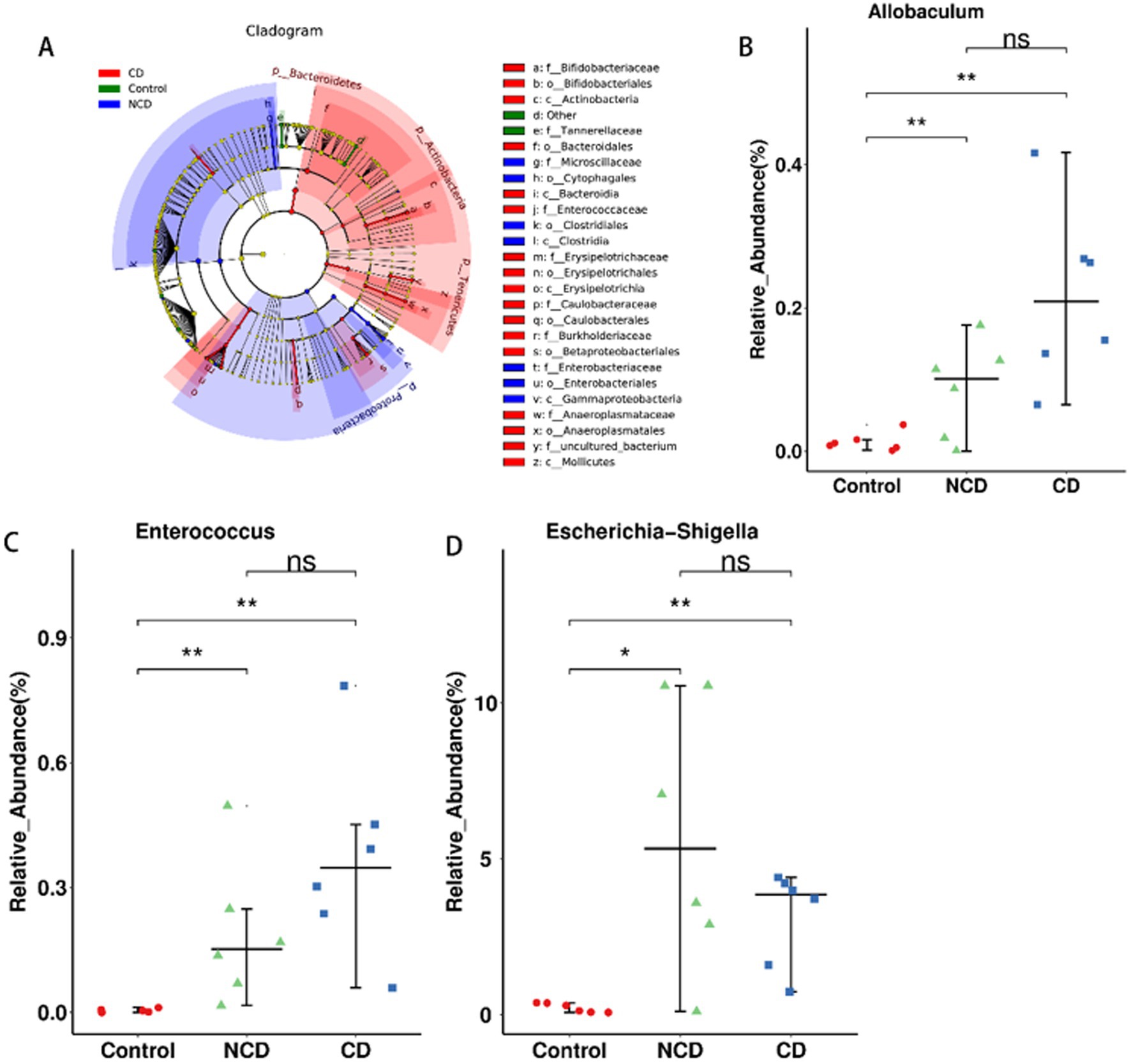
Figure 4. Differentially abundant bacterial taxa between the control and BDL mice. (A) LEfSe analysis among the three groups. LefSe, Linear discriminant analysis Effect Size. (B) Relative abundance of the phylum Allobaculum. (C) Relative abundance of the phylum Enterococcus. (D) Relative abundance of the phylum Escherichia-Shigella. Data are presented as the mean ± SEM. *p < 0.05, **p<0.01.
