- College of Life Science, Sichuan Agricultural University, Ya’an, China
Introduction: The gut microbiota is an emerging frontier in animal research, and researchers are increasingly transparent about its importance to animal health. Reptiles, particularly snakes, have not received the same attention given to other vertebrates, and the composition of their wild gut microbiome remains understudied.
Methods: In this study, the HiSeq high-throughput sequencing platform was used to sequence and analyze the 16S rRNA V4 region of the gut microbiota of three species (Gonyosoma coeruleum, Rhabdophis pentasupralabralis, Rhabdophis tigrinus).
Results: This study investigated alpha diversity analysis and showed that the gut microbiota richness of RP was significantly higher than that of the other two snakes. The dominant genus of Gonyosoma coeruleum (GC) and Rhabdophis tigrinus (RT) is Cetobacterium, while Enterobacteriaceae; g_uncultured is the dominant genus of Rhabdophis pentasupralabralis (RP). Tree clustering based on Bray-Curtis distances and Jaccard similarity coefficients indicated that the gut microbiota composition of RP and RT was more similar. The unique diet of RP promotes a diverse, competitive gut microbiota, while GC and RT displayed more stable networks linked to shared dietary habits. The functional heat map showed that the predicted functions of the gut microbes of the three snake species were different. These findings suggest that dietary preferences exert a stronger influence on gut microbial composition and function than host genetic background, and distantly related species with similar diets exhibit convergent gut microbiota characteristics.
1 Introduction
The intestinal tract of animals has a complex and diverse bacterial community whose functions vary from nutrient absorption to disease regulation, even influencing animals’ daily behavior and speciation (Dominguez-Bello et al., 2019). Simultaneously, gut microbiota composition is influenced by various factors, including species diversity, dietary patterns, environmental conditions, seasonal variations, and ecological niches (Xiao et al., 2022). Complex interactions exist between intestinal microorganisms, their metabolites, and host cells. Among them, short-chain fatty acids are the most crucial bacterial metabolites as they serve as direct energy sources for host cells, thereby stimulating the production of intestinal hormones and regulating food intake in the brain (Cani et al., 2019). Moreover, the high-protein diet of carnivores can enhance amino acid metabolism by intestinal microbes, leading to short-chain fatty acid (SCFA) production. This process may support intestinal barrier function, thereby aiding the host in adapting to a high-protein diet (Neis et al., 2015). Snakes, as quintessential carnivorous reptiles, possess protein-rich, carbohydrate-poor diets, along with a distinctive intermittent feeding pattern. Their digestive systems adhere to Krogh’s principle of comparative physiology, enabling highly efficient nutrient absorption (Glaudas et al., 2019). For instance, in Python bivittatus digestion, Firmicutes abundance and diversity exhibit significant increases (Costello et al., 2010). Energy metabolism-related enzymes produced by Fusobacteria, Bacteroidetes, and Firmicutes contribute to the degradation of various macromolecules in food, playing a pivotal role in nutrient availability for the host.
Recent studies have revealed that dietary habits and host genetics are the primary factors shaping reptile gut microbiota, exerting a significantly greater influence than environmental variables or conservation status. Reptilian gut microbiota exhibits relatively stable core components, predominantly composed of Bacteroidetes, Proteobacteria, and Firmicutes (Hoffbeck et al., 2023). Multiple studies indicate that host genetic background, whether through maternal transmission or genotype-environment interactions, profoundly influences microbial community structure (Goodrich et al., 2014). For instance, comparisons of farm-raised Naja atra, Ptyas mucosa, Elaphe carinata, and Deinagkistrodon acutus fed either chicken or mice have demonstrated that host species significantly shape the taxonomic composition and diversity of gut microbiota (Zhang et al., 2019). However, cross-species analyses suggest that dietary heterogeneity exerts a stronger influence on microbiota composition than phylogenetic relatedness (Barelli et al., 2015). A global meta-analysis of 113 vertebrate species further highlighted a strong correlation between host dietary patterns and gut microbiome variation (Xie et al., 2024). Dietary factors drive the adaptive evolution of microbial communities through selective pressures, with the host diet potentially playing a more dominant role than phylogenetic relationships (Jiang et al., 2017). Comparative studies have shown that, relative to herbivorous turtles, Caretta caretta shares more gut microbiota features with carnivorous marine mammals (Biagi et al., 2019). Additionally, Gekko japonicus exhibits significant differences in gut microbiota diversity between captive and wild populations, further implicating diet as a key determinant of microbial composition (Jiang et al., 2023). However, our understanding of differences in the composition and function of gut microbes in wild snakes caused by host genetics and dietary preferences is still limited, but it is of great significance for biodiversity conservation.
In the present study, we conducted an in-depth analysis of three snake species: Gonyosoma coeruleum (GC), Rhabdophis pentasupralabialis (RP), and Rhabdophis tigrinus (RT). Gonyosoma coeruleum, first described in 2021 (Liu et al., 2021), predominantly inhabits China (Yunnan Province), Vietnam, Thailand, western Malaysia, and southeastern Myanmar, and in 2022, it was thought to be equally distributed in China (Sichuan, Guizhou, and Hainan) (David et al., 2022). It usually inhabits forests in hills and low mountains and feeds mainly on rodents, birds, lizards, and frogs. Rhabdophis pentasupralabialis was named in 1983 (Zhao, 1995). It is a species-group nuchalis, genus Rhabdophis, mainly distributed in China (Sichuan Province; Jiulong City, Yunnan), feeding on earthworms and firefly larvae (Yoshida et al., 2020). Rhabdophis tigrinus is a wide-ranging species of the genus Rhabdophis, with a preference for moist areas, mainly in mountainous, hilly, and plain areas near water, but also far away from water. It prefers wet areas and mainly inhabits near water in mountainous, hilly, and plain areas, but is also found in wet and grassy mountainous areas far from water, mainly feeding on tailless amphibians, occasionally preying on rodents, fish, and birds (He and Yu, 2007; Figure 1A). RT and GC are generalist feeders, preying on vertebrates, whereas RP is a specialist feeder, restricted to invertebrates. All three species belong to the family Colubridae, with RP and RT classified under the genus Rhabdophis, while GC belongs to the genus Gonyosoma. In the phylogenetic tree constructed from seven nuclear loci and five mitochondrial genes, RP and RT are on the same branch, while GC is more distant (Pyron et al., 2013).
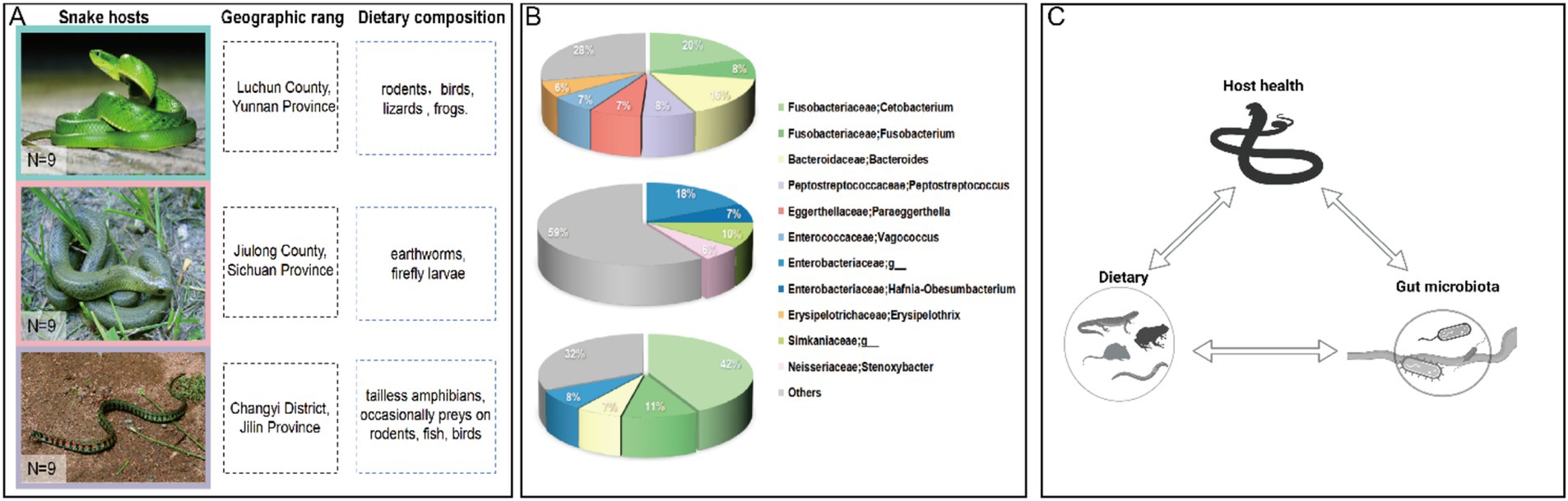
Figure 1. (A) Color-coded photographs of snake species, including GC framed in teal, RP framed in pink, and RT framed in light purple, alongside their respective study sites and dietary compositions (photographs courtesy of Fanyii Lai and our team). (B) Pie charts illustrate the composition of the snake gut microbiome at the genus level. Each color corresponds to specific taxonomic groups: for instance, green represents Fusobacteria, with different green shades denoting various genera within Fusobacteria (“Others” aggregates all microbial genera that did not meet the 5% threshold). (C) A Conceptual Model of Diet-Microbiota-Host Interactions in Snakes was created with BioRender (Song, 2025).
We hypothesize that: (1) Dietary diversity is positively correlated with microbiota α-diversity; (2) Specific prey types promote the enrichment of distinct functional microbial communities; (3) The genetic background of the host establishes the foundational structure of the gut microbiota, upon which diet further modulates microbial function and composition, with both factors collectively determining the final gut microbiota composition in snakes.
2 Materials and methods
2.1 Sample collection and DNA extraction
A total of nine adult male snakes, three for Gonyosoma coeruleum (Colubridae, Serpentes) were captured from Lvchun county, Yunnan province, China, in July 2015; three for Rhabdophis pentasupralabralis (Colubridae, Serpentes) were captured from Jiulong County, Sichuan province, China, in June 2015; and three for Rhabdophis tigrinus (Colubridae, Serpentes) were captured from Changyi District, Jilin province, China, in August 2015 (Figure 1A). The snake sampling procedure was approved by the Institutional Animal Care and Use Committee of the Sichuan Agricultural University (Approval No.20150036). Snakes were transported to the Sichuan Agricultural University laboratory for sampling without feeding during transportation. Before sampling, snakes were manually palpated to confirm no prey remained in the gastrointestinal tract. Aseptic dissection was performed to collect contents from the large intestine, small intestine, and cloaca of each individual. Samples from these three regions were pooled, resulting in 9 composite samples per snake (total n = 27). Specimens were immediately placed into 2 mL sterile collection tubes, snap-frozen in liquid nitrogen, and stored at −80°C. Genomic DNA from the gut tissues was extracted by using a TIANamp Stool DNA Kit (Tiangen Biotech, Beijing). DNA integrity was confirmed by observing high molecular weight bands (>10 kb) on agarose gels, while purity (A260/A280 = 1.8–2.0) and concentration were quantified using a NanoDrop 3,300 (Thermo Scientific). Microbial DNA stability under prolonged −80°C storage has been previously validated (Gavriliuc et al., 2021; Vandeputte et al., 2017), mitigating concerns about degradation in samples collected in 2015.
