Corrigendum: Exploring Peritumoral Neural Tracts by Using Neurite Orientation Dispersion and Density Imaging
- 1Institute of Neuroscience, National Yang Ming Chiao Tung University, Hsinchu, Taiwan
- 2Department of Neurosurgery, First Hospital of Jilin University, Changchun, China
- 3Department of Medical Imaging, Hualien Tzu Chi Hospital, Buddhist Tzu Chi Medical Foundation, Hualien, Taiwan
- 4Department of Radiology, School of Medicine, Tzu Chi University, Hualien, Taiwan
- 5GE Healthcare, Taipei, Taiwan
- 6Center for Geriatrics and Gerontology, Taipei Veterans General Hospital, Taipei, Taiwan
- 7School of Psychology and Cognitive Science, Institute of Cognitive Neuroscience, East China Normal University, Shanghai, China
- 8Institute of Science and Technology for Brain-Inspired Intelligence, Fudan University, Shanghai, China
- 9Brain Research Center, National Yang Ming Chiao Tung University, Hsinchu, Taiwan
Diffusion Tensor Imaging (DTI) tractography has been widely used in brain tumor surgery to ensure thorough resection and minimize functional damage. However, due to enhanced anisotropic uncertainty in the area with peritumoral edema, diffusion tractography is generally not practicable leading to high false-negative results in neural tracking. In this study, we evaluated the usefulness of the neurite orientation dispersion and density imaging (NODDI) derived tractography for investigating structural heterogeneity of the brain in patients with brain tumor. A total of 24 patients with brain tumors, characterized by peritumoral edema, and 10 healthy counterparts were recruited from 2014 to 2021. All participants underwent magnetic resonance imaging. Moreover, we used the images obtained from the healthy participants for calibrating the orientation dispersion threshold for NODDI-derived corticospinal tract (CST) reconstruction. Compared to DTI, NODDI-derived tractography has a great potential to improve the reconstruction of fiber tracking through regions of vasogenic edema. The regions with edematous CST in NODDI-derived tractography demonstrated a significant decrease in the intracellular volume fraction (VFic, p < 0.000) and an increase in the isotropic volume fraction (VFiso, p < 0.014). Notably, the percentage of the involved volume of the concealed CST and lesion-to-tract distance could reflect the motor function of the patients. After the tumor resection, four patients with 1–5 years follow-up were showed subsidence of the vasogenic edema and normal CST on DTI tractography. NODDI-derived tractography revealed tracts within the edematous area and could assist neurosurgeons to locate the neural tracts that are otherwise not visualized by conventional DTI tractography.
Introduction
The cerebral neoplasm may increase the intracranial pressure and cause neural tract deviation, infiltration, or destruction in the site of tumor growth and peritumoral areas (Assaf and Pasternak, 2008). Various magnetic resonance imaging (MRI) techniques are used to access and localize brain tumors to obtain a thorough resection and minimize functional loss. Among these techniques, diffusion tensor imaging (DTI) has been widely used to demonstrate the structural contents and peritumoral neural tracts. DTI is a non-invasive method that can probe the molecular diffusivity of water within the white matter to reflect the intravoxel architecture by measuring the water self-diffusion tensor (Basser et al., 1994; Basser, 1995). Herein, linking the anisotropic orientation determined by the principal eigenvector of the tensor has been widely applied to map the neuronal tracts (Mori et al., 1999; Mori and Van Zijl, 2002), which has also provided crucial information to neurosurgeons for neurosurgical planning and navigation (Berman et al., 2004; Bello et al., 2008). However, as a simplified model, DTI-derived tractography faces a substantial challenge in resolving the fiber tract within a voxel that comprises heterogeneous compartments including infiltrating cells and edematous tissues (Lecoeur et al., 2014; Chen et al., 2016; Gong et al., 2018; Ye et al., 2020).
Most aggressive brain tumors, such as malignant gliomas and metastatic tumors, are usually associated with peritumoral vasogenic edema (Lu et al., 2003). The latter generally infiltrates brain tissues with a lot of body fluid and alters the measures of diffusion characteristics, increasing the uncertainty of presurgical evaluation and leading to an enormous impact on patient management and surgical planning (Assaf and Pasternak, 2008; Lecoeur et al., 2014). Thus, it is crucial to identify the constituents of the infiltrated tissue and perform tractography within the area. However, the use of single ellipsoid DTI model makes this process challenging (Zhang et al., 2013). According to diffusion physics, diffusion-weighted images (DWI) with high b-value encoding can quantify the structural architecture and resolve structural heterogeneity (Callaghan et al., 1991; Lecoeur et al., 2014; Ye et al., 2020). Bi-exponential and higher-order models ascribe magnetic resonance (MR) signal attenuation into two processes, namely, restricted and hindered water diffusion in the intracellular and extracellular spaces, respectively, and provide a unique opportunity to reveal neural tracts within the hydro-environment compared with the conventional method. Previous studies have mentioned that multi-compartment diffusion models, such as the free water model (Lecoeur et al., 2013) and two-tensor unscented Kalman filter (UKF) (Chen et al., 2015, 2016) improve the tracking of the neuronal fiber in peritumoral vasogenic edema. This suggests that fiber tracking in the edema would benefit from the prior heterogeneity. Knowing the microstructure change in the peritumoral region may further help identify whether the fiber still existed, thus suggesting the need for an advanced biophysical diffusion model to explore the edematous fiber tracts (Liao et al., 2017).
Neurite orientation dispersion and density imaging (NODDI) is a clinically feasible three-compartment model that utilizes two b-value encodings (Zhang et al., 2012). The aforementioned model is capable of estimating three types of water diffusion behavior with different volume fractions, i.e., restricted (intracellular volume fraction), hindered (extracellular volume fraction), and free diffusion (isotropic volume fraction). These indices provide special measures that represent the axonal density and the dispersion of fiber orientations. NODDI is reportedly valuable for evaluating various neurological diseases, such as white matter microstructural changes in Alzheimer’s disease (Fu et al., 2020) and Parkinson’s disease (Mitchell et al., 2019). Moreover, it provides distinctive markers for multiple sclerosis (Crombe et al., 2018) and stroke (Adluru et al., 2014; Wang et al., 2019). Numerous studies have suggested that NODDI indices could provide unique contrast in brain tumors (Wen et al., 2015), which will help to differentiate between glioblastoma and solitary brain metastasis and reveal the differences between tumor infiltration and vasogenic edema (Kadota et al., 2020). Moreover, the NODDI indices are capable of characterizing white matter fibers involved in tumoral and edematous brain areas (Masjoodi et al., 2018), which implies that this multi-compartment model may potentially demonstrate neural tractography in peritumoral edema.
Although the peritumoral neural tracts involved in motor functions are crucial to neurosurgery, they are generally concealed by vasogenic edema and usually unseen with traditional diffusion modeling. Therefore, in this study, we aimed to use the NODDI model to investigate the structural heterogeneity in patients with vasogenic edema. First, we reconstructed the edematous corticospinal tract (CST) that were unseen with traditional diffusion modeling. Second, we provided evidence that the concealed tracts could reflect motor function, such as muscle strength, which would benefit surgical planning. Third, white matter integrity profiles were evaluated by the multi-compartment characteristics for better understanding the microstructure heterogeneity of the region of edema. Finally, we examined the disclosed tracts that could be detected following the release of the vasogenic edema.
Materials and Methods
Participants
We recruited a total of 24 patients with brain tumor and 10 healthy participants from 2014 to 2021. The enrolled patients were diagnosed with cerebral neoplasm and a large volume of perilesional vasogenic edema in the motor area, primary somatosensory cortex, or premotor area that affected the CST. The vasogenic edema was determined by peritumoral hyperintensity on T2-weighted fluid-attenuated inversion recovery (T2-FLAIR) images and no evidence of restricted water diffusion on diffusion-weighted imaging (DWI). Each patient underwent muscle strength measurement by using the Medical Research Council (MRC) scale of muscle strength. Motor function decline was defined as abnormal if the MRC grading was 4 or less. This study was approved by the local institutional review board, and all participants provided informed consent for the surgery.
MRI Acquisition and Imaging Preprocessing
Data Set I
The first dataset enrolled six patients and five healthy participants at the Tri-Service General Hospital, Taipei, Taiwan. MRI data were acquired on a 3-T GE machine (Discovery MR 750, GE Healthcare, Milwaukee, United States) with an eight-channel head coil. The clinical routine MR scanning protocols included a high-resolution 3-D Fast Spoiled Gradient Echo T1-weighted images (T1-weighted images (T1WI); field of view (FOV) = 224 × 224 × 146 mm3; voxel size = 1 × 1 × 1 mm3; and TE/TR/TI = 4.2/10.2/450 ms), 2-D axial view fast recovery fast spin-echo T2-weighted images (T2-weighted images (T2WI); FOV = 250 × 250 × 150 mm3; voxel size = 0.49 × 0.49 × 6 mm3; and TE/TR = 100.7/5,619 ms), 2-D axial view FSE T2-FLAIR (FOV = 250 × 250 × 150 mm3; voxel size = 0.49 × 0.49 × 6 mm3; and TE/TR/TI = 118.3/12,000/2,200 ms), and 2-D axial view T1WI following the injection of gadolinium-based contrast agent (gadolinium-enhanced T1WI (Gd-T1WI); FOV = 250 × 250 × 150 mm3; voxel size = 0.49 × 0.49 × 6 mm3; and TE/TR/TI = 31.1/3,465.2/928.8 ms). Two-shelled DWI were acquired using a single-shot spin-echo planer imaging sequence (FOV = 240 × 240 × 112 mm3; voxel size = 2.5 × 2.5 × 2.5 mm3; TE/TR = 88/6,000 ms; four null images; 30 directions of b = 1,000 s/mm2; and 60 directions of b = 2,000 s/mm2).
Data Set II
The second dataset included 18 patients and five healthy participants at The First Hospital of Jilin University, Jilin, China. MRI data were acquired on a 3-T Siemens machine (Magnetom Verio, Siemens, Erlangen, Germany) with a 16-channel head coil. The clinical MR scanning protocol included a 2-D axial view T1WI (FOV = 230 × 230 × 117 mm3; voxel size = 0.36 × 0.36 × 6.5 mm3; and TE/TR/TI = 9/1,500/791.8 ms), 2-D axial view T2WI (FOV = 230 × 230 × 117 mm3; voxel size = 0.6 × 0.6 × 6.5 mm3; and TE/TR = 95/6,000 ms), 2-D axial T2-FLAIR (FOV = 230 × 230 × 117 mm3; voxel size = 0.72 × 0.72 × 6.5 mm3; and TE/TR/TI = 81/7,500/2,297.9 ms), and 3-D magnetization-prepared rapid gradient-echo Gd-T1WI (FOV = 250 × 250 × 160 mm3; voxel size = 1 × 0.98 × 0.98 mm3; and TE/TR/TI = 3.5/2,300/1,070 ms). Two-shelled DWI were acquired using a single-shot spin-echo planer imaging sequence (FOV = 250 × 250 × 160 mm3; voxel size = 2.5 × 2.5 × 2.5 mm3; and TE/TR = 95/16,700 ms; four null images; 30 directions of b = 1,000 s/mm2; and 60 directions of b = 2,000 s/mm2). We also acquired three null diffusion images with opposite polarity (phase encoding form posterior to anterior).