Abundance of differential flora in the CD group of rats
In the next step of the analysis, we compared the abundance of differential flora in rats in the CD and NCD groups. We performed LEfSe analysis for all Bacterial levels between the two groups (Figure 5A). At the species level, we observed that several groups of bacteria in the NCD group, including c. Clostridia, o. Clostridiales, p. Firmicutes, and f. Ruminococcaceae, were significantly higher based on the linear discriminant analysis (LDA) score [(log10) > 4.30]. Moreover, further analysis using Wilcoxon’s comparative test showed that the differences in the relative abundance of these four groups were statistically significant (p < 0.05) (Figures 5B,D and Supplementary Figure 4). In addition to Anaeroplasma, Turicibacter, and Coprococcus_3, the abundance of these three groups was significantly higher in CD group than in NCD group (Supplementary Figure 3), and p. Bacteroidetes, c. Bacteroidia, o. Bacteroidales, c. Mollicutes, p. Tenericutes, c. Coriobacteriia, and o. Coriobacteriales were the most abundant microbiota in CD group (LDA score (log10) > 2.18). Three microbiota types, p. Bacteroidetes, c. Bacteroidia, and o. Bacteroidales, were the most abundant (LDA score (log10) > 4.34) in CD group. Further analysis using Wilcoxon’s comparative test indicate that the differences in the relative abundance of these five groups were statistically significant (p < 0.05) (Figure 5C and Supplementary Figure 5). Further comparative analysis of the relative abundance of these nine microbiota types in the three groups using the Kruskal–Wallis algorithm confirmed that only the relative abundance of c. Clostridia and o. Clostridiales was significantly lower in the CD group (Supplementary Figure 6), whereas the relative abundances of p. Bacteroidetes, c. Bacteroidia, o. Bacteroidales, c. Mollicutes, and p. Tenericutes were significantly higher in CD group.
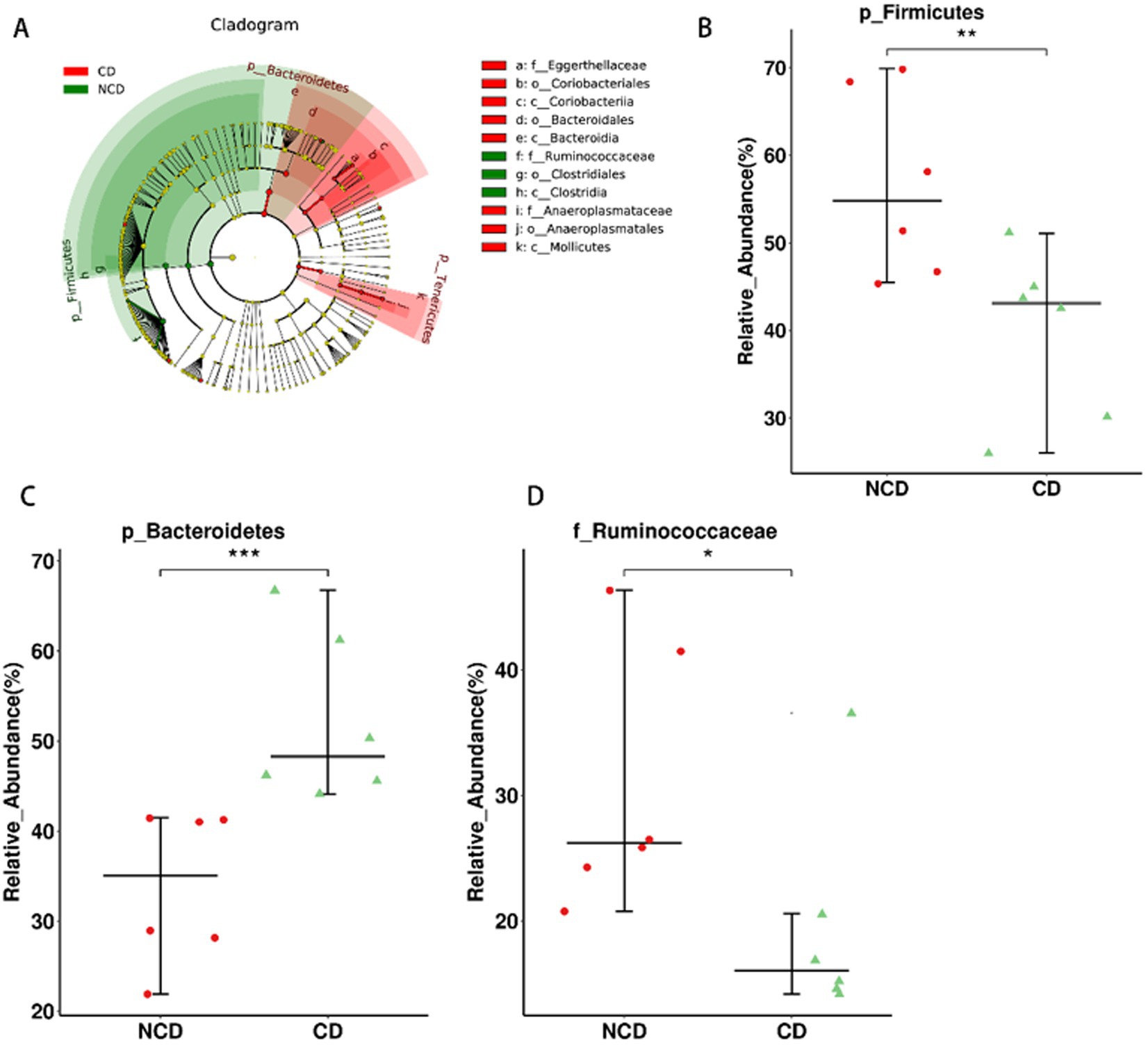
Figure 5. The relationship between dysbiosis markers and cognitive dysfunction. (A) Linear discriminant analysis effect size between the Group-CD and Group-NCD groups. (B–D) Relative abundance of gut microbiota at the species level. Data are presented as the mean ± SEM. *p < 0.05, **p < 0.01, ***p<0.001.
