- 1Industry Commons Foundation, Stockholm, Sweden
- 2Department of Informatics, University of Oslo, Oslo, Norway
- 3Ontology Engineering Group, Polytechnic University of Madrid, Madrid, Spain
- 4Unit for Social Semantics at Insight, University of Galway, Galway, Ireland
One of the greatest challenges in creating effective decision-making systems for connected enterprises is the management of cross-domain information. In manufacturing value networks where supply chains are increasingly intertwined, and closed-loop lifecycle management requires traversing several domains, ontologies are proving to be a reliable reference for cross-domain semantic interoperability. However, ontology development, implementation, and management are fragmented and difficult for new users of ontologies to grasp. This is a significant challenge in environments where ontologies are vital for managing effective data exchanges in complex industrial processes. The OntoCommons project has evolved an ontology ecosystem that aims to lower the entry barrier to using ontologies. Building on this ambition, we present a holistic approach to the integration and management of ontologies horizontally across manufacturing ecosystems, including the creation of reference documentation for manufacturing value networks and related standards, available tools for working with ontologies, and examples of vertical integration of knowledge from application level with domain-level and top-level ontology reference documentation. As a novel research direction, we propose a meta-level approach to ontology-driven knowledge management in manufacturing ecosystems. Based on evidence from recent breakthroughs, we present future and emerging research directions.
1 Introduction
In the context of manufacturing modelling, management and control, there is a long-standing consensus on the importance of collaboration in distributed manufacturing enterprises, with a process view of behaviour and interactions from the highest to the lowest level of manufacturing and integrated decision support systems. For successful Enterprise Integration, “the most promising applications are the improved designs for agile networks of companies which through better reference models can react to market demands quicker and with easier to predict results” (Ollero et al., 2002).
This paradigm has become more pertinent with increasing levels of information complexity, including environmental, organisational and technological factors that need to be taken into account and captured in manufacturing organisations’ decision-making models. Sustainable manufacturing ecosystems rely on the ability to model the entire lifecycle of complex systems for Closed Loop Lifecycle Management (CL2M) of products and materials, and this extends Product Lifecycle Management beyond traditional enterprise boundaries (Kiritsis, 2010). Panetto et al. (2019) respond to these evolving framework conditions by advocating for an integrated theory for the analysis and control of complex interdependent networked systems and suggest the future of manufacturing relies on collaboration networks supported by common reference models.
This approach has recently become a necessity for enterprise resilience in Intertwined Supply Networks (Ivanov and Dolgui, 2020), where production has growing dependencies on other domains. Ensuring resilience in manufacturing value networks requires, therefore, an ecosystemic view of Enterprise Modelling that unites well-informed decision-making, technological harmonisation, and socio-environmental responsibility in a common, multi-enterprise, multi-actor and multi-domain ecosystem (Magas and Kiritsis, 2021). In this paper, we shall refer to this approach as Ecosystem Integration.
Traditional approaches to Enterprise Integration imply a close coupling of the components of the enterprise system, while interoperability in complex product systems relies on a loose coupling that allows the autonomy of parts (Weichhart et al., 2021). In cross-domain industrial ecosystems, such as the Industry Commons, interoperability is the key horizontal enabler, and loose coupling is the default approach for the dynamically interacting constituent parts. This allows components of the ecosystem to be agile and adaptable to ongoing processes and open to coupling on the fly (Magas and Kiritsis, 2021).
Cross-Domain Ecosystem Interoperability (CDEI) relies on standardised reference documentation for knowledge management to support the complexity of dynamic network interactions. Aiming to support Industry Commons, the OntoCommons EcoSystem (OCES1) has been developed to bridge between knowledge domains with a system of translation and referencing that allows for interoperability (Magas and Kiritsis, 2021; Goldbeck et al., 2022). OCES adopts a pluralistic approach to ontological representation with harmonisation applied horizontally across domains and vertically within each domain, top-down from a Top Reference Ontology (TRO) and bottom-up from application demonstrators’ requirements specifications.
The OCES has stimulated the evolution of several novel research directions that consolidate the role of ontologies in Enterprise Integration, including the experiences of the Industrial Ontology Foundry2 since its creation in 2016 and 22 demonstrators developed in the framework of the OntoCommons project from a variety of industrial domains3. Novel ontologies have extended the ecosystem in answer to industry needs and novel framework conditions, including a legal ontology for the management of intellectual property for improved asset management developed as part of the project RE4DY4 and the Supply Chain Reference Ontology developed by the Industrial Ontologies Foundry that allows for an ecosystemic approach to manufacturing enterprise integration (Ameri et al., 2022). These have been further tested through collaborative prototyping and Test and Experimentation Facilities (TEFs) focusing on specific industrial use cases where decision-making at enterprise level is enhanced by access to cross-domain knowledge management and reference documentation. As a knowledge reference model for manufacturing value networks (MVNs), tests have demonstrated the possibility to connect directly from application level to the ontology ecosystem, integrating knowledge across MVNs. In this sense, Ecosystem Integration is achieved when every data node in a cross-domain manufacturing value network can access common knowledge reference models.
This paper presents a series of ontology-driven approaches that contribute to Ecosystem Integration in MVNs. Section 2 presents the efforts towards reference documentation for manufacturing in the Industrial Ontologies Foundry and related standardisation initiatives. Section 3 provides an overview of the available tools for working with ontologies from the perspective of MVNs. Section 4 provides two application use cases that connect knowledge at the application level with domain-level and top-level ontology reference documentation. Section 5 proposes a novel research direction for a meta-level approach to knowledge management in manufacturing ecosystems. The concluding Section 6 presents emerging and future research directions.
2 Industrial Ontologies Foundry (IOF)
The fourth industrial revolution is said to be driven by data collected from all kinds of elements of a value chain, including products, assets and processes, and consequently and continuously transformed to meaningful information, the foundation of responsible and informed decision making. This imposes the following challenges to be addressed by modern industrial information systems:
• Integrate data coming from various heterogeneous sources.
• Capture and explore the meaning of big industrial data and unlock the value of data sharing.
• Implement interoperability among all types of systems used in industry.
• Consider every aspect of the product life cycle from conception, design, and engineering, to manufacturing, supply and demand distribution networks, maintenance, customer service, and end-of-life decommissioning.
• Global manufacturing requires more explicit relations and communication among participants (both human and systems), which requires greater interoperability to allow these relations and communications to operate as expected.
To address these challenges, ontologies are considered the next-generation technology to enable connected information and interoperable industrial applications along a value chain. Although ontologies have been developed in the industrial manufacturing domain in many industrial sectors in the past decade, they have been disparately developed with inconsistent principles and viewpoints. As a result, developed industrial ontologies are incoherent and unsuitable for the connected information, semantic data modelling and interoperability challenges defined above.
With the goal to address these challenges and based on the successful experiences in developing coherent ontologies in the biological and biomedical domain (the OBO Foundry), an initiative called Industrial Ontologies Foundry (IOF) started in December 2016 to repeat the successful story of the OBO Foundry in the industrial manufacturing domain5. Modern advanced manufacturing, particularly with today’s complex cyber-physical product/systems and complex global value chains, requires diverse engineering disciplines, information technology and management, and the integration of Operations Technologies (OT) and Information Technologies (IT) systems.
The first IOF6 workshop was organised in December 2016 at the National Institute of Standards and Technology (NIST), Gaithersburg, USA, followed by weekly conference calls and yearly workshops. Following a workshop in 2017, the IOF charter was drafted and became available on the IOF website7. One of the most important messages from the charter that makes IOF unique from other efforts to create engineering and industrial ontologies is the intention of the IOF for its ontologies to be freely open Industry Commons and Standards. The charter includes not only publishing freely available ontologies but also provides principles, guidelines, and governance processes such that a suite of ontology modules can grow in an interoperable fashion.
The IOF is currently running under the Open Application Group Inc. (OAGi) standards organisation8 and is governed by three kinds of committees: a Governance Board (GB), a Technical Oversight Board (TOB), and Working Groups (WGs). There is 1 GB, one TOB, and as many WGs as the industrial interests of the community initiate and support.
Each WG develops an ontology or a suite of ontologies on a particular matter of Industrial interest. Some WGs or Task Forces may be responsible for developing or adapting domain-independent ontologies such as those for time or units of measurement. Figure 1 shows the types of ontologies anticipated within the IOF ontologies.
Starting from the top, IOF selected BFO (Basic Formal Ontology) as the Top-Level Ontology to be used by Domain Reference Ontologies. BFO is already an ISO standard9.
The IOF Core Mid-Level Ontology has recently been published and successfully used10.
The formations of the WGs are driven by industrial use cases. So far, the following WGs have been formed and are running:
• IOF Core
• Systems Engineering
• Supply Chain
• Maintenance
• Materials Science & Engineering
• Production Planning & Scheduling
• Product Service Systems
• MTConnect
The Supply Chain and Maintenance IOF WGs are almost ready to publish their respective Domain-Specific Reference Ontologies, such as the IOF Supply Chain and Maintenance Ontologies.
