- 1Faculty of Information Engineering and Automation, Kunming University of Science and Technology, Kunming, China
- 2Brain Cognition and Brain-Computer Intelligence Integration Group, Kunming University of Science and Technology, Kunming, China
- 3School of Information Engineering, Chinese People’s Armed Police Force Engineering University, Xi’an, China
- 4Faculty of Science, Kunming University of Science and Technology, Kunming, China
Brain signal patterns generated in the central nervous system of brain-computer interface (BCI) users are closely related to BCI paradigms and neural coding. In BCI systems, BCI paradigms and neural coding are critical elements for BCI research. However, so far there have been few references that clearly and systematically elaborated on the definition and design principles of the BCI paradigm as well as the definition and modeling principles of BCI neural coding. Therefore, these contents are expounded and the existing main BCI paradigms and neural coding are introduced in the review. Finally, the challenges and future research directions of BCI paradigm and neural coding were discussed, including user-centered design and evaluation for BCI paradigms and neural coding, revolutionizing the traditional BCI paradigms, breaking through the existing techniques for collecting brain signals and combining BCI technology with advanced AI technology to improve brain signal decoding performance. It is expected that the review will inspire innovative research and development of the BCI paradigm and neural coding.
1 Introduction
Brain-computer interface (BCI) is a revolutionizing human-computer interaction (Graimann et al., 2013), which directly bypasses peripheral nerves and muscles to establish a new communication and control channel between the brain and external devices (Wolpaw and Wolpaw, 2012). It has the potential to monitor, replace, improve/recover, enhance, and supplement damaged or impaired inputs or outputs of the central nervous system (CNS) (Ramsey and Millán José del, 2020).
In the BCI system, brain signal patterns generated in the CNS of the BCI user are closely related to BCI paradigms and neural coding (Allison et al., 2012), which are the foundation for decoding correctly the user’s intent. Therefore, BCI paradigms and neural coding are critical in BCI research. To date, there have been many references on brain signal processing and classification algorithms in BCI systems. For instance, Lotte et al. (2007, 2018) provided a comprehensive overview of the modern classification algorithms used in electroencephalogram (EEG) -based BCIs, and Bashashati et al. (2007) provided a comprehensive review of the signal processing techniques in BCI systems. However, there have been few references focusing on the definition and design principles of the BCI paradigm as well as the definition and modeling principles of BCI neural coding. For example, Abiri et al. (2019) reviewed EEG-based BCI paradigms, and Xu et al. (2021) reviewed the EEG-based BCI brain coding and decoding mechanisms. In addition to EEG-based BCI paradigms and neural coding, there are also other BCI paradigms and neural coding based on brain imaging techniques, such as intracortical local field potentials (LFP) (Hochberg et al., 2012; Willett et al., 2021, 2023), electroencephalogram (ECoG) (Luo et al., 2022; Branco et al., 2023; Metzger et al., 2023), functional near-infrared spectroscopy (fNIRS) (Abdalmalak et al., 2021; Paulmurugan et al., 2021; Eastmond et al., 2022), functional magnetic resonance imaging (fMRI) (Naselaris et al., 2011; Du et al., 2019), magnetoencephalography (MEG) (Xu et al., 2022; Bu et al., 2023), and hybrid brain-computer interface (hBCI) (Choi et al., 2017; Mussi and Adams, 2022). Therefore, we systematically elaborated on the definition and design principles of the BCI paradigm as well as the definition and modeling principles of BCI neural coding and introduced the existing main BCI paradigms and neural coding. Finally, the challenges and future research directions of the BCI paradigm and neural coding were discussed. It is expected that the review will inspire innovative research and development of the BCI paradigm and neural coding.
2 Definition and design principles of the BCI paradigm
2.1 Definition of BCI paradigm
Brain-computer interface paradigm is a set of specific mental tasks or external stimuli carefully selected/designed by the BCI developer to represent the user’s intentions under specific brain imaging techniques. The purpose of the BCI paradigm is to “write” the user’s intentions into brain signals, which represent or code the user’s intentions. It is expected that the brain imaging technology used can detect the neural coding of the user’s intentions, laying the foundation for subsequent “reading” or decoding of the user’s intentions (Chavarriaga et al., 2017; Wolpaw et al., 2020). It is worth noting that it is difficult for BCI to decode the arbitrary or random mental activity of the user, as well as the arbitrary or random external stimulus received by the user.
Specific mental tasks are implicit mental activities, such as motor imagery (MI), visual imagery, speech imagery, mental arithmetic, and reasoning; specific external stimuli are explicit attentional tasks, such as visual, auditory, and tactile stimuli. Specific features of brain signals are induced by mental tasks or external stimuli, and identify specific mental tasks and specific stimuli, which provide the basis for subsequent BCI decoding. Mental tasks or external stimuli correspond to specific brain functions and brain activities, which are closely related to specific brain regions and brain networks/brain circuits. Figure 1 illustrates the relationship between the BCI paradigm, specific brain functions, and structures. Special attention should be paid to the fact that BCI paradigms are usually discussed in the context of specific brain imaging techniques, which means that BCI paradigms are closely related to specific brain imaging techniques.
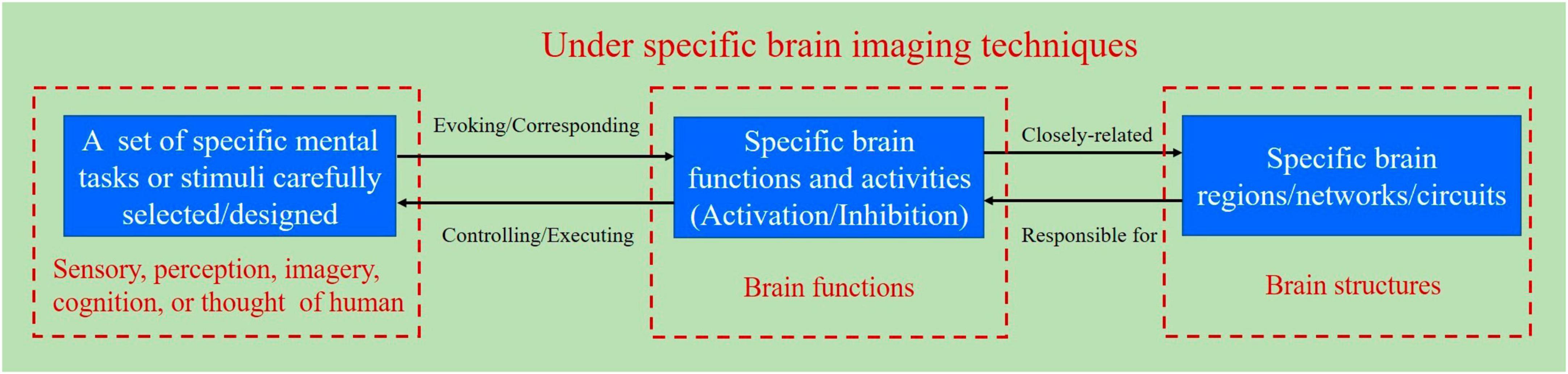
Figure 1. Schematic diagram for the relationship between the BCI paradigm, specific brain functions, and structures.
2.2 Principles for designing BCI paradigm
There have been several BCI paradigms so far, such as MI, steady-state visual evoked potential (SSVEP), and P300 paradigms. These paradigms have their advantages and disadvantages, and many researchers are still improving these paradigms. The innovative design of BCI paradigms is one of the critical contents of BCI research. To translate the designed BCI paradigms into practical applications, the principles for designing the BCI paradigm are based on user-centered design (Kübler et al., 2014; Chavarriaga et al., 2017; Lyu et al., 2023) and human factors engineering of BCI (Allison et al., 2012; Kübler et al., 2013, 2020; Liberati et al., 2015; Martin et al., 2018; Kübler, 2020; Ramsey and Millán José del, 2020; Wolpaw et al., 2020; Branco et al., 2021) to evaluate it. The principles for designing the BCI paradigm are proposed in Table 1.
2.2.1 CNS signals evoked by BCI paradigm specific tasks should have good separability
Brain-computer interface paradigms require users to perform specific mental tasks or receive specific external stimuli. Brain signal features evoked by the user performing the designed BCI paradigm task are significantly differentiable for different mental tasks or external stimuli under specific brain imaging techniques, or the relevant CNS activity better coded the mental tasks or external stimuli designed by the BCI paradigm. Better separability is the basis for subsequently achieving higher BCI decoding accuracy. It is worth noting that specific brain imaging techniques need to be considered in the innovative design of BCI paradigms.
When screening various mental tasks and external stimuli for combination, the classification performance of various mental tasks combinations or external stimuli combinations (Jang et al., 2022) needs to be evaluated to determine the most suitable mental tasks combinations or external stimuli combinations for customized BCI.
2.2.2 BCI paradigm tasks are easy for users to perform
Some mental tasks are easy to perform, while others are not, usually choosing tasks that users are proficient in and natural in their daily life and work. Mental tasks are designed to be as simple as possible, suitable for users, approved, and even enjoyed by users. Easy to perform BCI paradigm tasks can increase user acceptance of BCI technology and promote its translation into practical applications.
2.2.3 BCI paradigm tasks are safe for the user
The brain imaging technology involved in the BCI paradigm is required to be safe for users and not harmful to their physical and mental health (Liberati et al., 2015). In addition, external stimuli have a lower risk of causing brain diseases in users, and mental tasks and external stimuli are less likely to cause excessive fatigue in users, to reduce mental load.
2.2.4 BCI paradigm tasks bring the user a good experience and comfort level
The user experience and comfort of the BCI paradigm are related to the comfort of the sensors used to collect brain signals, as well as the experience and comfort of mental tasks or external stimuli. They are also related to the decoding performance (stability, accuracy, and speed of decoding) under the BCI paradigm, which affects the user’s acceptance of BCI. The BCI paradigm is required to have a high user rating for experience and comfort, which can be evaluated by an experience and comfort questionnaire. At present, the user experience and comfort of the existing BCI paradigm are not high, and the acceptance of BCI by users is not high.
2.2.5 Tasks specified by the BCI paradigm are consistent with tasks controlled by BCI
Brain-computer interface paradigm tasks are designed to avoid non-transparent mappings, which are inconsistencies between mental tasks and control commands, such as the use of left-handed MI to imagine the corresponding commands to control the robot’s movement to the right. Non-transparent mappings may lead to changes in autonomous intentions, which may affect the user’s performance during brain-computer interaction. For this reason, when designing a BCI paradigm task, it is important to consistent the mental task with the task controlled by the BCI.
2.2.6 BCI paradigm tasks are designed to the needs of specific user
During the screening of BCI paradigm tasks, the combination of mental tasks or external stimuli should be designed according to the specific needs of the application, the more mental tasks or external stimuli are not the better. It is necessary to just simply fulfill its requirements. In the case of rehabilitation training for movement disorders, it is appropriate to choose the MI of the corresponding limbs as the paradigm, but if it is to realize the simple communication of “YES” or “NO,” it is not appropriate to use the left or right limb MI as the mental task.
2.2.7 The overall user satisfaction of BCI paradigm tasks is high level
User satisfaction with BCI paradigm tasks is related to several factors that include the above-mentioned BCI paradigms should have good separability, be easy for the user to perform, be safe for the user, bring the user a good experience and comfort level, consistent with tasks controlled by BCI, and fit in the application needs of a specific user, which need to be considered and evaluated comprehensively to design a user-friendly BCI paradigm task.
The BCI paradigm task has a significant impact on whether potential BCI users accept and enjoy using the BCI system, and for this reason, the mental tasks that drive BCI need to be designed and optimized according to the user’s ability characteristics.