Discussion
The NORT is a valuable measure of cognition mainly used to assess learning and memory based on the differences in exploration time of novel and familiar objects (Lueptow, 2017). We demonstrated that rats in the model group had significantly reduced sciatic nerve conduction velocity 2 weeks after intraperitoneal injection of STZ, along with learning and memory deficits.
The gut microbiota plays an important role in promoting human health. Therefore, exploring the role and mechanisms of gut microbes in DPN combined with cognitive dysfunction is an emerging area of research. Microorganisms colonize the human gut and alter enteric nervous system function by regulating the metabolism and intestinal immune function (Ochoa-Reparaz and Kasper, 2016; Geijselaers et al., 2017; Iliodromiti et al., 2023). Intestinal flora imbalance can lead to the secretion of large amounts of amyloid, lipopolysaccharide (LPS), and inflammation-related molecules and disrupt the intestinal mucosal barrier, which contributes to the activation of microglia and triggers neuroinflammation in the CNS, which may lead to neurodegeneration (Cattaneo et al., 2017; Nguyen et al., 2023).
In this study, there was no significant difference in the alpha-diversity (composed of Shannon and Simpson indices) among the control rats, DPN rats with cognitive impairment, and DPN rats without cognitive impairment.
Previous studies have confirmed that the most predominant microbial taxa in this population are Firmicutes, Bacteroidetes, Aspergillus, Actinomycetes, and Clostridium. These bacteria are associated with diabetes, obesity (Jayapala and Lim, 2023), and AD (Patterson et al., 2015; Liu et al., 2020; Barta et al., 2023). A significant reduction in the abundance of thick-walled bacteria and actinomycetes at the phylum level was found in AD patients, in addition to an enrichment of allergic bacteria, which were highly correlated with biomarkers of AD pathology, suggesting that alterations in the AD microbiome may be associated with neuropathological changes in AD (Cenit et al., 2017). The proportion of Firmicutes was highest in samples from patients with type 2 diabetes alone (50.43%) and type 2 DPN (61.62%). However, studies have also reported that the abundance of thick-walled bacteria was reduced in the microbiome of patients with type 2 diabetes and AD (Cryan et al., 2020; Doumatey et al., 2020). In our study, the abundance of Firmicutes was also significantly reduced in rats in CD group. Firmicutes produce butyrate, a health-promoting molecule that is thought to increase insulin sensitivity and exert anti-inflammatory activity (Säemann et al., 2000; den Besten et al., 2013; Udayappan et al., 2016).
In our experiments, we determined that Ruminococcaceae, a member of Firmicutes, was also less abundant in CD group than in NCD group; both Ruminococcaceae and Clostridium are gut microbiota that are beneficial for cognitive function; Ruminococcaceae produces butyrate, inhibits histone deacetylase, attenuates the secretion of pro-inflammatory cytokines, and promotes cognitive function (Jiang et al., 2021).
In CD group, the relative abundance of Bacteroidetes was significantly higher. The phylum Bacteroidetes comprises a diverse and abundant group of Gram-negative commensal bacteria in the gut (Rajilić-Stojanović and de Vos, 2014). The main outer membrane component of Gram-negative bacteria is LPS, which stimulates a systemic inflammatory response upon transfer from the gut to the systemic circulation and releases pro-inflammatory cytokines. Dysregulation of the intestinal microecology can promote LPS entry into the systemic circulation by increasing intestinal permeability, leading to inflammation and metabolic dysfunction (Dheer et al., 2016). One study concluded that healthy mice injected directly with LPS developed memory deficits (Frühauf-Perez et al., 2018). The inflammatory response is a possible mechanism for the interaction between gut microbiota and the CNS.
Increased proportions of Bacteroides, Alistipes, Odoribacter, and Barnesiella have been observed in the microbiome of patients with AD (Angoorani et al., 2022). Recent studies have also revealed a reduction in alpha-diversity and an increase in the thick-walled Bacteries/Bacteroides phylum ratio (an important indicator of gut microbiota health) in AD model mice (Qiao et al., 2022).