More particularly, in Supply Chain operations, Ameri et al. (2022), in their paper, claim that “ontologies can be beneficial in the following ways for the supplier discovery and evaluation use case.
• Decision support/inference: Ontologies can support human experts during the sourcing process by providing answers to various queries about suppliers’ capabilities.
• Semantic integration and unification: Ontologies can help with the semantic integration of heterogeneous manufacturing capability data models generated by dispersed actors and systems. Ontologies can be used to create a controlled vocabulary for tagging and organising large amounts of data pertaining to the capabilities of manufacturing suppliers, thus making query and information retrieval more accurate and intelligent.
• Automation: Ontologies can enable machine agents to actively participate in the supply chain formation process by proving machine-understandable content.”
The IOF community and bodies have very closely collaborated with the OntoCommons community with the common objective of globally agreed Common Industrial Ontologies and associated commonly adopted principles about developing, sharing, maintaining, and using industrial ontologies.
3 Application software for ontology engineering
Application software is intended as computer programs, procedures, rules, and possibly associated documentation and data of an information processing system designed to help users perform particular tasks or handle particular types of problems11,12. As an evolving engineering profession, Ontology engineering has witnessed the emergence of a growing number of applications of its own. They are also frequently and commonly referred to as ontology engineering tools (or ontology development tools, even more casually and verbally), which are computer-based tools that are intended to assist the ontology lifecycle processes. Tools make it possible to automate routine, well-defined actions, which eases the cognitive load on the ontologist and frees them up to concentrate on the creative aspects of the process. Ontology engineering methods are frequently supported by tools, reducing the administrative burden associated with applying the method manually. They meant to make ontology engineering more systematic and range greatly in scope–from addressing an individual task or a single ontology lifecycle phase to covering and encompassing the complete lifecycle management of ontologies.
While there are comprehensive instructions for individual tools (e.g., on the IOF portal13) and numerous research articles on cutting-edge tools, generic technical writings on ontology engineering tools are somewhat scarce. Katifori et al. (2007) and Dudáš et al. (2018) surveyed state-of-the-art ontology visualisation methods and tools. d’Aquin and Noy (2012) provided a survey of the landscape of ontology libraries for finding or publishing ontologies. To the best of our knowledge, no state-of-the-art review focuses on the tools for the entire lifecycle management of ontologies. The rapid rate of change in ontology tools in general is one challenge. Another reason is that it is difficult to give concrete, up-to-date instances as details alter regularly.
Therefore, the purpose of this section is to serve as a compendium and guide to describe application software for ontology engineering that has been developing and evolving over the past few years. Furthermore, as the field of ontology engineering is not static and ever-evolving, tools must, inevitably, advance as the discipline matures. Nevertheless, it constitutes an essential component of meta-support infrastructure for the lifecycle management of ontologies and a valuable characterisation of the ontology engineering profession.
The next subsections describe the categories into which the ontology development tools can be classified. This categorisation and the classification of tools depicted in Figure 2 have been taken from the recent OntoCommons investigation about the ontology reference tooling landscape (Poveda-Villalón et al., 2022). This figure represents, for each ontology development or exploitation activity, the group of tools that can be used during such activity. Within each tool category, some examples of particular tools are provided. The categories of tools are located under each activity in a vertical position. That is, for example, for the “Ontology publication” activity, one may use systems of the category “Repository,” “Publication server,” or “Ontology FAIR validator.” These functionalities might be useful for more than one activity. For example, the category “Ontology editor” tools could be used for the activities included in its “vertical,” that is, they can be used for “Ontology conceptualisation,” “Ontology reuse,” “Encoding,” and “Evaluation.” It should be noted that one category can appear in more than one place in the figure. For example, the category “Repository” can be used both for looking for ontologies during the “Ontology Reuse” activity and for sharing and promoting the ontology in the “Ontology Publication” activity.
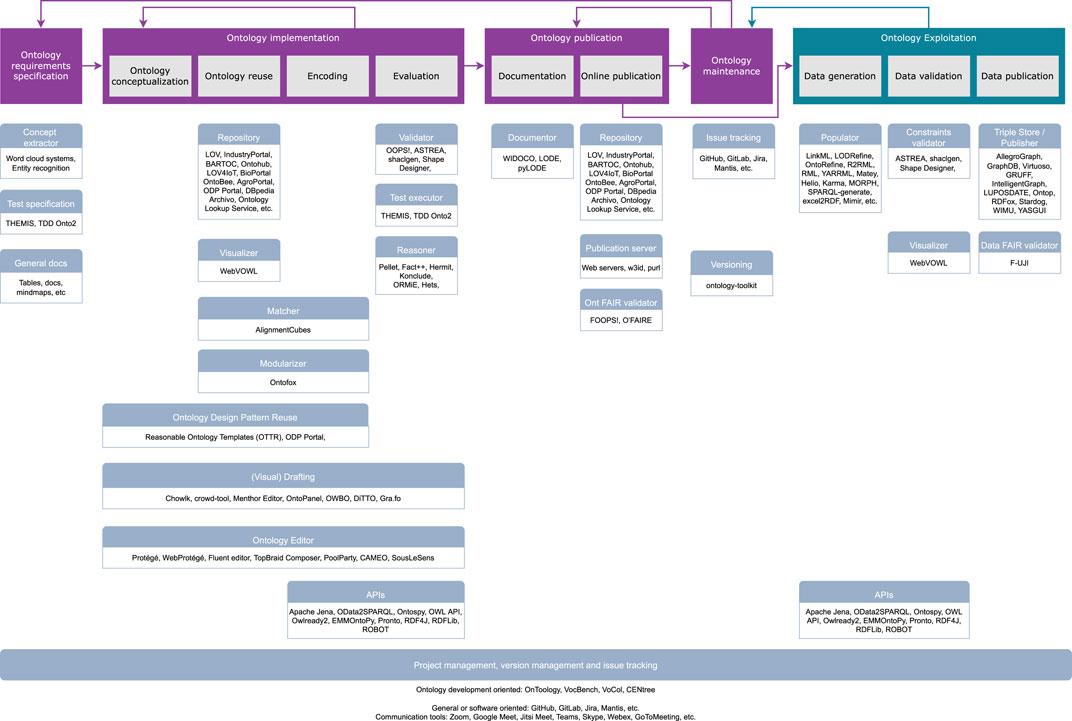
Figure 2. Categorisation of ontology engineering tools. OntoCommons D4.6 (Poveda-Villalón et al., 2022). Existing systems and tools for each category are provided as Supplementary Material to this manuscript.
3.1 Tools for ontology requirement specification
Tools for dealing with ontology requirement specification have been classified into four categories: concept extractors, constraint specification tools, test specification tools, and general documentation tools.
• Concept extractors. These tools can be used for processing long texts or several requirements to rank the most important terms.
• Test specification tools. These tools allow the generation of tests from the ontology requirements to be used later during the ontology evaluation.
• General documentation tools. These tools should be used to generate, store, and share the project documentation as resources, for example, the Ontology Requirement Specification Document or internal documents as meeting notes or annotations.
3.2 Tools for ontology implementation
This topic covers tools for creating and checking ontology designs and constructions.
• Drafting tools. These tools allow the design of ontological models and the generation of the corresponding code in a visual way oriented to graphical notations. Ontology editors providing GUIs are not considered specific visual drafting tools if no edition following a specific graphical notation is supported.
• Editing tools. Ontology editors allow for authoring, generating and modifying OWL and RDF(S) ontologies. Usually, ontology editors include a wide range of plugins or features for ontology authoring-related activities, including the merging and importing other ontologies. These systems can also be used in combination with plugins for specific validators or reasoners or just to verify ontology requirements against the model during the ontology evaluation activity.
• Source editing tools. These tools fetch ontology terms and axioms and support ontology reuse. They often allow users to input terms, fetch selected properties, annotations, and certain classes of related terms from source ontologies and save the results using the RDF/XML serialisation of the OWL.
• Matcher. These tools allow for the generation and exploration of ontology alignments.
• Ontology design pattern reuse. These tools allow for the reuse of ontology design patterns.
3.3 Tools for ontology publication
Ontology publication tools are divided into repository, modulariser, documenter, publication server, and triple store.
• Repository. Ontology repositories allow the registration and/or indexing of ontologies as well as searching and looking up ontology terms.
• Modulariser. These tools aim at generating modules from monolithic ontologies.
• Documenter. These tools facilitate the generation of human-oriented documentation from the OWL ontology code.
• Publication server. A publication server can be used to publish the ontology online following content negotiation mechanisms. In this sense, a general web server and domain configuration could be set up, or systems that facilitate the redirections and provide permanent URIs could be used.
• Triple store. These systems are used to store and optionally publish RDF data. In addition, they allow for querying RDF data. In some cases, the data could be stored in RDF (materialised), or the queries could be resolved in a virtualisation mode from different data sources.
