- 1Department of Neurosurgery, The Second Affiliated Hospital, Zhejiang University School of Medicine, Hangzhou, China
- 2Department of Neurosurgery, Tongde Hospital of Zhejiang Province, Hangzhou, China
Background: Accumulating evidence of clinical and neuroimaging studies indicated that migraine is related to brain structural alterations. However, it is still not clear whether the associations of brain structural alterations with migraine are likely to be causal, or could be explained by reverse causality confounding.
Methods: We carried on a bidirectional Mendelian randomization analysis in order to identify the causal relationship between brain structures and migraine risk. Summary-level data and independent variants used as instruments came from large genome-wide association studies of total surface area and average thickness of cortex (33,992 participants), gray matter volume (8,428 participants), white matter hyperintensities (50,970 participants), hippocampal volume (33,536 participants), and migraine (102,084 cases and 771,257 controls).
Results: We identified suggestive associations of the decreased surface area (OR = 0.85; 95% CI, 0.75–0.96; P = 0.007), and decreased hippocampal volume (OR = 0.74; 95% CI, 0.55–1.00; P = 0.047) with higher migraine risk. We did not find any significant association of gray matter volume, cortical thickness, or white matter hyperintensities with migraine. No evidence supporting the significant association was found in the reverse MR analysis.
Conclusion: We provided suggestive evidence that surface area and hippocampal volume are causally associated with migraine risk.
Introduction
Migraine is a common and complex neurological disease associated with significant psychosocial impact, and has been a leading burden for global population health (Leonardi et al., 2005; Ashina et al., 2021). This disease is usually diagnosed on the basis of clinical criteria and can be further divided into two subtypes, including with- and without-aura (Charles, 2018; Ashina et al., 2021). In recent years, accumulating evidence of clinical and neuroimaging studies indicated that brain structural alterations played a pivotal role in migraine. These T1-weighted magnetic resonance imaging (MRI) studies used voxel-based morphometry or surface-based morphometry to explore brain morphology differences in volume, thickness or surface area (SA) between migraine patients and healthy controls (DaSilva et al., 2007; Schmidt-Wilcke et al., 2008; Datta et al., 2011; Messina et al., 2013; Rocca et al., 2014; Coppola et al., 2017; Husøy et al., 2019; Amin et al., 2021; Kim et al., 2021). Currently, migraine has been hypothesized to be a both neuronal and vascular genetic disorder upon the mainstream opinion (Schwedt and Dodick, 2009; MacGregor, 2017). The latest and largest genome-wide association study (GWAS) meta-analysis, including 102,084 migraine cases and 771,257 controls, identified 123 risk loci associated with migraine, which were enriched in both vascular and central nervous system tissue/cell types (Hautakangas et al., 2022). And it supported the concept that migraine is a neurovascular disorder (Hautakangas et al., 2022).
Previous studies have revealed that cortex in migraine patients has peculiar anatomical, functional, and neurochemical properties (Barbanti et al., 2019). And the incidence of white matter hyperintensities obviously increased in migraine patients (Eikermann-Haerter and Huang, 2021). Hippocampus plays an important role in the processing of pain, pain-related attention, and stress response (Liu et al., 2018). Besides, the volume of hippocampus has been found to be related to migraine prognosis (Liu et al., 2018). Although the significant relationships between brain morphology alterations and migraine could be observed in previous studies, it remains unclear whether brain morphology alterations are the cause or consequence of migraine attacks. The relationship between hippocampal volume and migraines remains unclear, with some studies suggesting a positive correlation and others a negative one. Thus, it is necessary to clarify the direction of the association and provide evidence at the genetic level between brain structures and migraine.
Mendelian randomization (MR) is a genetic epidemiologic method by using genetic variants associated with exposures, which can avoid many of the potential methodological limitations of observational studies, such as reverse causation bias and confounding (Smith and Ebrahim, 2003). In view of the basis that both brain morphometry and migraine risk are influenced by genetic factors, using MR analysis to improve the knowledge of the relationship between brain morphometry alterations and migraine is promising. Recently, only one MR study have investigated the association between intracranial volume and migraine (Mitchell et al., 2022). Important brain traits like cortical SA, cortical thickness, and some other traits of interests were not assessed. Here, we carried out an MR study to explore the causal association between these important brain traits and migraine risk using the largest GWAS data.
Materials and methods
Study design
The MR study builds on three predominant assumptions (Figure 1). (1) Selected instrumental variables (IVs) are strongly and consistently associated with exposures. (2) There is no association between the IVs and confounders. (3) IVs impact outcomes through exposures directly, but not other pathways. Genetic variants are frequently utilized as IVs, due to their well-defined nature and resistance to alteration by environmental factors, thereby avoiding reverse causation (Burgess et al., 2017b). We conducted this bidirectional MR study to clarify the causal relationship between brain structures and migraine.
