- 1Key Laboratory of Southwest China Wildlife Resources Conservation (Ministry of Education), China West Normal University, Nanchong, China
- 2School of Electrical Information Engineering, Chengdu Textile College, Chengdu, China
Yunnan snub-nosed monkeys (Rhinopithecus bieti) are the highest elevation lived non-human primate, and their survival has been threatened for decades. To promote their population growth, a reserve provides a typical monkey population with supplemental food. However, the influences of this food provisioning on their gut microbiota and antibiotic resistance genes (ARGs) were unknown. Therefore, we investigated the gut microbiota and ARGs of the food-provisioned monkey population compared with another wild foraging population. We found that food provisioning significantly increased the gut microbiota diversity and changed the community composition, particularly increased both the Firmicutes abundance and Firmicutes/Bacteroidetes ratio. Meanwhile, the food provisioning decreased the complex and stable gut microbiota network. KEGG functions were also influenced by food provisioning, with wild foraging monkeys showing higher functions of metabolism and genetic information processing, especially the carbohydrate metabolism, while food-provisioned monkeys exhibited increased environmental information processing, cellular processes, and organismal systems, including valine, leucine, and isoleucine degradation. In addition, food provisioning increased the abundance of ARGs in the gut microbiota, with most increasing the abundance of bacA gene and changing the correlations between specific ARGs and bacterial phyla in each population. Our study highlights that even food provisioning could promote wildlife nutrient intake, and it is necessary to pay attention to the increased ARGs and potential effects on gut microbiota stability and functions for this human conservation measure.
Introduction
Gut microbiota play an important role in food digestion, absorption, and metabolism of the host, as well as in building the gut barrier and defense system (Lee and Hase, 2014; Zheng et al., 2020). They help maintain the normal functions of the gut and the health of the host (Jandhyala et al., 2015; Rosshart et al., 2017). In this relationship, the host provides a nutrient-rich and stable environment for the gut microbiota (Tsuji et al., 2008) and can influence the composition and functions of gut microbiota through various factors, such as diet, phylogeny, disease, age, and lifestyle (Gerritsen et al., 2011). Among these factors, diet, including its diversity and nutrient intake, is the most important one (Tomova et al., 2019; Cui et al., 2022).
Kilburn et al. (2020) found that a high-fat, high-protein diet increases the abundance of Bacteroidetes, and Wu et al. (2011) showed that a high-carbohydrate, high-fiber diet increases the abundance of Prevotella. In addition, previous studies have confirmed that diet can influence host health and resistance to disease by influencing gut microbiota (Makki et al., 2018; Catalkaya et al., 2020). All of these studies mainly related to human and rodents, while studies on non-human primates are relatively rare (Nagpal et al., 2018; Cui et al., 2022; Yang et al., 2023). This is important because non-human primates are the closest phylogenetic relatives of humans compared with other animals, making them valuable for understanding microbiota–host interactions and human coevolution (Ley et al., 2008; Yang et al., 2022). Therefore, it is necessary to investigate the impact of diet on the gut microbiota of non-human primates.
The Yunnan snub-nosed monkey (Rhinopithecus bieti) is a rare and endemic endangered species in China. It is the highest-distributed non-human primate and lives in the high-latitude forests (3,000–4,400 m) of northwestern Yunnan Province and southeastern Tibet Autonomous Region of China (Xia et al., 2020). Approximately 40 years ago, R. bieti was more endangered due to the threat of habitat reduction and fragmentation and hunting (Liu et al., 2009). With the establishment of the Yunnan Baima Snow Mountain National Nature Reserve, the population of R. bieti recovered and reached approximately 3,800 individuals (Zhao et al., 2019). To further increase the population and address the food shortage in the wild, the reserve has supplied artificial foods to one representative natural population of R. bieti. This supplementary food consists of native lichens, carrots, peanuts, apples, eggs, or pumpkin seeds (Zhu et al., 2016). Artificial food supply contributes greatly to the population expansion of R. bieti, but whether there is a negative effect on the health is unknown, especially for the gut microbiota.
Previous studies mainly explored the effect of diet on gut microbiota community composition and diversity, but they have widely ignored the impact on the gut microbiota network (Li et al., 2016; Huang et al., 2021). Microbiota network plays a critical role in shaping the overall functionality of the gut community (Weiss et al., 2016) because the positive and negative correlations within the network reflect interdependence and competitive exclusion, respectively (Wagg et al., 2019). Therefore, confirming the influence of food provisioning on gut microbiota network can help to understand its impact on gut microbiota.
Antibiotic resistance genes (ARGs) can be transmitted horizontally through mobile genetic elements or the food chain in the environment (Ramsamy et al., 2022). Their enrichment within gut microbiota can enhance the antibiotic resistance of pathogenic microbiota, leading to significant difficulty in potential treatment of infections (Allen et al., 2010). In food provisioning, the increased frequency of contact with humans and artificial food may facilitate the transfer of ARGs to gut microbiota (Allen et al., 2010; Hu et al., 2017). However, this potential effect on the abundance and diversity of ARGs has yet to be confirmed.
In this study, we compared two populations of R. bieti living in the Yunnan Baima Snow Mountain National Nature Reserve in China. One population foraged naturally, while the other received supplemental food. Both populations lived under similar environmental conditions. The aim of our study was to answer two questions: (1) How does food provisioning affect the gut microbiota community of R. bieti, especially the microbiota network? (2) How does the ARGs in the gut change due to food provisioning? Our results will help to evaluate the potential biological risks associated with food provisioning from the perspective of the gut microbiota community and ARGs and provide scientific support for the future conservation of the wild R. bieti population.
Materials and methods
Study area and food provisioning method
The Baima Snow Mountain National Nature Reserve (98°57′-99°25 E, 27°24′-28°36′ N) is located in Weixi County, Yunnan Province, China. The reserve has an annual average daily temperature of 9.4°C, ranging from a minimum of 2.7°C in January to a maximum of 16.2°C in June. The annual rainfall of the reserve is 1,371 mm, with 70% concentrated between June and October (Li et al., 2023). The typical vegetation is coniferous forest, deciduous broad-leaved forest (2,500–3,600 m), and subalpine fir forest (Zhu et al., 2016). The diet of wild R. bieti living in the reserve includes lichens, mature leaves, fruit seeds, young leaves, bamboo shoots, buds, flowers, insects, and fungi (Xia et al., 2022). The wild foraging (WF) population is located in Anyi (99°09′ E, 27°27′ N), and the food-provisioned (FP) population is located in Xianguqing (99°21′ E, 27°39′ N). The FP population consisted of approximately 80 individuals and has been provisioned food since 2009. Every day at 9:00 a.m. and 17:00 p.m., this population receives 10 kg of lichens and 4 kg of other food, including peanuts, apples, pumpkin seeds, and eggs. This supplementary food makes up approximately 40% of FP population daily diet, while the bamboo shoots make up 32% of WF population daily diet, which is significantly higher than that of the FP population (Xia et al., 2022).
Fecal sample collection
Between March and June, reserve forest rangers used telescopes to locate and observe the monkeys from a distance of approximately 200 meters. Once an individual monkey defecated and moved away, fecal sample was collected. Any adhering plant litter or soil was removed with sterilized tweezers. The collected fecal samples were immediately stored in liquid nitrogen and transported to the laboratory for storage in a −80°C freezer. In total, we collected 92 fecal samples, and 46 samples were collected for each population.
Enzyme activities and nutrients
Freeze-dried fecal samples (0.1 g) were mixed with 1 mL of ice-cold phosphate buffer (pH 7.5). The mixture was centrifuged at 12,000 r/min for 20 min, and the supernatant was used for enzyme activity and nutrient measurements. Protease (EC 3.4.21) activity was measured using the Folin-phenol method (McDonald and Chen, 1965); amylase (EC 3.2.1.1) activity was measured using the starch-iodine method (Xiao et al., 2006); lipase (EC 3.1.1.3) activity was measured using the polyvinyl alcohol olive oil emulsion hydrolysis method (Andersson, 1980); cellulase (EC 3.2.14) activity was measured using the 3,5-dinitrosalicylic acid method (Breuil and Saddler, 1985). The protein content was measured using the Coomassie brilliant blue dual-wavelength method (Sedmak and Grossberg, 1977); the glucose content was measured using the 3,5-dinitrosalicylic acid method (Borji et al., 2017). A suitable amount of freeze-dried feces was mixed with five times of its weight of sterile water, and then it was used to measure the pH.
DNA extraction and 16S rRNA sequencing
Total DNA was extracted from 0.25 g fecal samples using the E.Z.N.A.® soil DNA kit (Omega Bio-tek, Norcross, GA, USA), and the extracted DNA was then checked using 1% agarose gel and NanoDrop One Microvolume UV–VIS Spectrophotometer (Thermo Fisher Scientific, MA, USA). PCR amplifications were performed in triplicate to amplify the V3-V4 hypervariable region of the 16S rRNA gene using the primer pair 338F and 806R (Liu et al., 2016). The PCR products were examined and subsequently subjected to high throughput sequencing using Illumina Miseq PE300 platform by a commercial facility (Shanghai Majorbio Bio-Pharm Technology Corporation, Shanghai, China).
