- 1Department of Intensive Care and Oncological Emergencies and Thoracic Oncology Clinic, Institut Jules Bordet, Université Libre de Bruxelles, Brussels, Belgium
- 2Data Centre, Institut Jules Bordet, Université Libre de Bruxelles (ULB), Brussels, Belgium
- 3Department of Pulmonary and Thoracic Oncology, Centre Hospitalier Régional Universitaire de Lille, Lille, France
- 4Department of Pneumology, Grand Hôpital de Charleroi, site Saint-Joseph, Gilly, Belgium
- 5Department of Oncology, Hôpital Ambroise Paré, Mons, Belgium
- 6Department of Pneumology, CH Charleroi, Charleroi, Belgium
- 7Department of Medical Oncology and Hematology, Princess Margaret Cancer Centre, Toronto, ON, Canada
- 8Department of Pneumology, CHR St Joseph, Warquignies, Belgium
- 9Department of Pneumology, CH Mouscron, Mouscron, Belgium
- 10Laboratory of Molecular and Cellular Biology, Université de Liège, Gembloux, Belgium
Aim: Cisplatin doublets are standard 1st line treatment for advanced non-small cell lung cancer (NSCLC), without accurate predictor for response and survival, but important toxicity. Our aims were to identify predictive (for response) and prognostic (for survival) biological signatures in patients with NSCLC using messenger RNAs (mRNA) and miRNA expression.
Methods: Patients with pathologically proven untreated NSCLC, receiving 1st line cisplatin–vinorelbine and with an assessable lesion were eligible. A bronchial biopsy was lysed into Tripure Isolation Reagent on ice, snap frozen, and stored at −80°C. mRNA expression was analyzed using microarrays Agilent Technologies. miRNA expression was assessed using TaqMan Low Density Arrays (756 human miR panel, Applied Biosystems). Validation was performed by RT-PCR on the selected genes. Survival was measured from the registration date and response assessed by WHO criteria.
Results: Biopsies for transcriptomic analyses were obtained from 60 consecutive patients. No statistically significant differences were observed according to the main clinical characteristics, response rate (43 vs. 41%) or survival (median 25 vs. 29 months) between derivation and validation sets. In the derivation set (n = 38 patients), two mRNA and one miRNA predictive signatures for response were obtained. One mRNA and one miRNA prognostic signatures were derived from the first set, allowing an adequate distinction of patients with good and poor overall and progression-free survivals. None of these signatures could be validated in the validation set (n = 22 patients).
Conclusion: In this prospective study with advanced NSCLC treated with cisplatin–vinorelbine, we were able to derive with high throughput techniques predictive and prognostic signatures based on transcriptomic analyses. However, these results could not be reproduced in an independent validation set. The role of miRNA and mRNA as predictive or prognostic factors remains a research topic and the use of high throughput technology in that context questionable. The ClinicalTrials.gov study identifier is NCT00864266 (www.clinicaltrials.gov).
Introduction
Presently, non-small cell lung cancer (NSCLC) is the first cause of cancer death in industrialized countries. For most of the patients, the prognosis is poor (1). A few prognostic factors have been consistently reported in the literature, including performance status, gender, stage, or histology (2) but no prognostic score is allowing valid individual prognostic prediction. With a better understanding of the tumor biology and the development of high throughput techniques allowing multiple investigations on the same time, multiple prognostic biological signatures, based on messenger RNAs (mRNA) or miRNA analyses, have been proposed. However, most were constructed from retrospective studies, restricted to limited stages and surgical cases (3). Nevertheless, more than two-thirds of the patients are diagnosed at an advanced or metastatic stage for which only palliative chemotherapy can be provided. While the activity of first-line chemotherapy was demonstrated in randomized trials and meta-analyses (4), only 20–40% of the patients will exhibit an objective response. Customized therapy based on targeted agents has shown its effectiveness in tumors presenting with EGFR activating mutations (5) or ALK translocation (6). However, for most patients, there are no reliable predictive factor assessing chemosensitivity. This explains why a large number of patients are exposed uselessly to expensive and/or toxic drugs. While retrospective studies suggested that biomarkers could be helpful in predicting sensitivity to platinum derivatives (ERCC1 and XRCC1), taxanes (BRCA1), vincalcaloids (β tubulines), or gemcitabine (RRM1), two randomized trials failed to demonstrate that a genotypic approach based on ERCC1 or combined ERCC1 and RRM1 can help in predicting chemosensitivity and survival (7, 8).
The European Lung Cancer Working Party (ELCWP) initiated a prospective study aiming to find biological signatures that would predict response to chemotherapy and prognostic significance for progression-free and overall survival in advanced and metastatic NSCLC. First data of this study were previously published, concerning the miRNA analyses performed on a homogeneous derivation group of 38 patients treated with a same combination of cisplatin and vinorelbine. We were able to identify a two-miRNA signature predicting response to this chemotherapy regimen and a four-miRNA signature with prognostic value for survival (9).
The current study presents the results of our prospective study based on both mRNA and miRNA analyses and including the data from the initial derivation cohort and a validation cohort.