2.2 16S rRNA gene amplification
The V4 hypervariable region of the 16S rRNA gene was amplified with primers 515F/806R (Suzuki and Nachman, 2016), using a 30 μL reaction containing Phusion® High-Fidelity PCR Master Mix (New England Biolabs), primers (2 μm), and template DNA (10 ng). Cycling: 98°C 1 min; 30 × [98°C 10 s, 50°C 30 s, 72°C 30 s]; 72°C 5 min. Products were verified by 2% agarose gel. Amplicon Sequence Variant (ASV) was generated to classify taxa, replacing traditional Operational Taxonomic Units (OTUs). ASVs resolve single-nucleotide differences, minimize spurious taxa clustering, and enhance reproducibility compared to OTUs’ reliance on arbitrary 97% similarity thresholds. By leveraging ASV, this approach strengthens ecological inference capabilities for complex microbial communities (Callahan et al., 2017).
2.3 Illumina HiSeq platform sequencing
Amplicons (400–450 bp) were gel-purified (Thermo Scientific GeneJET Kit), normalized, and used to construct libraries with the TruSeq DNA PCR-Free Kit (Illumina). Library quality was verified via Qubit® 2.0 (Thermo Scientific) and Agilent Bioanalyzer 2100. Finally, the libraries were sequenced on an Illumina HiSeq2500 platform, generating 250 bp paired-end reads at Novogene (Beijing, China).
2.4 Statistical analysis
Paired-end reads were demultiplexed using sample-specific barcodes, followed by trimming of barcode and primer sequences with Cutadapt v4.0 (Hall and Beiko, 2018). Sequences were merged via FLASH v1.2.11 (Magoc and Salzberg, 2011), and quality filtering was conducted in QIIME2 to generate clean tags. ASV was resolved through DADA2 (Callahan et al., 2016), which implemented rigorous quality control, corrected amplicon errors, and denoised sequences. Chimeric sequences were identified and removed by alignment against the SILVA 132 reference database. Taxonomic annotation employed the Silva 132 database, and the generated BIOM format ASV table is used for more complex data analysis downstream.
The alpha and beta diversity analyses based on ASV tables compared microbial composition and abundance among the three snake species. Beta diversity was quantified using Bray–Curtis distance matrices. Due to microbial community data’s high-dimensional and non-normally distributed nature, nonparametric permutational multivariate analysis of variance (PERMANOVA) was employed as the primary method. In addition, PERMDISP tests were conducted to account for the potential confounding effects of intragroup dispersion on PERMANOVA results. Pairwise comparisons were carried out using ANOSIM with 999 permutations and Benjamini–Hochberg correction for multiple testing, providing quantitative assessments of intergroup differences. All analyses were performed in R, and visualizations were generated using the ggplot2 package (Tang et al., 2016). Phylogenetic trees were reconstructed using the Maximum Likelihood (ML) method based on mitochondrial cytochrome b (cytb) gene sequences (the sequences of RT and RP were measured by our group) from the three snake species (Das, 2006). Individual-level phylogenetic distance matrices were computed using MEGA7 (Kumar et al., 2016). Correlations between host phylogenetic distances and microbial community distances were evaluated via Mantel tests (999 permutations). Alpha diversity and beta diversity were calculated and visualized using R software.
To compare differences in microbiota abundance, the top 10 phyla and top 20 genus abundances were obtained and visualized using bar charts. Differences in the relative abundance of phyla and genera were expressed as mean ± SD, and one-way ANOVA (LSD test, p < 0.05) was used to compare the gut microbiota of different snakes. Significant differences in all genera were also analyzed using online LEfSe (LDA > 4). Membership and structure of samples at ASV were revealed by Venn diagrams and PCoA plots. Microbial functions were predicted using PICRUSt2 v2.3.0 (Douglas et al., 2020) via ASV phylogenetic placement. KEGG pathway predictions were retained only if NSTI scores <0.15. STAMP was used to analyze differential KEGG pathways between groups via two-sided Welch’s t-test (p < 0.05). Venn diagram analysis and visualization were performed using the online platform EVenn (Yang et al., 2024). Linear discriminant analysis (LDA) effect size (Lefse), and histograms were plotted using BioScience Cloud1 (Gao et al., 2024). For the gut bacterial network, we determined significant associations for genera with relative abundance >1% for each species by R4.3.1 using the Spearman correlation test (Bastian et al., 2009). The heatmap of predicted function analysis was performed using the OmicShare tools, a free online platform for data analysis.2
3 Results
3.1 Sequencing data quality of snakes
Following quality control, we obtained 788,913 high-quality reads from 27 intestinal samples. Samples averaged 29,219 reads, generating 4,012 amplicon sequence variants (ASVs). Taxonomic classification identified 35 phyla, 101 classes, 275 orders, 522 families, and 1,127 genera across the three wild snake species. Good’s coverage index exceeded 99.7% for all samples, indicating >99% bacterial community identification (Table 1).
3.2 Comparison of gut microbiota diversity and clustering differences
From Supplementary Figure S1 and Table 1, Observed_species (410.67 ± 244.73), Chao1 (417.99 ± 252.03), and ACE (417.72 ± 251.74) index of intestinal microorganisms in RP were significantly higher than GC (206.11 ± 44.14, 210.28 ± 44.94, 210.60 ± 44.44) and RT (234.67 ± 107.23, 239.39 ± 107.28, 240.40 ± 108.14). Venn analysis revealed 184 unique ASVs in GC compared to 106 in RT. In particular, the number of unique ASVs (955) in RP was significantly higher than in GC and RT. The number of ASVs shared by all snakes is 89 (Figure 2).
To explore the microbial community structure and similarity of different samples, we employed the Bray-Curtis distance and Jaccard similarity coefficients for clustering analyses. Our results showed that the branches of GC and RT showed some clustering in the graph and were separated from RP, possibly reflecting the similarity between GC and RT in terms of colony composition. The RP population was independent as a bifurcation, indicating that the structure of their microbial community was different from that of GC and RT (Figure 3A; Figure 3B). Principal coordinate analysis (PCoA) showed the first two principal coordinates explained 20.29 and 16.29% of the variance. The PCoA plot (Figure 3C) demonstrated significantly greater intragroup similarity compared to interspecific differences, showing clear interspecific clustering patterns. At the 95% confidence level, GC and RT exhibited more similar microbiota profiles than RP. PERMANOVA analysis confirmed significant overall compositional differences among groups (R2 = 0.33; p < 0.001), while PERMDISP (Figure 3D) indicated significant intragroup dispersion heterogeneity (p < 0.05), potentially reflecting individual physiological variation or microenvironmental dynamics. To account for dispersion effects and quantify intergroup differences, pairwise ANOSIM comparisons confirmed significant compositional differences (Figure 3C) for all comparisons (adjusted p < 0.001) with high R values (GC vs. RP: R = 0.78; GC vs. RT: R = 0.75; RP vs. RT: R = 0.77), indicating that intergroup dissimilarity exceeded intragroup variation. Mantel tests revealed a nonsignificant correlation (p = 0.667) between host phylogenetic distance (mitochondrial cytb-based) and microbial Bray–Curtis dissimilarity (Supplementary Figure S2).
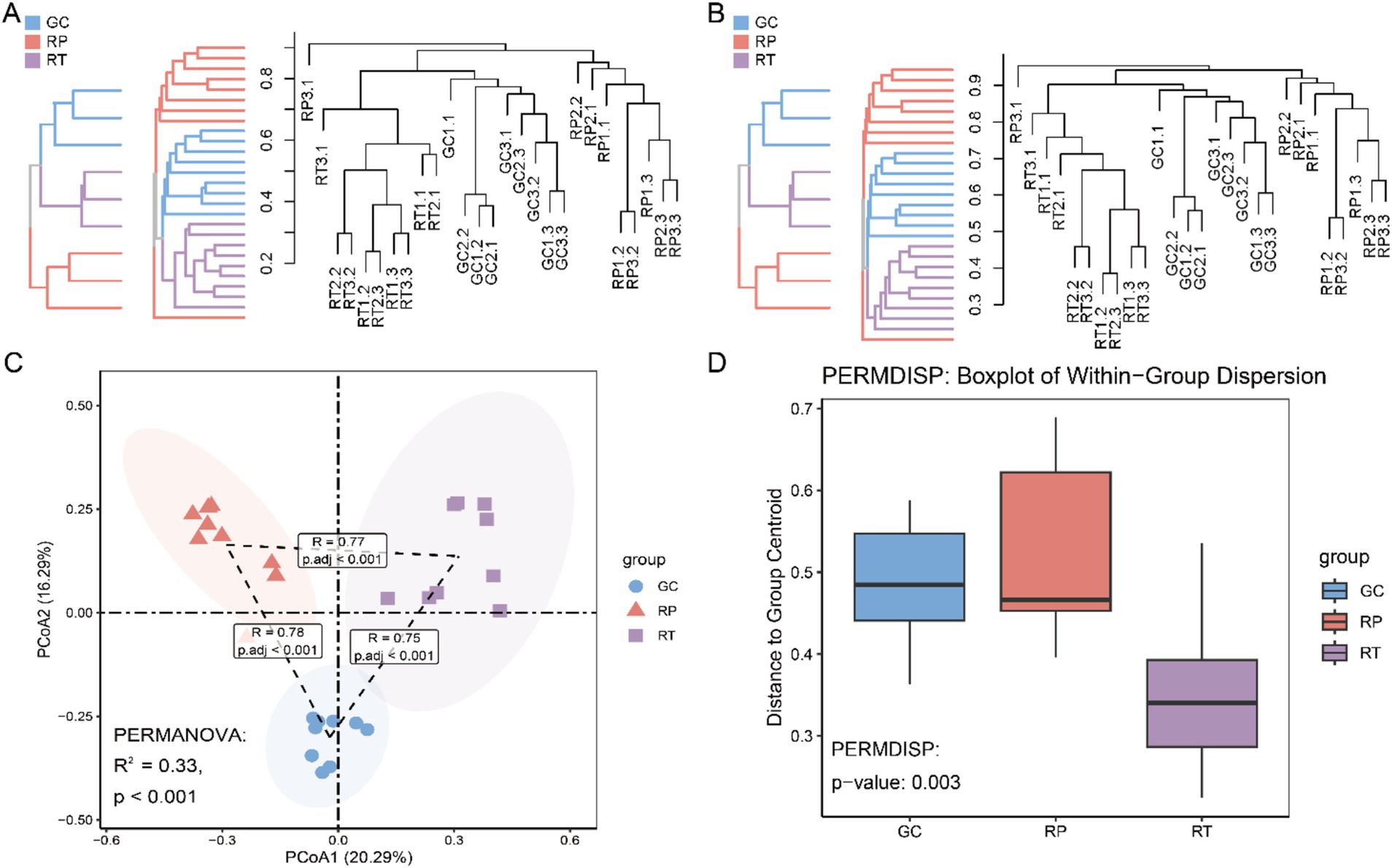
Figure 3. Based on the ASV abundance, UPGMA tree clustering based on (A) Bray-Curtis distance and (B) Jaccard similarity coefficient. (C) PCoA (Principal Coordinates Analysis) of the ASV abundance table, performed with Bray-Curtis distance and a 95% confidence interval. Adonis (PERMANOVA, permutational multivariate analysis of variance) was utilized to assess whether significant distribution differences existed among the three sample groups, supported by pairwise comparisons via ANOSIM. (D) Box plots displaying the outcomes of PERMDISP tests, which evaluate inter-group dispersion differences.