Imaging Preprocessing
All DWI data were processed by the in-house developed DWI preprocess pipeline (OGIO) that includes algorithms and functions from MRtrix31 FMRIB Software Library (FSL)2 and ANTs3. The overall imaging preprocessing workflow is summarized in Figure 1.
First, we used dwidenoise (Veraart et al., 2016a,b; Cordero-Grande et al., 2019) and mrdegibbs (Kellner et al., 2016) functions in MRtrix3 (Tournier et al., 2019) for imaging denoising and removing the Gibbs ringing artifact from the DWI. To correct the susceptibility-included off-resonance field, image distortion induced by the fast-switching gradient, slight head motion, and FSL functions (Jenkinson et al., 2012) TOPUP and EDDY (Graham et al., 2017) were performed on DWIs acquired with two opposite polarities. Finally, dwibiascorrect (Tustison et al., 2010) was utilized to correct performing B1 field inhomogeneity in the DWI volume series. The Brain Extraction Tool function (Smith, 2002) from FSL was used to create a brain mask from the average null DWIs. For T1WI, we used the mrdegibbs (Kellner et al., 2016) function for removing the Gibbs ringing artifact and N4BiasFieldCorrection (Tustison et al., 2010) from ANTS for B1 field inhomogeneity correction.
Model Fitting
All images were fitted with the NODDI model using the NODDI MATLAB toolbox4. The low-b-value images (<1,500 s/mm2) were used in DTI model fitting (with dtifit in FSL). We subsequently obtained the voxel-wise maps. We evaluated the first eigenvector for the principal directions on DTI tractography and used fractional anisotropy (FA) as the fiber tractography termination criteria. For NODDI-derived indices, we calculated the orientation dispersion index (ODI), fiber orientation (the principal directions from NODDI model), and the three absolute volume fractions of NODDI (intracellular volume fraction, VFic; extracellular volume fraction, VFec; isotropic volume fraction, VFiso), where VFic + VFec + VFiso = 1 (Figure 2).
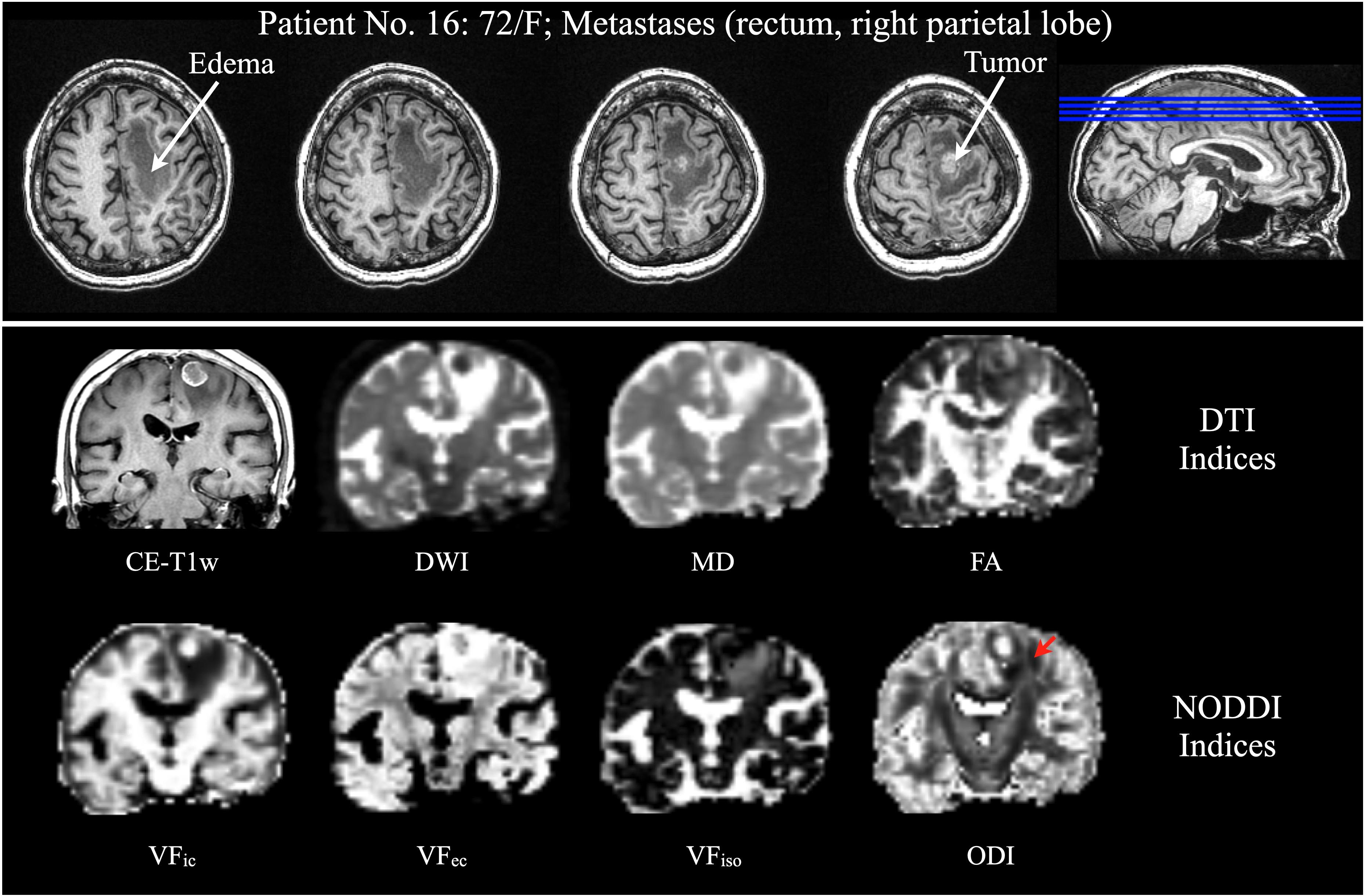
Figure 2. Patient No. 16, a 72-year-old woman with rectum metastasis in the right parietal lobe. (Top) Axial view of CE-T1WI displays an enhancing tumor in the right superior frontal lobe, with significant vasogenic edema. (Bottom) Coronal view of CE-T1WI, DWI, DTI indices (MD, and FA), and NODDI indices (VFic, VFec, and VFiso) could not reveal the CST except the ODI from NODDI (red arrow). CE-T1WI, contrast enhanced T1-weighted images; DWI, diffusion-weighted imaging; DTI, diffusion tensor imaging; MD, mean diffusivity; FA, fractional anisotropy; NODDI, neurite orientation dispersion and density imaging; VFic, intracellular volume fraction; VFec, extracellular volume fraction; and VFiso, isotropic volume fraction; CST, corticospinal tract; and ODI, orientation dispersion index.
ROI Selection
For CST extraction, four regions of interest (ROIs) were manually drawn in the Montreal Neurological Institute (MNI) space according to previous study (Cooper et al., 2015). The first and second ROIs (axial view of sensorimotor area and brainstem) were used for CST extraction. The third and fourth ROIs were the exclusion ROIs for excluding streamlines into the cerebellum and cross-hemisphere connection (Figure 3). Following an inspection of T1WI, T2WI, T2-FLAIR, DWI, and Gd-T1WI, the ROIs of lesion and edema for each patient were manually drawn by using ITK-SNAP5 (Yushkevich et al., 2006). All ROIs were manually drawn by a neuroradiologist (H-W Kao) with 15 years of experience.
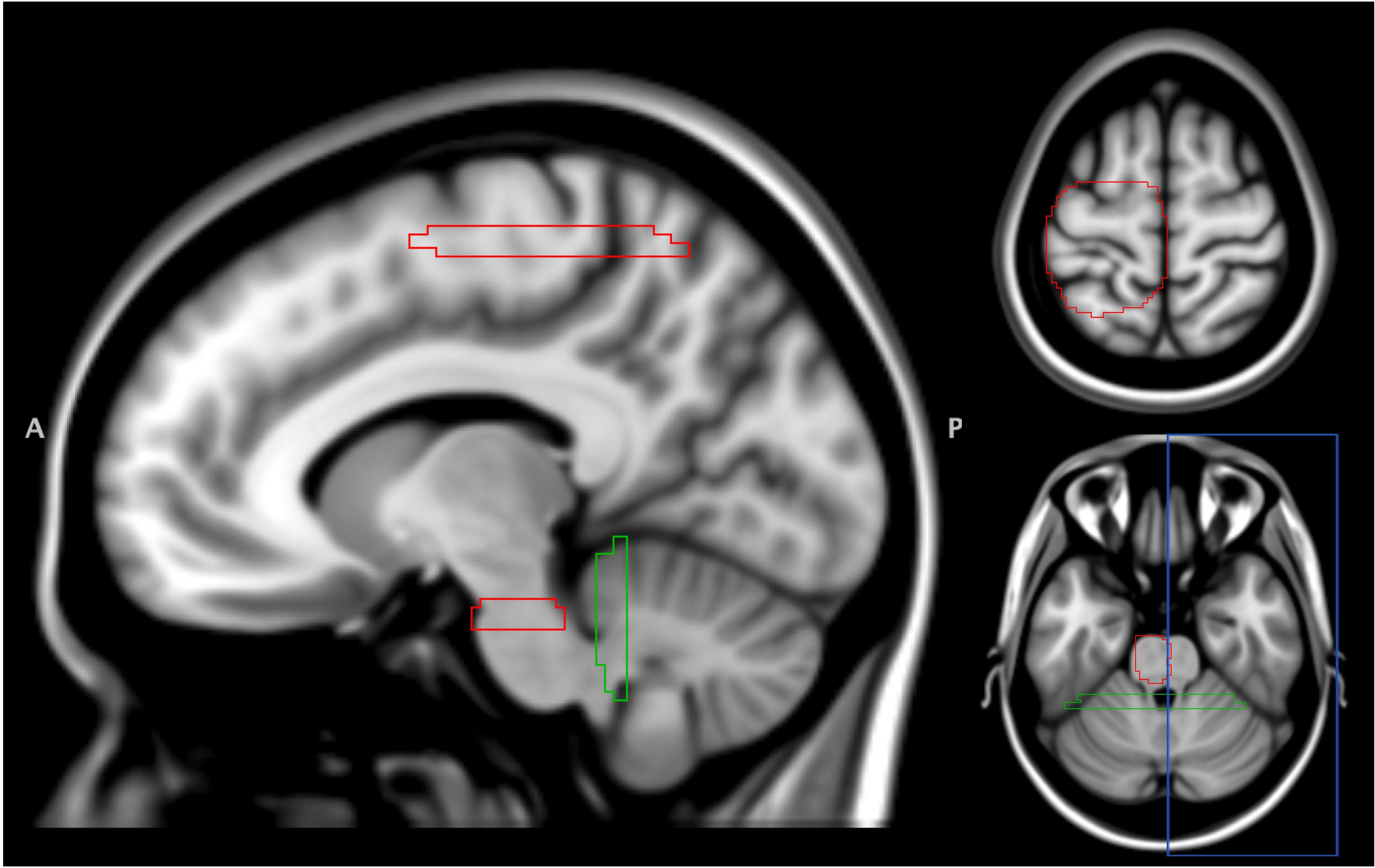
Figure 3. Regions of interest for CST extraction. A total of 4 ROIs were defined in the MNI152 T1 space. Two ROIs (sensorimotor area and brainstem, red) were used for CST extraction. Another two ROIs for excluding streamlines into cerebellum (green) and cross-hemisphere connection (blue). ROI, region of interest; CST, corticospinal tract.
