- 1Brain Research Center, Department of Acupuncture & Tuina, Chengdu University of Traditional Chinese Medicine, Chengdu, China
- 2Department of Radiology, Hospital of Chengdu University of Traditional Chinese Medicine, Chengdu, China
Primary dysmenorrhea (PDM) is a common complaint in women throughout the menstrual years. Acupuncture has been shown to be effective in dysmenorrhea; however, there are large interindividual differences in patients’ responses to acupuncture treatment. Fifty-four patients with PDM were recruited and randomized into real or sham acupuncture treatment groups (over the course of three menstrual cycles). Pain-related functional connectivity (FC) matrices were constructed at baseline and post-treatment period. The different neural mechanisms altered by real and sham acupuncture were detected with multivariate analysis of variance. Multivariate pattern analysis (MVPA) based on a machine learning approach was used to explore whether the different FC patterns predicted the acupuncture treatment response in the PDM patients. The results showed that real but not sham acupuncture significantly relieved pain severity in PDM patients. Real and sham acupuncture displayed differences in FC alterations between the descending pain modulatory system (DPMS) and sensorimotor network (SMN), the salience network (SN) and SMN, and the SN and default mode network (DMN). Furthermore, MVPA found that these FC patterns at baseline could predict the acupuncture treatment response in PDM patients. The present study verified differentially altered brain mechanisms underlying real and sham acupuncture in PDM patients and supported the use of neuroimaging biomarkers for individual-based precise acupuncture treatment in patients with PDM.
Introduction
Primary dysmenorrhea (PDM), cyclic menstrual pain in the absence of pelvic anomalies, is a common, and often debilitating, gynecological condition that affects between 45 and 95% of menstruating women (Coco, 1999). Despite the high incidence rate of PDM, it is unfortunately often underdiagnosed and poorly treated (O’Connell et al., 2006). Acupuncture, a traditional Chinese medicine procedure, has been widely used to alleviate diverse types of pain for over 2000 years (Zhao, 2008). The National Institutes of Health has also recommended acupuncture as an effective tool for certain health problems, including menstrual pain (Campbell and McGrath, 1999). Subsequently, the efficacy and safety of acupuncture for PDM have been reported in our systematic review (Yu et al., 2017) and several randomized controlled trials (RCTs) (Witt et al., 2008; Ma et al., 2013; Armour et al., 2017).
Although acupuncture has been shown to be effective in PDM, patients’ responses to acupuncture treatment vary widely between individuals (Liu et al., 2017; Liu et al., 2019; Tu et al., 2019b). In addition, responses to other analgesic therapies have also been characterized by robust individual differences (Coghill and Eisenach, 2003; Wager et al., 2011; Angst et al., 2012). If we can identify the interindividual differences in pain processing, this would greatly help to achieve improved and personalized treatment. By definition, pain is a subjective and highly personal experience, and treatment outcomes are likely to be affected by an individual’s baseline characteristics, such as demographic characteristics (e.g., sex, race, and age), levels of clinical pain and some objective biological markers (Wandner et al., 2012; Fillingim, 2017). Thus, baseline characteristics of individuals would be useful to predict the differential response to intervention strategies. Previous research has found that baseline clinical and demographic factors influence treatment response, but these characteristics have not achieved the accuracy required for prediction (Underwood et al., 2007; Azevedo et al., 2019; Witt et al., 2019). Some studies have focused on quantitative sensory testing (QST) in the prediction of analgesic effects, but with contradictory results (Grosen et al., 2013). In light of these studies showing limited individual predictive value for clinical measures, brain-based biomarkers have recently shown promise at predicting response to treatment (Chen et al., 2018; Reggente et al., 2018).
PDM has been proposed to be part of the central sensitization syndromes together with several chronic pain conditions, including fibromyalgia, irritable bowel syndrome, idiopathic low back pain, headache and migraine (Yunus, 2012; Iacovides et al., 2015). In recent years, using neuroimaging techniques, our group (Shen et al., 2019; Zhang et al., 2019) and other groups (Tu et al., 2009; Liu et al., 2018; Chen et al., 2019) have confirmed that PDM is associated with significant changes in the central nervous system’s anatomy, metabolism, and resting-state function. Although the exact mechanisms of the analgesic effects of acupuncture are not known, studies have postulated that acupuncture can alleviate pain by modulating brain regions and networks associated with pain processing (Chen et al., 2015; Maeda et al., 2017). Neuroimaging research has demonstrated that chronic pain may be associated with alterations in multiple brain networks, such as the default mode network (DMN), sensorimotor network (SMN), salience network (SN), and descending modulation pathways (DPMS) (Kucyi and Davis, 2015; Kucyi and Davis, 2017). These particular networks, involved in the cognitive, sensorimotor, and affective aspects of pain, have been implicated in the core symptomatology of chronic pain and treatment response (Shi et al., 2015; Wu et al., 2016; Low et al., 2017; Lee et al., 2019; Zhang et al., 2019). However, it remains largely unknown where and how specific changes in these pain-related networks give rise to symptom improvement in patients with PDM after acupuncture treatment and whether network-level markers can predict the clinical response before intervention.
In this study, we used a longitudinal study design to investigate brain plasticity following acupuncture treatment. Our main aim was to explore whether particular pretreatment functional connectivity (FC) patterns (including those of the DMN, SMN, SN and DPMS) would predict the real and sham acupuncture response in PDM patients. First, we assessed FC alterations after acupuncture over the course of three menstrual cycles. Second, the different FC alterations between real and sham acupuncture treatment were explored. Third, we used multivariate pattern analyses (MVPA) based on a machine learning approach (support vector regression, SVR) to explore whether the different FC patterns predicted the acupuncture treatment response in PDM patients. We hypothesized that there were different neural mechanisms underlying real and sham acupuncture treatment that could predict the treatment response in PDM patients.
Materials and Methods
Participants
Fifty-four patients with PDM were recruited from advertisements and word of mouth to participate in a dysmenorrhea study, and all participants were screened using telephone and in-person structured interviews. The Research Ethics Committee of Chengdu university of Traditional Chinses Medicine (CDUTCM) approved this study, and all participants gave written informed consent. The inclusion criteria for patients with PDM were (1) a regular menstrual cycle (27–32 days); (2) a history of PDM longer than 1 year; (3) no exogenous hormones or centrally acting medication in the last 6 months; (4) lower quadrant abdominal pain (including cramping, swelling, tingling, etc.) during menstruation in the last 6 months rated higher than 4 on a visual analog scale (VAS) (0 = not at all, 10 = the worst pain sensation); and (5) right-handedness, as confirmed by the Edinburgh Handedness Inventory (Oldfield, 1971). The exclusion criteria for patients with PDM were as follows: (1) other chronic pain conditions, such as low back pain; (2) organic pelvic disease or abnormalities found in gynecological ultrasonography; (3) visceral pain and other neurology that may cause hyperalgesia; (4) a positive pregnancy test or plan for pregnancy; (5) a neurologic or psychiatric disorder history; and (6) any contraindication for MRI scanning. Ten patients dropped out before the baseline clinical assessment and MRI scan, and nine patients dropped out during the treatment period. All the participants did not have any acupuncture experience. Finally, thirty-five patients (20 in the real and 15 in the sham acupuncture groups) completed all the clinical assessments and image scans and received real or sham acupuncture treatment during 3 menstrual cycles (Table 1). The details of the study design can be found in Figure 1.
Clinical Assessment
The primary outcome assessed in this trial was abdominal pain severity, as measured by the 0–10 VAS rom “no pain at all” to “unbearable pain (Larroy, 2002). In addition, the self-rating anxiety scale (SAS) and self-rating depression scale (SDS) were applied as secondary outcomes to evaluate the anxiety and depression levels of the PDM patients (Zung et al., 1965; Zung, 1971). All clinical outcomes were measured at baseline and after completion of three sessions of treatment during the periovulatory phase (days 12–16 of the menstrual cycle).
