- 1Department of Anesthesiology, Institute of Neurological Disease, Translational Neuroscience Center, West China Hospital, Sichuan University, Chengdu, China
- 2Institute of Neuroscience, Laboratory Zoology Department, Kunming Medical University, Kunming, China
- 3Department of Neurosurgery, The First Hospital of Kunming, Kunming, China
Traumatic brain injury (TBI) often leads to severe neurobehavioral impairment, but the underlying molecular mechanism remains to be elucidated. Here, we collected the sera from 23 patients (aged from 19 to 81 years old, third day after TBI as TBI-third group) subjected to TBI from The First Hospital of Kunming City, and the sera from 22 healthy donors (aged from 18 to 81 years old and as control group). Then, three samples from TBI-third group and three samples from control group were subjected to the protein microarray detection, and bioinformatics analysis. Then, enzyme-linked immunosorbent assay (ELISA) was used to verify significantly altered protein levels. Results showed that, when compared with the control group, all significantly differentially expressed proteins [DEPs, P < 0.05, FDR < 0.05, fold change (FC) > 2] contained 172 molecules in the TBI-third group, in which 65 proteins were upregulated, while 107 proteins were downregulated. The biological processes of these DEPs, mostly happened in the extracellular region and the extracellular region parts, are mainly involved in the regulation of cellular process, signaling and signal transduction, cell communication, response to stimuli, the immune system process and multicellular organismal development. Moreover, the essential molecular functions of them are cytokine activity, growth factor activity and morphogen activity. Additionally, the most significant pathways are enriched in cytokine–cytokine receptor interaction and PI3K-Akt signaling pathways among downregulated proteins, and pathways in cancer and cytokine–cytokine receptor interaction among upregulated proteins. Of these, we focused on the NGF, NT-3, IGF-2, HGF, NPY, CRP, MMP-9, and ICAM-2 with a high number of interactors in Protein–Protein Interaction (PPI) Network indicated by bioinformatics report. Furthermore, using ELISA test, we confirmed that all increase in the levels of NGF, NT-3, IGF-2, HGF, NPY, CRP, MMP-9, and ICAM-2 in the serum from TBI patients. Together, we determined the screened protein expressional profiles in serum for TBI patients, in which the cross-network between inflammatory factors and growth factors may play a crucial role in TBI damage and repair. Our findings could contribute to indication for the diagnosis and treatment of TBI in future translational medicine and clinical practice.
Introduction
Traumatic brain injury (TBI) refers to an intracranial injury when external forces act on the brain and bring about severe neurobehavioral impairment (Maas et al., 2008). In recent years, the incidence, mortality and disability of TBI have been on the rise (Bruns and Jagoda, 2009; Mondello et al., 2014). TBI has become a major cause of death and disability worldwide, especially in children and young people (Alves and Bullock, 2001; Hannay et al., 2004). The degree of brain damage in TBI is divided into mild, moderate and severe categories (Saatman et al., 2008). According to the severity, patients will present different physical, cognitive, social, emotional, and behavioral symptoms (Kushner, 1998; Whigham et al., 2011) and have outcomes from full recovery to permanent disability or death (Frey, 2003; Crooks et al., 2007; Brown et al., 2008; Maas et al., 2008; Nicholl and Lafrance, 2009). The current treatment measures are nothing more than drug treatment, emergency surgery or surgery after a few years (Maas et al., 2008; Kochanek and Tasker, 2009). With the development of medicine, although the current treatment measures have been greatly improved, they are not satisfactory. Consequently, further providing the molecular basis of TBI for clinical treatment has become inevitable.
Traumatic brain injury includes primary and secondary brain damage. Primary brain injury occurs during the initial period of injury due to displacement of the physical structure of the brain (Scalea, 2005). Secondary brain injury, which may be due to primary injury or independent of its cause (Porth and Gaspard, 2014), occurs gradually and involves a series of cellular processes including damage to blood–brain barrier, release of inflammatory factors, free radical overload, excessive release of neurotransmitter glutamate (excitotoxicity), influx of calcium and sodium ions, and mitochondrial dysfunction (Xiong et al., 1999; Park et al., 2008). Nowadays, some molecules have been found to participate in the development of secondary brain injury. The gene expression profile in the rat cerebral cortex with the secondary injury of TBI was analyzed by bioinformatics analysis and the expression change of the genes was found after 48 h of TBI, whose molecular functions were steroid biosynthesis, cell cycle, metal ion transport, inflammation and the apoptosis function (Ou et al., 2014). In addition, by using microarrays and bioinformatics analysis, Puhakka et al. (2017) confirmed 3 months after TBI miR-139-5p was downregulated and the NOTCH1 interactome was upregulated. Then, by genome-wide approach, Lamprecht et al. (2016) found that after TBI, sorting protein-related receptor with A-type repeats (SORLA) may be involved in the post-traumatic cascade linking TBI to Alzheimer’s disease. Moreover, the complement factor 9 (C9), complement factor B (CFB) and aldolase c (ALDOC) in the serum of rat TBI models rose in the early stage of injury, while hypoxia inducing factor (HIF) 1α, amyloid precursor protein (APP) and Williams-Beuren syndrome chromosome region 17 (WBSCR17) increased at the late stage of injury (Thelin et al., 2016). Furthermore, what proteins have changed in the serum of human with TBI? Bogoslovsky et al. (2016) enrolled 34 TBI subjects and 69 healthy volunteers and found an increase in glial fibrillary acidic protein (GFAP), microtubule-associated protein tau and amyloid β peptide (Aβ42) at days 0, 30, and 90 after TBI. In summary, there have been great changes in the serum proteins after TBI, but there are still few studies in this field, especially regarding the changes in human serum protein.
As the secondary injury gradually occurs and is more prominent to the body, the patient’s condition may gradually deteriorate (Sullivan et al., 2000; Reinert and Bullock, 2002). Of the various factors, the role of inflammatory factors cannot be ignored. Previous study has reported that the gene expression in the ipsilateral hippocampus of mice with mild TBI was changed, which mapped to many ontologies and molecular pathways related to inflammation and neurological physiology/pathology, including neurodegenerative disease (Tweedie et al., 2016). Simultaneously, Nwachuku et al. (2016) found that CSF concentrations of inflammatory biomarkers (IL-1β, IL-6, TNF-α, IFN-γ, IL-12p70, IL-10, and IL-8) had a significant association with 6-month neurological outcome, with the favorable outcome group having lower concentrations of these biomarkers on average, in comparison to the poor neurological outcome group after severe TBI.
Growth factor is a protein, or a steroid hormone, capable of stimulating cellular growth, proliferation, and differentiation (Hauer et al., 2009). At present, the role of growth factors in TBI have also been revealed. The expression of BDNF and its receptors at the acute phase following penetrating TBI of rat has been reported firstly to be altered (Rostami et al., 2014). Additionally, in the blast-induced traumatic brain injury of swine, vascular endothelial growth factor (VEGF) was discovered to increase (Ahmed et al., 2012). Moreover, the mRNA expression of components and targets of the TGF-β, BMP, and activin signaling pathways in the subventricular zone (SVZ) and the hippocampal dentate gyrus (DG) was significantly altered after cortical injury in mice, which then induced expression of Runt-related transcription factor-1 (Runx1) that can interact with intracellular TGF-β Smad signaling pathways and promotes microglial cell activation and proliferation and neural stem cell (NSC) proliferation after TBI (Logan et al., 2013).
Although some molecules have been found to be involved in repair and deterioration of the injury, a series of cellular processes are involved after TBI, especially in the development of secondary brain injury. Therefore, these processes cannot be the result of the action of one or more molecules. Accordingly, it is necessary to continue to explore the key players after TBI injury. In this study, serum protein microarray technology and bioinformatics analysis were used to investigate what molecules in the serum of TBI patients make a difference to these changes, and what functions and pathways these molecules are mainly enriched in. In particular, the expression of growth factors and inflammatory factors in serum of TBI patients was confirmed.
Materials and Methods
Ethics Statement and Grouping
Human serum samples used in this study were provided by The First Hospital of Kunming. The patients did not receive special treatment including radiotherapy and chemotherapy. The age of the patients ranged from 18 to 81 years old (mean 46.4 years old). These 23 serum samples were collected third day after TBI and served as TBI-third group. Moreover, the sera from 22 healthy volunteers without cerebral injury and other organic diseases were selected for the control group. Patients’ data was extracted by two independent reviewers, and the study type of the dataset was protein expression profile studies. Any discrepancies between reviewers were resolved by consensus or a third reviewer. This study was carried out in accordance with the recommendations of the Declaration of Helsinki, the Ethical Committee of The First Hospital of Kunming (reference number 2014-2). The protocol was approved by the Ethical Committee of The First Hospital of Kunming. All subjects gave written informed consent in accordance with the Declaration of Helsinki.