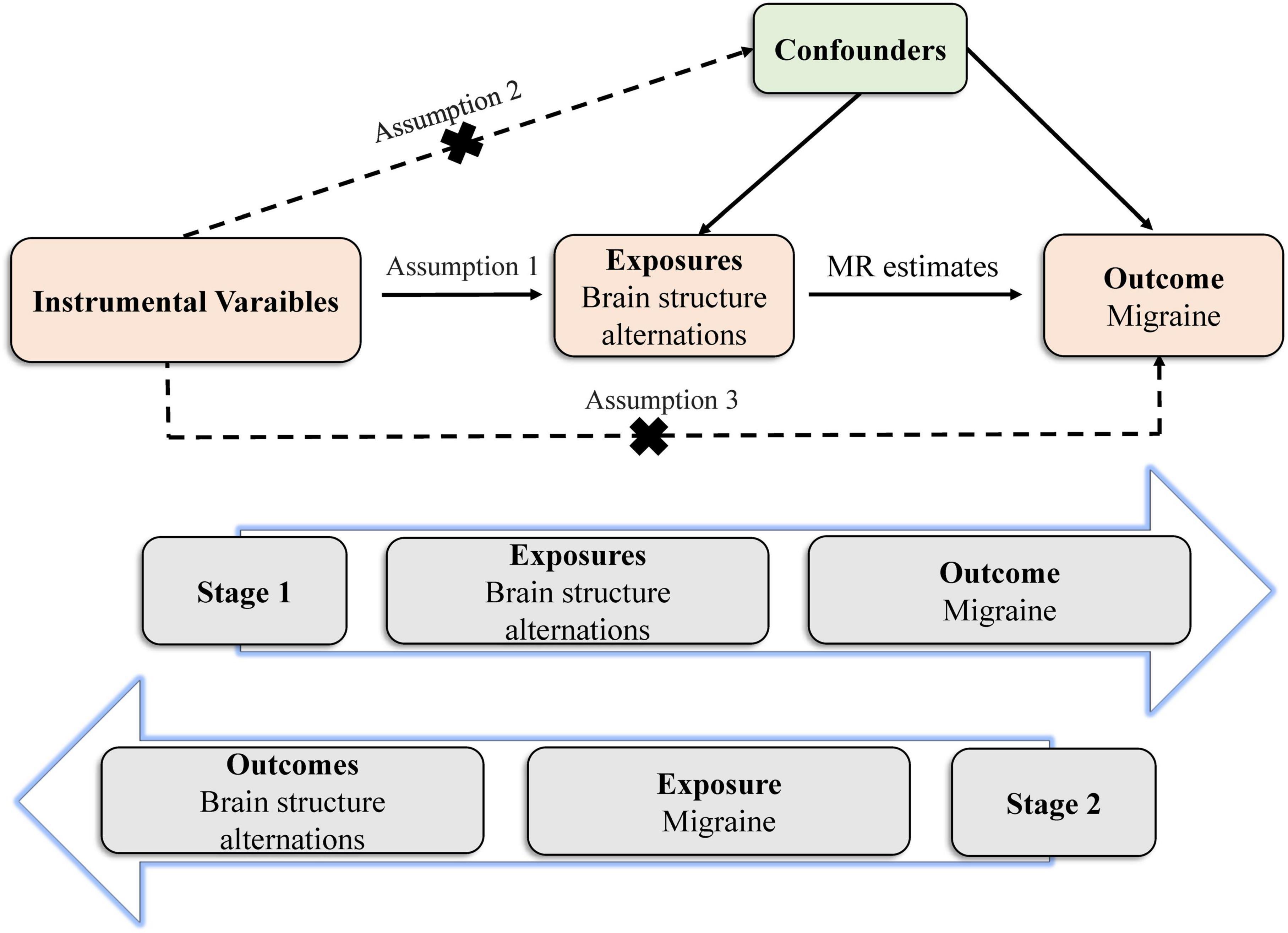
Figure 1. Principles of the Mendelian randomization study for brain structural alterations and migraine risks.