3 Definition and mechanisms models of BCI neural coding
Based on the definition and design principles of the BCI paradigm elaborated above, the following sections elaborate on the definition and design principles of BCI neural coding, the relationship between BCI paradigm, BCI neural coding, and BCI neural decoding, and the relationship between BCI neural coding, brain neural coding, and computer information coding.
3.1 Definition of BCI neural coding
Brain-computer interface neural coding refers to the coding of different user intentions into central neural signals under a specific BCI paradigm, which is characterized by distinguishable brain signal features. Brain signals with encoded intentions can be detected by specific brain imaging techniques, at last, user intentions can be recognized by BCI neural decoding algorithms. The process of BCI neural coding is shown in Figure 2.
It is worth noting that BCI neural coding is closely related to the chosen level and parameter settings of brain imaging technology, such that the level of technology used to record the ECoG signals and the hardware settings (including the sampling frequency and hardware filters) affect the types of features that can be extracted and analyses that can be performed (Hill et al., 2012; Shirhatti et al., 2016).
3.2 Principles for modeling BCI neural coding
Modeling BCI neural coding requires consideration of specific BCI paradigms, mechanisms of brain neural coding, neural signal features collected by different brain imaging techniques, and efficient decoding of the user’s intentions.
(1) Modeling neural coding under a specific BCI paradigm. Different BCI paradigms, such as SSVEP-BCI, P300-BCI, MI-BCI, and other paradigms have different neural coding models.
(2) Modeling BCI neural coding based on the mechanisms of brain neural coding. The mechanisms of brain neural coding characterize the hypothesized relationships between external stimuli or mental tasks and the response of specific neuronal populations, as well as the relationships between the electrical activity of neurons within neuronal populations (Johnson, 2000; Brown et al., 2004). An implantable BCI neural coding model can be modeled based on these relationships within the mechanisms of brain neural coding.
(3) Considering the features from the time domain, frequency domain, and spatial domain of neural signals collected using different brain imaging techniques when modeling BCI neural coding. Given the different temporal and spatial resolutions of brain imaging techniques, such as EEG, fNIRS, fMRI, MEG, ECoG, intracortical LFP, or Spikes, the measured brain activities (electrical activities of central neurons or metabolic activities of brain tissue) are different.
(4) BCI neural coding model being beneficial for subsequent neural decoding. The purpose of modeling BCI neural coding is to efficiently decode the user’s intentions.
3.3 Relationship between BCI paradigm, BCI neural coding, and BCI neural decoding
Brain-computer interface paradigm tasks are usually designed first, then the neural coding under the BCI paradigm is revealed, followed by the extraction of brain signal features from neural coding laws, and finally the neural decoding. The BCI paradigm with BCI neural coding is the basis or premise of BCI decoding. It should be emphasized that in BCI systems, there is no corresponding neural coding without the BCI paradigm, no neural decoding without BCI neural coding, or no high-performance neural decoding performance without good BCI paradigms and neural coding. Figure 3 illustrates the relationship between BCI paradigm, BCI neural coding, and BCI neural decoding.
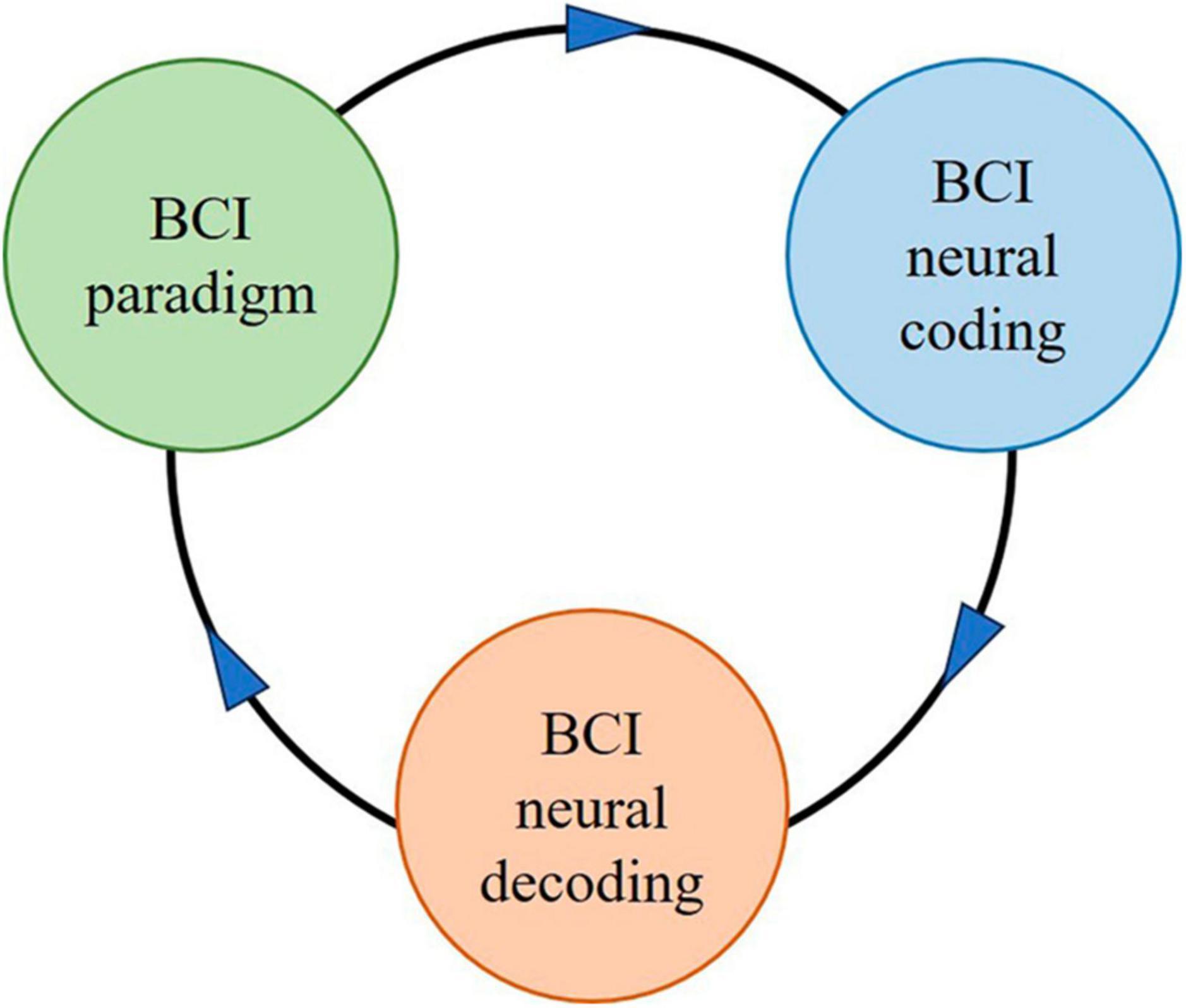
Figure 3. The schematic diagram for the relationship between BCI paradigm, BCI neural coding, and BCI neural decoding.
3.4 Relationship between BCI neural coding, brain neural coding, and computer information coding
Brain neural coding is the basis of BCI neural coding, which characterizes the hypothesized relationships between external stimuli / mental tasks and the responses of specific neurons or populations of neurons (Johnson, 2000; Brown et al., 2004). According to the theory that sensory and other information is represented in the brain by a network of neurons, it is believed that neurons can encode both digital and analog information (Thorpe, 1990). Computer information coding is the process of converting information from one form or format to another and can be used to represent the relationship of things, which can be represented by numbers, letters, special symbols, or combinations of them, to convert data into codes or coded characters that can be translated into the original data form. Figure 4 illustrates the relationship between BCI neural coding, brain neural coding, and computer information coding.
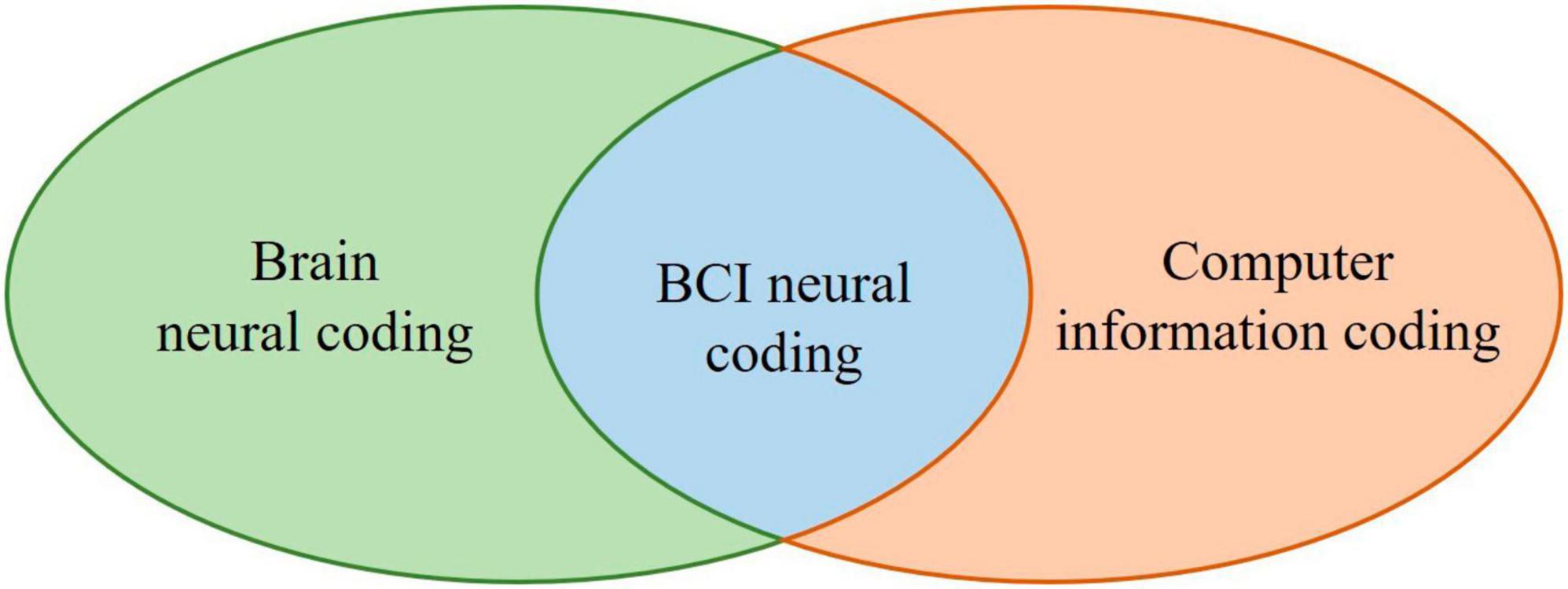
Figure 4. The schematic diagram for the relationship between BCI neural coding, brain neural coding, and computer information coding.
Inspired by the brain neural coding model in Figure 4, BCI neural coding models can be proposed, as shown in Table 2, and will be reviewed in sections 3.5–3.11. These BCI neural codes will eventually be transformed into computer information coding to be processed by computers.
3.5 Frequency/rate coding for BCI
Frequency/rate coding for intracortical BCI can be inspired by traditional models of neuronal firing rate coding. In most sensory systems, the firing rate increases, generally non-linearly, with increasing stimulus intensity (Kandel et al., 2000). Since the sequence of action potentials generated by a given stimulus varies from trial to trial, neuronal responses are typically treated statistically or probabilistically. The spike count rate for a single trial can be calculated from Equation (1) (Gerstner and Kistler, 2002).
The Dtrial is the duration of a trial, with typical values of 100 ms or 500 ms (Gerstner and Kistler, 2002), and Nspikes is the number of spikes occurring within the Dtrial.
