- 1Department of Child and Adolescent Health, School of Public Health, Harbin Medical University, Harbin, China
- 2Department of Biochemistry and Molecular & Cellular Biology, Georgetown University Medical Center, Washington, DC, United States
- 3College of Bioinformatics Science and Technology, Harbin Medical University, Harbin, China
Autism spectrum disorder (ASD) is a complex neurodevelopmental disorder. Aberrant DNA methylation has been observed in ASD but the mechanisms remain largely unknown. Here, we employed discordant monozygotic twins to investigate the contribution of DNA methylation to ASD etiology. Genome-wide DNA methylation analysis was performed using samples obtained from five pairs of ASD-discordant monozygotic twins, which revealed a total of 2,397 differentially methylated genes. Further, such gene list was annotated with Kyoto Encyclopedia of Genes and Genomes and demonstrated predominant activation of neurotrophin signaling pathway in ASD-discordant monozygotic twins. The methylation of SH2B1 gene was further confirmed in the ASD-discordant, ASD-concordant monozygotic twins, and a set of 30 pairs of sporadic case-control by bisulfite-pyrosequencing. The results showed that there was a greater DNA methylation difference in ASD-discordant monozygotic twins than ASD-concordant monozygotic twins. Further, verification of the Chr.16:28856743 of SH2B1 showed significant differences in DNA methylation between case and control. These results suggest abnormal methylation of SH2B1 is associated with ASD etiology. Our data suggest that it might be worthwhile to further explore the functions of SH2B1 and related genes of neurotrophin signaling pathway in ASD.
Introduction
Autism spectrum disorder (ASD) is a group of serious neurodevelopmental disorders defined in an individual by deficits in social interaction and communication, accompanied by restricted interests and stereotypical repetitive behaviors. ASD affects 1–2% of children and confers severe lifelong disability, thus ASD has become a growing clinical and public health problem worldwide (Kim et al., 2011; Elsabbagh et al., 2012; Hill et al., 2015). Traditionally, the etiology for ASD mainly attributed to different genetic variants such as de novo mutations, copy number variations (CNV), and single-nucleotide polymorphisms (SNP) (State and Levitt, 2011; Neale et al., 2012). Although focuses on research efforts have grown in intensity during the past decade and due to the highly clinical and etiological heterogeneous nature of ASD, no findings of biological or clinical markers have been definitively identified. This shows that additional epigenetic or environmental factors are an underlying cause for the susceptibility to ASD. A more recent twin study further suggested that an environmental contribution might be a potentially substantial role for ASD etiology (Hallmayer et al., 2011; Schendel et al., 2014). A large survey of over 14,000 children with ASD in Sweden revealed that heritable factors were found to contribute to about half of ASD risk and the other half were contributed from undetected genetic factors, environmental effects and/or stochastic effects.
The epigenetic mechanisms allow stable regulation of gene expression without altering the DNA sequence that is optimally positioned between the genome and the environment (Vogel Ciernia and LaSalle, 2016). Environmentally driven changes have also propelled the development of neuropsychiatric diseases via the alteration of epigenetic profiles, including classic ASD (Schanen, 2006; Grafodatskaya et al., 2010) and symptomatic ASD such as Rett syndrome and Fragile X syndrome. However, environmental modulation of epigenetic states is poorly understood. Since, monozygotic twins have a DNA sequence in common, the study of discordant monozygotic (MZ) twins provides an ideal model to investigate the value of epigenetic factors in the field of disease etiology. DNA methylation, as the most widely investigated process in epigenetic mechanisms, was considered as a key element that mediates reversible changes in gene expression regardless of DNA sequence variation (Henikoff and Matzke, 1997). Recent advances in high-throughput genomic technology expand our knowledge of DNA methylation. As a result, uncovering this complex relationship will be important for understanding the mechanism of DNA methylation in ASD. Lately, two studies have dissected several ASD-associated differentially methylated loci (Nguyen et al., 2010; Wong et al., 2014), and further support that the peripherally derived DNA can be used to identify disease-associated epigenetic changes. Nonetheless, to date, no study has been reported for the methylomic profile in the samples of Chinese ASD-discordant MZ twins, and it is necessary to investigate the significance of epigenetic mechanism in the Chinese population.
This study was designed to identify the genome-wide DNA methylation patterns in Chinese MZ twins discordant for ASD, and further provide the evidence for ASD associated epigenetic alterations.
Materials and Methods
Samples Recruitment
All the participants were recruited from the Children Development and Behavior Research Center (CDBRC), Harbin Medical University, Heilongjiang Province, China. Altogether, five pairs of ASD-discordant MZ twins, four pairs of ASD-concordant MZ twins and 30 pairs of sporadic patients with aged-, sex-matched controls were invited to participate. More than two experienced psychiatrists independently issued ASD diagnoses according to the criteria of international Diagnostic and Statistical Manual of Mental Disorders, 5th Ed (DSM-5). All probands were also administered by the Autism Diagnostic Observation Schedule-generic (ADOS) (Lord et al., 2000), and were all found to meet the criteria for autism. The ADOS, a semi-structured observational instrument, is made up for four modules to assess social and communicative abilities in individuals suspected of having ASD, in which two different cut-points that depend on symptom severity result in a diagnosis of (i) ASD (milder variant) or (ii) autism (more severe variant). Cases with Rett syndrome, tuberous sclerosis, fragile-X syndrome, and any other neurological conditions suspected to be associated with autism, were excluded by clinical examination and molecular genetic tests of the FMR1 gene (Khaniani et al., 2008). The standard biosecurity and institutional safety procedures for the blood collection have been carried out. This study was performed in accordance with the recommendations of Institutional Research Board of Harbin Medical University (HMUIRB2012006). The protocol was approved by the Institutional Research Board of Harbin Medical University. Safety and fairness principle has been fully considered in the study plans. All subjects gave written informed consent in accordance with the Declaration of Helsinki and the content of the research have no harm or risk.
DNA Extraction and Bisulfite Conversion
Genomic DNA was extracted from whole blood using the QIAamp DNA Mini Kit (Qiagen, Darmstadt, German), and assessed for integrity, quantity, purity and concentration by electrophoresis in a 1% agarose gel and Nanodrop2000 spectrophotometer. Bisulfite conversion of 500 ng of genomic DNA was performed using EZ 96-DNA methylation kit (Zymo Research, Irvine, CA, United States) consistent of manufacturer’s standard protocol. The bisulfite conversion reaction was conducted in duplicate to minimize potential bias caused by variable conversion efficiency, and pooled bisulfite-treated DNA was used for subsequent array experiments.
Infinium Human-Methylation 450BeadChip (450K) Array
Three pairs of ASD-discordant MZ twins for Genome-wide DNA methylation were measured via the Illumina Infinium Human Methylation 450BeadChip assay (Illumina, San Diego, CA, United States), which interrogated the DNA methylation profile of more than 485,000 methylation sites per sample at single-nucleotide resolution. Arrays were scanned by HiScan 2000 (Illumina). Illumina Genome Studio software (Illumina) was used to extract signal intensities for each probe and to perform initial control quality checks. There were no failed samples which needed to be excluded by examined 450K array control probes to assess bisulfite conversion, extension, hybridization, staining, specificity, negative control and others; The ChAMP pipeline was used to normalize and batch correct methylation array data (Johnson et al., 2007; Teschendorff et al., 2013; Morris et al., 2014). Probes that ambiguously mapped or had a high detection p-Value (>0.01), low bead count (<3 beads), and a low success rate (missing in >95% of the samples) were set to missing. Probes with SNPs (MAF ≥ 0.05 in 1000 Genomes Project) at CpG sites were excluded to avoid SNP (single-nucleotide polymorphism) effects on methylation measurement. To investigate if blood cell-composition was similar between the MZ twins, cell type counts (“CD8T,” “CD4T,” “NK,” “Bcell,” “Mono,” and “Gran”) were estimated using the method of Houseman et al. (2012). The difference in methylation level for each pair of twins was calculated by a paired t-test, and it was found that there was no significant difference in blood cell components between them (p > 0.05) (see Supplementary Table 5).