3.3 Bacterial community composition at different taxa levels
At the phylum level, dominant phyla were defined as >30% relative abundance, with subdominant phyla at >10%. The dominant phylum of GC was identified as Firmicutes (31.16% ± 13.29%), followed by Fusobacteria (27.67% ± 22.13%), Bacteroidetes (21.63% ± 5.41%), and Proteobacteria (11.01% ± 3.18%) as subdominant phyla. The dominant phylum of RP was Proteobacteria (42.30% ± 19.39%), with Firmicutes (14.75% ± 4.15%) representing the secondary dominant phylum. RT showed Fusobacteria dominance (54.70% ± 4.15%). The secondary dominant phyla were Proteobacteria (15.57% ± 4.17%), Bacteroidetes (14.49% ± 8.99%), and Firmicutes (13.00% ± 1.70%). (Figure 4A and Supplementary Table S1). LSD post hoc tests revealed significant intergroup differences in the dominant phylum of the three snakes at the phylum level. Fusobacteria abundance was significantly higher in RT versus RP, while Proteobacteria showed significantly greater abundance in RP compared to GC and RT (Supplementary Table S1).
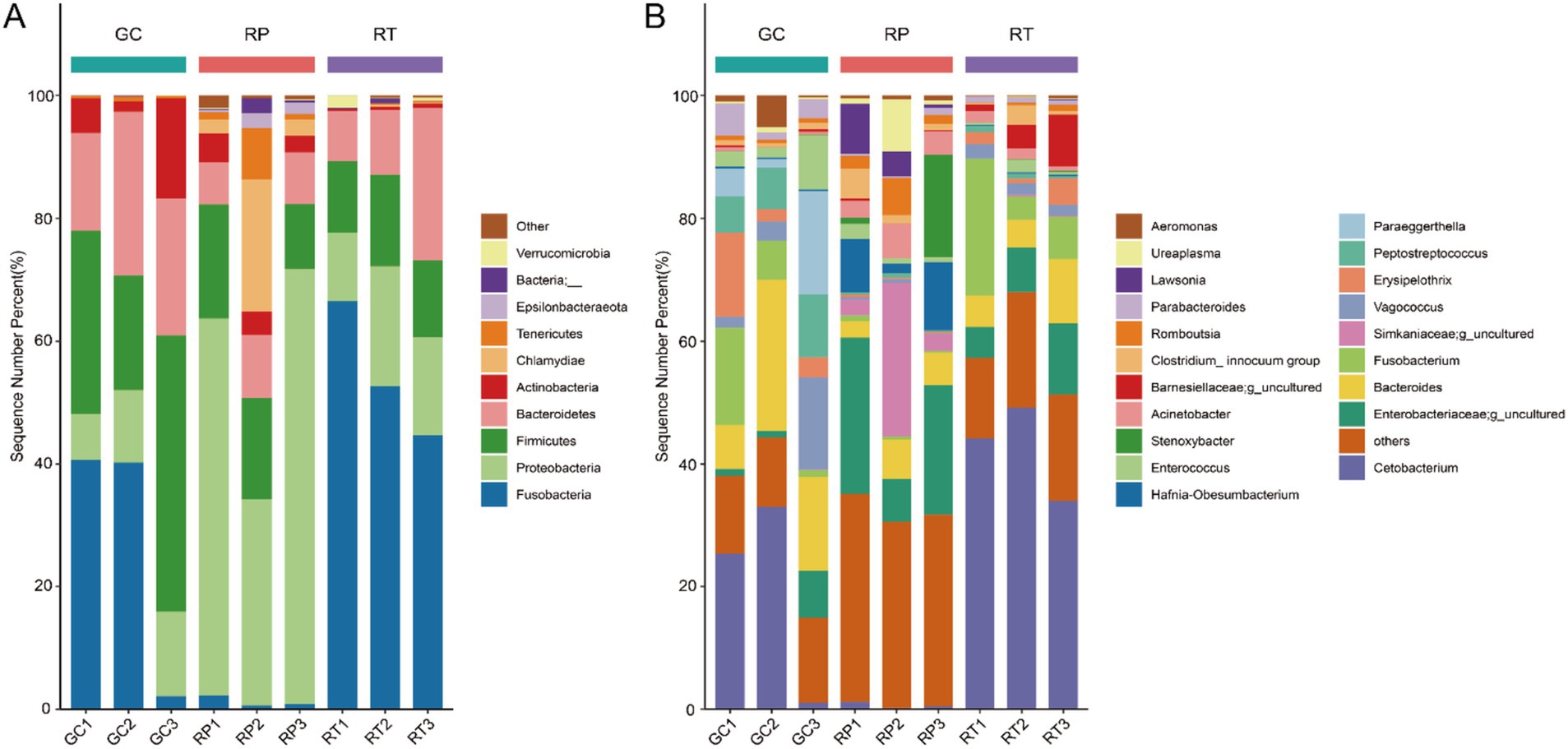
Figure 4. Taxonomic classifications of three groups at the (A) phylum and (B) genus levels. X-axis: three groups. Y-axis: Percent abundance of phylum and genus.
At the genus level, dominant genera were classified at >10% abundance, with subdominant genera >7%. The dominant genera of GC were identified as Cetobacterium (19.80% ± 16.68%) and Bacteroides (15.69% ± 8.72%), while the subdominant genera were Fusobacterium (7.77% ± 7.43%), Peptostreptococcus (7.61% ± 2.23%), and Paraeggerthella (7.58% ± 8.15%). The dominant genera of RP were Enterobacteriaceae; g_uncultured (17.88% ± 9.66%) and Simkaniaceae; g_uncultured (10.07% ± 12.93%), while Hafnia-Obesumbacterium (7.08% ± 9.85%) were the secondary dominant genera. The dominant genera of RT were identified as Cetobacterium (42.42% ± 7.72%) and Fusobacterium (10.99% ± 9.95%), while the secondary dominant genera were Enterobacteriaceae; g_uncultured (7.96%±3.35%) (Figure 4B and Supplementary Table S2).
The LEfSe (LDA > 4, p < 0.05) analysis was performed on all bacteria at the genus level. A total of 16 bacteria with a significant difference were found. Six were found in GC (Bacteroides, Paraeggerthella, Peptostreptococcus, Vagococcus, Erysipelothrix, Enterococcus), and seven were found in RP (Enterobacteriaceae; g_uncultured, Simkaniaceae; g_uncultured, Hafnia_Obesumbacterium, Stenoxybacter, Lawsonia, Ureaplasma, Acinetobacter), and three were found in RT (Cetobacterium, Fusobacterium, Barnesiellaceae; g_uncultured) (Figure 5). Besides that, Cetobacterium is one of 16 top taxa abundant in RT. This finding is also consistent with the changes in Cetobacterium mentioned above.
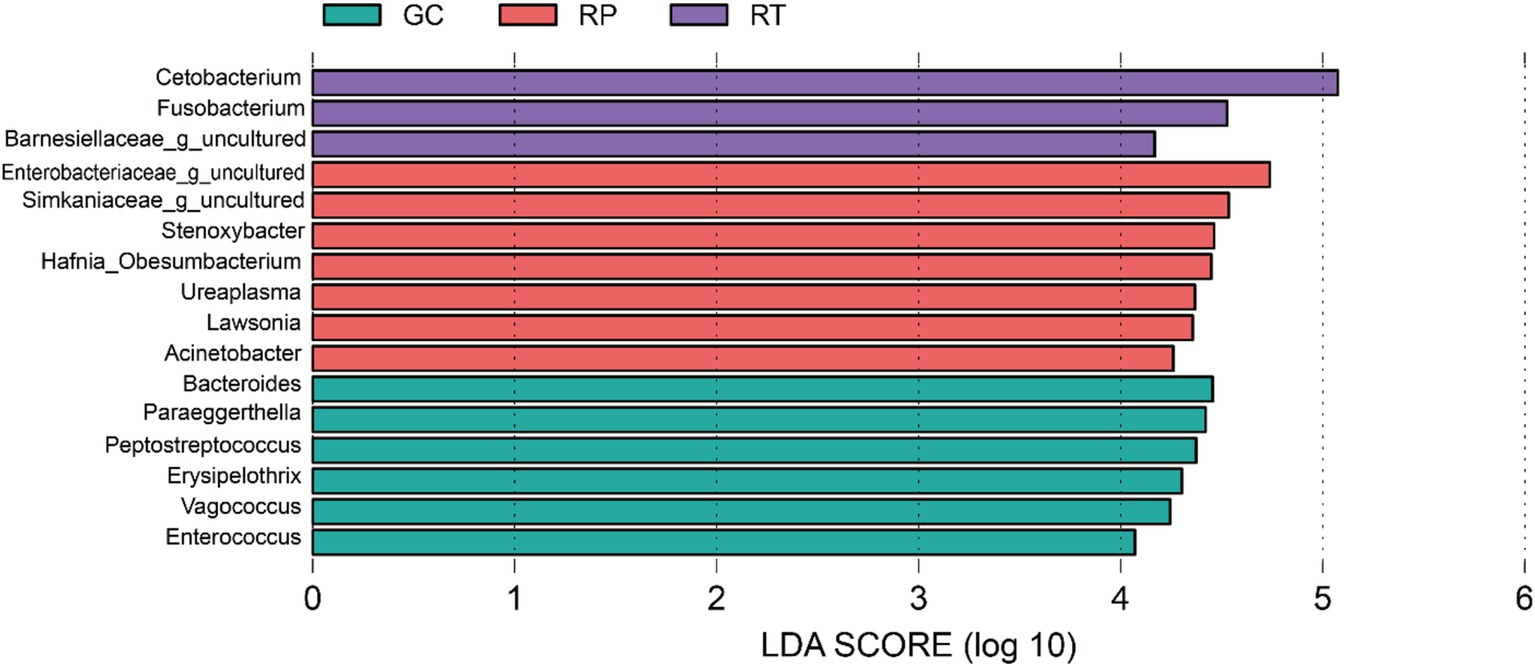
Figure 5. Differentially identified by linear discriminant analysis coupled with effect size (LEfSe).