The paired-end reads were quality-filtered and spliced using fastp v.0.20.0 (Chen et al., 2018) and FLASH v.1.2.11 (Magoč and Salzberg, 2011). The merged reads were then analyzed on QIIME2 v.2021.4 platform (Bolyen et al., 2019). DADA2 was employed to truncate the reads at 400 bp and perform quality control, read assembly, dereplication, chimera removal, and generation of amplicon sequence variants (ASVs) and the according abundance (Callahan et al., 2016). Singleton ASVs were removed, and the remaining ASVs were taxonomically classified using the feature-classifier classify-sklearn plugin with a confidence score of 0.8 (Bokulich et al., 2018) against the training classifier on Silva 16S rRNA v.138 dataset (Quast et al., 2012). Only bacterial ASVs were retained, and the reads for each sample were resampled to the same depth (3,996 reads).
Metagenomic sequencing and analysis
Each of 16 fecal samples from the feces of WF and FP monkeys was randomly selected for metagenomic sequencing (Supplementary Table S1). Sequencing was performed on an Illumina NovaSeq PE150 platform (Shanghai Majorbio Bio-Pharm Technology Corporation, Shanghai, China), generating 10G of raw data for each sample. The fastp v.0.20.0 was used to remove the adapters and low-quality reads, which were with a length of <50 bp, or an average quality value of <20, or having N bases (Chen et al., 2018). The reads belonging to R. bieti genome (NCBI accession number: GCF_001698545) were removed by BWA v.0.7.9a (Li and Durbin, 2009). The remaining reads were assembled into contigs using MEGAHIT v.1.1.2 (Li et al., 2015), and contigs exceeding 300 bp were used to predict open reading frames (ORFs) with Prodigal (Hyatt et al., 2010). Predicted ORFs with a length of ≥100 bp were retrieved and translated into amino acid sequences. CD-HIT v.4.6.1 (Fu et al., 2012) was employed to construct a non-redundant gene catalog with 90% identity and 90% coverage. The high-quality reads were then aligned to this catalog using SOAP aligner v.2.21 (Li et al., 2008), to calculate the gene abundance with a 95% identity threshold. The KEGG annotation was conducted using Diamond v.0.8.35 (Buchfink et al., 2015) against the Kyoto Encyclopedia of Genes and Genomes database1 with an e-value cutoff of 1e−5. Antibiotic resistance annotation for the non-redundant gene catalog was performed using Diamond v.0.8.35 against the ARDB database2 with an e-value cutoff of 1e−5.
Statistical analysis
Main statistical analyses were conducted using R v.4.3.1 (R Core Team, 2013). Phylogenetic tree was generated for ASV sequences using Qiime2 align-to-tree-mafft-fasttree command. Rooted and unrooted phylogenetic trees were used to calculate phylogenetic diversity (PD) and nearest taxon index with the ape and picante packages (Kembel et al., 2010; Paradis and Schliep, 2018). Bray–Curtis similarity among gut microbiota communities was calculated with the vegan package (Oksanen et al., 2007) and used for principal coordinate analysis (PCoA). Difference in gut microbiota community structure between WF and FP populations was tested using Adonis permutational multivariate analysis of variance (PERMANOVA) with 9,999 permutations. A group of biomarker taxa, which were most sensitive to the food provisioning treatment, were identified using a Random Forest model with the randomForest package (Liaw and Wiener, 2014). Differential ASV abundance between WF and FP gut microbiota was visualized using a Manhattan plot, and the p-values for this plot were adjusted using the FDR method (Kaler et al., 2020). The relationships between the microbiota community structure and gut environmental conditions including fecal enzyme activities and nutrients were tested by the Mantel test (Oksanen et al., 2007). To further explore the importance of enzyme activity and gut nutrient in explaining variance of the gut microbiota community structure, variance partition was performed based on the hierarchical partitioning theory using the rdacca.hp package (Lai et al., 2022). Gradient forests implemented through the gradientForest package were used to explore the individual contributions of gut environmental conditions to the variance of each main ASV taxon (Ellis et al., 2012). This analysis included only the 300 most abundant ASVs in WF or FP, and the overall predictive ability of these predictors was calculated as the average proportion of variance explained by the fitted forest.
Spearman’s correlations were calculated among the 500 most abundant ASVs in each population gut microbiota. Then, the results of Spearman’s correlation with |r| > 0.5 and p < 0.05 were considered significant and then used to construct the network by Gephi v.0.9.3 (Bastian et al., 2009). Meanwhile, 10,000 random networks, which had the same number of nodes and edges as the real networks, were generated for each real network to evaluate whether the significance of observed correlations was caused by the random incidence (Ju et al., 2014). The significance of differences between the properties of real and random networks was tested by the Z-test. To compare the gut network stability of the WF and FP populations, the average degree and natural connectivity were tracked as 0–80% nodes were randomly removed from each network, simulating microbial extinction (Peura et al., 2015). Linear discriminant analysis (LDA) effect size (LEfSe) analysis was used to identify differentially abundant KEGG pathway annotation between the gut microbiota of the WF and FP populations. KEGG pathway annotation biomarkers were detected by LEfSe with an LDA score threshold of >3.0, p < 0.05 using the microeco package (Liu et al., 2021). Finally, a Pearson correlation analysis was used to calculate the correlations between ARGs and bacterial phyla, and only correlations with |r| > 0.4 and p < 0.05 were considered significant. PCoA and PERMANOVA were also used to test the differences of compositions of KEGG pathways and ARGs between WF and FP populations.
Results
Food provisioning increased gut microbiota diversity and changed their community composition
We found that food provisioning significantly decreased amylase activity and protein concentration (Table 1). It also resulted in a decrease in glucose concentration and an increase in pH, although these changes were not statistically significant (Table 1). From these fecal samples, we got 4,298,438 high-quality sequences, and the average quality score was 38 (Supplementary Figure S1). After resampling to the same depth, 3,973 ASVs were identified from all the fecal samples. The WF population had 2,085 ASVs, while the FP population had 2,363 ASVs, and there were 475 ASVs shared by both populations. Food provisioning significantly enhanced gut microbiota Shannon–wiener diversity, richness, evenness, and phylogenetic diversity and had no significant effect on the nearest taxon index (Table 2). For both WF and FP populations, the gut microbiota phyla were dominated by Firmicutes, Bacteroidetes, Proteobacteria, Spirochaetes, and Actinobacteria (Figure 1A). Notably, Proteobacteria and Firmicutes were the dominant phyla in the WF and FP populations, respectively (Figure 1A). Both their abundance in the dominant population was higher than that observed in the other populations (both p < 0.001). Furthermore, we found that the Firmicutes/Bacteroidetes (F/B) was 0.562 in the WF population, which was significantly lower than 5.019 of FP population (Supplementary Figure S2).
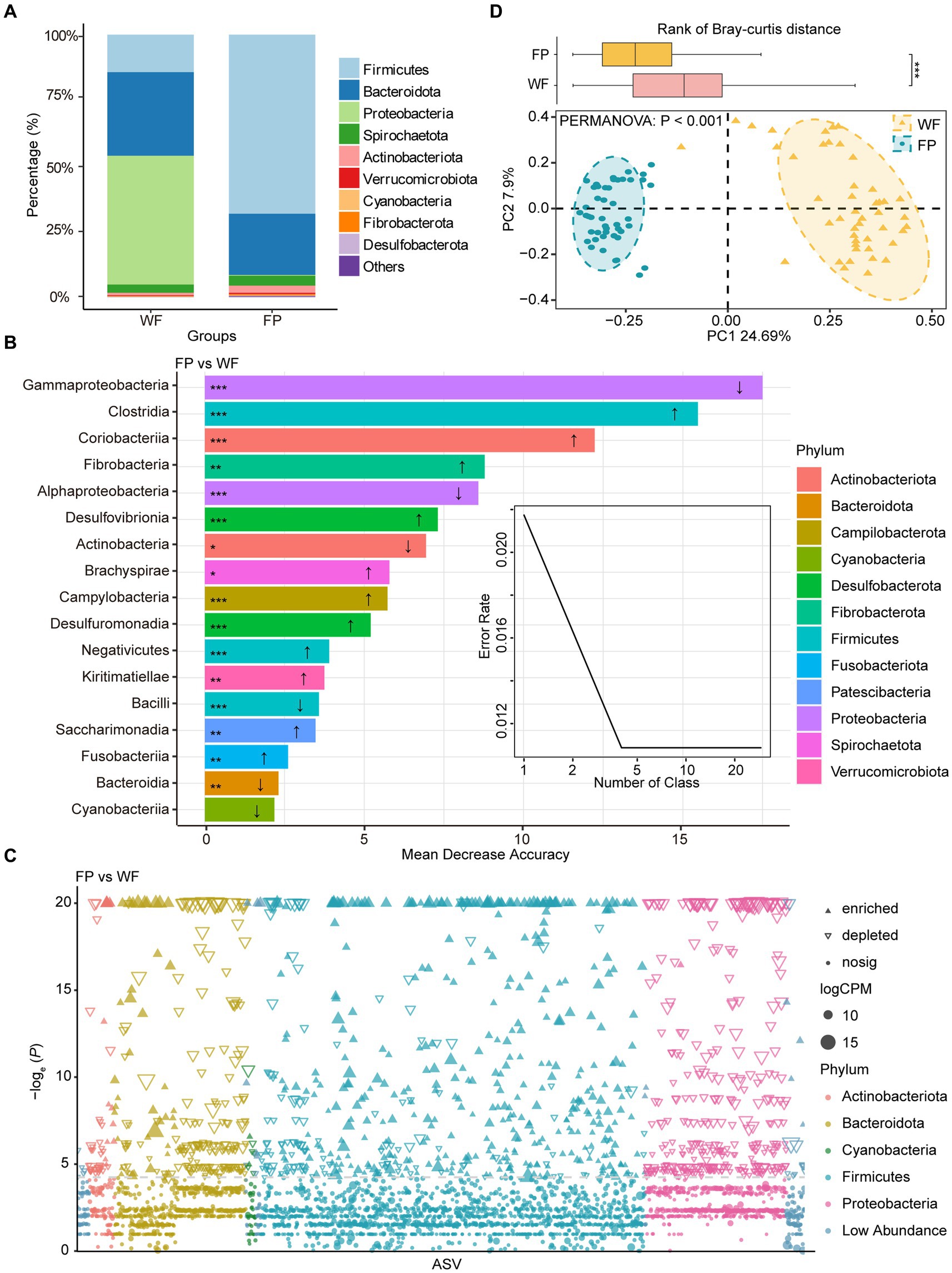
Figure 1. (A) Relative abundance of bacterial phyla in wild foraging (WF) and food-provisioned (FP) fecal samples. (B) The biomarker taxa listed in descending order of importance to the model accuracy, the up arrow means abundance increasing in FP fecal samples, vice versa. (C) Manhattan plots showing enriched or depleted ASVs in WF or FP fecal samples. Threshold = −loge (p − value) > 3. (D) PCoA of gut microbial communities and ellipses with different colors indicate 95% confidence intervals for each treatment. Boxplot above the PCoA ordination is the comparison of beta diversity of gut microbial communities based on Bray–curtis distance. ***p < 0.001; **p < 0.001; and *p < 0.05.