The time-dependent rate of issuance in a time-dependent stimulus can be calculated from Equation (2) (Gerstner and Kistler, 2002).
where NK is the number spike appearing on all repeated trials from time t and t+Δt, K is the number of retrials, t is the start time relative to the stimulus sequence, and Δt is the time interval, usually in the range of a millisecond or a few milliseconds. The FRtdapplies to both resting and time-dependent stimuli, but it is unlikely to be the coding scheme used by neurons in the brain (Gerstner and Kistler, 2002).
3.6 Time coding for BCI
Intracortical BCI time coding can be inspired by time coding models in neural coding (Butts et al., 2007; Gollisch and Meister, 2008). The time resolution of neural coding is on the millisecond time scale, suggesting that precise spike timing is an important element in neural coding (Theunissen and Miller, 1995). For example, the ability of many organisms to discriminate between stimuli (such as visual stimuli, auditory stimuli, tactile stimuli, gustatory stimuli, and olfactory stimuli) on a millisecond time scale suggests that time coding is also a model that functions in sensory systems (Gollisch and Meister, 2008).
The spiking activity features that can be extracted by time coding are time-to-first-spike after the stimulus onset (Victor, 2005; Carleton et al., 2010), phase-of-firing for background oscillations, features based on the second and higher statistical moments of the ISI probability distribution (Kostal et al., 2007), spike randomness, or precisely timed groups of spikes (Thorpe, 1990; Jolivet et al., 2006; Chen et al., 2009).
Information on the time structure of stimulus-evoked spike trains or dispensing rates is determined by the dynamics of the stimulus, the properties of the stimulus, and the nature of the neural coding process (Montemurro et al., 2008). In Equation (2) the code is rate coding if FRtdchanges slowly with time and time coding if it changes rapidly.
3.7 Phase-of-firing coding for BCI
Intracortical BCI phase coding can be inspired by phase-of-firing coding models in neural coding (Havenith et al., 2011). Phase-of-firing coding is a neural coding scheme that this type of code takes into account the spike count coding and a time label for each spike according to a time reference based on the phase of local ongoing oscillations at low (Montemurro et al., 2008) or high frequencies (Fries et al., 2007; Havenith et al., 2011). The feature of this code is that neurons adhere to a preferred order of spiking between a group of sensory neurons, resulting in a firing sequence (Havenith et al., 2011). For example, each neuron in the visual cortex has its own preferred/preferred relative firing time during the gamma oscillation cycle.
3.8 Intracortical neuronal population coding for BCI
Intracortical BCI neuron population coding can be inspired by the joint activity of multiple neurons to characterize stimuli or mental activity. In BCI intracortical neuronal population coding, each neuron has a distribution of responses over some set of inputs, and the responses of many neurons may be combined to determine corresponding stimulus or mental activity about the inputs. Neuronal population coding captures the essential features of neural coding (Wu et al., 2002).
Experimental studies have revealed that this coding paradigm is widely used in the sensor and motor areas of the brain. for example, Neurons are modulated to the moving/motor direction in the visual area medial time (Maunsell and Van Essen, 1983). However, Individual neurons fire fastest or slower in one direction depending on the distance of the target from the neuron’s “preferred” direction, the vectors of all neurons, and the coding of motion signals.
Typical neuronal population coding involves neurons with Gaussian modulation curves whose mean value varies linearly with stimulus intensity. Positional coding in a population of neurons can be used to encode continuous variables such as joint position, eye position, color, or sound frequency. Rate coding of the entire population ensures higher fidelity and accuracy than rate coding of individual neurons (Mathis et al., 2012).
3.9 Correlation coding for BCI
Intracortical BCI correlation coding can be inspired by the correlation coding model of neuronal firing (Panzeri et al., 1999). This model suggests that correlations between action potentials or “spikes” within a spike sequence may carry additional information beyond the simple timing of the spikes (Ahmadi et al., 2021; Zhang and Constandinou, 2023). Correlations may also carry information that is not present in the average firing rate of the two pairs of neurons (Decharms and Merzenich, 1996).
3.10 Sparse coding for BCI
Intracortical biometric sparse coding can be inspired by the sparse coding model of neural coding, in which each activity of a stimulus or mental activity is encoded by the strong activation of a relatively small set of neurons, which do not use the full set of available neurons, but rather a subset of them. In the BCI decoding stage, algorithms for sparse signal representation and processing can be used.
The fact that only a few neurons in a population of neurons respond to a given stimulus and that each neuron responds to only a few stimuli out of all possible stimuli, may be a biological selective response. Theoretical studies of sparse distributed memory have shown that sparse coding increases the capacity of associative memory by reducing the overlap between representations (Kanerva, 1988). Experimentally, sparse representations of sensory information have been observed in several systems, including visual (Vision, 2000), auditory (Hromádka et al., 2008), tactile (Crochet et al., 2011), and olfactory (Ito et al., 2008).
Sparsity may be focused on time sparsity, for example, the features extracted from all frequency bands during motion imagery are not all well separable (Olshausen and Field, 1996); It may also focus on spatial sparsity, as in the sparsity of neuronal populations activated, or the sparsity of brain regions/brain networks activated, such that it is the sensory-motor-related brain regions or brain networks that are predominantly activated during MI.
Most sparse coding models are based on linear generative models (Rehn and Sommer, 2007), as shown in Equation (3) (Lee et al., 2006).
where is the set of k-dimensional real input vectors, is the set of n k-dimensional basis vectors, and is the sparse n-dimensional vectors and sj is the weight of each input vector bj are combined linearly by Eq. (3) to approximate the inputs. Other models are based on match tracking and dictionary learning (Pati et al., 1993; Davis et al., 1994; Needell and Tropp, 2009).
3.11 Hybrid coding for BCI
To better or more fully characterize the relationship between the stimulus / mental activity and the neural response, a combination of several neural coding methods can be considered, which is a hybrid neural coding approach. To more accurately decode the stimulus or mental activity from the neural response, intracortical BCI hybrid coding schemes can combine two or more of the above models (Choi et al., 2017; Mussi and Adams, 2022). For example, global features such as pitch or formant transition profiles can be represented by both rate coding and place coding (Miller and Sachs, 1983).
4 Existing main BCI paradigms and neural coding
Brain-computer interface paradigms and neural coding are directly related to specific brain imaging techniques. The existing main BCI paradigms and neural coding models involve brain imaging techniques including the acquisition of intracortical LFP, ECoG, fNIRS, fMRI, MEG, EEG, and hybrid brain imaging techniques, as shown in Table 3.
4.1 Intracortical LFP-BCI paradigms and neural coding
The wrapping of intracortical electrodes has a significant effect on the collection of individual neuronal Spikes but not on LFP (Milekovic et al., 2018, 2019). LFP is expected to be used for long-term cortical control of an artificial device and is a low-frequency signal (<250 Hz) consisting of the sum of all the electrical activity in the region adjacent to the tip of the electrodes implanted in the cortex.
To date, most LFP-based BCI research has been carried out based on typical center-out arm movements (Rickert et al., 2005; Heldman et al., 2006; Wang, 2006), and some studies have employed point-to-point motor tasks (Ahmadi et al., 2021; Zhang and Constandinou, 2023). It has been shown that the M1 tune encodes movement direction (Georgopoulos et al., 1986) and velocity. There is a modest decrease in β-LFP amplitude beforehand movement and a large increase in HF-LFP spectral amplitude during hand movement. The time-domain characterization of LFP can be used to control computer cursors (Kennedy et al., 2004) and to encode motion parameters (Rickert et al., 2005; Ahmadi et al., 2021). Examples of the main existing LFP-BCI paradigms with neural coding studies are shown in Supplementary Table 1.
4.2 ECoG-BCI paradigms and neural coding
Electroencephalogram is the recording of the overall activity of a large population of neurons in a localized area by electrodes placed on the supernatural or subdural cortex, with time and spatial resolution of a few milliseconds and millimeters (ECoG is superior to MEG and EEG) (Katzner et al., 2009; Dubey and Ray, 2019), which is less affected by muscle activity and ocular artifacts (Ball et al., 2009), The ECoG has an excellent noise ratio. These advantages favor the coding of stimuli or mental tasks so that potential brain signal features are found to be well discriminated, Therefore, the brain imaging technique of ECoG is better suited for BCI.
The ECoG-BCI frequency coding model is the ECoG power spectrum associated with a specific event (stimulus or mental task), and studies have shown that the selection of appropriate electrodes and power can encode movement trajectories (Jang et al., 2022), and its temporal coding model is the peaks of the raw ECoG signals when time-locked to a specific event (a specific amount of time after stimulus presentation). It has been shown that ECoG high-frequency broadband (200–300 Hz) power variations carry a great deal of information about brain function and are coding information of robustness (Hermes et al., 2011, 2012; Siero et al., 2013, 2014; Vansteensel et al., 2016). In addition, visual, auditory, and tactile evoked ECoG potentials (Brunner et al., 2011; Hermes et al., 2015, 2017; Wittevrongel et al., 2018) and ECoG narrow-band (α, β, and γ) power variations (Crone et al., 1998; Pfurtscheller et al., 2003a; Miller et al., 2007; Kubánek et al., 2009; Brunner et al., 2011; Gunduz et al., 2012; Hermes et al., 2012, 2014; Burke et al., 2013, 2015; Brinkman et al., 2014, 2016) can characterize the function of specific brain regions and brain circuits, Their combination with high-frequency broad-band (Miller et al., 2009, 2016) power changes can sometimes improve decoding performance. Examples of the main existing ECoG-BCI paradigms with neural coding studies are shown in Supplementary Table 2.
4.3 fMRI-BCI paradigms and neural coding
Functional magnetic resonance imaging (Ogawa et al., 1990) has high spatial resolution (Logothetis et al., 2001), better robustness and user-friendliness, and individualized flexibility. The ability of fMRI to measure deep brain region structure and activity, map functional connectivity networks, and allow the use of amygdala and ventral striatum with BCI neurofeedback for user (Mehler et al., 2018) has become a core technique for mapping neuroplasticity (Seitz, 2010).
Spatial localization of brain functions using the fMRI-BCI space coding model to produce spatially distinct patterns of brain activation by engaging different combinations of brain regions during the time that subjects are receiving external stimuli or intentionally performing different mental activities (Yoo et al., 2004; Boly et al., 2007; Monti et al., 2010; Senden et al., 2019). Models of fMRI-BCI time coding reliably detect the onset, offset, and duration of single-trial fMRI responses evoked by various mental activities, which is the assignment of specific coding intervals for specific intentions (Monti et al., 2010). Models of fMRI-BCI amplitude coding using different fMRI signal levels within specific brain regions to encode different movement intentions (Yoo et al., 2001). The combination of fMRI-BCI hybrid coding models using the above signal features (spatial, temporal, and amplitude) can increase the degree of freedom to encode different units of information or increase the distinguishability of the evoked brain activation patterns, thus maximizing the decoding accuracy (Sorger et al., 2012). Examples of the main existing fMRI -BCI paradigms with neural coding studies are shown in Supplementary Table 3.
4.4 fNIRS-BCI paradigms and neural coding
Functional near-infrared spectroscopy measures the hemodynamic response of brain tissue during resting state (Abdalmalak et al., 2021; Paulmurugan et al., 2021; Eastmond et al., 2022), external stimulus, and mental activity, including changes in oxy-hemoglobin (HbO) and deoxyhemoglobin (HbR) concentration, with the main advantage of good portability, tolerating a certain degree of head movement of the subject, and a favorable ecological effect. fNIRS-BCI can also be used to facilitate the rehabilitation of patients with motor dysfunction and/or cognitive dysfunction, such as those suffering from stroke and spinal cord injury.