Reduced Representation Bisulfite Sequencing (RRBS)
Two pairs of ASD-discordant MZ twins for Genome-wide DNA methylation were assessed using RRBS (Gu et al., 2011), which contained over 1 million CpG sites. RRBS was an innovative method that specifically enriched genomic regions, which has a high density of potential methylation sites and enabled investigation of DNA methylation at single-nucleotide resolution, and coupled bisulfite conversion and next generation sequencing. 5 μg of genomic DNA was digested overnight with 4 μl MspI (New England Biolabs, Ipswich, MA, United States) to ensure complete digestion and was purified by phenol-chloroform extraction. After end repair and addition of 3′A overhangs, methylated-adaptors were ligated according to the manufacturer’s protocol and purified with AMPure beads (Agencourt Bioscience, Beverly, MA, United States). Two ranges of 150–175 and 175–225 bp adapter-ligated fractions were excised from a 2% agarose gel, respectively. Bisulfite conversion was conducted using the reagents and protocol of EpiTect Bisulfite Kit (Qiagen, Darmstadt, Germany). Subsequently, the bisulfite-converted DNA was amplified by PCR with PfuTurbo Cx Hotstart DNA polymerase to build the PCR-amplified RRBS libraries, which were analyzed by Illumina HiSeq2500 (Illumina). We then used whole genome bisulfite sequence mapping program (BSMAP version 2.74) to map the raw sequencing reads to GRCh37/hg19 reference genome and obtain methylation values of CpG sites. The numbers of unconverted and converted cytosine (C) reads covering the loci for each sample were extracted using python scripts methyratio.py in the BSMAP package.
Methylation Microarray and RRBS Data Processing
Our analysis were performed by R scripts1 and Perl scripts2. Some of the R packages were coded by us and can be applied with request. Overlap of 450K and RRBS data was generated by findOverlap function in GenomicRanges Bioconductor package (Lawrence et al., 2013). MethylKit Bioconductor package was used for methylation correlation and clustering analysis (Akalin et al., 2012; Lawrence et al., 2013). The relative methylation level of each interrogated CpG site was calculated as the ratio of the normalized signal from the methylated probe to the sum of the normalized signals of the methylated and unmethylated probes. A DNA methylation value, described as “β-value” for each CpG site, ranges from 0 (unmethylated) to 1 (fully methylated). β-values with detection p-Value > 0.01 were considered to fall below the minimum intensity and threshold and were consequently removed.
Differentially Methylated CpG Sites Screening
An analytical approach taking advantage of the discordant MZ twin design was applied in order to maximize our chances of identifying real within-twin differences in DNA methylation. Our analysis consisted of two separate tests. The first was a fold-change with the purpose of assessing the significance of DNA methylation differences between the affected and unaffected member in each twin pair, and the second was the one of assessing the size of methylation differences: a delta-β (Δβ) value was calculated representing the mean difference in methylation between the affected and unaffected. We set parameters (fold change ≥ 2 or ≤ 0.5 or |Δβ| ≥ 0.1) as cutoff, because we wanted to be slightly more inclusive and were willing to test a larger number of differentially methylated CpG sites in our downstream replication analyses. The CpG sites which met one of criterions were included, and the two differential loci lists were mapped to UniProt–SwissProt to obtain gene list. In DNA methylation analysis, genes are annotated based on the relative promoter regions, since the primary method for DNA methylation to regulate transcription is through inhibiting transcription binding sites to methylated cytosine at promoter sites upstream of start codon. Further, these genes were taken intersection across all twin pairs. GRCh37 (UCSC hg19, Feb/2009) was used as human genome reference sequence.
Functional Enrichment Analysis of Differentially Methylated Genes
The enrichment analysis for biological processes and gene networks relevant to the pathogenesis of ASD, based on the differentially methylated genes, was conducted using DAVID (version 6.7) online software3. To understand the functions and utilization of biological systems, the intersected list of differential methylation genes (Entrez gene ID annotated) was uploaded into the Kyoto Encyclopedia of Genes and Genomes (KEGG) pathway (Kanehisa et al., 2017), showing the molecular interactions and reaction networks. Hypergeometric model was used to calculate p value and to determine if the number of genes is greater than expected. P-value was subsequently adjusted for multiple testing.
Methylation Pyrosequencing
Pyrosequencing was employed to further validate differences in DNA methylation identified by 450K array and RRBS. Briefly, 500 ng DNA from each individual was treated with sodium bisulfite, which used the EZ96-DNA methylation kit in light of the manufacturer’s recommendation, and amplified by a bisulfite polymerase chain reaction. Quantitative DNA methylation analysis of each CpG was conducted using PSQ96 Pyrosequencer (Qiagen). Primers were designed with an online program MethPrimer4 for Primer F (5′-TTGTATGATTAGGGTAGTTTGTGTGG-3′), primer R (5′-ATTCTTTTCCTCCTTTAATTCTAACT-3′) and sequencing Primer (5′-GTATTGATGGTGATATT-3′) to amplify an amplicon with 174 bp, which corresponded to the region detected by the 450K array and RRBS. The sequence of primers was blasted against a gene bank in NCBI.
Results
Genome-Wide DNA Methylation Profiling Analysis
To determine the genome-wide DNA methylation signal in five pairs of MZ discordant autism, we applied two methods, Infinium 450K array and RRBS from individuals with an ADOS-confirmed diagnosis of autism and their control sibling. Demographic data of the samples involved in this study are presented in Supplementary Table 1. Metrics from array and RRBS passed standard quality control in our analyses. In each sample, % methylation had a bimodal distribution, which indicated that the majority of bases had either high or low methylation. Pairwise Pearson correlation coefficients were calculated among the ten samples within five ASD-discordant MZ twins, and to produce a correlation matrix, which easily compare correlation coefficients between pairs of samples. As expected, a high correlation was observed in genome-wide DNA methylation within each MZ twin (ranged between 0.97 and 1.00), indicating that ASD is not co-related with systemic changes in epigenetic programming (Figure 1A). In order to further verify the similarities observed in methylation status within five ASD-discordant MZ twins, an integrated heat map was generated, which displayed no significant differences in global DNA methylation profile (Figure 1B).