An increase in harmful microorganisms, such as Turicibacter and Bacteroides, is implicated in the development of ulcerative colitis (Li et al., 2021; Zhang Y. et al., 2021). The abundance of Turicibacter was positively correlated with the levels of pro-inflammatory cytokines such as interleukin (IL)-6, IL-1β, tumor necrosis factor-alpha (TNF-α), and interferon-gamma (Liang et al., 2019; Dong et al., 2022). By examining the effects of gut microbes on chemotherapy-induced inflammatory and behavioral side effects in female mice, it was shown that the relative abundance of Turicibacter spp. was associated with circulating inflammation (IL-1β) and behavioral outcomes (lethargy and anxiety-like behavior). In the absence of antibiotics, a relative increase in Turicibacter in the distal colon of chemotherapy-treated mice was associated with reduced motility (Grant et al., 2021). In contrast, fecal microbiota transplantation (FMT) protects against intestinal epithelial barrier disruption induced by dextran sodium sulfate by downregulating the relative abundance of Turicibacter (Wen et al., 2021). These findings suggest a complex role of Turicibacter in chronic inflammatory environment. Recent studies have proposed that Turicibacter can induce the production of intestinal-derived 5-hydroxytryptamine (Dunham et al., 2022), an important neurotransmitter in the PNS and CNS. Its abnormalities in the CNS may be associated with anorexia, stress, psychiatric classification, epilepsy, and dementia. Therefore, abnormalities in relation to Turicibacter may play a role in DPN-induced cognitive dysfunction.
We also established that the abundance of actinomycetes in the intestinal flora of CD group was relatively increased, mainly from Coriobacteriia and Coriobacteriales. Most bacteria belong to the class Coriobacteriia anaerobic bacteria, which often exist in the intestinal flora and are pathogenic (Hein et al., 1859). Coriobacteriales were a minority in normal mice, accounting for approximately 1% of the detected microorganisms. However, the low proportion of bacteria in this gut microbial ecosystem may be extremely important for host metabolism and should not be overlooked in future research.
Coriobacteriales, which are strongly associated with energy production or obesity, are abundant in obese people and obese mice and in mice consuming a “Western diet” or high-fat diet (Goodman et al., 2011). In a high-fat diet mouse model, it was revealed that the balance of Bifidobacteria/Coriobacteriales has an important effect on lipid metabolism in mice, and Bifidobacteria may indirectly affect lipid metabolism by inhibiting the number of Coriobacteriales (Martínez et al., 2009). Claus et al. reported the strongest correlation between red stink bugs and liver triglycerides among Actinomycetes. Furthermore, they found two correlations by calculating the correlation of bacteria in operational taxonomic unit (OTUs) of liver metabolic profiles, with the strongest OTUs belonging to the Coriobacteriales family, confirming a link between Coriobacteriales and liver triglycerides, glycogen, and glucose (Claus et al., 2011).
An analysis of the gut microbiota of mice exposed to the animal model of depression revealed that Coriobacteriia is associated with depressive symptoms in depressive mice. After ketamine treatment, 30 bacteria changed significantly, and the receiver operating characteristic curve showed that Actinobacteria and Coriobacteriia might be potential biomarkers of ketamine’s antidepressant efficacy (Huang et al., 2019).
Sleep quality is positively correlated with cognitive function, and sleep disturbance can lead to cognitive impairment. According to previous studies on sleep, Coriobacteriia is negatively correlated with total sleep time and sleep efficiency (Zhang R. et al., 2021).
In summary, our findings suggest that gut microbial disorders with persistently elevated abundance of the phyla Mycobacterium, Turicibacter, and Actinobacteria may be a causal factor in DPN combined with cognitive dysfunction. However, a limitation of this study is that we only performed correlation analysis; thus, it is difficult to determine the causal relationship between gut microbes and cognitive impairment, and the specific mechanisms that induce production need further investigation.
Data availability statement
The datasets presented in this study can be found in online repositories. The names of the repository/repositories and accession number(s) can be found at: NCBI – BioProjectPRJNA937393.
Ethics statement
The animal study was reviewed and approved by the Animal Ethics Committee of Laboratory Animal Center, Guangdong Academy of Chinese Medicine.
Author contributions
QH and GZ contributed to conception and design of the study. ZL and WH organized the database. ZL and AS performed the statistical analysis. WH and JD wrote the first draft of the manuscript. HX and AM wrote the sections of the manuscript. All authors contributed to manuscript revision, read, and approved the submitted version.
Conflict of interest
The authors declare that the research was conducted in the absence of any commercial or financial relationships that could be construed as a potential conflict of interest.
Publisher’s note
All claims expressed in this article are solely those of the authors and do not necessarily represent those of their affiliated organizations, or those of the publisher, the editors and the reviewers. Any product that may be evaluated in this article, or claim that may be made by its manufacturer, is not guaranteed or endorsed by the publisher.