Genetic instruments selection and data sources
Genetic variants associated with total cortical SA and average cortical thickness were obtained from a genome-wide meta-analysis, which were based on 51,665 predominantly healthy participants in the Enhancing NeuroImaging Genetics through Meta-Analysis Consortium and the UK Biobank. The total SA and average cortical thickness were measured on MRI (Grasby et al., 2020). A total of 12 SNPs associated with total SA and 2 SNPs associated with average cortical thickness were collected (P ≤ 8.3 × 10–10). For gray matter volume (GMV), we drew 8 SNPs (P ≤ 5 × 10–8) from a large GWAS of brain imaging-derived phenotypes from an open web server (the Oxford Brain Imaging Genetics),1 which included 33,224 participants from the UK Biobank (Smith et al., 2021). As for white matter hyperintensities (WMH), we obtained 24 SNPs as IVs of WMH from 50,970 individuals from Cohorts for Heart and Aging Research in Genomic Epidemiology consortium and from the UK Biobank (P < 5 × 10–8) (Sargurupremraj et al., 2020). The aggregated risk variants were then confirmed to be associated with WMH in another cohort of 1,738 young healthy adults (P = 2.5 × 10–7), which provided insight into the lifetime impact of WMH. In addition, we extracted six independent SNPs as IVs significantly associated with hippocampal volume (HV) from a genome-wide meta-analysis with 33,536 individuals (P ≤ 5 × 10–8), which accounted for as much as 18.76% of the variance in HV (Hibar et al., 2017). All IVs included were clumped for independence (r2 < 0.1; region size, 3000 kb) according to the Europeans data from the 1,000 Genomes Project. If these included SNPs were not available in the outcome datasets, proxy SNPs (r2 > 0.8) were acquired online as replacements.2
The summary-level data of migraine was obtained from the largest available genome-wide meta-analysis, combining five migraine study collections and comprising of 102,084 migraine cases and 771,257 controls of European ancestry (Hautakangas et al., 2022). A total of 123 independent SNPs associated with migraine were utilized as IVs in reverse analysis (P < 5 × 10–8). In this study, migraine phenotype was defined by self-reported information or second edition of international Classification of Headache Disorders. Logistic regression analyses were conducted by adjusting for age, sex, and at least for the four ancestry principal components. We also used a web tool3 to estimate the bias due to sample overlap and the calculated results were negligible (<1%). All participants gave written informed consent in these studies, and sites involved obtained approval from local research ethics committees or Institutional Review Boards.
Statistical analysis
In the main analyses, we applied the random-effects and fixed-effects inverse-variance weighted (IVW) approach to obtain causal estimates (Burgess et al., 2017a). We conducted several sensitivity analyses to identify potential pleiotropy. Cochran’s Q test was used to evaluate the heterogeneity among different instrumental variables (Burgess et al., 2017a). Weighted median method allowed less than 50% of the genetic variants to be invalid instrumental variables (Burgess et al., 2018). MR-Egger method was conducted to detect and adjust pleiotropic bias (Burgess and Thompson, 2017). To further control potential pleiotropy, we used the MR Pleiotropy Residual Sum and Outlier (MR-PRESSO) method to conduct a global test of heterogeneity and identify horizontal pleiotropy (Verbanck et al., 2018). Once the pleiotropic outlier instruments were identified, a repeated IVW analysis after removing these outlier instruments would be performed (Verbanck et al., 2018).
All tests were two sided and the Bonferroni-corrected significance threshold was set to P < 0.005 (correcting for 10 outcomes). The P-values between 0.005 and 0.05 was defined as suggestive of potential association between exposure and outcome. Odds ratios (ORs) are presented for each 1 standard deviation difference in all exposures. All analyses were conducted by using TwoSampleMR and MR-PRESSO packages in R software (Version 4.1.3).
Results
The main characteristics of datasets adopted in the MR analyses were shown in Supplementary Table 1. All F-statistics of these IVs were higher than the threshold of 10, suggesting no weak instrument bias in the present study. The summary information of SNPs on the five traits was shown in Supplementary Table 2.
In the random-effect IVW estimates, genetically increased cortical SA was potentially associated with a decreased risk of migraine (OR = 0.850; 95% CI, 0.754–0.957; P = 0.007; Figure 2). This association was robust in the weighted median and MR-Egger. And MR-PRESSO did not identify any potential SNP outliers and we did not observe evidence of horizontal pleiotropy in MR-Egger (P for intercept = 0.452). The Cochran’s Q test indicated significant heterogeneity (Cochran Q-derived P = 0.032). There was no significant evidence for association of cortical thickness with migraine (OR = 1.195; 95% CI, 0.958–1.491; P = 0.115).