Imaging Registration
To transform the ROIs from the MNI standard space to the individual space, T1WI was first co-registered with average null diffusion images using boundary-based registration to generate the transformation matrix from the DWI space to the T1WI space. Second, T1WI was then spatially normalized to the MNI152 T1 template in the standard space via linear affine transformation (FLIRT) and non-linear registration (FNIRT) (Zhang et al., 2001; Smith et al., 2004; Patenaude et al., 2011). The lesion mask was used to exclude the calculation in the nonlinear registration. Combining the two transformation matrices (DWI to T1WI and T1WI to MNI), we applied the inverse transformation matrix to obtain the ROIs in each individual’s native DWI space with the nearest-neighbor interpolation. All the spatial registration and normalization procedures were implemented in FSL.
Fiber Tractography and ODI Threshold Calibration
CST was reconstructed from the directions identified by both DTI and NODDI. The NODDI-derived CSTs were reconstructed by modifying the FIB file of DSI-studio with NODDI’s ODI and fiber orientation. All fiber tractography was done using the deterministic fiber algorithm (Yeh et al., 2013) implanted in the DSI-Studio6. Four ROIs (two for inclusion and two for exclusion, shown in Figure 3) were utilized for CST extraction. The step size was 0.5 mm; streamlines with lengths shorter than 30 mm or longer than 300 mm were discarded. A total of 3,000 streamlines were finally reconstructed.
To calibrate the ODI threshold, 10 healthy participants (five from each site) were included. We first reconstructed the DTI-derived CST with standard tracking termination criteria [fractional anisotropy (FA) ≤ 0.20; turning angle ≥ 60°] (Mori et al., 1999). Next, the NODDI-derived CSTs were reconstructed with ODI thresholds varying from 0.2 to 1. Moreover, the streamline similarity was evaluated using the dice similarity index (DSI) between DTI tractography and each ODI threshold CST. The DSI was defined as follows: . The optimal ODI threshold was determined with the maximized overlap.
In this study, assessment of the differences between DTI and NODDI-derived tractography was achieved by comparing the DTI-derived CST with the standard FA threshold (FA ≤ 0.20), DTI-derived CST with low FA (FA ≤ 0.10), and NODDI-derived CST with optimal ODI threshold on 24 patients.
Statistical Analyses
To evaluate the microstructure changes in the edema region, we applied the edema mask for extracting the voxels with streamline pass through. The following three conditions of edematous fiber tracts were classified in each patient: (1) DN condition, the volume of both DTI and NODDI-derived fiber tracts in the peritumoral edema; (2) D-only condition, the volume of only DTI fiber tracts in the peritumoral edema; and (3) N-only condition, the volume of only NODDI-derived fiber tracts in the peritumoral edema (Figure 4). We calculated the percentage of involved volume (PIV) in the three conditions of CST to assess the patient’s motor function. PIV was defined as follows: . The volume of CST was defined as the total volume of CST in DTI and NODDI-derived reconstruction. Moreover, we measured the lesion-to-tract distance (LTD) from the lesion boundaries to the nearest CST in a three-dimensional view with the in-house-developed viewer (GoViewer). To integrate the evaluation index with LTD, the volume and PIV of each condition were divided by LTD as weighted.
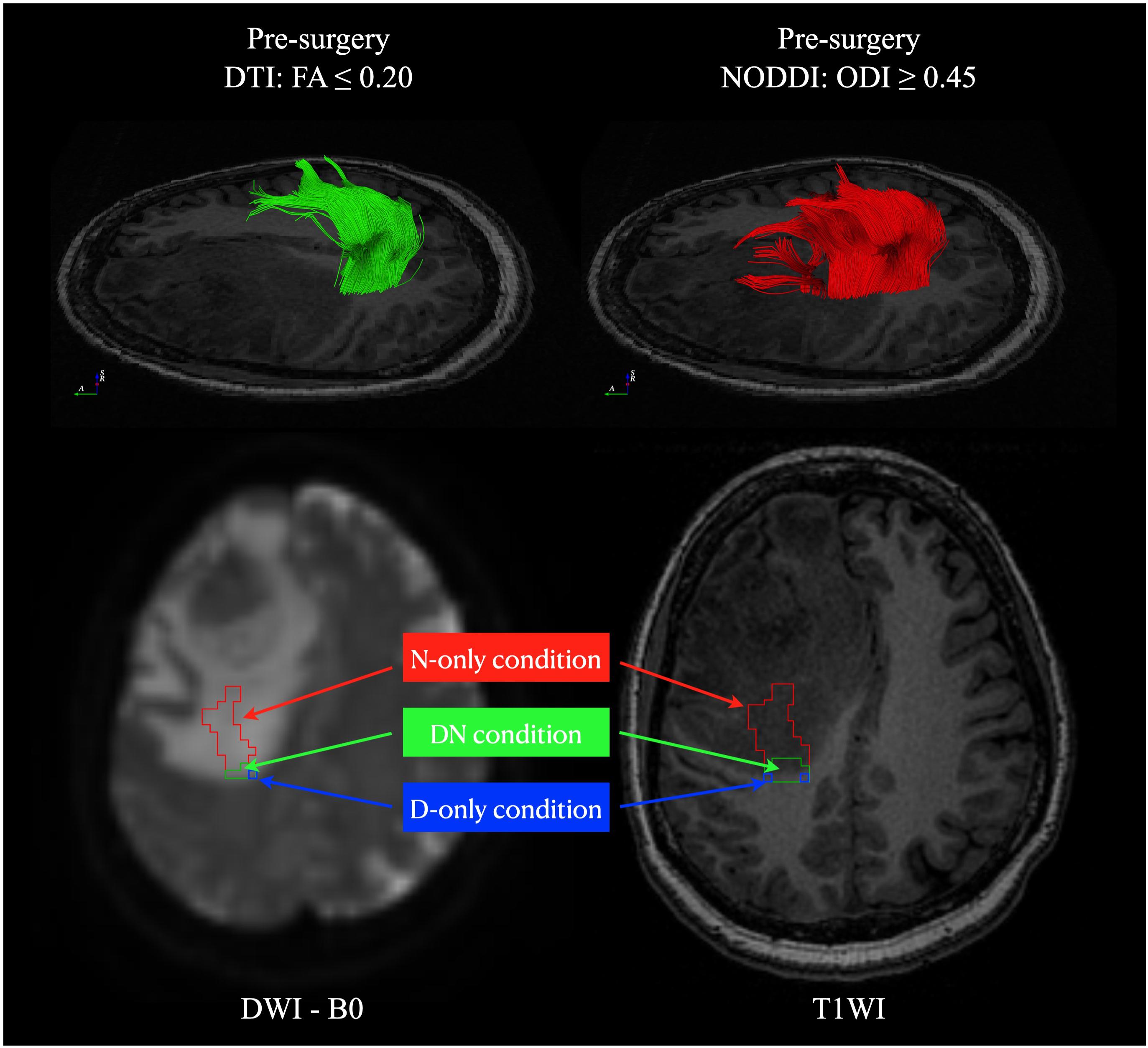
Figure 4. The fiber tracts volume condition definition. Three conditions of the fiber tracts located in areas overlapping with peritumoral edema were classified in each patient: (1) DN condition: the volume of both DTI and NODDI-derived fiber tracts in the peritumoral edema (green); (2) D-only condition: the volume of only DTI fiber tracts in the peritumoral edema (blue); (3) N-only condition: the volume of only NODDI-derived fiber tracts in the peritumoral edema (red). DTI, diffusion tensor imaging; NODDI, neurite orientation dispersion and density imaging.
We determined the diagnostic performance for differentiating the motor function using the receiver operating characteristic (ROC) curves. We calculated the sensitivity, specificity, and area under the ROC curve (AUC) to determine the diagnostic performance of each index. Moreover, Youden’s J statistic was used for determining the optimal cutoff threshold for the ROC curve. The Youden index is defined as Jmax=maxt{sensitivity(t) + specificity(t)−1}, where t denotes the classification threshold for which J is maximal from the ROC curve. Furthermore, we extracted the mean NODDI-derived volume fractions (VFic, VFec, and VFiso) in DN and N-only conditions. The Wilcoxon signed rank test was conducted to evaluate the differences in the microenvironment between the two conditions. All statistical analyses were analyzed using statistical software (SPSS, version 25; SPSS, Chicago, Ill). p-values < 0.05 were considered statistically significant.
Follow-Up Comparison
A total of four patients were recruited after imaging showing subsidence of the vasogenic edema: two patients with 1 year (from data set II) and another two with 5 years (from data set I) after the tumor resection. MRI data acquisition and imaging processing were the same as the initial. The DTI tractography of CST reconstruction was performed with the standard FA criteria (FA ≤ 0.20; turning angle ≥60°). To quantify the restoration of the CST in the follow-up compared to initial DTI- and NODDI-derived tractography, we compared the fiber tractography between pre- and post-surgery. We registered the pre-surgery and follow-up data by using linear registration (FLIRT). DSI was used for the streamline similarity comparison.
Results
The study included a total of 24 patients (9 men and 15 women). The mean age was 52 years, ranging from 32 to 72 years. Seven patients were diagnosed with meningiomas (four and three with grades I and II, respectively). Six patients had gliomas (one with grade I, one with grade II, and two each with grades III and IV). Six patients had metastases (two lung cancers, two rectal cancers, and two cancers of unknown origin). The other five patients had lymphoma, primitive neuroectodermal tumor, Ewing’s sarcoma, abscess, and gliosis. Among our patients, six patients experienced motor function decline. Table 1 summarizes the clinical information, imaging features, LTD, and pathological diagnoses of all patients.
ODI Threshold Calibration
The mean and maximum DSI values of CST reconstruction in the healthy participants were 0.8077 and 0.9164, respectively, with ODI ≥ 0.4482 ± 0.0316. We determined an optimal ODI threshold of 0.45 (Figure 5).