Supplementary material
The Supplementary material for this article can be found online at: https://www.frontiersin.org/articles/10.3389/fmicb.2023.1156591/full#supplementary-material
References
Angoorani, P., Ejtahed, H. S., Siadat, S. D., Sharifi, F., and Larijani, B. (2022). Is there any link between cognitive impairment and gut microbiota? A systematic review. Gerontology 68, 1201–1213. doi: 10.1159/000522381
Barta, B. P., Onhausz, B., Al Doghmi, A., Szalai, Z., Balázs, J., Bagyánszki, M., et al. (2023). Gut region-specific TNFR expression: TNFR2 is more affected than TNFR1 in duodenal myenteric ganglia of diabetic rats. World J. Diabetes 14, 48–61. doi: 10.4239/wjd.v14.i1.48
Cattaneo, A., Cattane, N., Galluzzi, S., Provasi, S., Lopizzo, N., Festari, C., et al. (2017). Association of brain amyloidosis with pro-inflammatory gut bacterial taxa and peripheral inflammation markers in cognitively impaired elderly. Neurobiol. Aging 49, 60–68. doi: 10.1016/j.neurobiolaging.2016.08.019
Cenit, M. C., Sanz, Y., and Codoñer-Franch, P. (2017). Influence of gut microbiota on neuropsychiatric disorders. World J. Gastroenterol. 23, 5486–5498. doi: 10.3748/wjg.v23.i30.5486
Claus, S. P., Ellero, S. L., Berger, B., Krause, L., Bruttin, A., Molina, J., et al. (2011). Colonization-induced host-gut microbial metabolic interaction. mBio 2:e00271-10. doi: 10.1128/mBio.00271-10
Cryan, J. F., O'Riordan, K. J., Sandhu, K., Peterson, V., and Dinan, T. G. (2020). The gut microbiome in neurological disorders. Lancet Neurol. 19, 179–194. doi: 10.1016/S1474-4422(19)30356-4
den Besten, G., van Eunen, K., Groen, A. K., Venema, K., Reijngoud, D. J., and Bakker, B. M. (2013). The role of short-chain fatty acids in the interplay between diet, gut microbiota, and host energy metabolism. J. Lipid Res. 54, 2325–2340. doi: 10.1194/jlr.R036012
Dheer, R., Santaolalla, R., Davies, J. M., Lang, J. K., Phillips, M. C., Pastorini, C., et al. (2016). Intestinal epithelial toll-like receptor 4 signaling affects epithelial function and colonic microbiota and promotes a risk for transmissible colitis. Infect. Immun. 84, 798–810. doi: 10.1128/IAI.01374-15
Dong, L., Du, H., Zhang, M., Xu, H., Pu, X., Chen, Q., et al. (2022). Anti-inflammatory effect of Rhein on ulcerative colitis via inhibiting PI3K/Akt/mTOR signaling pathway and regulating gut microbiota. Phytother. Res. 36, 2081–2094. doi: 10.1002/ptr.7429
Doumatey, A. P., Adeyemo, A., Zhou, J., Lei, L., Adebamowo, S. N., Adebamowo, C., et al. (2020). Gut microbiome profiles are associated with type 2 diabetes in urban Africans. Front. Cell. Infect. Microbiol. 10:63. doi: 10.3389/fcimb.2020.00063
Dunham, S. J. B., McNair, K. A., Adams, E. D., Avelar-Barragan, J., Forner, S., Mapstone, M., et al. (2022). Longitudinal analysis of the microbiome and metabolome in the 5xfAD mouse model of Alzheimer’s disease. mBio 13:e0179422. doi: 10.1128/mbio.01794-22
Frühauf-Perez, P. K., Temp, F. R., Pillat, M. M., Signor, C., Wendel, A. L., Ulrich, H., et al. (2018). Spermine protects from LPS-induced memory deficit via BDNF and TrkB activation. Neurobiol. Learn. Mem. 149, 135–143. doi: 10.1016/j.nlm.2018.02.012
Gao, X., Huynh, B. T., Guillemot, D., Glaser, P., and Opatowski, L. (2018). Inference of significant microbial interactions from longitudinal metagenomics data. Front. Microbiol. 9:2319. doi: 10.3389/fmicb.2018.02319
Gareau, M. G. (2014). Microbiota-gut-brain axis and cognitive function. Adv. Exp. Med. Biol. 817, 357–371. doi: 10.1007/978-1-4939-0897-4_16
Geijselaers, S. L. C., Sep, S. J. S., Claessens, D., Schram, M. T., van Boxtel, M. P. J., Henry, R. M. A., et al. (2017). The role of hyperglycemia, insulin resistance, and blood pressure in diabetes-associated differences in cognitive performance-the Maastricht study. Diabetes Care 40, 1537–1547. doi: 10.2337/dc17-0330
Goodman, A. L., Kallstrom, G., Faith, J. J., Reyes, A., Moore, A., Dantas, G., et al. (2011). Extensive personal human gut microbiota culture collections characterized and manipulated in gnotobiotic mice. Proc. Natl. Acad. Sci. U. S. A. 108, 6252–6257. doi: 10.1073/pnas.1102938108