3.4 Tools for ontology maintenance
This topic encompasses tools that are especially important in ontology maintenance where existing ontologies are being modified. Three categories are identified: validator, text executor, and populator.
• Validator. These tools assist ontology developers in evaluating their ontologies, looking for common errors, or checking constraints.
• Test executor. These tools execute the tests written by the tester to check whether the developed modules are providing the expected result as per requirement.
• Populator. These systems are an important support to escort the practitioners by leveraging instantiations in their own knowledge bases for exploitation.
3.5 Tools for ontology evaluation and ontology validation
• Constraint validators. These tools facilitate the validation of RDF data by checking constraints defined in shapes.
• Data FAIR validators. These tools assess the FAIR level of a dataset or to what extent the FAIR principles are met.
• Ontology FAIR validators. These tools assess the FAIR level of an ontology or to what extent the FAIR principles are met.
3.6 Tools for ontology use
Tools for ontology use are subdivided into four categories:
• API. These tools allow for the generation and management of ontologies and data and are normally used during the generation and/or evaluation.
• Query engine. A query engine sits on top of a database or server and executes queries against data.
• Reasoner. These tools facilitate ontology evaluation by looking for potential inconsistencies and incoherencies in the ontologies.
• Visualiser. These systems aim to provide a user-friendly ontology visualisation and navigation. Ontology editors providing GUIs are not considered specific visualisers. However, they can provide plugins for more elaborate visualisations. These systems are normally used to analyse existing ontologies, which is why the related activity is ontology reuse, but they can also be used for ontology documentation.
3.7 Tools for project management, version management and issue tracking
Tools related to project configuration management have been divided into two categories: versioning and issue tracking.
• Versioning. These tools facilitate the creation of versions and dependency handling, which can be both for general project management purposes and specific for ontologies.
• Issue tracking. These tools allow the reporting of bugs, new requirements, or potential enhancements of the ontologies. It should be noted that these systems are also considered for the whole project management, but a particular mention is made for the ontology maintenance activity.
3.8 Requirements following analysis
The review results suggested the need for a single point of entry for ontology tools and progress towards an ecosystem of tools, including:
• a study of the distinct kinds of services that are needed;
• the provision of a theoretical API for each of those services; and
• implementation of a global proof of concept, with each module remaining independent with its own principal investigator.
In ontology engineering work, there is a requirement for a platform to be supported in an integrated environment. We need a way to experiment with relators against the large repositories of knowledge patterns and frames that are currently used for interoperability or are discovered: it is not only a top-down problem. It is important to consider which feature can be taken from general or specific application software and that can be reused for ontology engineering.
As ontologists, we should know that configuration management is more than just managing files. So, not only do we need ontology configuration management tools, but configuration management tools are built using proper ontological analysis of the problem. The need is a software product line for ontology tools from where we could derive products by orchestrating the right tools and managing dependencies between ontology development sub-products.
In general, it can be observed that while a common advantage of ontology development-related tools is their availability as open source and free of charge, there is a lack of integration among most of the available systems. Some systems integrate many features in the form of plugins, such as TopBraid Composer, which provide free support for limited capabilities only. The equivalent version for open source is Protégé. However, since plugins are based on community efforts, no support or update is guaranteed for many plugins.
A trend towards integration systems, oriented to software development best practices, can be observed in the appearance of systems like OnToology, Vocol or VocBench. However, these tools do not completely solve the full integration of capabilities needed when building ontologies, so the definition of such orchestration systems is still an open problem for the community.
4 Referencing top-level ontologies from application level: two industrial use cases
One of the key challenges of OCES industrial use cases has been connecting knowledge at the application level with domain-level and top-level ontology reference documentation14. We present two use cases of industrial applications where we have demonstrated horizontal and vertical ontological alignment for each data instance loaded from production value networks in real-time.
4.1 The RE4DY use case for knowledge management of manufacturing value networks
In the RE4DY project, we started with a simple methodology to tackle the challenges of working with production, product and supply chain data. It was anticipated that a wide range of data types and structures would come into the system and the prototyping environment.
In this prototyping pipeline, we established some foundational concepts to build up to a flexible interface creation process that includes decision-making and change management, perspectives that we need to keep in focus when developing innovative views of the data. The steps include data models, data analysis, decision support, and change management as precursory procedures before exploring and evaluating different interfaces (Figure 3).
We first identify the data models, preparing for all kinds of data from industrial processes and how to bring semantics into the data. This can be related to how data pipelines are created between data lakes and data warehouses, inspired by newer concepts such as data lakehouses being able to move more freely between unstructured and structured data using interoperability and metadata layers.
Different data formats, schemas, and ontologies are explored in the data models stage to link industry knowledge with raw data and map incoming data to existing structures. The aim is to be able to explore data in ways that assist decision-making, such as with business intelligence tools. The prototyping environment should start to load the data to allow for pure explorative and statistical analysis using conventional visualisations, such as charts and diagrams, that can become part of innovative interfaces supporting decision-making.
One interesting concept when analysing data at this stage and connecting it to manufacturing data is the use of unit visualisation. This concept is well-defined in visualisation technology and will give a view where all items can be identified and rearranged according to different settings to give insights. Going from unit and raw data visualisation, one can start to discuss aggregation methods and statistics to be used in indicators. In the next step, we break down the indicators we want to communicate in the end result. In this way, we can evaluate the usefulness of the interfaces together with stakeholders in the context of their business operations at a very early stage. This can be considered part of a decision support system setup where KPIs will be the initial part of a workflow assessing the operation in comparison with different scenarios. The more indicators that are involved, the more challenges will be put on the input and output of the prototype to communicate different trade-offs of the different measures.
The change management perspective is often complicated and overlooked in data pipelines, resulting in a lot of work needing to be repeated. Changes occur in the information models, ontologies, the raw data, indicator settings, etc. It is recommended to build a timeline from the start to imagine not only how data is changing but also how revisions of documentation and models can be dealt with smoothly. This is favourable if the change management can be built into a pipeline, such as inspired by CI/CD. If not, the prototypes will be expensive to keep updated. It should also be possible to discuss change management as part of the problem and solution in innovative interfaces connected to manufacturing.
This is especially important in the context of digital twins, as these systems are defined by being “digital replicas.” If the digital twin needs to be updated manually as the physical phenomenon changes, this can be considered a warning signal that the twin is not sustainable. However, for prototyping and POCs, that is perfectly normal, just as long as it is clear that change management is not considered.
Working with system integration, cross-domain interfaces and new innovative ways of expressing data connections, often through a sustainable lens, putting together different data sources with some level of aggregation is necessary to gain new insights. In the polycarbonate sheet manufacturing use case developed for the RE4DY project, the settings ensured that a certain percentage of material was moved into the categories of waste and circular flows outside the main flow that was moved further along the chain (Figure 4). This is an efficient way to bring up the discussion about sideflows and create plans for circularity. Imagining several of these flows, it would be possible to abstract the stations from instances of actors to categories and feed the output from a circular flow back into the manufacturing process again.
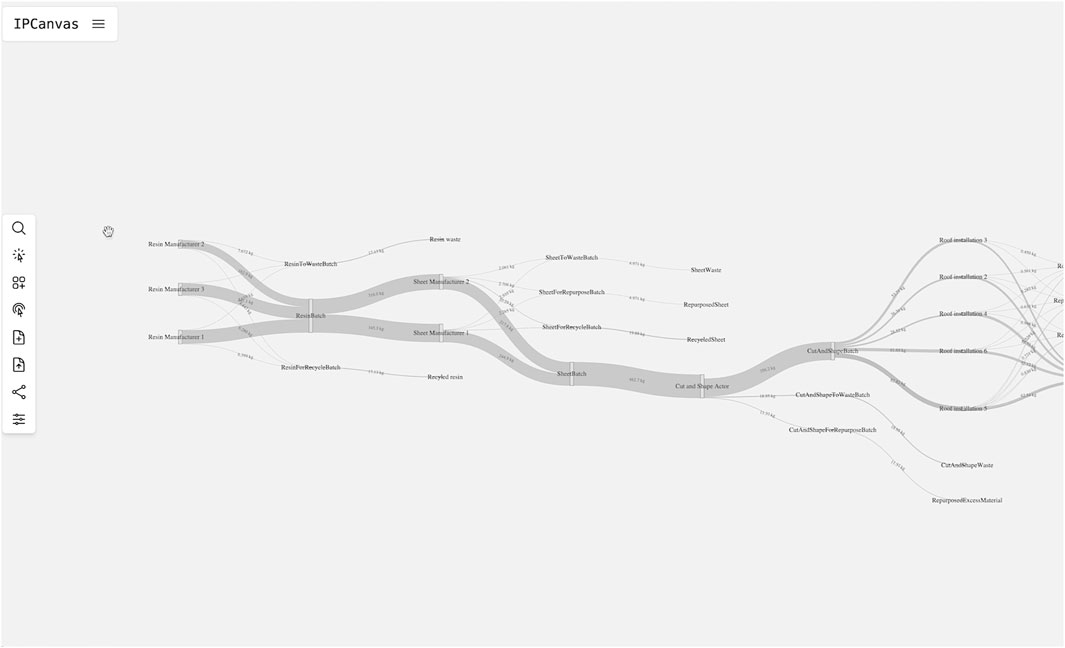
Figure 4. The Sankey chart is useful to show the flow from raw materials through the life cycle of the manufacturing process. In each station, the side streams are also shown distributed between waste and different circular flows.