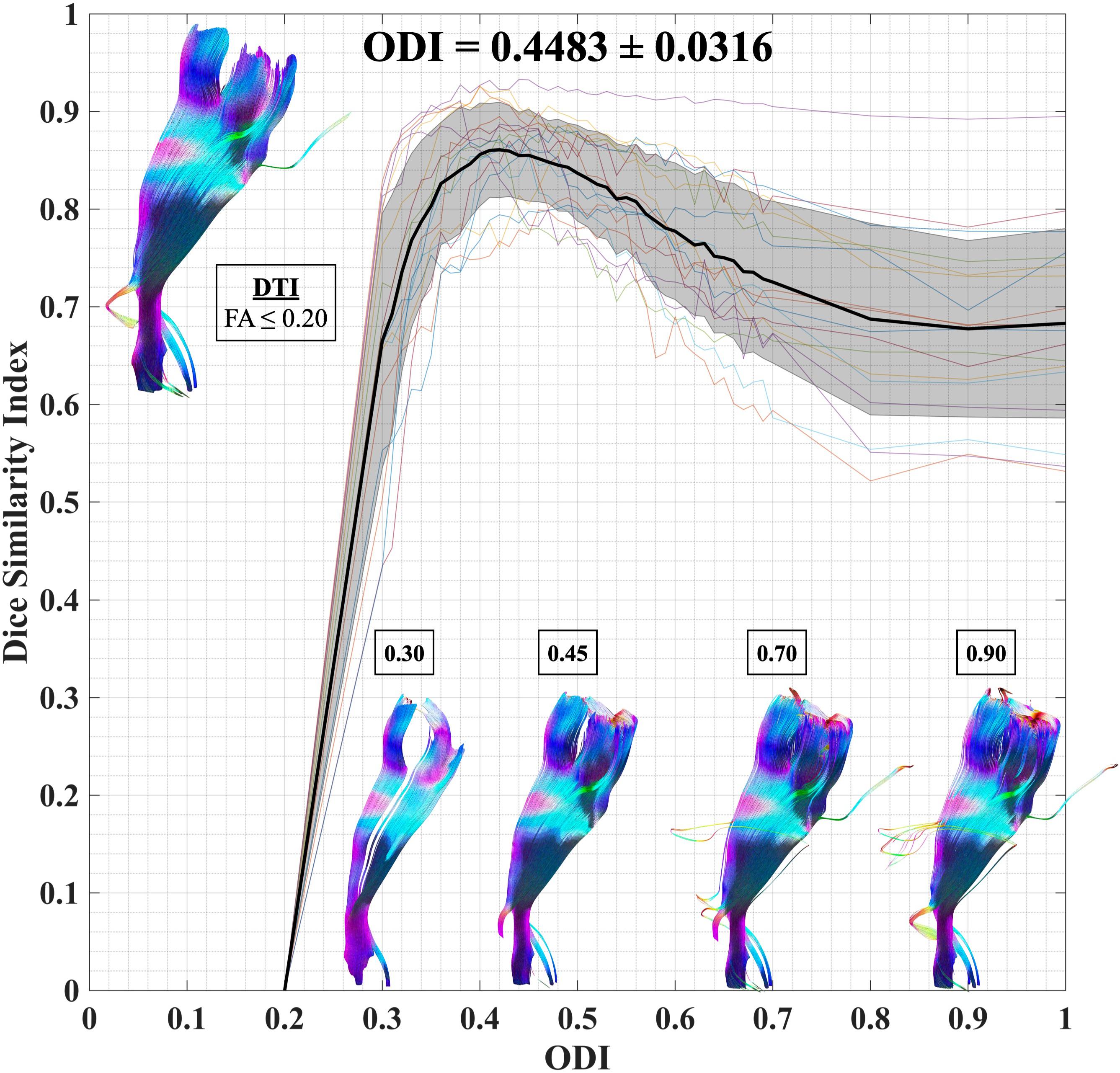
Figure 5. ODI threshold calibration for CST. We have studied the dice similarity index at each considered ODI threshold compared to the standard DTI termination criteria (FA ≤ 0.2; turning angle ≥ 60°). An optimal ODI ≥ 0.45 has been set for CST reconstruction. ODI, orientation dispersion index; CST, corticospinal tract; and DTI, diffusion tensor imaging.
Comparison Between DTI and NODDI
We compared the differences between DTI and NODDI, predominantly found in regions with vasogenic edema. DTI-derived tractography with standard FA displayed non-stabilized performance in the regions of vasogenic edema, comprising only sparse tracts. DTI tractography with low FA revealed better performance but was accompanied by several noisy streamlines. NODDI-derived tractography could reveal the CST in the regions of vasogenic edema, with fewer noisy streamlines (Figures 6, 7). Two patients (patient nos. 2 and 15) failed to reconstruct any of the CST in the regions with vasogenic edema while using DTI tractography (Supplementary Figure 1).
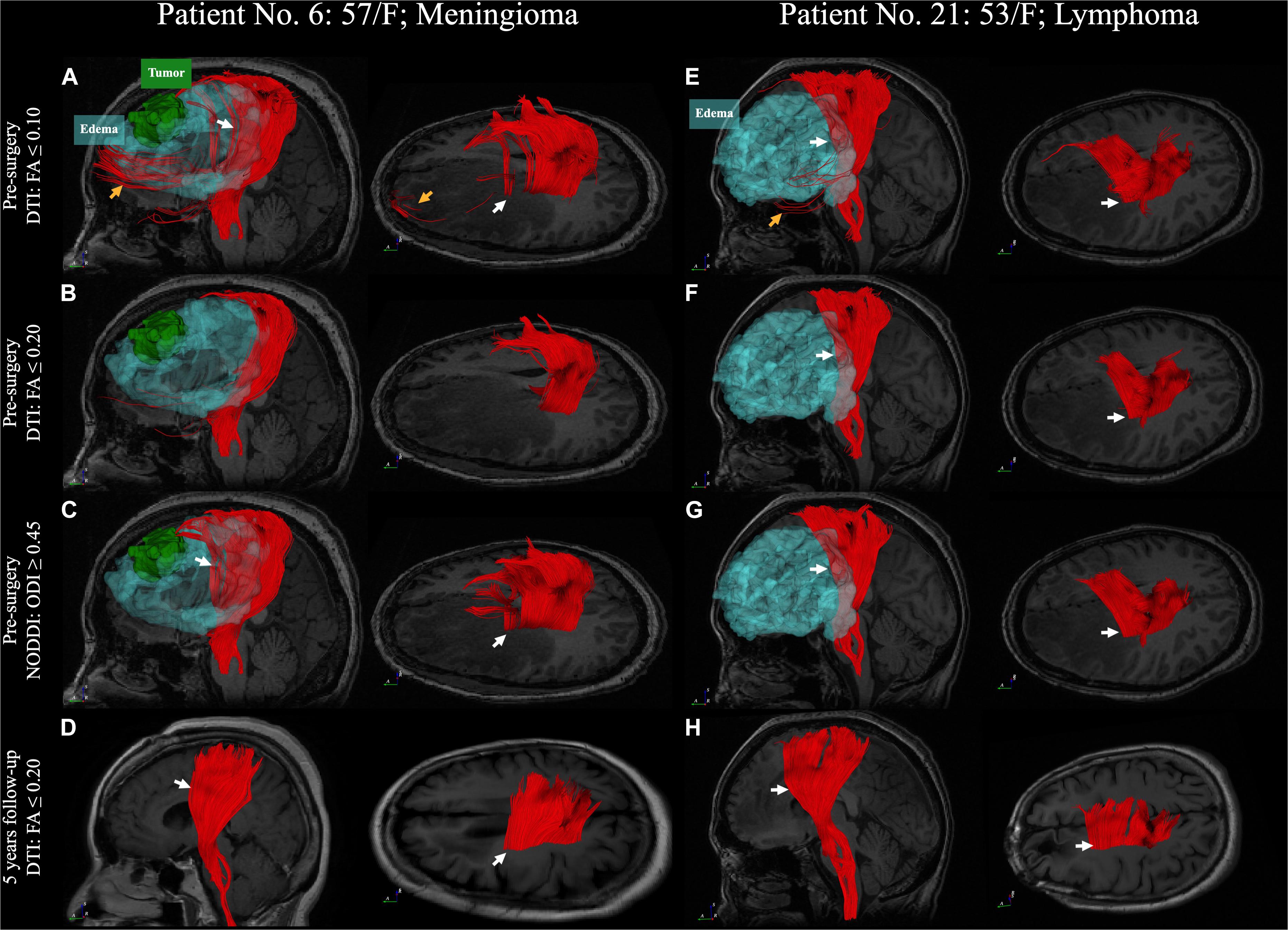
Figure 6. A comparison between DTI and NODDI-derived CST reconstruction with 5 years follow-up. (A–D) Patient No. 6, a 57-year-old woman with a meningioma (WHO grade II) in the left frontal lobe. (E–H) Patient No. 21, a 53-year-old woman with malignant B-cell lymphoma. DTI tractography with standard termination criteria (FA ≤ 0.2) has failed to reconstruct the CST in the region with peritumoral edema in the first case. (B) However, the CST has been successfully reconstructed in the second case (F, white arrow). DTI tractography with low FA criteria (FA ≤ 0.1) displays better performance in the region with peritumoral edema (white arrow). Nonetheless, it is accompanied by noisy streamlines (A,E, yellow arrow). NODDI-derived tractography with ODI reveals better performance in the region with edema and less noisy streamlines (C,G, white arrow). The DTI tractography with standard termination criteria reveals a CST similar to the pre-surgery NODDI-derived CST after the vasogenic edema has subsided in 5 years (D,H). DTI, diffusion tensor imaging; FA, fractional anisotropy; NODDI, neurite orientation dispersion and density imaging; CST, corticospinal tract; WHO, World Health Organization and ODI, orientation dispersion index.