The fNIRS-BCI time coding model extracts time-domain features of hemodynamic responses associated with a specific event (such as external stimulus or mental task), for example, the mean, peak, and variance of HbO and HbR (Holper and Wolf, 2011; Hwang et al., 2014; Naseer et al., 2014; Hong et al., 2015). Models of fNIRS -BCI amplitude coding using different fNIRS signal levels within specific brain regions to encode different movement intentions (Coyle et al., 2007; Kaiser et al., 2014). Examples of the main existing fNIRS -BCI paradigms with neural coding studies are shown in Supplementary Table 4.
4.5 MEG-BCI paradigms and neural coding
Magnetoencephalography is a non-invasive neuroimaging technique for detecting weak magnetic field changes generated by the electrical activity of central neurons (Xu et al., 2022; Bu et al., 2023). The technique has high time (less than 1 ms) and spatial [2–5 μm (Cetin and Temurtas, 2021)] resolution and low sensitivity to artifacts generated by muscle activity, but its comfort, aesthetics, and ease of use leave much to be desired.
The MEG-BCI time coding model characterizes mental tasks or external stimuli by information such as the peaks of the time-domain waveforms of the MEG signals as well as the time points. The frequency coding model characterizes a specific event (mental activity or external stimulus) by the MEG power spectral features (Mellinger et al., 2007; Chen and Bai, 2009; Halme and Parkkonen, 2016). MEG signals are complex non-linear and non-stationary signals, which the single time or frequency coding model will lose some feature information, and a time-frequency coding model can be used to obtain the relationship between signal frequency over time (Chholak et al., 2019). MEG-BCI space coding models can be used to downsize the data using spatial filtering methods to differentiate between mental activities or external stimuli (Rathee et al., 2021). Examples of the main existing MEG-BCI paradigms with neural coding studies are shown in Supplementary Table 5.
4.6 EEG-BCI paradigms and neural coding
4.6.1 MI paradigms and neural coding
Mental tasks involved in MI paradigms include slow, non-fine, non-dexterous MI, fast, fine, and highly dexterous MI, MI involving a unilateral limb, coordinated MI involving multiple limbs, a single or repetitive MI, as well as kinematics or kinetics parameters imagery. The neural coding of MI paradigms can be encoded using (1) time-domain features such as movement-related potential (MRP) or movement-related cortical potential (MRCP) (movement preparation potentials, movement monitoring potentials, and end-of-movement rebound potentials); (2) frequency-domain features coding, such as neural oscillatory power change features of μ/β/γ and other rhythms, which are commonly used event-related-desynchronization/synchronization (ERD/ERS); and (3) space-domain features coding, such as the primary motor area of the hemispheres, the auxiliary motor area, and the premotor area. In addition, the neural coding of some MI paradigms has yet to be studied in depth.
4.6.1.1 Slow, non-fine, non-dexterous MI
Slow, non-fine, and non-dexterous movements usually involve gross limb movements that do not require rapidity, fine coordination, and a high degree of dexterity.
Slow movements are slow and do not require rapid responses or high rates of execution, which include slow walking, strolling, and soothing stretches are all slow movements (Pfurtscheller et al., 2003b; Müller-Putz et al., 2007). Non-fine movements do not require a high degree of fine coordination or precise control of fine muscle groups. Comparatively, they favor holistic and basic movements. Such as simple hand lifting, striding, and simple dance movements (Pfurtscheller et al., 1997; Ramoser et al., 2000; Kaiser et al., 2014). Non-dexterous movements do not require high skills or complex combinations of movements. They focus more on the simplicity and ease of realization of the movements. For example, balancing simple objects, simple stretching, and bending movements (Neuper et al., 2009).
4.6.1.2 Fast, fine, and highly dexterous movement imagery
Fast, fine, and highly dexterous movements usually involve the movement of fine limbs, the execution of which requires fast, accurate movements with a high degree of skill and coordination These types of movements often require long periods of training and practice to achieve a high level of skill.
Rapid movements are executed at a high speed. It requires rapid reaction and movement execution. The users can react quickly in a short period and complete the movement at a high rate. Fine movements require a high degree of precision and care. The users need to control the movement accurately, including the coordination of small muscle groups and precise handling of details. Highly dexterous movements demonstrate exceptional skill and flexibility. The performer can perform the movement with grace and agility. Implantable acquisition of brain signals with high space resolution is usually used to encode and decode such movements, and scalp EEG makes it difficult to encode and decode such MI with stability and high precision.
4.6.1.3 MI involving the unilateral limb
In daily life, some movements involve only the unilateral limb, such as tapping movements of the right or left index finger, internal or external rotation of the wrist, flexion or extension of the wrist, clenching of the fist, pinching of the thumb against the other fingers, and extension of the arm. These movement exercises favor unilateral limb strength, balance, and coordination, and help improve symmetry and motor control (Pfurtscheller et al., 1997; Ramoser et al., 2000; Neuper et al., 2009; Hashimoto and Ushiba, 2013). The difficulty of recognizing different imagined movements in a single limb is greater compared to the recognition of imagined movements in different limbs. For example, recognizing various imagined movements in the affected limb of hemiparetic patients poses a greater challenge.
4.6.1.4 Coordinated MI involving multiple limbs
In daily life, coordinated movement usually involves synergistic movements of multiple limbs to cooperate in time and space to effectively accomplish a desired task (Zhang et al., 2022). Examples include walking and threading a needle. These types of movements usually require some training making good coordination and overall control between limbs (Müller-Putz et al., 2007; Hashimoto and Ushiba, 2013). The neural and decoding of coordinated motor involving at least two limbs is to be studied in depth.
4.6.1.5 A single or repetitive MI
A single MI BCI paradigm is different from the repetitive or continuous MI BCI paradigm, and the BCI coding is different. The Synchronous MI-BCI requires subjects to perform imagery tasks according to a temporal sequence designed by the researcher, and it is typically used to build classification models. The asynchronous MI-BCI is much more challenging, where subjects’ imaginative mental activity can be self-paced rather than performing the imaginative task according to a temporal sequence designed by the researcher.
4.6.1.6 Kinematic or kinetic parameters imagery
The kinematic parameters of the limb include the velocity of movement, trajectory of movement, and time of movement. The kinetic parameters of the limb include the driving force of movement and acceleration of movement. For example, the speed of the right index finger tap (such as slow, medium, and fast), reaching and grasping processes, space navigation, and grip size (Flint et al., 2022). Compared to kinematic parameter imagery, kinetic parameter imagery coding and decoding have been relatively less studied and more difficult.
4.6.2 External stimulus paradigms and neural coding
4.6.2.1 P300-BCI paradigms and neural coding
In the P300-BCI paradigm, the probability of a target/target stimulus (novel stimulus with small probability) is no more than 20%, and the probability of a non-target stimulus (standard stimulus with large probability) is no less than 80%. When a user is exposed to a target stimulus during 220–500 ms (latency) a positive peak of 5–10 microvolts is induced, most significant at the midline location (Pz, Cz, and Fz in the 10/20 international system). This component characterizes the target stimulus. The visual P300-BCI speller was first implemented by Farwell and Donchin (1988), and subsequently, there have been many variants of the P300-BCI paradigm, mainly differences in visual stimulus characterization and presentation. In addition to the visual P300-BCI paradigm, there are also auditory P300-BCI (Furdea et al., 2009; Klobassa et al., 2009) and tactile P300-BCI (Müller-Putz et al., 2006; Brouwer and Van Erp, 2010).
Although the P300-BCI can provide effective input of characters, the practicality still faces challenges. The system’s online transmission rate is low, which makes it difficult to meet the current real-time needs. The inseparability of external stimuli is tied to attention such as vision and hearing, and offline training tends to be prolonged, which causes fatigue to the users.
4.6.2.2 SSVEP-BCI paradigms and neural coding
In the SSVEP-BCI paradigm, when a subject gazes at a visual stimulus of a certain flicker frequency [low band (<12 Hz), middle band (12–30 Hz), and high band (>30 Hz)], a stabilizing potential component of the same frequency as the stimulus frequency or its higher harmonic frequencies is induced. The SSVEP-BCI paradigm can be traced back to as early as a 1995 conference report (McMillan et al., 1995), but is not the paradigm that is now commonly used; the paradigm that is now commonly used comes from a 1999 conference report (Ming and Shangkai, 1999). Subsequently, the SSVEP-BCI paradigm has many innovative designs such as frequency modulation combined with phase and amplitude modulation (Chen et al., 2015; Zhang et al., 2021).
Although SSVEP-BCI has high performance (for example, significant features, stable amplitude, high interference immunity, high information transfer rate, less training, and large instruction set), it requires highly accurate eye control (Herrmann, 2001; Chang et al., 2014), and may lead to subject fatigue when using low blinking frequency (Molina and Mihajlovic, 2010; Müller et al., 2011; Volosyak et al., 2011) the interaction of SSVEP is unnatural, and the user’s satisfaction remains to be further improved. SSVEP-BCIs must essentially have flickering external visual stimuli, and thus cannot be separated from visual attention. Examples of the main existing EEG-BCI paradigms with neural coding studies are shown in Supplementary Table 6.
4.7 hBCI paradigms and coding
A hybrid Brain-Computer Interface (hBCI) aims to improve the usability or efficacy of BCI systems hBCI consists of a mix of a BCI system (the main system) and an add-on system that assists the BCI (Li et al., 2019). which can be a non-external stimulus or non-psychological task-driven system, or an AI system (e.g., a deep learning-based machine vision or computer vision system) can be mixed to improve the accuracy of target recognition by the main system BCI and increase the number of brain-controlled/other commands. As shown in Figure 5.
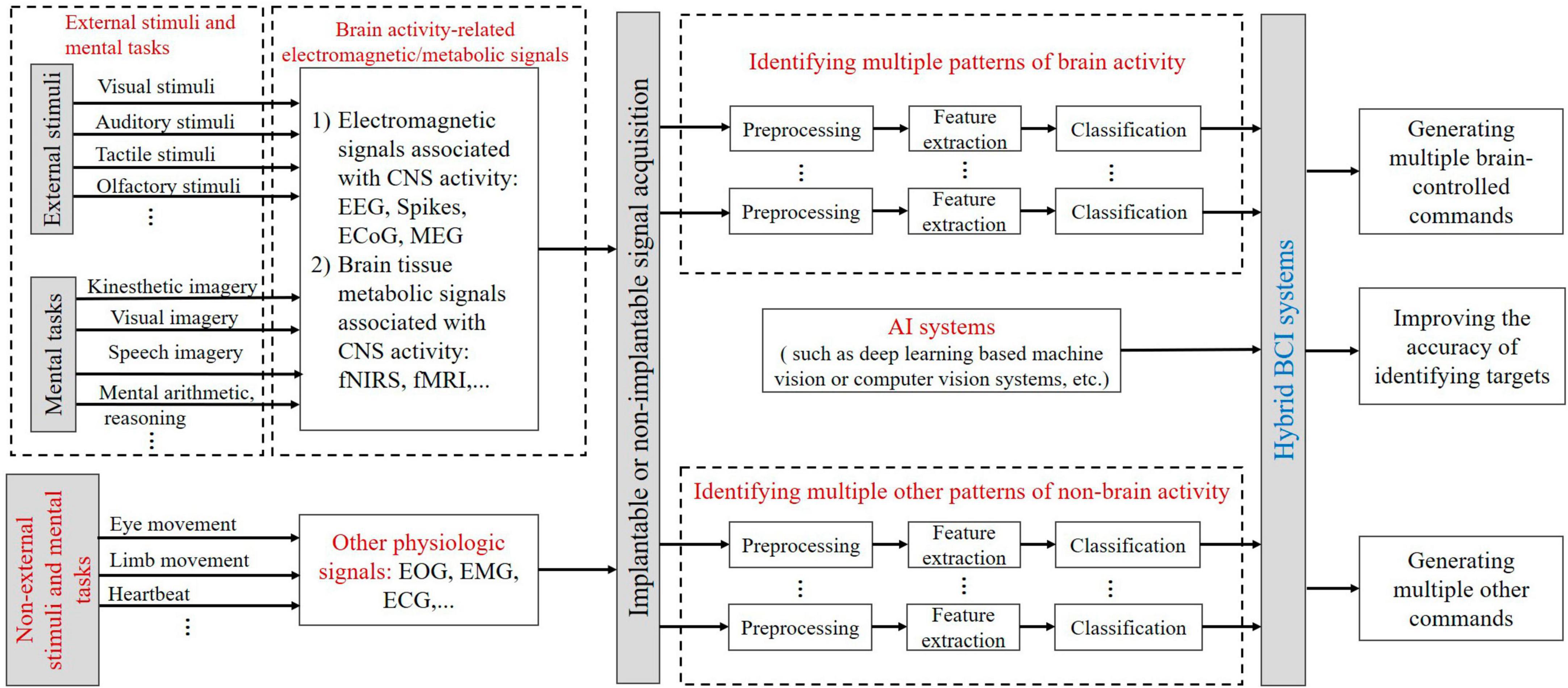
Figure 5. The schematic for hybrid BCI paradigms and coding. EOG, electrooculogram; EMG, electromyogram; ECG, electrocardiogram.