3.4 Co-occurrence network
To characterize microbial interactions, we constructed genus-level co-occurrence networks based on Spearman correlation analysis (ρ > |0.6|, p < 0.05) for taxa exceeding 1% relative abundance, with node degree filtering (>2 connections). The network’s basic topological properties were then analyzed (Figure 6A and Supplementary Table S3). Among the three snake species, RP exhibited the most complex network, with the highest number of nodes (73), total links (386), negative links (28.76%), and average degree (10.575). This indicates extensive interactions and a highly competitive microbial community. In contrast, GC displayed the smallest network with the fewest nodes, links, and negative associations, suggesting a predominantly collaborative microbial structure. Modular analysis revealed similar modularity across all networks, with GC showing the highest modularity (0.516) and clustering coefficient (0.631), indicating a tendency to form localized cooperative groups.
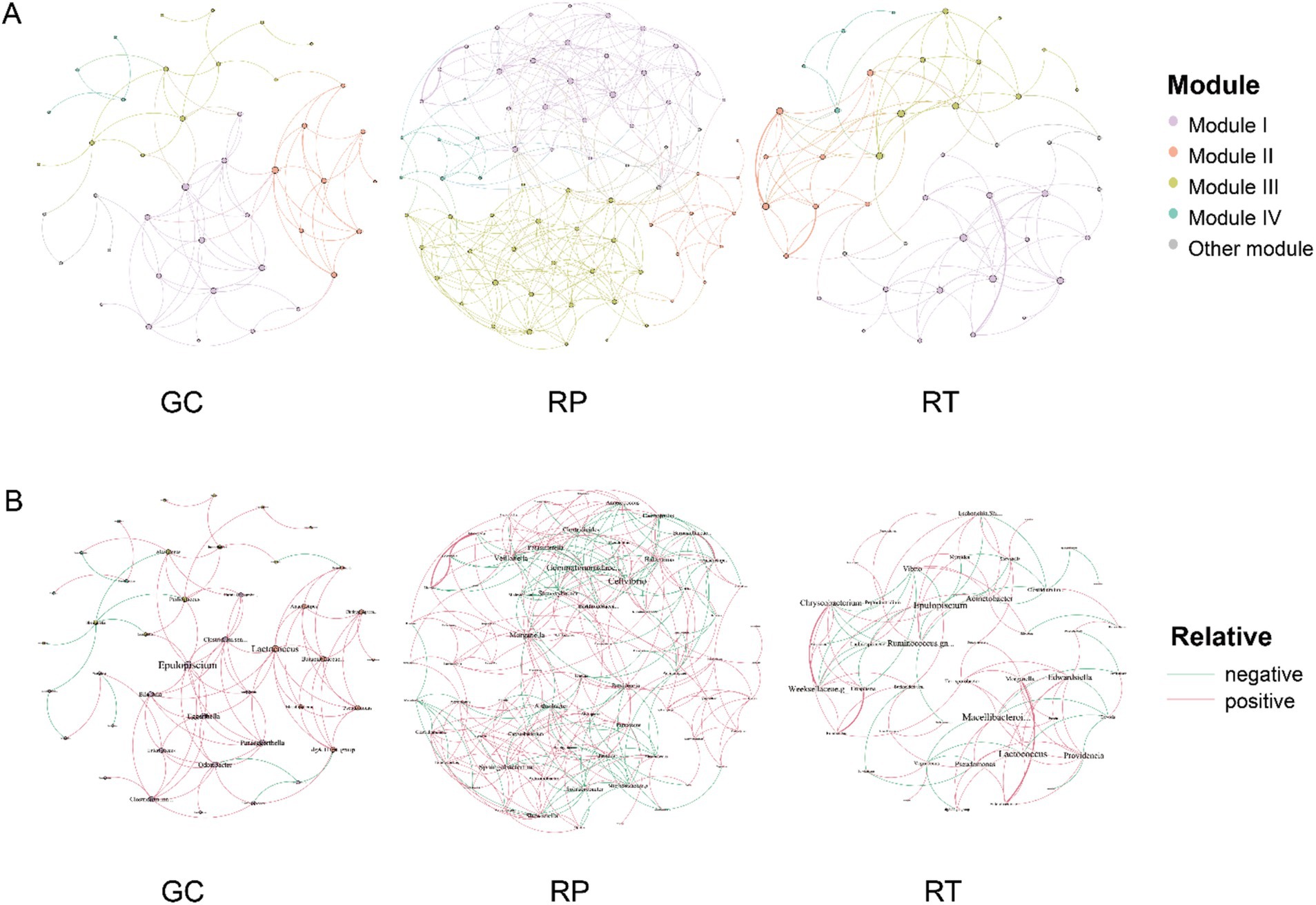
Figure 6. Symbiotic networks of gut bacterial communities: the gut bacterial networks of three snake species are shown: GC, RP, and RT. the networks are based on Spearman’s correlation between enriched taxa (relative abundance of genera >1%), indicating strong connectivity (|r| > 0.6) and significant (p < 0.05) correlation (p< 0.05) correlation. Node and name size are proportional to the number (degree) of links. Network of frog gut samples colored according to modularity (A), with red and green lines indicating positive and negative correlations, respectively (B).
There were variations in the key taxa between networks (Figure 6B). In GC, Epulopiscium (p_Firmicutes), Lactococcus (p_Firmicutes), and Odoribacter (p_Bacteroidetes) were identified as critical taxa. For RP, key genera included Cellvibrio (p_Proteobacteria), Gemmatimonadaceae.g_uncultured (p_Gemmatimonadetes), and Sphingobacterium (p_Bacteroidetes). In RT, Epulopiscium (p_Firmicutes), Lactococcus (p_Firmicutes), and Macellibacteroides (p_Bacteroidetes) played an important role. Proteobacteria and Firmicutes were the two dominant clades in the symbiotic network of GC (37.7%; 35.5%), RP (39.1%; 25.6%), and RT (35.5%; 31.1%). Notably, these dominant taxa mainly interact positively with other genera, highlighting their integrating role in the network.
3.5 Differences in the function of a bacterial community prediction
The heatmap analysis demonstrated higher relative abundances of core metabolic pathways (carbohydrate metabolism, energy metabolism, and metabolism of cofactors and vitamins) and genetic information processing (replication, repair, and translation) in the GC group compared with RP and RT groups (Figure 7A). STAMP analysis further identified distinct functional specializations: (1) RP showed elevated cell motility compared to GC and RT; (2) GC and RT groups displayed higher abundances of signaling molecules and interaction pathways than RP; (3) The RT group demonstrated significantly increased excretory system activity relative to GC, alongside upregulated metabolism of cofactors and vitamins and biosynthesis of secondary metabolites compared to RP (Figure 7B).
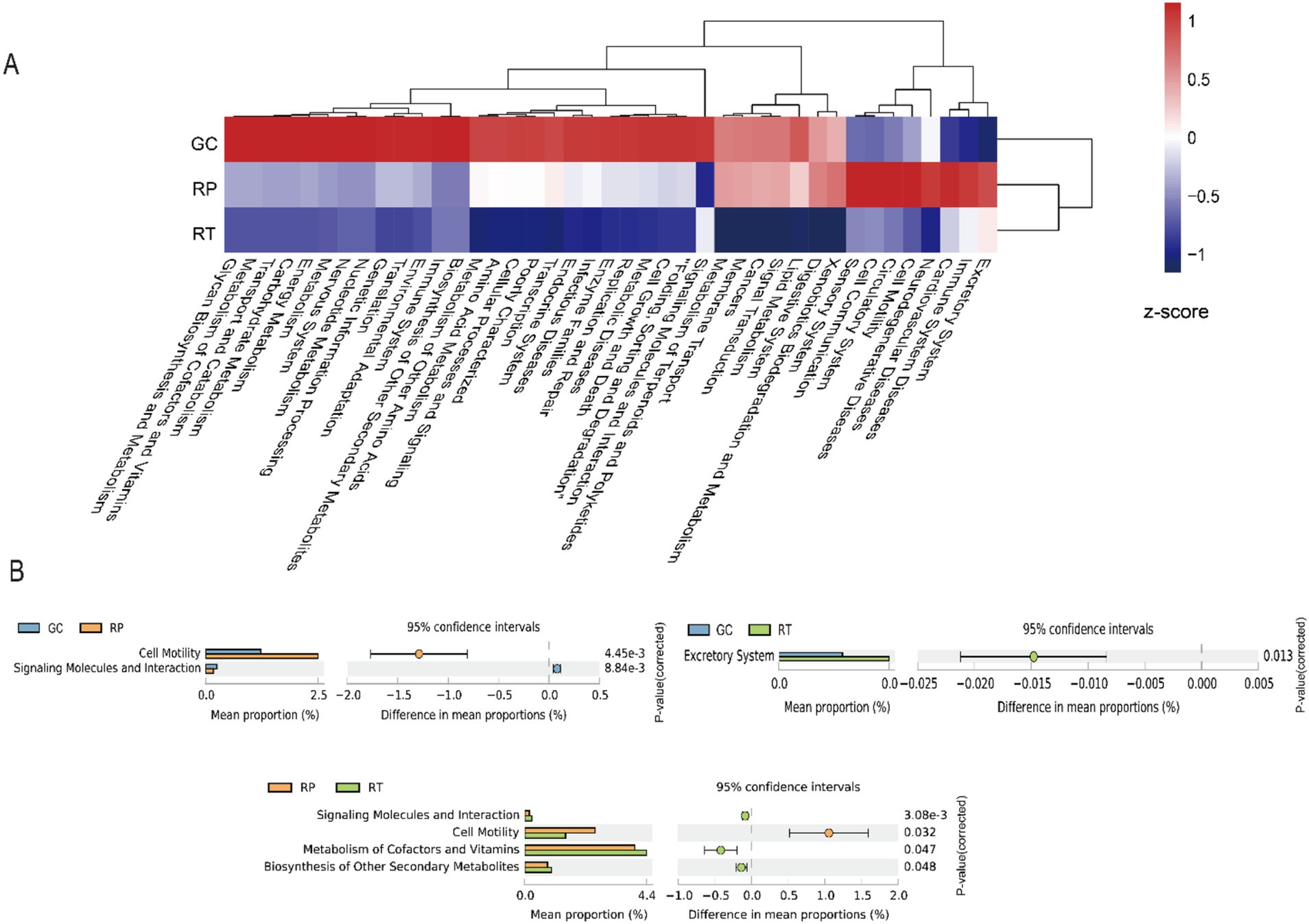
Figure 7. Predicted function pathways analysis among three snakes groups. (A) Heatmap of predicted function pathways. (B) Differences among groups of predicted function pathways were compared through software STAMP and two-side Welch’s t-test (p < 0.05).