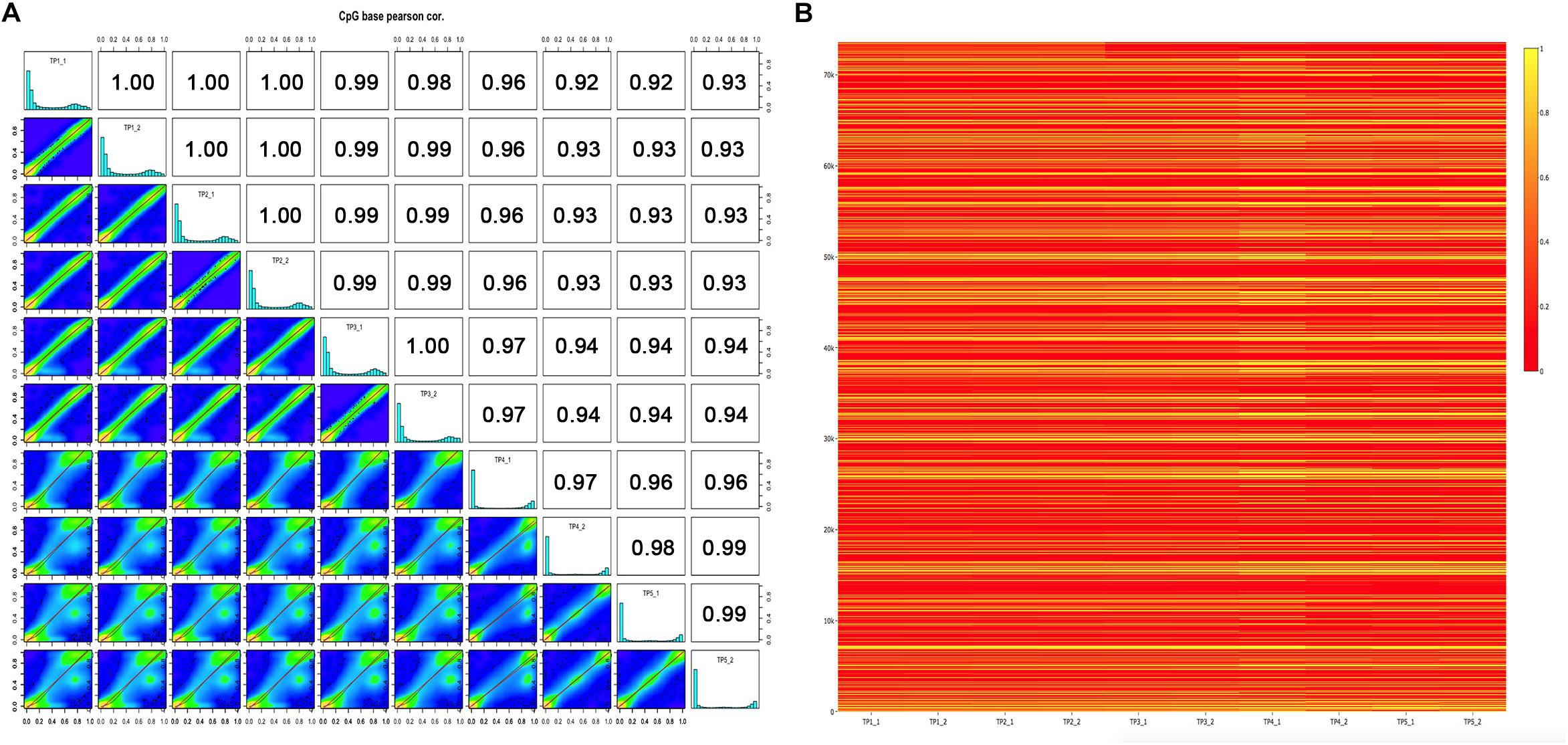
Figure 1. DNA methylation levels of five ASD-discordant monozygotic twins obtained in 450K array and RRBS. (A) Scatter plots for sample pairs. Scatter plots of % methylation values for each pair in five ASD-discordant monozygotic twins. Numbers on upper right corner denote pair-wise Pearson’s correlation scores. The histograms on the diagonal are % methylation histograms per cytosine for each sample. Most of the bases have either high or low methylation. (B) Heat map of methylation CpG sites of five ASD-discordant monozygotic twins. Scaled methylation values are color-coded according to the legend on the right.
Identification of Differentially Methylated CpG Sites
Although there were no significant differences in global DNA methylation levels, DNA methylation at individual CpG sites indicated substantial differential methylated CpGs within ASD-discordant MZ twins pairs, as the phenotypes involved in the comparisons were different. A myriad of sites throughout the genome exhibiting disease-associated differential DNA methylation have been observed within co-twins by combined analysis method (difference and fold change), and these have been further mapped to genes (Table 1). Next, the intersected genes were obtained from two sets of genome-wide methylation data. In the 450K array dataset, there were 2,581 differentially methylated genes intersected in three ASD-discordant MZ pairs and 10,506 differentially methylated genes intersected from RRBS data in two ASD-discordant MZ pairs. Overall, 2,397 intersected genes were identified between the two datasets of five pairs of ASD-discordant MZ twins.
Functional Enrichment Analysis of Differentially Methylated Genes
We performed functional enrichment analysis based on the Kyoto Encyclopedia of Genes and Genomes (KEGG) for the 2,397 differentially methylated intersected genes (see Supplementary Table 2) to examine whether common networks or pathways were over-represented in the list of genes associated with the differentially methylated CpG sites for autism. The results showed that 27 pathways were significantly enriched (p.adjust < 0.05) (Figure 2A and Table 2). Of these pathways, the neurotrophin signaling pathway (KEGG: hsa04722) was selected for further study, because it is involved in many processes in the central nervous system and plays a critical role in the neurogenesis, differentiation and synaptic plasticity (Pardo and Eberhart, 2007; Segura et al., 2015). Indeed, accumulating evidence suggests that neurotrophic factors might play a crucial role in the ASD pathogenesis and represent a group of candidate genes for ASD etiology (Nickl-Jockschat and Michel, 2011). Given these properties, the neurotrophin signal pathway is promising candidates for influencing ASD pathophysiology. Thirty-five genes were enriched in this pathway, including NFKB1, IRAK4, CDC42, KRAS, MAP3K3, MAP3K1, BCL2, GAB1, CAMK2D, PIK3CA, CAMK2B, SH2B1, SHC3, PIK3R3, MAP2K7, AKT3, PIK3R1, MAP2K5, NTF3, BAD, MAPK10, IRS1, TP73, PTPN11, NTRK3, MAPK1, CRKL, MAPK12, PLCG1, RPS6KA1, RPS6KA2, MAPK14, MAPK7, ABL1, and PDPK1 (Figure 2B).