Collection of Human Serum Samples
Grouping information and all the patients’ information is shown in Tables 1, 2, respectively. The whole blood of the patients was collected with coagulant tubes. After blood collection, the coagulant tubes (SANLI, Jiangsu, China) were gently reversed, and the blood was mixed four to five times and then stood upright at room temperature until the blood was completely solidified (usually about 1 h). Within 2 h after blood collection, all the serum samples were retrieved and transferred to the laboratory of the Institute of Neuroscience, Kunming Medical University. After the filtering process according to the standards, the coagulant tubes of 23 human serum samples on the TBI-third group were centrifugated with 1,000 × g for 10 min, and the serum was isolated. The collected serum was transferred to 1.5 ml microtubes (Axygen, United States) and divided into 200–300 μl/tube. All samples were immediately frozen in liquid nitrogen, and then stored at -80°C. Moreover, the serum obtained from control group underwent the same procedures. Finally, the samples were filled in a vessel with dry ice and transported to Shanghai Kangcheng Biological Engineering Co., Ltd. for further study.
Protein Microarray Analysis
To detect the DEPs, three samples in TBI-third group and three samples in the control group (these six patients’ basic information is shown in Supplementary Data Sheet S1) were performed the protein microarray analysis. Firstly, the serum samples were diluted with PH = 8.0 1 × PBS five times, and were dialyzed. Then the protein concentration of the serum samples was measured according to the operating instructions of the KCTM BCA protein quantification kit (KangChen Bio-tech, Shanghai, China). Next, the samples and 1× labeling reagent were added into new tubes, mixed, and incubated for 30 min at room temperature. After that, stop solution was used for stopping the label reaction and the biotin labeled samples were dialyzed three times with dialysis tubes. Meanwhile, the RayBio® Biotin label-based human antibody array 1 and 2 (including 1,000 antibodies: cytokines, chemokines, adipokines, growth factors, proteases, soluble antibodies, adhesion factors and other proteins, which all are shown in Supplementary Data Sheet S2) were balanced for 1 h at room temperature and placed at the vacuum dryer for 1 h. Then 400 ul 1× blocking buffer was added into each chip well to incubate on a shaker for 1 h at room temperature. After discarding the blocking buffer, 400 μl of the sample was added to each well and incubated overnight at 4°C with shaking. When the sample was removed, 1× wash buffer I and II were used for washing the arrays 5 min/time for three times, respectively. After that, 400 ul 1× Cy3 equivalent (diluted by 1× blocking buffer) was added into each well and incubated for 2 h at room temperature with shaking and protection from light. Similarly, the arrays were washed with 1× wash buffer I and II. Finally, the InnoScan 300 Microarray Scanner (Parc d’Activités Activestre; Carbonne-France) with wave length 532 nm and resolution 10 μm was used for detecting the fluorescent signal on the arrays. The RayBio® Analysis Tool software and data analysis software of AAH-BLG-1000 were used to extract and analyze the data. The obtained fluorescence intensity data first subtracted the background and was normalized to the Positive Control signals. Differentially expressed proteins (DEPs) were obtained by comparison of the signal values between groups based on P < 0.05 by t-test, FDR < 0.05, and fold change (FC) > 2 or <0.5. In TBI-third group, the proteins more than twice the relative expression of that in the control group were defined as up-regulated proteins, and the proteins that were less than 0.5 times the relative expression of that in the control group were defined as down-regulated proteins.
Gene Ontology and KEGG Pathway Analysis of DEPs
In this study, we applied Gene Ontology (GO) analysis and Kyoto Encyclopedia of Genes and Genomes (KEGG) Pathway to analyze the DEPs by using String online tools1. GO analysis was employed to annotate genes and gene products including molecular function, biological process, and cellular component. KEGG is a knowledge base for systematic analysis of gene functions, comprising a series of genome and enzymatic approaches and genomic information with higher order functional information (Kanehisa and Goto, 2000). Accordingly, it was used for systematic analysis of gene function and related high-level genome functional information of DEPs.
Integration of Protein–Protein Interaction (PPI) Network Analysis
STRING version 10.0 covers 9,643,763 proteins obtained from 2,031 organisms (Szklarczyk et al., 2015). The STRING database (see footnote 1) is utilized to assess and predict the PPIs comprising direct (physical) and indirect (functional) associations. In order to assess the interactional relationships and build a PPI network among the DEPs, STRING tool was employed and established a PPI network according to the function and pathway enrichment analysis. P < 0.05 was considered statistically significant.
Automatic Blood Biochemical Examination
The Beckman Coulter Chemistry Analyzer (AU480) (Beckman Coulter K.K., Tokyo, Japan) was used to detect the serum level of the indicators that reflect the liver and kidney function and lipid status. The Lis 2.2 software (Shanghai Medical InfoTech Co., Ltd., China) was operated for result display. Reagent detection, ion calibration, and quality control must be performed every time the sample is tested. Therefore, these three steps were performed first. Firstly, the standard reagents (Beckman Coulter Laboratory Systems Co., Ltd., Suzhou, China) were placed in the corresponding positions and the sample cups (Kangjie Medical Devices Co., Ltd., Jiangsu, China) filled with W1 cleaning solution and W2 cleaning solution (Beckman Coulter, Inc., Brea, CA, United States) were laid in the stat table. Then the reagent check was started. After, ISE High Serum Standard and ISE Low Serum Standard (Beckman Coulter, Inc., Brea, CA, United States) were then placed in the S-H and S-L positions on the stat table, respectively, for all ion calibrations. Subsequently, the quality control test was conducted. Quality control is the detection of the stability of the reagents. The sample cups with Control Serum 1 and Control Serum 2 (Beckman Coulter Ireland Inc., Co., Clare, Ireland) were placed on the sample rack (Beckman Coulter K.K., Tokyo, Japan) for quality control tests. After the tests were completed, the result was transmitted to the Lis 2.2 software. When all the tests were passed, blood biochemical examination of the samples could be performed. Clean sample cups with 300 μl of the serum sample were put on the sample racks in turn, and the items to be detected were selected for sample examination. The results were also displayed on the Lis 2.2 software.
Enzyme-Linked Immunosorbent Assay (ELISA)
In order to determine the levels of human NGF, NT-3, HGF, IGF-2, NPY, CRP, ICAM-2, and MMP-9 in the patients’ serum, the enzyme-linked immunosorbent assay (ELISA) kits were purchased from MEIMIAN (Kete Biological Technology Co., Ltd., Jiangsu, China) and used according to the manufacturer’s instructions. Firstly, the microtiter plates were coated with purified NGF, NT-3, HGF, IGF-2, NPY, CRP, ICAM-2, and MMP-9 antibodies, respectively. Then blank wells, the standard wells and sample wells were set. In the sample wells, 25 μl of the sample diluent and 25 μl of a sample to be tested were added to the bottom of the wells (the final dilution of the sample is two times). At the same time, 50 ul of the standards of known concentration were added into the standards wells. Nothing was added to the blank well. After that, 100 μl HRP-conjugated reagent was added to each well except the blank well, and the plates were covered with adhesive films, mixed gently and incubated for 60 min at 37°C in the incubator (Thermo, Marietta, OH, United States). Next, the plates were rinsed five times with 1× washing solution and patted dry. Then 50 μl of substrate A and 50 μl of substrate B were added to each well and gently mixed. After incubating in the dark at 37°C for 15 min, the reaction was stopped by 50 μl of stop solution (blue at this time turned yellow). Within 15 min, the absorbance (optical density value) of each well was measured with a spectrophotometer (Thermo Fisher Scientific, Vantaa, Finland) at a wavelength of 450 nm. Finally, the linear regression equations of the standard curves were calculated using the concentration and the OD value of the standards and then the concentration of NGF, NT-3, HGF, IGF-2, NPY, CRP, ICAM-2, and MMP-9 in the serum was calculated.
Statistical Analysis
The measurement data were statistically analyzed with an independent sample t-test using SPSS 16.0 software (IBM Corporation, Armonk, NY, United States). P < 0.05 was considered as statistical significance. The concentrations of NGF, NT-3, HGF, IGF-2, NPY, CRP, ICAM-2, and MMP-9 in the serum were shown by mean ± standard error (SEM) and 95% confidence interval (CI). In addition to the concentrations of these molecules, other measurement data were represented as mean ± standard deviation (SD). The enumeration data was also analyzed by SPSS 16.0, and represented with the percentage.