Acupuncture Treatment
Patients were randomized using a computer-generated, random-allocation sequence and then assigned to either the real acupuncture group or the sham acupuncture group. All patients and study staff were blinded to the treatment groups. Only the acupuncturist, who had to know whether to deliver real or sham treatment, was not blinded.
Real Acupuncture Treatment
For the real acupuncture treatment group, sanyinjiao (SP6) was selected based on data mining from our previous review and expert opinions. SP6 is located on the tibial aspect of the leg, posterior to the medial border of the tibia and 3 cun (proportional bone cun), above the medial malleolus (World Health Organization, 2008). The acupuncture procedures were as follows: after the skin was cleaned with tincture of iodine and alcohol, 0.25 × 40 mm stainless needles (Hwatuo, Suzhou, China) were inserted 1.0–1.2 cun and gently twisted, lifted and thrust with even amplitude, force and speed four to six times until deqi was obtained (soreness, numbness, distension and heaviness). Needles were retained at the acupoints for 30 min, and the above manipulation was repeated twice every 10 min for 30 s each time.
Sham Acupuncture Treatment
An adjacent sham acupoint located at the midpoint between the stomach and gall bladder meridians in the same level of SP6 and xuanzhong (GB39) was selected in the sham group (Ma et al., 2010). The patients in this group underwent an acupuncture procedure similar to the patients in the real acupuncture group, but no needle manipulation was performed after needle insertion, and deqi sensation was not obtained. The real and sham acupuncture targets are displayed in Supplementary Figure S1.
The acupuncture interventions for both groups were performed by two licensed acupuncturists with over 3 years of experience. Acupuncture treatments started 7 days before the beginning of menses and did not stop until the onset of the next menstruation. All participants received acupuncture treatment once a day, there were 7 days in a session, and there were 3 sessions over 3 menstrual cycles.
Imaging Acquisition
All participants underwent two MRI scans on the same 3.0-Tesla magnetic resonance scanner (Discovery MR750, General Electric, Milwaukee, WI, United States) in the Department of Radiology at the Affiliated Hospital of CDUTCM at baseline and the forth periovulatory phase after each clinical assessment. Tight, but comfortable, foam padding was used to minimize head motion, and earplugs were used to reduce scanner noise. Sagittal 3D T1-weighted images were acquired using a brain volume sequence with the following parameters: repetition time (TR) = 8.16 ms; echo time (TE) = 3.18 ms; flip angle (FA) = 7°; field of view (FOV) = 256 × 256 mm; matrix = 256 × 256; slice thickness = 1 mm, no gap; and 188 sagittal slices. The resting-state functional fMRI (rs-fMRI) datasets were obtained in 7 min with a gradient-recalled echo-planar imaging pulse sequence. The rs-fMRI imaging parameters were TR = 2000 ms, TE = 30 ms, FA = 90°, acquisition matrix = 64 × 64, FOV = 240 × 240 mm, thickness = 4.0 mm, voxel size = 3.5 × 3.5 × 4.02 mm3, gap = 0.5 mm, NEX = 1.0, and number of slices = 33. A total of 210 volumes were acquired. All subjects were scanned during the first three days of the menstrual phase. During the data scans, all subjects were instructed to relax and maintain closed eyes, and all participants reported that they did not fall asleep during the scanning.
fMRI Data Preprocessing
MRI data were preprocessed and analyzed using the SPM8 toolbox1 implemented in MATLAB 8.0 (Mathworks Inc., Sherborn, MA, United States). Structural images were coregistered with resting-state functional images. Conventional preprocessing steps were performed, which included (1) removing the first 5 time points; (2) slice timing correction; (3) realignment (participants with head motion greater than 1.5mm maximum displacement in any direction (x, y, z) or 1.5° of angular motion were excluded); (4) normalization of images with a T1 template in the Montreal Neurological Institute (MNI) atlas space and resampling to 3? × 3 × 3mm3 cubic voxels; (5) linear detrending; (6) nuisance covariate regression including six motion parameters, average signals of cerebrospinal fluid and white matter, and time points having spike motion of framewise displacement (FD) > 0.5; (7) temporal filtering (bandpass 0.01–0.1 Hz); and (8) spatial smoothing using a Gaussian kernel of 6-mm full-width at half-maximum (FWHM). The images with all preprocessing steps were used for region of interest (ROI) to ROI functional connectivity analysis, while the images after the first four preprocessing steps were used for group independent component analysis (GICA). There were no differences in head motion parameters (FD) within or between groups.
ROI Selection and Functional Connectivity Analyses
First, GICA analysis was conducted using group ICA of the fMRI toolbox (GIFT 3.0b, Medical Image Analysis Lab, University of New Mexico, Albuquerque, NM, United States) implemented in MATLAB 8.0 and SPM8. A set of independent components (ICs) were identified as intrinsic resting-state networks in all subjects at both the baseline and post-treatment periods (Calhoun et al., 2001). The optimal number of ICs was automatically estimated by minimum description length (MDL) criteria in GIFT, and the median of the MDL over all subjects was 66 (Li et al., 2006). The ICA components were calculated by infomax algorithms, and spatial maps were transformed to z-scores.
Second, the default mode network (DMN), sensorimotor network (SMN), and salience network (SN) were chosen as components of interest to be evaluated from the resting-state data. ten ROIs in these resting-state networks (RSNs) were manually chosen from the 66 extracted ICs, which were identified as anatomically and functionally classical RSNs by two experienced neuroimaging researchers (YJ and YSY) (Smith et al., 2009). Four ROIs in the DMN, including the medial prefrontal cortex (mPFC), posterior cingulate cortex (PCC) and bilateral inferior parietal cortices (IPC), are involved in pain rumination (Kumbhare et al., 2017). Four ROIs in the SN, including the bilateral dorsolateral prefrontal cortices (dlPFC) and bilateral anterior insula (aINS) represent the sustained activation during attention to pain (Kucyi and Davis, 2015, 2017), and descending pathways that modulate the transmission of ascending nociceptive signals (Hemington et al., 2016; Davis et al., 2017). Bilateral primary somatosensory cortices (S1) and bilateral thalami in the SMN represent the major ascending pathways of pain (Tracey and Mantyh, 2007; Davis et al., 2017). In addition, two key regions in the descending pain modulatory system, the periaqueductal gray (PAG) and rostroventral medulla (RVM) (Goksan et al., 2018), were also selected as ROIs for the pain-related FC matrix in PDM. The ten cortical ROIs were saved as masks by one sample t-test by using SPM8. The subcortical ROI of the thalamus in the SMN was acquired from automated anatomical labeling, and the PAG and RVM masks were acquired from the DPMS network mask in standard space (Goksan et al., 2018; Figure 2).
Third, an ROI-wise FC matrix was constructed using the REST toolbox2. The averaged time series in each ROI were extracted, and the Pearson correlations with time series in other ROIs were calculated. Then, the FCs were transformed to Z values (Lowe et al., 1998). Thus, a 14 × 14 FC pattern matrix for each individual was separately obtained for further analysis.
Statistical Analysis
Demographic and Clinical Features
Two independent t-tests were performed to compare the demographic and clinical traits between the two groups, and paired t-tests were employed to determine whether the alterations in VAS scores were significant after treatment in each group (SPSS 20.0; SPSS Inc., Chicago, IL, United States). Pearson correlation analyses were employed to explore associations between duration of disease, baseline VAS, SDS, and SAS scores, and changes of the VAS scores. The significance level was set at P < 0.05.
Functional Connectivity Difference Analysis
The paired t-tests were performed to detect the FC matrix alterations after treatment in each treatment group. In addition, we employed a 2 × 2 (group × time) multivariate analysis of covariance (MANCOVA) on the FC matrix to detect whether there were different neural mechanisms underlying the different treatments, with age and duration of disease as covariates (Hand and Taylor, 1987; Davis, 2002). Traditional Pearson correlation analyses were used to explore the associations between the FCs of MANCOVA and VAS changes after three sessions of acupuncture. The significance level was set at P < 0.05 and corrected for multiple comparisons by the false discovery rate (FDR) approach. The in-house script was write in MATLAB to conduct the MANCOVA in the study. The codes for MANCOVA of ROI-wise functional connectivity can be downloaded from https://github.com/cdutcmysy/ROIwise_FC_mancova.