4 Discussion
4.1 The relationship between gut microbiota and dietary preference
Human evolutionary studies have revealed that long-term dietary shifts not only shape the adaptive evolution of the host genome but also drive the co-evolution of gut microbial communities. Comparative studies across mammalian species have demonstrated that dietary patterns are a key determinant of gut microbiota composition. For instance, Bacteroides enterotypes exhibit a strong positive correlation with the intake of animal proteins, amino acids, and saturated fats, whereas the abundance of Firmicutes and Proteobacteria is positively associated with dietary fiber consumption (Ley et al., 2008b). Importantly, experimental evidence from other vertebrates underscores that diet exerts a more profound influence on gut microbiota structure than host phylogeny or environmental factors (Colston and Jackson, 2016). Fusobacterium is enriched in captive lizards that feed earthworms, but the abundance of Elizabethkingia, Halomonas, Morganella, and Salmonella in captive lizards that feed loaches is higher (Jiang et al., 2017).
Previous studies have identified Proteobacteria as a common bacterial phylum in various snake species, including Agkistrodon piscivorus (Colston et al., 2015), Crotalus horridus (McLaughlin et al., 2015), Rhabdophis subminiatus (Tang et al., 2019), Laticauda laticaudata, Trimeresurus flavomaculatus, and Boiga dendrophila (Smith et al., 2021), as well as Ptyas mucosus (Wei et al., 2023) all contained Proteobacteria phyla. The dominant bacterial phyla of the three snake species include Proteobacteria and Firmicutes (Figure 1B). Notably, Fusobacteria (54%) was dominant in both RT and GC. RP exhibited a significantly higher abundance of Proteobacteria (42%) compared to GC and RT. This likely relates to their primarily invertebrate-based diet. Indeed, significant differences in microbial diversity and community structure exist between vertebrate and invertebrate hosts (Brooks et al., 2016). Insect hosts typically harbor microbiota dominated by Proteobacteria, a pattern also observed in insectivorous lizards such as Japalura sensu lato, Shinisaurus crocodilurus, Phrynocephalus vlangalii, and Takydromus septentrionalis. Conversely, omnivorous species (e.g., Liolaemus parvus) and herbivores (e.g., Phymaturus williamsi) exhibit lower proportions of Proteobacteria in their intestines (Tian et al., 2022). Proteobacteria not only enhance cellulolytic activity, degrade various aromatic compounds, and promote nutrient absorption but also play a dual role in intestinal immunity (Reid et al., 2011). Under healthy conditions, they contribute to anti-infection and anti-inflammatory processes; however, excessive proliferation can disrupt gut microbial homeostasis, serving as a key marker of dysbiosis (Shin et al., 2015).
Fusobacteria is commonly found in scavengers, being particularly enriched in the hindgut of omnivores and carnivores. For instance, Fusobacteria constitute about 21% of the gut microbiota in black vultures and 31% in turkey vultures. This relationship is mutually beneficial, as Fusobacteria thrive in a protein-rich, anaerobic environment while the scavenger host benefits from the bacterial degradation of carrion to obtain nutrients (Roggenbuck et al., 2014). Fusobacteria are also frequently isolated from infected reptiles (Stevens et al., 2009). Research on the digestive microbiota of Alligator mississippiensis has revealed that Fusobacteria is a core phylum in its gut ecosystem. This bacterial group may contribute to the development of digestive organs and nutrient absorption and is involved in amino acid metabolism. However, it might interfere with the host’s efficiency in protein degradation (Keenan et al., 2013). In carnivorous species such as tigers and lions, Fusobacteria is distinctly more prevalent than in omnivores (e.g., gibbons, golden monkeys, chimpanzees, Assam macaques, giant pandas, black bears, and red pandas), suggesting a close association with a specialized meat-based diet (Chen et al., 2018). Fusobacteria are prevalent in GC and RT. We hypothesize that wild snakes, which typically experience long intervals between meals and consume prey much larger than themselves, may have adapted to support bacteria specialized in carrion digestion. This prolonged digestive process, sometimes lasting several days, could favor the presence of Fusobacteria, which aid in breaking down carrion and efficiently extracting nutrients for the host.
In GC and RT, the dominant bacterial genera were Cetobacterium and Fusobacterium, while Bacteroides was a dominant genus found only in GC. In contrast, Enterobacteriaceae; g_uncultured, and Simkaniaceae; g_uncultured were dominant only in RP. The diets of GC and RT appear more similar, as reflected by the similarities in their dominant gut genera compared to RP. To further investigate how diet differences impact gut microbiota composition among these three snake species, we performed LEfSe analysis to identify genera with higher relative abundance. The results indicated that Cetobacterium, Enterobacteriaceae; g_uncultured, Simkaniaceae; g_uncultured, and Bacteroides were primarily responsible for the observed microbial differences among the three snakes. The Enterobacteriaceae are facultatively anaerobic bacteria capable of both respiratory and fermentative metabolism. Previous studies have reported Enterobacteriaceae in the guts of various earthworm species, where they function as a highly active group within the gut microbiota, particularly in environments lacking added sugars (Meier et al., 2018). Enterobacteriaceae are highly active fermenters in the earthworm gut, utilizing glucose-derived carbon, which suggests that they may also metabolize mucus and plant-derived sugars during gut transit (Wust et al., 2011). Previous studies on snakes have identified Enterobacteriaceae as abundant in species such as Lycodon rufozonatus (Shang et al., 2023), Hydrophis curtus, and H. cyanocinctus (Zhong et al., 2022). Simkaniaceae, a member of the phylum Chlamydiae, was first recognized as a dominant bacterial group in studies of snake microbiota. We hypothesize that Simkaniaceae may play a role in regulating host physiology and the immune response, although its exact ecological functions require further investigation.
Bacteroides is strictly anaerobic, with roles in lactose fermentation and carbohydrate metabolism. These metabolic capabilities allow them to contribute significantly to the digestive processes of both herbivores and carnivores, engaging in multiple metabolic pathways (Turnbaugh et al., 2006). Sceloporus aeneus, S. bicanthalis, S. spinosus, and S. grammicus harbor mainly Bacteroides and Parabacteroides (Hernandez et al., 2024). Dietary habits strongly influence the structure of gut microbial communities; for instance, a carbohydrate-rich diet promotes the growth of Prevotella, whereas a protein- and fat-rich diet supports the proliferation of Bacteroides (Wu et al., 2011). This relationship is further substantiated by the observed increase in Bacteroides within the gut microbiota of animals that consume protein- and fat-rich diets, which reflects the interplay between protein fermentation and carbohydrate metabolism (David et al., 2014). Notably, Bacteroides not only serves as a dominant genus in GC but also shows a high relative abundance in both RP and RT, collectively confirming the carnivorous nature of all three snake species. Interestingly, previous studies have shown that Fusobacteria are commonly found in the intestines of omnivorous and carnivorous freshwater fish, where they play roles in biofilm formation, digestive organ development, and nutrient absorption (Larsen et al., 2014), and function as a dominant phylum in fish gut microbiota (Chen et al., 2018; Eichmiller et al., 2016). In our study, RT exhibited a notable abundance of Fusobacteria, especially Cetobacterium, mirroring findings in fish studies. Cetobacterium is the dominant genus in the gut microbiota of most fish species, such as common carp (Cyprinus carpio) (van Kessel et al., 2011), and Nile tilapia (Oreochromis niloticus) (Ray et al., 2017). Cetobacterium metabolites, particularly short-chain fatty acids (SCFAs) like acetate, propionate, and butyrate, along with vitamin B12, have been shown to benefit fish health (Bhute et al., 2020; Wang et al., 2021; Xie et al., 2021).
4.2 Cluster perspective: the effect of dietary preference on the gut microbiota
Although dietary diversity is generally assumed to correlate positively with gut microbial α-diversity, our data reveal that the obligate feeder RP exhibits significantly higher Observed_species, Chao1, and ACE indices than the vertebrate-feeding GC and RT. This unexpected pattern likely reflects the metabolic demands associated with specialized feeding strategies, particularly the degradation of complex chitin in invertebrate exoskeletons. Statistical validation via PERMANOVA (R2 = 0.33, p < 0.001) confirmed significant microbial differentiation among species. Notably, this evolutionary convergence parallels findings in myrmecophagous mammals (Delsuc et al., 2014) and amphibians (Brunetti et al., 2023), suggesting that dietary specialization promotes cross-species functional convergence through either elevated α-diversity or conserved enrichment of core degradative taxa (e.g., chitinolytic communities).
Host genetics, dietary divergence, and physiological traits all influence gut microbiota composition (Benson et al., 2010; Moeller et al., 2020; Spor et al., 2011). However, our study demonstrates that dietary divergence plays a dominant role in snakes. Take the genus Elaphe as an example: despite the high morphological and habitat similarity between E. schrenckii and E. anomala and their historical classification as conspecific subspecies, significant genus-level microbiota differences exist between them (Lu et al., 2019). This pattern of dietary influence extends even to phylogenetically distant species, as hosts with similar diets exhibit convergent microbial communities (Brooks et al., 2016). The dendrogram based on ASVs demonstrated that RT and GC shared more similar microbiota compared to RT and its conspecific RP. Moreover, the Mantel test indicated no significant correlation between host phylogenetic distance (based on the cytb gene) and microbiota dissimilarity (p = 0.667). This is consistent with our PCoA results, where PCoA2 effectively distinguishes RT from the other species due to its elevated abundance of Cetobacterium, a genus associated with aquatic prey consumption. In contrast, GC clusters along PCoA1 are driven by its higher proportion of Bacteroides, which reflects its vertebrate-based diet. Interestingly, Cetobacterium is also a dominant genus in GC, potentially due to the following factors: (1) All samples were collected during the rainy season, a period of increased amphibian activity that may have led GC to consume more frogs. (2) Prey size in the GC and RT groups was significantly larger than in the RP group. Their intermittent feeding strategy may favor symbiont colonization, as frequent feeding-digestion cycles tend to select for rapidly growing taxa (e.g., Enterobacteriaceae), whereas intermittent feeding supports the proliferation of symbiotic bacteria such as Cetobacterium. In summary, the specialized feeding behavior of RP not only enhances its gut microbial α-diversity but also drives functional specialization within its microbiome, leading to significant compositional differences compared to closely related species that predominantly consume vertebrates. The mechanism by which dietary specialization promotes the convergent evolution of microbial functions appears to be conserved across different taxonomic groups, as evidenced in myrmecophagous mammals and amphibians (Brunetti et al., 2023; Delsuc et al., 2014), thereby offering a novel perspective for understanding host-microbe co-evolution.