Results
Baseline Data of the Patients
Baseline characteristics of all patients are shown in Table 2. The patients in the control group do not suffer from cerebral injury or another organic disease. In addition to traumatic brain damage, the patients of TBI-third group also aren’t afflicted with other diseases. The percentage of male and female who were embedded in the control group was 53% and 47%, respectively, but 85% and 15% in TBI-third group, respectively. The ratio of male and female included in the two groups was not consistent. However, the mean age of patients in the control group and TBI-third group were 44.4 ± 20.56 and 46.4 ± 16.24 years old, respectively, with no statistical difference (P = 0.733). Besides, the difference in creatinine (CREA), urea nitrogen (BUN), and uric acid (UA) between the two groups was statistically significant (P < 0.05), but they were all within the normal reference range. Therefore, the kidney function was not considered to be impaired in both groups. At the same time, the total protein (TP), albumin (ALB), globulin (GLB), albumin/globulin ratio (A/G), alanine aminotransferase (ALT), aspartate aminotransferase (AST), alkaline phosphatase (ALP), gamma-glutamyltranspeptidase (GGT), total bilirubin (TBIL), direct bilirubin (DBIL) and indirect bilirubin (IBIL), which all reflected the liver function, in the serum of control group and TBI-third group were also completely within the normal reference range, indicating good liver function in both groups. Moreover, the triglyceride, total cholesterol (TC), low density lipoprotein cholesterol (LDL-C) and high-density lipoprotein cholesterol (HDL-C), which reflected the state of blood lipid, did not differ between the two groups (P > 0.05). In addition to HDL-C that was less than the lower limit of the normal reference range in control group, TG, TC and LDL-C in control group, and TG, TC, LDL-C, and HDL-C in TBI-third group were all within the normal reference range, suggesting that the blood lipid level in both groups were almost normal. Furthermore, the Glasgow Coma Scale (GCS) scores of patients in control group and TBI-third groups were 15 ± 0 points and 13.25 ± 0.39 points, respectively, and the difference was statistically significant (P = 0.000). Additionally, CT examination revealed that the TBI patients had lesions in the forehead (70%), partes temporalis (45%), facies parietalis (35%), and occiput (15%). Hematoma occurred in 85% of the patients’ lesions, which appeared in intracerebral (45%), epidural (30%), and subdural (10%). For the treatment of hematoma, 70% of patients were treated conservatively, and only 30% of patients were treated by surgery to relieve hematoma compression. Blood examination and coagulative function evaluation showed that platelet (PLT), fibrinogen (FIB), activated partial thrombin time (APTT), prothrombin time (PT), and international normalized ratio (INR) of TBI patients were within the normal reference range, illustrating that the TBI patients had normal coagulation function and no possibility of spontaneous bleeding, that meant the hematomas were caused by trauma. Simultaneously, blood examination showed that the hemoglobin (HGB) in TBI patients was 126.73 ± 16.21 g/L, demonstrating that there was no massive hemorrhage after TBI. What’s more, the white blood cells (WBCs) were (9.06 ± 4.48) × 109/L, and the proportions of neutrophil granulocyte (NEUT), lymphocyte (LYM), and monocyte (MONO) were (74.53 ± 9.27)%, (18.95 ± 7.87)%, and (5.55 ± 2.48)%, respectively. It can be seen that WBC and NEUT were elevated, while LYM and MONO were within normal reference range, revealing that there existed acute inflammatory response in TBI-third patients.
Heat Map Analysis of DEPs
The expression levels of all significantly DEPs were shown in the heat map, which comprised 107 downregulated proteins and 65 upregulated proteins (Figures 1A-a,b,B-a,b and Supplementary Data Sheet S3). Moreover, the top 10 of downregulated proteins are Follistatin-like 1, beta-NGF, Lefty-A, MMP-20, EG-VEGF/PK1, Decorin, Pref-1, HAI-2, MCP-4/CCL13 and IL-22 (Figure 1A-c and Supplementary Data Sheet S3), and the top 10 of upregulated proteins are I-309, SAA, Notch-1, S100 A8/A9, D-Dimer, IL-31, NT-4, CRP, MMP-9 and SCG3 (Figure 1B-c and Supplementary Data Sheet S3).
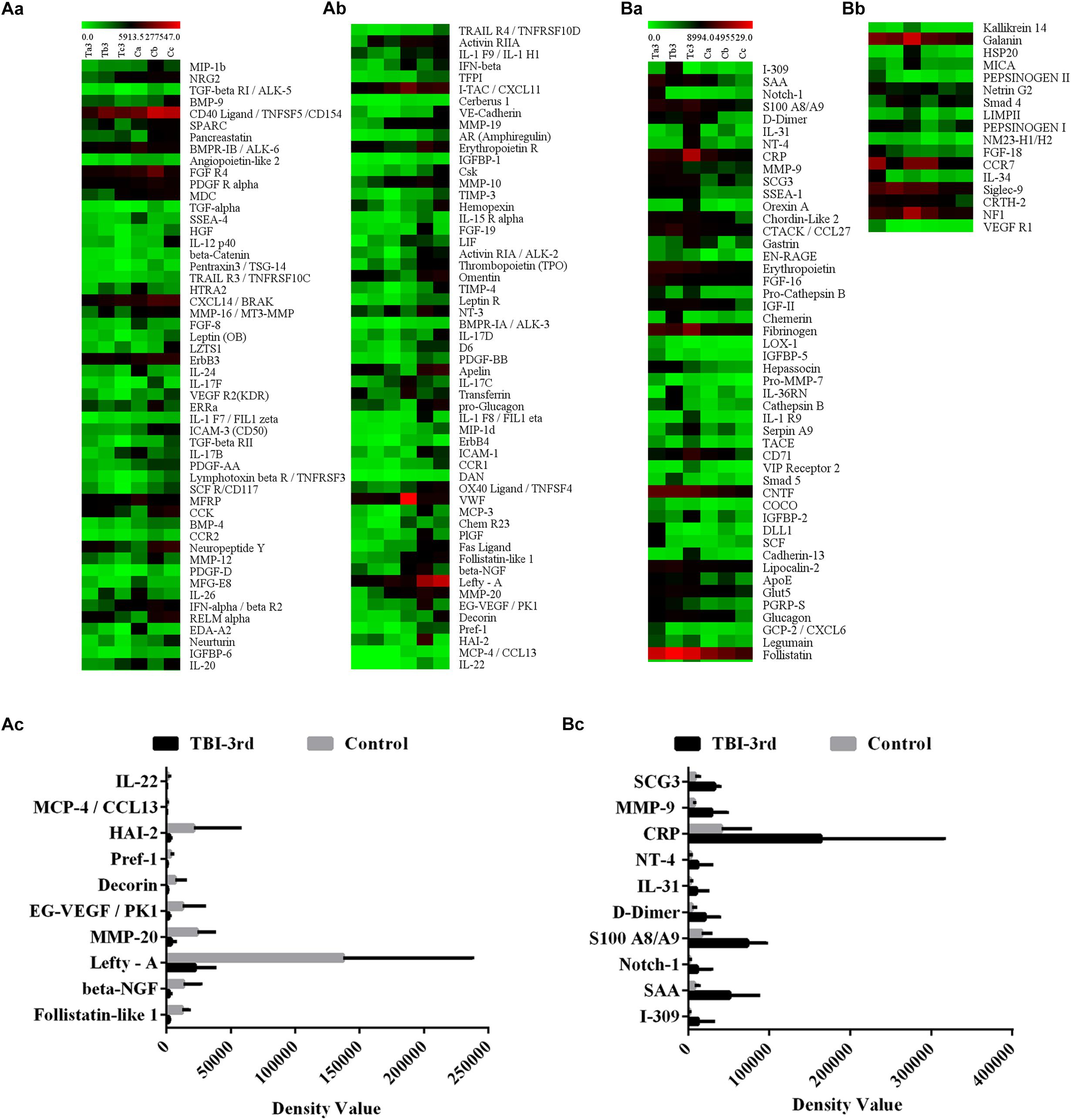
Figure 1. The expression profile of all the differentially expressed proteins (DEPs) in TBI-third group and control group. Here the protein expression was detected by protein microarray. In the heatmap, each column represents a sample, and each row represents a single protein. (A-a,A-b) Represent the down-regulated proteins, and the (B-a,B-b) represent the up-regulated proteins. (A-c,B-c) Show the top 10 of the lowest fold change proteins and the top 10 of the highest fold change proteins, respectively. The color scale shows the relative expression level of proteins in certain slide: The colors from green (left) to red (right) indicate the low to high relative expression levels, in which the green at the far left is the lowest relative expression level and the red at the far right is the highest relative expression level.