As can be seen from Figure 5, BCI paradigms of the main system can be subsets of different external stimuli and mental tasks. For example, the P300 paradigm can be designed by visual, auditory, tactile, or olfactory stimuli according to the Oddball paradigm. The P300 paradigm, the SSVEP paradigm, and the kinesthetic imagery paradigm can be combined. BCI paradigms of the main system induce electromagnetic/metabolic activity signals related to brain activity, and multiple brain activity patterns in these brain signals can encode external stimuli and mental tasks. Non-external stimuli and mental tasks such as eye movement, limb movement, or heartbeat in the additional system can be characterized by other non-brain activity physiological signal patterns. The AI system in the add-on system can enhance the level of intelligence of the main BCI system.
5 Challenges and future research directions for BCI paradigms and neural coding
So far, existing BCI paradigms and neural codes have limitations that hinder the translational application of BCI. For this reason, the innovative design and improvement of BCI paradigms and neural codes is one of the key tasks in the development of BCI systems.
5.1 User-centered design and evaluation for BCI paradigms and neural coding
The end user of BCI is the user, while the user itself is the source of the CNS signals that drive the BCI. The user is the most complex, active, and highly adaptive subsystem essential to the BCI system. Therefore, BCI systems are the most typical human-in-the-loop systems (the human brain is directly connected or coupled to the machine, a closed-loop system with direct brain-machine interaction), and the design and evaluation (usability and satisfaction) of BCI paradigms and coding need to be user-centered, taking into account BCI human factors engineering (Lyu et al., 2023).
Brain-computer interface paradigms and neural coding are closely related to the neural mechanisms of the user’s mental activities/tasks (Liberati et al., 2015). The performance of a BCI system (such as effectiveness and efficiency) is closely related to the user’s mental activity, such that the performance of a movement-imagery BCI system is largely dependent on the user’s effectiveness or ability to perform MI (Kübler et al., 2014; Martin et al., 2018).
It is worth noting that to evaluate the first principle of BCI paradigm design proposed in section 2.2, which states that CNS signals evoked by BCI paradigm specific tasks should have good separability, any innovatively designed BCI paradigm, and neural coding model typically requires offline data analysis and model establishment, and ultimately must be validated and evaluated by neural decoding in an online BCI system.
5.2 Revolutionizing the traditional BCI paradigms
The BCI paradigm, from a perspective of communication principles and technology, is a coding protocol in which brain intentions are encoded into signals generated by neural activity through specific external stimuli or mental tasks.
So far, BCI has been developed for more than 50 years. However, current BCI paradigms are more limited, and the transformation faces great challenges, which need to significantly improve, we need to break through the traditional classical BCI paradigms (such as SSVEP-BCI, P300-BCI, and MI-BCI), and add new BCI paradigms that are more natural and more effective to interact with the user. In recent years, many important advances have been made in the innovation of BCI paradigms (Willett et al., 2021, 2023; Metzger et al., 2023).
5.2.1 Speech-BCI paradigm
Speech is the primary mode of human communication, and the speech BCI paradigm is one of the more natural BCI paradigms. Speech BCI has the potential to decode the neural activity triggered by attempted speech into text or sound, thus promising to restore rapid communication for paralyzed patients (Metzger et al., 2023; Willett et al., 2023).
5.2.2 Handwriting imagery-BCI paradigm for input text
To date, a major focus of BCI research has been the restoration of motor skills to gross limbs such as reaching and grasping or typing with computer cursor clicks. Willett et al. (2021) developed an intracortical brain-computer interface that decodes attempted handwriting actions via neural activity in the hand junction area of the motor cortex and uses a recursive neural network decoding method to translate neural activity in the motor cortex into text in real-time.
5.3 Breaking through the existing techniques for collecting brain signals
The performances of the BCI paradigm and neural coding are directly related to the level of brain signal collection technology, which requires a breaking through of brain signal collection technology. How brain signals are acquired is crucial for the BCI paradigm and neural coding, which is related to the quality of the collected signals and the final BCI control effect. With the continuous development of micro-nano processing technology and electrode materials, electrodes for invasive BCI tend to be flexible, miniaturized, high-throughput, and integrated. Currently, the research and development of hydrogel EEG electrodes are more active (Xue et al., 2023), stereotactic EEG (sEEG) (Herff et al., 2020), and in-ear EEG electrodes (Wang et al., 2023) have also made positive progress. In addition, minimally invasive endovascular stent-electrode techniques (Oxley et al., 2016, 2021), minimally invasive local-skull electrophysiological modification methods (Sun et al., 2022), and other schemes have been proposed to innovate brain signal acquisition.
5.4 Combining BCI technology with advanced AI technology to improve brain signal decoding performance
Currently, classical machine learning still has an advantage in BCI neural decoding, but deep learning also has the potential to enhance BCI decoding performance. Some studies have introduced suitable deep learning algorithms in decoding brain signals, and these studies show that BCI technology combined with advanced AI techniques is expected to significantly improve brain signal decoding performance (Willett et al., 2021, 2023; Metzger et al., 2023). Figure 6 illustrates the introduction of AI into BCI to improve the intelligence of BCI and facilitate the clinical translational application of BCI.
6 Conclusion
In the BCI technology system, BCI paradigms and neural coding are some of the key and important contents of BCI research and development. In the paper, the definition of BCI paradigms and seven design principles, as well as the definition and coding model of BCI neural coding, including BCI frequency/rate coding, time coding, phase coding, intracortical neuron population coding, correlation coding, sparse coding, and hybrid coding model are shown more systematically and clearly. The existing main BCI paradigms and neural coding are presented, including intracortical LFP-BCI, ECoG-BCI, fNIRS-BCI, fMRI-BCI, MEG-BCI, EEG-BCI, and hybrid BCI paradigms and neural coding. Finally, user-centered design and evaluation for BCI paradigms and neural coding, revolutionizing the traditional BCI paradigms, breaking through the existing techniques for collecting brain signals and combining BCI technology with advanced AI technology to improve brain signal decoding performance are discussed. It is expected that this paper will inspire the innovative research and development of BCI paradigms and neural coding.
Author contributions
PT: Writing—original draft. PD: Investigation, Writing—review and editing, Conceptualization, Project administration, Validation. FW: Investigation, Writing—review and editing. AG: Validation, Writing—review and editing. TL: Validation, Writing—review and editing. LZ: Validation, Writing—review and editing. LS: Validation, Writing—review and editing. YF: Conceptualization, Funding acquisition, Investigation, Project administration, Supervision, Validation, Writing—review and editing.
Funding
The author(s) declare financial support was received for the research, authorship, and/or publication of this article. This study was partially funded by the National Natural Science Foundation of China (Grant Nos. 82172058, 62376112, 81771926, 61763022, and 62366026).
Conflict of interest
The authors declare that the research was conducted in the absence of any commercial or financial relationships that could be construed as a potential conflict of interest.
Publisher’s note
All claims expressed in this article are solely those of the authors and do not necessarily represent those of their affiliated organizations, or those of the publisher, the editors and the reviewers. Any product that may be evaluated in this article, or claim that may be made by its manufacturer, is not guaranteed or endorsed by the publisher.
Supplementary material
The Supplementary Material for this article can be found online at: https://www.frontiersin.org/articles/10.3389/fnins.2023.1345961/full#supplementary-material
References
Abdalmalak, A., Milej, D., Norton, L., Debicki, D. B., Owen, A. M., and Lawrence, K. S. (2021). The potential role of fNIRS in evaluating levels of consciousness. Front. Hum. Neurosci. 15:703405. doi: 10.3389/fnhum.2021.703405
Abiri, R., Borhani, S., Sellers, E. W., Jiang, Y., and Zhao, X. (2019). A comprehensive review of EEG-based brain–computer interface paradigms. J. Neural Eng. 16:011001.
Ahmadi, N., Constandinou, T. G., and Bouganis, C. S. (2021). Impact of referencing scheme on decoding performance of LFP-based brain-machine interface. J. Neural Eng. 18:016028. doi: 10.1088/1741-2552/abce3c
Allison, B. Z., Dunne, S., Leeb, R., Millan, J., and Nijholt, A. (2012). Towards practical brain-computer interfaces: Bridging the gap from research to real-world applications. Berlin: Springer Science & Business Media.
Ball, T., Kern, M., Mutschler, I., Aertsen, A., and Schulze-Bonhage, A. (2009). Signal quality of simultaneously recorded invasive and non-invasive EEG. Neuroimage 46, 708–716.
Bashashati, A., Fatourechi, M., Ward, R. K., and Birch, G. E. (2007). A survey of signal processing algorithms in brain–computer interfaces based on electrical brain signals. J. Neural Eng. 4:R32.
Boly, M., Coleman, M. R., Davis, M. H., Hampshire, A., Bor, D., Moonen, G., et al. (2007). When thoughts become action: An fMRI paradigm to study volitional brain activity in non-communicative brain injured patients. Neuroimage 36, 979–992. doi: 10.1016/j.neuroimage.2007.02.047
Branco, M. P., Geukes, S. H., Aarnoutse, E. J., Ramsey, N. F., and Vansteensel, M. J. (2023). Nine decades of electrocorticography: A comparison between epidural and subdural recordings. Eur. J. Neurosci. 57, 1260–1288. doi: 10.1111/ejn.15941
Branco, M. P., Pels, E. G., Sars, R. H., Aarnoutse, E. J., Ramsey, N. F., Vansteensel, M. J., et al. (2021). Brain-computer interfaces for communication: Preferences of individuals with locked-in syndrome. Neurorehabil. Neural Repair 35, 267–279.