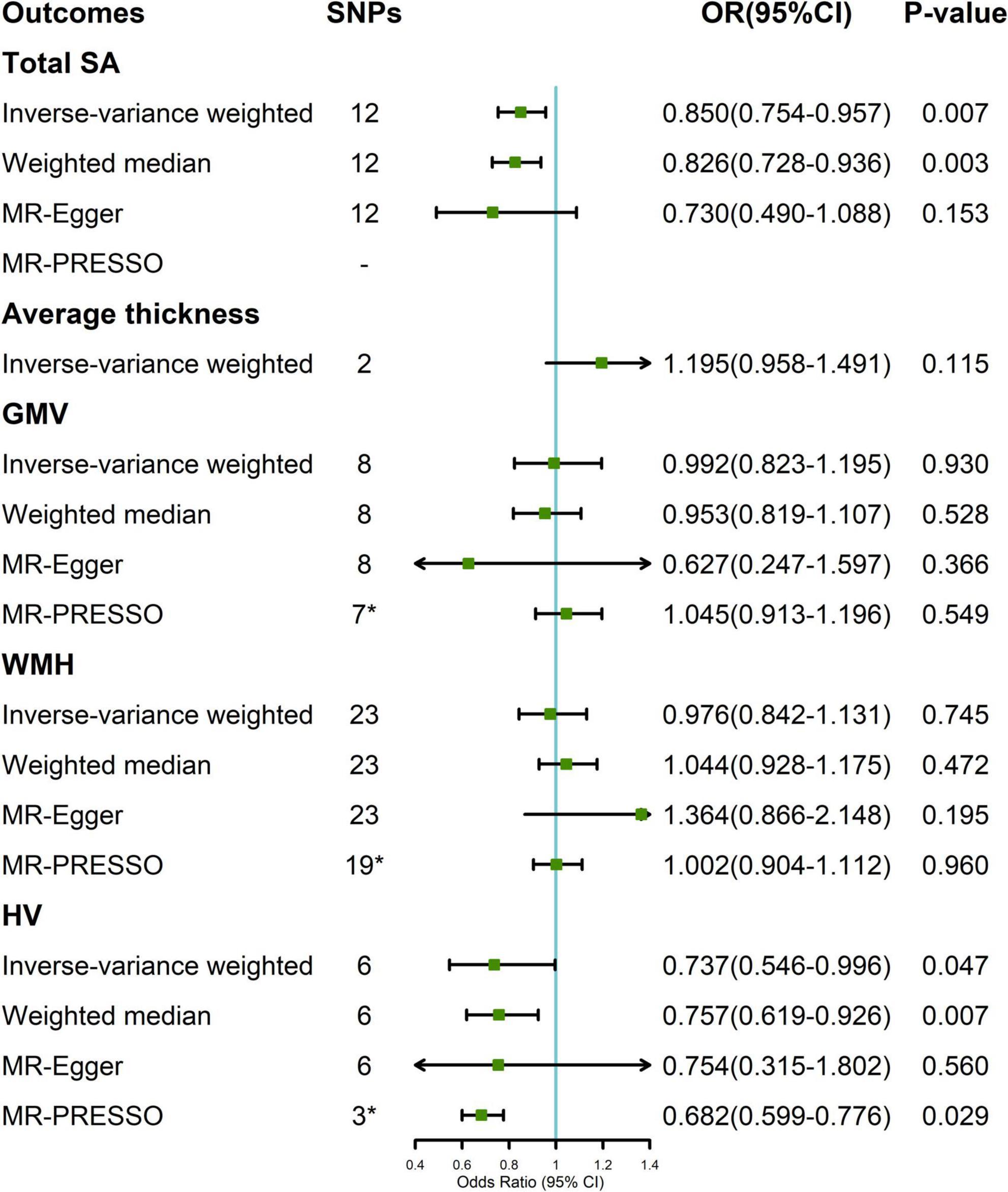
Figure 2. Association between genetically determined brain structural alterations and migraine risks. *MR-PRESSO outlier detected (Supplementary Table 2). CI, confidence interval; OR, odds ratio; SNP, single nucleotide polymorphism; WMH, white matter hyperintensities.
A suggestive association was found between genetically determined HV and migraine (OR = 0.737; 95% CI, 0.546–0.996; P = 0.047; Figure 2). The Cochran’s Q test indicated significant heterogeneity (Cochran Q-derived P < 0.001). There was no indication of horizontal pleiotropy in the association of HV with migraine as measured by MR-Egger (P for intercept = 0.959). Three SNPs (rs11979341, rs61921502, and rs7020341) were detected in the MR-PRESSO test, and the result remained suggestive (P = 0.029). Genetically determined GMV and WMH were not causally associated with migraine in the IVW method. The lack of causality was also confirmed in the weighted median, MR-Egger estimates, and MR-PRESSO.
There were no any significant effects of migraine on brain structural alternation in the reverse estimates (Supplementary Table 3).
Discussion
This MR analysis revealed a suggestive causal association of genetically decreased SA and HV with higher risk of migraine. In addition, there was no evidence supporting the association between genetic liability to migraine and brain structural alterations in the reverse MR analysis.