Materials and Methods
To be eligible for the study, patients had to meet the following inclusion criteria: histologically confirmed NSCLC (generally, this information was available after registration due to the design of the protocol), to have a bronchial biopsy for biomarkers analysis, to be a candidate for standard first-line chemotherapy. Other key eligibility criteria were: age ≥ 18 years; presence of a measurable lesion; no prior chemotherapy; no history of cancer other than non-melanoma skin cancer, in situ cervical cancer or cured malignancy (interval > 5 years without recurrence). Signed informed consent had to be obtained prior to registration. The ethics committees of the participating institutions had approved the study protocol, in accordance with current legislation. The ClinicalTrials.gov study identifier is NCT00864266.
After registration at the ELCWP data center, biopsies and complete tumoral work-up, a therapeutic choice was made by the physician in charge of the patient. If the indication of chemotherapy for NSCLC was confirmed, the choice of treatment was left to the investigator, based on clinical practice guidelines of the ELCWP (10), with a preferential option for the combination of cisplatin (60 mg/m2 day 1) plus vinorelbine (25 mg/m2 days 1 and 8), every 3 weeks in order to obtain a homogeneous group for biomarkers analysis. For the present analysis, the selected group of patients required histologically confirmed NSCLC whose response to chemotherapy with cisplatin–vinorelbine was evaluable according to WHO criteria (11) and adequate biopsy obtained for transcriptomic analysis. Evaluation of response was performed every three cycles and in case of objective response, patients were treated until best response. All charts were reviewed during regular meetings by at least three independent ELCWP investigators. Patients with early progression or death due to malignant disease prior to evaluation or toxicity and treatment cessation due to toxicity were considered as treatment failures. Survival was measured from the registration date until death from any cause or last date known to be alive. Progression-free survival was measured from date of registration until date of first progression or death.
Biopsy Procedure
The procedure for collecting and processing bronchial biopsies was standardized. Any patient with pulmonary lesion consistent with the diagnosis of lung cancer and for which bronchoscopy was considered, was offered the protocol before any treatment has been applied. The sequence of diagnostic bronchoscopy was identical to a standard one, with the exception of additional samples for the study. A minimum of two tumoral biopsies were collected if the tumor was accessible during endoscopy. For each tumor biopsy, a control sample was taken in a macroscopically healthy bronchial area, remote from the tumor. Among the biopsies, the first sample was fixed in formalin and embedded in paraffin for histological diagnosis. The second one was treated for transcriptomic analyses (mRNA and miRNA) by high throughput techniques. It was directly lysed in Tripure (Roche Diagnostics, Indianapolis, IN, USA) on ice and snap frozen with liquid nitrogen. If possible, a third series of biopsies was collected and directly frozen in liquid nitrogen in order to store it in a tissue bank for further molecular biology analyses. Both series of biopsies collected for molecular biology were stored at −80°C.
Nucleic Acid Isolation
This procedure allowed isolation of total RNA for both mRNAs and miRNAs expression analyses. RNA isolation was performed using the Tripure reagent (Roche Diagnostics). We added 20 μg of glycogen (Roche Diagnostics) as carrier and the separation between the organic and the aqueous phases was achieved on Phase Lock Gel (Eppendorf, Hamburg, Germany), optimizing the recovery of nucleic acids. RNA was assessed for quantity and purity on the NanoDrop ND-1000 spectrophotometer (NanoDrop Technologies, Rockland, DE, USA) and for integrity on the Agilent 2100 bioanalyser with RNA 6000 NanoAssay (Agilent Technologies, Palo Alto, CA, USA). The extracted RNAs were stored at −80°C. The RNA was used to assess expression of the mRNAs using microarrays (Agilent Technologies) and of the miRNAs by Taqman Low Density arrays (Applied Biosystem).
Messenger RNAs Expression Analysis (Microarrays)
Messenger RNAs were reverse-transcribed by using a T7 primer coupled with oligo-dT primers and Moloney murine leukemia virus-reverse transcriptase (MMLV-RT). The cDNAs were then in vitro transcribed into labeled cRNA by the T7 RNA polymerase with fluorescent nucleotides, Cy5 for samples or Cy3 for reference RNA dyes, by using the Low input RNA Fluorescent Linear Amplification Plus kit (Agilent Technologies). RNAs spike-in (Agilent Technologies) served as positive controls to monitor the whole microarray workflow (sample amplification, labeling and microarray processing). An amount of 100 ng of starting total RNA was engaged for each sample and 100 ng of pooled reference RNAs was amplified in parallel in the same experiment with the same Master-Mix. Labeled cRNA was checked for quantity and dye incorporation by the NanoDrop ND-1000 spectrophotometer (NanoDrop Technologies) and the Gaussian distribution of sample sizes was assessed by migration profiles on the Agilent 2100 bioanalyser with RNA 6000 NanoAssay (Agilent Technologies). Thereafter, the labeled cRNAs was hybridized on an Agilent oligonucleotide microarray (Two Colors Whole Human Genome 4 × 44 K arrays, Agilent Technologies) for 17 h at 65°C in a rotating oven according to the provider’s protocol (Agilent Technologies). After disassembling the hybridization chambers, the slides were washed and the signal was read by confocal laser scanning (Agilent Technologies). Grid positioning, spots localization, outliers flagging, fluorescence intensities quantification, background level assessment, and correction of the values according to the background followed by linear and Lowess data normalization were performed by using Feature Extraction software (Agilent Technologies). Quality control metrics were calculated by using this software and the arrays, which reached the 12/12 points among the 12 qualitative experimental aspects assessed (reproducibility of spike-in RNAs, maximum acceptable background, quantification of the outliers, reproducibility of the replicated spots on the arrays, etc.) were included in the analysis. Statistical analyses of microarray data were performed with the Genespring GX software (Agilent Technologies).