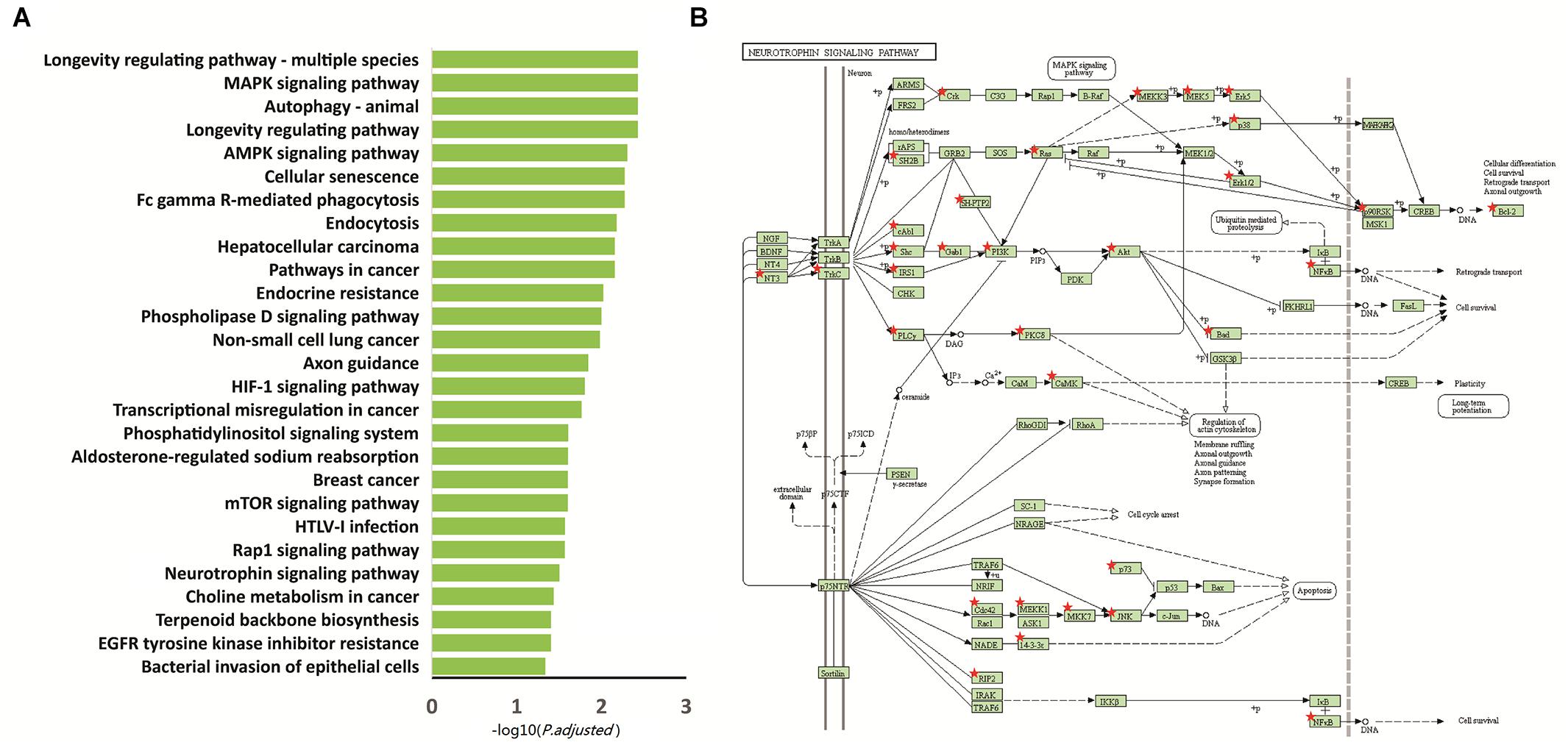
Figure 2. Functional enrichment analysis of the 2,397 intersected differential genes. (A) Relevant pathway enriched of 2,397 intersected differential genes in KEGG pathway. (B) Intersected differential genes enriched in the neurotrophin signaling pathway. Red star: different methylation genes.
Methylation Pyrosequencing
Further analyses sought to determine if these 35 candidate genes were highly represented among differentially methylated genes that are relevant to neurological functions and disorders in other samples. We compared these genes that displayed differentially methylated CpGs in neurotrophin signaling pathway to the electronic database (PubMed). An extensive literature review was conducted on these genes that are linked to ASD. Among them, 30 (85.7%) genes had been implicated in genetics studies on ASD (Xu et al., 2012; Supplementary Table 3). Of particular note is SH2B1, an interesting gene chosen for further bisulfite-pyrosequencing analysis, owing to the location in 16p11.2, which is one of the most frequent genetic etiologies of ASD. Furthermore, there is a greater incidence of obesity in the ASD population and SH2B1 is reported to be causative for obesity. Following up on this result, a replication analysis was verified for three methylation sites (Chr.16:28856729, Chr.16:28856735, and Chr.16:28856743) of SH2B1 in ASD-discordant MZ twins, ASD-concordant MZ twins and a set of 30 pairs sporadic case-control sample.
Five ASD-Discordant MZ Twins
To obtain high-resolution information about specific CpG sites differentially methylated in autism, further validation of disease-associated DNA methylation (Chr.16:28856729, Chr.16:28856735, and Chr.16:28856743) located in SH2B1 was verified by bisulfite-pyrosequencing within five ASD-discordant MZ twins. The size of absolute methylation difference (|Δβ| ≥ 0.1) was used as a cutoff. The Chr.16:28856729 was identified in twin pair 2 exhibiting autism, compared to the unaffected sibling (|Δβ| = 0.1199). The Chr.16:28856743 methylation difference was shown to be associated with ASD in both twin pair 2 (|Δβ| = 0.2436) and twin pair 5 (|Δβ| = 0.2266). The Chr.16:28856735 did not reveal any methylation difference in bisulfite-pyrosequencing, which is likely a disagreement between the high throughput approaches and bisulfite-pyrosequencing. While no overall significant difference existed in DNA methylation at the 450K array and RRBS nominated sites were observed across the five ASD-discordant MZ twins, it was striking that 2 out of the 5 (40%) pairs tested showed marked methylation difference and were clear outliers at SH2B1 (Table 3).
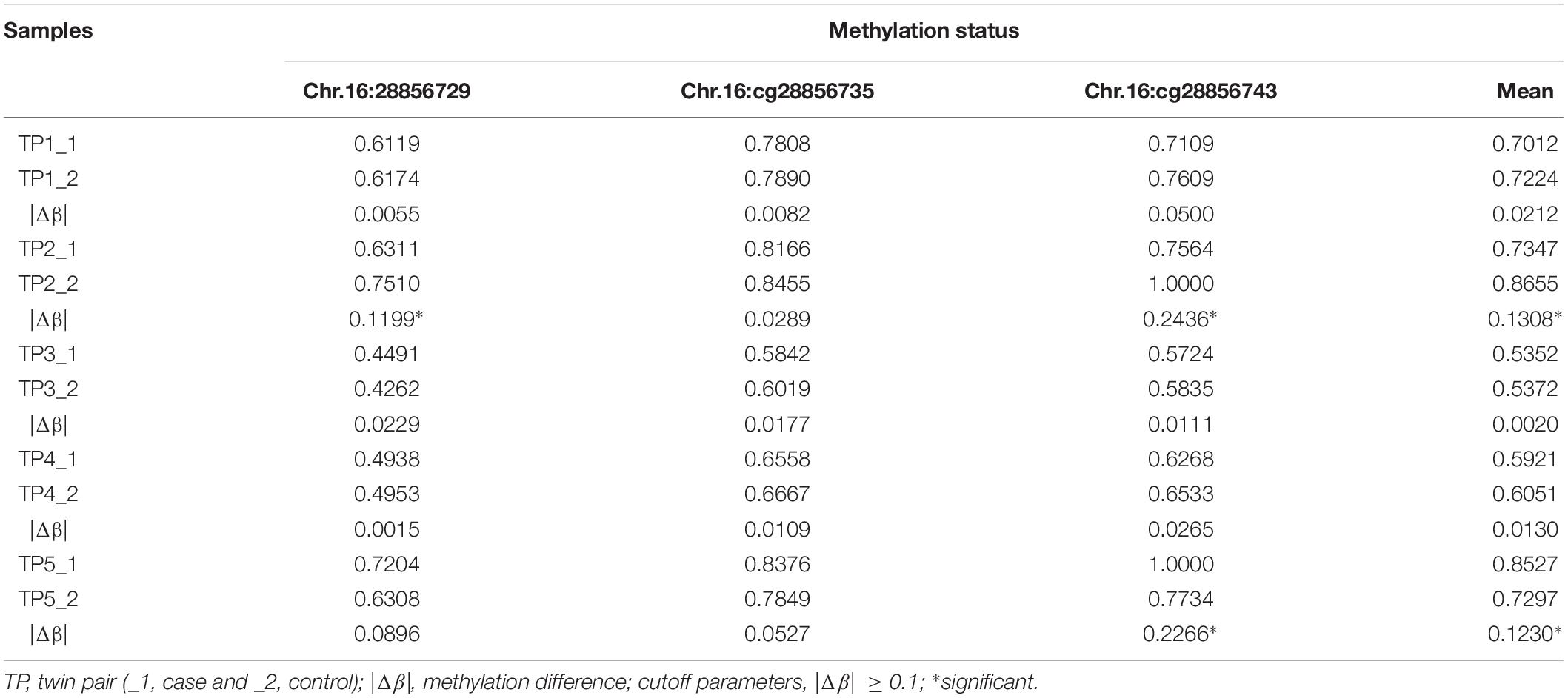
Table 3. Methylation status identified candidate sites of SH2B1 in five ASD-discordant MZ twins by pyrosequencing.