Figure 5 shows an operational flowchart useful for tracking down and navigating connections and processes between the actors and the different products.
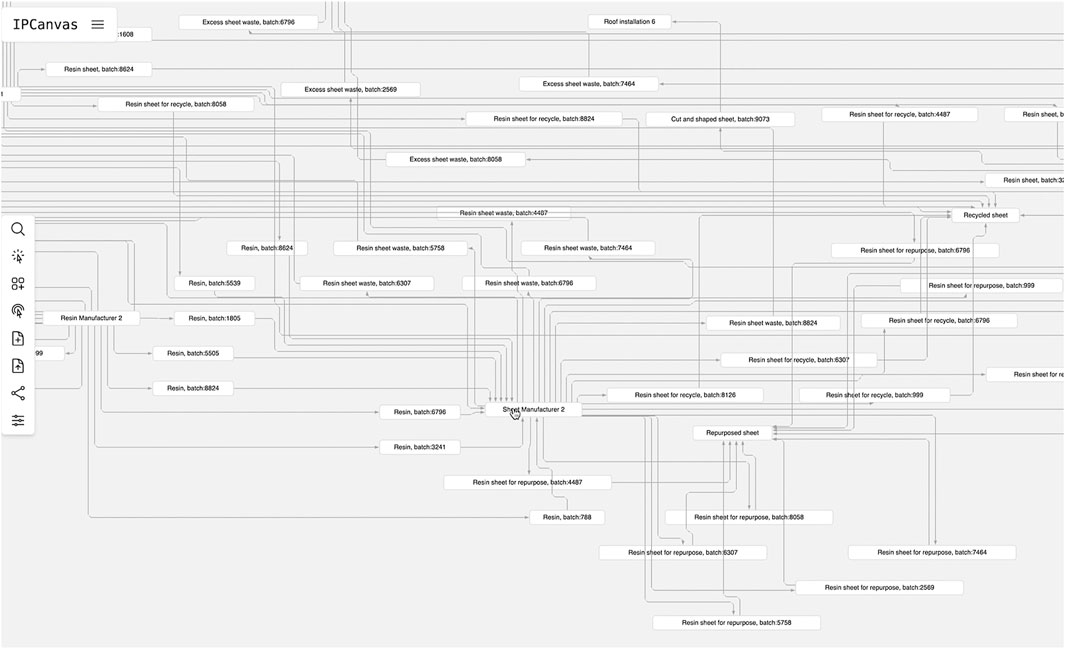
Figure 5. In this view, we can zoom in and follow different tracks from actors or products in the supply chain.
During an ontology development and implementation process, it is useful to link to ontologies in the prototyping environment. This can stimulate discussions on innovative applications of the ontology as well as clearly visualise the connections.
In the use case of manufacturing polycarbonate sheets, several ontologies were loaded into the runtime. First, the IP ontology was loaded and mapped to the immaterial instance of polycarbonate, an entity protected by IPR. Then, the Supply Chain Reference Ontology (SCRO15) was loaded to map the material entity to the supply chain. By this connection, the link to the top-level ontology BFO16 could be visualised for both the material and immaterial assets (Figure 6).
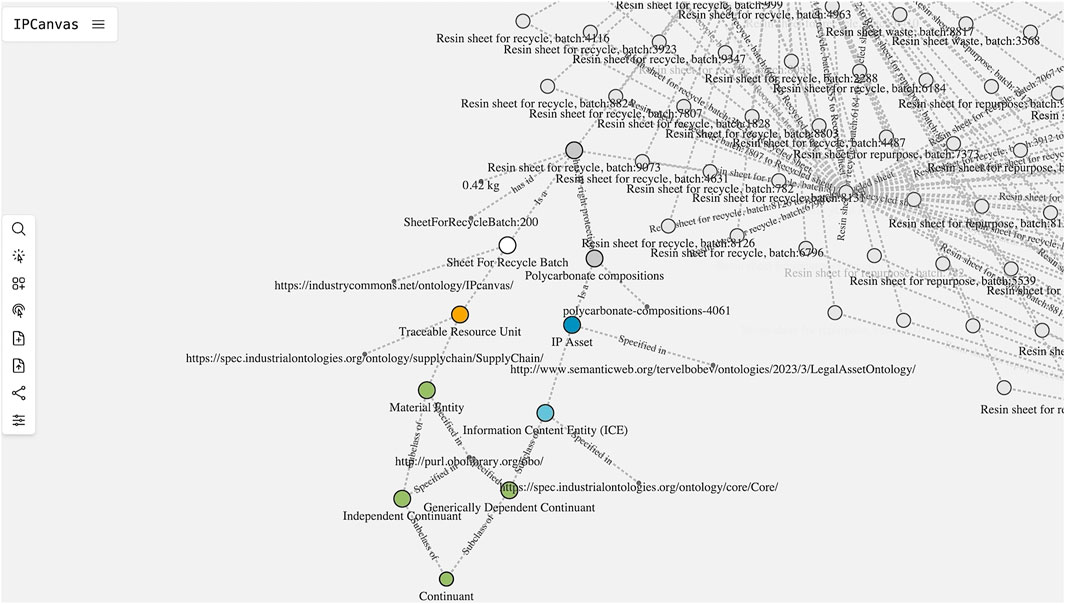
Figure 6. The IP ontology (in blue) and the supply chain ontology (in orange) are connected from the application level and up to the BFO top-level ontology (in green).
4.2 Basajaun use case and prototyping in OntoCommons
The Basajaun project is a Horizon2020 project that develops wood-based building components and follows these through the wooden value supply chain, with several building use cases to break down the supply and demand of bio-based material. One of the buildings is built physically in Bordeaux with the aim of connecting real production data from the full life cycle of the building. As the project ends a few months after the building has been constructed, a few months of data have been collected by the sensors in the digital twin of the building. At that stage, exploring the digital twin to trace wood back to the forest for each component will also be possible. By projecting the maintenance of the building as well as its decommissioning phase, it will be possible to show a favourable circular perspective when using bio-based materials. This will also promote the importance of the origin of wood, including the perspective of certification and deforestation. With the data, several innovative visualisations have been produced, such as the Sankey diagram of the wood flow from Forest to building and the Woodlaunch application that uses a 3D node-based flow visualisation.
As Basajaun has been selected as a demonstrator in the OntoCommons project, there have been activities to connect the wood supply chain data to semantic structures. Since horizontal alignment is very challenging in a long value supply chain reaching across a great number of domains, a traditional approach to information modelling would be unsustainable. Looking into the state-of-the-art industrial interoperability, as defined in the OPC UA standard, the work on companion information models using XML schema is very time-consuming and far from complete when it comes to cross-domain concepts that are agile enough for a broad supply chain collaboration. Aligning using full semantic interoperability could be the only way to future-proof this work, as the chain must change and be extended in a manageable way.
Connecting actors along a value supply chain trying to bring in state-of-the-art semantic cross-domain concepts requires a user-centric perspective from the start. Going too fast into horizontal alignment will risk the most important part of succeeding factors in this process, namely, that there needs to be real industrial actors in the alignment work. Solving this problem in the paperwork will not help, nor will the handover of complex concepts in documented form after years of project research. The transformation towards industrial interoperability must start with the people working at the daily operations, where reaching over the border to neighbouring domains could feel like an insurmountable challenge, especially considering today’s optimised just-in-time global supply chains. There is simply no time to risk disruption or incentivise too much change for the sake of environmental improvement if it is not mandated by laws or regulations. However, the interest is often there among the drivers of the organisation, and if they do not have time and resources to immediately transition to a sustainable industrial setup, they can at least take small steps towards measures that focus on environmental indicators.
In the Basajaun project, a prototyping approach was taken for the co-creation of information models and semantic alignment. Looking into how ontologies can be layered from the Application level, through domain level connections and finally connected to more abstract mid- and top-level ontologies, it is possible to start at the application level to cater for common understanding among actors along the supply chain, introducing concepts of types and vocabulary. Being facilitated by someone who has some knowledge of semantics, such as the Ontology Translator (Goldbeck et al., 2022), it is possible to guide users into existing linked data and ontologies, introducing the concept of namespaces.
It has been important at this stage to make a fully functional system without reaching full semantic interoperability, rather a level of compatibility where the actors feel that they can connect to other actors, reasoning about environmental indicators and responsibility. Having quick results will help the actors feel invested from the start without introducing too much complexity. However, from the beginning, there needs to be a clear plan on how to connect the work with defining terminology for the ontology ecosystem.