Grant, C. V., Loman, B. R., Bailey, M. T., and Pyter, L. M. (2021). Manipulations of the gut microbiome alter chemotherapy-induced inflammation and behavioral side effects in female mice. Brain Behav. Immun. 95, 401–412. doi: 10.1016/j.bbi.2021.04.014
Groeneveld, O., Reijmer, Y., Heinen, R., Kuijf, H., Koekkoek, P., Janssen, J., et al. (2018). Brain imaging correlates of mild cognitive impairment and early dementia in patients with type 2 diabetes mellitus. Nutr. Metab. Cardiovasc. Dis. 28, 1253–1260. doi: 10.1016/j.numecd.2018.07.008
He, Z., Liu, Y., Li, Z., Sun, T., Li, Z., Manyande, A., et al. (2023). Gut microbiota regulates circadian oscillation in hepatic ischemia–reperfusion injury-induced cognitive impairment by interfering with hippocampal lipid metabolism in mice. Hepatol. Int. 16. doi: 10.1007/s12072-023-10509-w
Hein, S., von Irmer, J., Gallei, M., Meusinger, R., and Simon, J. (1859). Two dedicated class C radical S-adenosylmethionine methyltransferases concertedly catalyse the synthesis of 7,8-dimethylmenaquinone. Biochim. Biophys. Acta Bioenerg. 1859, 300–308. doi: 10.1016/j.bbabio.2018.01.010
Huang, N., Hua, D., Zhan, G., Li, S., Zhu, B., Jiang, R., et al. (2019). Role of Actinobacteria and Coriobacteriia in the antidepressant effects of ketamine in an inflammation model of depression. Pharmacol. Biochem. Behav. 176, 93–100. doi: 10.1016/j.pbb.2018.12.001
Iannone, L. F., Preda, A., Blottière, H. M., Clarke, G., Albani, D., Belcastro, V., et al. (2019). Microbiota-gut brain axis involvement in neuropsychiatric disorders. Expert. Rev. Neurother. 19, 1037–1050. doi: 10.1080/14737175.2019.1638763
Iliodromiti, Z., Triantafyllou, A. R., Tsaousi, M., Pouliakis, A., Petropoulou, C., Sokou, R., et al. (2023). Gut microbiome and neurodevelopmental disorders: a link yet to be disclosed. Microorganisms 11:487. doi: 10.3390/microorganisms11020487
Jayapala, H. P. S., and Lim, S. Y. (2023). N-3 polyunsaturated fatty acids and gut microbiota. Comb. Chem. High Throughput Screen. 26, 892–905. doi: 10.2174/1386207325666220701121025
Jiang, Y., Li, K., Li, X., Xu, L., and Yang, Z. (2021). Sodium butyrate ameliorates the impairment of synaptic plasticity by inhibiting the neuroinflammation in 5XFAD mice. Chem. Biol. Interact. 341:109452. doi: 10.1016/j.cbi.2021.109452
Lee, M. C., and Tracey, I. (2013). Imaging pain: a potent means for investigating pain mechanisms in patients. Br. J. Anaesth. 111, 64–72. doi: 10.1093/bja/aet174
Li, Q., Cui, Y., Xu, B., Wang, Y., Lv, F., Li, Z., et al. (2021). Main active components of Jiawei Gegen Qinlian decoction protects against ulcerative colitis under different dietary environments in a gut microbiota-dependent manner. Pharmacol. Res. 170:105694. doi: 10.1016/j.phrs.2021.105694
Li, Z., Sun, T., He, Z., Li, Z., Zhang, W., Wang, J., et al. (2022). SCFAs ameliorate chronic postsurgical pain-related cognition dysfunction via the ACSS2-HDAC2 Axis in rats. Mol. Neurobiol. 59, 6211–6227. doi: 10.1007/s12035-022-02971-8
Liang, Y. N., Yu, J. G., Zhang, D. B., Zhang, Z., Ren, L. L., Li, L. H., et al. (2019). Indigo naturalis ameliorates dextran sulfate sodium-induced colitis in mice by modulating the intestinal microbiota community. Molecules 24:4086. doi: 10.3390/molecules24224086
Liu, T., Li, Z., He, J., Yang, N., Han, D., Li, Y., et al. (2020). Regional metabolic patterns of abnormal postoperative behavioral performance in aged mice assessed by H-1-NMR dynamic mapping method. Neurosci. Bull. 36, 25–38. doi: 10.1007/s12264-019-00414-4
Liu, Y., Li, Z., Sun, T., He, Z., Xiang, H., and Xiong, J. (2022). Gut microbiota-generated short-chain fatty acids are involved in Para-chlorophenylalanine-induced cognitive disorders. Front. Microbiol. 13:1028913. doi: 10.3389/fmicb.2022.1028913
Lueptow, L. M. (2017). Novel object recognition test for the investigation of learning and memory in mice. J. Vis. Exp. e55718. doi: 10.3791/55718