Four ASD-Concordant MZ Twins
The methylation status of the identified candidate sites of SH2B1 were tested by bisulfite-pyrosequencing in four ASD-concordant MZ twins. The results showed that no methylation differences at SH2B1 were observed in ASD-concordant MZ twins, which showed a consistent methylation profile across ASD-concordant MZ twins. The results further suggest that greater DNA methylation differences occurred in ASD-discordant MZ twins than in ASD-concordant MZ twins (Table 4).
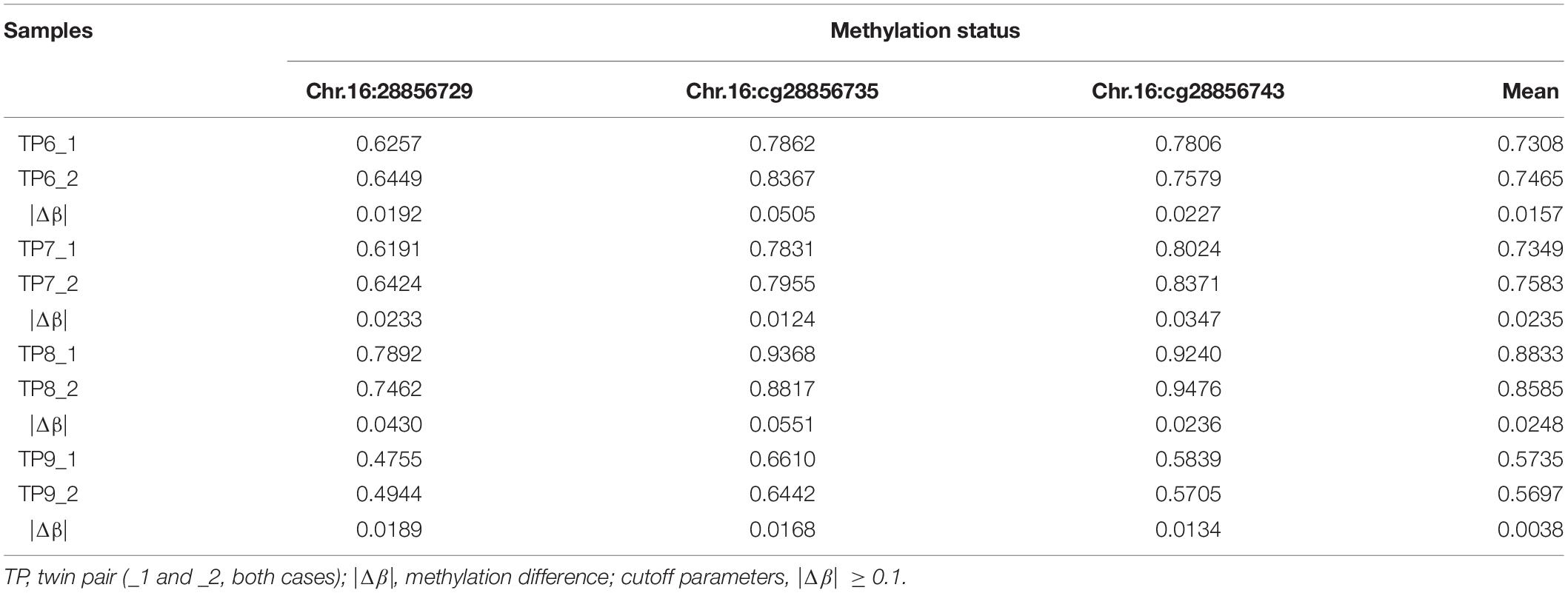
Table 4. Methylation status identified candidate sites of SH2B1 in four ASD-concordant MZ twins by pyrosequencing.
Thirty Pairs of Sporadic Age- and Sex- Match Autism and Controls
To further confirm the accuracy of high throughput data, bisulfite-pyrosequencing was performed on Chr.16:28856729, Chr.16:28856735, and Chr.16:28856743 located at SH2B1 in 30 pairs of sporadic ADOS-diagnosis autism, in comparison to the respective age- and sex-matched controls (see Supplementary Table 4). The DNA methylation on Chr.16:28856743 showed was significantly different between autism and unaffected control (paired t-test, p = 0.035). The mean of these three methylation differential sites also showed statistical significance (paired t-test, p = 0.023), which illustrated the correlation between autism and methylation at this region (Figure 3 and Supplementary Table 4).