A Random Forest model was used to identify the most sensitive taxa to food provisioning (Figure 1B). The error curve stabilized with involving the 17 most sensitive classes, which belonged to 12 phyla, and these classes were identified as biomarker taxa (Figure 1B). Among these, the relative abundances of 11 classes, namely, Clostridia, Coriobacteriia, Fibrobacteria, Desulfovibrionia, Brachyspirae, Campylobacteria, Desulfuromonadia, Negativicutes, Kiritimatiellae, Saccharimonadia, and Fusobacteriia, were significantly increased by food provisioning. Conversely, five classes, namely, Gammaproteobacteria, Alphaproteobacteria, Actinobacteria, Bacilli, and Bacteroidia, were significantly reduced by food provisioning (Figure 1B). The Manhattan plot highlighted that food provisioning had a major impact on the ASVs from Bacteroidota, Firmicutes, and Proteobacteria. Food provisioning reduced 179, 126, and 332 ASVs in these phyla, respectively, and increased 39, 395, and 2 ASVs, respectively (Figure 1C). The PCoA showed a distinct clustering and separation of microbiota communities in the gut between WF and FP populations. PERMANOVA confirmed a significant effect of food provisioning on community structure (p < 0.001; Figure 1D). Beta-diversity of gut microbiota communities based on Bray–Curtis dissimilarity showed that the WF population had a higher dispersion (Figure 1D).
Mantel tests revealed a significant correlation between the gut microbiota community and a combination of the gut enzyme activities and nutrient contents in the WF population (r = 0.334, p < 0.001) but not in the FP population (r = 0.156, p = 0.051; Supplementary Figure S3). For the WF population, of these individual environmental factors, only pH significantly predicted the variance of microbiota community (Supplementary Figure S4). In contrast, no individual factors significantly predicted the variance of gut microbiota community of FP population. For the 300 most abundant ASVs, our gradient forest analysis successfully explained the variance of only 69 ASVs in the WF population and 30 ASVs in the FP population (Supplementary Figure S5). In addition, a t-test showed significantly higher explanation for the WF population (Figure 2). This suggests that the predominant ASVs in the WF population have a tighter correlation with gut environmental conditions compared with the FP population, and it was compatible with the results of the Mantel test.
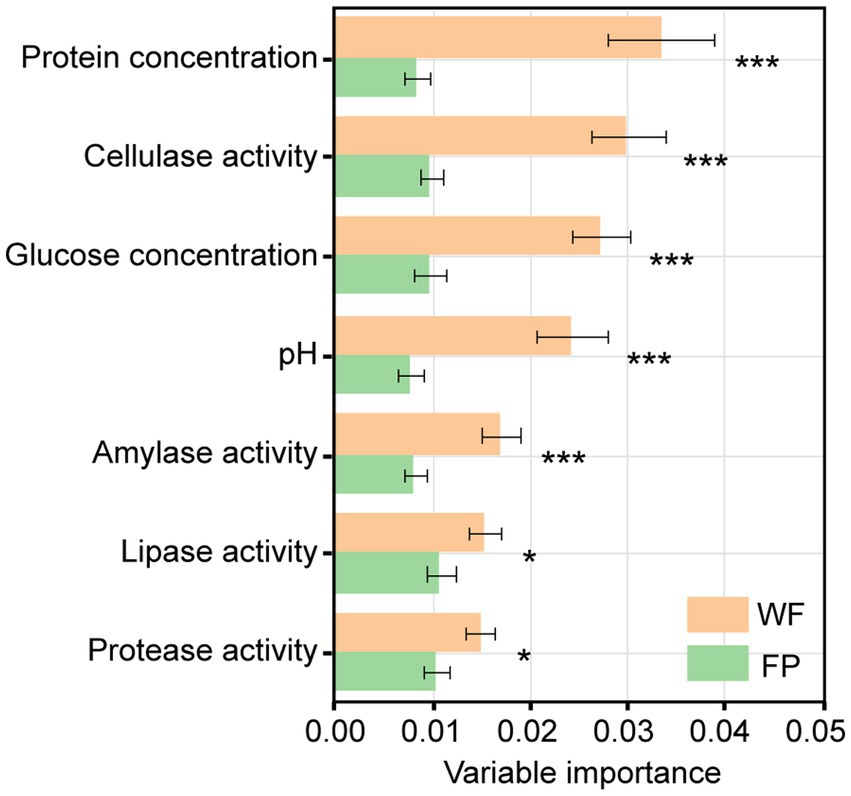
Figure 2. Importance of individual variables of gut enzyme activities and nutrients across models for all predominant ASV taxa. Asterisk means the significant t-test of the importance between WF and FP. ***p < 0.001; **p < 0.001; and *p < 0.05.
Food provisioning decreased gut microbiota network complexity and stability
Comparison of the main properties of real network, including clustering coefficient, modularity, average path length, and network diameter, with those of random network indicated that the real networks were non-random (Table 3). We found that the positive correlation dominated the gut microbiota network of both populations (Table 3). However, compared with the WF population, the gut microbiota network in the FP population had fewer nodes, links, average degree, linkage density, and network diameter (Figure 3A; Table 3). Thus, the WF population had a more complex gut microbiota network. Furthermore, a robustness test showed that the WF population maintained higher average degree and natural connectivity than the FP population as we removed 0–80% of the nodes from the networks (Figure 3B). This demonstrates a greater stability of the gut microbiota network of WF population.
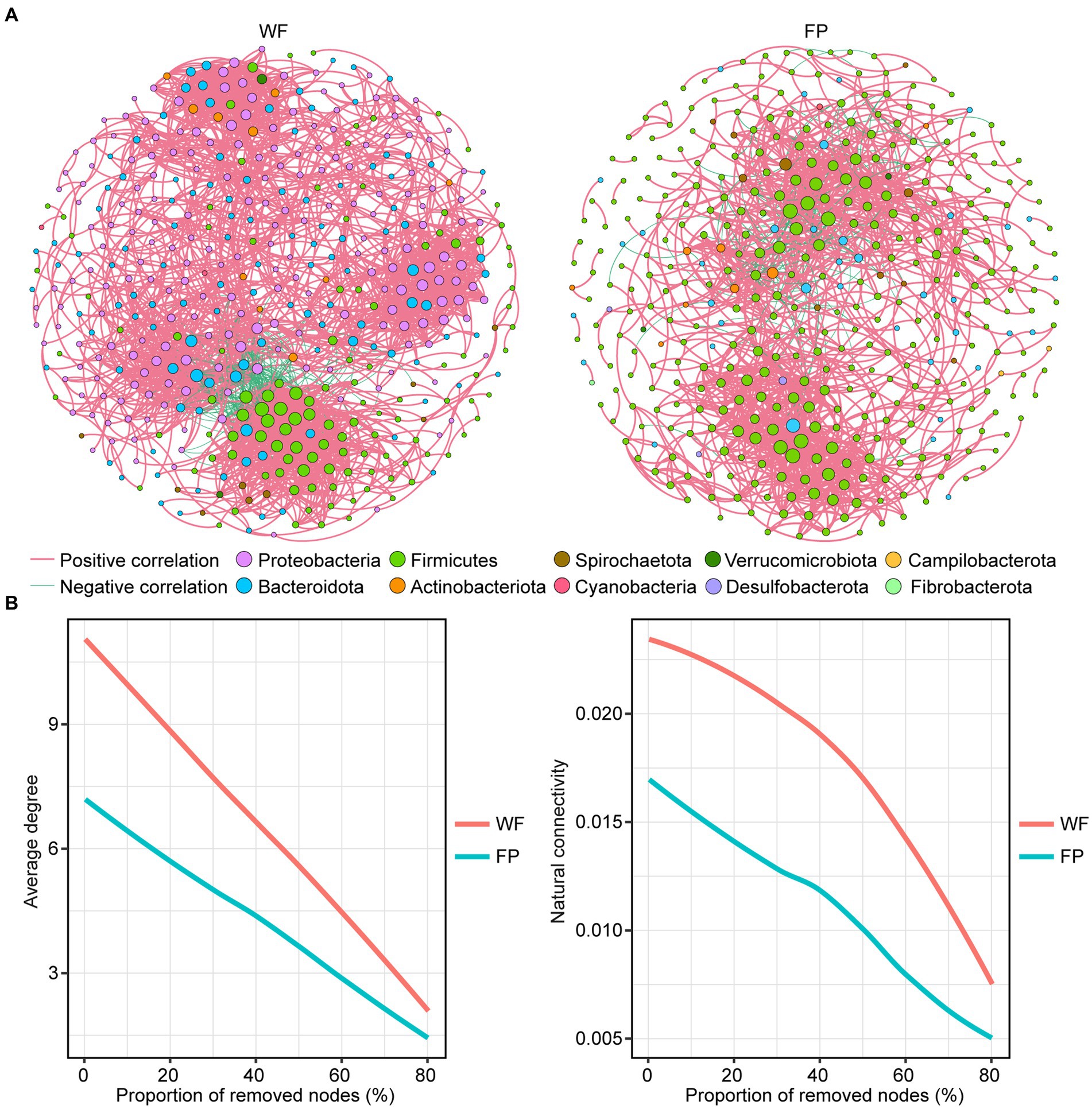
Figure 3. (A) Correlation network of gut microbes in WF and FP populations. The node size indicates the relative abundance of the ASV. (B) Robustness analysis for gut microbial communities between WF and FP populations by removing the same proportion of nodes.