miRNA Expression Analysis
The miRNA were reverse-transcribed and amplified by PCR using the multiplex RT TaqMan MicroRNA Low Density Array (LDA) kit (Applied Biosystem, Foster City, CA, USA). An amount of 700 ng total RNA was used for each sample. The global miRNA profiling for human miRNA (756 miR probes) was then performed by using the TaqMan LDA Human microRNA Panel (Applied Biosystem). All the quality control tests were validated: blanks and reproducibility [standard deviation of cycle threshold (CT) < 1] with the two small nucleolar house-keeping RNAs RNU48 (SNORD48) and RNU44 (SNORD44). The amount of RNA from each sample was calibrated to RNU48 that had the smallest standard deviation of all miRNA. This value gave a delta CT (ΔCT) value for each miR (miR CT value – RNU48 CT value). The average ΔCT was calculated for responders and non-responders and the delta ΔCTs (ΔΔCTs) corresponded to the difference in ΔCT between the two categories. Fold changes (FC) were calculated as 2−ΔΔCT for up-regulated, as a decrease in one CT value was equivalent to a twofold increase in the starting amount of cDNA, and by 2ΔΔCT for down-regulated miRNA.
Internal Validation of the Genes Found in Microarrays Analyses by RT-qPCR
The reverse transcriptase quantitative PCR (RT-qPCR) was done by TaqMan. The qPCR reaction consisted to use the Mesa Green qPCR Master-Mix Plus diluted 2×, 300 nM of forward and reverse primers, and diluted template cDNA in a range of 5 and 10×, in function of the RNA quantity assessed by the NanoDrop ND-1000 spectrophotometer. Cycling was performed at the following conditions: 15 min at 42°C, 3 min at 95°C followed by 40 rounds of 15 s at 95°C, 20 s at 60°C, and 40 s at 72°C. The assay included two no-template controls that consisted of the same samples without the reverse transcription and a control of potential non-specific amplifications by using melting curves.
Statistical Considerations
The primary objective of the study was to identify a molecular signature to predict response to chemotherapy in patients with NSCLC. The secondary objectives were to identify prognostic signatures for survival and progression-free survival. Statistical considerations are detailed at www.elcwp.org. The lack of data and the absence of guidelines in this particular setting led us to make assumptions on the study power with different scenarios. So, assuming the existence of a predictive signature, we calculated the power to detect the effect of the signature on response, with varying rates of patients with a predictive signature of response (between 10 and 50%) for standard chemotherapy and for three different values for objective response rate overall. We also targeted a response rate among patients predicted to be responders of 75% at least. The power, including a minimum of 15 patients evaluable for objective response to perform the genetic analysis in the derivation group, was more than 80% in almost all circumstances with predicted response rates above 20% (power 0.90–1.00) except in the situation of low rates (<20%, power 0.47–0.80) of patients predicted to be responders, but in this case the signature can be considered as not sensitive enough and then without clinical usefulness. According to the protocol, the signature should be confirmed in an independent validation group.
Differences between responders and non-responders according to clinical characteristics were assessed by t-tests or a Fisher’s Exact tests. We applied t-tests for comparing mRNA expression and Wilcoxon test for miRNA expression between responders and non-responders, after adjusting for multiplicity testing using the Benjamini–Hochberg (BH) method (12). False discovery rate was set to 5%. The signature for response was derived using logistic regression with stepwise variable selection. The associations between overall survival and mRNA or miRNA expression levels were estimated by using the Kaplan–Meier method and a log-rank test. Cox proportional hazard regression models were applied to estimate the hazard ratios. The signatures for overall survival were derived using Cox proportional hazards models with stepwise variable selection.
Results
From 1/04/2009 to 12/06/2013, 308 patients, with a high suspicion of lung cancer based on lung lesion on chest CT scan, were prospectively registered (Figure 1). Seven patients further denied their initial consent, leaving 301 patients assessable for the study. In 25 cases, the diagnosis of lung cancer could not be confirmed. The two main reasons were malignant tumor from another site (n = 13) or a benign disease (n = 6). Despite the clinical suspicion, no pathologic confirmation could be obtained in six cases. A pathological diagnosis of lung cancer was found in 276 patients, either on samples obtained at bronchoscopy or during a subsequent procedure. Among them, there were 39 small cell lung cancers leaving 237 NSCLC for further analyses.