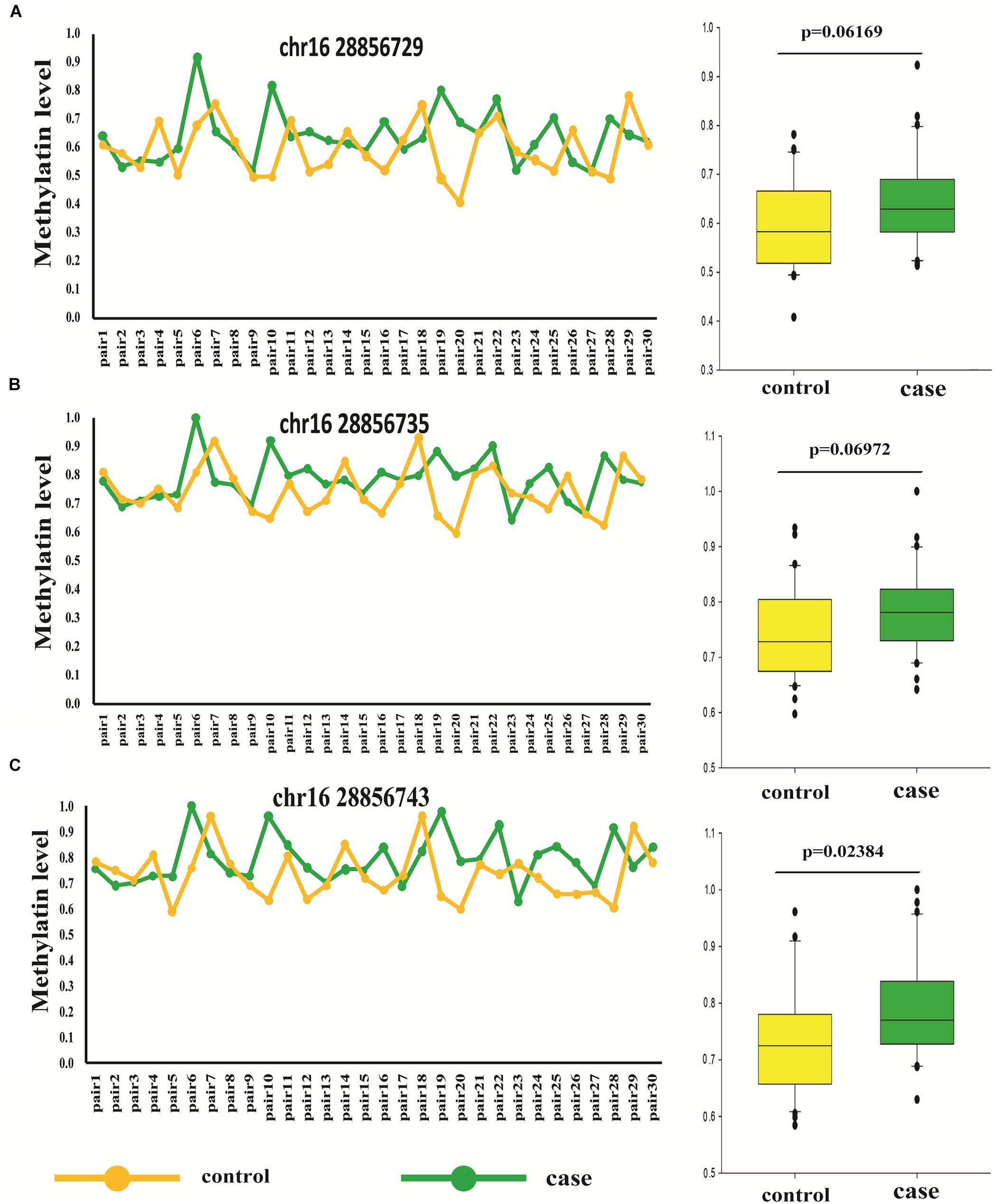
Figure 3. Different methylation loci status of SH2B1 in autism and controls by pyrosequencing (pair t-test). (A) Methylation level of Chr.16:28856729 of SH2B1 in case and control. (B) Methylation level of Chr.16:28856735 of SH2B1 in case and control. (C) Methylation level of Chr.16:28856743 of SH2B1 in case and control. Green, case and yellow, control.
Discussion
In summary, our study showed the novel finding of the epigenetic effect on ASD risk, while genetic factors were controlled. Differences of disease-associated DNA methylation were analyzed comprehensively in Chinese MZ twins discordant for ASD, using two genome-wide approaches. No alterations appeared between affected and unaffected twins in global DNA methylation, whereas, considerable disease-associated differences between-twins at specific loci across the genome have been successfully observed. We identified disease-associated DNA methylation genes which were not previously implicated in ASD. In addition, our data replicated other studies which revealed differential methylated genes implicated in ASD (Nguyen et al., 2010). Pathway analysis of the intersected differential genes highlighted a significant enrichment of epigenetic disruption of neurotrophin signaling pathway, which is relevant to psychiatric disease and neurodevelopment disorders. Specifically, SH2B1, one of the candidate genes whose methylation status was disturbed and further confirmed by bisulfite-pyrosequencing. Overall, our data are proved to support the role of DNA methylation differences in ASD etiology.
There are two reasons to choose SH2B1 for further analysis. First, SH2B1 is located in 16p11.2, which is the one of the most common chromosomal abnormal regions in autism (Veenstra-Vanderweele et al., 2004). The mounting evidences indicated that microdeletion and microduplication in 16p11.2 had been closely related to a wide range of neurodevelopmental conditions including ASD (Kumar et al., 2008; Weiss et al., 2008). Tabet et al. (2012) reported three children of a family with autism, including two monozygotic twins, carrying different rearrangements on the chromosome 16p11.2. Notably, our preliminary research using Affymetrix CytoScan HD platform had demonstrated CNV in 16p11.2 in Han Chinese individuals with ASD (Gazzellone et al., 2014), which suggested that the epigenetic factors of SH2B1 located in 16p11.2 was also involved in ASD.
Second, pediatric overweight and obesity are significant public health concerns. Childhood obesity may persist into adolescence or adulthood and have an increased risk of development related metabolic disorders, including impaired glucosetolerance, hyperinsulinemia, dyslipidemia, type 2 diabetes, non-alcoholic fatty liver disease, and cardiovascular disease (Quattrin et al., 2005; Weiss et al., 2009; Reilly and Kelly, 2011; Katzmarzyk et al., 2012). It is noteworthy that there is a greater incidence of obesity in the ASD population as compared to the general population (Curtin et al., 2005, 2010; Cunningham et al., 2014; de Vinck-Baroody et al., 2015; Dreyer Gillette et al., 2015; Hill et al., 2015). Particularly, the region harboring the SH2B1 gene was reported in early-onset obesity (Bochukova et al., 2010). SH2B1 also participated in neurodevelopmental and other phenotypes, which could promote neurite outgrowth of PC12 cells, hippocampal and cortical neurons (Chen et al., 2015). Deletions of the 16p11.2 harboring SH2B1 were pathogenic and were linked to developmental delay in addition to obesity (Bachmann-Gagescu et al., 2010). Maillard et al. (2015) also showed that the 16p11.2 locus regulated brain structures common to autism and obesity. Criado et al. (2017) indicated that identification of factors of obesity risk in children with ASD served as a prerequisite for addressing long-term individual health burdens and societal costs in this population. The data reported here suggested that epigenetic changes of SH2B1 might confer risk of ASD.
However, there are considerable limitations of the study. First, DNA was extracted from blood rather than the brain tissue. Although we know methylation is the characteristic of tissue-specificity, it is difficult to obtain the brain tissue of ASD. Moreover, the mounting evidences have supported disease-associated methylation loci could be identified from peripheral samples. BCL2 previously reported in brain tissue (Nguyen et al., 2010) was also identified in peripheral blood from our study. Second, the sample size in each group was small. In our study, there were 9 pairs of ASD MZ twins involved. Although ASD-discordant MZ twins and ASD-concordant MZ twins are relatively rare, further evaluation in larger sample is warranted to confirm our findings. Finally, since methylation in regulating gene expression plays a vital role, it is reasonable that CNV and methylomic variation could mediate disease susceptibility through the alteration of gene dosage (Wong et al., 2014). If gene dosage alteration of SH2B1 was obtained, we can further confer the risk of ASD. RNA was not available from peripheral blood when collecting samples, so we have no RNA expression data. Therefore, we could not directly evaluate SH2B1 gene expression. In future study, CNV and RNA expression data should be further added in order to identify discrete SH2B1 showing methylation differences between autism and controls.
The present study suggest that it may also be worthwhile to further explore how SH2B1 and related genes on neurotrophin signaling pathway affect ASD and risk of obesity in the ASD population.
Ethics Statement
This study was carried out in accordance with the recommendations of the Institutional Research Board of the Harbin Medical University (HMUIRB2012006). The protocol was approved by the Institutional Research Board of the Harbin Medical University. Safety and fairness principle has been fully considered in the study plans. All subjects gave written informed consent in accordance with the Declaration of Helsinki and the contents of the research have no harm or risk.