MVPA-Based Clinical Symptom Prediction Analysis
MANCOVA found differences in FC between the real and sham treatments for PDM. We proposed that these different FC patterns could reveal the neural mechanisms underlying the effects of acupuncture treatment for PDM and that these FC patterns at baseline would be used for predicting the treatment response to acupuncture in patients with PDM. Here, MVPA based on linear support vector regression (SVR, implemented by LIBSVM3) (Chang and Lin, 2011) was employed to verify our hypothesis. We set the change in pain severity (VAS change and VAS change rate) as the dependent variable and FCs from the MANCOVA results at baseline as independent variables (predictors) in all participants and regressed out the effects of age, treatment method and duration of disease. A leave-one-out cross-validation (LOOCV) method was used for prediction to ensure separation between training and testing samples (Plitt et al., 2015). We calculated the squared prediction-outcome correlation (R2) as well as the mean absolute error (MAE) to evaluate the SVR predictive ability (Wager et al., 2013; Lindquist et al., 2017). Furthermore, we employed the permutation test to verify that the predictor was not from a random chance (repeated 5000 times).
Results
Demographic and Clinical Features
There were no significant differences in age, BMI, duration of disease, and baseline SAS, SDS or VAS scores between the real and sham acupuncture treatment groups (all P > 0.05; see Table 1). The VAS at the post-treatment period was lower in the real group than in the sham group, and the VAS change score and VAS change rate were significantly higher in the real acupuncture treatment group than in the sham acupuncture treatment group. Paired t-tests showed that the VAS change in the real acupuncture treatment group was significant (t = 9.90, P < 0.01), while the VAS change in the sham acupuncture group was not significant (t = 2.10, P = 0.06). In addition, there were no significant associations between duration of disease and baseline VAS, SDS, and SAS scores and post-treatment VAS score changes in either group (all P > 0.05; see Table 2).
FC Matrix Alterations After Real and Sham Acupuncture Treatment
As illustrated in Figures 3A–C, after real acupuncture treatment, both increased and decreased FCs were found; specifically, FCs between the mPFC-right S1, PAG-bilateral thalami, PAG-RVM, RVM-bilateral thalami and RVM-DMN (all four ROIs in the DMN) were increased, while FC between the left S1-right aINS was decreased after real acupuncture treatment. For the sham acupuncture treatment group, increased FCs were found in the right S1-right aINS, left IPC-bilateral aINS, right dlPFC-left S1, right dlPFC-PCC and right dlPFC-right IPC, while FC between the PAG-RVM was decreased after sham treatment (Figures 3D–F). Interestingly, we did not find the same patterns of altered FC in the real and sham acupuncture treatment groups.
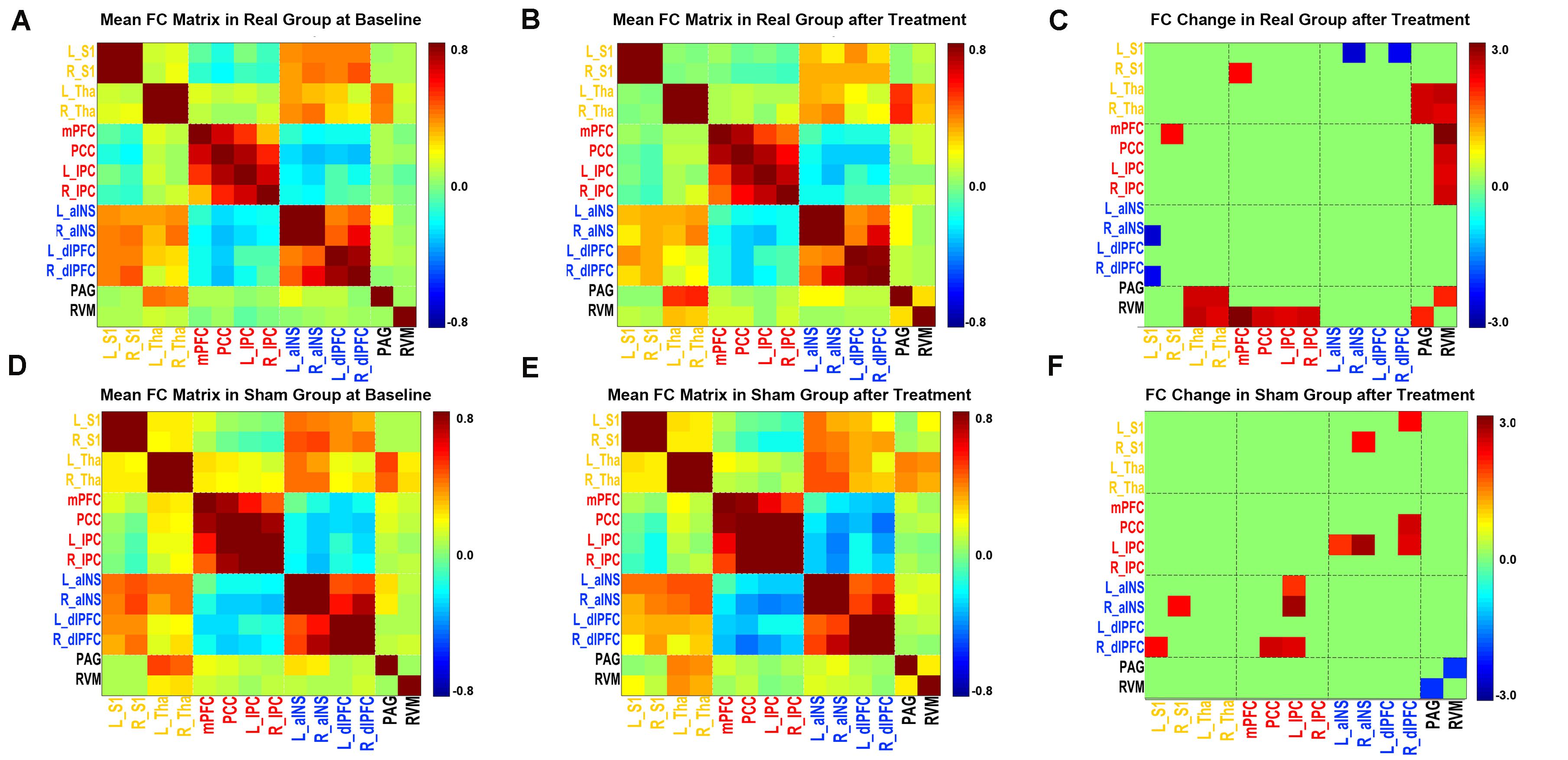
Figure 3. FC matrix pattern in each group. (A,D) The FC matrix pattern at baseline. (B,E) The FC matrix pattern after treatment. The color bar indicates the correlation coefficient between two regions. (C,F) FC alterations after acupuncture treatment; the color bar indicates the T value. (A,B,C) Real acupuncture group. (D,E,F) Sham acupuncture group. Abbreviations: FC, functional connectivity; L, left side; R, right side; S1, primary somatosensory cortex; Tha, thalamus; mPFC, medial prefrontal cortex; PCC, posterior cingulate cortex; IPC, inferior parietal cortex; aINS, anterior insula; dlPFC, dorsolateral prefrontal cortex; RVM, rostroventral medulla; PAG, periaqueductal gray.
Group Differences in the FC Matrix
The MANCOVA analysis results are displayed in Figure 4 and Supplementary Figure S2. Eight paired FCs showed significant differences between the two groups, including the right aINS-bilateral S1, right dlPFC-bilateral S1, right dlPFC-left IPC, RVM-bilateral thalami and RVM-PAG. Specifically, FCs that were decreased in the real group but increased in the sham group were located in the SMN-SN and DMN-SN, while FCs that were increased in the real group but decreased in the sham group were found in the DPMS-SMN and within the DPMS pathway.