The literatures on the association between brain structural alterations and migraine remained inconclusive. Several observational studies demonstrated that the global or regional SA decreased in migraine cases compared with healthy controls (Messina et al., 2013; Petrusic et al., 2018; Planchuelo-Gómez et al., 2020). And it frequently involved the visual motion processing, pain processing, and executive function regions (Messina et al., 2013; Planchuelo-Gómez et al., 2020). Cortical SA is usually thought to be congenital and is largely independent of environmental or external factors (Kapellou et al., 2006; Frye et al., 2010; Messina et al., 2013). Some authors supposed that cortical SA could be a good biomarker to distinguish migraine patients from healthy controls (Petrusic et al., 2018). Our results of bidirectional MR analysis further supported the causal association between cortical SA and migraine risk. However, the causal relationship was only found in total SA instead of regional SA due to low variance explained by SNPs. Thus, further studies are required to identify the association between migraine and the related regional SA.
Cortical thickness is a marker reflecting gray matter integrity, which can be determined by the number of their neurogenic divisions. In previous literatures, variation in regional or global cortex thickness was controversial (DaSilva et al., 2007; Messina et al., 2013; Husøy et al., 2019; Torres-Ferrus et al., 2021). A possible explanation was that the sample of included subjects was rather small, while the traits to be compared were many, which might lead to false positive results in this way of comparison. Besides, cortical thickness might undergo considerable changes postnatally. For patients with migraine, several factors might affect cortical thickness, such as age, disease duration, frequency of the attacks, and even the scanning timing (attack phase vs. interictal phase). Some authors hypothesized that increased cortical thickness was a compensatory mechanism to meet the requirement for increased sensory processing of migraine attacks. Similar results of increased cortical thickness were found in other neuropsychiatric disorders, including schizophrenia, autism spectrum disorder, early-stage Parkinson’s disease and so on (Biundo et al., 2013; Williams et al., 2022). Although the direction of the association in our analysis was in accordance with previous studies, the result was not significant (P = 0.115). In addition, only two available SNPs were applied as IVs in the causal estimate, which might influence the result.
Besides, the decrease in HV was suggestively associated with an increased risk of migraine, which was in lined with most of previous studies (Liu et al., 2013, 2017; Chong et al., 2017). At the same time, observational evidence showed that HV had been negatively associated with the frequency and severity of migraine attacks (Maleki et al., 2013; Liu et al., 2017). Mitchell et al. (2022) suggested that migraine attacks might influence HV in a longitudinal study. However, this reverse relationship was not verified in our study or a recent MR study. WMH and GMV were reported to be associated with migraine. Some studies suggested that a patent foramen ovale might be associated with migraines, accompanied by changes in the gray matter and destruction of the white matter (Signoriello et al., 2018; Cao et al., 2022). Possible mechanisms implicated in the pathophysiology of this phenomenon include microembolus-triggered cortical spreading depression, the vasoactive substance hypothesis, impaired cerebral autoregulation, and a common genetic basis (Signoriello et al., 2018; Cao et al., 2022). However, these association were not discovered in our bidirectional MR analysis. In fact, null findings in our study do not truly reflect lack of associations, since MR analyses are dependent on the power of the original GWAS to a large extent (Pierce and Burgess, 2013).
There are some strengths in our study. Firstly, the use of bidirectional MR design permitted an examination of the reverse causation. Secondly, several sensitivity analyses, including pleiotropy robust methods were applied to ensure the valid estimation of the causal effect size. In addition, we used well-powered GWAS data of migraine, which had more statistical power to detect associations than past smaller study. Finally, our results were in lined with a recent MR study based on voxel-based morphometry, which additionally increased the robustness of our results (Mitchell et al., 2022).
This study also has some limitations. Firstly, we only used total cortical SA and average thickness to explore the causal association between cortical structure and migraine, since the fraction of variance explained by SNPs was low in regional SA and thickness. Secondly, sample overlapping may occur between exposure and outcomes population, especially for cohort of UK biobank, which may potentially bias the results. But recently, one study showed that two-sample MR can be applied safely and robustly in a single large dataset using large biobanks (Minelli et al., 2021). Thirdly, most of participants in this study were of European descents, which limited our findings to extend to other ancestries.
In conclusion, we provided suggestive evidence that decreased cortical SA, and decreased HV are suggestively associated with higher migraine risk, and we did not find any significant effect of migraine on brain structural alternation in the reverse estimates. Future investigation into the brain regions is recommended, which helps to clarify the underlying mechanisms and point to new therapies against migraine.
Data availability statement
The original contributions presented in this study are included in the article/Supplementary material, further inquiries can be directed to the corresponding author.
Ethics statement
Ethical review and approval was not required for the study on human participants in accordance with the local legislation and institutional requirements. Written informed consent for participation was not required for this study in accordance with the national legislation and the institutional requirements.
Author contributions
XG: drafting/revision of the manuscript, study design, and analysis and interpretation of data. DW: analysis and interpretation of data. CY: acquisition of data. YH: revision of the manuscript. All authors contributed to the article and approved the submitted version.