GO Function Analysis of Differential Expressed Proteins
The GO is a set of dynamic controlled vocabulary and used to describe the role of gene and protein with three major categories of information including biological process, cellular component, and molecular function. Biological process mainly involves a biological objective to which the gene or gene product contributes, and this process may be accomplished by one or more ordered assemblies of molecular functions. In this study, the DEPs were analyzed in relevant biological processes by gene annotations. The results indicated that the biological processes of downregulated proteins were involved in 212 categories, and the top 10 of biological processes were shown in Table 3A and Supplementary Data Sheet S4. Moreover, the biological processes of upregulated proteins were mainly 115 categories, and the top 10 biological processes were screened and shown in Table 3A and Supplementary Data Sheet S5. Among the three categories of GO, cellular component is related to the activation of a gene product. In this study, we found that the most expression of downregulated proteins and upregulated proteins both happened in the extracellular region, extracellular region part and extracellular space (Table 3B and Supplementary Data Sheets S4, S5). Molecular function is characterized by the biochemical activity of a gene product consisting of specific binding to ligands or structures. The molecular functions of downregulated proteins were mainly involved in receptor binding, protein binding, cytokine activity, growth factor activity, chemoattractant activity, cytokine receptor binding, C-C chemokine receptor activity cytokine binding, morphogen activity, and BMP binding (Table 3C and Supplementary Data Sheet S4), while the molecular function of upregulated proteins were consisting of protein binding, receptor binding, endopeptidase activity, growth factor activity, lipoprotein particle binding, and interleukin-6 receptor binding (Table 3C and Supplementary Data Sheet S5).
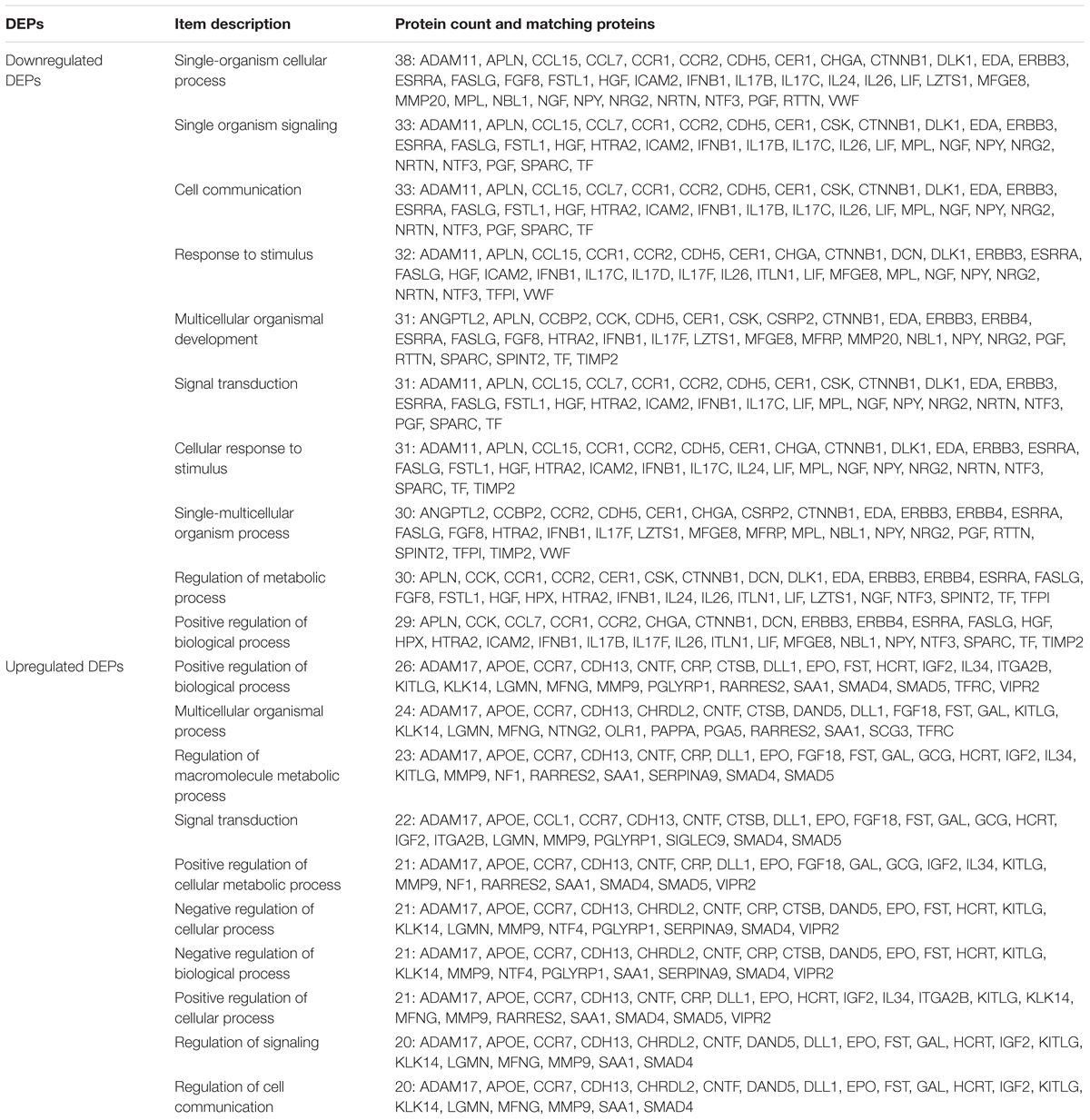
Table 3A. The top 10 biological process based on protein count of differentially expressed proteins (DEPs).
PPI Network Analysis of the DEPs
The PPI network with obvious interaction relationship for all DEPs included 109 nodes and 293 edges, in which the network of downregulated proteins included 58 nodes and 82 edges, and the PPI network of upregulated proteins composed of 51 nodes and 74 edges (Figure 2). Additionally, the top five pairs of all DEPs with the greatest combined score were TF-TFRC (0.999), CCK-GAST (0.993), NRG2-ERBB3 (0.991), GCG-GAST (0.99), and CTNNB1-CDH5 (0.988) (Figure 2 and Supplementary Data Sheet S6). Moreover, the top 10 proteins with the max number of interactors in the PPI network of all DEPs were MMP9 (30), HGF (25), CRP (19), NGF (18), NPY (17), VWF (17), IGF-2 (15), KITLG (15), TF (15), and EPO (13, the same as GCG and SAA1) (Figure 2 and Supplementary Data Sheet S6). Meanwhile, the top five pairs with the greatest combined score were NRG2-ERBB3 (0.991), CTNNB1-CDH5 (0.988), NRG2-ERBB4 (0.984), CCL7-CCR2 (0.978), CCL7-CCR1 (0.959) in the downregulated proteins, and GCG-GAST (0.99), EPO-KITLG (0.982), SMAD5-SMAD4 (0.968), GCG-VIPR2 (0.951), IGF2-PAPPA (0.948) in upregulated proteins (Figure 2 and Supplementary Data Sheet S6). Then the top five proteins with the max number of interactors were HGF (15), NGF (10), VWF (8), CTNNB1 (7), NPY (7) in the PPI network of downregulated proteins, and CRP (12), MMP9 (11), APOE (8), GCG (8), SAA1 (8) in the PPI network of upregulated proteins (Figure 2 and Supplementary Data Sheet S6). In summary, NGF (18), NT-3 (7), IGF-2 (15), HGF (18), NPY (17), CRP (19), MMP-9 (30) and ICAM-2 (2) hold the high number of interactors (shown in the brackets) and might be the core protein in PPI Network of all DEPs. Therefore, they were considered to be meaningful proteins and were for further study. Here values in brackets are the combined score value or the number of interactors.