To not overload the system for supply chain connections, a level of aggregation was proposed. Many systems will emit many messages every minute, and at that level, we need to establish boundaries of responsibility between systems. The supply chain connection will not deal with the level of local digital twins (for example, where a sensor in a building or factory is collecting values for the local digital twin), but for the supply chain level, it is important not to overwhelm the system and agree on the least granular level for the required insight needed at the current increment.
Another kind of aggregation is where packages are sent between actors, and the digitalisation is yet not sufficiently sophisticated to map between individuals sent through the chain. In this case, one must accept some rough estimates in tracing as the different steps in transformation will fork back to the origin.
Finally, a building is a complex information structure that requires some aggregation to be managed in a supply chain context. A building represented by different subdivisions can be represented using IFC (Industry Foundation Classes), the open format used in the industry for transferring information modelling data. A suitable way to deal with building management in the supply chain context is to break down the building’s data according to the bill of material (BOM) used for material assessment in manufacturing. The list of materials contains rows with aggregations and can be used to map out the demand for materials from the building design. This breakdown is also useful for the Life Cycle Assessment (LCA) analysis of the full building.
Another consideration for ontologies in relation to the supply chain is the concept of time and space. In all the KPIs discussed, this factor is relevant as both aggregation needs time, and any environmental calculation should include transport between the actors in the chain. One area of future research is to connect ontologies to the NGSI-LD standard used in a smart city context that focuses on Context Information Management (CIM) but can be suitable for supply chains as well. It is based on JSON-LD and was used as inspiration for the data payloads implemented in Basajaun as it supports IoT principles with time and spatial data in the specification17.
4.2.1 Connecting the Basajaun building model to existing ontologies and the ontology ecosystem
The Supply Chain Reference Ontology (SCRO) described in Section 2 of this paper is a new initiative connected to the IOF (industry ontology foundry) for modern and modular ontology adaptation suitable for the industry. This effort is also connected to the top-level ontology BFO and adheres fully to the layering of ontologies, where hooking into such a system will help create consistency connected to first-order logic.
There are many domains that need to be mapped to the SCRO when connecting a full supply chain. When working with buildings, there is a connection between the IFC format used in the industry and the ifcOWL, the ontology version of IFC. As this ontology contains thousands of classes, it is challenging to implement and align to the ontological ecosystem (Figure 7). Work has been done to connect ifcOWL to BFO, which is helpful in guidance on how to further connect with the SCRO18.
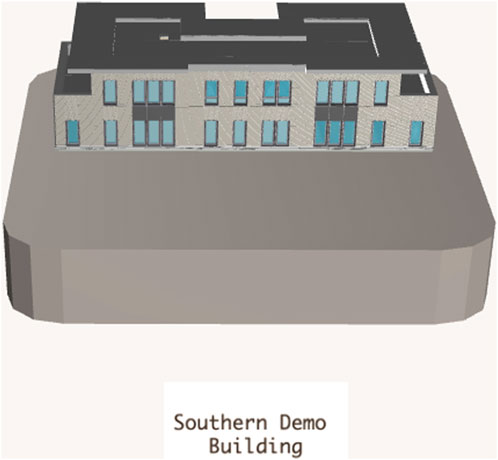
Figure 7. The BIM model from the Basajaun project contains hundreds of thousands of objects and is divided into three IFC models–facade, structure and interior partitions.
In this prototyping work, we used the source code and data from the Basajaun project to show how it is possible to break down the IFC data into categories and map these to the ontology, chain it back through the chain of superclasses, and finally connect with BFO (Figure 8).
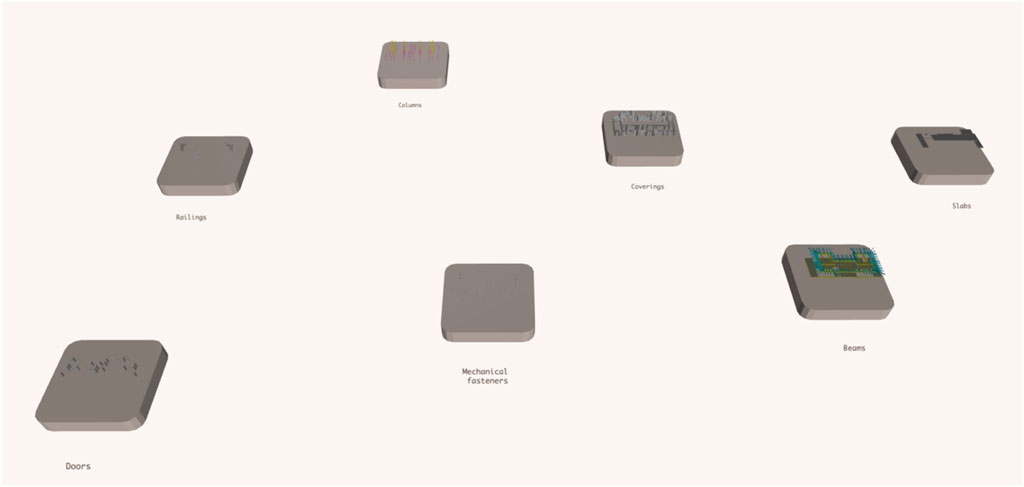
Figure 8. This visualisation breaks up the BIM model into semantic sections to display the 3D representation of each category (only a few examples are shown here). The Mechanical Fastener, containing all the bolts in the structure, is hardly visible in the picture.
By mapping the volumes to a semantic model, we can break down the demand side according to different materials and connect it to the supply side of the flow. In the end, switching to an environmental perspective, we want to bring forward values such as energy, emissions and embedded carbon to compare and break down the sustainable value supply chain in a transparent way.
To map the instances in the building to their classes, we can extrude the nodes on the amount of instances for each class (Figure 9). There are two columns that are higher: the Building Element Proxy and the Mechanical Fastener. The Building Element Proxy is simply a non-semantic instance and shows that more work is needed to bring out the meaning of a large part of the model into this visualisation. The Mechanical Fastener is, as shown in a previous example, all the bolts in the structural model. This gives us good insights using the semantic connection.
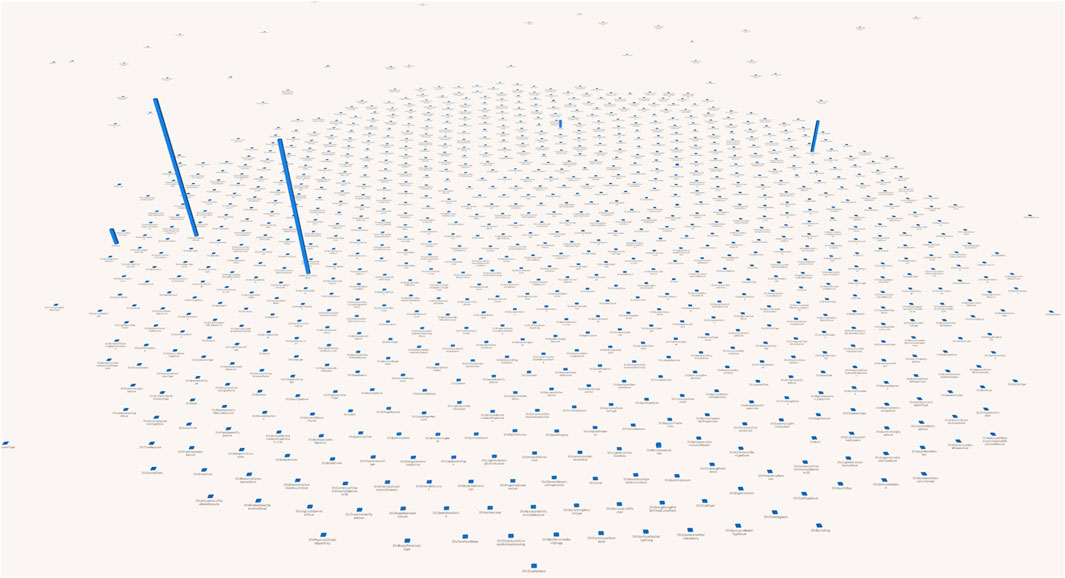
Figure 9. Loading the ifcOWL ontology with the classes that represent building elements gives this overview. The two larger columns show examples of one useful semantic connection, the bolts of the structural model, and one missing semantic link, the Building Element Proxy.
Loading a small selection of the instances directly into the visualisation will directly show that aggregation is needed, as data is overwhelming. In Figure 10 the selection of the Building Element Proxy and the Mechanical Fastener are centred around their semantic classes.
