- 1Graduate School of Health Sciences, Kyoto Tachibana University, Kyoto, Japan
- 2Department of Rehabilitation, Kyoto Kuno Hospital, Kyoto, Japan
- 3Department of Physical Therapy, Faculty of Health Sciences, Kyoto Tachibana University, Kyoto, Japan
- 4Department of Rehabilitation, Tesseikai Neurosurgical Hospital, Shijonawate, Japan
- 5Graduate School of Environment and Information Sciences, Yokohama National University, Yokohama, Japan
Introduction: Neurofeedback (NFB) training and transcranial direct current stimulation (tDCS) have been shown to individually improve motor imagery (MI) abilities. However, the effect of combining both of them with MI has not been verified. Therefore, the aim of this study was to examine the effect of applying tDCS directly before MI with NFB.
Methods: Participants were divided into an NFB group (n = 10) that performed MI with NFB and an NFB + tDCS group (n = 10) that received tDCS for 10 min before MI with NFB. Both groups performed 60 MI trials with NFB. The MI task was performed 20 times without NFB before and after training, and μ-event-related desynchronization (ERD) and vividness MI were evaluated.
Results: μ-ERD increased significantly in the NFB + tDCS group compared to the NFB group. MI vividness significantly increased before and after training.
Discussion: Transcranial direct current stimulation and NFB modulate different processes with respect to MI ability improvement; hence, their combination might further improve MI performance. The results of this study indicate that the combination of NFB and tDCS for MI is more effective in improving MI abilities than applying them individually.
1. Introduction
Motor imagery (MI) is the cognitive process of imagining the execution of a movement without actually moving (Lotze and Halsband, 2006; Olsson and Nyberg, 2010); it can improve skill (Ladda et al., 2021) and muscle strength (Yue and Cole, 1992) despite the lack of movement. Therefore, it is used in rehabilitation and sports training (MacIntyre et al., 2018). It has been shown that MI shares some of the neural activities with actual motor execution (Lotze et al., 1999; Hanakawa et al., 2003; Nakano et al., 2012), which likely supports the effectiveness of MI. Particularly, MI involves abundant neural activity (Ruffino et al., 2016); therefore, it promotes neuroplasticity and is used in the rehabilitation of neurological diseases such as stroke (Guerra et al., 2017) and Parkinson’s disease (Tinaz et al., 2022). Further, it improves upper limb function in patients who have had stroke (Villa-Berges et al., 2023) and reduces bradykinesia in patients with Parkinson’s disease (Tamir et al., 2007). Specifically, upper limb function is less likely to improve compared with lower limb function in patients who have had stroke (Liu et al., 2012), and MI may be an effective novel intervention method. However, as MI is a purely mental process, it does not provide any feedback (Bai et al., 2014); thus, its effectiveness varies among individuals (van der Meulen et al., 2014). This issue can be overcome by using neurofeedback (NFB) (Hwang et al., 2009; Yu et al., 2015; Nakano et al., 2018). NFB is a technique for improving performance by feeding back brain activity recorded during task execution to the participants (Hampson et al., 2020). In particular, closed-loop adaptations of NFB are used for MI; they promote the active regulation of brain activity (Al-Wasity et al., 2021; Grigorev et al., 2021) by providing brain activity (Sitaram et al., 2019) and performance feedback (Riahi et al., 2022). Thus, positive effects of NFB on MI have been reported. Furthermore, NFB improves MI in stroke (Dettmers et al., 2016) and Parkinson’s disease (Tinaz et al., 2018, 2022), and may also address the problem of MI in patients with neurological diseases. Neurological diseases, such as stroke, decrease MI ability (Dettmers et al., 2012; Braun et al., 2017); thus, NFB for MI may be an effective novel treatment method.
On the other hand, transcranial direct current stimulation (tDCS), a method to directly modify brain states, has attracted increasing attention. tDCS is a reversible, focal, non-invasive brain stimulation technique that modulates neuroplasticity by applying a direct current to the head via electrodes (Nitsche and Paulus, 2001). In neurons, it changes the resting membrane potential toward depolarization or hyperpolarization, depending on the direction of the current flow relative to the direction of the axons (Lefaucheur et al., 2017). Further, it has been shown that anodal tDCS increases cortical excitability immediately below the electrode, whereas cathodal tDCS decreases cortical excitability (Nitsche and Paulus, 2000). These neural effects affect exercise performance, motor learning (Reis and Fritsch, 2011; Buch et al., 2017), and cognitive function (Pope et al., 2015; Imburgio and Orr, 2018). In addition, tDCS alters various symptoms, including attention function in children with paralysis (Alharbi et al., 2021), mental status in patients with depression (Palm et al., 2016), and pain intensity in patients with chronic pain (Pacheco-Barrios et al., 2020). tDCS has also been used to treat neurological diseases because it modulates neuroplasticity (Di Pino et al., 2014). By directly modulating brain states, it improves motor functions, such as gait (Chieffo et al., 2016) and upper limb function (Pires et al., 2023).
Recently, the effects of the combination of MI and tDCS have been investigated. In healthy young adults, the combination of MI and tDCS enhances event-related desynchronization (ERD) in the primary motor cortex (M1) (Wei et al., 2013; Xie et al., 2021) and improves connectivity in the sensorimotor cortex (Baxter et al., 2017). These changes in brain states improve MI performance (Moghadas Tabrizi et al., 2019). In addition, in stroke patients, the combination of MI and tDCS promotes neuroplasticity (Hong et al., 2017) and improves the Fugl-Meyer Assessment score (Chew et al., 2020; Kashoo et al., 2022). While it has been reported that the combinations of MI and NFB as well as MI and tDCS improve MI performance, the effect of combining both NFB and tDCS with MI has not yet been verified, although this combination may provide a new approach to maximizing MI performance.
Therefore, this study aimed to investigate the combined effect of tDCS and NFB in MI tasks in healthy young adults.
2. Materials and methods
2.1. Participants
Twenty healthy young men (age: 20.20 ± 0.70 years, height: 172.65 ± 6.77 cm, and body weight: 63.00 ± 8.52 kg) without a history of motor or cognitive dysfunction and metal implants in the head were recruited for this study. Supplementary Table 1 summarizes their demographic data. The Edinburgh Handedness Inventory (Oldfield, 1971) was used to confirm that all participants were right-handed.
This study was conducted in accordance with the Helsinki Declaration, and informed consent was obtained from all participants. Additionally, this research was approved by the Research Ethics Committee of Kyoto Tachibana University (approval no. 21-47).
2.2. Sample size calculation
The sample size was determined as 16 using the G*Power software (Faul et al., 2007) by considering an effect size as “medium” at 0.40 (Cohen, 1998), α = 0.05, and power (1-β) = 0.80 at 95% confidence level.
2.3. Study protocol
This study was a randomized controlled trial. First, in the pre-evaluation phase, all participants performed the MI task 20 times without NFB. Second, for the training phase, they were randomly divided into two groups as follows: (i) NFB group (n = 10, age: 20.40 ± 0.70 years, height: 173.70 ± 4.50 cm, and body weight: 64.40 ± 5.42 kg) that performed 60 MI tasks with NFB and (ii) tDCS + NFB group (n = 10, age: 20.00 ± 0.67 years, height: 171.60 ± 8.60 cm, and body weight: 61.60 ± 10.93 kg) that performed 60 MI tasks with NFB after receiving tDCS for 10 min (Yamaguchi et al., 2020). Finally, in the post-evaluation phase, all participants performed the MI task without NFB 20 times again. The self-reported MI vividness was assessed during the pre- and post-evaluation phases. Additionally, EEG was recorded during the MI tasks and ERD was evaluated during the pre-evaluation, training, and post-evaluation phases (Figure 1).