Funding
This study was supported by the National Natural Science Foundation of China (81870964) and Zhejiang Province Traditional Chinese Medicine Science and Technology Project (2023ZR075).
Acknowledgments
The authors thank the IHGC, ENIGMA consortiums, BIG40, and ISGC for providing summary statistics data.
Conflict of interest
The authors declare that the research was conducted in the absence of any commercial or financial relationships that could be construed as a potential conflict of interest.
Publisher’s note
All claims expressed in this article are solely those of the authors and do not necessarily represent those of their affiliated organizations, or those of the publisher, the editors and the reviewers. Any product that may be evaluated in this article, or claim that may be made by its manufacturer, is not guaranteed or endorsed by the publisher.
Supplementary material
The Supplementary Material for this article can be found online at: https://www.frontiersin.org/articles/10.3389/fnins.2023.1148458/full#supplementary-material
Abbreviations
GMV, gray matter volume; GWAS, genome-wide association study; HV, hippocampal volume; IV, instrumental variable; IVW, inverse-variance weighted; MR, Mendelian randomization; MRI, magnetic resonance imaging; MR-PRESSO, MR pleiotropy residual sum and outlier; OR, odds ratio; SA, surface area; SNP, single nucleotide polymorphism; WMH, white matter hyperintensities.
Footnotes
- ^ https://open.win.ox.ac.uk/ukbiobank/big40/
- ^ https://ldlink.nci.nih.gov/
- ^ https://sb452.shinyapps.io/overlap/
References
Amin, F. M., De Icco, R., Al-Karagholi, M. A., Raghava, J., Wolfram, F., and Larsson, H. B. W. (2021). Investigation of cortical thickness and volume during spontaneous attacks of migraine without aura: A 3-Tesla MRI study. J. Headache Pain 22:98. doi: 10.1186/s10194-021-01312-9
Ashina, M., Terwindt, G. M., Al-Karagholi, M. A., de Boer, I., Lee, M. J., Hay, D. L., et al. (2021). Migraine: Disease characterisation, biomarkers, and precision medicine. Lancet 397, 1496–1504. doi: 10.1016/S0140-6736(20)32162-0
Barbanti, P., Fofi, L., Aurilia, C., and Egeo, G. (2019). Does the migraine attack start in the cortex and is the cortex critical in the migraine process? Neurol. Sci. 40(Suppl. 1), 31–37. doi: 10.1007/s10072-019-03838-y
Biundo, R., Calabrese, M., Weis, L., Facchini, S., Ricchieri, G., and Gallo, P. (2013). Anatomical correlates of cognitive functions in early Parkinson’s disease patients. PLoS One 8:e64222. doi: 10.1371/journal.pone.0064222
Burgess, S., and Thompson, S. G. (2017). Interpreting findings from Mendelian randomization using the MR-Egger method. Eur. J. Epidemiol. 32, 377–389. doi: 10.1007/s10654-017-0255-x
Burgess, S., Small, D. S., and Thompson, S. G. (2017b). A review of instrumental variable estimators for Mendelian randomization. Stat. Methods Med. Res. 26, 2333–2355. doi: 10.1177/0962280215597579
Burgess, S., Bowden, J., Fall, T., Ingelsson, E., and Thompson, S. G. (2017a). Sensitivity analyses for robust causal inference from Mendelian randomization analyses with multiple genetic variants. Epidemiology 28, 30–42. doi: 10.1097/EDE.0000000000000559
Burgess, S., Zuber, V., Gkatzionis, A., and Foley, C. N. (2018). Modal-based estimation via heterogeneity-penalized weighting: Model averaging for consistent and efficient estimation in Mendelian randomization when a plurality of candidate instruments are valid. Int. J. Epidemiol. 47, 1242–1254. doi: 10.1093/ije/dyy080
Cao, W., Shen, Y., Zhong, J., Chen, Z., Wang, N., and Yang, J. (2022). The patent foramen Ovale and migraine: Associated mechanisms and perspectives from MRI Evidence. Brain Sci. 12:941. doi: 10.3390/brainsci12070941
Charles, A. (2018). The pathophysiology of migraine: Implications for clinical management. Lancet Neurol. 17, 174–182. doi: 10.1016/S1474-4422(17)30435-0
Chong, C. D., Dumkrieger, G. M., and Schwedt, T. J. (2017). Structural co-variance patterns in migraine: A cross-sectional study exploring the role of the hippocampus. Headache 57, 1522–1531. doi: 10.1111/head.13193