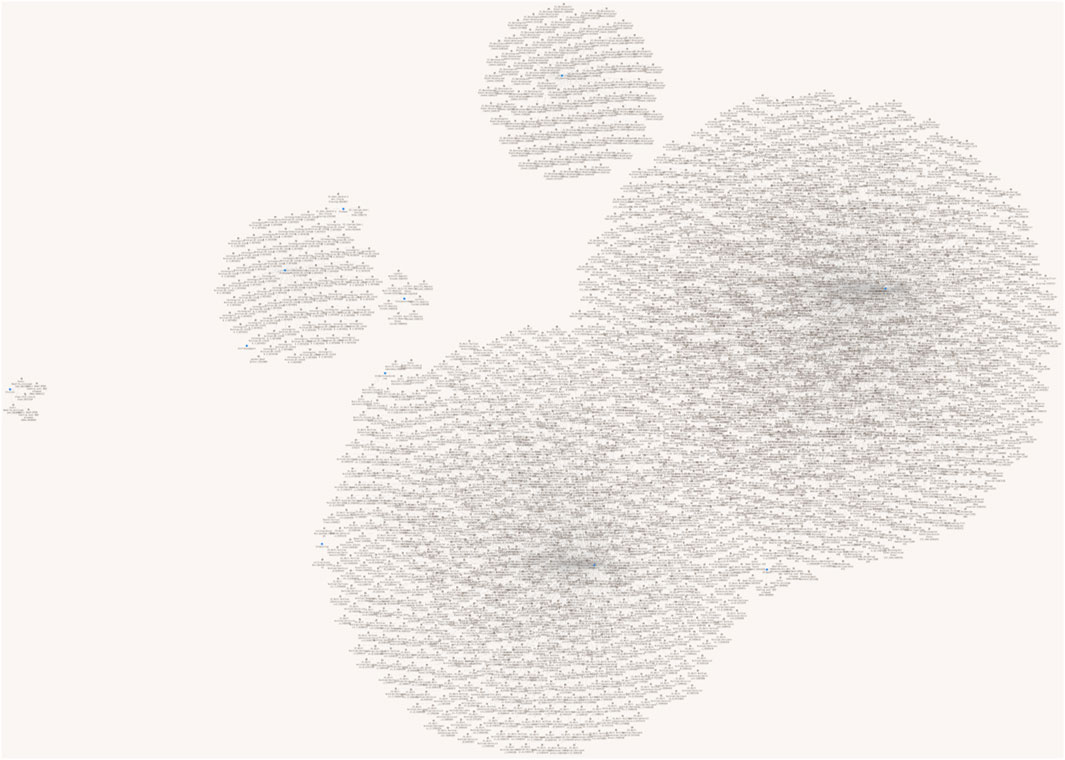
Figure 10. Loading a small selection of instances connected to their classes could be useful. However, the volume of data could also quickly overwhelm the visualisation for BIM models.
Finally, when navigating along the superclass hierarchy, Figure 11 shows how the IfcElement from extended ifcOWL connects to the Material Entity class of the top-level ontology BFO, as proposed in recent efforts to align the two ontologies.
As the SCRO ontology is also aligned to the BFO, the work is simplified from here on to continue to find a suitable way to connect the building information to a supply chain context. Figure 12 shows the orange nodes representing classes from SCRO but without any specific connection.
In this visualisation, we worked on quantifying the extreme number of instances coming in from the BIM model into different categories, for example, how each bolt is represented and instantiated. Working with supply chain demand and sustainable assessment, it is valuable to break down the wood and steel by amount, volume or even with LCA indicators. A semantic connection can be very powerful when scaling up to large-scale assessment. With a proper semantic connection, we can simplify the assessment of sustainable material flows.
5 Novel research direction: proposal for a meta-level approach to the management of ontologies
Findings from the overview of ontology engineering tools in Section 3 indicate that ontology development, implementation, and management are fragmented and difficult for new users of ontologies to grasp (Skjæveland et al., 2020). This is a significant challenge in environments where ontologies are vital for managing effective data exchanges in complex industrial processes. This section proposes a direction towards meta-approaches to strengthen the efficiency of developing, implementing and managing ontology solutions over their life cycle. It aims to initiate discussions and novel research towards a functional and efficient meta-support infrastructure for the life cycle management of ontologies and stimulate meta-platform development and standardisations for the ontology community towards more effective life cycle management and more significant adoption of ontologies in production processes.
Managing a collection of different ontologies in an organisation can be challenging as each ontology has a unique life cycle that involves a combination of tools. This can be especially difficult in organisations involving production and supply chain interactions with numerous producers, each with its unique setup for ontology management tools and standards. Each ontology can be managed by its combination of tools for each phase, and often, not all steps are covered by many ontologies. There is also a potential need to add future support processes such as analysis engines for statistics and learning/best practices to support effective implementation. Future changes in standards and collaborative procedures will demand the adaptability of both the tools and processes.
During the OntoCommons project,19 a survey was conducted to identify the gaps between the use of different processes and tools towards enabling innovation and suggest further research directions. Examples of improved processes and tools described by the participants are skills/learning/practices management support, need/challenges evaluation support, standard effective and accurate tagging and search, and ontology “assembling” support, allowing users to find/evaluate and reuse existing ontologies. Tools and routines were also mentioned for coordinating distributed development efforts and managing FAIR administration and IPR management. Lastly, requests were stated to support effective “packaging” of the ontology context, allowing more effective sales/distribution and deployment–a package containing the contents/reference to the ontology itself and including supporting references and data covering knowledge bases, expertise documentation, etc.
The conditions described above demand approaches supporting high levels of flexibility, scalability, and interoperability with many current and future standards, supporting new types of workflows and collaborative contexts.
In answer to the above challenges, we present a meta-level approach for managing ontologies called the OntoTwin.
5.1 The OntoTwin approach
The OntoTwin approach aims towards solutions that can:
• Act as a meta-data (portfolio) manager to support the processes and data managed in the ontology lifecycles and function as an efficient distributed repository for data and information.
• Be modular in their design and allow for the dynamic addition of potentially new information contexts, such as community of practice/learning platforms, reuse by external markets, access by repositories, sustainability benchmarks, and IP management.
• Allow integrated interoperability with future external information management systems and technologies into the ontology lifecycle information management flows.
Core concepts of the OntoTwin approach build on the research in eMaintenance solutions for advanced technical equipment (ICT concepts for managing future challenges in e-maintenance20) and EU H2020 project CIRC4Life21. They include i) the main application framework, ii) functions, iii) elements and iv) support tools.
The main application framework manages the information/data flows between the different functions and tools and the events controlling the interaction between the different parts of the system. It allows the user to navigate between several workflows and processes in parallel. In a final implementation of OntoTwin approaches, the user can, by access level, select and “install” their custom setup of tools and processes in the form of functions and tools.
The function represents a collection of information and tools to manage a given process flow or main functionality in the system–a function can represent a process step in the schema above (Requirement specification, Implementation) containing their different tools. The function acts as a canvas for users to bring a unique combination of elements and tools to their workflows.
Elements can be regarded as individual information contexts–not only a single database record itself but an “environmental context” involving integrating additional support data and relations, allowing the element to connect by relationships, allowing for the functional clustering of many disparate types of information contexts if needed by the user–information contexts often residing on a multitude of external systems. The user can access many elements covering different information types, connectivity options and data, categorised according to discipline and process flow to an ontology.
The element is one of the primary managers of information and data in the system, responsible for managing the core data in the element and updating the related services, such as related external databases, knowledge graphs, statistics and vector/LLM systems.
Support tools represent tools and functions considered neutral and useable regardless of discipline or process area. Examples of tools are multimedia resources, visualisation tools and editors, binaries, integrated web browser functions to interact with external web tools, REST connection tools to allow for integration of external REST-accessible data and access to external databases for integration into the UI for the user. Often, functions and elements can contain several interacting sub-functions, elements and support tools, allowing the development of highly functional dashboards and navigation facilities.
5.2 Usage scenario
Figure 13 describes a usage scenario of the OntoTwin concept that presents the hierarchies of components–functions, elements and tools. The application framework is in green, functions are in light blue, elements are in yellow, and tools are in pink.
The user first selects the main functionality of the system–in this case, to manage the ontologies repository. After a search, the user selects which ontology to be managed. The core detail information is presented with an updated list of the selected ontology’s connected resources, representing elements or sub-functions. There is no limit to the number of related elements or their types that also can be updated over time. As illustrated, an element can consist of other elements and different tools. In this case, the market offering for the ontology is presented with statistics, initial analysis of use, error reporting, etc. The different elements, functions and tools can be reused in many systems outside the OntoTwin context.
5.3 Core data and information management approaches
The elements are the system’s primary data and information managers, who control the access, updates, and insertions of data from the connected data sources and support systems. The primary storage formats within the element data storage facility are JSON(-LD) and XML in the current version. This allows elements to directly follow the future standards for data exchange based on JSON(-LD), allows the data to be used in a modular approach, and simplifies the interoperability with future systems following standards developed by GAIA-X and IDSA, such as the Manufacturing Data Space 4.022.
Using meta-data and connectivity information, the environment can facilitate remote access to web-based tools and remote data through REST calls and direct database connectivity to external databases. Database connectivity allows interoperability with all relevant database systems in the market today, assuming that the element has access to the correct access information and profile. In all these cases, the element GUI must be designed to represent the data managed in the individual element. Specialised elements can act as agent representatives of complex data management tasks involving the interaction of several external/internal systems to collect and analyse data from different sources.