Effect of food provisioning on the predicted KEGG pathways
KEGG functional analysis revealed that the predicted genes mapped to 6 level 1, 46 level 2, and 446 level 3 pathways. PERMANOVA analysis identified a significant effect of food provision on the overall KEGG pathway composition at all levels (levels 1–3, p < 0.001; Supplementary Figure S6). Analyzing KEGG level 1 revealed that the FP population had higher pathways involved in metabolism and genetic information processing, while the WF population had higher pathways involved in environmental information processing, cellular processes, and organismal systems (Figure 4A). At KEGG level 2, the FP population had higher 8 pathways, including the metabolisms of glycan and nucleotide, carbohydrate, and other pathways types such as cell growth and death and global metabolic and overview maps. The WF population had higher 10 pathways, including the metabolisms of cofactor and vitamin, amino acid, lipid, energy, and other amino acids, and other pathways types such as signal transduction, xenobiotic degradation, and metabolism (Figure 4B). At KEGG level 3, the FP population had higher 21 pathways, including the biosynthesis of amino acids, secondary metabolites, aminoacyl-tRNA, peptidoglycan, and lysine, and the metabolisms of starch and sucrose, pyrimidine, galactose, amino sugar, and nucleotide sugar, cysteine and methionine, fructose and mannose, and even the metabolic pathways. The WF population had higher 18 pathways, including the degradation of valine, leucine and isoleucine, benzoate, fatty acid, and lysine and the metabolisms of sulfur, fatty acid, tryptophan, glutathione, glyoxylate, and dicarboxylate (Figure 4C).
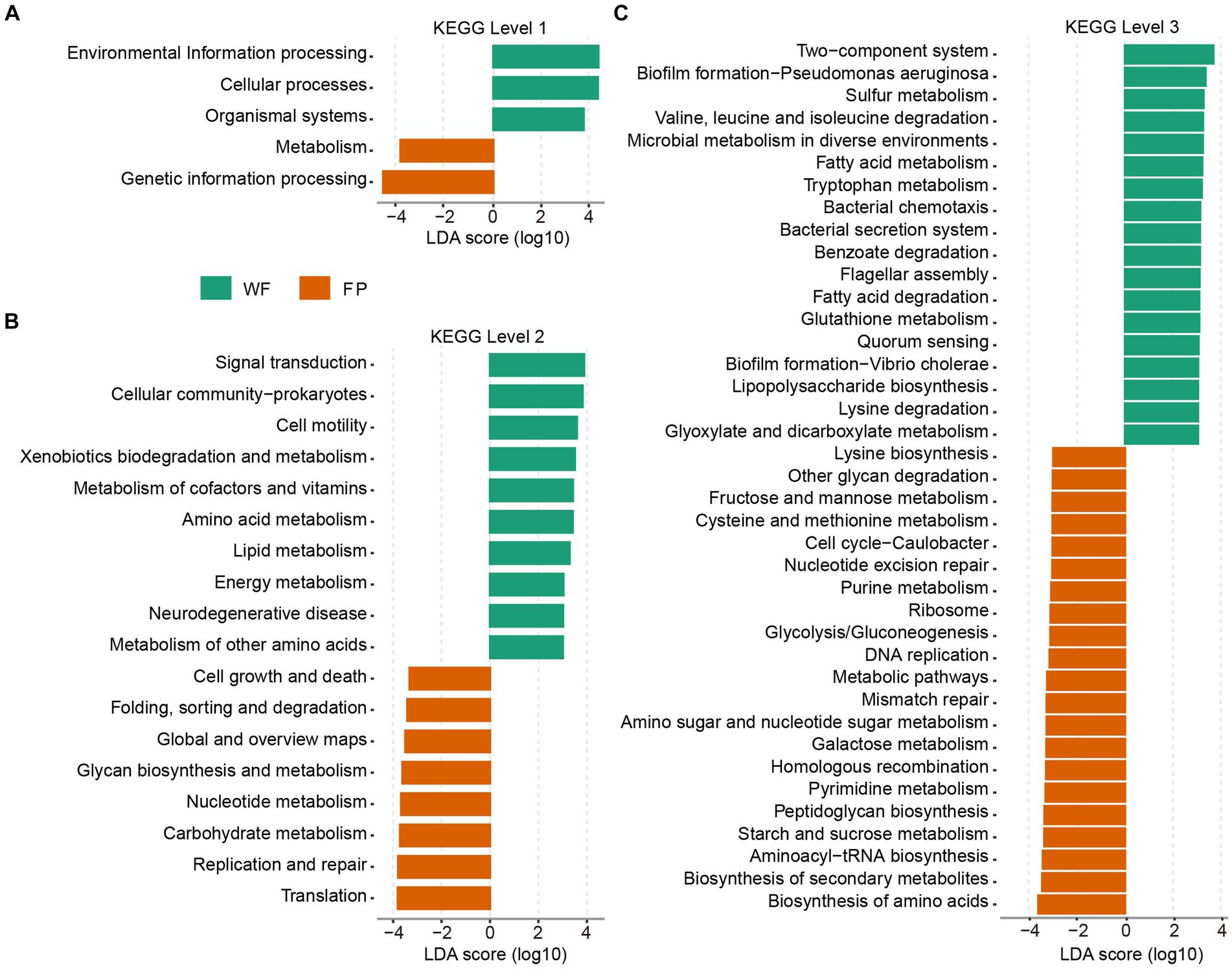
Figure 4. Plots of KEGG pathways comparisons between the WF (green) and FP (orange) populations at levels (A) 1, (B) 2, and (C) 3 analyzed by LEfSe analysis. LDA > 3.0, p < 0.05.
Increased abundance and altered composition of ARGs in the gut microbiota with food provisioning
Analysis of ARGs in the gut microbiota of the WF and FP populations revealed that vancomycin, tetracycline, and multidrug were the major antibiotic types (Figure 5A). Remarkably, the WF population had a higher abundance of multidrug, while the FP population had higher levels of vancomycin and tetracycline (Figure 5A). Compared with the WF population, food provisioning significantly enhanced the total abundance of ARGs by 81.7% (Figure 5B; Supplementary Figure S7), while food provisioning did change the Shannon diversity but reduced the richness of ARGs (Supplementary Figure S7). ARGs in both WF and FP populations were dominated by the bacA gene (Figure 5B). Food provisioning further increased the abundance of bacA with the greatest increase in the abundance compared with other ARG genes (Figure 5C). In addition, tetW, tetQ, tet40, and tetPB were increased, while mexB, mexF, mdtG, ksgA, and acrB were reduced by food provisioning (Figure 5C). PERMANOVA revealed a change in ARG composition with food provision (Supplementary Figure S8; p < 0.001). Our correlation network analysis identified positive correlations between 19 ARGs and 7 phyla taxa in the WF population and between 30 ARGs and 6 phyla taxa in the FP population (Figure 6). Interestingly, Verrucomicrobiota was the only phylum common to both networks (Figure 6). In each population, specific phyla had the most correlated ARGs: Patescibacteria in WF (9 types) and Proteobacteria in FP (22 types).