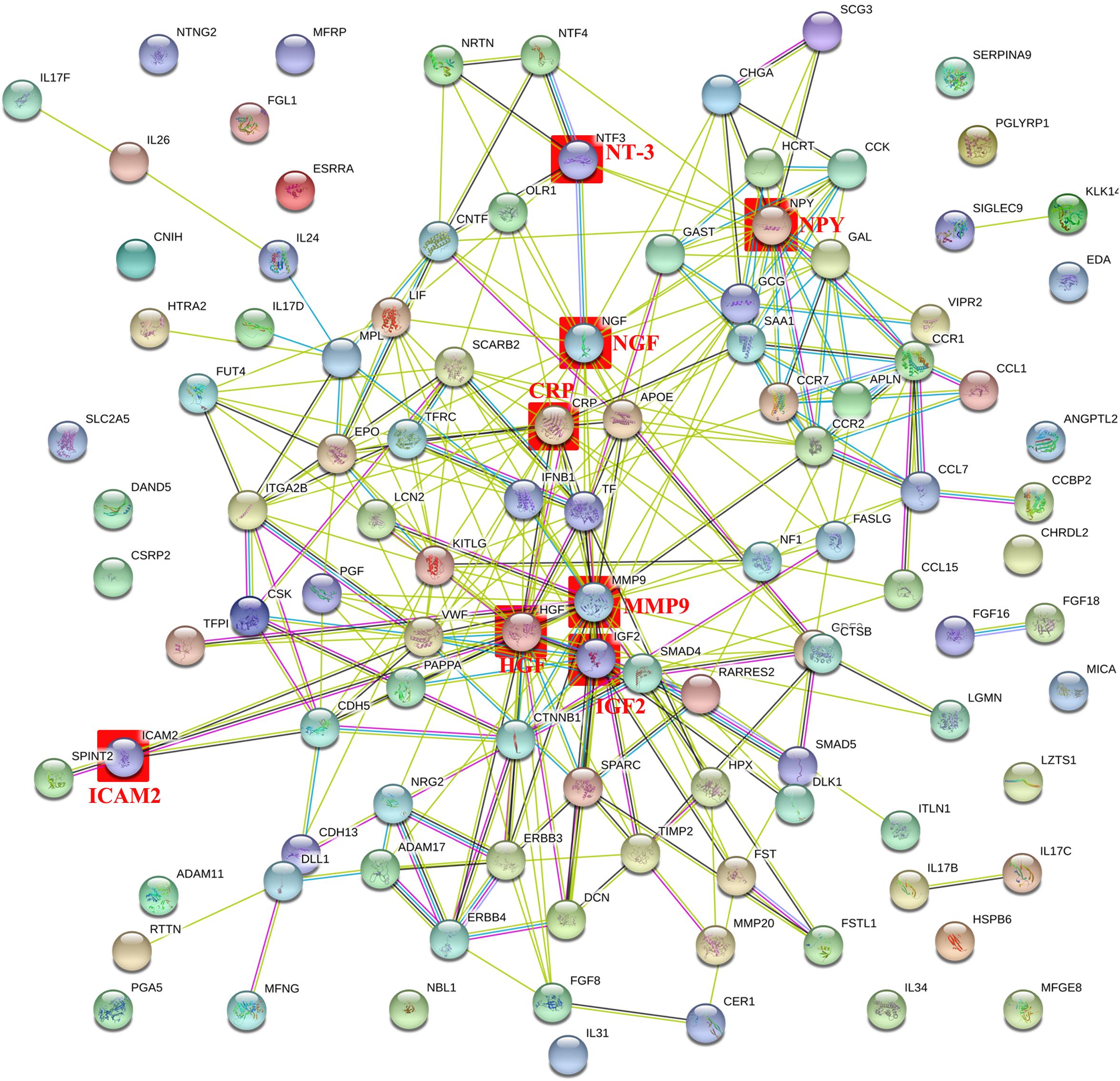
Figure 2. Intermolecular interactions of differentially expressed proteins (DEPs). The intermolecular interactions of all DEPs are shown in this figure. The lines reflect interaction relationship of DEPs. Network edges: line color indicates the type of interaction evidence from the interaction sources, line thickness indicates the strength of data support, and line shape indicates the predicted mode of action. The interaction sources: text mining, experiments, databases, co-expression, neighborhood, gene fusion, and co-occurrence. The minimum required interaction score was medium confidence 0.400. The max number of interactors to show: none or query proteins only in first shell and none in second shell. The network display mode: interactive svg (a scalable vector graphic). The set organism: homo sapiens. Colored nodes: query proteins and first shell of interactors, white nodes: second shell of interactors, empty nodes: proteins of unknown 3D structure, filled nodes: proteins with known or predicted 3D structure.
Kyoto Encyclopedia of Genes and Genomes (KEGG) Analyses for Differentially Expressed Proteins (DEPs)
To analyze the enriched pathways of DEPs, KEGG analysis was performed. The results showed that the significant enriched pathways of the downregulated DEPs were cytokine–cytokine receptor interaction, PI3K-Akt signaling pathway, proteoglycans in cancer, Rap1 signaling pathway, Ras signaling pathway, and Jak-STAT signaling pathway (Table 4). Meanwhile, the enriched pathways of the upregulated DEPs were showed as follows: pathways in cancer, cytokine–cytokine receptor interaction, hematopoietic cell lineage and Notch signaling pathway (Table 4). Among these pathways, they were either relevant to growth or inflammation.
Changes of Expression of Some Growth Factors and Inflammation-Related Factors After TBI Injury
Because of the high number of interactors of NGF, NT-3, IGF-2, HGF, NPY, CRP, MMP-9, and ICAM-2 in PPI Network, we think they may play a pivotal role in traumatic brain injury. In our study, therefore, their expression in serum was verified by ELISA. 39 sera from 19 patients in control group and 20 patients in TBI-third group were detected. Compared with control group, CRP, ICAM-2, and MMP-9 from innate immune system were significantly increased in TBI-third group, and the P-values were 0.008, 0.045, 0.03, respectively (Figures 3A–C). In addition, HGF, IGF-2, NGF, and NT-3 with growth factor activity were also up-regulated in TBI-third group in comparison with control group, and the difference was statistically significant, and the P-value were 0.038, 0.042, 0.006, 0.009, respectively (Figures 3D–G). Furthermore, NPY with neuropeptide hormone activity was significantly more in TBI-third group than that in control group (P = 0.009) (Figure 3H). Moreover, the mean ± SEM value and the 95% CI of the concentration of the eight proteins in the serum of both groups were shown Table 5.
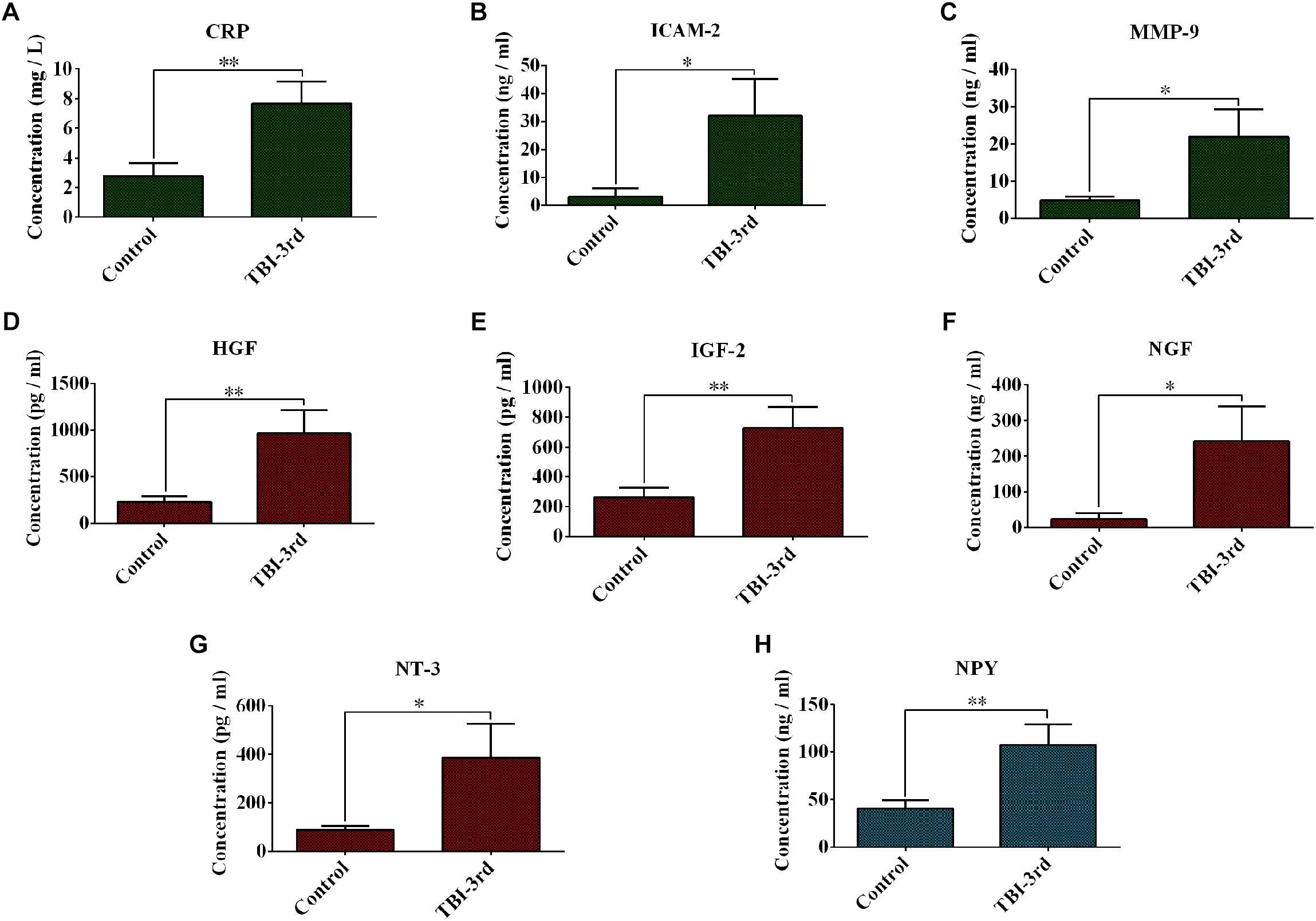
Figure 3. Changes of expression of some growth factors and inflammation-related factors in the serum. (A–H) Separately showed the serum concentration of CRP, ICAM-2, MMP-9, HGF, IGF-2, NGF, NT-3, and NPY in control group and TBI-third group. The data was represent as mean ± SEM. ∗TBI vs. Control, ∗P < 0.05, ∗∗P < 0.01; ∗∗∗P < 0.001.