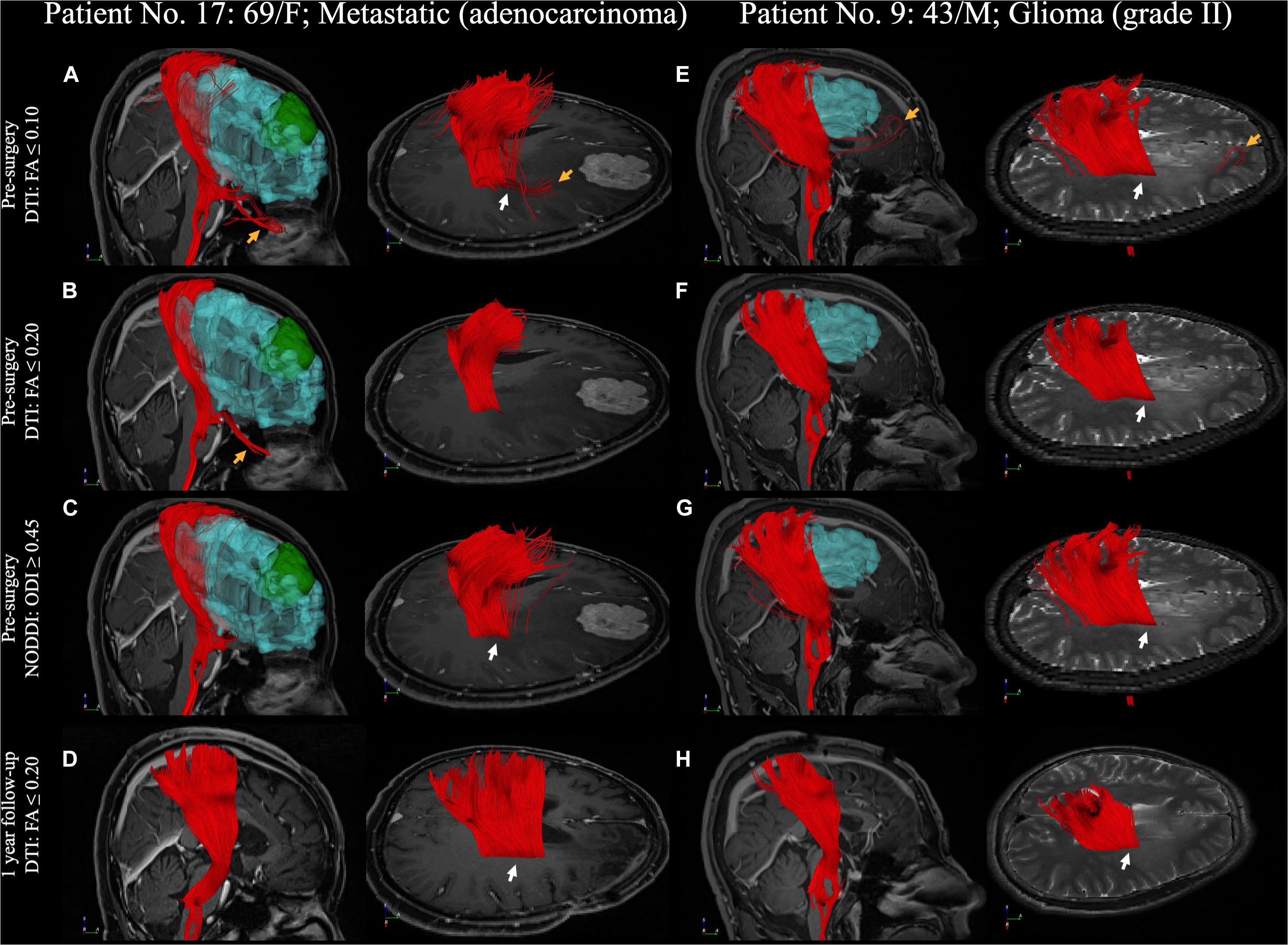
Figure 7. A comparison between DTI and NODDI-derived CST reconstruction with 1-year follow-up. (A–D) Patient No. 17, a 69-year-old woman with an adenocarcinoma metastatic in the right frontal lobe. (E–H) Patient No. 9, a 43-year-old man with WHO grade II glioma in right parietal lobe. CST reconstruction in DTI and NODDI-derived tractography in pre-surgery and 1 year after tumor resection. White arrow indicated the fiber tracts in the peritumoral edema. DTI, diffusion tensor imaging; FA, fractional anisotropy; NODDI, neurite orientation dispersion and density imaging; CST, corticospinal tract; WHO, World Health Organization and ODI, orientation dispersion index.
ROC Diagnostic Performance Analysis
In ROC diagnostic performance analysis, we found that LTD, D-only volume, D-only PIV, N-only volume, N-only volume-LTD (N-only volume with weighted LTD), N-only PIV, and N-only PIV-LTDs (N-only PIV with weighted LTD) had significant differences between patients with and without surgery-related paresis. The PIV of the N-only condition weighted by LTD had the best diagnostic performance for determining the presence of motor function decline. The threshold value of >0.0128 yielded a sensitivity, specificity, and AUC of 100, 100%, and 1, respectively, for patients with motor function decline (Figure 8). The threshold value and diagnostic performance of each index in motor function decline are summarized in Table 2. The statistical details of group comparison between patients with and without motor function decline can be found in Supplementary Table 2.
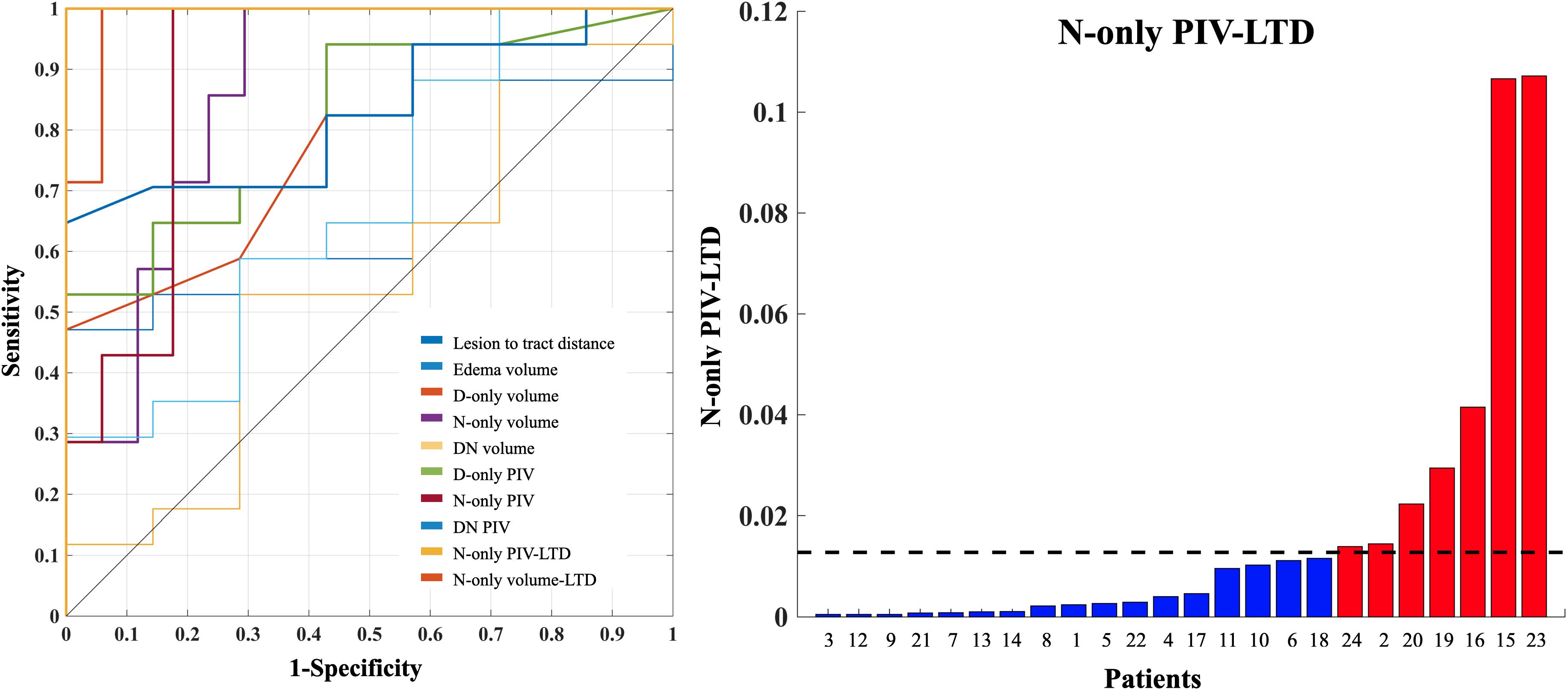
Figure 8. The PIV-LTD of N-only condition and ROC diagnostic performance of motor functions. The PIV of N-only condition weighted by LTD had the best diagnostic performance for determining the presence of motor function decline (left, gold line). An optimal threshold of PIV-LTD of N-only condition >0.0128 has yielded a sensitivity, specificity, and AUC of 100%, 100%, and 1 for differentiating patients with motor function decline (right). The red and blue color bars indicate patients with and without motor function decline. The black dash line indicates the optimal threshold, determined by using the Youden index. PIV, percentage of involved volume; LTD, lesion-to-tract distance; ROC, receiver operating characteristic; AUC, area under the receiver operating characteristic curve.
NODDI Fraction Comparison
In a 2-D barycentric coordinate system, the distribution of the N-only condition (mean VFic = 0.1501; mean VFec = 0.5752; and mean VFiso = 0.2738) was presented in the left upper shift relative to that in the DN condition (mean VFic = 0.2100; mean VFec = 0.5809; and mean VFiso = 0.2091). The statistical comparison of NODDI indices between the N-only condition and DN condition showed no significant difference in VFec (p = 0.4751). Moreover, we observed a significant increase and decrease of VFiso (p < 0.0006) and VFic (p < 0.0036) in the N-only condition when comparing it to the DN condition (Figure 9).
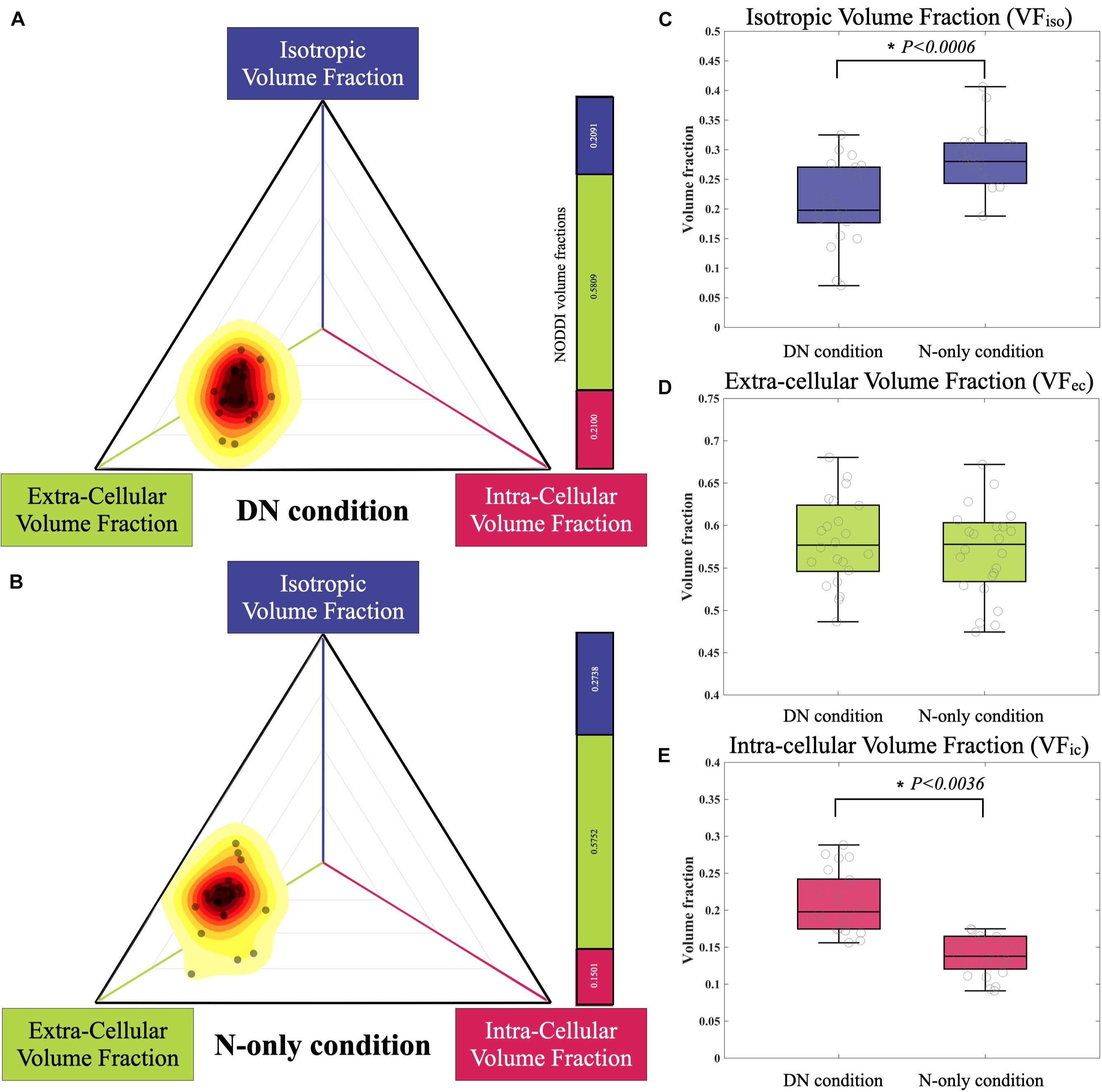
Figure 9. The distribution of NODDI-derived volume fraction in the DN condition and N-only condition are plotted with a 2-D barycentric coordinate system. (A) The N-only condition and (B) distribution reveal a left upper shift in the coordinate system, compared to the DN condition. There is no significant difference in the VFec (p = 0.4751, D). However, there are significant differences in the VFic (p < 0.0036, E) and VFiso (p = 0.0006, C). NODDI, neurite orientation dispersion and density imaging.