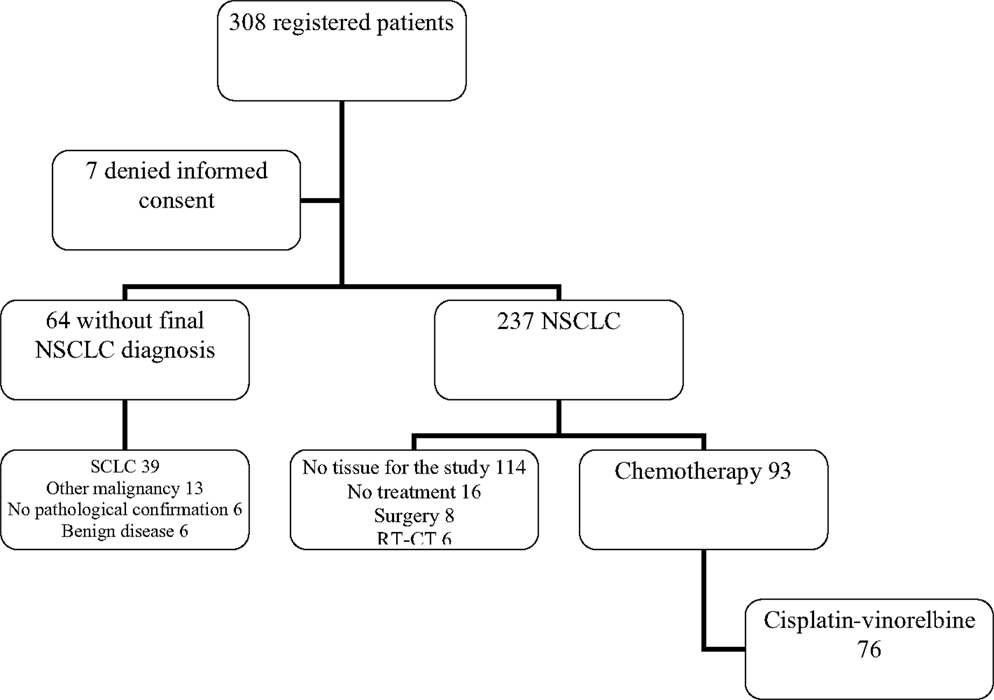
Figure 1. Flow chart of registered patients. NSCLC, non-small cell lung cancer; SCLC, small cell lung cancer; RT-CT, radiochemotherapy.
Among the 237 NSCLC, no tissue sample eligible for the genomic analyses can be obtained in 114 cases. From the remaining 123 patients, 16 did not receive any treatment (exclusive palliative care and/or rapid alteration of the general status not allowing any anticancer therapy) and 14 were treated with curative intent including a local treatment not allowing evaluation of chemotherapy activity (eight surgery and six radiochemotherapy).
Among 93 patients benefiting from chemotherapy, 76 did receive a combination of cisplatin (60 mg/m2 day 1) and vinorelbine (25 mg/m2 days 1 and 8). From these 76 patients (Figure 2), eight were not assessable for response and at the time of analysis, one patient was still under therapy and not yet assessed. Sixty-seven patients were evaluable for response but only 55 samples were adequate for performing microarrays (mRNA), the other 11 showing either insufficient quantity (n = 2), poor quality (n = 9), or unassessable for technological consideration (n = 1). The first 34 patients were included in the derivation group and the further 21 ones in the validation one. Sixty patients could be included in the miRNA analyses, the first 38 for the derivation group and the further consecutive 22 for the validation one. In seven cases, RNA was in insufficient quantity. The main characteristics of the patients are reported in the Table 1. No statistically significant differences were observed between the derivation and validation sets concerning clinical characteristics, response rate, or survival times.