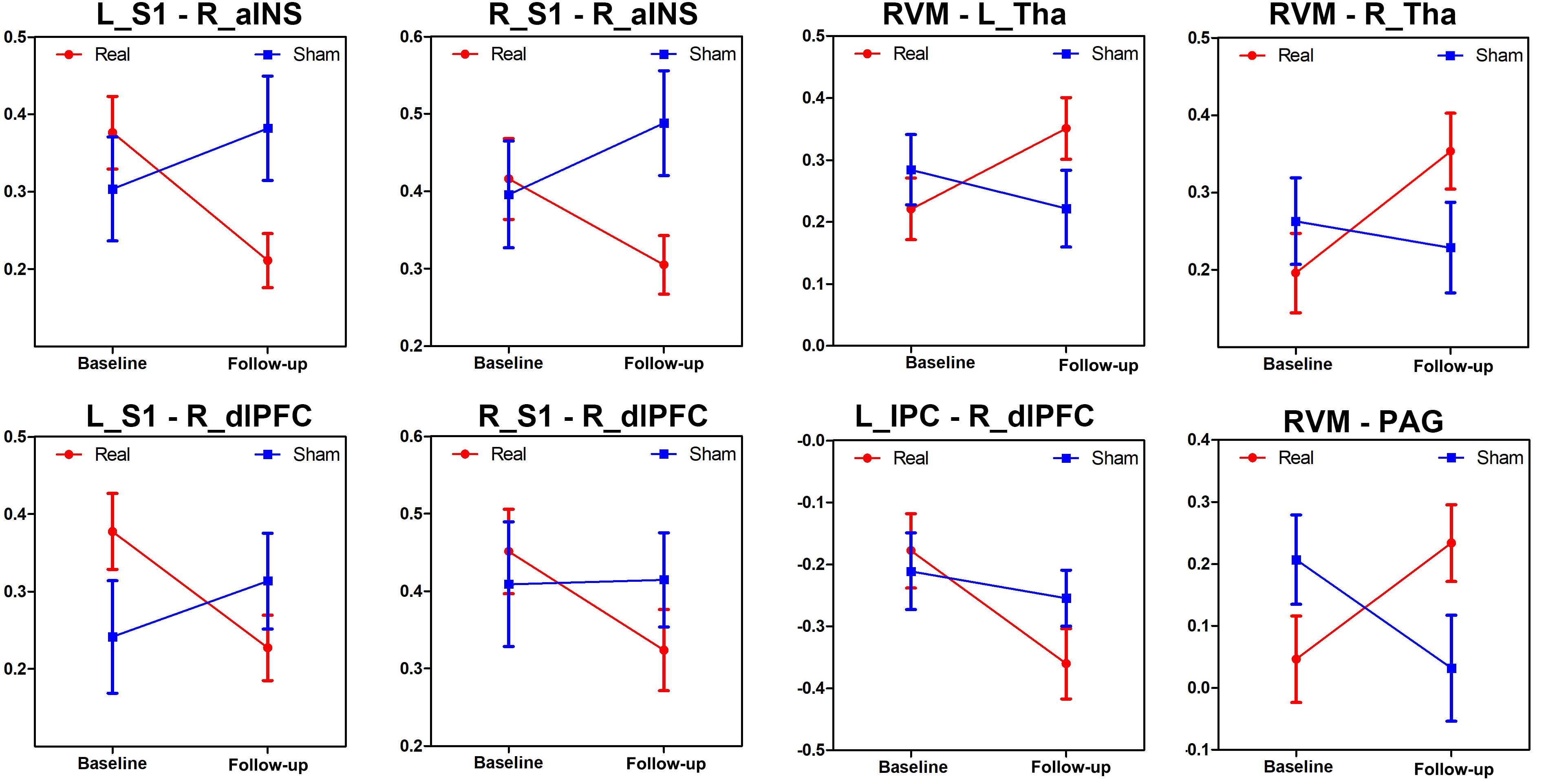
Figure 4. Changes in the different brain connections following real and sham acupuncture for PDM. The line charts display the different alterations between real and sham acupuncture for PDM in these FCs after treatment. Abbreviations: FC, functional connectivity; L, left side; R, right side; S1, primary somatosensory cortex; aINS, anterior insula; dlPFC, dorsolateral prefrontal cortex; RVM, rostroventral medulla; Tha, thalamus; PAG, periaqueductal gray; IPC, inferior parietal cortex.
Clinical Prediction Results
As we found significant group differences in FC alterations between the real and sham groups, we used the baseline FCs as predictors of the treatment response and controlled for the effect of age, duration and treatment method. The SVR analyses revealed that the eight baseline FC patterns predicted the VAS change scores (R2 = 0.27, P = 0.002, MAE = 0.36; Figure 5A) and VAS change rate after treatment (R2 = 0.30, P = 0.0009, MAE = 2.26; Figure 5B). The permutation tests confirmed that the results could not be obtained by chance (P < 0.001). Conversely, the traditional bivariate correlation analyses did not find any significant associations between these differences in FC and VAS changes after acupuncture treatment in the patients with PDM (all P > 0.05; see Table 2).
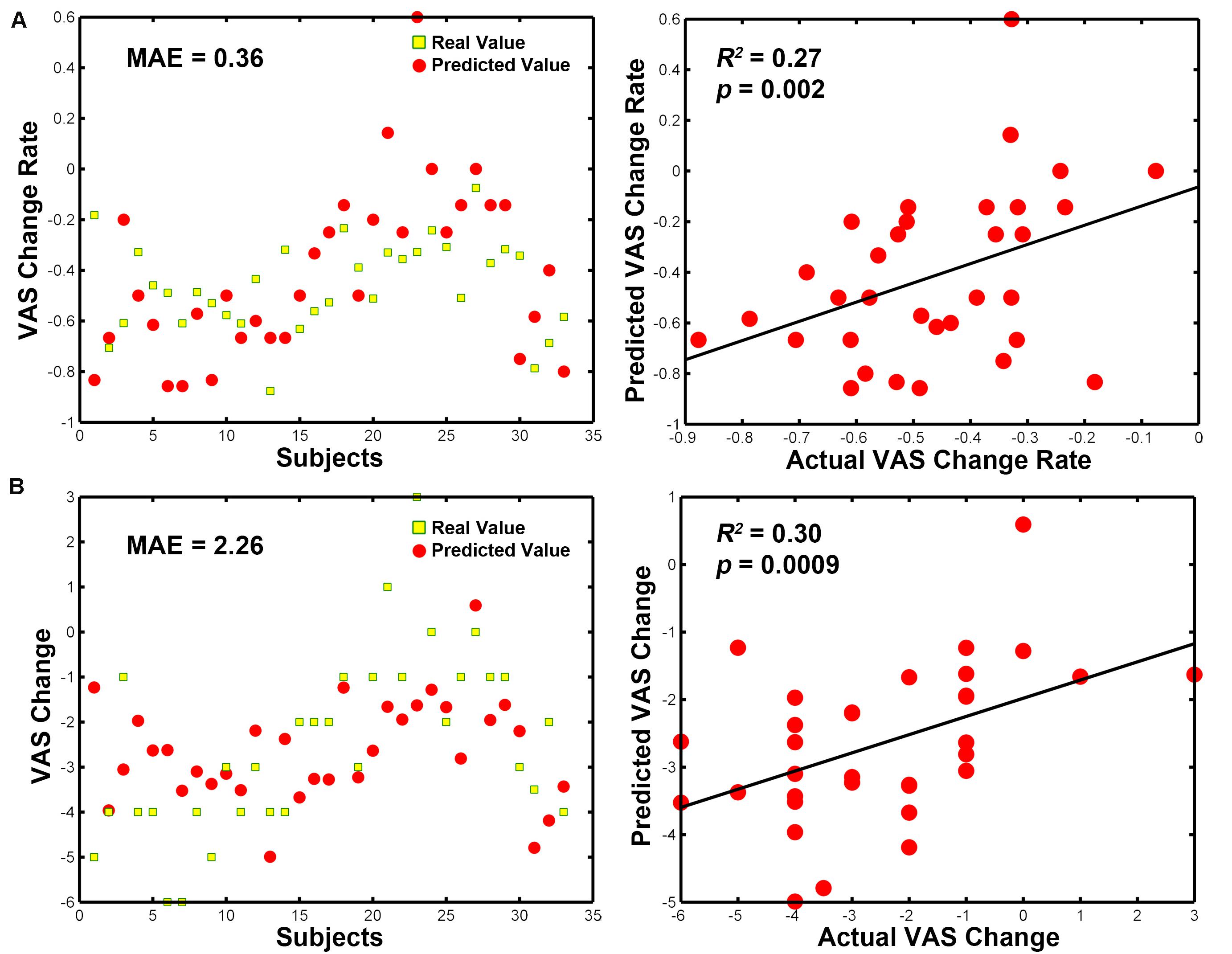
Figure 5. MVPA-based clinical prediction results. (A) Baseline FC patterns predict VAS change scores after treatment. (B) Baseline FC patterns predict VAS change rates after treatment. Abbreviations: MVPA, multivariate pattern analysis; MAE, mean absolute error; VAS, visual analog scale.