5.4 Example of potential ontology elements structure–OSysRec
OSysRec is a systems reconfiguration ontology to support model-based systems engineering (Qasim et al., 2023). The OSysRec ontology is complex and is designed to support multidisciplinary solutions during extended periods with ongoing modifications and updates to advanced systems. Due to these demands, the ontology must be flexible and adaptable for future changes and support large volumes of complex data. Figure 14 illustrates the overall structure and contents of an OSysRec implementation.
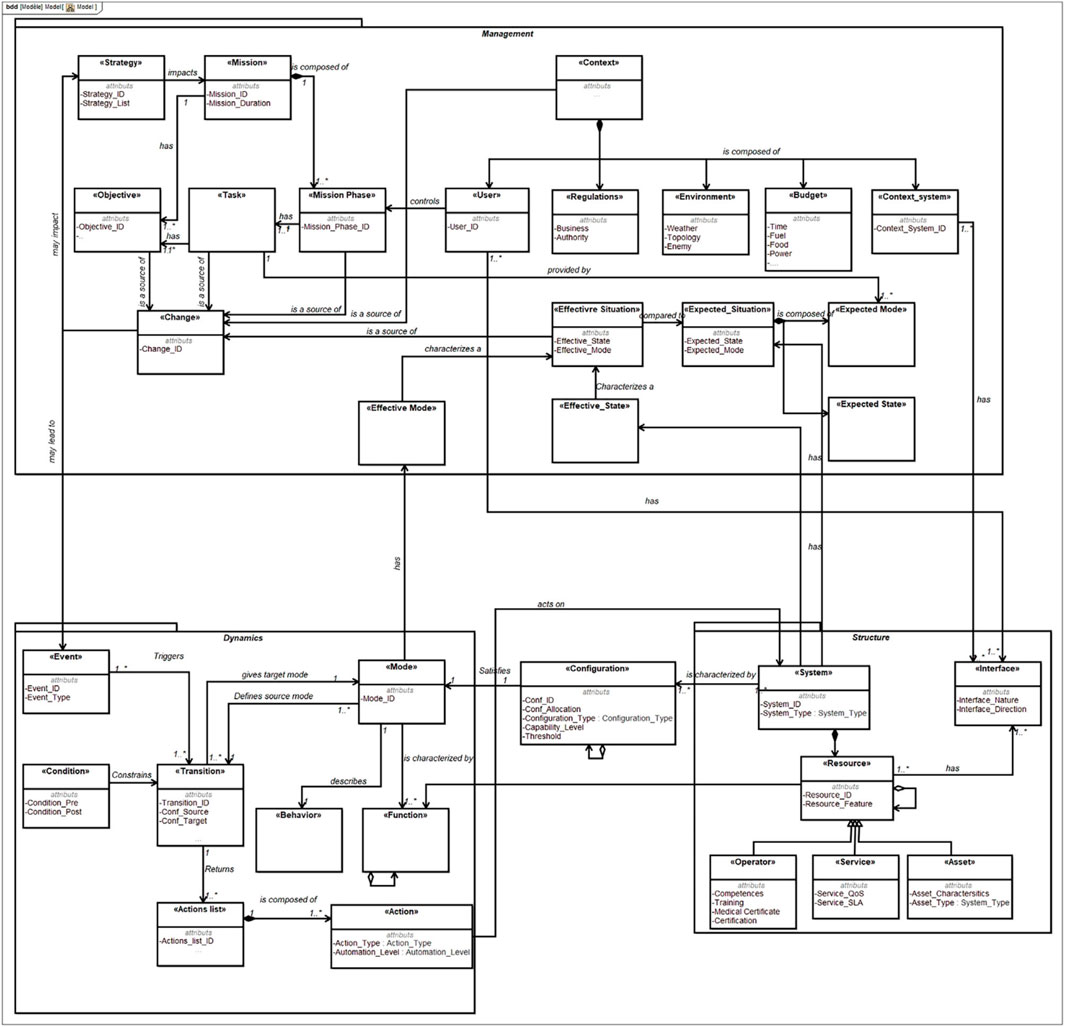
Figure 14. Overall structure and contents of an OSysRec implementation (Qasim et al., 2023).
Each one of the concepts in Figure 14 can be represented by an element containing not only the internal and external attribute/support data for the concept but also its structured references. The data in the concept/element can be stored in external systems, and over time, additional concepts/elements can be added–knowledge bases, connections to external analysis systems, etc. The OSysRec ontology for a system can be managed in the OntoTwin environment as a network structure of elements representing individual concepts. The OntoTwin environment can be a support/governance infrastructure for larger-scale implementation of the OSysRec ontologies over time.
5.5 Interoperability with external specialised support systems
Another vital role of the individual element is to manage the interactions with external specialised support systems–amongst the most important are Knowledge Graphs, technical statistics and vector database systems. These external support systems can be managed in parallel–updating an element of data, such as knowledge graph data, vector data, and extensive data statistics, can trigger updates of the external support systems in parallel.
Knowledge Graphs are utilised to present and manage the relationships between ontologies and components in ontologies. Network visualisations can be added as tools or elements and used as environmental components interconnected with the datasets.
External statistics databases are vital for reporting and analysis both internally and externally.
Vector databases allow for interactions, searches, and analyses similar to ChatGPT, vital in knowledge and skills management systems searches and ad hoc analysis functionalities.
Other specialised support systems are distributed ledgers for IP management and interplanetary File Systems. The interoperability mentioned above with an external marketplace system is an example of an external support system to the OntoTwin environment.
5.6 Example of a potential OntoTwin deployment
A complete deployment of an OntoTwin system can result in the approach presented in Figure 15 interacting with external systems with data “assembly/stitching” and presentation/services provisioning. The schema shows the vital functions for a potential future OntoTwin system to be functional and scalable. A future system must cover standardised search meta-data systems, approaches for interoperability with legacy data systems, and the ability to integrate knowledge/skills/practices resources into the systems and workflows. Environments for managing the lifecycles of ontologies will not function in a vacuum in the future–the ability to interoperate with other systems and contexts will be critical for overall system effectiveness.
5.7 Risks and challenges of the OntoTwin approach
Due to the modularity, adaptability and flexibility of the approaches used in the OntoTwin concepts, the demands on effective and sustainable governance infrastructures increase not only in the context of management of ever-growing availability of element types and functions over time but also in the need for coordinated interaction with external support systems and services not easily foreseen today. Due to the modularity of the environment, OntoTwin solutions can be integrated into other systems as a vital sub-component, placing high demands on adaptability and sustainable operation over time. Thus, effective and accurate standardisation/governance approaches will be needed in those implementations beyond enterprise internal solutions, such as sustainable supply chain solutions, advanced repositories/escrow solutions and modular management systems. Due to these factors, the systems specification phases can increase in complexity and demand more resources and collaborative efforts in large-scale implementations.
6 Emerging and future research directions
The prototypes described in Section 4.1 have highlighted the need for a Resilience Ontology that incorporates resilience elements from various domains. Research is currently in progress on resilience priority criteria from industrial use cases within the project RE4DY, and an international Task Force is in place to build the relevant ontology reference documentation. It is anticipated that a Resilience Ontology may require dedicated ongoing research due to the level of complexity in spanning several domains and catering for a rapidly evolving number of use cases.
Advancements towards support to MVN resilience have already been made within RE4DY with the first version of a Legal Ontology of IP Rights (Bobev, 2023), referenced in Section 4.1. This ontology responds to the industrial need for the management and tracking of proprietary intellectual property and acts as a horizontal enabler for Ecosystem Integration across domains. Further research has been suggested in conjunction with the international Task Force on expanding this ontology to an overarching Legal Ontology.
Recent systems engineering literature referenced in Section 5.4, demonstrates the benefits of cross-domain ontology frameworks for the integration of structure, dynamics and management reference models within the growing field of Model-Based Systems Engineering (MBSE) that applies modelling principles, methods, languages, and tools to the entire lifecycle of large, complex, interdisciplinary, sociotechnical systems (Qasim et al., 2023). These novel engineering approaches point to the need for further research into the role of ontologies and meta-models in MBSE as a useful direction for building the resilience of manufacturing enterprises.
The recent Materials 2030 Manifesto23 and Roadmap24 promoting the creation of a Materials Commons for data sharing with communities of interest across domains has highlighted the need for research into a unified materials ontology. It is envisaged that research would be built with reference to OCES and the EMMO (European Materials Modelling Ontology).
Recent prototyping of commercial systems using knowledge graphs conducted by the IKEA demonstrator team within the OntoCommons project shows promising directions for more ethical approaches to B2C digital environments and optimisation of data-driven applications. Results from live prototyping include the removal of cookies and complete anonymisation of user interaction with the commercial catalogue; the addition of features in 3 days that would previously take months, with notable labour savings; substantially improved time to market that allows for quick iterations and improvements; no data migration, no training of large data sets over periods of months, no expense of running statistical models hosted in the cloud; substantial reduction of data carbon footprint (Kari et al., 2023).