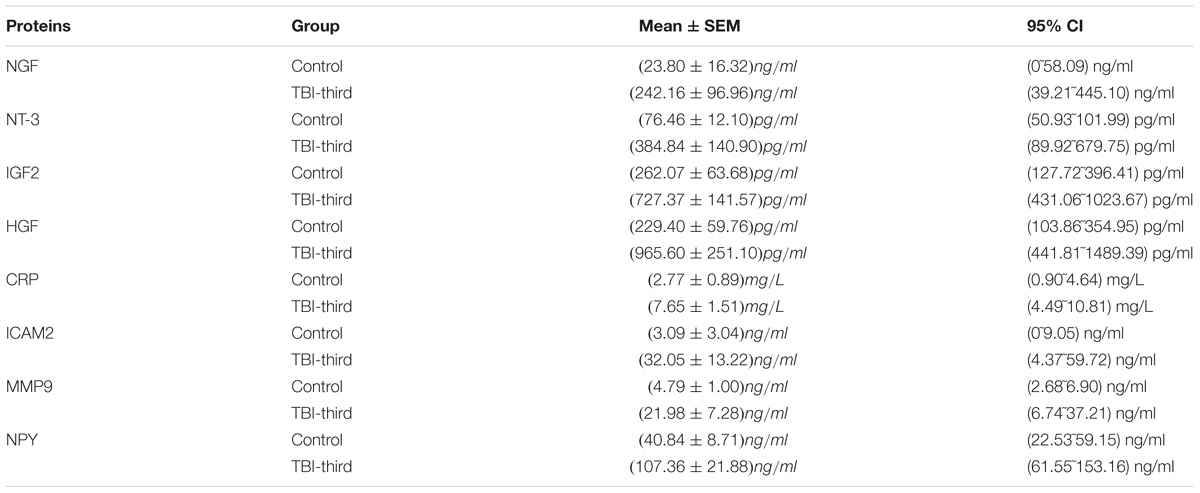
Table 5. The mean ± SEM and 95% confidence interval (CI) of the concentration of NGF, NT-3, IGF-2, HGF, CRP, ICAM-2, MMP-9, and NPY in the serum.
Discussion
In this study, the serum samples of TBI patients and healthy volunteers were subjected to protein microarray analysis and it was found that there were significant changes in many proteins, especially growth factors and inflammatory factors in the serum after TBI injury compared with control group. As everyone knows, clinical data is often influenced by many factors. In order to ensure the scientificalness of the study, the mean age and age distribution of patients in TBI-third group were the same as those in the control group. Moreover, the major indicators such as liver function, renal function and blood lipids in both groups were within normal reference range, which indicated that the liver and renal function of all cases are normal. Furthermore, all volunteers in the control group must have no other organic-related diseases, and all patients in the TBI-third group have mild-moderate damage with 11–15 points of GCS but without other organic diseases except for TBI injury. Therefore, the baseline data of both groups were almost consistent.
At the same time, in this study, the degree of traumatic brain injury was determined by CT performance and GCS score. Because of the rapid acquisition of CT images and independent changes in the patient’s consciousness, routine CT examinations are known as the “gold standard” for the diagnosis of acute brain injury (Toyama et al., 2005). However, CT showed poor brain parenchymal lesions, and it only had a good effect on diagnosing large-area hemorrhagic changes, large-area brain contusion and other relatively wide-ranging lesions. There is a big limitation on the diagnosis of microbleeds in brain tissue, so that the severity of traumatic brain injury was often missed or judged too lightly (Brophy et al., 2011). Moreover, in the acute phase of TBI, the patients may be in a coma or critically ill and then unable to complete the large-scale tests. Therefore, CT performance combined with the clinical behavioral observations to assess the degree of damage is more reliable. The most common clinical behavioral observation is the GCS score, which scored according to coma severity (Becker et al., 2018). In this study, CT showed that the injured sites of the patients with TBI were in forehead, partes temporalis, facies parietalis and occiput, and 85% of the patients had the intracerebral, epidural or subdural hematoma. In addition, the GCS scores of these patients were 11–15 points, that is, mild and moderate coma. Therefore, the serum samples from TBI patients used for in this study also were credible.
Through bioinformatics analysis, we found the biological process of DEPs, the most of which happened in the extracellular region, extracellular region part and extracellular space, was involved in positive/negative regulation of cellular process, signaling and signal transduction, cell communication, response to stimulus, immune system process, multicellular organismal development, metabolic process, and biological process. Moreover, the molecular functions of them were involved in receptor binding, protein binding, cytokine activity, growth factor activity, chemoattractant activity, cytokine receptor binding, C-C chemokine receptor activity, cytokine binding, morphogen activity, BMP binding, and interleukin-6 receptor binding. Additionally, the most significant pathways were enriched in cytokine–cytokine receptor interaction and PI3K-Akt signaling pathway among downregulated proteins, and pathways in cancer and cytokine–cytokine receptor interaction among upregulated proteins. To sum up, these DEPs were much enriched in the biological processes and pathways related with growth and inflammation. As noted above, the secondary injury of traumatic brain injury involves a series of cellular processes (Xiong et al., 1999; Park et al., 2008), which are the result of the involvement of these DEPs. By binding with other molecules (e.g., protein, receptor, and cytokine) and the activity of growth factors and inflammatory factors, they are involved in positively or negatively regulating cellular process, signal transduction, cell communication, response to stimulus, immune system process and multicellular organismal development, etc. Then, in turn, they further promote the damage repair, or suppress or even worsen damage.
The role of growth factors and inflammatory factors in the body is mutual resistance. Growth factors can promote the recovery of neurologic damage. In contrast, inflammatory factors destroy the repair. If the growth factors and anti-inflammatory factors predominate, the prognosis of TBI patients is better; however, if the injury factors such as inflammation prevail, the prognosis will be worse. After TBI, both physical and chemical factors activate the innate immune system to cause a cascade of inflammatory reactions at the site of the injury. Inflammatory factors accumulate around the injury and diffuse into the cerebrospinal fluid and the blood, which is inextricably linked to the patient’s neurological outcome. In our study, WBC and NEUT in TBI-third group were increased and more than the upper limit of normal reference range, which demonstrated that acute inflammatory reaction existed. What’s more, inflammation-related factors including CRP, ICAM-2, and MMP-9 were also elevated, suggesting that after traumatic brain injury, the innate immune system was activated, then the inflammatory factors damaged the blood–brain barrier and were released into the blood. Previous studies also reported similar results. After TBI, neurological function of rats was impaired, while immediately administrating with propofol for 2 h after TBI could effectively improve neurological function (Liu et al., 2016). Simultaneously, the expressions of IL-1β, IL-6, and TNF-α within 1 week after operation were significantly augmented in the injured cortex, but markedly decreased after propofol treatment (Liu et al., 2016). In addition, after TBI injury, some interventions, such as administrated with breviscapine via the right lateral ventricle or co-transplanting NSCs with olfactory ensheathing cells (OECs) into the area surrounding the injury site, could reduce interleukin-6 expression, and significantly improved neurobehavioral dysfunction in the short-term (Liu et al., 2014; Jiang et al., 2017).
On the other hand, however, it has also been reported that growth factors were involved in nerve repair. In traumatic brain injury, neurological function improvement was associated with the upregulated BDNF expression, while the blockade of BDNF exacerbated neurological function deficits (Xiong et al., 2017). Similarly, after spinal cord injury (SCI), the level of BDNF also gradually increased with partial functional restoration, and BDNF overexpressing facilitated the recovery of locomotor function, while BDNF knockdown wound led to opposite outcome (Gao et al., 2012; Chen et al., 2016). Moreover, GDNF and CNTF played an essential role in neuroplasticity after TBI and the SCI, which could downregulate the expression of BAX and BAD signaling to suppress apoptosis, therefore, to improve neurological function and survival (Qin et al., 2016; Qiu and Wang, 2016; Feng et al., 2017). Furthermore, the upregulation of NGF and NT-3 in neurons also could enhance neurons’ survival and neurite outgrowth, as well as promoted motor and sensory function recovery in hind limbs after SCI (Yang et al., 2009; Wang et al., 2016; Xiong et al., 2016). At present, we investigated the factors associated with growth and nerve repair by ELISA, including NGF, NT-3, HGF, IGF-2, NPY in serum, and they were found to be elevated in TBI-third group compared to the control group, which pointed out that the protective factors were playing a neuroprotective role after TBI injury.