MahmoudianDehkordi, S., Arnold, M., Nho, K., Ahmad, S., Jia, W., Xie, G., et al. (2019). Altered bile acid profile associates with cognitive impairment in Alzheimer's disease-an emerging role for gut microbiome. Alzheimers Dement. 15, 76–92. doi: 10.1016/j.jalz.2018.07.217
Martínez, I., Wallace, G., Zhang, C., Legge, R., Benson, A. K., Carr, T. P., et al. (2009). Diet-induced metabolic improvements in a hamster model of hypercholesterolemia are strongly linked to alterations of the gut microbiota. Appl. Environ. Microbiol. 75, 4175–4184. doi: 10.1128/AEM.00380-09
Nguyen, N. M., Cho, J., and Lee, C. (2023). Gut microbiota and Alzheimer's disease: how to study and apply their relationship. Int. J. Mol. Sci. 24:4047. doi: 10.3390/ijms24044047
Nossa, C. W., Oberdorf, W. E., Yang, L., Aas, J. A., Paster, B. J., Desantis, T. Z., et al. (2010). Design of 16S rRNA gene primers for 454 pyrosequencing of the human foregut microbiome. World J. Gastroenterol. 16, 4135–4144. doi: 10.3748/wjg.v16.i33.4135
Ochoa-Reparaz, J., and Kasper, L. H. (2016). The second brain: is the gut microbiota a link between obesity and central nervous system disorders? Curr. Obes. Rep. 5, 51–64. doi: 10.1007/s13679-016-0191-1
Olson, C. A., Vuong, H. E., Yano, J. M., Liang, Q. Y., Nusbaum, D. J., and Hsiao, E. Y. (2018). The gut microbiota mediates the anti-seizure effects of the ketogenic diet. Cells 173, 1728–1741.e13. doi: 10.1016/j.cell.2018.04.027
Patterson, E., Marques, T. M., O'Sullivan, O., Fitzgerald, P., Fitzgerald, G. F., Cotter, P. D., et al. (2015). Streptozotocin-induced type-1-diabetes disease onset in Sprague-Dawley rats is associated with an altered intestinal microbiota composition and decreased diversity. Microbiology 161, 182–193. doi: 10.1099/mic.0.082610-0
Qiao, L., Chen, Y., Song, X., Dou, X., and Xu, C. (2022). Selenium nanoparticles-enriched lactobacillus casei ATCC 393 prevents cognitive dysfunction in mice through modulating microbiota-gut-brain Axis. Int. J. Nanomedicine 17, 4807–4827. doi: 10.2147/IJN.S374024
Radulescu, C. I., Garcia-Miralles, M., Sidik, H., Bardile, C. F., Yusof, N., Lee, H. U., et al. (2019). Manipulation of microbiota reveals altered callosal myelination and white matter plasticity in a model of Huntington disease. Neurobiol. Dis. 127, 65–75. doi: 10.1016/j.nbd.2019.02.011
Rajilić-Stojanović, M., and de Vos, W. M. (2014). The first 1000 cultured species of the human gastrointestinal microbiota. FEMS Microbiol. Rev. 38, 996–1047. doi: 10.1111/1574-6976.12075
Rogers, G. B., Keating, D. J., Young, R. L., Wong, M. L., Licinio, J., and Wesselingh, S. (2016). From gut dysbiosis to altered brain function and mental illness: mechanisms and pathways. Mol. Psychiatry 21, 738–748. doi: 10.1038/mp.2016.50
Säemann, M. D., Böhmig, G. A., Osterreicher, C. H., Burtscher, H., Parolini, O., Diakos, C., et al. (2000). Anti-inflammatory effects of sodium butyrate on human monocytes: potent inhibition of IL-12 and up-regulation of IL-10 production. FASEB J. 14, 2380–2382. doi: 10.1096/fj.00-0359fje
Salguero, M. V., Al-Obaide, M. A. I., Singh, R., Siepmann, T., and Vasylyeva, T. L. (2019). Dysbiosis of gram-negative gut microbiota and the associated serum lipopolysaccharide exacerbates inflammation in type 2 diabetic patients with chronic kidney disease. Exp. Ther. Med. 18, 3461–3469. doi: 10.3892/etm.2019.7943
Sampson, T. R., Debelius, J. W., Thron, T., Janssen, S., Shastri, G. G., Ilhan, Z. E., et al. (2016). Gut microbiota regulate motor deficits and neuroinflammation in a model of Parkinson’s disease. Cells 167, 1469–1480.e12. doi: 10.1016/j.cell.2016.11.018
Segata, N., Izard, J., Waldron, L., Gevers, D., Miropolsky, L., Garrett, W. S., et al. (2011). Metagenomic biomarker discovery and explanation. Genome Biol. 12:R60. doi: 10.1186/gb-2011-12-6-r60
Selvarajah, D., Wilkinson, I. D., Davies, J., Gandhi, R., and Tesfaye, S. (2011). Central nervous system involvement in diabetic neuropathy. Curr. Diab. Rep. 11, 310–322. doi: 10.1007/s11892-011-0205-z
Sharma, M., Chan, H. K., Lavilla, C. A. Jr., Uy, M. M., Froemming, G. R. A., and Okechukwu, P. N. (2023). Induction of a single-dose of Streptozotocin (50 mg) in rat model causes insulin resistance with type 2 diabetes mellitus. Fundam. Clin. Pharmacol. doi: 10.1111/fcp.12892