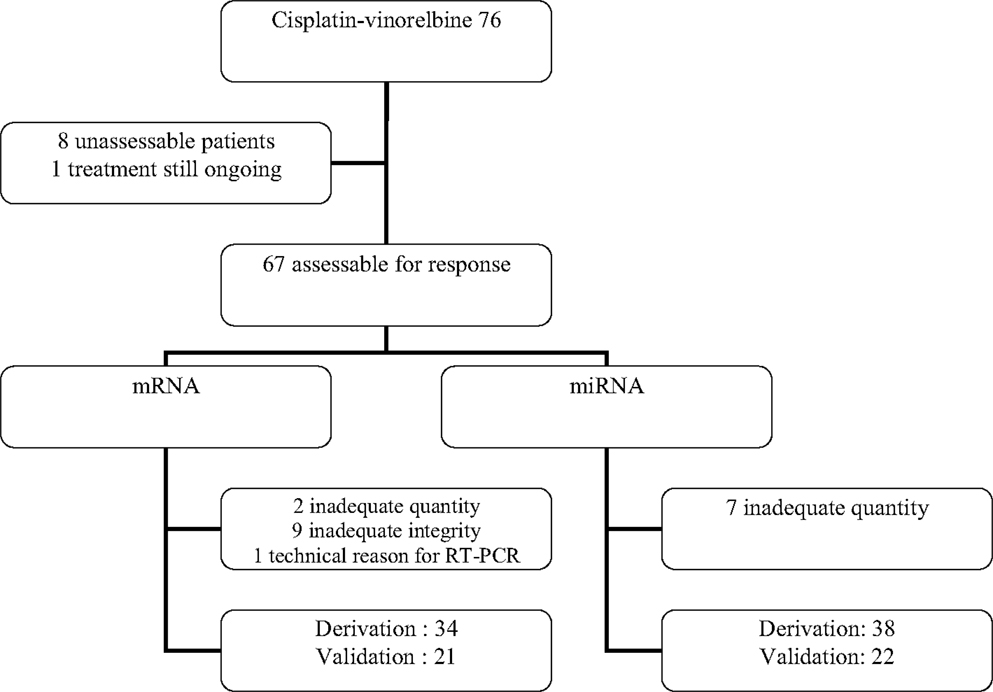
Figure 2. Flow chart of the patients treated with cisplatin–vinorelbine for transcriptomic analysis.
mRNA Analyses
Derivation group
Constructing a signature predicting response to cisplatin–vinorelbine. Thirty-four patients were assessable for response and mRNA analyses in the derivation group. Among those 34 patients, we observed 14 objective responses (41%) confirmed by an independent panel of ELCWP investigators. Data were imported in Genespring as Agilent two-color data, preprocessed by Feature Extraction software with normalization and baseline to median transformation of all samples. All quality indicators appeared correct with and without baseline transformation.
We removed from the 41,093 probes the 14,523 that were not expressed in any of the samples, leaving 26,570 probes for the analyses. If we restricted to only the probes with detected intensities in all of the samples of at least one experimental condition (response/non-response), then out of the 41,093 probes, 26,567 would have been retained. If we had restricted only to the probes with detected intensities in all of the samples for both response and non-response, then out of the 41,093 probes, 24,609 probes would have been retained, but potentially interesting biological changes between response and non-response might be missed. Therefore, we have decreased the stringency of the filter such that even if the gene is only expressed (associated with detected or marginal intensity) in all of the samples in any experimental condition (response/non-response), the probe will pass the filter. So, only undetected probes were removed.
We applied t-tests (asymptotic p-value) for comparing mRNAs expression according to response, after adjusting for multiplicity testing [false discovery rate (FDR) according to BH method <0.05]. We found 115 mRNAs differentially expressed between responders and non-responders (Table 2), 50 mRNAs having a FC >2 and 19 with a FC >3 (Table 3).
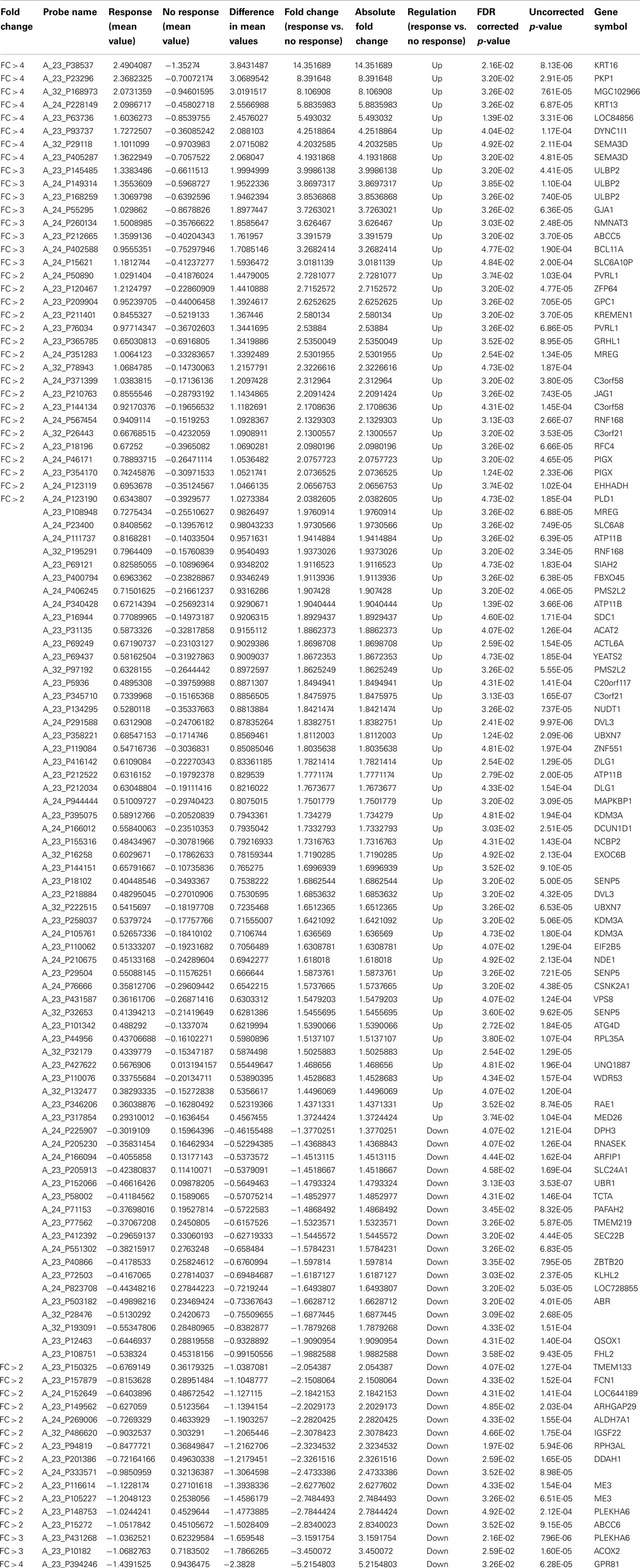
Table 2. List of 115 genes differentially expressed between responders and non-responders in the derivation group.