Author Contributions
SL contributed to the array and molecular biology experiments, carried out the data analysis, and wrote the manuscript. ZL, YW, and MZ carried out the data analysis. XY, XL, and XZ contributed to the array and molecular biology experiments. YH, CS, and ZG contributed to the sample collection and data collection. YZ and LW were responsible for the study design, protocol development, interpretation of data, and revised the manuscript. All authors have approved the final version of the manuscript.
Funding
This study was funded by grants from the National Natural Science Foundation of China (81202221 and 81874269), the China Postdoctoral Science Foundation (2014M561375), and the Postdoctoral Science Foundation of Heilongjiang Province (LBH-Z14153).
Conflict of Interest Statement
The authors declare that the research was conducted in the absence of any commercial or financial relationships that could be construed as a potential conflict of interest.
Acknowledgments
We thank all the patients and their parents for their support and participation. We also thank the NIH Fellows Editorial Board (FEB) for editing and polishing the manuscript.
Supplementary Material
The Supplementary Material for this article can be found online at: https://www.frontiersin.org/articles/10.3389/fnins.2019.00712/full#supplementary-material
Footnotes
- ^ http://www.r-project.org
- ^ https://www.perl.org/
- ^ https://david.ncifcrf.gov/
- ^ https://www.urogene.org/methprimer/
References
Akalin, A., Kormaksson, M., Li, S., Garrett-Bakelman, F. E., Figueroa, M. E., Melnick, A., et al. (2012). methylKit: a comprehensive R package for the analysis of genome-wide DNA methylation profiles. Genome Biol. 13:R87. doi: 10.1186/gb-2012-13-10-r87
Bachmann-Gagescu, R., Mefford, H. C., Cowan, C., Glew, G. M., Hing, A. V., Wallace, S., et al. (2010). Recurrent 200-kb deletions of 16p11.2 that include the SH2B1 gene are associated with developmental delay and obesity. Genet. Med. 12, 641–647. doi: 10.1097/GIM.0b013e3181ef4286
Bochukova, E. G., Huang, N., Keogh, J., Henning, E., Purmann, C., Blaszczyk, K., et al. (2010). Large, rare chromosomal deletions associated with severe early-onset obesity. Nature 463, 666–670. doi: 10.1038/nature08689
Chen, K. W., Chang, Y. J., and Chen, L. (2015). SH2B1 orchestrates signaling events to filopodium formation during neurite outgrowth. Commun. Integr. Biol. 8:e1044189. doi: 10.1080/19420889.2015.1044189
Criado, K. K., Sharp, W. G., McCracken, C. E., De Vinck-Baroody, O., Dong, L., Aman, M. G., et al. (2017). Overweight and obese status in children with autism spectrum disorder and disruptive behavior. Autism 22, 450–459. doi: 10.1177/1362361316683888
Cunningham, S. A., Kramer, M. R., and Narayan, K. M. (2014). Incidence of childhood obesity in the United States. N. Engl. J. Med. 370, 403–411. doi: 10.1056/NEJMoa1309753
Curtin, C., Anderson, S. E., Must, A., and Bandini, L. (2010). The prevalence of obesity in children with autism: a secondary data analysis using nationally representative data from the National Survey of Children’s Health. BMC Pediatr. 10:11. doi: 10.1186/1471-2431-10-11
Curtin, C., Bandini, L. G., Perrin, E. C., Tybor, D. J., and Must, A. (2005). Prevalence of overweight in children and adolescents with attention deficit hyperactivity disorder and autism spectrum disorders: a chart review. BMC Pediatr. 5:48. doi: 10.1186/1471-2431-5-48
de Vinck-Baroody, O., Shui, A., Macklin, E. A., Hyman, S. L., Leventhal, J. M., and Weitzman, C. (2015). Overweight and obesity in a sample of children with autism spectrum disorder. Acad. Pediatr. 15, 396–404. doi: 10.1016/j.acap.2015.03.008
Dreyer Gillette, M. L., Borner, K. B., Nadler, C. B., Poppert, K. M., Odar Stough, C., Swinburne Romine, R., et al. (2015). Prevalence and health correlates of overweight and obesity in children with autism spectrum disorder. J. Dev. Behav. Pediatr. 36, 489–496.
Elsabbagh, M., Divan, G., Koh, Y. J., Kim, Y. S., Kauchali, S., Marcin, C., et al. (2012). Global prevalence of autism and other pervasive developmental disorders. Autism Res. 5, 160–179. doi: 10.1002/aur.239
Gazzellone, M. J., Zhou, X., Lionel, A. C., Uddin, M., Thiruvahindrapuram, B., Liang, S., et al. (2014). Copy number variation in Han Chinese individuals with autism spectrum disorder. J. Neurodev. Disord. 6:34. doi: 10.1186/1866-1955-6-34
Grafodatskaya, D., Chung, B., Szatmari, P., and Weksberg, R. (2010). Autism spectrum disorders and epigenetics. J. Am. Acad. Child Adolesc. Psychiatry 49, 794–809. doi: 10.1016/j.jaac.2010.05.005
Gu, H., Smith, Z. D., Bock, C., Boyle, P., Gnirke, A., and Meissner, A. (2011). Preparation of reduced representation bisulfite sequencing libraries for genome-scale DNA methylation profiling. Nat. Protoc. 6, 468–481. doi: 10.1038/nprot.2010.190
Hallmayer, J., Cleveland, S., Torres, A., Phillips, J., Cohen, B., Torigoe, T., et al. (2011). Genetic heritability and shared environmental factors among twin pairs with autism. Arch. Gen. Psychiatry 68, 1095–1102.
Henikoff, S., and Matzke, M. A. (1997). Exploring and explaining epigenetic effects. Trends Genet. 13, 293–295. doi: 10.1016/s0168-9525(97)01219-5
Hill, A. P., Zuckerman, K. E., and Fombonne, E. (2015). Obesity and autism. Pediatrics 136, 1051–1061.
Houseman, E. A., Accomando, W. P., Koestler, D. C., Christensen, B. C., Marsit, C. J., Nelson, H. H., et al. (2012). DNA methylation arrays as surrogate measures of cell mixture distribution. BMC Bioinformatics 13:86. doi: 10.1186/1471-2105-13-86
Johnson, W. E., Li, C., and Rabinovic, A. (2007). Adjusting batch effects in microarray expression data using empirical Bayes methods. Biostatistics 8, 118–127. doi: 10.1093/biostatistics/kxj037
Kanehisa, M., Furumichi, M., Tanabe, M., Sato, Y., and Morishima, K. (2017). KEGG: new perspectives on genomes, pathways, diseases and drugs. Nucleic. Acids Res. 5, D353–D361. doi: 10.1093/nar/gkw1092
Katzmarzyk, P. T., Shen, W., Baxter-Jones, A., Bell, J. D., Butte, N. F., Demerath, E. W., et al. (2012). Adiposity in children and adolescents: correlates and clinical consequences of fat stored in specific body depots. Pediatr. Obes. 7, e42–e61. doi: 10.1111/j.2047-6310.2012.00073.x
Khaniani, M. S., Kalitsis, P., Burgess, T., and Slater, H. R. (2008). An improved diagnostic PCR assay for identification of cryptic heterozygosity for CGG triplet repeat alleles in the fragile X gene (FMR1). Mol. Cytogenet. 1:5. doi: 10.1186/1755-8166-1-5
Kim, Y. S., Leventhal, B. L., Koh, Y. J., Fombonne, E., Laska, E., Lim, E. C., et al. (2011). Prevalence of autism spectrum disorders in a total population sample. Am. J. Psychiatry 168, 904–912. doi: 10.1176/appi.ajp.2011.10101532
Kumar, R. A., KaraMohamed, S., Sudi, J., Conrad, D. F., Brune, C., Badner, J. A., et al. (2008). Recurrent 16p11.2 microdeletions in autism. Hum. Mol. Genet. 17, 628–638. doi: 10.1093/hmg/ddm376
Lawrence, M., Huber, W., Pages, H., Aboyoun, P., Carlson, M., Gentleman, R., et al. (2013). Software for computing and annotating genomic ranges. PLoS Comput. Biol. 9:e1003118. doi: 10.1371/journal.pcbi.1003118
Lord, C., Risi, S., Lambrecht, L., Cook, E. H. Jr., Leventhal, B. L., DiLavore, P. C., et al. (2000). The autism diagnostic observation schedule-generic: a standard measure of social and communication deficits associated with the spectrum of autism. J. Autism Dev. Disord. 30, 205–223.