7 Conclusion
Advancements stimulated by the development of the OCES are paving the way for harmonised cross-domain interoperability and knowledge management in the Industry Commons. Successful Ecosystem Integration will allow for the modelling of the current and emerging market possibilities and can lead to breakthrough innovation that is fully trackable and traceable across the ecosystem. To achieve this, the work conducted with the OntoCommons and RE4DY demonstrators indicates that Ecosystem Integration of manufacturing value networks does not rely on a single reference model but requires further research into effective management of a network of ontologies that can collectively contribute to a greater understanding of complex enterprise systems.
Data availability statement
The raw data supporting the conclusion of this article will be made available by the authors, without undue reservation.
Author contributions
MM: Writing–original draft. DK: Writing–original draft. MP-V: Writing–original draft. LY: Writing–original draft. S-EB: Writing–original draft. AR: Writing–original draft.
Funding
The author(s) declare financial support was received for the research, authorship, and/or publication of this article. The work presented in this paper is funded by the EU H2020 project OntoCommons (958371) and Horizon Europe project RE4DY (101058384). Use cases are provided by the H2020 project Basajaun (862942).
Acknowledgments
The authors wish to acknowledge the valuable contributions of the OntoCommons project partners to the development of the OntoCommons EcoSystem (OCES).
Conflict of interest
The authors declare that the research was conducted in the absence of any commercial or financial relationships that could be construed as a potential conflict of interest.
Publisher’s note
All claims expressed in this article are solely those of the authors and do not necessarily represent those of their affiliated organizations, or those of the publisher, the editors and the reviewers. Any product that may be evaluated in this article, or claim that may be made by its manufacturer, is not guaranteed or endorsed by the publisher.
Supplementary material
The Supplementary Material for this article can be found online at: https://www.frontiersin.org/articles/10.3389/fmtec.2024.1331197/full#supplementary-material
Footnotes
2https://industrialontologies.org
3https://ontocommons.eu/ontocommons-demonstrators
5Open Biological and Biomedical Ontology Foundry Web Site. http://obofoundry.org
6Industrial Ontologies Foundry (IOF) Website. https://industrialontologies.org
7IOF Charter. https://industrialontologies.org/iof-charter/
9https://www.iso.org/standard/74572.html
10https://ceur-ws.org/Vol-3240/paper3.pdf
11ISO/IEC 16350-2015 Information technology–Systems and software engineering–Application management, 4.6; IEEE 828-2012 IEEE Standard for Configuration Management in Systems and Software Engineering, 2.1.
12ISO/IEC 19770-1:2012 Information technology–Software asset management–Part 1: Processes- and tiered assessment of conformance, 3.14.
13https://industrialontologies.org/helpful-materials-on-ontologies/
14For a comprehensive description of OCES use cases, see https://ontocommons.eu/demonstrators and associated reporting on OntoCommons resuts https://ontocommons.eu/results
15https://github.com/iofoundry/ontology/tree/master/supplychain
16http://basic-formal-ontology.org/
17https://www.etsi.org/committee/cim
18https://content.iospress.com/articles/applied-ontology/ao210254
20https://www.diva-portal.org/smash/record.jsf?pid=diva2%3A1005717&dswid=8421
21CIRC4LIFE Report on Technical Implementation of ecoPoints Management, LCA and EPCIS Systems Interoperability https://25cd04c9-5fc8-4b44-8c3c-9ad39fc8bbac.usrfiles.com/ugd/25cd04_7e091aeb45ad4ad5aaa8c6e6a82e9aa2.pdf
22https://manufacturingdataspace-csa.eu/
23https://www.ami2030.eu/wp-content/uploads/2022/06/advanced-materials-2030-manifesto-Published-on-7-Feb-2022.pdf
24https://www.ami2030.eu/wp-content/uploads/2022/12/2022-12-09_Materials_2030_RoadMap_VF4.pdf
References
Ameri, F., Sormaz, D., Psarommatis, F., and Kiritsis, D. (2022). Industrial ontologies for interoperability in agile and resilient manufacturing. Int. J. Prod. Res. 60 (2), 420–441. doi:10.1080/00207543.2021.1987553
Bobev, T. (2023). “Challenges in IP and industry: digesting a datafied world with a legal ontology,” in Ontology Commons addressing challenges of the Industry 5.0 transition (Oslo, Norway: University of Oslo). https://www.youtube.com/watch?v=Osg00-oiKJs&t=7s.
d’Aquin, M., and Noy, N. F. (2012). Where to publish and find ontologies? A survey of ontology libraries. J. Web Semant. 11, 96–111. doi:10.1016/j.websem.2011.08.005
Dudáš, M., Lohmann, S., Svátek, V., and Pavlov, D. (2018). Ontology visualization methods and tools: a survey of the state of the art. Knowl. Eng. Rev. 33, e10. doi:10.1017/S0269888918000073
Goldbeck, G., Simperler, A., Bull, L., Gao, D., Ghedini, E., Karray, M., et al. (2022). The translator in knowledge management for innovation – towards industry commons. https://zenodo.org/records/7041697.
Ivanov, D., and Dolgui, A. (2020). Viability of intertwined supply networks: extending the supply chain resilience angles towards survivability. A position paper motivated by COVID-19 outbreak. Int. J. Prod. Res. 2020 (10), 2904–2915. doi:10.1080/00207543.2020.1750727
Kari, K., Piper, T., and Ashok, A. (2023). IKEA knowledge graph. Ontology commons addressing challenges of the industry 5.0 transition. Oslo, Norway: University of Oslo. https://www.youtube.com/watch?v=Qb6k-Z2JYXw.
Katifori, A., Halatsis, C., Lepouras, G., Vassilakis, C., and Giannopoulou, E. (2007). Ontology visualization methods - a survey. ACM Comput. Surv. 39 (4), 10. doi:10.1145/1287620.1287621
Kiritsis, D. (2010). Closed-loop PLM for intelligent products in the era of the Internet of things. Computer-Aided Design 43 (5), 479–501.
Magas, M., and Kiritsis, D. (2021). Industry Commons: an ecosystem approach to horizontal enablers for sustainable cross-domain industrial innovation (a positioning paper). https://www.tandfonline.com/doi/full/10.1080/00207543.2021.1989514.
Ollero, A., Morel, G., Bernus, P., Nof, S. Y., Sasiadek, J., Boverie, S., et al. (2002). Milestone report of the manufacturing and instrumentation coordinating committee: from MEMS to enterprise systems. https://www.sciencedirect.com/science/article/abs/pii/S1367578802000263.
Panetto, H., Iung, B., Ivanov, D., Weichhart, G., and Wang, X. (2019). Challenges for the cyber-physical manufacturing enterprises of the future. https://www.sciencedirect.com/science/article/pii/S1367578818302086.
Poveda-Villalón, M., Yang, L., Breslin, J., Magas, M., García-Castro, R., Simsek, U., et al. (2022). D4.6 Ontology ecosystem reference implementation. https://ontocommons.eu/.
Qasim, L., Hein, A. M., Olaru, S., Garnier, J. L., and Jankovic, M. (2023). System reconfiguration ontology to support model-based systems engineering: approach linking design and operations. Syst. Eng. 26 (4), 347–364. doi:10.1002/sys.21661
Skjæveland, M. G., Slaughter, L. A., and Kindermann, C. (2020). OntoCommons D4.3 - report on landscape analysis of ontology engineering tools. https://zenodo.org/records/6504670.
Keywords: ontology, ecosystems, Semantic interoperability, manufacturing, lifecycle management, industry commons
Citation: Magas M, Kiritsis D, Poveda-Villalón M, Yang L, Björling S-E and Rudenå A (2024) Ecosystem integration: the use of ontologies in integrating knowledge across manufacturing value networks. Front. Manuf. Technol. 4:1331197. doi: 10.3389/fmtec.2024.1331197
Received: 31 October 2023; Accepted: 29 January 2024;
Published: 21 March 2024.
Edited by:
Dimitris Mourtzis, University of Patras, GreeceReviewed by:
Greg Zacharewicz, Mines-Telecom Institute Alès, FranceGünther Schuh, RWTH Aachen University, Germany
Copyright © 2024 Magas, Kiritsis, Poveda-Villalón, Yang, Björling and Rudenå. This is an open-access article distributed under the terms of the Creative Commons Attribution License (CC BY). The use, distribution or reproduction in other forums is permitted, provided the original author(s) and the copyright owner(s) are credited and that the original publication in this journal is cited, in accordance with accepted academic practice. No use, distribution or reproduction is permitted which does not comply with these terms.
*Correspondence: Michela Magas, bWljaGVsYS5tYWdhc0BpbmR1c3RyeWNvbW1vbnMubmV0