Discussion
This study in patients with PDM used MVPA recognition to identify neurobiological predictors of acupuncture treatment response. We first verified the efficacy of real but not sham acupuncture treatment in the patients with PDM. Then, we identified FCs involving the SMN-SN, DMN-SN, DPMS-SMN and DPMS pathway that showed different alterations after real and sham acupuncture treatment in PDM patients. Furthermore, MVPA revealed that these pretreatment multivariate FC patterns in the SMN, SN, DMN and DPMS significantly predicted individual patient pain symptoms after 3 menstrual cycles of intensive acupuncture treatment. Conversely, pretreatment clinical variables and traditional correlation analyses were not able to predict posttreatment pain severity in these patients with PDM. These findings have implications for identifying who will benefit most from acupuncture, as well as for understanding the pathophysiology of PDM as it relates to acupuncture effects.
The present study verified the effect of acupuncture on reducing menstrual pain intensity in PDM (Yu et al., 2017). In the present study, for the real acupuncture treatment, we selected sanyinjiao (SP6) as the acupuncture target based on data mining from our previous review (Yu et al., 2015) and expert opinions, while for the sham acupuncture treatment, an adjacent non-acupoint was selected. We found that real acupuncture was superior to sham acupuncture for pain relief. The results indicated that correct acupuncture point locations are important contributors to the treatment effects and that the acupuncture effect of pain relief cannot be explained solely in terms of placebo effects (Smith et al., 2011; Vickers et al., 2018). Subsequently, we detected the potential brain mechanisms underlying the real and sham acupuncture treatment effects in PDM.
To date, no study has explored the underlying mechanisms of pain reduction by acupuncture in patients with PDM. For other types of chronic pain, Lee et al. found that both real and sham (phantom) acupuncture could reduce pain ratings in patients with low back pain (LBP), but the reduced pain intensity was associated with reduced FC between the mPFC and posterior insula in the real acupuncture group, while the decreased pain intensity was associated with enhanced FC between the PCC and aINS (Lee et al., 2019). The results indicated differential brain influences on real and sham acupuncture in LBP. Here, we constructed the pain-related functional connectivity matrices in patients with PDM by combining the data-driven approach (GICA) and hypothesis-based ROI selection method with rs-fMRI data. The findings showed that altered pain-related FCs after real acupuncture were located in FC between the DPMS-SMN and DPMS-DMN and within the DPMS, while altered FCs after sham acupuncture were found in FC between the SN-SMN and SN-DMN and within the DPMS. In general, real acupuncture modulated the DPMS-associated brain network more, while sham acupuncture modulated the SN-related brain network more. These results indicated that real acupuncture could modulate widespread brain networks associated with the DPMS, while sham acupuncture had a more focused modulation in the salience network associated with analgesia in patients with PDM. Furthermore, MANCOVA was used to detect the different brain functional modulatory mechanisms between real and sham acupuncture treatment in patients with PDM.
We found eight functional couplings that showed differences in alterations after real and sham treatment of PDM. As illustrated in Figure 3, real acupuncture increased but sham acupuncture decreased FC between the DPMS and SMN and FC within the DPMS (RVM-PAG), while real acupuncture reduced but sham acupuncture enhanced FC between the SN and SMN, and the SN and DMN. Our study was consistent with previous findings of different modulatory effects in the central nervous system between real and sham acupuncture in a healthy population. Cao et al. found that real acupuncture could increase pain intensity thresholds, which were associated with increased neural activity in the insula (Cao et al., 2018). A recent neuroimaging meta-analysis about acupuncture effects in the brain in healthy individuals found that in 29 out of 33 studies, real acupuncture, compared to sham acupuncture, resulted in more/different modulatory effects on neurological components measured by neuroimaging, including somatosensory, affective, and cognitive aspects (Scheffold et al., 2015). The dlPFC is known to be associated with center control and top-down processes for pain control (Wager et al., 2004; Kong et al., 2006), as well as anticipation of pain relief and expectation-related placebo analgesia (Krummenacher et al., 2010). The SMN represents the major ascending pathways of pain (Tracey and Mantyh, 2007; Davis et al., 2017), while the DMN is involved in the self-regulation of pain, such as mind wandering (Kucyi et al., 2013; Kucyi and Davis, 2015). Multiple neuroimaging studies have suggested that acupuncture may achieve analgesic effects by modulating the SN, DMN and SMN (Chen et al., 2015; Shi et al., 2015; Lee et al., 2019; Zhang et al., 2019). The DPMS is considered the key system for pain modulation (Millan, 2002) and has also been found to be modulated by acupuncture treatment in migraine (Li et al., 2016). The different modulatory effects of real and sham acupuncture in the pain-associated brain networks indicated different and complex neural mechanisms of real and sham acupuncture in patients with PDM. The different alterations in these pain-related FCs resulted in different treatment responses, which also indicated the potential use of these FCs as predictors for acupuncture treatment response in PDM patients.
Our research is in line with the growing interest in predictive modeling using neuroimaging and machine learning methods (Janssen et al., 2018; Keshavan et al., 2020). Most machine learning studies have focused on differential diagnosis, identifying brain signatures that discriminate patients from healthy controls, and further established objective signs of disease pathology (Lai, 2019). Another important application of machine learning has been the use of brain characteristics to predict therapeutic outcomes and offer personalized tailoring of interventions (Gao et al., 2018; Leaver et al., 2018; Mithani et al., 2019). Here, using MVPA (Yang et al., 2012), a widely applied machine learning approach, we found that several pain-related FC patterns at baseline could be a useful predictor for acupuncture treatment response (both VAS changes and the change rate) in PDM patients. Recently, Tu et al. also used MVPA-based rs-FCs to predict real and sham acupuncture treatment responses in chronic LBP, and the rs-FC characteristics were significantly predictive of the differential responses to real and sham treatment in LBP (Tu et al., 2019a). In our study, the predictive power and strength of the MVPA approach was validated in several ways. First, we found that baseline clinical or demographic features were unable to predict the outcome responses of the PDM patients. Second, the traditional univariate correlations between FCs and VAS changes were not significant. Given the nature of MVPA approaches, these techniques provide improved sensitivity to subtle and spatially distributed brain differences that would likely remain undetected with the use of conventional univariate approaches (Fan et al., 2006; Haxby, 2012). Therefore, we demonstrated the feasibility and reliability of the MVPA model for predicting clinical symptom changes after acupuncture treatment in patients with PDM.
Limitations
The present study also has several limitations. First, the sample size in this study was small, especially in the sham acupuncture group, and previous studies have also found a significant analgesic effect of sham acupuncture (Tu et al., 2019a). Our study found that the VAS change in the sham acupuncture group was nearly significant (P = 0.06), and further studies with larger sample sizes are needed to address this point. Second, as the PDM is a cyclic chronic pain, the present study only explored the “trait pain” (average pain experienced over time) in the patients with PDM, and future studies should also explore the mechanism of the acupuncture-related analgesic effect on “state pain” (pain at the menstrual period) in PDM patients (Low et al., 2018). Third, to increase the statistical power, we combined the two treatment groups as a pooled group in the MVPA analysis after controlling for the effect of treatment. Nevertheless, we did not find that baseline FCs predicted the treatment response in the single groups. Further studies with larger sample sizes are needed to further detect the different predictors for real and sham treatment effects in PDM.