Maillard, A. M., Ruef, A., Pizzagalli, F., Migliavacca, E., Hippolyte, L., Adaszewski, S., et al. (2015). The 16p11.2 locus modulates brain structures common to autism, schizophrenia and obesity. Mol. Psychiatry 20, 140–147. doi: 10.1038/mp.2014.145
Morris, T. J., Butcher, L. M., Feber, A., Teschendorff, A. E., Chakravarthy, A. R., Wojdacz, T. K., et al. (2014). ChAMP: 450k chip analysis methylation pipeline. Bioinformatics 30, 428–430. doi: 10.1093/bioinformatics/btt684
Neale, B. M., Kou, Y., Liu, L., Ma’ayan, A., Samocha, K. E., Sabo, A., et al. (2012). Patterns and rates of exonic de novo mutations in autism spectrum disorders. Nature 485, 242–245. doi: 10.1038/nature11011
Nguyen, A., Rauch, T. A., Pfeifer, G. P., and Hu, V. W. (2010). Global methylation profiling of lymphoblastoid cell lines reveals epigenetic contributions to autism spectrum disorders and a novel autism candidate gene, RORA, whose protein product is reduced in autistic brain. FASEB J. 24, 3036–3051. doi: 10.1096/fj.10-154484
Nickl-Jockschat, T., and Michel, T. M. (2011). The role of neurotrophic factors in autism. Mol. Psychiatry 16, 478–490. doi: 10.1038/mp.2010.103
Quattrin, T., Liu, E., Shaw, N., Shine, B., and Chiang, E. (2005). Obese children who are referred to the pediatric endocrinologist: characteristics and outcome. Pediatrics 115, 348–351. doi: 10.1542/peds.2004-1452
Reilly, J. J., and Kelly, J. (2011). Long-term impact of overweight and obesity in childhood and adolescence on morbidity and premature mortality in adulthood: systematic review. Int. J. Obes. 35, 891–898. doi: 10.1038/ijo.2010.222
Schendel, D. E., Gronborg, T. K., and Parner, E. T. (2014). The genetic and environmental contributions to autism: looking beyond twins. JAMA 311, 1738–1739.
Segura, M., Pedreno, C., Obiols, J., Taurines, R., Pamias, M., Grunblatt, E., et al. (2015). Neurotrophin blood-based gene expression and social cognition analysis in patients with autism spectrum disorder. Neurogenetics 16, 123–131. doi: 10.1007/s10048-014-0434-9
State, M. W., and Levitt, P. (2011). The conundrums of understanding genetic risks for autism spectrum disorders. Nat. Neurosci. 14, 1499–1506. doi: 10.1038/nn.2924
Tabet, A. C., Pilorge, M., Delorme, R., Amsellem, F., Pinard, J. M., Leboyer, M., et al. (2012). Autism multiplex family with 16p11.2p12.2 microduplication syndrome in monozygotic twins and distal 16p11.2 deletion in their brother. Eur. J. Hum. Genet. 20, 540–546. doi: 10.1038/ejhg.2011.244
Teschendorff, A. E., Marabita, F., Lechner, M., Bartlett, T., Tegner, J., Gomez-Cabrero, D., et al. (2013). A beta-mixture quantile normalization method for correcting probe design bias in Illumina Infinium 450 k DNA methylation data. Bioinformatics 29, 189–196. doi: 10.1093/bioinformatics/bts680
Veenstra-Vanderweele, J., Christian, S. L., and Cook, E. H. Jr. (2004). Autism as a paradigmatic complex genetic disorder. Annu. Rev. Genomics Hum. Genet. 5, 379–405. doi: 10.1146/annurev.genom.5.061903.180050
Vogel Ciernia, A., and LaSalle, J. (2016). The landscape of DNA methylation amid a perfect storm of autism aetiologies. Nat. Rev. Neurosci. 17, 411–423. doi: 10.1038/nrn.2016.41
Weiss, L. A., Shen, Y., Korn, J. M., Arking, D. E., Miller, D. T., Fossdal, R., et al. (2008). Association between microdeletion and microduplication at 16p11.2 and autism. N. Engl. J. Med. 358, 667–675.
Weiss, R., Shaw, M., Savoye, M., and Caprio, S. (2009). Obesity dynamics and cardiovascular risk factor stability in obese adolescents. Pediatr. Diabetes 10, 360–367. doi: 10.1111/j.1399-5448.2008.00504.x
Wong, C. C., Meaburn, E. L., Ronald, A., Price, T. S., Jeffries, A. R., Schalkwyk, L. C., et al. (2014). Methylomic analysis of monozygotic twins discordant for autism spectrum disorder and related behavioural traits. Mol. Psychiatry 19, 495–503. doi: 10.1038/mp.2013.41
Keywords: autism spectrum disorder, monozygotic twins, discordant, DNA methylation, SH2B1
Citation: Liang S, Li Z, Wang Y, Li X, Yang X, Zhan X, Huang Y, Gao Z, Zhang M, Sun C, Zhang Y and Wu L (2019) Genome-Wide DNA Methylation Analysis Reveals Epigenetic Pattern of SH2B1 in Chinese Monozygotic Twins Discordant for Autism Spectrum Disorder. Front. Neurosci. 13:712. doi: 10.3389/fnins.2019.00712
Received: 01 November 2018; Accepted: 24 June 2019;
Published: 17 July 2019.
Edited by:
Leonard C. Schalkwyk, University of Essex, United KingdomReviewed by:
Claus Jürgen Scholz, Labor Dr. Wisplinghoff, GermanyEmma Louise Dempster, University of Exeter, United Kingdom
Copyright © 2019 Liang, Li, Wang, Li, Yang, Zhan, Huang, Gao, Zhang, Sun, Zhang and Wu. This is an open-access article distributed under the terms of the Creative Commons Attribution License (CC BY). The use, distribution or reproduction in other forums is permitted, provided the original author(s) and the copyright owner(s) are credited and that the original publication in this journal is cited, in accordance with accepted academic practice. No use, distribution or reproduction is permitted which does not comply with these terms.
*Correspondence: Lijie Wu, d3VsaWppZWh5ZEAxMjYuY29t; Yan Zhang, dHlvemhhbmdAZW1zLmhyYm11LmVkdS5jbg==