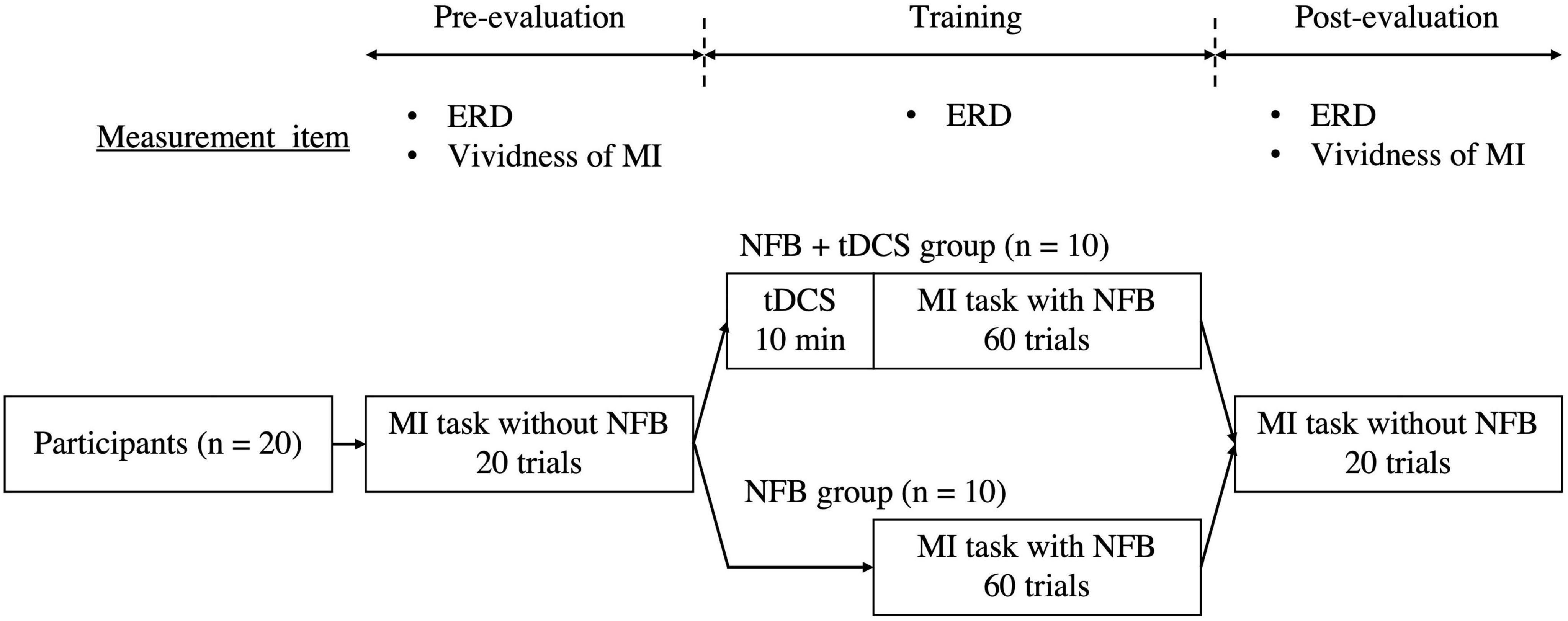
Figure 1. Study protocol. Participants were divided into an NFB group (n = 10) performing the MI task with NFB alone and a tDCS + NFB group (n = 10) performing the MI task with NFB after 10 min of tDCS. ERD and MI vividness were evaluated before and after the training phase. In the training phase, ERD during the MI task was also evaluated. MI, motor imagery; NFB, neurofeedback; tDCS, transcranial direct current stimulation; ERD, event-related desynchronization.
2.4. Motor imagery
During the training phase, a bar graph was displayed on a monitor to provide real-time feedback based on the ERD (Figure 2). Participants were asked to imagine dorsiflexion of the left wrist joint at 100% maximum voluntary contraction strength from a first-person perspective. The imagery task consisted of a resting period (5 s) followed by an imagery period (5 s), and participants repeated the imagery task 20 times during the pre- and post-evaluation phases. In the training phase, three sets of 20 imagery tasks were performed. Several MI studies consisted of short repetitions of the imagery and rest periods (Xie et al., 2021; Sawai et al., 2022); we followed a similar protocol. The degree of MI improves with its repetition (Pineda et al., 2003); therefore, few trials may erase the effect of tDCS. Conversely, numerous trials can cause mental fatigue, which may reduce the degree of MI (Nakashima et al., 2021). Therefore, we set the number of trials at 60, which has been used in previous studies (Maghsoudi and Shalbaf, 2022; Sawai et al., 2022).
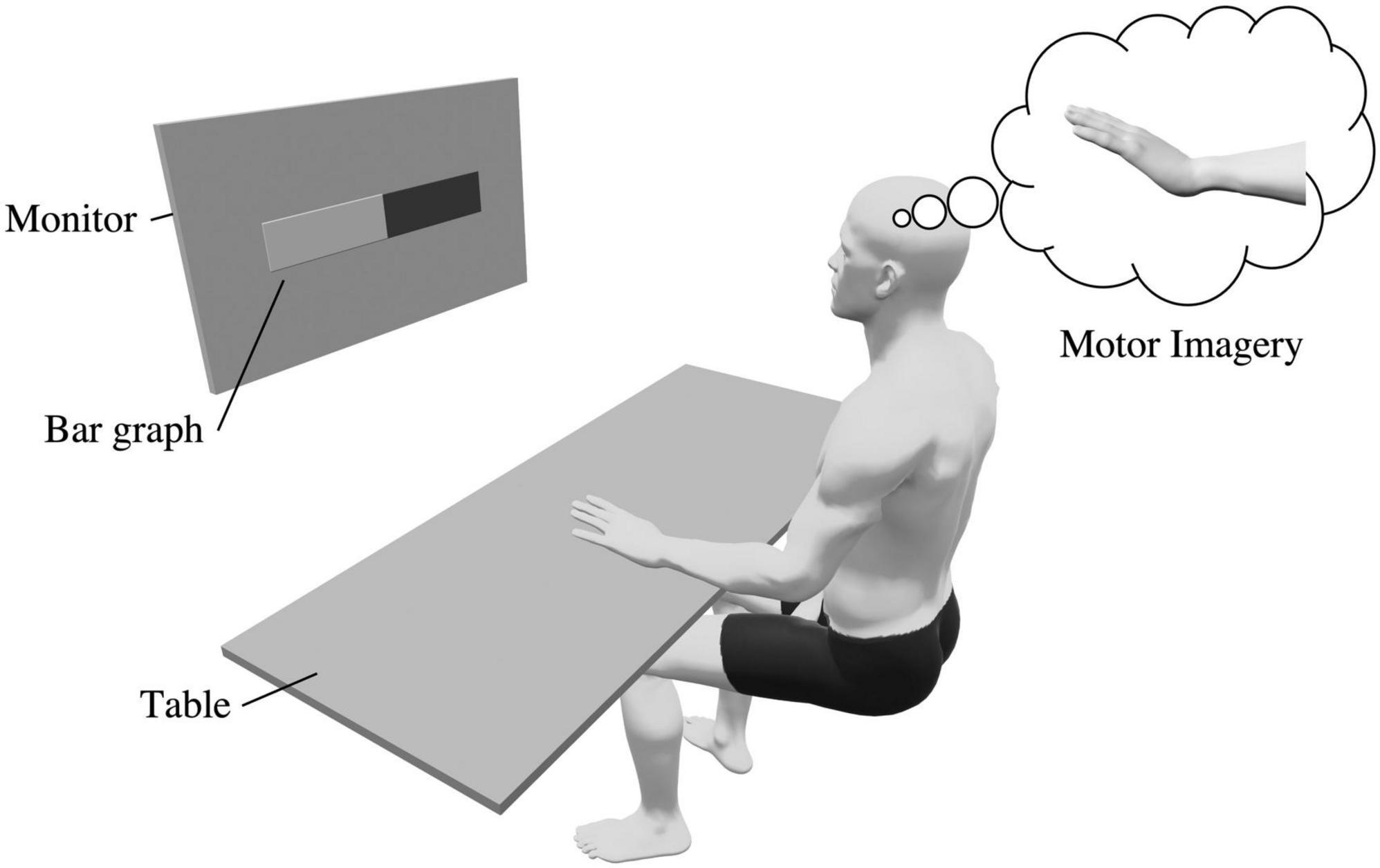
Figure 2. Experimental setup. Participants were seated in a chair, placed their left hand on a desk, and imagined a kinesthetic wrist dorsiflexion movement. During the training phase, a bar graph was displayed on the monitor and the ERD was fed back to the participant.