Brinkman, L., Stolk, A., Dijkerman, H. C., Lange, F. P., and Toni, I. (2014). Distinct roles for alpha-and beta-band oscillations during mental simulation of goal-directed actions. J. Neurosci. 34, 14783–14792. doi: 10.1523/JNEUROSCI.2039-14.2014
Brinkman, L., Stolk, A., Marshall, T. R., Esterer, S., Sharp, P., Dijkerman, H. C., et al. (2016). Independent causal contributions of alpha-and beta-band oscillations during movement selection. J. Neurosci. 36, 8726–8733. doi: 10.1523/JNEUROSCI.0868-16.2016
Brouwer, A. M., and Van Erp, J. B. (2010). A tactile P300 brain-computer interface. Front. Neurosci. 4:1440. doi: 10.3389/fnins.2010.00019
Brown, E. N., Kass, R. E., and Mitra, P. P. (2004). Multiple neural spike train data analysis: State-of-the-art and future challenges. Nat. Neurosci. 7, 456–461. doi: 10.1038/nn1228
Brunner, P., Ritaccio, A. L., Emrich, J. F., Bischof, H., and Schalk, G. (2011). Rapid communication with a “P300” matrix speller using electrocorticographic signals (ECoG). Front. Neurosci. 5:5. doi: 10.3389/fnins.2011.00005
Bu, Y., Harrington, D. L., Lee, R. R., Shen, Q., Angeles-Quinto, A., Ji, Z., et al. (2023). Magnetoencephalogram-based brain–computer interface for hand-gesture decoding using deep learning. Cereb. Cortex 33, 8942–8955. doi: 10.1093/cercor/bhad173
Burke, J. F., Merkow, M. B., Jacobs, J., Kahana, M. J., and Zaghloul, K. A. (2015). Brain computer interface to enhance episodic memory in human participants. Front. Hum. Neurosci. 8:1055. doi: 10.3389/fnhum.2014.01055
Burke, J. F., Zaghloul, K. A., Jacobs, J., Williams, R. B., Sperling, M. R., Sharan, A. D., et al. (2013). Synchronous and asynchronous theta and gamma activity during episodic memory formation. J. Neurosci. 33, 292–304. doi: 10.1523/JNEUROSCI.2057-12.2013
Butts, D. A., Weng, C., Jin, J., Yeh, C., Lesica, N. A., Alonso, J., et al. (2007). Temporal precision in the neural code and the timescales of natural vision. Nature 449, 92–95.
Carleton, A., Accolla, R., and Simon, S. A. (2010). Coding in the mammalian gustatory system. Trends Neurosci. 33, 326–334.
Cetin, O., and Temurtas, F. (2021). A comparative study on classification of magnetoencephalography signals using probabilistic neural network and multilayer neural network. Soft Comput. 25, 2267–2275.
Chang, M. H., Baek, H. J., Lee, S. M., and Park, K. S. (2014). An amplitude-modulated visual stimulation for reducing eye fatigue in SSVEP-based brain–computer interfaces. Clin. Neurophysiol. 125, 1380–1391. doi: 10.1016/j.clinph.2013.11.016
Chavarriaga, R., Fried-Oken, M., Kleih, S., Lotte, F., and Scherer, R. (2017). Heading for new shores! Overcoming pitfalls in BCI design. Brain Comput. Interfaces 4, 60–73. doi: 10.1080/2326263X.2016.1263916
Chen, G., Wang, L. P., and Tsien, J. Z. (2009). Neural population-level memory traces in the mouse hippocampus. PLoS One 4:e8256. doi: 10.1371/journal.pone.0008256
Chen, X., and Bai, O. (2009). “Towards multi-dimensional robotic control via noninvasive brain-computer interface,” in Proceedings of the 2009 ICME international conference on complex medical engineering, (Piscataway, NJ), 1–5.
Chen, X., Wang, Y., Gao, S., Jung, T., and Gao, X. (2015). Filter bank canonical correlation analysis for implementing a high-speed SSVEP-based brain–computer interface. J. Neural Eng. 12:046008. doi: 10.1088/1741-2560/12/4/046008
Chholak, P., Niso, G., Maksimenko, V. A., Kurkin, S. A., Frolov, N. S., Pitsik, E. N., et al. (2019). Visual and kinesthetic modes affect motor imagery classification in untrained subjects. Sci. Rep. 9:9838. doi: 10.1038/s41598-019-46310-9
Choi, I., Rhiu, I., Lee, Y., Yun, M. H., and Nam, C. S. (2017). A systematic review of hybrid brain-computer interfaces: Taxonomy and usability perspectives. PLoS One 12:e0176674. doi: 10.1371/journal.pone.0176674
Coyle, S. M., Ward, T. E., and Markham, C. M. (2007). Brain–computer interface using a simplified functional near-infrared spectroscopy system. J. Neural Eng. 4:219.
Crochet, S., Poulet, J. F., Kremer, Y., and Petersen, C. C. (2011). Synaptic mechanisms underlying sparse coding of active touch. Neuron 69, 1160–1175. doi: 10.1016/j.neuron.2011.02.022
Crone, N. E., Miglioretti, D. L., Gordon, B., and Lesser, R. P. (1998). Functional mapping of human sensorimotor cortex with electrocorticographic spectral analysis. II. Event-related synchronization in the gamma band. Brain 121, 2301–2315. doi: 10.1093/brain/121.12.2301
Davis, G. M., Mallat, S. G., and Zhang, Z. (1994). Adaptive time-frequency decompositions. Opt. Eng. 33, 2183–2191.
Decharms, R. C., and Merzenich, M. M. (1996). Primary cortical representation of sounds by the coordination of action-potential timing. Nature 381, 610–613.
Du, C., Li, J., Huang, L., and He, H. (2019). Brain encoding and decoding in fMRI with bidirectional deep generative models. Engineering 5, 948–953.
Dubey, A., and Ray, S. (2019). Cortical electrocorticogram (ECoG) is a local signal. J. Neurosci. 39, 4299–4311.
Eastmond, C., Subedi, A., De, S., and Intes, X. (2022). Deep learning in fNIRS: A review. Neurophotonics 9, 041411–041411.
Farwell, L. A., and Donchin, E. (1988). Talking off the top of your head: Toward a mental prosthesis utilizing event-related brain potentials. Electroencephalogr. Clin. Neurophysiol. 70, 510–523. doi: 10.1016/0013-4694(88)90149-6
Flint, R. D., Li, Y., Wang, P., Vaidya, M., Barry, A., Ghassemi, M., et al. (2022). Noninvasively recorded high-gamma signals improve synchrony of force feedback in a novel neurorehabilitation brain–machine interface for brain injury. J. Neural Eng. 19:036024. doi: 10.1088/1741-2552/ac7004
Furdea, A., Halder, S., Krusienski, D. J., Bross, D., Nijboer, F., Birbaumer, N., et al. (2009). An auditory oddball (P300) spelling system for brain-computer interfaces. Psychophysiology 46, 617–625. doi: 10.1111/j.1469-8986.2008.00783.x
Georgopoulos, A. P., Schwartz, A. B., and Kettner, R. E. (1986). Neuronal population coding of movement direction. Science 233, 1416–1419.
Gerstner, W., and Kistler, W. M. (2002). Spiking neuron models: Single neurons, populations, plasticity. Cambridge: Cambridge University Press.
Gollisch, T., and Meister, M. (2008). Rapid neural coding in the retina with relative spike latencies. Science 319, 1108–1111. doi: 10.1126/science.1149639
Graimann, B., Allison, B., and Pfurtscheller, G. (2013). Brain-computer interfaces: Revolutionizing human-computer interaction. Berlin: Springer Publishing Company.
Gunduz, A., Brunner, P., Daitch, A., Leuthardt, E. C., Ritaccio, A. L., Pesaran, B., et al. (2012). Decoding covert spatial attention using electrocorticographic (ECoG) signals in humans. Neuroimage 60, 2285–2293. doi: 10.1016/j.neuroimage.2012.02.017
Halme, H. L., and Parkkonen, L. (2016). Comparing features for classification of MEG responses to motor imagery. PLoS One 11:e0168766. doi: 10.1371/journal.pone.0168766
Hashimoto, Y., and Ushiba, J. (2013). EEG-based classification of imaginary left and right foot movements using beta rebound. Clin. Neurophysiol. 124, 2153–2160. doi: 10.1016/j.clinph.2013.05.006
Havenith, M. N., Yu, S., Biederlack, J., Chen, N. H., Singer, W., and Nikolić, D. (2011). Synchrony makes neurons fire in sequence, and stimulus properties determine who is ahead. J. Neurosci. 31, 8570–8584. doi: 10.1523/JNEUROSCI.2817-10.2011
Heldman, D. A., Wang, W., Chan, S. S., and Moran, D. W. (2006). Local field potential spectral tuning in motor cortex during reaching. IEEE Trans. Neural Syst. Rehabil. Eng. 14, 180–183.
Herff, C., Krusienski, D. J., and Kubben, P. (2020). The potential of stereotactic-EEG for brain-computer interfaces: Current progress and future directions. Front. Neurosci. 14:123. doi: 10.3389/fnins.2020.00123
Hermes, D., Miller, K. J., Vansteensel, M. J., Edwards, E., Ferrier, C. H., Bleichner, M. G., et al. (2014). Cortical theta wanes for language. Neuroimage 85, 738–748. doi: 10.1016/j.neuroimage.2013.07.029
Hermes, D., Miller, K. J., Wandell, B. A., and Winawer, J. (2015). Stimulus dependence of gamma oscillations in human visual cortex. Cereb. Cortex 25, 2951–2959.
Hermes, D., Siero, J. C., Aarnoutse, E. J., Leijten, F. S., Petridou, N., and Ramsey, N. F. (2012). Dissociation between neuronal activity in sensorimotor cortex and hand movement revealed as a function of movement rate. J. Neurosci. 32, 9736–9744.
Hermes, D., Trenité, D. G., and Winawer, J. (2017). Gamma oscillations and photosensitive epilepsy. Curr. Biol. 27, R336–R338.
Hermes, D., Vansteensel, M. J., Albers, A. M., Bleichner, M. G., Benedictus, M. R., Orellana, C. M., et al. (2011). Functional MRI-based identification of brain areas involved in motor imagery for implantable brain–computer interfaces. J. Neural Eng. 8:025007. doi: 10.1088/1741-2560/8/2/025007
Herrmann, C. S. (2001). Human EEG responses to 1–100 Hz flicker: Resonance phenomena in visual cortex and their potential correlation to cognitive phenomena. Exp. Brain Res. 137, 346–353. doi: 10.1007/s002210100682
Hill, N. J., Gupta, D., Brunner, P., Gunduz, A., Adamo, M. A., Ritaccio, A., et al. (2012). Recording human electrocorticographic (ECoG) signals for neuroscientific research and real-time functional cortical mapping. J. Vis. Exp. 26:e3993. doi: 10.3791/3993
Hochberg, L. R., Bacher, D., Jarosiewicz, B., Masse, N. Y., Simeral, J. D., Vogel, J., et al. (2012). Reach and grasp by people with tetraplegia using a neurally controlled robotic arm. Nature 485, 372–375.
Holper, L., and Wolf, M. (2011). Single-trial classification of motor imagery differing in task complexity: A functional near-infrared spectroscopy study. J. Neuroeng. Rehabil. 8, 1–13. doi: 10.1186/1743-0003-8-34
Hong, K. S., Naseer, N., and Kim, Y. H. (2015). Classification of prefrontal and motor cortex signals for three-class fNIRS–BCI. Neurosci. Lett. 587, 87–92. doi: 10.1016/j.neulet.2014.12.029
Hromádka, T., DeWeese, M. R., and Zador, A. M. (2008). Sparse representation of sounds in the unanesthetized auditory cortex. PLoS Biol. 6:e16. doi: 10.1371/journal.pbio.0060016
Hwang, H., Lim, J., Kim, D., and Im, C. (2014). Evaluation of various mental task combinations for near-infrared spectroscopy-based brain-computer interfaces. J. Biomed. Opt. 19:077005. doi: 10.1117/1.JBO.19.7.077005
Ito, I., Ong, R. C., Raman, B., and Stopfer, M. (2008). Sparse odor representation and olfactory learning. Nat. Neurosci. 11, 1177–1184.