Conclusion
The present study verified the different brain mechanisms underlying real and sham acupuncture in PDM patients. In addition, the pain-related FC patterns at baseline could predict the acupuncture treatment response for patients with PDM. The findings supported the use of neuroimaging biomarkers for individual-based precise acupuncture treatment in patients with PDM.
Data Availability Statement
The raw data supporting the conclusions of this article will be made available by the authors, without undue reservation.
Ethics Statement
The studies involving human participants were reviewed and approved by the Research Ethics Committee of Chengdu University of Traditional Chinses Medicine. The patients/participants provided their written informed consent to participate in this study.
Author Contributions
JY and FL designed the study. SL, WW, XG, JT, QZ, and MX collected the data. SY and MX analyzed the data. SY contributed to the original draft. JY, FZ, and FL reviewed and edited the manuscript. All authors contributed to the article and approved the submitted version.
Funding
This work was supported by the programs of the National Natural Science Foundation of China (Nos. 81574089, 81973960, 81973966, and 81590951), the Fok Ying-Tong Education Foundation (No. 151043), the Initiative Postdocs Supporting Program (No. BX20190046), and the China Postdoctoral Science Foundation Grant (No. 2019M663454).
Conflict of Interest
The authors declare that the research was conducted in the absence of any commercial or financial relationships that could be construed as a potential conflict of interest.
Acknowledgments
We would like to acknowledge the generous support and contribution of all our trial participants.
Supplementary Material
The Supplementary Material for this article can be found online at: https://www.frontiersin.org/articles/10.3389/fnins.2020.559191/full#supplementary-material
Footnotes
- ^ http://www.fil.ion.ucl.ac.uk/spm
- ^ http://www.restfmri.net
- ^ https://www.csie.ntu.edu.tw/∼cjlin/libsvm/
References
Angst, M. S., Phillips, N. G., Drover, D. R., Tingle, M., Ray, A., Swan, G. E., et al. (2012). Pain sensitivity and opioid analgesia: a pharmacogenomic twin study. Pain 153, 1397–1409. doi: 10.1016/j.pain.2012.02.022
Armour, M., Dahlen, H. G., Zhu, X., Farquhar, C., and Smith, C. A. (2017). The role of treatment timing and mode of stimulation in the treatment of primary dysmenorrhea with acupuncture: an exploratory randomised controlled trial. PLoS One 12:e0180177. doi: 10.1371/journal.pone.0180177
Azevedo, D. C., Ferreira, P. H., Santos, H. O., Oliveira, D. R., Souza, J. V. L., and Costa, L. O. P. (2019). Baseline characteristics did not identify people with low back pain who respond best to a movement system impairment-based classification treatment. Braz. J. Phys. Ther. 24, 358–364.
Calhoun, V. D., Adali, T., Pearlson, G. D., and Pekar, J. J. (2001). A method for making group inferences from functional MRI data using independent component analysis. Hum. Brain Mapp. 14, 140–151. doi: 10.1002/hbm.1048
Campbell, M. A., and McGrath, P. J. (1999). Non-pharmacologic strategies used by adolescents for the management of menstrual discomfort. Clin. J. Pain 15, 313–320. doi: 10.1097/00002508-199912000-00008
Cao, J., Tu, Y., Orr, S. P., Lang, C., Park, J., Vangel, M., et al. (2018). Analgesic effects evoked by real and imagined acupuncture: a neuroimaging study. Cereb. Cortex 29, 3220–3231. doi: 10.1093/cercor/bhy190
Chang, C. C., and Lin, C. J. (2011). LIBSVM: a library for support vector machines. ACM Trans. Intell. Syst. Tech. 2, 1–27. doi: 10.1145/1961189.1961199
Chen, J., Wang, Z., Tu, Y., Liu, X., Jorgenson, K., Ye, G., et al. (2018). Regional homogeneity and multivariate pattern analysis of cervical spondylosis neck pain and the modulation effect of treatment. Front. Neurosci. 12:900. doi: 10.3389/fnins.2018.00900
Chen, T., Mu, J., Xue, Q., Yang, L., Dun, W., Zhang, M., et al. (2019). Whole-brain structural magnetic resonance imaging–based classification of primary dysmenorrhea in pain-free phase: a machine learning study. Pain 160, 734–741. doi: 10.1097/j.pain.0000000000001428
Chen, X., Spaeth, R. B., Freeman, S. G., Scarborough, D. M., Hashmi, J. A., Wey, H.-Y., et al. (2015). The modulation effect of longitudinal acupuncture on resting state functional connectivity in knee osteoarthritis patients. Mol. Pain 11:67.
Coghill, R. C., and Eisenach, J. (2003). Individual differences in pain sensitivity: implications for treatment decisions. Anesthesiology 98, 1312–1314. doi: 10.1097/00000542-200306000-00003
Davis, C. S. (2002). Normal-theory methods: multivariate analysis of variance. Statist. Methods Anal. Repeat. Meas. 133, 73–102. doi: 10.1007/978-0-387-21573-0_4
Davis, K. D., Flor, H., Greely, H. T., Iannetti, G. D., Mackey, S., Ploner, M., et al. (2017). Brain imaging tests for chronic pain: medical, legal and ethical issues and recommendations. Nat. Rev. Neurol. 13:624. doi: 10.1038/nrneurol.2017.122
Fan, Y., Shen, D., Gur, R. C., Gur, R. E., and Davatzikos, C. (2006). COMPARE: classification of morphological patterns using adaptive regional elements. IEEE Trans. Med. Imaging 26, 93–105. doi: 10.1109/tmi.2006.886812
Fillingim, R. B. (2017). Individual differences in pain: understanding the mosaic that makes pain personal. Pain 158:S11.
Gao, S., Calhoun, V. D., and Sui, J. (2018). Machine learning in major depression: from classification to treatment outcome prediction. CNS Neurosci. Ther. 24, 1037–1052. doi: 10.1111/cns.13048
Goksan, S., Baxter, L., Moultrie, F., Duff, E., Hathway, G., Hartley, C., et al. (2018). The influence of the descending pain modulatory system on infant pain-related brain activity. eLife 7:e37125.
Grosen, K., Fischer, I. W. D., Olesen, A. E., and Drewes, A. (2013). Can quantitative sensory testing predict responses to analgesic treatment? Eur. J. Pain 17, 1267–1280. doi: 10.1002/j.1532-2149.2013.00330.x
Hand, D. J., and Taylor, C. C. (1987). Multivariate Analysis of Variance and Repeated Measures: A Practical Approach for Behavioral Scientists. London: CRC press.
Haxby, J. V. (2012). Multivariate pattern analysis of fMRI: the early beginnings. Neuroimage 62, 852–855. doi: 10.1016/j.neuroimage.2012.03.016
Hemington, K. S., Wu, Q., Kucyi, A., Inman, R. D., and Davis, K. D. (2016). Abnormal cross-network functional connectivity in chronic pain and its association with clinical symptoms. Brain Struct. Funct. 221, 4203–4219. doi: 10.1007/s00429-015-1161-1
Iacovides, S., Avidon, I., and Baker, F. C. (2015). What we know about primary dysmenorrhea today: a critical review. Hum. Reprod. Update 21, 762–778. doi: 10.1093/humupd/dmv039
Janssen, R. J., Mourão-Miranda, J., and Schnack, H. G. (2018). Making individual prognoses in psychiatry using neuroimaging and machine learning. Biol. Psychiatry 3, 798–808. doi: 10.1016/j.bpsc.2018.04.004
Keshavan, M. S., Collin, G., Guimond, S., Kelly, S., Prasad, K. M., and Lizano, P. (2020). Neuroimaging in Schizophrenia. Neuroimaging Clin. N. Am. 30, 73–83.