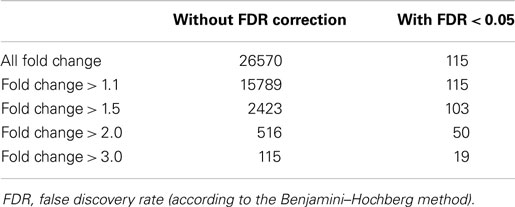
Table 3. Number of genes differentially expressed in responders and non-responders in the derivation group.
We restricted further analyses to the mRNAs with a fold change of at least two. Among the 50 mRNAs differentially expressed, 34 were up-regulated and 16 down-regulated in non-responders in comparison with responders. We calculated the area under the ROC curve for each mRNA to predict response (genes detailed in Table 4). After a stepwise variable selection on the 50 mRNAs, two variables (FCN1 and RNF168) were retained. The area under the ROC curve, when including both FCN1 and RNF168, was 0.97 [95% confidence interval (CI) 0.91–1]. It allowed constructing a signature predicting response to cisplatin–vinorelbine summarized as −2*FCN1 + 5*RNF168. At the best threshold of >2.3, the signature had 93% (13/14) sensitivity, 100% (20/20) specificity, 100% (13/13) positive predictive value, and 95% (20/21) negative predictive value (Table 5).
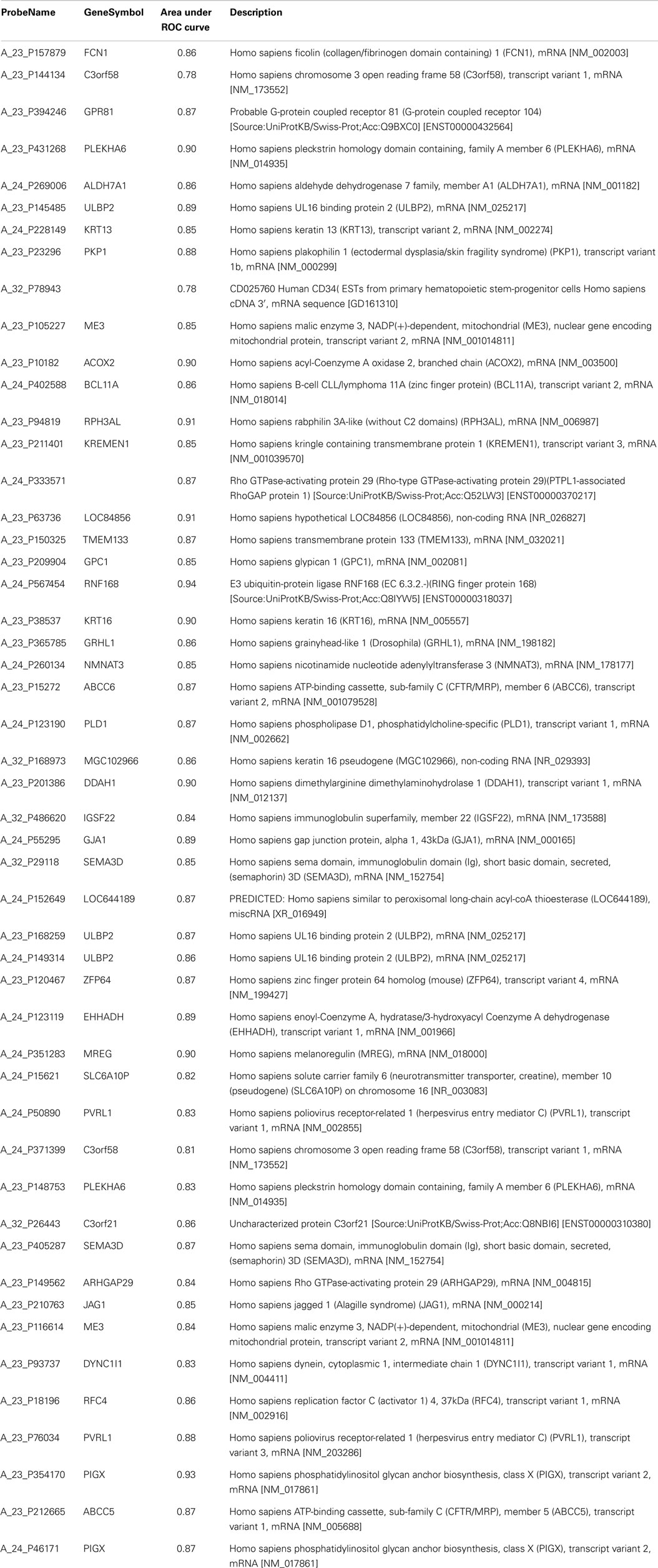
Table 4. Area under ROC curve for the 50 genes differentially expressed between responders and non-responders with a fold change > 2.