Coppola, G., Petolicchio, B., Di Renzo, A., Tinelli, E., Di Lorenzo, C., and Parisi, V. (2017). Cerebral gray matter volume in patients with chronic migraine: Correlations with clinical features. J. Headache Pain 18:115. doi: 10.1186/s10194-017-0825-z
DaSilva, A. F., Granziera, C., Snyder, J., and Hadjikhani, N. (2007). Thickening in the somatosensory cortex of patients with migraine. Neurology 69, 1990–1995. doi: 10.1212/01.wnl.0000291618.32247.2d
Datta, R., Detre, J. A., Aguirre, G. K., and Cucchiara, B. (2011). Absence of changes in cortical thickness in patients with migraine. Cephalalgia 31, 1452–1458. doi: 10.1177/033310241142102
Eikermann-Haerter, K., and Huang, S. Y. (2021). White matter lesions in migraine. Am. J. Pathol. 191, 1955–1962. doi: 10.1016/j.ajpath.2021.02.007
Frye, R. E., Liederman, J., Malmberg, B., McLean, J., Strickland, D., and Beauchamp, M. S. (2010). Surface area accounts for the relation of gray matter volume to reading-related skills and history of dyslexia. Cereb. Cortex 20, 2625–2635. doi: 10.1093/cercor/bhq010
Grasby, K. L., Jahanshad, N., Painter, J. N., Colodro-Conde, L., Bralten, J., and Hibar, D. P. (2020). The genetic architecture of the human cerebral cortex. Science 367:eaay6690. doi: 10.1126/science.aay669
Hautakangas, H., Winsvold, B. S., Ruotsalainen, S. E., Bjornsdottir, G., Harder, A. V. E., and Kogelman, L. J. A. (2022). Genome-wide analysis of 102,084 migraine cases identifies 123 risk loci and subtype-specific risk alleles. Nat. Genet. 54, 152–160. doi: 10.1038/s41588-021-00990-0
Hibar, D. P., Adams, H. H. H., Jahanshad, N., Chauhan, G., Stein, J. L., and Hofer, E. (2017). Novel genetic loci associated with hippocampal volume. Nat. Commun. 8:13624. doi: 10.1038/ncomms13624
Husøy, A. K., Håberg, A. K., Rimol, L. M., Hagen, K., Vangberg, T. R., and Stovner, L. J. (2019). Cerebral cortical dimensions in headache sufferers aged 50 to 66 years: A population-based imaging study in the Nord-Trøndelag health study (HUNT-MRI). Pain 160, 1634–1643. doi: 10.1097/j.pain.0000000000001550
Kapellou, O., Counsell, S. J., Kennea, N., Dyet, L., Saeed, N., and Stark, J. (2006). Abnormal cortical development after premature birth shown by altered allometric scaling of brain growth. PLoS Med. 3:e265. doi: 10.1371/journal.pmed.0030265
Kim, S. K., Nikolova, S., and Schwedt, T. J. (2021). Structural aberrations of the brain associated with migraine: A narrative review. Headache 61, 1159–1179. doi: 10.1111/head.14189
Leonardi, M., Steiner, T. J., Scher, A. T., and Lipton, R. B. (2005). The global burden of migraine: Measuring disability in headache disorders with WHO’s classification of functioning, disability and health (ICF). J. Headache Pain 6, 429–440. doi: 10.1007/s10194-005-0252-4
Liu, H. Y., Chou, K. H., and Chen, W. T. (2018). Migraine and the hippocampus. Curr. Pain Headache Rep. 22:13. doi: 10.1007/s11916-018-0668-6
Liu, H. Y., Chou, K. H., Lee, P. L., Fuh, J. L., Niddam, D. M., and Lai, K. L. (2017). Hippocampus and amygdala volume in relation to migraine frequency and prognosis. Cephalalgia 37, 1329–1336. doi: 10.1177/033310241667862
Liu, J., Lan, L., Li, G., Yan, X., Nan, J., and Xiong, S. (2013). Migraine-related gray matter and white matter changes at a 1-year follow-up evaluation. J. Pain 14, 1703–1708. doi: 10.1016/j.jpain.2013.08.013
Maleki, N., Becerra, L., Brawn, J., McEwen, B., Burstein, R., and Borsook, D. (2013). Common hippocampal structural and functional changes in migraine. Brain Struct. Funct. 218, 903–912. doi: 10.1007/s00429-012-0437-y
Messina, R., Rocca, M. A., Colombo, B., Valsasina, P., Horsfield, M. A., and Copetti, M. (2013). Cortical abnormalities in patients with migraine: A surface-based analysis. Radiology 268, 170–180. doi: 10.1148/radiol.13122004
Minelli, C., Del Greco, M. F., van der Plaat, D. A., Bowden, J., Sheehan, N. A., and Thompson, J. (2021). The use of two-sample methods for Mendelian randomization analyses on single large datasets. Int. J. Epidemiol. 50, 1651–1659. doi: 10.1093/ije/dyab084