2.5. Neurofeedback
In the training phase, EEG was recorded during MI and ERD values were fed back to the participants in real-time using an electroencephalograph (EEG-9100; Nihon Kohden Corp., Tokyo, Japan) and an active dry electrodes system (Miyuki Giken, Co., Ltd., Tokyo, Japan). EEG was recorded from 19 channel locations (Fp1, Fp2, F7, F3, Fz, F4, F8, T3, C3, Cz, C4, T4, T5, P3, Pz, P4, T6, O1, and O2) according to the international 10–20 system, and reference electrodes were attached to both earlobes. The sampling rate was set at 1,000 Hz. The EEG data were analyzed using Microsoft Visual Studio (Microsoft Corp., Redmond, WA, USA), and the ERD in the μ-wave band (8–13 Hz) of the sensorimotor-related region (C4) was calculated using frequency analysis. ERD in the μ-wave band of C4 is associated with MI (Pineda et al., 2003; Enomae et al., 2017). The ERD E(t) at a given time t was calculated using the following equation using the resting μ-wave activity Rrest and the μ-wave activity Rimage(t) during the imagery task (Pfurtscheller and Lopes da Silva, 1999):
Here, ERD indicates a decrease in μ-wave activity during MI compared to the resting state. Therefore, E(t) can take values from -∞ to 1 with ERD occurring between 0 and 1.
The ERD feedback, computed using the aforementioned methodology, was visually presented to the participants as a bar graph (Figure 2; Ono et al., 2013). The bar graph changed in real time as the ERD increased or decreased. During the training phase, participants were instructed to imagine the wrist dorsiflexion movement so that the ERD bar displayed on the monitor would increase. The NFB procedure is described in the Supplementary Text and Supplementary Figure 1.
2.6. tDCS
In the tDCS + NFB group, we applied tDCS for 10 min before the MI task in the training phase. We used a battery-powered stimulator (DC-STIMULATOR PLUS; neuroConn GmbH, Ilmenau, Germany) and a pair of sponge surface electrodes immersed in 0.9% NaCl saline. tDCS increases EEG activity and corticospinal tract excitability related to MI (Ang et al., 2015; Kaneko et al., 2016), thus, ensuring its reliability and validity. The stimulation intensity was set to 2.0 mA and the ramp time to 15 s. The anode electrode (25 cm2, stimulation intensity; 2.0 mA) was placed over the right primary motor cortex and the cathode electrode (50 cm2, stimulation intensity; −2.0 mA) was placed on the right upper arm (Figure 3; Yamaguchi et al., 2020). Generally, greater stimulation intensity induces greater changes in the electric field (Bikson et al., 2015). However, guidelines for tDCS use indicate that tDCS with an intensity of up to 2.0 mA is safe for humans (Antal et al., 2017). Therefore, we set the stimulation intensity at 2.0 mA, which is safe and maximally effective. In an electric field modeling study, a tDCS of 2.0 mA at M1 produced an electric field change of 0.369 V/m (Evans et al., 2020); a similar electric field change likely occurred in this study. The current density was 0.08 mA/cm2 and the total surface charge density was 0.048 C/cm2, which is below the threshold for tissue damage (Nitsche et al., 2003).
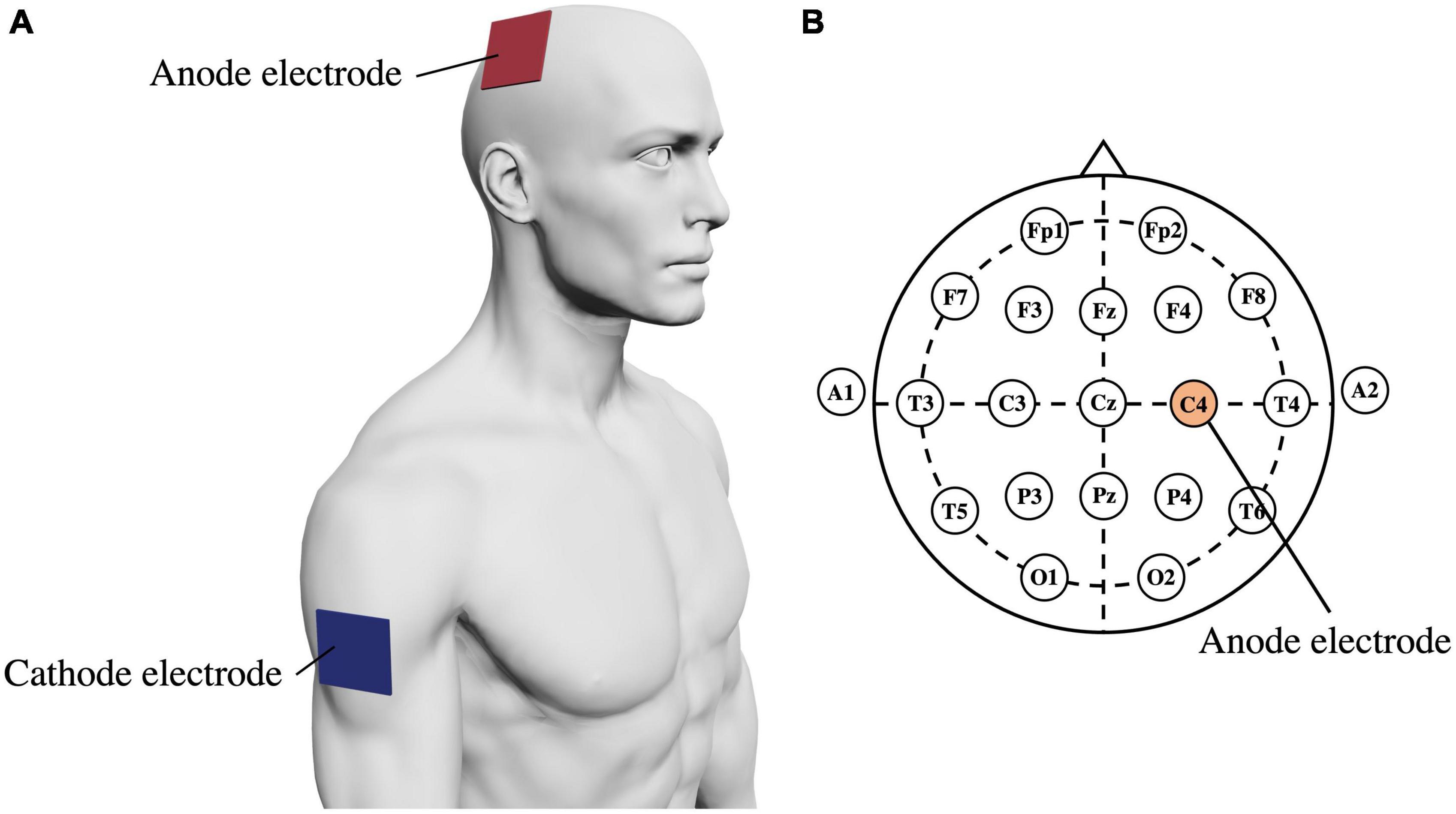
Figure 3. (A) Electrode placement for tDCS. The anode electrode is placed over the right primary motor cortex, and the cathode electrode is placed on the right upper arm. (B) Electrode placement under the international 10–20 system. The anode electrode is placed on C4.