4.3 Diet-microbiota network associations in wild snakes
Network analysis provides new perspectives on microbial interactions (Grond et al., 2019), and differences in diet can lead to significant changes in gut microbial diversity and network structure (Ley et al., 2008a; Youngblut et al., 2019). Additionally, the study demonstrates that diet types (herbivorous, omnivorous, and carnivorous) play a dominant role in the gastrointestinal microbiome networks of Nematoda, Arthropoda, and Chordata, with their impact being significantly greater than host phylogeny (Ma and Shi, 2024). Network stability can be quantitatively assessed using modularity and the negative edge ratio (Hernandez et al., 2021; Newman, 2006). High modularity is positively correlated with ecological network stability (Newman, 2006), whereas a low negative edge ratio reduces competitive interference, facilitating host adaptation to environmental fluctuations (Grilli et al., 2016). Although not in the same genus, GC and RT exhibited highly stable microbial networks potentially attributable to their overlapping feeding strategies (vertebrate prey species). The observed dietary diversification appears to promote synergistic inter-colony interactions (91.11% positive links in GC vs. 76.1% in RT) and may contribute to enhanced modular structures (modularity > 0.5). Conversely, RP’s specialized diet (earthworms vs. fireflies exclusively) coincided with heightened inter-colony competition (28.76% negative interactions), forming a complex network (73 nodes, 386 links) with reduced modularity (0.498) that demonstrated comparatively lower stability than GC/RT. These patterns may indicate that dietary diversity supports colony stability, while dietary similarity could drive functional convergence of microbial communities across phylogenetically divergent hosts - a phenomenon analogous to that reported in migratory birds (Wang et al., 2022). Our findings align with the hypothesis that the host diet selects key species to establish stable functional taxa (Youngblut et al., 2019), though internal resource competition within these taxa might modulate network resilience (Feng et al., 2017). Notably, GC and RT shared core hub taxa Epulopiscium (Firmicutes) and Lactococcus (Firmicutes), which could maintain host homeostasis through putative nutrient decomposition (Parata et al., 2020) and metabolic regulation (Li et al., 2023). The RP network displayed the smallest diameter (6) and relatively low density (0.147), suggesting a configuration where centralized microbial connectivity might be particularly vulnerable to keystone node perturbations (e.g., Cellvibrio, Sphingobacterium). Cellvibrio and Sphingobacteriaceae are jointly involved in initial chitinolysis to provide energy to the host (Wieczorek et al., 2019), potentially supplying energy substrates for the host. Monitoring abundance fluctuations in these core microbiota (e.g., Cellvibrio, Sphingobacterium) may provide insights for ecological risk assessment in RP systems.
4.4 The function composition of the gut microbiota to diet
KEGG pathway analysis indicated that RT exhibits enhanced capabilities in cofactor/vitamin metabolism and secondary metabolite biosynthesis, which may reflect its adaptation to high-protein diets. For example, the enrichment of Cetobacterium somerae in its gut could support host hemoglobin production via vitamin B12 synthesis (Qi et al., 2023), although this function requires validation through the host erythrocyte parameters. In contrast, the relatively low abundance of signal molecule interaction pathways in RP might indicate a need for a stable gut microbial community. Moreover, the slightly higher abundances of amino acid metabolism and environmental adaptation functions observed in the gut microbiota of GC likely reflect the metabolic demands associated with processing the diverse components of terrestrial prey. Similar patterns have been observed in other systems. For instance, the functional divergence between the gut microbial communities of baleen and toothed whales (Sanders et al., 2015) suggests that substrate types transmitted through food chains (e.g., krill as the primary food for baleen whales, which in turn feed on phytoplankton) may drive metabolic specialization. In snakes, differences in prey origin, such as the ingestion of insect exoskeletons rich in chitin, may influence microbial functions through the food chain dynamics. Notably, the host genetic background may influence microbial functions by modulating the gut’s physical environment (Figure 1C). For instance, the enrichment of cell motility functions in RP might be linked to its frequent feeding and digestive cycles. Similarly, the persistence of carnivorous gut characteristics during the dietary transition in giant pandas suggests that host genes constrain microbial functions (Hu et al., 2017; Nie et al., 2019). It is plausible to infer that the food chain plays a significant role in shaping gut microbial composition, much like the evolutionary transition of the giant panda’s carnivorous gut into a specialized bamboo-based system—a process reflecting the long-term co-evolution of dietary habits and species.
Limited by the elusive behavior and capture difficulty of wild snakes, we obtained only three biological replicates per species (n = 3). While this small sample size aligns with precedents (Colston et al., 2015; Li et al., 2021; Tang et al., 2019; Zhang et al., 2024), it may constrain statistical power and generalizability. Furthermore, by focusing exclusively on carnivorous snakes (GC, RT, RP), our findings may lack broader relevance compared to studies across dietary guilds. Nevertheless, our data suggest a predictive link between microbial networks and snake dietary specificity, though experimental validation is required to confirm causality. Additionally, using PICRUSt2 on 16S rRNA data infers only potential metabolic capabilities rather than actual activities or host–microbe interactions. For example, although RT showed predicted enrichment in excretory system functions (e.g., the urea cycle), validation through serum urea level measurements or assessments of intestinal ammonia concentrations is required. Future studies should integrate multi-omics approaches, such as metagenomics and metabolomics, to elucidate the quantitative relationships between dietary components and microbial network parameters, thereby unraveling the link between dietary specificity and microbial adaptation. Such an approach would further validate the functionality of the gut microbiota and explore its ecological roles.
5 Conclusion
This study analyzed the gut microbiota of three snake species, revealing distinct microbial compositions and network structures. The invertebrate-feeding RP exhibited higher alpha diversity, although its less stable microbial network was more susceptible to prey scarcity. Network analysis highlighted differences in key taxa: Epulopiscium and Lactococcus were identified as keystone taxa in GC and RT; their presence and abundance underscore the critical role of dietary preferences in shaping gut microbial communities. Cellvibrio and Sphingobacterium in RP could serve as monitoring indicators. Functional predictions further reflected adaptations of the gut microbiota to distinct ecological niches, suggesting that dietary habits influence microbial assemblages through food chain dynamics.
Data availability statement
The original contributions presented in the study are publicly available. This data can be found here: https://www.ncbi.nlm.nih.gov/, accession number: PRJNA1191237.
Ethics statement
The animal study was approved by the Sichuan Agricultural University Animal Ethical and Welfare Committee. The study was conducted in accordance with the local legislation and institutional requirements.
Author contributions
GZ: Data curation, Funding acquisition, Resources, Supervision, Writing – original draft, Writing – review & editing. HS: Data curation, Formal analysis, Investigation, Visualization, Writing – original draft, Writing – review & editing. MD: Data curation, Investigation, Writing – review & editing. JW: Data curation, Investigation, Resources, Writing – review & editing. JL: Data curation, Investigation, Resources, Writing – review & editing. SY: Data curation, Investigation, Resources, Writing – review & editing. FW: Investigation, Methodology, Writing – review & editing. JJ: Investigation, Methodology, Writing – review & editing. JC: Investigation, Methodology, Writing – review & editing. WT: Investigation, Methodology, Writing – review & editing.
Funding
The author(s) declare that financial support was received for the research and/or publication of this article. This study was supported by Zhu GX with a grant from the National Natural Science Foundation of China (NSFC 32270477). Additional support was provided to Zhu GX by a grant from the Sichuan Provincial Natural Science Foundation (2025ZNSFSC0280).
Conflict of interest
The authors declare that the research was conducted in the absence of any commercial or financial relationships that could be construed as a potential conflict of interest.
Generative AI statement
The author(s) declare that no Gen AI was used in the creation of this manuscript.
Publisher’s note
All claims expressed in this article are solely those of the authors and do not necessarily represent those of their affiliated organizations, or those of the publisher, the editors and the reviewers. Any product that may be evaluated in this article, or claim that may be made by its manufacturer, is not guaranteed or endorsed by the publisher.
Supplementary material
The Supplementary material for this article can be found online at: https://www.frontiersin.org/articles/10.3389/fmicb.2025.1559646/full#supplementary-material
Footnotes
References
Barelli, C., Albanese, D., Donati, C., Pindo, M., Dallago, C., Rovero, F., et al. (2015). Habitat fragmentation is associated to gut microbiota diversity of an endangered primate: implications for conservation. Sci. Rep. 5:14862. doi: 10.1038/srep14862
Bastian, M., Heymann, S., and Jacomy, M. (2009). Gephi: an Opensource software for exploring and manipulating networks. Proc. Int. Aaai Conf. Web Soc. Media 3, 361–362. doi: 10.1609/icwsm.v3i1.13937
Benson, A. K., Kelly, S. A., Legge, R., Ma, F., Low, S. J., Kim, J., et al. (2010). Individuality in gut microbiota composition is a complex polygenic trait shaped by multiple environmental and host genetic factors. Proc. Natl. Acad. Sci. USA 107, 18933–18938. doi: 10.1073/pnas.1007028107
Bhute, S. S., Escobedo, B., Haider, M., Mekonen, Y., Ferrer, D., Hillyard, S. D., et al. (2020). The gut microbiome and its potential role in paradoxical anaerobism in pupfishes of the Mojave Desert. Anim. Microbiome. 2:20. doi: 10.1186/s42523-020-00037-5
Biagi, E., D'Amico, F., Soverini, M., Angelini, V., Barone, M., Turroni, S., et al. (2019). Faecal bacterial communities from Mediterranean loggerhead sea turtles (Caretta caretta). Environ. Microbiol. Rep. 11, 361–371. doi: 10.1111/1758-2229.12683
Brooks, A. W., Kohl, K. D., Brucker, R. M., van Opstal, E. J., and Bordenstein, S. R. (2016). Phylosymbiosis: relationships and functional effects of microbial communities across host evolutionary history. PLoS Biol. 14:e2000225. doi: 10.1371/journal.pbio.2000225
Brunetti, A. E., Lyra, M. L., Monteiro, J. P. C., Zurano, J. P., Baldo, D., Haddad, C. F. B., et al. (2023). Convergence of gut microbiota in myrmecophagous amphibians. Proc. Biol. Sci. 290:20232223. doi: 10.1098/rspb.2023.2223
Callahan, B. J., McMurdie, P. J., and Holmes, S. P. (2017). Exact sequence variants should replace operational taxonomic units in marker-gene data analysis. ISME J. 11, 2639–2643. doi: 10.1038/ismej.2017.119