Sun, T., Du, H., Li, Z., Xiong, J., Liu, Y., Li, Y., et al. (2022). Decoding the contributions of gut microbiota and cerebral metabolism in acute liver injury mice with and without cognitive dysfunction. CNS Neurosci. Ther. doi: 10.1111/cns.14069
Tarragon, E., Lopez, D., Estrada, C., Gonzalez-Cuello, A., Ros, C. M., Lamberty, Y., et al. (2014). Memantine prevents reference and working memory impairment caused by sleep deprivation in both young and aged Octodon degus. Neuropharmacology 85, 206–214. doi: 10.1016/j.neuropharm.2014.05.023
Udayappan, S., Manneras-Holm, L., Chaplin-Scott, A., Belzer, C., Herrema, H., Dallinga-Thie, G. M., et al. (2016). Oral treatment with Eubacterium hallii improves insulin sensitivity in db/db mice. NPJ Biofilms Microbiomes 2:16009. doi: 10.1038/npjbiofilms.2016.9
Wen, X., Wang, H. G., Zhang, M. N., Zhang, M. H., Wang, H., and Yang, X. Z. (2021). Fecal microbiota transplantation ameliorates experimental colitis via gut microbiota and T-cell modulation. World J. Gastroenterol. 27, 2834–2849. doi: 10.3748/wjg.v27.i21.2834
Wilkinson, I. D., Selvarajah, D., Greig, M., Shillo, P., Boland, E., Gandhi, R., et al. (2013). Magnetic resonance imaging of the central nervous system in diabetic neuropathy. Curr. Diab. Rep. 13, 509–516. doi: 10.1007/s11892-013-0394-8
Yang, B., Sun, T., Chen, Y., Xiang, H., Xiong, J., and Bao, S. (2022). The role of gut microbiota in mice with bile duct ligation-evoked Cholestatic liver disease-related cognitive dysfunction. Front. Microbiol. 13:909461. doi: 10.3389/fmicb.2022.909461
Zhang, R., Gao, S., Wang, S., Zhang, J., Bai, Y., He, S., et al. (2021). Gut microbiota in patients with type 1 narcolepsy. Nature Sci. Sleep 13, 2007–2018. doi: 10.2147/NSS.S330022
Zhang, Y., Jiang, D., Jin, Y., Jia, H., Yang, Y., Kim, I. H., et al. (2021). Glycine attenuates Citrobacter rodentium-induced colitis by regulating ATF6-mediated endoplasmic reticulum stress in mice. Mol. Nutr. Food Res. 65:e2001065. doi: 10.1002/mnfr.202001065
Zhang, L., Wang, Y., Xiayu, X., Shi, C., Chen, W., Song, N., et al. (2017). Altered gut microbiota in a mouse model of Alzheimer’s disease. J. Alzheimer’s Dis. 60, 1241–1257. doi: 10.3233/JAD-170020
Zheng, P., Zeng, B., Liu, M., Chen, J., Pan, J., Han, Y., et al. (2019). The gut microbiome from patients with schizophrenia modulates the glutamate-glutamine-GABA cycle and schizophrenia-relevant behaviors in mice. Sci. Adv. 5:eaau8317. doi: 10.1126/sciadv.aau8317
Zheng, P., Zeng, B., Zhou, C., Liu, M., Fang, Z., Xu, X., et al. (2016). Gut microbiome remodeling induces depressive-like behaviors through a pathway mediated by the host's metabolism. Mol. Psychiatry 21, 786–796. doi: 10.1038/mp.2016.44
Keywords: diabetic peripheral neuropathy, cognitive function, gut microbiota, 16S rRNA sequencing, rat
Citation: Huang W, Lin Z, Sun A, Deng J, Manyande A, Xiang H, Zhao GF and Hong Q (2023) The role of gut microbiota in diabetic peripheral neuropathy rats with cognitive dysfunction. Front. Microbiol. 14:1156591. doi: 10.3389/fmicb.2023.1156591
Edited by:
Lin Shi, Shaanxi Normal University, ChinaReviewed by:
Kiran Veer Sandhu, University College Cork, IrelandSidharth Prasad Mishra, University of South Florida, United States
Copyright © 2023 Huang, Lin, Sun, Deng, Manyande, Xiang, Zhao and Hong. This is an open-access article distributed under the terms of the Creative Commons Attribution License (CC BY). The use, distribution or reproduction in other forums is permitted, provided the original author(s) and the copyright owner(s) are credited and that the original publication in this journal is cited, in accordance with accepted academic practice. No use, distribution or reproduction is permitted which does not comply with these terms.
*Correspondence: Gao Feng Zhao, emhhb2dhb2ZlbmdfOTdAMTYzLmNvbQ==; Qingxiong Hong, aG9uZ3Fpbmd4aW9uZ0BnenVjbS5lZHUuY24=
†These authors have contributed equally to this work