Mitchell, B. L., Diaz-Torres, S., Bivol, S., Cuellar-Partida, G., International Headache Genetics Consortium, and Gerring, Z. F. (2022). Elucidating the relationship between migraine risk and brain structure using genetic data. Brain 145, 3214–3224. doi: 10.1093/brain/awac105
Petrusic, I., Dakovic, M., Kacar, K., and Zidverc-Trajkovic, J. (2018). Migraine with aura: Surface-based analysis of the cerebral cortex with magnetic resonance imaging. Korean J. Radiol. 19, 767–776. doi: 10.3348/kjr.2018.19.4.767
Pierce, B. L., and Burgess, S. (2013). Efficient design for Mendelian randomization studies: Subsample and 2-sample instrumental variable estimators. Am. J. Epidemiol. 178, 1177–1184. doi: 10.1093/aje/kwt084
Planchuelo-Gómez, Á, García-Azorín, D., Guerrero, ÁL., Rodríguez, M., Aja-Fernández, S., and de Luis-García, R. (2020). Gray matter structural alterations in chronic and episodic migraine: A morphometric magnetic resonance imaging study. Pain Med. 21, 2997–3011. doi: 10.1093/pm/pnaa271
Rocca, M. A., Messina, R., Colombo, B., Falini, A., Comi, G., and Filippi, M. (2014). Structural brain MRI abnormalities in pediatric patients with migraine. J. Neurol. 261, 350–357. doi: 10.1007/s00415-013-7201-y
Sargurupremraj, M., Suzuki, H., Jian, X., Sarnowski, C., Evans, T. E., and Bis, J. C. (2020). Cerebral small vessel disease genomics and its implications across the lifespan. Nat. Commun. 11:6285. doi: 10.1038/s41467-020-19111-2
Schmidt-Wilcke, T., Gänssbauer, S., Neuner, T., Bogdahn, U., and May, A. (2008). Subtle grey matter changes between migraine patients and healthy controls. Cephalalgia 28, 1–4. doi: 10.1111/j.1468-2982.2007.01428
Schwedt, T. J., and Dodick, D. W. (2009). Advanced neuroimaging of migraine. Lancet Neurol. 8, 560–568. doi: 10.1016/S1474-4422(09)70107-3
Signoriello, E., Cirillo, M., Puoti, G., Signoriello, G., Negro, A., and Koci, E. (2018). Migraine as possible red flag of PFO presence in suspected demyelinating disease. J. Neurol. Sci. 390, 222–226. doi: 10.1016/j.jns.2018.04.042
Smith, G. D., and Ebrahim, S. (2003). ‘Mendelian randomization’: Can genetic epidemiology contribute to understanding environmental determinants of disease? Int. J. Epidemiol. 32, 1–22. doi: 10.1093/ije/dyg070
Smith, S. M., Douaud, G., Chen, W., Hanayik, T., Alfaro-Almagro, F., and Sharp, K. (2021). An expanded set of genome-wide association studies of brain imaging phenotypes in UK Biobank. Nat. Neurosci. 24, 737–745. doi: 10.1038/s41593-021-00826-4
Torres-Ferrus, M., Pareto, D., Gallardo, V. J., Cuberas-Borrós, G., Alpuente, A., and Caronna, E. (2021). Cortical metabolic and structural differences in patients with chronic migraine. An exploratory 18FDG-PET and MRI study. J. Headache Pain 22:75. doi: 10.1186/s10194-021-01289-5
Verbanck, M., Chen, C. Y., Neale, B., and Do, R. (2018). Detection of widespread horizontal pleiotropy in causal relationships inferred from Mendelian randomization between complex traits and diseases. Nat. Genet. 50, 693–698. doi: 10.1038/s41588-018-0099-7
Keywords: brain structure, NeuroImage, Mendelian randomization, migraine, cortex
Citation: Guo X, Wang D, Ying C and Hong Y (2023) Association between brain structures and migraine: A bidirectional Mendelian randomization study. Front. Neurosci. 17:1148458. doi: 10.3389/fnins.2023.1148458
Received: 20 January 2023; Accepted: 10 February 2023;
Published: 03 March 2023.
Edited by:
Jing Li, Capital Medical University, ChinaReviewed by:
Jun Zhou, Chengdu University of Traditional Chinese Medicine, ChinaConstantina Aggeli, National and Kapodistrian University of Athens, Greece
Copyright © 2023 Guo, Wang, Ying and Hong. This is an open-access article distributed under the terms of the Creative Commons Attribution License (CC BY). The use, distribution or reproduction in other forums is permitted, provided the original author(s) and the copyright owner(s) are credited and that the original publication in this journal is cited, in accordance with accepted academic practice. No use, distribution or reproduction is permitted which does not comply with these terms.
*Correspondence: Yuan Hong, aHkwOTA0QHpqdS5lZHUuY24=