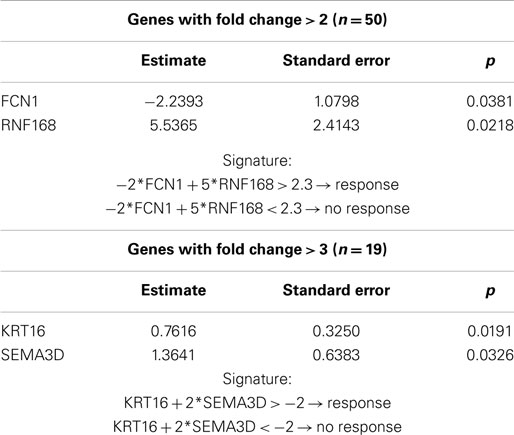
Table 5. Results of the stepwise regression and construction of signature predicting response in patients treated with cisplatin–vinorelbine based on mRNA analyses.
As a FC >3 is another limit accepted in the literature, we performed the analysis with the same methodology, restricted to the 19 mRNAs with a FC above 3. Three were down-regulated and 16 up-regulated in non-responders in comparison with responders. After a stepwise variable selection on the 19 mRNAs, two variables (KRT16 and SEMA3D) were retained. KRT16 had a FC of 14, SEMA3D a FC of 4 (Table 2). The area under the ROC curve, when including both KRT16 and SEMA3D, was 0.95 (95% CI 0.89–1). The signature (KRT16 + 2*SEMA3D) allowed predicting response with 100% (14/14) sensitivity, 100% negative predictive value (14/14), 70% specificity (14/20), and 70% (14/20) positive predictive value with a best cut-off of −2 (Table 5).
The mRNAs found in the two signatures were further validated in the same patients by RT-qPCR (Table 6). The following forward (Fw) and reverse (Rev) primers were used for the selected genes FCN1 (Fw: TAGAGCTGGGGGACTCTTCA; Rev: CCACCTTCACCTCTGGACAT), RNF168 (Fw: TTGGCAGAGGAGGAAGAAGA; Rev: TCAAGGGAGAAGCCGAGATA), KRT16 (Fw: GTGAAGATCCGTGACTGGTA; Rev: GCAATGATCTTGTTCCTCAG), and SEMA3D (Fw: TGGAATTGTCTCTGAAGCAGC; Rev: TGCGCAAGGTTTCCCATAAG). They were for the reference genes HPRT (Fw: GGTCAGGCAGTATAATCCAAAG; Rev: AAGGGCATATCCTACAACAAAC) and β-actin (Fw: CGCCGCCAGCTCACCATG; Rev: CACGATGGAGGGGAAGACGG). Using this methodology, we could confirm in that group of patients that the four genes were differentially expressed with a high statistical significance between responders and non-responders. The p-value were respectively 0.006 (FCN1), 0.008 (RNF168), 0.002 (KRT16), and 0.005 (SEMA3D). When normalized with the reference gene HPRT, all except RNF168 retained their statistical significance with respective p-value of <0.01 (FCN1-HPRT), 0.68 (RNF168-HPRT), 0.002 (KRT16-HPRT), and 0.06 (SEMA3D-HPRT).
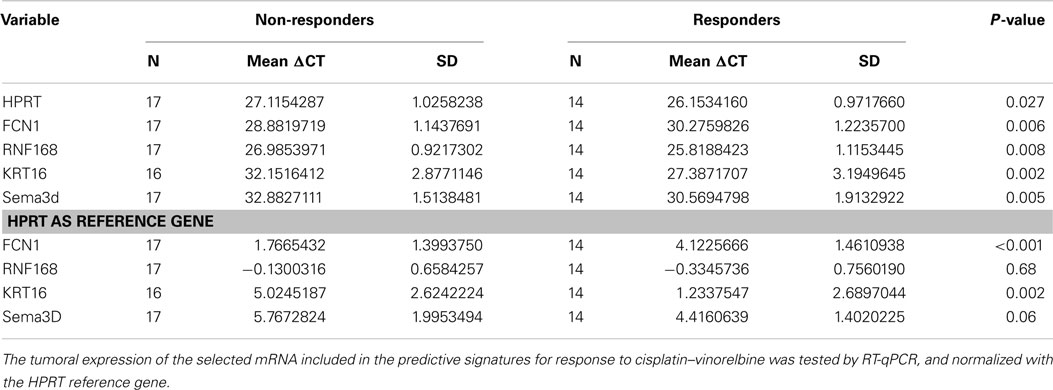
Table 6. Internal validation of the four genes found in the two predictive signatures for response by RT-qPCR in the derivation set.