2.7. Evaluation
During both the pre- and post-evaluation phases, as well as the training phase, the mean of the maximum values in each trial was used to evaluate the ERD. In addition, the subjective MI vividness was evaluated using the Visual Analog Scale (VAS) during the pre- and post-evaluation phases. Participants rated the vividness by making a mark on a 100-mm horizontal line, where 0 equaled “not at all” and 100 equaled “very vivid image.” MI vividness correlates with cortical excitability (Moriuchi et al., 2020) and has been used in MI studies involving healthy participants as a simple and subjective MI assessment (Iso et al., 2021; Sawai et al., 2022).
2.8. Statistical analysis
First, the normality of all data was examined using the Shapiro–Wilk test. Because a non-normal distribution was detected, unpaired t-tests were performed to compare the demographic data of age, height, and weight between groups. Additionally, the Mann–Whitney U-test was used to compare ERD values during training between groups. We also compared VAS and ERD values using a two-factor analysis of variance with the factors group (tDCS + NFB group, NFB group) and time (pre, post). The Bonferroni’s method was used for post-hoc tests. SPSS ver. 28.0 (IBM Corp., Armonk, NY, USA) was used for all statistical analyses. The statistical significance level was set at p < 0.05.
3. Results
Demographic data, including age, height, and weight, did not differ significantly between the groups (p > 0.05, Supplementary Table 1). ERD during the training phase was significantly higher in the tDCS + NFB group than in the NFB group (p < 0.05; Figure 4A). The two-factor analysis of variance for ERD during the evaluation phases revealed a significant interaction between the group and time factors (F = 5.16, p < 0.05). Post-hoc test results showed that ERD in the tDCS + NFB and NFB groups increased significantly before and after training (p < 0.05), and that ERD in the post-evaluation phase was significantly higher in the tDCS + NFB group than in the NFB group (p < 0.05; Figure 4B). Finally, the two-factor analysis of variance for the VAS revealed a significant main effect of time, with significantly increased values after training (F = 16.90, p < 0.05; Figure 4C).
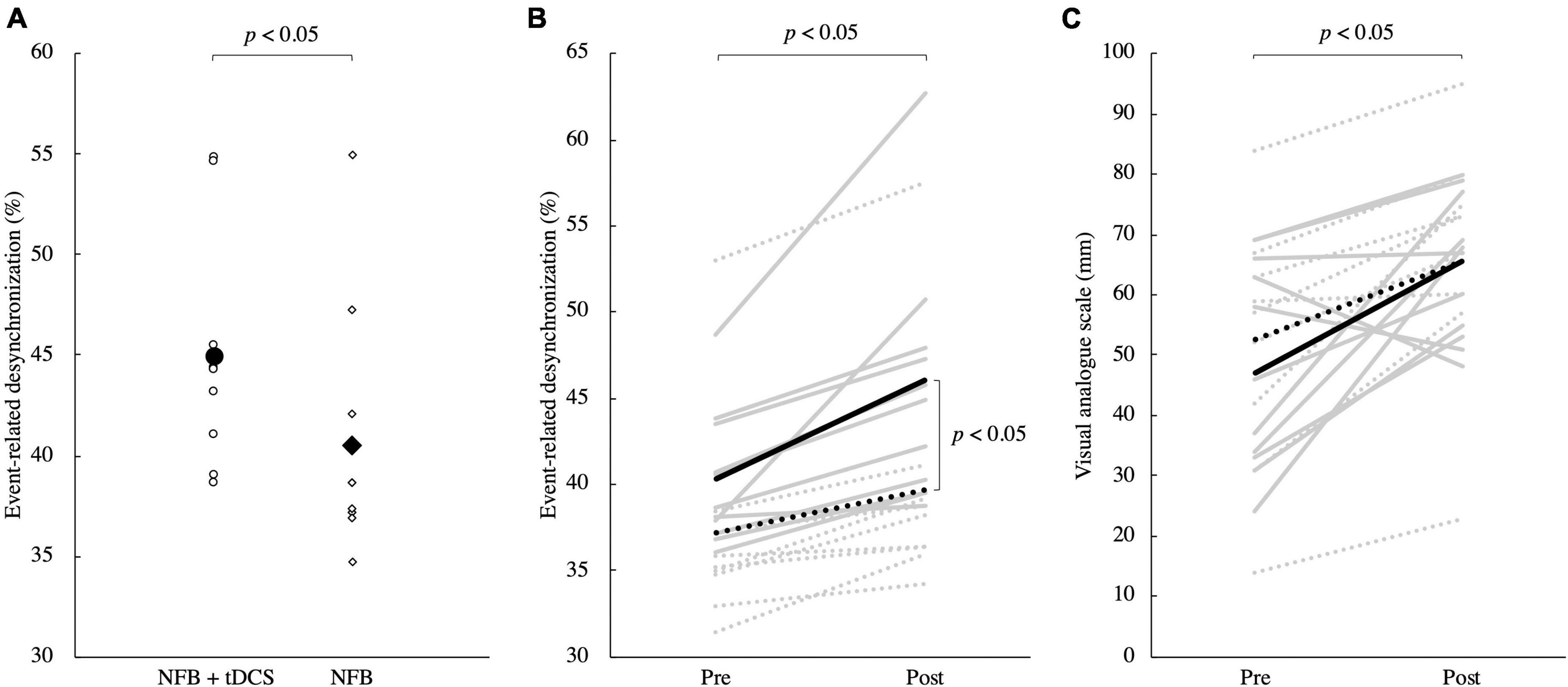
Figure 4. (A) ERD in the training phase. Round markers indicate the NFB + tDCS group, and rhombic markers indicate the NFB group. The NFB + tDCS group had significantly higher ERD during MI in the training phase than the NFB group (p < 0.05). (B) ERD changes between pre- and post-evaluation. The solid line shows the data for the NFB + tDCS group, and the dotted line shows the data for the NFB group. The gray line shows the data for each participant and the black line shows the mean for each group. There was a significant interaction between group and time, with a significant increase in ERD in the NFB + tDCS group compared to that in the NFB group (p < 0.05). Furthermore, ERD after training was significantly higher in the NFB + tDCS group than in the NFB group (p < 0.05). (C) Changes in VAS scores. The solid line shows the data for the NFB + tDCS group, and the dotted line shows the data for the NFB group. VAS increased after training in both groups (p < 0.05). MI, motor imagery; NFB, neurofeedback; tDCS, transcranial direct current stimulation; ERD, event-related desynchronization; VAS, visual analog scale.