Tract Verifications After Releasing the Vasogenic Edema
After the tumor resection, two patients with 5-year follow-up (Figure 6) and two with 1-year follow-up (Figure 7) showed reduced vasogenic edema and normal CST on DTI tractography with standard termination criteria (FA ≤ 0.20). The similarity comparison showed that NODDI-derived tractography performed the highest similarity and lowest standard deviation (0.607 ± 0.035) with post-surgery fiber tractography (Figure 10).
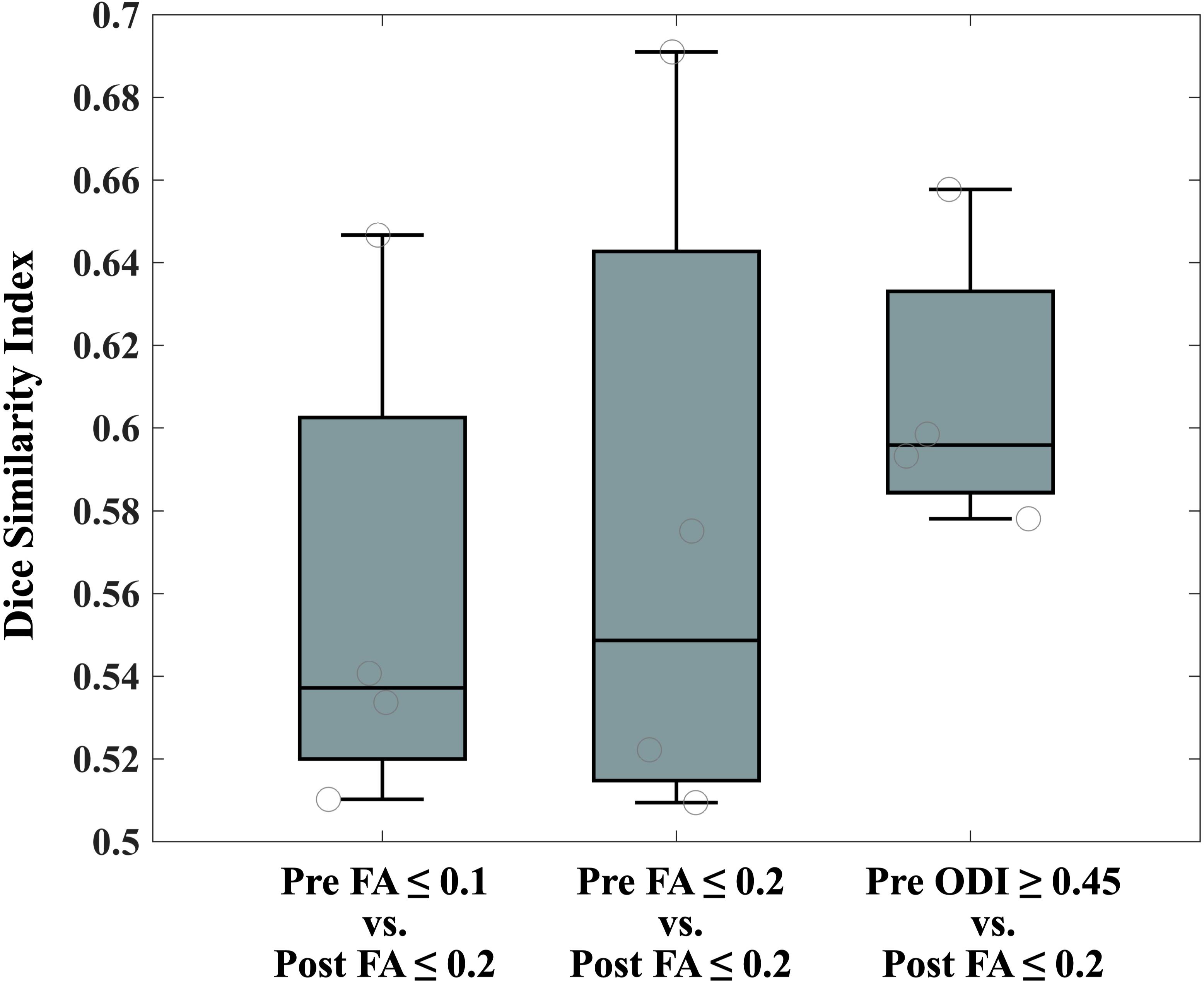
Figure 10. The similarity comparison of CST reconstruction between pre- and post-surgery. CST, corticospinal tract.
Discussion
Specific functional tracts like CST that are concealed by peritumoral edema are meaningful to neurosurgeons. However, they have not yet been systematically disclosed. We applied an advanced NODDI model to different diffusion contents to highlight the neural anisotropy and tractography. Compared to conventional DTI tractography, NODDI-derived fiber tractography provided better performance in heterogeneous areas. Notably, the involved volume of the concealed tracts and LTD directly reflected their functional performance, which, in turn, would facilitate pre-surgery planning and post-surgery recovery assessment. After the vasogenic edema subsided, we verified the neural tracts in four patients during the 1–5-year follow-up.
DTI tractography effectively maps neural tracts within the human brain. However, it has been proven unreliable in revealing the fiber tracts in the peritumoral edema region (Zhang et al., 2013; Ye et al., 2020). Nonetheless, specific functional fiber tracts that are located in areas overlapping with peritumoral edema are crucial to the functional protection of the patients. Previous studies have mentioned that the single-tensor model has a high probability of failure in revealing the fiber tracts when the image voxel gets affected by the vasogenic edema (partial volume effect) (Lecoeur et al., 2013; Zhang et al., 2013; Liao et al., 2017). This necessitates the deconstruction of DWI signals. Lecoeur et al. (2013) proposed removing the free water compartment for tensor correction and achieved significantly improved tractography in the presence of edema. This concept was extended to further studies, such as free water modeling (Gong et al., 2018), two-tensor unscented UKF (Chen et al., 2015, 2016; Liao et al., 2017), and DBSI (Ye et al., 2020). Following the exclusion of the free water compartment, the above-mentioned models demonstrated the possibility of tracing a larger volume of fiber bundles. Derived from the NODDI model, the ODI summarized the angular variation of neurites by excluding the isotropic diffusivity. Therefore, the ODI showed a more apparent trend in fiber direction in the area with vasogenic edema when compared to the DTI-derived FA map (Figure 2). It also displayed higher sensitivity and specificity of CST reconstruction in the NODDI-derived tractography than did DTI tractography (Figure 6). We specified on CST reconstruction to systematically compare the results and validate them through follow-up data. Previous studies have suggested that the vasogenic edema may be resolved in 4 days after total removal and that it disappeared entirely by 2–3 weeks post-operation (Stevens et al., 1983; Shirotani et al., 1994). Despite a follow-up in only four patients, the tractography results and the similarity comparison suggested that free water fraction exclusion could improve fiber tracking in regions with vasogenic edema.
Conventionally, the FA threshold, one of the stopping criteria for DTI tractography, was chosen rather arbitrarily, ranging from 0.15 to 0.30 for general tracking purposes (Stadlbauer et al., 2007; Bello et al., 2008). Stadlbauer et al. (2007) conducted a study on brain tumor infiltration and associated vasogenic edema. They demonstrated that an increase in the FA threshold stepped up the distance between the reconstructed fiber bundles and the tumors and decreased the number of detectable fiber bundles. Accordingly, the FA threshold was usually selected at 0.15 or 0.10 to display the peritumoral neural tracts (Bello et al., 2008). Despite the high tracking sensitivity of low FA, low specificity increases the probability of noisy streamline reconstruction. This, in turn, concurrently increases the uncertainty of neurosurgery. Therefore, surgeons should be more careful while applying the results mentioned above in surgical planning and navigation.
Following the exposure of the fiber tracts located in areas overlapping with peritumoral edema, we calculated the CST volume and LTD associated with motor functions. Numerous studies have suggested that the CST volume may reflect clinical outcomes, such as stroke recovery (Auriat et al., 2015) and amyotrophic lateral sclerosis (Wang et al., 2006). Sollmann et al. (2019) and Yu et al. (2020) suggested that the LTD was related to surgery-related transient impairment and may well be regarded as a presumptive predictor of outcome, attributable in part to tumor edema. In this study, we removed the edematous signal to highlight the percentage of the involved volume of the CST. The combination of PIV and LTD index revealed a direct association with motor function decline. Our results suggested that an increase in volume in the edema area and decrease in LTD may reflect motor function decline (Figure 8).
Furthermore, the fiber tracts located in areas overlapping with peritumoral edema that only existed in NODDI-derived tractography (N-only condition) significantly increased and decreased in VFiso and VFic when compared with the DN-only condition (Figure 9). In the DTI model, the FA index was calculated by a total signal in each voxel. Unlike DTI, the NODDI model separated the total signal into three compartments: VFic, VFec, and VFiso. However, in the calculation of NODDI’s ODI, only VFic and VFec were used (Zhang et al., 2012). While comparing the NODDI indices between DN and N-only conditions, we found that there were no significant differences in the VFec, but there were in VFic and VFiso. The region with increasing isotropic volume fraction (increased water content of the surrounding tissue) causes the FA’s decrease and failure to reconstruct the CST in DTI tractography. This suggested that by excluding the isotropic volume fraction (edema effect), NODDI-derived tractography could reveal the fiber tracts located in areas overlapping with peritumoral edema. Based on the biophysical model, the increase in VFiso may reflect the severity of vasogenic edema. Similarly, the decrease in VFic (neurite density index) may reflect focal neurologic deficits (neuron injury or axonal loss) and could also refer to the cognitive function decline (Kaal and Vecht, 2004; Parker et al., 2018). The edematous fiber tracts that only existed in DTI-derived tractography (D-only condition) found significant differences in the comparison between patients with and without motor function decline. However, as the D-only volume was relatively small (D-only volume = 31.45 ± 54.56 mm3), the estimation of the differences within these regions may come from the variability of the fiber orientation estimated by the DTI model.