Kong, J., Gollub, R. L., Rosman, I. S., Webb, J. M., Vangel, M. G., Kirsch, I., et al. (2006). Brain activity associated with expectancy-enhanced placebo analgesia as measured by functional magnetic resonance imaging. J. Neurosci. 26, 381–388. doi: 10.1523/jneurosci.3556-05.2006
Krummenacher, P., Candia, V., Folkers, G., Schedlowski, M., and Schönbächler, G. (2010). Prefrontal cortex modulates placebo analgesia. Pain 148, 368–374. doi: 10.1016/j.pain.2009.09.033
Kucyi, A., and Davis, K. D. (2015). The dynamic pain connectome. Trends Neurosci. 38, 86–95. doi: 10.1016/j.tins.2014.11.006
Kucyi, A., and Davis, K. D. (2017). The neural code for pain: from single-cell electrophysiology to the dynamic pain connectome. Neuroscientist 23, 397–414. doi: 10.1177/1073858416667716
Kucyi, A., Salomons, T. V., and Davis, K. D. (2013). Mind wandering away from pain dynamically engages antinociceptive and default mode brain networks. Proc. Natl. Acad. Sci. U.S.A. 110, 18692–18697. doi: 10.1073/pnas.1312902110
Kumbhare, D. A., Elzibak, A. H., and Noseworthy, M. D. (2017). Evaluation of chronic pain using magnetic resonance (MR) neuroimaging approaches. Clin. J. Pain 33, 281–290. doi: 10.1097/ajp.0000000000000415
Lai, C. H. (2019). The neural markers of MRI to differentiate depression and panic disorder. Prog. Neuropsychopharmacol. Biol. Psychiatry 91, 72–78. doi: 10.1016/j.pnpbp.2018.04.013
Larroy, C. (2002). Comparing visual-analog and numeric scales for assessing menstrual pain. Behav. Med. 27, 179–181. doi: 10.1080/08964280209596043
Leaver, A. M., Wade, B., Vasavada, M., Hellemann, G., Joshi, S. H., Espinoza, R., et al. (2018). Fronto-temporal connectivity predicts ECT outcome in major depression. Front. Psychiatry 9:92. doi: 10.3389/fpsyt.2018.00092
Lee, J., Eun, S., Kim, J., Lee, J.-H., and Park, K. (2019). Differential influence of acupuncture somatosensory and cognitive/affective components on functional brain connectivity and pain reduction during low back pain state. Front. Neurosci. 13:1062. doi: 10.3389/fnins.2019.01062
Li, Y. O., Adali, T., and Calhoun, V. D. (2006). “Sample dependence correction for order selection in fMRI analysis,” in Proceedings of the IEEE International Symposium on Biomedical Imaging: Nano To Macro, (Arlington, VA: IEEE), 1072–1075.
Li, Z., Liu, M., Lan, L., Zeng, F., Makris, N., Liang, Y., et al. (2016). Altered periaqueductal gray resting state functional connectivity in migraine and the modulation effect of treatment. Sci. Rep. 6:20298.
Lindquist, M. A., Krishnan, A., Lopez-Sola, M., Jepma, M., Woo, C. W., Koban, L., et al. (2017). Group-regularized individual prediction: theory and application to pain. Neuroimage 145, 274–287. doi: 10.1016/j.neuroimage.2015.10.074
Liu, J., Mu, J., Chen, T., Zhang, M., and Tian, J. (2019). White matter tract microstructure of the mPFC-amygdala predicts interindividual differences in placebo response related to treatment in migraine patients. Hum. Brain Mapp. 40, 284–292. doi: 10.1002/hbm.24372
Liu, J., Mu, J., Liu, Q., Dun, W., Zhang, M., and Tian, J. (2017). Brain structural properties predict psychologically mediated hypoalgesia in an 8-week sham acupuncture treatment for migraine. Hum. Brain Mapp. 38, 4386–4397. doi: 10.1002/hbm.23667
Liu, P., Liu, Y., Wang, G., Li, R., Wei, Y., Fan, Y., et al. (2018). Changes of functional connectivity of the anterior cingulate cortex in women with primary dysmenorrhea. Brain Imaging Behav. 12, 710–717. doi: 10.1007/s11682-017-9730-y
Low, I., Kuo, P.-C., Liu, Y.-H., Tsai, C.-L., Chao, H.-T., Hsieh, J.-C., et al. (2017). Altered brain complexity in women with primary dysmenorrhea: a resting-state magneto-encephalography study using multiscale entropy analysis. Entropy 19:680. doi: 10.3390/e19120680
Low, I., Wei, S.-Y., Lee, P.-S., Li, W.-C., Lee, L.-C., Hsieh, J.-C., et al. (2018). Neuroimaging studies of primary Dysmenorrhea. Adv. Exp. Med.Biol. 1099, 179–199.
Lowe, M. J., Mock, B. J., and Sorenson, J. A. (1998). Functional connectivity in single and multislice echoplanar imaging using resting-state fluctuations. Neuroimage 7, 119–132. doi: 10.1006/nimg.1997.0315
Ma, Y.-X., Ma, L.-X., Liu, X.-L., Ma, Y.-X., Lv, K., Wang, D., et al. (2010). A comparative study on the immediate effects of electroacupuncture at Sanyinjiao (SP6), Xuanzhong (GB39) and a non-meridian point, on menstrual pain and uterine arterial blood flow, in primary dysmenorrhea patients. Pain Med. 11, 1564–1575. doi: 10.1111/j.1526-4637.2010.00949.x
Ma, Y. X., Ye, X. N., Liu, C. Z., Cai, P. Y., Li, Z. F., Du, D. Q., et al. (2013). A clinical trial of acupuncture about time-varying treatment and points selection in primary dysmenorrhea. J. Ethnopharmacol. 148, 498–504. doi: 10.1016/j.jep.2013.04.045
Maeda, Y., Kim, H., Kettner, N., Kim, J., Cina, S., Malatesta, C., et al. (2017). Rewiring the primary somatosensory cortex in carpal tunnel syndrome with acupuncture. Brain 140, 914–927. doi: 10.1093/brain/awx015
Mithani, K., Mikhail, M., Morgan, B. R., Wong, S., Weil, A. G., Deschenes, S., et al. (2019). Connectomic profiling identifies responders to vagus nerve stimulation. Ann. Neurol. 86, 743–753. doi: 10.1002/ana.25574
O’Connell, K., Davis, A. R., and Westhoff, C. (2006). Self-treatment patterns among adolescent girls with dysmenorrhea. J. Pediatr Adolescent Gynecol. 19, 285–289. doi: 10.1016/j.jpag.2006.05.004
Oldfield, R. C. (1971). The assessment and analysis of handedness: the Edinburgh inventory. Neuropsychologia 9, 97–113. doi: 10.1016/0028-3932(71)90067-4
Plitt, M., Barnes, K. A., Wallace, G. L., Kenworthy, L., and Martin, A. (2015). Resting-state functional connectivity predicts longitudinal change in autistic traits and adaptive functioning in autism. Proc. Natl. Acad. Sci. U.S.A. 112, E6699–E6706.
Reggente, N., Moody, T. D., Morfini, F., Sheen, C., Rissman, J., O’neill, J., et al. (2018). Multivariate resting-state functional connectivity predicts response to cognitive behavioral therapy in obsessive-compulsive disorder. Proc. Natl. Acad. Sci. U.S.A. 115, 2222–2227. doi: 10.1073/pnas.1716686115
Scheffold, B. E., Hsieh, C.-L., and Litscher, G. (2015). Neuroimaging and neuromonitoring effects of electro and manual acupuncture on the central nervous system: a literature review and analysis. Evid. Based Comp. Alternat. Med. 2015:641742.