Callahan, B. J., McMurdie, P. J., Rosen, M. J., Han, A. W., Johnson, A. J. A., and Holmes, S. P. (2016). DADA2: high-resolution sample inference from Illumina amplicon data. Nat. Methods 13, 581–583. doi: 10.1038/nmeth.3869
Cani, P. D., Van Hul, M., Lefort, C., Depommier, C., Rastelli, M., and Everard, A. (2019). Microbial regulation of organismal energy homeostasis. Nat. Metab. 1, 34–46. doi: 10.1038/s42255-018-0017-4
Chen, B., Gao, L., and Pan, Q. (2018). Woody forages effect the intestinal bacteria diversity of golden pompano Trachinotus ovatus. AMB Express 8:29. doi: 10.1186/s13568-018-0550-2
Chen, X., Li, Q., Li, G., Xu, F., Han, L., Jiang, Y., et al. (2018). The distal gut bacterial Community of some Primates and Carnivora. Curr. Microbiol. 75, 213–222. doi: 10.1007/s00284-017-1368-x
Colston, T. J., and Jackson, C. R. (2016). Microbiome evolution along divergent branches of the vertebrate tree of life: what is known and unknown. Mol. Ecol. 25, 3776–3800. doi: 10.1111/mec.13730
Colston, T. J., Noonan, B. P., and Jackson, C. R. (2015). Phylogenetic analysis of bacterial communities in different regions of the gastrointestinal tract of Agkistrodon piscivorus, the cottonmouth Snake. PLoS One 10:e128793:e0128793. doi: 10.1371/journal.pone.0128793
Costello, E. K., Gordon, J. I., Secor, S. M., and Knight, R. (2010). Postprandial remodeling of the gut microbiota in Burmese pythons. ISME J. 4, 1375–1385. doi: 10.1038/ismej.2010.71
Das, J. (2006). The role of mitochondrial respiration in physiological and evolutionary adaptation. BioEssays 28, 890–901. doi: 10.1002/bies.20463
David, P., Campbell, P. D., Deuti, K., Hauser, S., Luu, V. Q., Nguyen, T. Q., et al. (2022). On the distribution of Gonyosoma prasinum (Blyth, 1854) and Gonyosoma coeruleum Liu, Hou, ye Htet Lwin, Wang amp; Rao, 2021, with a note on the status of Gonyosoma gramineum Gnther, 1864 (Squamata: Serpentes: Colubridae). Zootaxa 5154, 175–197. doi: 10.11646/zootaxa.5154.2.4
David, L. A., Maurice, C. F., Carmody, R. N., Gootenberg, D. B., Button, J. E., Wolfe, B. E., et al. (2014). Diet rapidly and reproducibly alters the human gut microbiome. Nature 505, 559–563. doi: 10.1038/nature12820
Delsuc, F., Metcalf, J. L., Wegener Parfrey, L., Song, S. J., Gonzalez, A., and Knight, R. (2014). Convergence of gut microbiomes in myrmecophagous mammals. Mol. Ecol. 23, 1301–1317. doi: 10.1111/mec.12501
Dominguez-Bello, M. G., Godoy-Vitorino, F., Knight, R., and Blaser, M. J. (2019). Role of the microbiome in human development. Gut 68, 1108–1114. doi: 10.1136/gutjnl-2018-317503
Douglas, G. M., Maffei, V. J., Zaneveld, J. R., Yurgel, S. N., Brown, J. R., Taylor, C. M., et al. (2020). PICRUSt2 for prediction of metagenome functions. Nat. Biotechnol. 38, 685–688. doi: 10.1038/s41587-020-0548-6
Eichmiller, J. J., Hamilton, M. J., Staley, C., Sadowsky, M. J., and Sorensen, P. W. (2016). Environment shapes the fecal microbiome of invasive carp species. Microbiome 4:44. doi: 10.1186/s40168-016-0190-1
Feng, K., Zhang, Z., Cai, W., Liu, W., Xu, M., Yin, H., et al. (2017). Biodiversity and species competition regulate the resilience of microbial biofilm community. Mol. Ecol. 26, 6170–6182. doi: 10.1111/mec.14356
Gao, Y., Zhang, G., Jiang, S., and Liu, Y. (2024). Wekemo Bioincloud: A user-friendly platform for meta-omics data analyses. iMeta 3:e175. doi: 10.1002/imt2.175
Gavriliuc, S., Stothart, M. R., Henry, A., and Poissant, J. (2021). Long-term storage of feces at −80 degrees C versus −20 degrees C is negligible for 16S rRNA amplicon profiling of the equine bacterial microbiome. Peerj 9:e10837. doi: 10.7717/peerj.10837
Glaudas, X., Glennon, K. L., Martins, M., Luiselli, L., Fearn, S., Trembath, D. F., et al. (2019). Foraging mode, relative prey size and diet breadth: a phylogenetically explicit analysis of snake feeding ecology. J. Anim. Ecol. 88, 757–767. doi: 10.1111/1365-2656.12972
Goodrich, J. K., Waters, J. L., Poole, A. C., Sutter, J. L., Koren, O., Blekhman, R., et al. (2014). Human genetics shape the gut microbiome. Cell 159, 789–799. doi: 10.1016/j.cell.2014.09.053
Grilli, J., Rogers, T., and Allesina, S. (2016). Modularity and stability in ecological communities. Nat. Commun. 7:12031. doi: 10.1038/ncomms12031
Grond, K., Santo Domingo, J. W., Lanctot, R. B., Jumpponen, A., Bentzen, R. L., Boldenow, M. L., et al. (2019). Composition and drivers of gut microbial communities in Arctic-breeding shorebirds. Front. Microbiol. 10:2258. doi: 10.3389/fmicb.2019.02258
Hall, M., and Beiko, R. G. (2018). 16S rRNA gene analysis with QIIME2. Methods Mol. Biol. 1849, 113–129. doi: 10.1007/978-1-4939-8728-3_8
Hernandez, M., Ancona, S., Hereira-Pacheco, S., Diaz De La Vega-Perez, A. H., Alberdi, A., and Navarro-Noya, Y. E. (2024). Seasonal dietary changes relate to gut microbiota composition depending on the host species but do not correlate with gut microbiota diversity in arthropod-eating lizards. Mol. Ecol. 33:e17426. doi: 10.1111/mec.17426
Hernandez, D. J., David, A. S., Menges, E. S., Searcy, C. A., and Afkhami, M. E. (2021). Environmental stress destabilizes microbial networks. ISME J. 15, 1722–1734. doi: 10.1038/s41396-020-00882-x
Hoffbeck, C., Middleton, D. M. R. L., Nelson, N. J., and Taylor, M. W. (2023). 16S rRNA gene-based meta-analysis of the reptile gut microbiota reveals environmental effects, host influences and a limited core microbiota. Mol. Ecol. 32, 6044–6058. doi: 10.1111/mec.17153
Hu, Y., Wu, Q., Ma, S., Ma, T., Shan, L., Wang, X., et al. (2017). Comparative genomics reveals convergent evolution between the bamboo-eating giant and red pandas. Proc. Natl. Acad. Sci. USA 114, 1081–1086. doi: 10.1073/pnas.1613870114
Jiang, X., Dai, Y., Wang, Y., Guo, K., Du, Y., Gao, J., et al. (2023). Dietary and sexual correlates of gut microbiota in the Japanese gecko, Gekko japonicus (Schlegel, 1836). Animals 13. doi: 10.3390/ani13081365
Jiang, H., Ma, J., Li, J., Zhang, X., Li, L., He, N., et al. (2017). Diets Alter the gut microbiome of crocodile lizards. Front. Microbiol. 8:2073. doi: 10.3389/fmicb.2017.02073
Keenan, S. W., Engel, A. S., and Elsey, R. M. (2013). The alligator gut microbiome and implications for archosaur symbioses. Sci. Rep. 3:2877. doi: 10.1038/srep02877
Kumar, S., Stecher, G., and Tamura, K. (2016). MEGA7: molecular evolutionary genetics analysis version 7.0 for bigger datasets. Mol. Biol. Evol. 33, 1870–1874. doi: 10.1093/molbev/msw054
Larsen, A. M., Mohammed, H. H., and Arias, C. R. (2014). Characterization of the gut microbiota of three commercially valuable warmwater fish species. J. Appl. Microbiol. 116, 1396–1404. doi: 10.1111/jam.12475
Ley, R. E., Hamady, M., Lozupone, C., Turnbaugh, P. J., Ramey, R. R., Bircher, J. S., et al. (2008a). Evolution of mammals and their gut microbes. Science 320, 1647–1651. doi: 10.1126/science.1155725
Ley, R. E., Lozupone, C. A., Hamady, M., Knight, R., and Gordon, J. I. (2008b). Worlds within worlds: evolution of the vertebrate gut microbiota. Nat. Rev. Microbiol. 6, 776–788. doi: 10.1038/nrmicro1978
Li, D., Sun, Y., and Xu, F. (2021). Interpopulation differences in the composition and characteristics of fecal microbiota in Ptyas dhumnades. Chin. J. Zoolo. 56, 696–706. doi: 10.13859/j.cjz.202105007
Li, S., Yang, H., Jin, Y., Hao, Q., Liu, S., Ding, Q., et al. (2023). Dietary cultured supernatant mixture of Cetobacterium somerae and Lactococcus lactis improved liver and gut health, and gut microbiota homeostasis of zebrafish fed with high-fat diet. Fish Shellfish Immunol. 142:109139. doi: 10.1016/j.fsi.2023.109139
Liu, S., Hou, M., Lwin, Y. H., Wang, Q., and Rao, D. (2021). A new species of Gonyosoma Wagler, 1828 (Serpentes, Colubridae), previously confused with G. prasinum (Blyth, 1854). Evol. Syst. 5, 129–139. doi: 10.3897/evolsyst.5.66574
Lu, S., Li, P., Lu, Y., Yu, Q., and Zhou, Z. (2019). Fecal microbial diversity of three Elaphe snake species under artificial breeding conditions. Chin. J. Zool. 54, 270–278. doi: 10.13859/j.cjz.201902013
Ma, Z., and Shi, P. (2024). Critical complex network structures in animal gastrointestinal tract microbiomes. Anim. Microb. 6:23. doi: 10.1186/s42523-024-00291-x
Magoc, T., and Salzberg, S. L. (2011). FLASH: fast length adjustment of short reads to improve genome assemblies. Bioinformatics 27, 2957–2963. doi: 10.1093/bioinformatics/btr507
McLaughlin, R. W., Cochran, P. A., and Dowd, S. E. (2015). Metagenomic analysis of the gut microbiota of the timber rattlesnake, Crotalus horridus. Mol. Biol. Rep. 42, 1187–1195. doi: 10.1007/s11033-015-3854-1
Meier, A. B., Hunger, S., and Drake, H. L. (2018). Differential engagement of fermentative taxa in gut contents of the earthworm Lumbricus terrestris. Appl. Environ. Microbiol. 84:16. doi: 10.1128/AEM.01851-17
Moeller, A. H., Ivey, K., Cornwall, M. B., Herr, K., Rede, J., Taylor, E. N., et al. (2020). The Lizard gut microbiome changes with temperature and is associated with heat tolerance. Appl. Environ. Microbiol. 86:10. doi: 10.1128/AEM.01181-20
Neis, E. P. J. G., Dejong, C. H. C., and Rensen, S. S. (2015). The role of microbial amino acid metabolism in host metabolism. Nutrients 7, 2930–2946. doi: 10.3390/nu7042930
Newman, M. E. J. (2006). Modularity and community structure in networks. Proc. Natl. Acad. Sci. USA 103, 8577–8582. doi: 10.1073/pnas.0601602103
Nie, Y., Wei, F., Zhou, W., Hu, Y., Senior, A. M., Wu, Q., et al. (2019). Giant pandas are macronutritional carnivores. Curr. Biol. 29, 1677–1682.e2. doi: 10.1016/j.cub.2019.03.067
Parata, L., Nielsen, S., Xing, X., Thomas, T., Egan, S., and Verges, A. (2020). Age, gut location and diet impact the gut microbiome of a tropical herbivorous surgeonfish. FEMS Microbiol. Ecol. 96. doi: 10.1093/femsec/fiz179
Pyron, R. A., Burbrink, F. T., and Wiens, J. J. (2013). A phylogeny and revised classification of Squamata, including 4161 species of lizards and snakes. BMC Evol. Biol. 13:93. doi: 10.1186/1471-2148-13-93
Qi, X., Zhang, Y., Zhang, Y., Luo, F., Song, K., Wang, G., et al. (2023). Vitamin B (12) produced by Cetobacterium somerae improves host resistance against pathogen infection through strengthening the interactions within gut microbiota. Microbiome 11:135. doi: 10.1186/s40168-023-01574-2
Ray, C., Bujan, N., Tarnecki, A., and Davis, D. A. (2017). Analysis of the gut microbiome of Nile Tilapia Oreochromis niloticus L. fed diets supplemented with Previda® and Saponin. J. Fisheriessci. 11:36. doi: 10.21767/1307-234X.1000116
Reid, N. M., Addison, S. L., Macdonald, L. J., and Lloyd-Jones, G. (2011). Biodiversity of active and inactive bacteria in the gut flora of wood-feeding huhu beetle larvae (Prionoplus reticularis). Appl. Environ. Microbiol. 77, 7000–7006. doi: 10.1128/AEM.05609-11
Roggenbuck, M., Baerholm Schnell, I., Blom, N., Baelum, J., Bertelsen, M. F., Sicheritz-Ponten, T., et al. (2014). The microbiome of New World vultures. Nat. Commun. 5:5498. doi: 10.1038/ncomms6498
Sanders, J. G., Beichman, A. C., Roman, J., Scott, J. J., Emerson, D., McCarthy, J. J., et al. (2015). Baleen whales host a unique gut microbiome with similarities to both carnivores and herbivores. Nat. Commun. 6:8285. doi: 10.1038/ncomms9285
Shang, Y., Zhong, H., Liu, G., Wang, X., Wu, X., Wei, Q., et al. (2023). Characteristics of microbiota in different segments of the digestive tract of Lycodon rufozonatus. Animals 13. doi: 10.3390/ani13040731
Shin, N., Whon, T. W., and Bae, J. (2015). Proteobacteria: microbial signature of dysbiosis in gut microbiota. Trends Biotechnol. 33, 496–503. doi: 10.1016/j.tibtech.2015.06.011
Smith, S. N., Colston, T. J., and Siler, C. D. (2021). Venomous snakes reveal ecological and phylogenetic factors influencing variation in gut and oral microbiomes. Front. Microbiol. 12:657754. doi: 10.3389/fmicb.2021.657754
Song, H. (2025). Confirmation of publication and licensing rights. Available online at: https://BioRender.com/j88i726 (Accessed March 15, 2025).