Therefore, after TBI injury, it is a trend to inhibit its damage factors, especially the progression of inflammatory factors, and promote its protective factors, especially the growth factors and neuropeptides, which will contribute to clinical diagnosis and targeted therapies of TBI in translational medicine and clinical practice.
Conclusion
In this study, we reported the protein expressional profiles in serum from TBI patients, in which the network among inflammatory factors and growth factors may play a crucial role in TBI damage and repair, which, consequently, may contribute to diagnosis and treatment in future translational medicine and clinical practice.
Author Contributions
T-HW was participated in the guidance and design of the study and the revision of the paper. X-YH and Q-QD were responsible for the design of the study, the manuscript writing and revision, ELISA, statistical analysis, and data description. FW was performed the data ordering and analysis, supervised the experiments, and revised the manuscript. NZ, S-JF, and Y-KL were performed the sample collection, data analysis, and revised the manuscript. All authors have read and approved the final version of the manuscript.
Funding
This study was supported by the Program Innovative Research Team in Science and Technology in Yunnan Province (Grant No. 2017HC007).
Conflict of Interest Statement
The authors declare that the research was conducted in the absence of any commercial or financial relationships that could be construed as a potential conflict of interest.
Acknowledgments
We would like to thank Professor Ping Su for his support for sample collection. We also are grateful to Xue-Fei Han and Ya Jiang for the blood biochemical examination. Finally, we express our gratitude to Ping Dai for collection and collation of some clinical data.
Supplementary Material
The Supplementary Material for this article can be found online at: https://www.frontiersin.org/articles/10.3389/fnins.2018.01049/full#supplementary-material
Data Sheet S1 | Basic information of patients for protein chips.
Data Sheet S2 | Human protein chip (1000 proteins included).
Data Sheet S3 | The Differential Expressed Proteins (DEPs).
Data Sheet S4 | The GO and Pathway analysis of downregulated DEPs.
Data Sheet S5 | The GO and Pathway analysis of upregulated DEPs.
Data Sheet S6 | The interactions in tabular form.
Abbreviations
BAD, Bcl-2 associated death promoter; BAX, Bcl-2 associated X protein; BDNF, brain-derived neurotrophic factor; BMPs, bone morphogenetic proteins; CNTF, ciliary neurotrophic factor; CRP, C-reactive protein; CT, computed tomography; GDNF, glial cell-derived neurotrophic factor; HGF, hepatocyte growth factor; HRP, horse radish peroxidase; ICAM2, intercellular adhesion molecule 2; IGF2, insulin like growth factor 2; MMP9, matrix metallopeptidase 9; NGF, nerve growth factor; NPY, neuropeptide Y; NT-3, neurotrophin 3; TGF-β, transforming growth factor β.
Footnotes
References
Ahmed, F., Gyorgy, A., Kamnaksh, A., Ling, G., Tong, L., Parks, S., et al. (2012). Time-dependent changes of protein biomarker levels in the cerebrospinal fluid after blast traumatic brain injury. Electrophoresis 33, 3705–3711. doi: 10.1002/elps.201200299
Alves,ÓL., and Bullock, R. (2001). “Excitotoxic damage in traumatic brain injury,” in Brain Injury. Molecular and Cellular Biology of Critical Care Medicine, Vol. 2, eds R. S. B. Clark and P. Kochanek (Boston, MA: Springer), 1–36. doi: 10.1007/978-1-4615-1721-4_1
Becker, A., Peleg, K., Olsha, O., Givon, A., and Kessel, B. (2018). Analysis of incidence of traumatic brain injury in blunt trauma patients with glasgow coma scale of 12 or less. Chin. J. Traumatol. 21, 152–155. doi: 10.1016/j.cjtee.2018.01.004
Bogoslovsky, T., Wilson, D., Chen, Y., Hanlon, D., Gill, J., Jeromin, A., et al. (2016). Increases of plasma levels of glial fibrillary acidic protein, tau, and amyloid β up to 90 days after traumatic brain injury. J. Neurotrauma 34, 66–73. doi: 10.1089/neu.2015.4333
Brophy, G. M., Mondello, S., Papa, L., Robicsek, S. A., Gabrielli, A., Rd, T. J., et al. (2011). Biokinetic analysis of ubiquitin C-terminal hydrolase-L1 ( UCH-L1 ) in severe traumatic brain injury patient biofluids. J. Neurotrauma 28, 861–870. doi: 10.1089/neu.2010.1564
Brown, A. W., Elovic, E. P., Kothari, S., Flanagan, S. R., and Kwasnica, C. (2008). Congenital and acquired brain injury. 1. Epidemiology, pathophysiology, prognostication, innovative treatments, and prevention. Arch. Phys. Med. Rehabil. 89(3 Suppl. 1), S3–S8. doi: 10.1016/j.apmr.2007.12.001
Bruns, J. J., and Jagoda, A. S. (2009). Mild traumatic brain injury. Mt. Sinai J. Med. 76, 129–137. doi: 10.1002/msj.20101
Chen, M. R., Dai, P., Wang, S. F., Song, S. H., Wang, H. P., Zhao, Y., et al. (2016). BDNF overexpression exhibited bilateral effect on neural behavior in SCT mice associated with AKT signal pathway. Neurochem. Res. 41, 2585–2597. doi: 10.1007/s11064-016-1970-5
Crooks, C. Y., Zumsteg, J. M., and Bell, K. R. (2007). Traumatic brain injury: a review of practice management and recent advances. Phys. Med. Rehabil. Clin. North Am. 18, 681–710. doi: 10.1016/j.pmr.2007.06.005
Feng, G. Y., Liu, J., Wang, Y. C., Wang, Z. Y., Hu, Y., Xia, Q. J., et al. (2017). Effects of alpha-synuclein on primary spinal cord neurons associated with apoptosis and CNTF expression. Cell. Mol. Neurobiol. 37, 817–829. doi: 10.1007/s10571-016-0420-x
Frey, L. C. (2003). Epidemiology of posttraumatic epilepsy: a critical review. Epilepsia 44, 11–17. doi: 10.1046/j.1528-1157.44.s10.4.x
Gao, L., Li, L. H., Xing, R. X., Ou, S., Liu, G. D., Wang, Y. P., et al. (2012). Gastrocnemius-derived BDNF promotes motor function recovery in spinal cord transected rats. Growth Factors 30, 167–175. doi: 10.3109/08977194.2012.678842
Hannay, H., Lezak, M., Howieson, D., Lorig, D., Loring, D., Howieson, D., et al. (2004). Neuropsychological Assessment. New York, NY: Oxford University Press.
Hauer, A. D., Habets, K. L., van Wanrooij, E. J., Vos, P. D., Krueger, J., Reisfeld, R. A., et al. (2009). Vaccination against TIE2 reduces atherosclerosis. Atherosclerosis 204, 365–371. doi: 10.1016/j.atherosclerosis.2008.09.039
Jiang, L., Hu, Y., He, X., Lv, Q., Wang, T. H., and Xia, Q. J. (2017). Breviscapine reduces neuronal injury caused by traumatic brain injury insult: partly associated with suppression of interleukin-6 expression. Neural Regen. Res. 12, 90–95. doi: 10.4103/1673-5374.198990
Kanehisa, M., and Goto, S. (2000). KEGG: kyoto encyclopedia of genes and genomes. Nucleic Acids Res. 28, 27–30. doi: 10.1093/nar/28.1.27
Kochanek, P. M., and Tasker, R. C. (2009). Pediatric neurointensive care: 2008 update for the Rogers’ Textbook of Pediatric Intensive Care. Pediatr. Crit. Care Med. 10, 517–523. doi: 10.1097/PCC.0b013e3181ab88cd
Kushner, D. (1998). Mild traumatic brain injury: toward understanding manifestations and treatment. Arch. Intern. Med. 158, 1617–1624. doi: 10.1001/archinte.158.15.1617
Lamprecht, M. R., Elkin, B. S., Kesavabhotla, K., Crary, J. F., Hammers, J. L., Huh, J. W., et al. (2016). Strong correlation of genome-wide expression after traumatic brain injury In Vitro and In Vivo implicates a role for SORLA. J. Neurotrauma 34, 97–108. doi: 10.1089/neu.2015.4306
Liu, F., Chen, M. R., Liu, J., Zou, Y., Wang, T. Y., Zuo, Y. X., et al. (2016). Propofol administration improves neurological function associated with inhibition of pro-inflammatory cytokines in adult rats after traumatic brain injury. Neuropeptides 58, 1–6. doi: 10.1016/j.npep.2016.03.004
Liu, S. J., Zou, Y., Belegu, V., Lv, L. Y., Lin, N., Wang, T. Y., et al. (2014). Co-grafting of neural stem cells with olfactory en sheathing cells promotes neuronal restoration in traumatic brain injury with an anti-inflammatory mechanism. J. Neuroinflammation 11:66. doi: 10.1186/1742-2094-11-66
Logan, T. T., Villapol, S., and Symes, A. J. (2013). TGF-β superfamily gene expression and induction of the runx1 transcription factor in adult neurogenic regions after brain injury. PLoS One 8:e59250. doi: 10.1371/journal.pone.0059250
Maas, A. I., Stocchetti, N., and Bullock, R. (2008). Moderate and severe traumatic brain injury in adults. Lancet Neurol. 7, 728–741. doi: 10.1016/S1474-4422(08)70164-9
Mondello, S., Schmid, K., Berger, R. P., Kobeissy, F., Italiano, D., Jeromin, A., et al. (2014). The challenge of mild traumatic brain injury: role of biochemical markers in diagnosis of brain damage. Med. Res. Rev. 34, 503–531. doi: 10.1002/med.21295
Nicholl, J., and Lafrance, W. C. J. (2009). Neuropsychiatric sequelae of traumatic brain injury. Semin. Neurol. 29, 247–255. doi: 10.1055/s-0029-1223878
Nwachuku, E. L., Puccio, A. M., Adeboye, A., Chang, Y. F., Kim, J., and Okonkwo, D. O. (2016). Time course of cerebrospinal fluid inflammatory biomarkers and relationship to 6-month neurologic outcome in adult severe traumatic brain injury. Clin. Neurol. Neurosurg. 149, 1–5. doi: 10.1016/j.clineuro.2016.06.009
Ou, S., Liu, G. D., Zhou, L. S., Xia, X., Bai, S. R., Li, J., et al. (2014). Bioinformatics analysis of gene expression profiles in the rat cerebral cortex following traumatic brain injury. Eur. Rev. Med. Pharmacol. Sci. 18, 101–107.