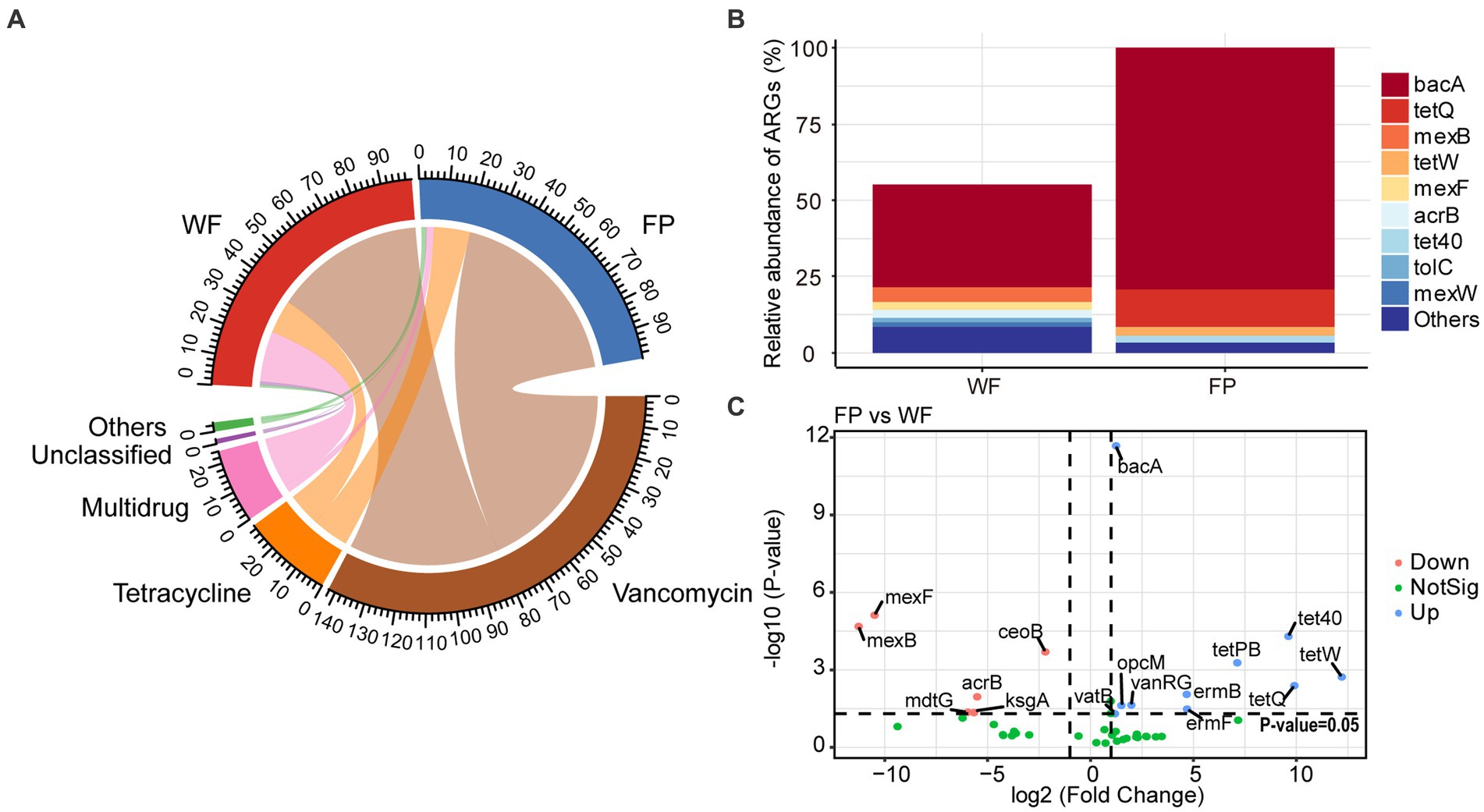
Figure 5. (A) Relative abundance of classification of antibiotic resistance genes (ARGs) according to antibiotic resistance type. (B) Relative abundance of predominant ARGs comparing to the total abundance of FP population. (C) Volcano plot revealed the abundance of differentially ARGs between FP and WF populations.
Discussion
Previous studies show that diet can influence enzyme activities and nutrient concentrations in the gut (Basolo et al., 2020; Dagar et al., 2023). In this study, food provisioning led to a decrease in gut amylase activity. Similar results were found in studies with nutrient-rich diets (Prakash and Srinivasan, 2012; Saravanan et al., 2014). This could be due to the fact that food provisioning provides sufficient energy, which reduces the body’s need for amylase. In addition, the WF population had higher gut protein concentrations, possibly due to their high intake of protein-rich diet (Xia et al., 2022).
We found that food provisioning increased gut microbiota diversity (Table 2). This could be due to the additional nutrients and carbohydrate substrates provided to the monkeys’ gut, which created more ecological niches for microbiota, ultimately leading to higher diversity (Louis et al., 2007). Food provisioning offered a wider variety of food to the monkey that could also potentially contribute to the increased gut microbiota diversity (Shepherd et al., 2018). However, the increase in ARGs observed in the FP monkeys (Figure 5) suggests another potential impact that increased indirect contact with humans through provisioning of food which could expand the monkeys’ bacterial sources.
Proteobacteria dominated the gut microbiota of WF monkeys and had higher abundance than that of FP monkeys (Figure 1A). Furthermore, Proteobacteria also contained the most sensitive biomarker class (Gammaproteobacteria) response to food provisioning (Figures 1B,C). This is likely due to the high bamboo shoot content in WF diets. Bamboo shoots are rich in unique secondary metabolites such as cyanogenic glycosides (Chongtham et al., 2011). Previous study showed that Proteobacteria had the genes encoding bamboo-degrading enzymes (Sang-A-Gad et al., 2011; Xia et al., 2020), and this is confirmed by the high Proteobacteria abundance in bamboo-eating giant pandas (Zhu et al., 2018). Firmicutes dominated the gut microbiota of FP monkeys, exceeding that of WF monkeys (Figure 1A), and the Firmicutes/Bacteroidetes (F/B) ratio was higher in FP monkeys (Supplementary Figure S2). This raises concerns about potential FP obesity, as the high F/B ratio is a marker of obesity (Crovesy et al., 2020), and we should further monitor the individual obesity of monkeys under food provisioning.
The composition of gut microbiota differed between WF and FP monkeys (Figure 1C). It may be caused by the different diet composition and overall high nutrient intake (Louis et al., 2007). However, according to Cui et al. (2022), a study focusing on another non-human primate, Macaca mulatta, suggests that nutrient level exerts a stronger influence on gut microbiota than diet composition. This highlights the need for further exploration of how these factors differentially impact the gut microbiota of R. bieti. Interestingly, the FP population displayed lower community dissimilarity within their population compared with WF population (Figure 1C). This result could be explained by the food provisioning that leads to a more consistent diet among individuals, thereby reducing variation in gut microbiota communities (Li et al., 2016). Notably, both Mantel test and gradient forest analysis revealed that the gut microbiota community of the WF population exhibited a stronger correlation with their gut environment. According to the nutrient niche theory, we hypothesize that the increased nutrient intake from food provisioning could make the gut environment more conducive to the growth of gut microbiota, which enhances the resistance of the microbiota community to the changes in the gut environment. This seems to reduce the linkage between the gut environmental condition and gut microbiota community composition (Freter et al., 1983), while this observation figures out that the mechanism behind this result needs further investigation.
The analysis of gut microbiota networks revealed that the positive correlation dominated both populations, suggesting that microbiota growth in their gut mainly relies on collaboration (Layeghifard et al., 2017). Further comparison of the networks revealed that the gut of WF population had a more complex microbiota network (Figure 3A; Table 3). This is mainly because most microbiota require nutrient exchange to grow normally, and when their overall nutrient intake is lower, as in the case of the WF population, their interdependence will increase, which promotes the complexity of their networks (Layeghifard et al., 2017; Zengler and Zaramela, 2018). The network robustness test conducted in our study showed that the gut microbiota network degree and natural connectivity of the WF population after continuous removal of ASVs were always higher than those of the FP population (Figure 3B). This suggests that WF monkeys exhibit greater resilience and stability in their gut microbiota communities (Coyte et al., 2015).
The KEGG pathway analysis showed that food provisioning significantly changed the composition of gut KEGG functions (Supplementary Figure S6), enriching the pathway-related metabolism and genetic information processing (Figure 4A). This is due to the fact that the FP population had higher nutrient intake. In this condition, higher nutrient provides gut microbiota with more raw materials to build their cellular components and enzymes, which needs more gene expression and protein synthesis (Okie et al., 2020). Furthermore, higher nutrient could also enhance to build the pathways for efficient and diverse metabolic processes. However, this metabolic enrichment was not evenly distributed. The KEGG level 2 analysis revealed this enrichment on metabolism pathways that were related to global metabolic and overview maps and metabolisms of carbohydrate, glycan, and nucleotide, while pathways related to xenobiotic degradation and metabolism and metabolisms of cofactor, vitamin, amino acid, lipid, energy, and other amino acids were all depleted. Our food provisioning, including carbohydrate-rich peanut and pumpkin seeds, could trigger the activity of carbohydrate metabolism (Li et al., 2017). Conversely, the low nutrient intake of the WF population forced them to upregulate the pathways involved in breaking down and utilization of other substrates such as xenobiotics, cofactors, vitamins, amino acids, lipids, and energy-generating molecules to meet the energy needs for gut microbiota. Drilling down to KEGG level 3, we observed enhanced degradation pathways in the WF population, specifically for benzoate, valine, leucine, isoleucine, lysine, and fatty acids (Figure 4C). We hypothesized that the food sources of WF population contained more plant toxins such as alkaloids, which potentially induced higher microbiota detoxification efforts, including geraniol breakdown (Chen and Viljoen, 2010). In addition, the higher protein content in WF diets likely provided them with a rich amino acid pool, increasing the degradation of valine, leucine, isoleucine, and lysine (Madsen et al., 2017).