Shen, Z., Yu, S., Wang, M., She, T., Yang, Y., Wang, Y., et al. (2019). Abnormal amygdala resting-state functional connectivity in primary dysmenorrhea. NeuroReport 30, 363–368. doi: 10.1097/wnr.0000000000001208
Shi, Y., Liu, Z., Zhang, S., Li, Q., Guo, S., Yang, J., et al. (2015). Brain network response to acupuncture stimuli in experimental acute low back pain: an fMRI study. Evid. Based Comp.Alternat. Med. 2015:210120.
Smith, C. A., Zhu, X., He, L., and Song, J. (2011). Acupuncture for primary dysmenorrhoea. Cochrane Database Syst. Rev. 9:CD007854.
Smith, S. M., Fox, P. T., Miller, K. L., Glahn, D. C., Fox, P. M., Mackay, C. E., et al. (2009). Correspondence of the brain’s functional architecture during activation and rest. Proc. Natl. Acad. Sci. U.S.A. 106, 13040–13045.
Tracey, I., and Mantyh, P. W. (2007). The cerebral signature for pain perception and its modulation. Neuron 55, 377–391. doi: 10.1016/j.neuron.2007.07.012
Tu, C.-H., Niddam, D. M., Chao, H.-T., Liu, R.-S., Hwang, R.-J., Yeh, T.-C., et al. (2009). Abnormal cerebral metabolism during menstrual pain in primary dysmenorrhea. Neuroimage 47, 28–35. doi: 10.1016/j.neuroimage.2009.03.080
Tu, Y., Jung, M., Gollub, R. L., Napadow, V., Gerber, J., Ortiz, A., et al. (2019a). Abnormal medial prefrontal cortex functional connectivity and its association with clinical symptoms in chronic low back pain. Pain 160, 1308–1318. doi: 10.1097/j.pain.0000000000001507
Tu, Y., Ortiz, A., Gollub, R. L., Cao, J., Gerber, J., Lang, C., et al. (2019b). Multivariate resting-state functional connectivity predicts responses to real and sham acupuncture treatment in chronic low back pain. Neuroimage Clin. 23:101885. doi: 10.1016/j.nicl.2019.101885
Underwood, M., Morton, V., Farrin, A., and Team, U. B. T. (2007). Do baseline characteristics predict response to treatment for low back pain? Secondary analysis of the UK BEAM dataset [ISRCTN32683578]. Rheumatology 46, 1297–1302. doi: 10.1093/rheumatology/kem113
Vickers, A. J., Vertosick, E. A., Lewith, G., Macpherson, H., Foster, N. E., Sherman, K. J., et al. (2018). Acupuncture for chronic pain: update of an individual patient data meta-analysis. J. Pain 19, 455–474. doi: 10.1016/j.jpain.2017.11.005
Wager, T. D., Atlas, L. Y., Leotti, L. A., and Rilling, J. K. (2011). Predicting individual differences in placebo analgesia: contributions of brain activity during anticipation and pain experience. J. Neurosci. 31, 439–452. doi: 10.1523/jneurosci.3420-10.2011
Wager, T. D., Atlas, L. Y., Lindquist, M. A., Roy, M., Woo, C. W., and Kross, E. (2013). An fMRI-based neurologic signature of physical pain. N. Engl. J. Med. 368, 1388–1397. doi: 10.1056/nejmoa1204471
Wager, T. D., Rilling, J. K., Smith, E. E., Sokolik, A., Casey, K. L., Davidson, R. J., et al. (2004). Placebo-induced changes in FMRI in the anticipation and experience of pain. Science 303, 1162–1167. doi: 10.1126/science.1093065
Wandner, L. D., Scipio, C. D., Hirsh, A. T., Torres, C. A., and Robinson, M. E. (2012). The perception of pain in others: how gender, race, and age influence pain expectations. J. Pain 13, 220–227. doi: 10.1016/j.jpain.2011.10.014
Witt, C. M., Reinhold, T., Brinkhaus, B., Roll, S., Jena, S., and Willich, S. N. (2008). Acupuncture in patients with dysmenorrhea: a randomized study on clinical effectiveness and cost-effectiveness in usual care. Am. J. Obstet. Gynecol. 198, 166.e1–166.e8.
Witt, C. M., Vertosick, E. A., Foster, N. E., Lewith, G., Linde, K., Macpherson, H., et al. (2019). The effect of patient characteristics on acupuncture treatment outcomes: an individual patient data meta-analysis of 20,827 chronic pain patients in randomized controlled trials. Clin. J. Pain 35, 428–434. doi: 10.1097/ajp.0000000000000691
World Health Organization (2008). WHO Standard Acupuncture Point Locations in the Western Pacific Region. Manila: World Health Organization.
Wu, T.-H., Tu, C.-H., Chao, H.-T., Li, W.-C., Low, I., Chuang, C.-Y., et al. (2016). Dynamic changes of functional pain connectome in women with primary dysmenorrhea. Sci. Rep. 6:24543.
Yang, Z., Fang, F., and Weng, X. (2012). Recent developments in multivariate pattern analysis for functional MRI. Neurosci. Bull. 28, 399–408. doi: 10.1007/s12264-012-1253-3
Yu, S., Yang, J., Yang, M., Gao, Y., Chen, J., Ren, Y., et al. (2015). Application of acupoints and meridians for the treatment of primary dysmenorrhea: a data mining-based literature study. Evid. Based Comp. Alter. Med. 2015:752194.
Yu, S.-Y., Lv, Z.-T., Zhang, Q., Yang, S., Wu, X., Hu, Y.-P., et al. (2017). Electroacupuncture is beneficial for primary dysmenorrhea: the evidence from meta-analysis of randomized controlled trials. Evid. Based Comp. Alter. Med. 2017:1791258.
Yunus, M. B. (2012). The prevalence of fibromyalgia in other chronic pain conditions. Pain Res. Treat. 2012:584573.
Zhang, Q., Yu, S., Wang, Y., Wang, M., Yang, Y., Wei, W., et al. (2019). Abnormal reward system network in primary dysmenorrhea. Mol. Pain 15:1744806919862096.
Zhang, Y., Zhang, H., Nierhaus, T., Pach, D., Witt, C. M., and Yi, M. (2019). Default mode network as a neural substrate of acupuncture: evidence, challenges and strategy. Front. Neurosci. 13:100. doi: 10.3389/fnins.2019.00100
Zhao, Z.-Q. (2008). Neural mechanism underlying acupuncture analgesia. Prog. Neurobiol. 85, 355–375. doi: 10.1016/j.pneurobio.2008.05.004
Zung, W. W. (1971). A rating instrument for anxiety disorders. Psychosomatics 12, 371–379. doi: 10.1016/s0033-3182(71)71479-0
Keywords: functional connectivity, primary dysmenorrhea, machine learning, multivariate pattern analyses, acupuncture
Citation: Yu S, Xie M, Liu S, Guo X, Tian J, Wei W, Zhang Q, Zeng F, Liang F and Yang J (2020) Resting-State Functional Connectivity Patterns Predict Acupuncture Treatment Response in Primary Dysmenorrhea. Front. Neurosci. 14:559191. doi: 10.3389/fnins.2020.559191
Received: 29 May 2020; Accepted: 14 August 2020;
Published: 08 September 2020.
Edited by:
Damiana Scuteri, University of Calabria, ItalyReviewed by:
Cheng-Hao Tu, China Medical University, TaiwanCun-Zhi Liu, Beijing University of Chinese Medicine, China
Copyright © 2020 Yu, Xie, Liu, Guo, Tian, Wei, Zhang, Zeng, Liang and Yang. This is an open-access article distributed under the terms of the Creative Commons Attribution License (CC BY). The use, distribution or reproduction in other forums is permitted, provided the original author(s) and the copyright owner(s) are credited and that the original publication in this journal is cited, in accordance with accepted academic practice. No use, distribution or reproduction is permitted which does not comply with these terms.
*Correspondence: Fanrong Liang, YWN1cmVhc2VyY2hAMTI2LmNvbQ==; Jie Yang, amVubnlfeWFuZ19qaWVAMTI2LmNvbQ==
†These authors have contributed equally to this work