Park, E., Bell, J. D., and Baker, A. J. (2008). Traumatic brain injury: can the consequences be stopped? CMAJ 178, 1163–1170. doi: 10.1503/cmaj.080282
Porth, C. M., and Gaspard, K. J. (2014). Essentials of Pathophysiology: Concepts of Altered Health States, 4th Edn. Philadelphia, PA: Wolters Kluwer.
Puhakka, N., Bot, A. M., Vuokila, N., Debski, K. J., Lukasiuk, K., and Pitkänen, A. (2017). Chronically dysregulated NOTCH1 interactome in the dentate gyrus after traumatic brain injury. PLoS One 12:e0172521. doi: 10.1371/journal.pone.0172521
Qin, S., Yong, Y., Xia, Q. J., Na, L., Wang, Y. C., Jia, L., et al. (2016). Bone marrow stromal cells promote neuronal restoration in rats with traumatic brain injury: involvement of GDNF regulating BAD and BAX signaling. Cell. Physiol. Biochem. 38, 748–762. doi: 10.1159/000443031
Qiu, D. L., and Wang, T. H. (2016). The Expression implication of GDNF in ventral horn and associated remote cortex in rhesus monkeys with hemisected spinal cord injury. Iran. J. Basic Med. Sci. 19, 970–976.
Reinert, M., and Bullock, R. (2002). Clinical trials in head injury. J. Neurotrauma 19, 503–557. doi: 10.1089/089771502753754037
Rostami, E., Krueger, F., Plantman, S., Davidsson, J., Agoston, D., Grafman, J., et al. (2014). Alteration in BDNF and its receptors, full-length and truncated TrkB and p75(NTR) following penetrating traumatic brain injury. Brain Res. 1542, 195–205. doi: 10.1016/j.brainres.2013.10.047
Saatman, K. E., Duhaime, A. C., Bullock, R., Maas, A. I., Valadka, A., and Manley, G. T. (2008). Classification of traumatic brain injury for targeted therapies. J. Neurotrauma 25, 719–738. doi: 10.1089/neu.2008.0586
Scalea, T. M. (2005). “Does it matter how head injured patients are resuscitated?,” in Neurotrauma: Evidence-Based Answer to Common Questions, eds A. B. Valadka and B. T. Andrew (New York, NY: Thieme), 3–5.
Sullivan, P. G., Rabchevsky, A. G., Hicks, R. R., Gibson, T. R., Fletcherturner, A., and Scheff, S. W. (2000). Dose-response curve and optimal dosing regimen of cyclosporin A after traumatic brain injury in rats. Neuroscience 101, 289–295. doi: 10.1016/S0306-4522(00)00380-8
Szklarczyk, D., Franceschini, A., Wyder, S., Forslund, K., Heller, D., Huerta-Cepas, J., et al. (2015). STRING v10: protein-protein interaction networks, integrated over the tree of life. Nucleic Acids Res. 43, D447–D452. doi: 10.1093/nar/gku1003
Thelin, E. P., Just, D., Frostell, A., Häggmark-Månberg, A., Risling, M., Svensson, M., et al. (2016). Protein profiling in serum after traumatic brain injury in rats reveals potential injury markers. Behav. Brain Res. 340, 71–80. doi: 10.1016/j.bbr.2016.08.058
Toyama, Y., Kobayashi, T., Nishiyama, Y., Satoh, K., Ohkawa, M., and Seki, K. (2005). CT for acute stage of closed head injury. Radiat. Med. 23, 309–316.
Tweedie, D., Rachmany, L., Dong, S. K., Rubovitch, V., Lehrmann, E., Zhang, Y., et al. (2016). Mild traumatic brain injury-induced hippocampal gene expressions: the identification of target cellular processes for drug development. J. Neurosci. Methods 272, 4–18. doi: 10.1016/j.jneumeth.2016.02.003
Whigham, K. B., Burns, T. G., and Lageman, S. K. (2011). “National Institute of Neurological Disorders and Stroke,” in Encyclopedia of Clinical Neuropsychology, eds J. S. Kreutzer, J. DeLuca, and B. Caplan (New York, NY: Springer), 1707–1710. doi: 10.1007/978-0-387-79948-3_642
Wang, Y. C., Feng, G. Y., Xia, Q. J., Hu, Y., Xu, Y., Xiong, L. L., et al. (2016). Knockdown of α-synuclein in cerebral cortex improves neural behavior associated with apoptotic inhibition and neurotrophin expression in spinal cord transected rats. Apoptosis 21, 404–420. doi: 10.1007/s10495-016-1218-5
Xiong, L. L., Hu, Y., Zhang, P., Zhang, Z., Li, L. H., Gao, G. D., et al. (2017). Neural stem cell transplantation promotes functional recovery from traumatic brain injury via brain derived neurotrophic factor-mediated neuroplasticity. Mol. Neurobiol. 55, 2696–2711. doi: 10.1007/s12035-017-0551-1
Xiong, L. L., Li, Y., Shang, F. F., Chen, S. W., Chen, H., Ju, S. M., et al. (2016). Chondroitinase administration and pcDNA3.1-BDNF-BMSC transplantation promote motor functional recovery associated with NGF expression in spinal cord-transected rat. Spinal Cord 54, 1088–1095. doi: 10.1038/sc.2016.55
Xiong, Y., Peterson, P. L., and Lee, C. P. (1999). Effect of N-acetylcysteine on mitochondrial function following traumatic brain injury in rats. J. Neurotrauma 16, 1067–1082. doi: 10.1089/neu.1999.16.1067
Keywords: protein network analysis, the serum, ELISA, patients, traumatic brain injury
Citation: He X-Y, Dan Q-Q, Wang F, Li Y-K, Fu S-J, Zhao N and Wang T-H (2019) Protein Network Analysis of the Serum and Their Functional Implication in Patients Subjected to Traumatic Brain Injury. Front. Neurosci. 12:1049. doi: 10.3389/fnins.2018.01049
Received: 16 October 2018; Accepted: 24 December 2018;
Published: 31 January 2019.
Edited by:
Dietrich Ernst Lorke, Florida International University, United StatesReviewed by:
Claus Jürgen Scholz, Independent Researcher, GermanyZhihui Yang, University of Florida, United States
Copyright © 2019 He, Dan, Wang, Li, Fu, Zhao and Wang. This is an open-access article distributed under the terms of the Creative Commons Attribution License (CC BY). The use, distribution or reproduction in other forums is permitted, provided the original author(s) and the copyright owner(s) are credited and that the original publication in this journal is cited, in accordance with accepted academic practice. No use, distribution or reproduction is permitted which does not comply with these terms.
*Correspondence: Ting-Hua Wang, dGluZ2h1YV9uZXVyb25AMjYzLm5ldA== Nan Zhao, emhhby1uYW5AMTYzLmNvbQ==
†These authors have contributed equally to this work