This study confirmed that the food provisioning increased the abundance of ARGs in the gut of R. bieti (Figure 5B). This may be because both human contact and artificial food intake during food provisioning could increase the horizontal transformation of ARGs to the gut (Allen et al., 2010; Hu et al., 2017). Another possible reason might be due to the dietary influence as Yan et al. (2022) found that high-fat/low-fiber diet could also increase both abundance and diversity of ARGs of the gut of cynomolgus monkeys (Macaca fascicularis). However, our food provisioning had no effect on the Shannon diversity and even reduced the richness of ARGs (Supplementary Figure S7). While the bacA was the most abundant and increased ARGs in our study (Figure 5B), this ARG type abundance was not changed by the high-fat diet in the study of Yan et al. (2022). Thus, we speculated that the horizontal transformation was the main mechanism to enrich the abundance of ARGs in the gut of R. bieti, but both possible mechanisms should be investigated in the future. In this study, bacA was dominated in both WF and FP populations among the ARGs. This result is also found from human (Feng et al., 2018; Li et al., 2021) and other animals (Thomas et al., 2021; Zhang et al., 2022). Meanwhile, the most increased ARGs by food provisioning were also the bacA (Figure 5B), which might be due to its high abundance in human guts (Feng et al., 2018; Li et al., 2021), facilitating its more and effortless transfer to FP monkeys. Another finding is that the abundance of tetracycline ARGs, including tetPB, tet40, tetW, and tetQ, was increased by food provisioning (Figure 5C). This could be partly explained by the result about the suppression of gut protein on tetracycline ARGs, which was observed by Zhang et al. (2016). As the FP population had lower gut protein concentration compared with the WF population, this factor might contribute to the increased abundance of these specific ARGs. Our analysis of ARG correlation networks with gut bacterial phyla revealed that food provisioning could alter the correlations between ARGs and specific bacterial phyla (Figure 6). Notably, Patescibacteria had the most correlations with ARGs in the WF population, while Proteobacteria had the highest correlations in the FP population, with a higher number of connections than the WF population. Both phyla have been previously implicated in harboring ARGs (Chen et al., 2017; Kim et al., 2023). We hypothesized that the richer nutritional environment in the FP population might stimulate ARG transfer to Proteobacteria, but further research was needed to unravel the underlying mechanisms.
Conclusion
Our study demonstrated the complex impact of food provisioning on the gut microbiota of Yunnan snub-nosed monkeys. We observed that while food provisioning increases the diversity of gut microbiota, it also changed the gut microbiota community composition, leading to greater homogeneity of community composition among individuals. The network analysis revealed that food provisioning reduced the complexity and stability of the gut microbiota network, potentially weakening its resilience to disturbance. Furthermore, metagenomic sequencing revealed that food provisioning significantly increases the abundance of ARGs in the gut, potentially raising the risk of drug resistance in this wildlife. These findings underscored the necessity of a comprehensive approach when considering food provisioning for wildlife. While increasing diversity may seem beneficial (Fan and Pedersen, 2021), it was crucial to simultaneously monitor the potential drawbacks, including altered community structure, changed network stability, and increased ARGs, to truly ensure the benefits of this conservation practice.
Data availability statement
The datasets presented in this study can be found in online repositories. The sequences data is deposited in National Microbiology Data Center (NMDC) with accession number NMDC10018711. (https://nmdc.cn/resource/genomics/project/detail/NMDC10018711).
Author contributions
LL: Conceptualization, Data curation, Formal analysis, Methodology, Software, Writing – original draft, Writing – review & editing. SJ: Conceptualization, Investigation, Methodology, Software, Validation, Writing – review & editing. YT: Investigation, Project administration, Resources, Writing – review & editing. DL: Data curation, Investigation, Resources, Supervision, Writing – review & editing. MQ: Conceptualization, Data curation, Software, Supervision, Writing – review & editing.
Funding
The author(s) declare financial support was received for the research, authorship, and/or publication of this article. We are grateful to the financial supports of the Open Project Funding of the Key Laboratory of Southwest China Wildlife Resource Conservation (Ministry of Education) (XNYB21-04), the National Natural Science Foundation of China (No. 32070454), the Second Tibetan Plateau Scientific Expedition and Research Program (No. 2019QZKK0501), the Natural Science Foundation of Sichuan Province (2023NSFSC1223 and 2023NSFSC1152), and the Innovation Team Funds of China West Normal University (KCXTD2023-5).
Conflict of interest
The authors declare that the research was conducted in the absence of any commercial or financial relationships that could be construed as a potential conflict of interest.
Publisher’s note
All claims expressed in this article are solely those of the authors and do not necessarily represent those of their affiliated organizations, or those of the publisher, the editors and the reviewers. Any product that may be evaluated in this article, or claim that may be made by its manufacturer, is not guaranteed or endorsed by the publisher.
Supplementary material
The Supplementary material for this article can be found online at: https://www.frontiersin.org/articles/10.3389/fmicb.2024.1361218/full#supplementary-material
Footnotes
References
Allen, H. K., Donato, J., Wang, H. H., Cloud-Hansen, K. A., Davies, J., and Handelsman, J. (2010). Call of the wild: antibiotic resistance genes in natural environments. Nat. Rev. Microbiol. 8, 251–259. doi: 10.1038/nrmicro2312
Andersson, R. E. (1980). Microbial lipolysis at low temperatures. Appl. Environ. Microb. 39, 36–40. doi: 10.1128/aem.39.1.36-40.1980
Basolo, A., Hohenadel, M., Ang, Q. Y., Piaggi, P., Heinitz, S., Walter, M., et al. (2020). Effects of underfeeding and oral vancomycin on gut microbiome and nutrient absorption in humans. Nat. Med. 26, 589–598. doi: 10.1038/s41591-020-0801-z
Bastian, M., Heymann, S., and Jacomy, M. (2009). Gephi: an open source software for exploring and manipulating networks. Stanford, CA: AAAI.
Bokulich, N. A., Kaehler, B. D., Rideout, J. R., Dillon, M., Bolyen, E., Knight, R., et al. (2018). Optimizing taxonomic classification of marker-gene amplicon sequences with QIIME 2’s q2-feature-classifier plugin. Microbiome 6, 90–17. doi: 10.1186/s40168-018-0470-z
Bolyen, E., Rideout, J. R., Dillon, M. R., Bokulich, N. A., Abnet, C. C., Al-Ghalith, G. A., et al. (2019). Reproducible, interactive, scalable and extensible microbiome data science using QIIME 2. Nat. Biotechnol. 37, 852–857. doi: 10.1038/s41587-019-0209-9
Borji, A., Borji, F., and Jourani, A. (2017). A new method for the determination of sucrose concentration in a pure and impure system: spectrophotometric method. Int. J. Anal. Chem. 2017, 8214120–8214126. doi: 10.1155/2017/8214120
Breuil, C., and Saddler, J. N. (1985). Comparison of the 3,5-dinitrosalicylic acid and Nelson-Somogyi methods of assaying for reducing sugars and determining cellulase activity. Enzym. Microb. Technol. 7, 327–332. doi: 10.1016/0141-0229(85)90111-5
Buchfink, B., Xie, C., and Huson, D. H. (2015). Fast and sensitive protein alignment using DIAMOND. Nat. Methods 12, 59–60. doi: 10.1038/nmeth.3176
Callahan, B. J., McMurdie, P. J., Rosen, M. J., Han, A. W., Johnson, A. J. A., and Holmes, S. P. (2016). DADA2: high-resolution sample inference from Illumina amplicon data. Nat. Methods 13, 581–583. doi: 10.1038/nmeth.3869
Catalkaya, G., Venema, K., Lucini, L., Rocchetti, G., Delmas, D., Daglia, M., et al. (2020). Interaction of dietary polyphenols and gut microbiota: microbial metabolism of polyphenols, influence on the gut microbiota, and implications on host health. Food Front. 1, 109–133. doi: 10.1002/fft2.25
Chen, B., He, R., Yuan, K., Chen, E., Lin, L., Chen, X., et al. (2017). Polycyclic aromatic hydrocarbons (PAHs) enriching antibiotic resistance genes (ARGs) in the soils. Environ. Pollut. 220, 1005–1013. doi: 10.1016/j.envpol.2016.11.047
Chen, W., and Viljoen, A. M. (2010). Geraniol — A review of a commercially important fragrance material. S. Afr. J. Bot. 76, 643–651. doi: 10.1016/j.sajb.2010.05.008
Chen, S., Zhou, Y., Chen, Y., and Gu, J. (2018). Fastp: an ultra-fast all-in-one FASTQ preprocessor. Bioinformatics 34, i884–i890. doi: 10.1093/bioinformatics/bty560
Chongtham, N., Bisht, M. S., and Haorongbam, S. (2011). Nutritional properties of bamboo shoots: potential and prospects for utilization as a health food. Compr. Rev. Food Sci. Food Saf. 10, 153–168. doi: 10.1111/j.1541-4337.2011.00147.x