Spor, A., Koren, O., and Ley, R. (2011). Unravelling the effects of the environment and host genotype on the gut microbiome. Nat. Rev. Microbiol. 9, 279–290. doi: 10.1038/nrmicro2540
Stevens, D. A., Hamilton, J. R., Johnson, N., Kim, K. K., and Lee, J. (2009). Halomonas, a newly recognized human pathogen causing infections and contamination in a Dialysis center three new species. Medicine 88, 244–249. doi: 10.1097/MD.0b013e3181aede29
Suzuki, T. A., and Nachman, M. W. (2016). Spatial heterogeneity of gut microbial composition along the gastrointestinal tract in natural populations of house mice. PLoS One 11:e163720:e0163720. doi: 10.1371/journal.pone.0163720
Tang, Z., Chen, G., and Alekseyenko, A. V. (2016). PERMANOVA-S: association test for microbial community composition that accommodates confounders and multiple distances. Bioinformatics 32, 2618–2625. doi: 10.1093/bioinformatics/btw311
Tang, W., Zhu, G., Shi, Q., Yang, S., Ma, T., Mishra, S. K., et al. (2019). Characterizing the microbiota in gastrointestinal tract segments of Rhabdophis subminiatus: dynamic changes and functional predictions. Microbiology 8:e789. doi: 10.1002/mbo3.789
Tian, Z., Pu, H., Cai, D., Luo, G., Zhao, L., Li, K., et al. (2022). Characterization of the bacterial microbiota in different gut and oral compartments of splendid japalure (Japalura sensu lato). BMC Vet. Res. 18:205. doi: 10.1186/s12917-022-03300-w
Turnbaugh, P. J., Ley, R. E., Mahowald, M. A., Magrini, V., Mardis, E. R., and Gordon, J. I. (2006). An obesity-associated gut microbiome with increased capacity for energy harvest. Nature 444, 1027–1031. doi: 10.1038/nature05414
van Kessel, M. A. H. J., Dutilh, B. E., Neveling, K., Kwint, M. P., Veltman, J. A., Flik, G., et al. (2011). Pyrosequencing of 16S rRNA gene amplicons to study the microbiota in the gastrointestinal tract of carp (Cyprinus carpio L). AMB Express 1:9. doi: 10.1186/2191-0855-1-41
Vandeputte, D., Tito, R. Y., Vanleeuwen, R., Falony, G., and Raes, J. (2017). Practical considerations for large-scale gut microbiome studies. FEMS Microbiol. Rev. 41, S154–S167. doi: 10.1093/femsre/fux027
Wang, A., Zhang, Z., Ding, Q., Yang, Y., Bindelle, J., Ran, C., et al. (2021). Intestinal Cetobacterium and acetate modify glucose homeostasis via parasympathetic activation in zebrafish. Gut Microbes 13, 1–15. doi: 10.1080/19490976.2021.1900996
Wang, B., Zhong, H., Liu, Y., Ruan, L., Kong, Z., Mou, X., et al. (2022). Diet drives the gut microbiome composition and assembly processes in winter migratory birds in the Poyang Lake wetland, China. Front. Microbiol. 13:973469. doi: 10.3389/fmicb.2022.973469
Wei, Y., Zhou, M., Fang, W., Liu, Q., Mao, H., Chen, B., et al. (2023). Differences in the luminal and mucosal gut microbiomes and metabolomes of oriental rat snake (Ptyas mucosus). Appl. Microbiol. Biotechnol. 107, 3257–3271. doi: 10.1007/s00253-023-12524-1
Wieczorek, A. S., Schmidt, O., Chatzinotas, A., von Bergen, M., Gorissen, A., and Kolb, S. (2019). Ecological functions of agricultural soil Bacteria and microeukaryotes in chitin degradation: a case study. Front. Microbiol. 10:1293. doi: 10.3389/fmicb.2019.01293
Wu, G. D., Chen, J., Hoffmann, C., Bittinger, K., Chen, Y., Keilbaugh, S. A., et al. (2011). Linking long-term dietary patterns with gut microbial enterotypes. Science 334, 105–108. doi: 10.1126/science.1208344
Wust, P. K., Horn, M. A., and Drake, H. L. (2011). Clostridiaceae and Enterobacteriaceae as active fermenters in earthworm gut content. ISME J. 5, 92–106. doi: 10.1038/ismej.2010.99
Xiao, K., Liang, X., Lu, H., Li, X., Zhang, Z., Lu, X., et al. (2022). Adaptation of gut microbiome and host metabolic systems to lignocellulosic degradation in bamboo rats. ISME J. 16, 1980–1992. doi: 10.1038/s41396-022-01247-2
Xie, Y., Xu, S., Xi, Y., Li, Z., Zuo, E., Xing, K., et al. (2024). Global meta-analysis reveals the drivers of gut microbiome variation across vertebrates. Imetaomics 1. doi: 10.1002/imo2.35
Xie, M., Zhou, W., Xie, Y., Li, Y., Zhang, Z., Yang, Y., et al. (2021). Effects of Cetobacterium somerae fermentation product on gut and liver health of common carp (Cyprinus carpio) fed diet supplemented with ultra-micro ground mixed plant proteins. Aquaculture 543:736943. doi: 10.1016/j.aquaculture.2021.736943
Yang, M., Chen, T., Liu, Y., and Huang, L. (2024). Visualizing set relationships: EVenn's comprehensive approach to Venn diagrams. iMeta 3:e184. doi: 10.1002/imt2.184
Yoshida, T., Ujiie, R., Savitzky, A. H., Jono, T., Inoue, T., Yoshinaga, N., et al. (2020). Dramatic dietary shift maintains sequestered toxins in chemically defended snakes. Proc. Natl. Acad. Sci. USA 117, 5964–5969. doi: 10.1073/pnas.1919065117
Youngblut, N. D., Reischer, G. H., Walters, W., Schuster, N., Walzer, C., Stalder, G., et al. (2019). Host diet and evolutionary history explain different aspects of gut microbiome diversity among vertebrate clades. Nat. Commun. 10:2200. doi: 10.1038/s41467-019-10191-3
Zhang, B., Ren, J., Yang, D., Liu, S., and Gong, X. (2019). Comparative analysis and characterization of the gut microbiota of four farmed snakes from southern China. Peerj 7:e6658. doi: 10.7717/peerj.6658
Zhang, J., Tan, S., Lyu, B., Yu, M., Lan, Y., Tang, R., et al. (2024). Differences in gut microbial composition and characteristics among three populations of the bamboo Pitviper (Viridovipera stejnegeri). Ecol. Evol. 14:e70742. doi: 10.1002/ece3.70742
Zhao, E. (1995). Discussion on several species and subspecies of snakes in China. J. Railway Teach. Coll. 2, 36–39.
Keywords: gut microbiota, dietary preference, Snake, 16S r RNA, evolution
Citation: Zhu G, Song H, Duan M, Wang J, Luo J, Yang S, Wu F, Jiang J, Chen J and Tang W (2025) Dietary preferences affect the gut microbiota of three snake species (Squamata: Colubridae). Front. Microbiol. 16:1559646. doi: 10.3389/fmicb.2025.1559646
Edited by:
Le Liu, Southern Medical University, ChinaReviewed by:
Tiziana Maria Mahayri, Academy of Sciences of the Czech Republic (ASCR), CzechiaXiaolong Hu, Jiangxi Agricultural University, China
Hong Wu, Tianjin Normal University, China
Copyright © 2025 Zhu, Song, Duan, Wang, Luo, Yang, Wu, Jiang, Chen and Tang. This is an open-access article distributed under the terms of the Creative Commons Attribution License (CC BY). The use, distribution or reproduction in other forums is permitted, provided the original author(s) and the copyright owner(s) are credited and that the original publication in this journal is cited, in accordance with accepted academic practice. No use, distribution or reproduction is permitted which does not comply with these terms.
*Correspondence: Guangxiang Zhu, Wmh1R1gwNzExQDE2My5jb20=
†These authors share first authorship