Jang, S. J., Yang, Y. J., Ryun, S., Kim, J. S., Chung, C. K., and Jeong, J. (2022). Decoding trajectories of imagined hand movement using electrocorticograms for brain–machine interface. J. Neural Eng. 19:056011.
Jolivet, R., Rauch, A., Lüscher, H., and Gerstner, W. (2006). Predicting spike timing of neocortical pyramidal neurons by simple threshold models. J. Comput. Neurosci. 21, 35–49. doi: 10.1007/s10827-006-7074-5
Kaiser, V., Bauernfeind, G., Kreilinger, A., Kaufmann, T., Kübler, A., Neuper, C., et al. (2014). Cortical effects of user training in a motor imagery based brain–computer interface measured by fNIRS and EEG. Neuroimage 85, 432–444. doi: 10.1016/j.neuroimage.2013.04.097
Kandel, E. R., Koester, J. D., Sarah, H., Mack, S. H., and Siegelbaum, S. A. (2000). Principles of neural science. New York, NY: McGraw-Hill.
Katzner, S., Nauhaus, I., Benucci, A., Bonin, V., Ringach, D. L., and Carandini, M. (2009). Local origin of field potentials in visual cortex. Neuron 61, 35–41.
Kennedy, P. R., Kirby, M. T., Moore, M. M., King, B., and Mallory, A. (2004). Computer control using human intracortical local field potentials. IEEE Trans. Neural Syst. Rehabil. Eng. 12, 339–344.
Klobassa, D. S., Vaughan, T. M., Brunner, P., Schwartz, N. E., Wolpaw, J. R., Neuper, C., et al. (2009). Toward a high-throughput auditory P300-based brain–computer interface. Clin. Neurophysiol. 120, 1252–1261. doi: 10.1016/j.clinph.2009.04.019
Kostal, L., Lansky, P., and Rospars, J. P. (2007). Neuronal coding and spiking randomness. Eur. J. Neurosci. 26, 2693–2701.
Kubánek, J., Miller, K. J., Ojemann, J. G., Wolpaw, J. R., and Schalk, G. (2009). Decoding flexion of individual fingers using electrocorticographic signals in humans. J. Neural Eng. 6:066001.
Kübler, A. (2020). The history of BCI: From a vision for the future to real support for personhood in people with locked-in syndrome. Neuroethics 13, 163–180.
Kübler, A., Holz, E. M., Riccio, A., Zickler, C., Kaufmann, T., Kleih, S. C., et al. (2014). The user-centered design as novel perspective for evaluating the usability of BCI-controlled applications. PLoS One 9:e112392. doi: 10.1371/journal.pone.0112392
Kübler, A., Nijboer, F., and Kleih, S. (2020). Hearing the needs of clinical users. Handb. Clin. Neurol. 168, 353–368.
Kübler, A, Zickler, C., Holz, E., Kaufmann, T., Riccio, A., and Mattia, D. (2013). Applying the user-centred design to evaluation of Brain-Computer Interface controlled applications. Biomed. Eng. 58, 3234–3234.
Lee, H., Battle, A., Raina, R., and Ng, A. (2006). “Efficient sparse coding algorithms,” in Proceeding of the advances in neural information processing systems, (Cambridge, MA: MIT Press), 19.
Li, Z., Zhang, S., and Pan, J. (2019). Advances in hybrid brain-computer interfaces: Principles, design, and applications. Comput. Intell. Neurosci. 2019.
Liberati, G., Pizzimenti, A., Simione, L., Riccio, A., Schettini, F., Inghilleri, M., et al. (2015). Developing brain-computer interfaces from a user-centered perspective: Assessing the needs of persons with amyotrophic lateral sclerosis, caregivers, and professionals. Appl. Ergon. 50, 139–146. doi: 10.1016/j.apergo.2015.03.012
Logothetis, N. K., Pauls, J., Augath, M., Trinath, T., and Oeltermann, A. (2001). Neurophysiological investigation of the basis of the fMRI signal. Nature 412, 150–157.
Lotte, F., Bougrain, L., Cichocki, A., Clerc, M., Congedo, M., Rakotomamonjy, A., et al. (2018). A review of classification algorithms for EEG-based brain–computer interfaces: A 10 year update. J. Neural Eng. 15, 031005. doi: 10.1088/1741-2552/aab2f2
Lotte, F., Congedo, M., Anatole, L., Lamarche, F., and Arnaldi, B. (2007). A review of classification algorithms for EEG-based brain–computer interfaces. J. Neural Eng. 4, R1–R13. doi: 10.1088/1741-2560/4/2/R01
Luo, S., Rabbani, Q., and Crone, N. E. (2022). Brain-computer interface: Applications to speech decoding and synthesis to augment communication. Neurotherapeutics 19, 263–273. doi: 10.1007/s13311-022-01190-2
Lyu, X., Ding, P., Li, S., Dong, Y., Su, L., Zhao, L., et al. (2023). Human factors engineering of BCI: An evaluation for satisfaction of BCI based on motor imagery. Cogn. Neurodyn. 17, 105–118.
Martin, S., Armstrong, E., Thomson, E., Vargiu, E., Sol, M., Dauwalder, S., et al. (2018). A qualitative study adopting a user-centered approach to design and validate a brain computer interface for cognitive rehabilitation for people with brain injury. Assist. Technol. 30, 233–241. doi: 10.1080/10400435.2017.1317675
Mathis, A., Herz, A. V., and Stemmler, M. B. (2012). Resolution of nested neuronal representations can be exponential in the number of neurons. Phys. Rev. Lett. 109:018103. doi: 10.1103/PhysRevLett.109.018103
Maunsell, J. H., and Van Essen, D. C. (1983). Functional properties of neurons in middle temporal visual area of the macaque monkey. I. Selectivity for stimulus direction, speed, and orientation. J. Neurophysiol. 49, 1127–1147.
McMillan, G. R., Calhoun, G. L., Middendorf, M. S., Schnurer, J., Ingle, D., and Nasman, V. (1995). “Direct brain interface utilizing self-regulation of steady-state visual evoked response (SSVER),” in Proceedings of the RESNA ‘95 Annual Conference, (Vancouver, BC), 693–695.
Mehler, D. M., Sokunbi, M. O., Habes, I., Barawi, K., Subramanian, L., Range, M., et al. (2018). Targeting the affective brain—a randomized controlled trial of real-time fMRI neurofeedback in patients with depression. Neuropsychopharmacology 43, 2578–2585. doi: 10.1038/s41386-018-0126-5
Mellinger, J., Schalk, G., Braun, C., Preissl, H., Rosenstiel, W., Birbaumer, N., et al. (2007). An MEG-based brain–computer interface (BCI). Neuroimage 36, 581–593.
Metzger, S. L., Littlejohn, K. T., Silva, A. B., Moses, D. A., Seaton, M. P., Wang, R., et al. (2023). A high-performance neuroprosthesis for speech decoding and avatar control. Nature 620, 1037–1046. doi: 10.1038/s41586-023-06443-4
Milekovic, T., Bacher, D., Sarma, A. A., Simeral, J. D., Saab, J., Pandarinath, C., et al. (2019). Volitional control of single-electrode high gamma local field potentials by people with paralysis. J. Neurophysiol. 121, 1428–1450. doi: 10.1152/jn.00131.2018
Milekovic, T., Sarma, A. A., Bacher, D., Simeral, J. D., Saab, J., Pandarinath, C., et al. (2018). Stable long-term BCI-enabled communication in ALS and locked-in syndrome using LFP signals. J. Neurophysiol. 120, 343–360. doi: 10.1152/jn.00493.2017
Miller, K. J., Leuthardt, E. C., Schalk, G., Rao, R. P., Anderson, N. R., Moran, D. W., et al. (2007). Spectral changes in cortical surface potentials during motor movement. J. Neurosci. 27, 2424–2432.
Miller, K. J., Schalk, G., Hermes, D., Ojemann, J. G., and Rao, R. P. (2016). Spontaneous decoding of the timing and content of human object perception from cortical surface recordings reveals complementary information in the event-related potential and broadband spectral change. PLoS Comput. Biol. 12:e1004660.
Miller, K. J., Zanos, S., Fetz, E. E., and Nijs, M d, and Ojemann, J. G. (2009). Decoupling the cortical power spectrum reveals real-time representation of individual finger movements in humans. J. Neurosci. 29, 3132–3137. doi: 10.1523/JNEUROSCI.5506-08.2009
Miller, M. I., and Sachs, M. B. (1983). Representation of stop consonants in the discharge patterns of auditory-nerve fibers. J. Acoust. Soc. Am. 74, 502–517.
Ming, C., and Shangkai, G. (1999). “An EEG-based cursor control system,” in Proceedings of the 1999 IEEE engineering in medicine and biology 21st annual conference and the 1999 annual fall meeting of the biomedical engineering society, (Piscataway, NJ).
Molina, G. G., and Mihajlovic, V. (2010). Spatial filters to detect steady-state visual evoked potentials elicited by high frequency stimulation: BCI application. Biomed. Tech. 55, 173–182. doi: 10.1515/BMT.2010.013
Montemurro, M. A., Rasch, M. J., Murayama, Y., Logothetis, N. K., and Panzeri, S. (2008). Phase-of-firing coding of natural visual stimuli in primary visual cortex. Curr. Biol. 18, 375–380. doi: 10.1016/j.cub.2008.02.023
Monti, M. M., Vanhaudenhuyse, A., Coleman, M. R., Boly, M., Pickard, J. D., Tshibanda, L., et al. (2010). Willful modulation of brain activity in disorders of consciousness. N. Engl. J. Med. 362, 579–589.
Müller, S. M., Diez, P. F., Bastos-Filho, T. F., Sarcinelli-Filho, M., Mut, V., and Laciar, E. (2011). “SSVEP-BCI implementation for 37–40 Hz frequency range,” in Proceedings of the 2011 annual international conference of the IEEE engineering in medicine and biology society, (Piscataway, NJ), 6352–6355. doi: 10.1109/IEMBS.2011.6091568
Müller-Putz, G. R., Scherer, R., Neuper, C., and Pfurtscheller, G. (2006). Steady-state somatosensory evoked potentials: Suitable brain signals for brain-computer interfaces? IEEE Trans. Neural Syst. Rehabil. Eng. 14, 30–37. doi: 10.1109/TNSRE.2005.863842
Müller-Putz, G. R., Zimmermann, D., Graimann, B., Nestinger, K., Korisek, G., and Pfurtscheller, G. (2007). Event-related beta EEG-changes during passive and attempted foot movements in paraplegic patients. Brain Res. 1137, 84–91. doi: 10.1016/j.brainres.2006.12.052
Mussi, M. G., and Adams, K. D. (2022). EEG hybrid brain-computer interfaces: A scoping review applying an existing hybrid-BCI taxonomy and considerations for pediatric applications. Front. Hum. Neurosci. 16:1007136. doi: 10.3389/fnhum.2022
Naseer, N., Hong, M. J., and Hong, K. S. (2014). Online binary decision decoding using functional near-infrared spectroscopy for the development of brain–computer interface. Exp. Brain Res. 232, 555–564. doi: 10.1007/s00221-013-3764-1
Naselaris, T., Kay, K. N., Nishimoto, S., and Gallant, J. L. (2011). Encoding and decoding in fMRI. Neuroimage 56, 400–410.
Needell, D., and Tropp, J. A. (2009). CoSaMP: Iterative signal recovery from incomplete and inaccurate samples. Appl. Comput. Harmon. Anal. 26, 301–321.