Coyte, K. Z., Schluter, J., and Foster, K. R. (2015). The ecology of the microbiome: networks, competition, and stability. Science 350, 663–666. doi: 10.1126/science.aad2602
Crovesy, L., Masterson, D., and Rosado, E. L. (2020). Profile of the gut microbiota of adults with obesity: a systematic review. Eur. J. Clin. Nutr. 74, 1251–1262. doi: 10.1038/s41430-020-0607-6
Cui, Z., Holmes, A. J., Zhang, W., Hu, D., Shao, Q., Wang, Z., et al. (2022). Seasonal diet and microbiome shifts in wild rhesus macaques are better correlated at the level of nutrient components than food items. Integr. Zool. 17, 1147–1161. doi: 10.1111/1749-4877.12601
Dagar, V. S., Mishra, M., Sharma, A., Sankar, M., Verma, A., Saxena, T., et al. (2023). Alterations in the gut enzymes of Helicoverpa armigera (Hübner) induced by dietary Artemisia annua L. essential oil. Int. J. Trop. Insect Sci 43, 1295–1303. doi: 10.1007/s42690-023-01035-1
Ellis, N., Smith, S. J., and Pitcher, C. R. (2012). Gradient forests: calculating importance gradients on physical predictors. Ecology 93, 156–168. doi: 10.1890/11-0252.1
Fan, Y., and Pedersen, O. (2021). Gut microbiota in human metabolic health and disease. Nat. Rev. Microbiol. 19, 55–71. doi: 10.1038/s41579-020-0433-9
Feng, J., Li, B., Jiang, X., Yang, Y., Wells, G. F., Zhang, T., et al. (2018). Antibiotic resistome in a large-scale healthy human gut microbiota deciphered by metagenomic and network analyses. Environ. Microbiol. 20, 355–368. doi: 10.1111/1462-2920.14009
Freter, R., Brickner, H., Fekete, J., Vickerman, M. M., and Carey, K. E. (1983). Survival and implantation of Escherichia coli in the intestinal tract. Infect. Immun. 39, 686–703. doi: 10.1128/iai.39.2.686-703.1983
Fu, L., Niu, B., Zhu, Z., Wu, S., and Li, W. (2012). CD-HIT: accelerated for clustering the next-generation sequencing data. Bioinformatics 28, 3150–3152. doi: 10.1093/bioinformatics/bts565
Gerritsen, J., Smidt, H., Rijkers, G. T., and de Vos, W. M. (2011). Intestinal microbiota in human health and disease: the impact of probiotics. Genes Nutr. 6, 209–240. doi: 10.1007/s12263-011-0229-7
Hu, Y., Gao, G. F., and Zhu, B. (2017). The antibiotic resistome: gene flow in environments, animals and human beings. Front. Med. 11, 161–168. doi: 10.1007/s11684-017-0531-x
Huang, K., Wang, J., Huang, J., Zhang, S., Vogler, A. P., Liu, Q., et al. (2021). Host phylogeny and diet shape gut microbial communities within bamboo-feeding insects. Front. Microbiol. 12:633075. doi: 10.3389/fmicb.2021.633075
Hyatt, D., Chen, G. L., LoCascio, P. F., Land, M. L., Larimer, F. W., and Hauser, L. J. (2010). Prodigal: prokaryotic gene recognition and translation initiation site identification. BMC Bioinformatics 11, 1–11. doi: 10.1186/1471-2105-11-119
Jandhyala, S. M., Talukdar, R., Subramanyam, C., Vuyyuru, H., Sasikala, M., and Reddy, D. N. (2015). Role of the normal gut microbiota. World J. Gastroenterol. 21, 8787–8803. doi: 10.3748/wjg.v21.i29.8787
Ju, F., Xia, Y., Guo, F., Wang, Z., and Zhang, T. (2014). Taxonomic relatedness shapes bacterial assembly in activated sludge of globally distributed wastewater treatment plants. Environ. Microbiol. 16, 2421–2432. doi: 10.1111/1462-2920.12355
Kaler, A. S., Gillman, J. D., Beissinger, T., and Purcell, L. C. (2020). Comparing different statistical models and multiple testing corrections for association mapping in soybean and maize. Front. Plant Sci. 10:1794. doi: 10.3389/fpls.2019.01794
Kembel, S. W., Cowan, P. D., Helmus, M. R., Cornwell, W. K., Morlon, H., Ackerly, D. D., et al. (2010). Picante: R tools for integrating phylogenies and ecology. Bioinformatics 26, 1463–1464. doi: 10.1093/bioinformatics/btq166
Kilburn, L. R., Koester, L. R., Schmitz-Esser, S., Serão, N. V. L., and Rossoni Serão, M. C. (2020). High-fat diets led to OTU-level shifts in fecal samples of healthy adult dogs. Front. Microbiol. 11:564160. doi: 10.3389/fmicb.2020.564160
Kim, J. J., Seong, H. J., Johnson, T. A., Cha, C.-J., Sul, W. J., and Chae, J.-C. (2023). Persistence of antibiotic resistance from animal agricultural effluents to surface water revealed by genome-centric metagenomics. J. Hazard. Mater. 457:131761. doi: 10.1016/j.jhazmat.2023.131761
Lai, J., Zou, Y., Zhang, J., and Peres-Neto, P. R. (2022). Generalizing hierarchical and variation partitioning in multiple regression and canonical analyses using the rdacca. hp R package. Methods Ecol. Evol. 13, 782–788. doi: 10.1111/2041-210X.13800
Layeghifard, M., Hwang, D. M., and Guttman, D. S. (2017). Disentangling interactions in the microbiome: a network perspective. Trends Microbiol. 25, 217–228. doi: 10.1016/j.tim.2016.11.008
Lee, W.-J., and Hase, K. (2014). Gut microbiota–generated metabolites in animal health and disease. Nat. Chem. Biol. 10, 416–424. doi: 10.1038/nchembio.1535
Ley, R. E., Hamady, M., Lozupone, C., Turnbaugh, P. J., Ramey, R. R., Bircher, J. S., et al. (2008). Evolution of mammals and their gut microbes. Science 320, 1647–1651. doi: 10.1126/science.1155725
Li, H., and Durbin, R. (2009). Fast and accurate short read alignment with burrows–wheeler transform. Bioinformatics 25, 1754–1760. doi: 10.1093/bioinformatics/btp324
Li, J., Hou, Q., Zhang, J., Xu, H., Sun, Z., Menghe, B., et al. (2017). Carbohydrate staple food modulates gut microbiota of Mongolians in China. Front. Microbiol. 8:484. doi: 10.3389/fmicb.2017.00484
Li, H., Li, T., Beasley, D. E., Heděnec, P., Xiao, Z., Zhang, S., et al. (2016). Diet diversity is associated with beta but not alpha diversity of pika gut microbiota. Front. Microbiol. 7:1169. doi: 10.3389/fmicb.2016.01169
Li, R., Li, Y., Kristiansen, K., and Wang, J. (2008). SOAP: short oligonucleotide alignment program. Bioinformatics 24, 713–714. doi: 10.1093/bioinformatics/btn025
Li, D., Liu, C. M., Luo, R., Sadakane, K., and Lam, T. W. (2015). MEGAHIT: an ultra-fast single-node solution for large and complex metagenomics assembly via succinct de Bruijn graph. Bioinformatics 31, 1674–1676. doi: 10.1093/bioinformatics/btv033
Li, J., Si, H., Du, H., Guo, H., Dai, H., Xu, S., et al. (2021). Comparison of gut microbiota structure and Actinobacteria abundances in healthy young adults and elderly subjects: a pilot study. BMC Microbiol. 21:13. doi: 10.1186/s12866-020-02068-z
Li, H. B., Sun, J., Li, L. H., Zhou, Y., Fang, X. L., Li, B.-Y., et al. (2023). Effects of provisioning on the activity budget and foraging strategies of black-and-white snub-nosed monkeys (Rhinopithecus bieti) in the Baima Snow Mountain nature reserve, Yunnan, China 85:e23548. doi: 10.1002/ajp.23548
Liaw, A., and Wiener, M. (2014). Package ‘random Forest’: Breiman and Cutler’s random forests for classification and regression. R Development Core Team 4, 6–10.
Liu, C., Cui, Y., Li, X., and Yao, M. (2021). microeco: an R package for data mining in microbial community ecology. FEMS Microbiol. Ecol. 97:fiaa 255. doi: 10.1093/femsec/fiaa255
Liu, Z., Ren, B., Wu, R., Zhao, L., Hao, Y., Wang, B., et al. (2009). The effect of landscape features on population genetic structure in Yunnan snub-nosed monkeys (Rhinopithecus bieti) implies an anthropogenic genetic discontinuity. Mol. Ecol. 18, 3831–3846. doi: 10.1111/j.1365-294X.2009.04330.x
Liu, C., Zhao, D., Ma, W., Guo, Y., Wang, A., Wang, Q., et al. (2016). Denitrifying sulfide removal process on high-salinity wastewaters in the presence of Halomonas sp. Appl. Microbiol. Biot. 100, 1421–1426. doi: 10.1007/s00253-015-7039-6
Louis, P., Scott, K. P., Duncan, S. H., and Flint, H. J. (2007). Understanding the effects of diet on bacterial metabolism in the large intestine. J. Appl. Microbiol. 102, 1197–1208. doi: 10.1111/j.1365-2672.2007.03322.x
Madsen, L., Myrmel, L. S., Fjære, E., Liaset, B., and Kristiansen, K. (2017). Links between dietary protein sources, the gut microbiota, and obesity. Front. Physiol. 8:1047. doi: 10.3389/fphys.2017.01047
Magoč, T., and Salzberg, S. L. (2011). FLASH: fast length adjustment of short reads to improve genome assemblies. Bioinformatics 27, 2957–2963. doi: 10.1093/bioinformatics/btr507
Makki, K., Deehan, E. C., Walter, J., and Bäckhed, F. (2018). The impact of dietary fiber on gut microbiota in host health and disease. Cell Host Microbe 23, 705–715. doi: 10.1016/j.chom.2018.05.012
McDonald, C. E., and Chen, L. L. (1965). The Lowry modification of the Folin reagent for determination of proteinase activity. Anal. Biochem. 10, 175–177. doi: 10.1016/0003-2697(65)90255-1
Nagpal, R., Shively, C. A., Appt, S. A., Register, T. C., Michalson, K. T., Vitolins, M. Z., et al. (2018). Gut microbiome composition in non-human Primates consuming a Western or Mediterranean diet. Front. Nutr. 5:28. doi: 10.3389/fnut.2018.00028
Okie, J. G., Poret-Peterson, A. T., Lee, Z. M. P., Richter, A., Alcaraz, L. D., Eguiarte, L. E., et al. (2020). Genomic adaptations in information processing underpin trophic strategy in a whole-ecosystem nutrient enrichment experiment. eLife 9:e49816. doi: 10.7554/eLife.49816
Oksanen, J., Kindt, R., Legendre, P., O’Hara, B., Stevens, M. H. H., Oksanen, M. J., et al. (2007). The vegan package. Commun. Ecol. Package 10:719.