4. Discussion
This study aimed to investigate the combined effect of tDCS and NFB in MI tasks in healthy young adults. The results showed that the ERD in the NFB + tDCS group during the training phase was significantly higher than that of the NFB group. Additionally, the ERD of the NFB + tDCS group was significantly higher than that of the NFB group in the pre- and post-training phases. Furthermore, the MI vividness was significantly increased before and after training. These results indicate that the combination of NFB and tDCS improves performance in NFB-assisted MI tasks.
The μ-ERD in the training phase of the NFB + tDCS group was significantly higher than that of the NFB group. The μ-ERD in the motor cortex measured in this study indicates the degree of MI (Wei et al., 2013; Kasuga et al., 2015; Xie et al., 2021), suggesting that the addition of tDCS to an NFB-assisted MI task can maximize MI performance. μ-ERD is stronger when MI is successful (Chen et al., 2021), and NFB training provides feedback on the μ-ERD to promote active control, which improves μ-ERD and MI performance. Additionally, tDCS has been shown to increase the probability of neurons firing in response to MI-related input signals (Matsumoto et al., 2010), which in turn increases neuronal depolarization and desynchronization, and enhances μ-ERD. These findings suggest that NFB increases the input signal to the motor cortex via MI, and tDCS may further amplify this signal. Therefore, it is likely that the NFB and tDCS mutually contribute to the neural MI activity, resulting in an increased μ-ERD in the training phase of the NFB + tDCS group.
The μ-ERD in the NFB + tDCS group was significantly increased after training compared to that in the NFB group. In the pre- and post-evaluation phases, NFB was not provided in both groups. Therefore, these results suggest that the combination of NFB and tDCS increases the learning efficacy in the post-evaluation phase. NFB has been reported to promote neuroplasticity, resulting in long-term potentiation (LTP) and long-term depression (LTD) of neural networks (Mrachacz-Kersting et al., 2021). Specific neural networks are then modified to become preferential signal input destinations (Yger et al., 2015). Through these actions, NFB facilitates learning. It has also been shown that tDCS, similar to NFB, promotes neuroplasticity and enhances learning (Monte-Silva et al., 2013; Rroji et al., 2015; Barbati et al., 2022). However, tDCS produces a change in electrical polarity that is considerably lower than the potential changes required for depolarization as required for LTP in NFB. Therefore, tDCS promotes its learning effect when activity-dependent LTP is occurring (Fritsch et al., 2010). In this study, μ-ERD after training was significantly improved in the NFB + tDCS group compared to that in the NFB group, which suggests that NFB increased the activity of the neural network related to MI, and tDCS may have reinforced this effect.
On the other hand, the vividness of MI significantly increased in both the NFB and NFB + tDCS groups after training. However, there was no significant difference between the two groups, which indicates that the impact of combining NFB and tDCS on subjective MI evaluation is minimal. Repeated MI improves the vividness of MI (Iso et al., 2021). Nevertheless, studies of MI with simultaneous action observation (Ono et al., 2013) and repetitive peripheral magnetic stimulation (Sawai et al., 2022) reported no difference in the improvement of MI vividness between groups compared to that in MI alone. Similarly, in this study, there was no difference between the NFB and the NFB + tDCS groups. Therefore, the effect of repeated MI on the improvement of MI vividness may be substantial, while the effect of NFB and tDCS on the improvement of MI vividness may be minimal.
There are several limitations to this study: first, the tDCS electrodes used in this study were relatively large (25 cm2); thus, we cannot rule out the possibility that other areas adjacent to the motor cortex were affected. Future studies may reveal the effects of tDCS using more targeted stimulation methods. Second, in this study, the task was to imagine a relatively simple dorsiflexion movement of the wrist joint. While μ-ERD was improved in the NFB + tDCS group compared to that in the NFB group, it has been reported that ceiling effects occur in simple tasks, which reduce the effects of tDCS (Furuya et al., 2014). Therefore, the effects of tDCS may be augmented by using more elaborate MI tasks. Third, no group solely received applied tDCS. Therefore, it is unclear whether this result was attributed to the combination of tDCS and NFB or tDCS alone. Fourth, our sample size was small. Therefore, this study is positioned as a feasibility study because of the limited generalizability.
In conclusion, the results of this study indicate that NFB + tDCS increases neural activity not only during, but also after training, and improves MI performance. For immediate MI performance, NFB increased the signal input to the motor cortex, and tDCS increased the firing probability of neurons in response to the signal input. Regarding the learning effect of MI, NFB increases neural activity that produces LTP, and tDCS enhances LTP through subthreshold electrical polarity changes. Therefore, since NFB and tDCS modulate different processes during MI tasks, the concurrent use of these two methods may have augmented MI performance. The present study suggests that this method can be widely applied as a new training technique to improve MI abilities. NFB exerts an inconsistent impact on MI training for the rehabilitation of stroke (Kern et al., 2022) and Parkinson’s disease (Tinaz et al., 2022). Therefore, our results may address this issue. Particularly, the application of our results to patients who have had stroke may help to resolve the lack of improvement in upper limb function (Liu et al., 2012). Further validation in neurological diseases, such as stroke and Parkinson’s disease, as well as in children with cerebral palsy (Behboodi et al., 2022), where the effects of NFB on MI is unclear, will provide more clinical results and advance neurorehabilitation.
Data availability statement
The raw data supporting the conclusions of this article will be made available by the authors, without undue reservation.
Ethics statement
The studies involving human participants were reviewed and approved by the Kyoto Tachibana University. The patients/participants provided their written informed consent to participate in this study.
Author contributions
SS and HN: conceptualization, formal analysis, data curation, writing—original draft preparation, and visualization. SS, SF, RY, and HN: methodology and validation. SS, SM, SF, RY, KS, and HN: investigation and writing—review and editing. SM, KS, and HN: resources. HN: supervision, project administration, and funding acquisition. All authors contributed to manuscript revision, read, and approved the submitted version.
Funding
This work was supported by the JSPS KAKENHI Grant Number: JP20K11173.
Acknowledgments
We thank all the volunteers who participated in this study. We also thank editage (http://www.editage.com) for editing and reviewing this manuscript for English language.
Conflict of interest
The authors declare that the research was conducted in the absence of any commercial or financial relationships that could be construed as a potential conflict of interest.
Publisher’s note
All claims expressed in this article are solely those of the authors and do not necessarily represent those of their affiliated organizations, or those of the publisher, the editors and the reviewers. Any product that may be evaluated in this article, or claim that may be made by its manufacturer, is not guaranteed or endorsed by the publisher.