Neuper, C., Scherer, R., Wriessnegger, S., and Pfurtscheller, G. (2009). Motor imagery and action observation: Modulation of sensorimotor brain rhythms during mental control of a brain–computer interface. Clin. Neurophysiol. 120, 239–247. doi: 10.1016/j.clinph.2008.11.015
Ogawa, S., Lee, T. M., Kay, A. R., and Tank, D. W. (1990). Brain magnetic resonance imaging with contrast dependent on blood oxygenation. Proc. Natl. Acad. Sci. U.S.A. 87, 9868–9872.
Olshausen, B. A., and Field, D. J. (1996). Emergence of simple-cell receptive field properties by learning a sparse code for natural images. Nature 381, 607–609. doi: 10.1038/381607a0
Oxley, T. J., Opie, N. L., John, S. E., Rind, G. S., Ronayne, S. M., Wheeler, T. L., et al. (2016). Minimally invasive endovascular stent-electrode array for high-fidelity, chronic recordings of cortical neural activity. Nat. Biotechnol. 34, 320–327. doi: 10.1038/nbt.3428
Oxley, T. J., Yoo, P. E., Rind, G. S., Ronayne, S. M., Lee, C. M., Bird, C., et al. (2021). Motor neuroprosthesis implanted with neurointerventional surgery improves capacity for activities of daily living tasks in severe paralysis: First in-human experience. J. Neurointerv. Surg. 13, 102–108.
Panzeri, S., Schultz, S. R., Treves, A., and Rolls, E. T. (1999). Correlations and the encoding of information in the nervous system. Proc. R. Soc. Lond. Ser. B Biol. Sci. 266, 1001–1012.
Pati, Y. C., Rezaiifar, R., and Krishnaprasad, P. S. (1993). “Orthogonal matching pursuit: Recursive function approximation with applications to wavelet decomposition,” in Proceedings of 27th Asilomar conference on signals, systems and computers, (Piscataway, NJ), 40–44.
Paulmurugan, K., Vijayaragavan, V., Ghosh, S., Padmanabhan, P., and Gulyás, B. (2021). Brain–computer interfacing using functional near-infrared spectroscopy (fNIRS). Biosensors 11:389.
Pfurtscheller, G., Graimann, B., Huggins, J. E., Levine, S. P., and Schuh, L. A. (2003a). Spatiotemporal patterns of beta desynchronization and gamma synchronization in corticographic data during self-paced movement. Clin. Neurophysiol. 114, 1226–1236. doi: 10.1016/s1388-2457(03)00067-1
Pfurtscheller, G., Müller, G. R., Pfurtscheller, J., Gerner, H. J., and Rupp, R. (2003b). ‘Thought’–control of functional electrical stimulation to restore hand grasp in a patient with tetraplegia. Neurosci. Lett. 351, 33–36. doi: 10.1016/s0304-3940(03)00947-9
Pfurtscheller, G., Neuper, C., Flotzinger, D., and Pregenzer, M. (1997). EEG-based discrimination between imagination of right and left hand movement. Electroencephalogr. Clin. Neurophysiol. 103, 642–651.
Ramoser, H., Muller-Gerking, J., and Pfurtscheller, G. (2000). Optimal spatial filtering of single trial EEG during imagined hand movement. IEEE Trans. Rehabil. Eng. 8, 441–446.
Rathee, D., Raza, H., Roy, S., and Prasad, G. (2021). A magnetoencephalography dataset for motor and cognitive imagery-based brain-computer interface. Sci. Data 8:120. doi: 10.1038/s41597-021-00899-7
Rehn, M., and Sommer, F. T. (2007). A network that uses few active neurones to code visual input predicts the diverse shapes of cortical receptive fields. J. Comput. Neurosci. 22, 135–146. doi: 10.1007/s10827-006-0003-9
Rickert, J., Oliveira, S. C., Vaadia, E., Aertsen, A., Rotter, S., and Mehring, C. (2005). Encoding of movement direction in different frequency ranges of motor cortical local field potentials. J. Neurosci. 25, 8815–8824.
Senden, M., Emmerling, T. C., Hoof, R., Frost, M. A., and Goebel, R. (2019). Reconstructing imagined letters from early visual cortex reveals tight topographic correspondence between visual mental imagery and perception. Brain Struct. Funct. 224, 1167–1183. doi: 10.1007/s00429-019-01828-6
Shirhatti, V., Borthakur, A., and Ray, S. (2016). Effect of reference scheme on power and phase of the local field potential. Neural Comput. 28, 882–913. doi: 10.1162/NECO_a_00827
Siero, J. C., Hermes, D., Hoogduin, H., Luijten, P. R., Petridou, N., and Ramsey, N. F. (2013). BOLD consistently matches electrophysiology in human sensorimotor cortex at increasing movement rates: A combined 7T fMRI and ECoG study on neurovascular coupling. J. Cereb. Blood Flow Metab. 33, 1448–1456. doi: 10.1038/jcbfm.2013.97
Siero, J. C., Hermes, D., Hoogduin, H., Luijten, P. R., Ramsey, N. F., and Petridou, N. (2014). BOLD matches neuronal activity at the mm scale: A combined 7 T fMRI and ECoG study in human sensorimotor cortex. Neuroimage 101, 177–184. doi: 10.1016/j.neuroimage.2014.07.002
Sorger, B., Reithler, J., Dahmen, B., and Goebel, R. (2012). A real-time fMRI-based spelling device immediately enabling robust motor-independent communication. Curr. Biol. 22, 1333–1338. doi: 10.1016/j.cub.2012.05.022
Sun, Y., Shen, A., Sun, J., Du, C., Chen, X., Wang, Y., et al. (2022). Minimally invasive local-skull electrophysiological modification with piezoelectric drill. IEEE Trans. Neural Syst. Rehabil. Eng. 30, 2042–2051. doi: 10.1109/TNSRE.2022.3192543
Theunissen, F., and Miller, J. P. (1995). Temporal encoding in nervous systems: A rigorous definition. J. Comput. Neurosci. 2, 149–162. doi: 10.1007/BF00961885
Thorpe, S. J. (1990). “Spike arrival times: A highly efficient coding scheme for neural networks,” in Parallel processing in neural systems, eds G. Hartmann, R. Eckmiller, and G. Hauske (North-Holland: Elsevier), 91–94.
Vansteensel, M. J., Pels, E. G., Bleichner, M. G., Branco, M. P., Denison, T., Freudenburg, Z. V., et al. (2016). Fully implanted brain–computer interface in a locked-in patient with ALS. N. Engl. J. Med. 375, 2060–2066. doi: 10.1056/NEJMoa1608085
Vision, N. (2000). Sparse coding and decorrelation in primary visual cortex during. Science 287:1273.
Volosyak, I., Valbuena, D., Lüth, T., Malechka, T., and Gräser, A. (2011). BCI demographics II: How many (and what kinds of) people can use a high-frequency SSVEP BCI? IEEE Trans. Neural Syst. Rehabil. Eng. 19, 232–239. doi: 10.1109/TNSRE.2011.2121919
Wang, W. (2006). Motor cortical representation of hand position, velocity and rotation during reaching. St. Louis, MO: Washington University.
Wang, Z., Shi, N., Zhang, Y., Zheng, N., Li, H., Jiao, Y., et al. (2023). Conformal in-ear bioelectronics for visual and auditory brain-computer interfaces. Nat. Commun. 14:4213. doi: 10.1038/s41467-023-39814-6
Willett, F. R., Avansino, D. T., Hochberg, L. R., Henderson, J. M., and Shenoy, K. V. (2021). High-performance brain-to-text communication via handwriting. Nature 593, 249–254. doi: 10.1038/s41586-021-03506-2
Willett, F. R., Kunz, E. M., Fan, C., Avansino, D. T., Wilson, G. H., Choi, E. Y., et al. (2023). A high-performance speech neuroprosthesis. Nature 620, 1031–1036.
Wittevrongel, B., Khachatryan, E., Fahimi, H. M., Camarrone, F., Carrette, E., De Taeye, L., et al. (2018). Decoding steady-state visual evoked potentials from electrocorticography. Front. Neuroinform. 12:65. doi: 10.3389/fninf.2018.00065
Wolpaw, J. R., and Wolpaw, E. W. (2012). Brain-computer interfaces: Principles and practice. Oxford: Oxford University Press.
Wolpaw, J. R., Millán, J. R., and Ramsey, N. F. (2020). Brain-computer interfaces: Definitions and principles. Handb. Clin. Neurol. 168, 15–23.
Wu, S., Amari, S., and Nakahara, H. (2002). Population coding and decoding in a neural field: A computational study. Neural Comput. 14, 999–1026. doi: 10.1162/089976602753633367
Xu, H., Gong, A., Ding, P., Luo, J., Chen, C., and Fu, Y. (2022). Key technologies for intelligent brain-computer interaction based on magnetoencephalography. Sheng Wu Yi Xue Gong Cheng Xue Za Zhi 39, 198–206. doi: 10.7507/1001-5515.202108069
Xu, L., Xu, M., Jung, T. P., and Ming, D. (2021). Review of brain encoding and decoding mechanisms for EEG-based brain–computer interface. Cogn. Neurodyn. 15, 569–584.
Xue, H., Wang, D., Jin, M., Gao, H., Wang, X., Xia, L., et al. (2023). Hydrogel electrodes with conductive and substrate-adhesive layers for noninvasive long-term EEG acquisition. Microsyst. Nanoeng. 9:79. doi: 10.1038/s41378-023-00524-0
Yoo, S. S., Choi, B. G., Chung, K. I., and Lee, C. (2001). Neural substrates of motor imagery: Event-related functional MRI study. J. Korean Neuropsychiatr. Assoc. 1247–1250.
Yoo, S. S., Fairneny, T., Chen, N. K., Choo, S. E., Panych, L. P., Park, H., et al. (2004). Brain–computer interface using fMRI: Spatial navigation by thoughts. Neuroreport 15, 1591–1595.
Zhang, D., Liu, S., Wang, K., Zhang, J., Chen, D., Zhang, Y., et al. (2021). Machine-vision fused brain machine interface based on dynamic augmented reality visual stimulation. J. Neural Eng. 18:056061. doi: 10.1088/1741-2552/ac2c9e
Zhang, M., Wu, J., Song, J., Fu, R., Ma, R., Jiang, Y., et al. (2022). Decoding coordinated directions of bimanual movements from EEG signals. IEEE Trans. Neural Syst. Rehabil. Eng. 31, 248–259. doi: 10.1109/TNSRE.2022.3220884
Keywords: brain-computer interface (BCI) paradigm, neural coding, brain imaging technology, neural decoding, BCI
Citation: Tai P, Ding P, Wang F, Gong A, Li T, Zhao L, Su L and Fu Y (2024) Brain-computer interface paradigms and neural coding. Front. Neurosci. 17:1345961. doi: 10.3389/fnins.2023.1345961
Received: 28 November 2023; Accepted: 28 December 2023;
Published: 15 January 2024.
Edited by:
Yongcheng Li, Chinese Academy of Sciences (CAS), ChinaReviewed by:
Xiaogang Chen, Chinese Academy of Medical Sciences and Peking Union Medical College, ChinaRui Zhang, Zhengzhou University, China
Copyright © 2024 Tai, Ding, Wang, Gong, Li, Zhao, Su and Fu. This is an open-access article distributed under the terms of the Creative Commons Attribution License (CC BY). The use, distribution or reproduction in other forums is permitted, provided the original author(s) and the copyright owner(s) are credited and that the original publication in this journal is cited, in accordance with accepted academic practice. No use, distribution or reproduction is permitted which does not comply with these terms.
*Correspondence: Peng Ding, ausarschorr@foxmail.com; Yunfa Fu, fyf@ynu.edu.cn