Limitations
Despite the good performance of the NODDI model in revealing the fiber tracts located in areas overlapping with peritumoral edema, our study has some limitations. First, our sample populations included a variety of tumor types. Previous studies have suggested that patients with glioma presumably had tumor-infiltrated edema, which was different from the pure vasogenic edema (Lu et al., 2003, 2004; Min et al., 2013). However, there were no significant differences found in the volume of edema with CST (DN-condition and N-only condition) in our dataset, which may suggest that patients in our dataset with edematous CST may not have infiltration by the tumor cell. The group comparison between vasogenic edema and edematous CST analysis can be found in Supplementary Material. Second, our MRI data were recruited from two different centers since these datasets were hard to obtain. Although the quantitative measurement that we used from the NODDI model, such as ODI, was normalized and calibrated from 10 healthy participants (five participants from each center), the variability from the different scanners still needed to be taken seriously. Third, our main purpose was to investigate the effect of edema on routinely employed tractography in clinical practice. The effect of crossing fiber in human white matter (Jeurissen et al., 2013) should be considered, and it would be necessary to utilize a model that focuses on solving crossing fiber-like constrained spherical deconvolution (CSD) (Tournier et al., 2008) in the future studies. Fourth, the NODDI protocol we used in this study is more time-consuming than the regular clinical DTI. The suggested NODDI protocol was encoded with two shells, 30 directions with 700 s/mm2 and 60 directions with 2,000 s/mm2 (Zhang et al., 2012), which involved approximately 15 min in our scanner with |Gmax|=45mT/m. The balance of the trade-off between acquisition time and imaging resolution was shifted toward lower imaging resolution to decrease the acquisition time. Thus, we used 2.5 mm3 as the isotropic voxel size, which is an acceptable protocol (Mohd Taib et al., 2017). Fifth, the optimal ODI threshold of 0.45 was only calibrated for the CST. The ODI thresholds were calibrated for the corpus callosum (0.478) and CST (0.467) in the study conducted by Figini et al. (2014), with values closer to our optimal values. However, the ODI threshold may differ while reconstructing other tracts. Finally, the correlation with intraoperative stimulation was absent. The changes of the post-operative CST examination in our study could be affected by multiple factors, especially the surgical approach. Future studies will require a larger sample size and direct cortical stimulation for validating the ability of NODDI-derived fiber tractography in the region of vasogenic edema in a more direct way.
Conclusion
Our results demonstrated that NODDI-derived fiber tractography had a great potential to improve the reconstruction of fiber tracking through regions of vasogenic edema. Increasing the isotropic volume fraction or decreasing the intracellular volume fraction may cause the DTI tractography failure to reveal the fiber tracts located in areas with peritumoral edema. Moreover, the volume of edematous CST that was reconstructed by NODDI-derived tractography could provide an accurate microstructure index for clinical quantitative measurements and diagnostic performance. Therefore, this technique may help neurosurgeons to define a better and safe margin for tumor resection.
Data Availability Statement
The raw data supporting the conclusions of this article will be made available by the authors, without undue reservation.
Ethics Statement
The studies involving human participants were reviewed and approved by Institutional Review Board, Tri-Service General Hospital (TSGHIRB No. 1-102-05-109) Institutional Review Board, First Hospital of Jilin University (2017-465). The patients/participants provided their written informed consent to participate in this study. Written informed consent was obtained from the individual (s) for the publication of any potentially identifiable images or data included in this article.
Author Contributions
SC, H-WK, and C-PL contributed to the conception and design of the study. XL and H-WK organized the database. SC, C-YEL, C-CH, Y-CK, K-TK, C-CHH, and C-YL provided the methodology support. SC wrote the first draft of the manuscript. SC, H-WK and C-PL wrote sections of the manuscript. YL and GZ provide the additional clinical suggestions during the revision. All authors contributed to manuscript revision, read, and approved the submitted version.
Funding
C-YL was partially supported by the Shanghai Municipal Science and Technology Major Project (No.2018SHZDZX01), the National Key Research and Development Program of China (No. 2018YFC0910503), the Young Scientists Fund of the National Natural Science Foundation of China (No. 81801774), the Natural Science Foundation of Shanghai (No. 18ZR1403700), ZJ Lab, and Shanghai Center for Brain Science and Brain-Inspired Technology. C-PL was supported by the Ministry of Science and Technology (MOST) of Taiwan (grants MOST 110-2321-B-010-004, MOST 110-2321-B-010-007, MOST 108-2321-B-010-010-MY2, and MOST 110-2634-F-010-001), Veterans General Hospitals and University System of Taiwan Joint Research Program (VGHUST110-G1-1-3). This work was financially supported by the Brain Research Center, National Yang Ming Chiao Tung University from the Featured Areas Research Center Program within the framework of the Higher Education Sprout Project by the Ministry of Education (MOE) in Taiwan (110BRC-B501).
Conflict of Interest
C-YEL was employed by GE Healthcare.
The remaining authors declare that the research was conducted in the absence of any commercial or financial relationships that could be construed as a potential conflict of interest.
Publisher’s Note
All claims expressed in this article are solely those of the authors and do not necessarily represent those of their affiliated organizations, or those of the publisher, the editors and the reviewers. Any product that may be evaluated in this article, or claim that may be made by its manufacturer, is not guaranteed or endorsed by the publisher.
Supplementary Material
The Supplementary Material for this article can be found online at: https://www.frontiersin.org/articles/10.3389/fnins.2021.702353/full#supplementary-material
Footnotes
- ^ https://www.mrtrix.org/
- ^ https://fsl.fmrib.ox.ac.uk/fsl/fslwiki/
- ^ http://picsl.upenn.edu/ANTS
- ^ http://www.nitrc.org/projects/noddi_toolbox/
- ^ http://www.itksnap.org
- ^ http://dsi-studio.labsolver.org
References
Adluru, G., Gur, Y., Anderson, J. S., Richards, L. G., Adluru, N., and Dibella, E. V. R. (2014). “Assessment of white matter microstructure in stroke patients using NODDI,” in Proceedings of the 2014 36th Annual International Conference of the IEEE Engineering in Medicine and Biology Society, (Piscataway, NY: IEEE).
Assaf, Y., and Pasternak, O. (2008). Diffusion tensor imaging (DTI)-based white matter mapping in brain research: a review. J. Mol. Neurosci. 34, 51–61. doi: 10.1007/s12031-007-0029-0
Auriat, A. M., Borich, M. R., Snow, N. J., Wadden, K. P., and Boyd, L. A. (2015). Comparing a diffusion tensor and non-tensor approach to white matter fiber tractography in chronic stroke. Neuroimage Clin. 7, 771–781. doi: 10.1016/j.nicl.2015.03.007
Basser, P. J. (1995). Inferring microstructural features and the physiological state of tissues from diffusion-weighted images. NMR Biomed. 8, 333–344. doi: 10.1002/nbm.1940080707
Basser, P. J., Mattiello, J., and Lebihan, D. (1994). MR diffusion tensor spectroscopy and imaging. Biophys. J. 66, 259–267. doi: 10.1016/s0006-3495(94)80775-1
Bello, L., Gambini, A., Castellano, A., Carrabba, G., Acerbi, F., Fava, E., et al. (2008). Motor and language DTI Fiber Tracking combined with intraoperative subcortical mapping for surgical removal of gliomas. NeuroImage 39, 369–382. doi: 10.1016/j.neuroimage.2007.08.031
Berman, J. I., Berger, M. S., Mukherjee, P., and Henry, R. G. (2004). Diffusion-tensor imaging—guided tracking of fibers of the pyramidal tract combined with intraoperative cortical stimulation mapping in patients with gliomas. J. Neurosurg. 101, 66–72. doi: 10.3171/jns.2004.101.1.0066
Callaghan, P. T., Coy, A., Macgowan, D., Packer, K. J., and Zelaya, F. O. (1991). Diffraction-like effects in NMR diffusion studies of fluids in porous solids. Nature 351, 467–469. doi: 10.1038/351467a0
Chen, Z., Tie, Y., Olubiyi, O., Rigolo, L., Mehrtash, A., Norton, I., et al. (2015). Reconstruction of the arcuate fasciculus for surgical planning in the setting of peritumoral edema using two-tensor unscented Kalman filter tractography. NeuroImage Clin. 7, 815–822. doi: 10.1016/j.nicl.2015.03.009
Chen, Z., Tie, Y., Olubiyi, O., Zhang, F., Mehrtash, A., Rigolo, L., et al. (2016). Corticospinal tract modeling for neurosurgical planning by tracking through regions of peritumoral edema and crossing fibers using two-tensor unscented Kalman filter tractography. Int. J. Comput. Assisted Radiol. Surg. 11, 1475–1486. doi: 10.1007/s11548-015-1344-5
Cooper, M., Thapar, A., and Jones, D. K. (2015). ADHD severity is associated with white matter microstructure in the subgenual cingulum. Neuroimage Clin. 7, 653–660. doi: 10.1016/j.nicl.2015.02.012
Cordero-Grande, L., Christiaens, D., Hutter, J., Price, A. N., and Hajnal, J. V. (2019). Complex diffusion-weighted image estimation via matrix recovery under general noise models. NeuroImage 200, 391–404. doi: 10.1016/j.neuroimage.2019.06.039
Crombe, A., Planche, V., Raffard, G., Bourel, J., Dubourdieu, N., Panatier, A., et al. (2018). Deciphering the microstructure of hippocampal subfields with in vivo DTI and NODDI: applications to experimental multiple sclerosis. NeuroImage 172, 357–368. doi: 10.1016/j.neuroimage.2018.01.061
Figini, M., Baselli, G., Riva, M., Bello, L., Zhang, H., and Bizzi, A. (2014). “NODDI performs better than DTI in brain tumors with vasogenic edema,” in Proceedings of the Joint Annual Meeting ISMRM-ESMRMB, (Milan).
Fu, X., Shrestha, S., Sun, M., Wu, Q., Luo, Y., Zhang, X., et al. (2020). Microstructural white matter alterations in mild cognitive impairment and alzheimer’s disease. Clin. Neuroradiol. 30, 569–579.
Gong, S., Zhang, F., Norton, I., Essayed, W. I., Unadkat, P., Rigolo, L., et al. (2018). Free water modeling of peritumoral edema using multi-fiber tractography: application to tracking the arcuate fasciculus for neurosurgical planning. PLoS One 13:e0197056. doi: 10.1371/journal.pone.0197056
Graham, M. S., Drobnjak, I., Jenkinson, M., and Zhang, H. (2017). Quantitative assessment of the susceptibility artefact and its interaction with motion in diffusion MRI. PLoS One 12:e0185647. doi: 10.1371/journal.pone.0185647
Jenkinson, M., Beckmann, C. F., Behrens, T. E. J., Woolrich, M. W., and Smith, S. M. (2012). FSL. NeuroImage 62, 782–790.
Jeurissen, B., Leemans, A., Tournier, J.-D., Jones, D. K., and Sijbers, J. (2013). Investigating the prevalence of complex fiber configurations in white matter tissue with diffusion magnetic resonance imaging. Hum. Brain Map. 34, 2747–2766. doi: 10.1002/hbm.22099
Kaal, E. C. A., and Vecht, C. J. (2004). The management of brain edema in brain tumors. Curr. Opin. Oncol. 16, 593–600.