Supplementary material
The Supplementary Material for this article can be found online at: https://www.frontiersin.org/articles/10.3389/fnins.2023.1148336/full#supplementary-material
References
Alharbi, R., Aloyuni, S., Kashoo, F., Waly, M., Singh, H., and Ahmad, M. (2021). Does transcranial direct current stimulation affect selective visual attention in children with left-sided infantile hemiplegia? A randomized, controlled pilot study. Brain Impair. 22, 152–164. doi: 10.1017/BrImp.2020.20
Al-Wasity, S., Vogt, S., Vuckovic, A., and Pollick, F. E. (2021). Upregulation of supplementary motor area activation with fMRI neurofeedback during motor imagery. eNeuro 8:ENEURO.0377-18.2020. doi: 10.1523/ENEURO.0377-18.2020
Ang, K. K., Guan, C., Phua, K. S., Wang, C., Zhao, L., Teo, W. P., et al. (2015). Facilitating effects of transcranial direct current stimulation on motor imagery brain-computer interface with robotic feedback for stroke rehabilitation. Arch. Phys. Med. Rehabil. 96, S79–S87. doi: 10.1016/j.apmr.2014.08.008
Antal, A., Alekseichuk, I., Bikson, M., Brockmöller, J., Brunoni, A. R., and Chen, R. (2017). Low intensity transcranial electric stimulation: safety, ethical, legal regulatory and application guidelines. Clin. Neurophysiol. 128, 1774–1809. doi: 10.1016/j.clinph.2017.06.001
Bai, O., Huang, D., Fei, D. Y., and Kunz, R. (2014). Effect of real-time cortical feedback in motor imagery-based mental practice training. NeuroRehabilitation. 34, 355–363. doi: 10.3233/NRE-131039
Barbati, S. A., Podda, M. V., and Grassi, C. (2022). Tuning brain networks: the emerging role of transcranial direct current stimulation on structural plasticity. Front. Cell. Neurosci. 16:945777. doi: 10.3389/fncel.2022.945777
Baxter, B. S., Edelman, B. J., Sohrabpour, A., and He, B. (2017). Anodal transcranial direct current stimulation increases bilateral directed brain connectivity during motor-imagery based brain-computer interface control. Front. Neurosci. 11:691. doi: 10.3389/fnins.2017.00691
Behboodi, A., Lee, W. A., Hinchberger, V. S., and Damiano, D. L. (2022). Determining optimal mobile neurofeedback methods for motor neurorehabilitation in children and adults with non-progressive neurological disorders: a scoping review. J. Neuroeng. Rehabil. 19:104. doi: 10.1186/s12984-022-01081-9
Bikson, M., Truong, D. Q., Mourdoukoutas, A. P., Aboseria, M., Khadka, N., Adair, D., et al. (2015). Modeling sequence and quasi-uniform assumption in computational neurostimulation. Prog. Brain Res. 222, 1–23. doi: 10.1016/bs.pbr.2015.08.005
Braun, N., Kranczioch, C., Liepert, J., Dettmers, C., Zich, C., Büsching, I., et al. (2017). Motor imagery impairment in postacute stroke patients. Neural Plast. 2017:4653256. doi: 10.1155/2017/4653256
Buch, E. R., Santarnecchi, E., Antal, A., Born, J., Celnik, P. A., Classen, J., et al. (2017). Effects of tDCS on motor learning and memory formation: a consensus and critical position paper. Clin. Neurophysiol. 128, 589–603. doi: 10.1016/j.clinph.2017.01.004
Chen, Y. Y., Lambert, K. J. M., Madan, C. R., and Singhal, A. (2021). Mu oscillations and motor imagery performance: a reflection of intra-individual success, not inter-individual ability. Hum. Mov. Sci. 78:102819. doi: 10.1016/j.humov.2021.102819
Chew, E., Teo, W. P., Tang, N., Ang, K. K., Ng, Y. S., Zhou, J. H., et al. (2020). Corrigendum: using transcranial direct current stimulation to augment the effect of motor imagery-assisted brain-computer interface training in chronic stroke patients-cortical reorganization considerations. Front. Neurol. 11:605141. doi: 10.3389/fneur.2020.605141
Chieffo, R., Comi, G., and Leocani, L. (2016). Noninvasive neuromodulation in poststroke gait disorders: rationale, feasibility, and state of the art. Neurorehabil. Neural Repair. 30, 71–82. doi: 10.1177/1545968315586464
Cohen, J. (1998). Statistical Power Analysis for the Behavioral Sciences, 2nd Edn. New York, NY: Routledge.
Dettmers, C., Benz, M., Liepert, J., and Rockstroh, B. (2012). Motor imagery in stroke patients, or plegic patients with spinal cord or peripheral diseases. Acta Neurol. Scand. 126, 238–247. doi: 10.1111/j.1600-0404.2012.01680.x
Dettmers, C., Braun, N., Büsching, I., Hassa, T., Debener, S., and Liepert, J. (2016). Neurofeedback-based motor imagery training for rehabilitation after stroke. Nervenarzt 87, 1074–1081. doi: 10.1007/s00115-016-0185-y
Di Pino, G., Pellegrino, G., Assenza, G., Capone, F., Ferreri, F., Formica, D., et al. (2014). Modulation of brain plasticity in stroke: a novel model for neurorehabilitation. Nat. Rev. Neurol. 10, 597–608. doi: 10.1038/nrneurol.2014.162
Enomae, T., Fujimori, H., and Tanaka, H. (2017). Analysis of the switching information on motor imagery for asynchronous BCI. Int. J. Affect. Eng. 16, 131–137. doi: 10.5057/ijae.IJAE-D-16-00019
Evans, C., Bachmann, C., Lee, J. S. A., Gregoriou, E., Ward, N., and Bestmann, S. (2020). Dose-controlled tDCS reduces electric field intensity variability at a cortical target site. Brain Stimul. 13, 125–136. doi: 10.1016/j.brs.2019.10.004
Faul, F., Erdfelder, E., Lang, A. G., and Buchner, A. (2007). G*Power 3: a flexible statistical power analysis program for the social, behavioral, and biomedical sciences. Behav. Res. Methods. 39, 175–191. doi: 10.3758/bf03193146
Fritsch, B., Reis, J., Martinowich, K., Schambra, H. M., Ji, Y., Cohen, L. G., et al. (2010). Direct current stimulation promotes BDNF-dependent synaptic plasticity: potential implications for motor learning. Neuron 66, 198–204. doi: 10.1016/j.neuron.2010.03.035
Furuya, S., Klaus, M., Nitsche, M. A., Paulus, W., and Altenmüller, E. (2014). Ceiling effects prevent further improvement of transcranial stimulation in skilled musicians. J. Neurosci. 34, 13834–13839. doi: 10.1523/JNEUROSCI.1170-14.2014
Grigorev, N. A., Savosenkov, A. O., Lukoyanov, M. V., Udoratina, A., Shusharina, N. N., Kaplan, A. Y., et al. (2021). A BCI-based vibrotactile neurofeedback training improves motor cortical excitability during motor imagery. IEEE Trans. Neural Syst. Rehabil. Eng. 29, 1583–1592. doi: 10.1109/TNSRE.2021.3102304
Guerra, Z. F., Lucchetti, A. L. G., and Lucchetti, G. (2017). Motor imagery training after stroke: a systematic review and meta-analysis of randomized controlled trials. J. Neurol. Phys. Ther. 41, 205–214. doi: 10.1097/NPT.0000000000000200
Hampson, M., Ruiz, S., and Ushiba, J. (2020). Neurofeedback. Neuroimage 218:116473. doi: 10.1016/j.neuroimage.2019.116473
Hanakawa, T., Immisch, I., Toma, K., Dimyan, M. A., Van Gelderen, P., and Hallett, M. (2003). Functional properties of brain areas associated with motor execution and imagery. J. Neurophysiol. 89, 989–1002. doi: 10.1152/jn.00132.2002