Paradis, E., and Schliep, K. (2018). ape 5.0: an environment for modern phylogenetics and evolutionary analyses in R. Bioinformatics 35, 526–528. doi: 10.1093/bioinformatics/bty633
Peura, S., Bertilsson, S., Jones, R. I., and Eiler, A. (2015). Resistant microbial cooccurrence patterns inferred by network topology. Appl. Environ. Microb. 81, 2090–2097. doi: 10.1128/AEM.03660-14
Prakash, U. N. S., and Srinivasan, K. (2012). Fat digestion and absorption in spice-pretreated rats. J. Sci. Food Agric. 92, 503–510. doi: 10.1002/jsfa.4597
Quast, C., Pruesse, E., Yilmaz, P., Gerken, J., Schweer, T., Yarza, P., et al. (2012). The SILVA ribosomal RNA gene database project: improved data processing and web-based tools. Nucleic Acids Res. 41, D590–D596. doi: 10.1093/nar/gks1219
R Core Team (2013). R: A language and environment for statistical computing. Vienna, Austria: R Foundation for Statistical Computing.
Ramsamy, Y., Mlisana, K. P., Amoako, D. G., Abia, A. L. K., Ismail, A., Allam, M., et al. (2022). Mobile genetic elements-mediated Enterobacterales-associated carbapenemase antibiotic resistance genes propagation between the environment and humans: a one health South African study. Sci. Total Environ. 806:150641. doi: 10.1016/j.scitotenv.2021.150641
Rosshart, S. P., Vassallo, B. G., Angeletti, D., Hutchinson, D. S., Morgan, A. P., Takeda, K., et al. (2017). Wild mouse gut microbiota promotes host fitness and improves disease resistance. Cell 171, 1015–1028.e13. doi: 10.1016/j.cell.2017.09.016
Sang-A-Gad, P., Guharat, S., and Wananukul, W. (2011). A mass cyanide poisoning from pickling bamboo shoots. Clin. Toxicol. 49, 834–839. doi: 10.3109/15563650.2011.618456
Saravanan, G., Ponmurugan, P., Deepa, M. A., and Senthilkumar, B. (2014). Anti-obesity action of gingerol: effect on lipid profile, insulin, leptin, amylase and lipase in male obese rats induced by a high-fat diet. J. Sci. Food Agric. 94, 2972–2977. doi: 10.1002/jsfa.6642
Sedmak, J. J., and Grossberg, S. E. (1977). A rapid, sensitive, and versatile assay for protein using Coomassie brilliant blue G250. Anal. Biochem. 79, 544–552. doi: 10.1016/0003-2697(77)90428-6
Shepherd, E. S., DeLoache, W. C., Pruss, K. M., Whitaker, W. R., and Sonnenburg, J. L. (2018). An exclusive metabolic niche enables strain engraftment in the gut microbiota. Nature 557, 434–438. doi: 10.1038/s41586-018-0092-4
Thomas, J. C., Kieran, T. J., Finger, J. W., Bayona-Vásquez, N. J., Oladeinde, A., Beasley, J. C., et al. (2021). Unveiling the gut microbiota and resistome of wild cotton mice, Peromyscus gossypinus, from heavy metal- and radionuclide-contaminated sites in the Southeastern United States. Microbiol. Spectrum 9:e0009721. doi: 10.1128/spectrum.00097-21
Tomova, A., Bukovsky, I., Rembert, E., Yonas, W., Alwarith, J., Barnard, N. D., et al. (2019). The effects of vegetarian and vegan diets on gut microbiota. Front. Nutr. 6:47. doi: 10.3389/fnut.2019.00047
Tsuji, M., Suzuki, K., Kinoshita, K., and Fagarasan, S. (2008). Dynamic interactions between bacteria and immune cells leading to intestinal IgA synthesis. Semin. Immunol. 20, 59–66. doi: 10.1016/j.smim.2007.12.003
Wagg, C., Schlaeppi, K., Banerjee, S., Kuramae, E. E., and van der Heijden, M. G. (2019). Fungal-bacterial diversity and microbiome complexity predict ecosystem functioning. Nat. Commun. 10:4841. doi: 10.1038/s41467-019-12798-y
Weiss, S., Van Treuren, W., Lozupone, C., Faust, K., Friedman, J., Deng, Y., et al. (2016). Correlation detection strategies in microbial data sets vary widely in sensitivity and precision. ISME J. 10, 1669–1681. doi: 10.1038/ismej.2015.235
Wu, G. D., Chen, J., Hoffmann, C., Bittinger, K., Chen, Y. Y., Keilbaugh, S. A., et al. (2011). Linking long-term dietary patterns with gut microbial enterotypes. Science 334, 105–108. doi: 10.1126/science.1208344
Xia, W., Liu, G., Wang, D., Chen, H., Zhu, L., and Li, D. (2022). Functional convergence of Yunnan snub-nosed monkey and bamboo-eating panda gut microbiomes revealing the driving by dietary flexibility on mammal gut microbiome. Comput. Struct. Biotechnol. J. 20, 685–699. doi: 10.1016/j.csbj.2022.01.011
Xia, W., Zhang, C., Zhuang, H., Ren, B., Zhou, J., Shen, J., et al. (2020). The potential distribution and disappearing of Yunnan snub-nosed monkey: influences of habitat fragmentation. Glob Ecol. Conserv. 21:e00835. doi: 10.1016/j.gecco.2019.e00835
Xiao, Z., Storms, R., and Tsang, A. (2006). A quantitative starch-iodine method for measuring alpha-amylase and glucoamylase activities. Anal. Biochem. 351, 146–148. doi: 10.1016/j.ab.2006.01.036
Yan, Y., Li, H., Fayyaz, A., and Gai, Y. (2022). Metagenomic and network analysis revealed wide distribution of antibiotic resistance genes in monkey gut microbiota. Microbiol. Res. 254:126895. doi: 10.1016/j.micres.2021.126895
Yang, S., Fan, Z., Li, J., Wang, X., Lan, Y., Yue, B., et al. (2023). Assembly of novel microbial genomes from gut metagenomes of rhesus macaque (Macaca mulatta). Gut Microbes 15:2188848. doi: 10.1080/19490976.2023.2188848
Yang, S., Liu, Y., Yang, N., Lan, Y., Lan, W., Feng, J., et al. (2022). The gut microbiome and antibiotic resistome of chronic diarrhea rhesus macaques (Macaca mulatta) and its similarity to the human gut microbiome. Microbiome 10:29. doi: 10.1186/s40168-021-01218-3
Zengler, K., and Zaramela, L. S. (2018). The social network of microorganisms -how auxotrophies shape complex communities. Nat. Rev. Microbiol. 16, 383–390. doi: 10.1038/s41579-018-0004-5
Zhang, P., Lu, G., Sun, Y., Yan, Z., Dang, T., and Liu, J. (2022). Metagenomic analysis explores the interaction of aged microplastics and roxithromycin on gut microbiota and antibiotic resistance genes of Carassius auratus. J. Hazard. Mater. 425:127773. doi: 10.1016/j.jhazmat.2021.127773
Zhang, Q., Wu, Y., Wang, J., Wu, G., Long, W., Xue, Z., et al. (2016). Accelerated dysbiosis of gut microbiota during aggravation of DSS-induced colitis by a butyrate-producing bacterium. Sci. Rep. 6:27572. doi: 10.1038/srep27572
Zhao, X., Ren, B., Li, D., Xiang, Z., Garber, P. A., and Li, M. (2019). Effects of habitat fragmentation and human disturbance on the population dynamics of the Yunnan snub-nosed monkey from 1994 to 2016. Peer J 7:e6633. doi: 10.7717/peerj.6633
Zheng, D., Liwinski, T., and Elinav, E. (2020). Interaction between microbiota and immunity in health and disease. Cell Res. 30, 492–506. doi: 10.1038/s41422-020-0332-7
Zhu, P., Ren, B., Garber, P. A., Xia, F., Grueter, C. C., and Li, M. (2016). Aiming low: a resident male’s rank predicts takeover success by challenging males in Yunnan snub-nosed monkeys. Am. J. Primatol. 78, 974–982. doi: 10.1002/ajp.22567
Keywords: food provisioning, Yunnan snub-nosed monkey, gut microbiota, antibiotic resistance genes, microbial network
Citation: Li L, Jing S, Tang Y, Li D and Qin M (2024) The effects of food provisioning on the gut microbiota community and antibiotic resistance genes of Yunnan snub-nosed monkey. Front. Microbiol. 15:1361218. doi: 10.3389/fmicb.2024.1361218
Edited by:
Wei Zhu, Chinese Academy of Sciences (CAS), ChinaReviewed by:
Yantian Ma, Nanchang University, ChinaChuanfa Liu, The Chinese University of Hong Kong, China
Copyright © 2024 Li, Jing, Tang, Li and Qin. This is an open-access article distributed under the terms of the Creative Commons Attribution License (CC BY). The use, distribution or reproduction in other forums is permitted, provided the original author(s) and the copyright owner(s) are credited and that the original publication in this journal is cited, in accordance with accepted academic practice. No use, distribution or reproduction is permitted which does not comply with these terms.
*Correspondence: Mingsen Qin, cWlubXMwOEBvdXRsb29rLmNvbQ==