Kadota, Y., Hirai, T., Azuma, M., Hattori, Y., Khant, Z. A., Hori, M., et al. (2020). Differentiation between glioblastoma and solitary brain metastasis using neurite orientation dispersion and density imaging. J. Neuroradiol. 47, 197–202. doi: 10.1016/j.neurad.2018.10.005
Kellner, E., Dhital, B., Kiselev, V. G., and Reisert, M. (2016). Gibbs-ringing artifact removal based on local subvoxel-shifts. Magnet. Reson. Med. 76, 1574–1581. doi: 10.1002/mrm.26054
Lecoeur, J., Caruyer, E., Elliott, M., Brem, S., Macyszyn, L., and Verma, R. (2014). “Addressing the challenge of edema in fiber tracking,” in Proceedings of the MICCAI 2014 DTI Tractography Challenge, (Boston).
Lecoeur, J., Caruyer, E., Macyszyn, L., and Verma, R. (2013). “Improving white matter tractography by resolving the challenges of edema,” in Proceedings of the MICCAI 2013 DTI Tractography Challenge, (Nagoya).
Liao, R., Ning, L., Chen, Z., Rigolo, L., Gong, S., Pasternak, O., et al. (2017). Performance of unscented Kalman filter tractography in edema: analysis of the two-tensor model. NeuroImage Clin. 15, 819–831. doi: 10.1016/j.nicl.2017.06.027
Lu, S., Ahn, D., Johnson, G., and Cha, S. (2003). Peritumoral diffusion tensor imaging of high-grade gliomas and metastatic brain tumors. AJNR Am. J. Neuroradiol. 24, 937–941.
Lu, S., Ahn, D., Johnson, G., Law, M., Zagzag, D., and Grossman, R. I. (2004). Diffusion-Tensor MR imaging of intracranial neoplasia and associated peritumoral edema: introduction of the tumor infiltration index. Radiology 232, 221–228. doi: 10.1148/radiol.2321030653
Masjoodi, S., Hashemi, H., Oghabian, M. A., and Sharifi, G. (2018). Differentiation of edematous, tumoral and normal areas of brain using diffusion tensor and neurite orientation dispersion and density imaging. J. Biomed. Phys. Eng. 8, 251–260.
Min, Z.-G., Niu, C., Rana, N., Ji, H.-M., and Zhang, M. (2013). Differentiation of pure vasogenic edema and tumor-infiltrated edema in patients with peritumoral edema by analyzing the relationship of axial and radial diffusivities on 3.0T MRI. Clin. Neurol. Neurosurg. 115, 1366–1370. doi: 10.1016/j.clineuro.2012.12.031
Mitchell, T., Archer, D. B., Chu, W. T., Coombes, S. A., Lai, S., Wilkes, B. J., et al. (2019). Neurite orientation dispersion and density imaging (NODDI) and free-water imaging in Parkinsonism. Hum. Brain Map. 40, 5094–5107. doi: 10.1002/hbm.24760
Mohd Taib, N., Kamil, W., Shuaib, I. L., Magosso, E., and Mat Isa, S. (2017). Determination of optimum combination of voxel size and b-value for brain diffusion tensor imaging. Sains Malaysiana 46, 67–74. doi: 10.17576/jsm-2017-4601-09
Mori, S., Crain, B. J., Chacko, V. P., and Van Zijl, P. C. (1999). Three-dimensional tracking of axonal projections in the brain by magnetic resonance imaging. Ann. Neurol. 45, 265–269. doi: 10.1002/1531-8249(199902)45:2<265::aid-ana21>3.0.co;2-3
Mori, S., and Van Zijl, P. C. M. (2002). Fiber tracking: principles and strategies - a technical review. NMR Biomed. 15, 468–480. doi: 10.1002/nbm.781
Parker, T. D., Slattery, C. F., Zhang, J., Nicholas, J. M., Paterson, R. W., Foulkes, A. J. M., et al. (2018). Cortical microstructure in young onset Alzheimer’s disease using neurite orientation dispersion and density imaging. Hum. Brain Map. 39, 3005–3017. doi: 10.1002/hbm.24056
Patenaude, B., Smith, S. M., Kennedy, D. N., and Jenkinson, M. (2011). A Bayesian model of shape and appearance for subcortical brain segmentation. NeuroImage 56, 907–922. doi: 10.1016/j.neuroimage.2011.02.046
Shirotani, T., Shima, K., and Chigasaki, H. (1994). Resolution of Peritumoral Brain Edema Following Excision of Meningioma. Vienna: Springer, 416–418.
Smith, S. M. (2002). Fast robust automated brain extraction. Hum. Brain Map. 17, 143–155. doi: 10.1002/hbm.10062
Smith, S. M., Jenkinson, M., Woolrich, M. W., Beckmann, C. F., Behrens, T. E. J., Johansen-Berg, H., et al. (2004). Advances in functional and structural MR image analysis and implementation as FSL. NeuroImage 23, S208–S219.
Sollmann, N., Fratini, A., Zhang, H., Zimmer, C., Meyer, B., and Krieg, S. M. (2019). Associations between clinical outcome and tractography based on navigated transcranial magnetic stimulation in patients with language-eloquent brain lesions. J. Neurosurg. 132, 1033–1042. doi: 10.3171/2018.12.jns182988
Stadlbauer, A., Nimsky, C., Buslei, R., Salomonowitz, E., Hammen, T., Buchfelder, M., et al. (2007). Diffusion tensor imaging and optimized fiber tracking in glioma patients: histopathologic evaluation of tumor-invaded white matter structures. NeuroImage 34, 949–956. doi: 10.1016/j.neuroimage.2006.08.051
Stevens, J. M., Ruiz, J. S., and Kendall, B. E. (1983). Observation on peritumoural oedema in meningioma. Part I: distribution, spread and resolution of vasogenic oedema seen on computed tomography. Neuroradiology 25, 71–80. doi: 10.1007/bf00333295
Tournier, J.-D., Smith, R., Raffelt, D., Tabbara, R., Dhollander, T., Pietsch, M., et al. (2019). MRtrix3: a fast, flexible and open software framework for medical image processing and visualisation. NeuroImage 202:116137.
Tournier, J. D., Yeh, C.-H., Calamante, F., Cho, K.-H., Connelly, A., and Lin, C.-P. (2008). Resolving crossing fibres using constrained spherical deconvolution: validation using diffusion-weighted imaging phantom data. NeuroImage 42, 617–625. doi: 10.1016/j.neuroimage.2008.05.002
Tustison, N. J., Avants, B. B., Cook, P. A., Yuanjie, Z., Egan, A., Yushkevich, P. A., et al. (2010). N4ITK: improved N3 Bias Correction. IEEE Trans. Med. Imaging 29, 1310–1320. doi: 10.1109/tmi.2010.2046908
Veraart, J., Fieremans, E., and Novikov, D. S. (2016a). Diffusion MRI noise mapping using random matrix theory. Magnet. Reson. Med. 76, 1582–1593. doi: 10.1002/mrm.26059
Veraart, J., Novikov, D. S., Christiaens, D., Ades-Aron, B., Sijbers, J., and Fieremans, E. (2016b). Denoising of diffusion MRI using random matrix theory. NeuroImage 142, 394–406. doi: 10.1016/j.neuroimage.2016.08.016
Wang, S., Poptani, H., Bilello, M., Wu, X., Woo, J. H., Elman, L. B., et al. (2006). Diffusion tensor imaging in amyotrophic lateral sclerosis: volumetric analysis of the corticospinal tract. Am. J. Neuroradiol. 27, 1234–1238.
Wang, Z., Zhang, S., Liu, C., Yao, Y., Shi, J., Zhang, J., et al. (2019). A study of neurite orientation dispersion and density imaging in ischemic stroke. Magnet. Reson. Imaging 57, 28–33. doi: 10.1016/j.mri.2018.10.018
Wen, Q., Kelley, D.a.C, Banerjee, S., Lupo, J. M., Chang, S. M., Xu, D., et al. (2015). Clinically feasible NODDI characterization of glioma using multiband EPI at 7 T. NeuroImage Clin. 9, 291–299. doi: 10.1016/j.nicl.2015.08.017
Ye, Z., Gary, S. E., Sun, P., Mustafi, S. M., Glenn, G. R., Yeh, F. C., et al. (2020). The impact of edema and fiber crossing on diffusion MRI metrics assessed in an ex vivo nerve phantom: multi-tensor model vs. diffusion orientation distribution function. NMR Biomed. 34:e4414.
Yeh, F.-C., Verstynen, T. D., Wang, Y., Fernández-Miranda, J. C., and Tseng, W.-Y. I. (2013). Deterministic diffusion fiber tracking improved by quantitative anisotropy. PLoS One 8:e80713. doi: 10.1371/journal.pone.0080713
Yu, Q., Lin, K., Liu, Y., and Li, X. (2020). Clinical uses of diffusion tensor imaging fiber tracking merged neuronavigation with lesions adjacent to corticospinal tract : a retrospective cohort study. J. Korean Neurosurg. Soc. 63, 248–260. doi: 10.3340/jkns.2019.0046
Yushkevich, P. A., Piven, J., Hazlett, H. C., Smith, R. G., Ho, S., Gee, J. C., et al. (2006). User-guided 3D active contour segmentation of anatomical structures: significantly improved efficiency and reliability. NeuroImage 31, 1116–1128. doi: 10.1016/j.neuroimage.2006.01.015
Zhang, H., Schneider, T., Wheeler-Kingshott, C. A., and Alexander, D. C. (2012). NODDI: Practical in vivo neurite orientation dispersion and density imaging of the human brain. NeuroImage 61, 1000–1016. doi: 10.1016/j.neuroimage.2012.03.072
Zhang, H., Wang, Y., Lu, T., Qiu, B., Tang, Y., Ou, S., et al. (2013). Differences between generalized q-sampling imaging and diffusion tensor imaging in the preoperative visualization of the nerve fiber tracts within peritumoral edema in brain. Neurosurgery 73, 1044–1053.
Keywords: neurite orientation dispersion and density imaging, diffusion tensor imaging, fiber tractography, vasogenic edema, brain tumor, neurosurgery
Citation: Chong ST, Liu X, Kao H-W, Lin C-YE, Hsu C-CH, Kung Y-C, Kuo K-T, Huang C-C, Lo C-YZ, Li Y, Zhao G and Lin C-P (2021) Exploring Peritumoral Neural Tracts by Using Neurite Orientation Dispersion and Density Imaging. Front. Neurosci. 15:702353. doi: 10.3389/fnins.2021.702353
Received: 29 April 2021; Accepted: 17 August 2021;
Published: 27 September 2021.
Edited by:
Nico Sollmann, University of California, San Francisco, United StatesReviewed by:
Andrey Zhylka, Eindhoven University of Technology, NetherlandsSandro M. Krieg, Technical University of Munich, Germany
Giuseppe Barisano, University of Southern California, United States
Copyright © 2021 Chong, Liu, Kao, Lin, Hsu, Kung, Kuo, Huang, Lo, Li, Zhao and Lin. This is an open-access article distributed under the terms of the Creative Commons Attribution License (CC BY). The use, distribution or reproduction in other forums is permitted, provided the original author(s) and the copyright owner(s) are credited and that the original publication in this journal is cited, in accordance with accepted academic practice. No use, distribution or reproduction is permitted which does not comply with these terms.
*Correspondence: Ching-Po Lin, chingpolin@gmail.com; Hung-Wen Kao, cohimeow@gmail.com