Hong, X., Lu, Z. K., Teh, I., Nasrallah, F. A., Teo, W. P., Ang, K. K., et al. (2017). Brain plasticity following MI-BCI training combined with tDCS in a randomized trial in chronic subcortical stroke subjects: a preliminary study. Sci. Rep. 7:9222. doi: 10.1038/s41598-017-08928-5
Hwang, H. J., Kwon, K., and Im, C. H. (2009). Neurofeedback-based motor imagery training for brain-computer interface (BCI). J. Neurosci. Methods 179, 150–156. doi: 10.1016/j.jneumeth.2009.01.015
Imburgio, M. J., and Orr, J. M. (2018). Effects of prefrontal tDCS on executive function: methodological considerations revealed by meta-analysis. Neuropsychologia 117, 156–166. doi: 10.1016/j.neuropsychologia.2018.04.022
Iso, N., Moriuchi, T., Fujiwara, K., Matsuo, M., Mitsunaga, W., Hasegawa, T., et al. (2021). Hemodynamic signal changes during motor imagery task performance are associated with the degree of motor task learning. Front. Hum. Neurosci. 15:603069. doi: 10.3389/fnhum.2021.603069
Kaneko, F., Shibata, E., Hayami, T., Nagahata, K., and Aoyama, T. (2016). The association of motor imagery and kinesthetic illusion prolongs the effect of transcranial direct current stimulation on corticospinal tract excitability. J. Neuroeng. Rehabil. 13, 1–8. doi: 10.1186/s12984-016-0143-8
Kashoo, F. Z., Al-Baradie, R. S., Alzahrani, M., Alanazi, A., Manzar, M. D., Gugnani, A., et al. (2022). Effect of transcranial direct current stimulation augmented with motor imagery and upper-limb functional training for upper-limb stroke rehabilitation: a prospective randomized controlled trial. Int. J. Environ. Res. Public Health 19:15199. doi: 10.3390/ijerph192215199
Kasuga, S., Matsushika, Y., Kasashima-Shindo, Y., Kamatani, D., Fujiwara, T., Liu, M., et al. (2015). Transcranial direct current stimulation enhances mu rhythm desynchronization during motor imagery that depends on handedness. Laterality 20, 453–468. doi: 10.1080/1357650X.2014.998679
Kern, K., Vukelić, M., Guggenberger, R., and Gharabaghi, A. (2022). Oscillatory neurofeedback networks and poststroke rehabilitative potential in severely impaired stroke patients. Neuroimage Clin. 37:103289. doi: 10.1016/j.nicl.2022.103289
Ladda, A. M., Lebon, F., and Lotze, M. (2021). Using motor imagery practice for improving motor performance - A review. Brain Cogn. 150:105705. doi: 10.1016/j.bandc.2021.105705
Lefaucheur, J. P., Antal, A., Ayache, S. S., Benninger, D. H., Brunelin, J., Cogiamanian, F., et al. (2017). Evidence-based guidelines on the therapeutic use of transcranial direct current stimulation (tDCS). Clin. Neurophysiol. 128, 56–92. doi: 10.1016/j.clinph.2016.10.087
Liu, M., Fujiwara, T., Shindo, K., Kasashima, K., Otaka, Y., and Tsuji, T. (2012). Newer challenges to restore hemiparetic upper extremity after stroke: HANDS therapy and BMI neurorehabilitation. Hong Kong Physiother J. 30, 83–92. doi: 10.1016/j.hkpj.2012.05.001
Lotze, M., and Halsband, U. (2006). Motor imagery. J. Physiol. Paris. 99, 386–395. doi: 10.1016/j.jphysparis.2006.03.012
Lotze, M., Montoya, P., Erb, M., Hülsmann, E., Flor, H., Klose, U., et al. (1999). Activation of cortical and cerebellar motor areas during executed and imagined hand movements: an fMRI study. J. Cogn. Neurosci. 11, 491–501. doi: 10.1162/089892999563553
MacIntyre, T. E., Madan, C. R., Moran, A. P., Collet, C., and Guillot, A. (2018). Motor imagery, performance and motor rehabilitation. Prog. Brain Res. 240, 141–159. doi: 10.1016/bs.pbr.2018.09.010
Maghsoudi, A., and Shalbaf, A. (2022). hand motor imagery classification using effective connectivity and hierarchical machine learning in EEG signals. J. Biomed. Phys. Eng. 12, 161–170. doi: 10.31661/jbpe.v0i0.1264
Matsumoto, J., Fujiwara, T., Takahashi, O., Liu, M., Kimura, A., and Ushiba, J. (2010). Modulation of mu rhythm desynchronization during motor imagery by transcranial direct current stimulation. J. Neuroeng. Rehabil. 7:27. doi: 10.1186/1743-0003-7-27
Moghadas Tabrizi, Y., Yavari, M., Shahrbanian, S., and Gharayagh Zandi, H. (2019). Transcranial direct current stimulation on prefrontal and parietal areas enhances motor imagery. NeuroReport 30, 653–657. doi: 10.1097/WNR.0000000000001253
Monte-Silva, K., Kuo, M. F., Hessenthaler, S., Fresnoza, S., Liebetanz, D., Paulus, W., et al. (2013). Induction of late LTP-like plasticity in the human motor cortex by repeated non-invasive brain stimulation. Brain Stimul. 6, 424–432. doi: 10.1016/j.brs.2012.04.011
Moriuchi, T., Nakashima, A., Nakamura, J., Anan, K., Nishi, K., Matsuo, T., et al. (2020). The vividness of motor imagery is correlated with corticospinal excitability during combined motor imagery and action observation. Front. Hum. Neurosci. 14:581652. doi: 10.3389/fnhum.2020.581652
Mrachacz-Kersting, N., Ibáñez, J., and Farina, D. (2021). Towards a mechanistic approach for the development of non-invasive brain-computer interfaces for motor rehabilitation. J. Physiol. 599, 2361–2374. doi: 10.1113/JP281314
Nakano, H., Kodama, T., Murata, S., Nakamoto, T., Fujihara, T., and Ito, Y. (2018). Effect of auditory neurofeedback training on upper extremity function and motor imagery ability in a stroke patient: a single case study. Int. J. Clin. Res Trials. 3:126. doi: 10.15344/2456-8007/2018/126
Nakano, H., Ueta, K., Osumi, M., and Morioka, S. (2012). Brain activity during the observation, imagery, and execution of tool use: an fNIRS/EEG study. J. Nov. Physiother. S1:9. doi: 10.4172/2165-7025.S1-009
Nakashima, A., Moriuchi, T., Matsuda, D., Hasegawa, T., Nakamura, J., Anan, K., et al. (2021). Corticospinal excitability during motor imagery is diminished by continuous repetition-induced fatigue. Neural Regen. Res. 16, 1031–1036. doi: 10.4103/1673-5374.300448
Nitsche, M. A., Liebetanz, D., Lang, N., Antal, A., Tergau, F., and Paulus, W. (2003). Safety criteria for transcranial direct current stimulation (tDCS) in humans. Clin. Neurophysiol. 114, 2220–2222. doi: 10.1016/s1388-2457(03)00235-9
Nitsche, M. A., and Paulus, W. (2000). Excitability changes induced in the human motor cortex by weak transcranial direct current stimulation. J. Physiol. 527(Pt. 3), 633–639. doi: 10.1111/j.1469-7793.2000.t01-1-00633.x
Nitsche, M. A., and Paulus, W. (2001). Sustained excitability elevations induced by transcranial DC motor cortex stimulation in humans. Neurology 57, 1899–1901. doi: 10.1212/wnl.57.10.1899
Oldfield, R. C. (1971). The assessment and analysis of handedness: the Edinburgh inventory. Neuropsychologia 9, 97–113. doi: 10.1016/0028-3932(71)90067-4
Olsson, C. J., and Nyberg, L. (2010). Motor imagery: if you can’t do it, you won’t think it. Scand. J. Med. Sci. Sports 20, 711–715. doi: 10.1111/j.1600-0838.2010.01101.x
Ono, T., Kimura, A., and Ushiba, J. (2013). Daily training with realistic visual feedback improves reproducibility of event-related desynchronisation following hand motor imagery. Clin. Neurophysiol. 124, 1779–1786. doi: 10.1016/j.clinph.2013.03.006
Pacheco-Barrios, K., Cardenas-Rojas, A., Thibaut, A., Costa, B., Ferreira, I., Caumo, W., et al. (2020). Methods and strategies of tDCS for the treatment of pain: current status and future directions. Expert Rev. Med. Devices 17, 879–898. doi: 10.1080/17434440.2020.1816168
Palm, U., Hasan, A., Strube, W., and Padberg, F. (2016). tDCS for the treatment of depression: a comprehensive review. Eur. Arch. Psychiatry Clin. Neurosci. 266, 681–694. doi: 10.1007/s00406-016-0674-9
Pfurtscheller, G., and Lopes da Silva, F. H. (1999). Event-related EEG/MEG synchronization and desynchronization: basic principles. Clin. Neurophysiol. 110, 1842–1857. doi: 10.1016/s1388-2457(99)00141-8
Pineda, J. A., Silverman, D. S., Vankov, A., and Hestenes, J. (2003). Learning to control brain rhythms: making a brain-computer interface possible. IEEE Trans. Neural Syst. Rehabil. 11, 181–184. doi: 10.1109/TNSRE.2003.814445
Pires, R., Baltar, A., Sanchez, M. P., Antonino, G. B., Brito, R., Berenguer-Rocha, M., et al. (2023). Do higher transcranial direct current stimulation doses lead to greater gains in upper limb motor function in post-stroke patients? Int. J. Environ. Res, Public Health 20:1279. doi: 10.3390/ijerph20021279
Pope, P. A., Brenton, J. W., and Miall, R. C. (2015). Task-specific facilitation of cognition by anodal transcranial direct current stimulation of the prefrontal cortex. Cereb. Cortex 25, 4551–4558. doi: 10.1093/cercor/bhv094
Reis, J., and Fritsch, B. (2011). Modulation of motor performance and motor learning by transcranial direct current stimulation. Curr. Opin. Neurol. 24, 590–596. doi: 10.1097/WCO.0b013e32834c3db0
Riahi, N., Ruth, W., D’Arcy, R. C. N., and Menon, C. (2022). A method for using neurofeedback to guide mental imagery for improving motor skill. IEEE Trans. Neural Syst. Rehabil. Eng. 31:99. doi: 10.1109/TNSRE.2022.3218514
Rroji, O., van Kuyck, K., Nuttin, B., and Wenderoth, N. (2015). Anodal tDCS over the primary motor cortex facilitates long-term memory formation reflecting use-dependent plasticity. PLoS One. 10:e0127270. doi: 10.1371/journal.pone.0127270
Ruffino, C., Papaxanthis, C., and Lebon, F. (2016). Neural plasticity during motor learning with motor imagery practice: review and perspectives. Neuroscience 341, 61–78. doi: 10.1016/j.neuroscience.2016.11.023
Sawai, S., Fujikawa, S., Ushio, R., Tamura, K., Ohsumi, C., Yamamoto, R., et al. (2022). Repetitive peripheral magnetic stimulation combined with motor imagery changes resting-state EEG activity: a randomized controlled trial. Brain Sci. 12:1548. doi: 10.3390/brainsci12111548
Sitaram, R., Ros, T., Stoeckel, L., Haller, S., Scharnowski, F., Lewis-Peacock, J., et al. (2019). Author correction: closed-loop brain training: the science of neurofeedback. Nat. Rev. Neurosci. 20:314. doi: 10.1038/s41583-019-0161-1
Tamir, R., Dickstein, R., and Huberman, M. (2007). Integration of motor imagery and physical practice in group treatment applied to subjects with Parkinson’s disease. Neurorehabil. Neural Repair. 21, 68–75. doi: 10.1177/1545968306292608
Tinaz, S., Kamel, S., Aravala, S. S., Elfil, M., Bayoumi, A., Patel, A., et al. (2022). Neurofeedback-guided kinesthetic motor imagery training in Parkinson’s disease: randomized trial. Neuroimage Clin. 34:102980. doi: 10.1016/j.nicl.2022.102980
Tinaz, S., Para, K., Vives-Rodriguez, A., Martinez-Kaigi, V., Nalamada, K., and Sezgin, M. (2018). Insula as the interface between body awareness and movement: a neurofeedback-guided kinesthetic motor imagery study in Parkinson’s disease. Front. Hum. Neurosci. 12:496. doi: 10.3389/fnhum.2018.00496
van der Meulen, M., Allali, G., Rieger, S. W., Assal, F., and Vuilleumier, P. (2014). The influence of individual motor imagery ability on cerebral recruitment during gait imagery. Hum. Brain Mapp. 35, 455–470. doi: 10.1002/hbm.22192
Villa-Berges, E., Laborda Soriano, A. A., Lucha-López, O., Tricas-Moreno, J. M., Hernández-Secorún, M., Gómez-Martínez, M., et al. (2023). Motor imagery and mental practice in the subacute and chronic phases in upper limb rehabilitation after stroke: a systematic review. Occup. Ther. Int. 2023:3752889. doi: 10.1155/2023/3752889
Wei, P., He, W., Zhou, Y., and Wang, L. (2013). Performance of motor imagery brain-computer interface based on anodal transcranial direct current stimulation modulation. IEEE Trans. Neural Syst. Rehabil. Eng. 21, 404–415. doi: 10.1109/TNSRE.2013.2249111
Xie, J., Peng, M., Lu, J., Xiao, C., Zong, X., Wang, M., et al. (2021). Enhancement of event-related desynchronization in motor imagery based on transcranial electrical stimulation. Front. Hum. Neurosci. 15:635351. doi: 10.3389/fnhum.2021.635351
Yamaguchi, T., Moriya, K., Tanabe, S., Kondo, K., Otaka, Y., and Tanaka, S. (2020). Transcranial direct-current stimulation combined with attention increases cortical excitability and improves motor learning in healthy volunteers. J. Neuroeng. Rehabil. 17:23. doi: 10.1186/s12984-020-00665-7
Yger, P., Stimberg, M., and Brette, R. (2015). Fast learning with weak synaptic plasticity. J. Neurosci. 35, 13351–13362. doi: 10.1523/JNEUROSCI.0607-15.2015
Yu, T., Xiao, J., Wang, F., Zhang, R., Gu, Z., Cichocki, A., et al. (2015). Enhanced motor imagery training using a hybrid BCI with feedback. IEEE Trans. Biomed. Eng. 62, 1706–1717. doi: 10.1109/TBME.2015.2402283
Keywords: motor imagery, neurofeedback, brain-computer interface, tDCS, combination
Citation: Sawai S, Murata S, Fujikawa S, Yamamoto R, Shima K and Nakano H (2023) Effects of neurofeedback training combined with transcranial direct current stimulation on motor imagery: A randomized controlled trial. Front. Neurosci. 17:1148336. doi: 10.3389/fnins.2023.1148336
Received: 20 January 2023; Accepted: 16 February 2023;
Published: 02 March 2023.
Edited by:
Kishor Lakshminarayanan, Vellore Institute of Technology (VIT), IndiaReviewed by:
Abdul Hanif Khan Yusof Khan, University of Putra Malaysia, MalaysiaFaizan Z. Kashoo, Majmaah University, Saudi Arabia
Copyright © 2023 Sawai, Murata, Fujikawa, Yamamoto, Shima and Nakano. This is an open-access article distributed under the terms of the Creative Commons Attribution License (CC BY). The use, distribution or reproduction in other forums is permitted, provided the original author(s) and the copyright owner(s) are credited and that the original publication in this journal is cited, in accordance with accepted academic practice. No use, distribution or reproduction is permitted which does not comply with these terms.
*Correspondence: Hideki Nakano, bmFrYW5vLWhAdGFjaGliYW5hLXUuYWMuanA=