We measured the diagnostic performance of both signatures by comparing their area under the ROC curve. No statistical difference was noted (p = 0.58).
Constructing a prognostic signature for survival and progression-free survival. Using the same statistical methodology, we constructed a signature specifically predicting overall survival using the mRNAs with a FC >3. The signature included two mRNAs (KRT16 and ULBP2) and was designed as −3*KRT16 + 2*ULBP2. At the best cut-off of 1, it allowed distinguishing patients with poor and good overall survival (HR 22.2, 95% CI 4.6–107.7; log-rank and Wilcoxon test p < 0.001) (Figure 3, Table 7). Respective median survival times were for the “good overall survival group” of 40 months (95% CI 25–52 months) and for the “poor overall survival group” of 15 months (95% CI 7–19 months). The same signature was statistically significantly predicting progression-free survival (HR 3.8, 95% CI 1.5–9.3; p < 0.001). Respective median progression-free survival times were for the “good overall survival group” of 18.6 months (95% CI, 12.3–27.3 months) and for the “poor overall survival group” of 8.1 months (95% CI, 5.8–15.5 months).
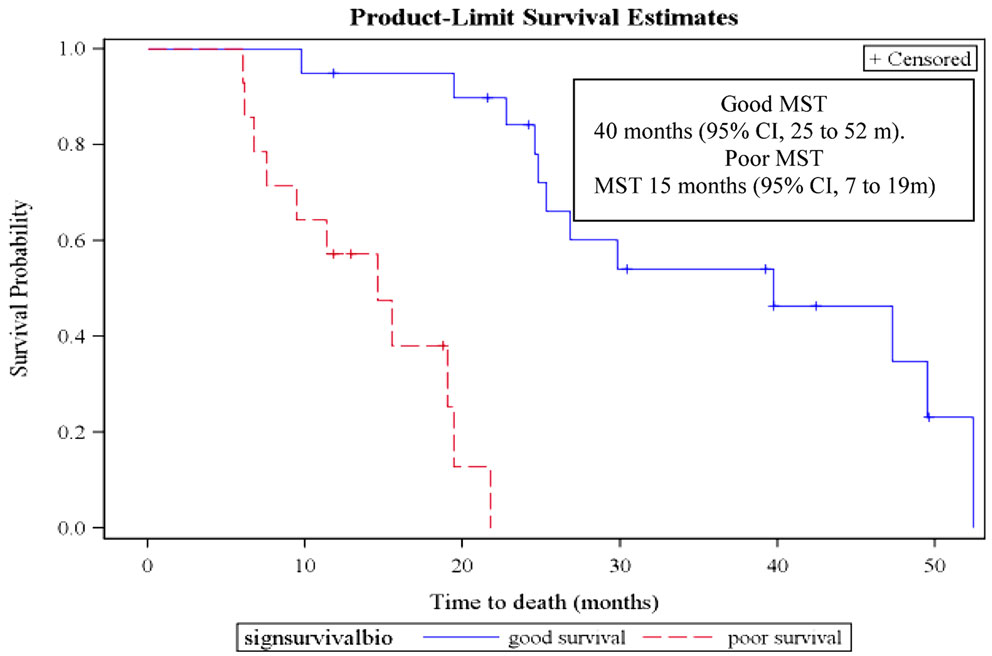
Figure 3. Overall survival curves according to the mRNA prognostic signature in the derivation group. MST, median survival time. Patients from the derivation group could be dichotomized according to the mRNA prognostic signature (−3*KRT16 + 2*ULBP2). A value >1 is predicting a poor overall survival while a value <1 is associated with a good overall survival.
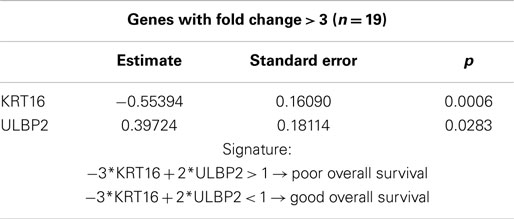
Table 7. Results of the stepwise Cox’s proportional hazards model regression and construction of signature predicting survival in patients treated with cisplatin–vinorelbine, based on mRNA analyses in the derivation set.
Validation group
Reverse transcriptase quantitative PCR for the five genes included in the two predictive signatures and the one prognostic signature (FCN1, RNF168, KRT16, SEMA3D, and ULBP2) were performed on 22 samples from 22 patients treated with cisplatin–vinorelbine. One sample was excluded as the CT values for the two reference genes (HPRT and β-actin) were above 32, leaving 21 patients assessable for validating the signatures. Nine objective responses were observed. Whatever correcting for actin or HPRT, no gene was anymore differentially expressed with statistical significance between responders and non-responders (Table 8). However, we observed a statistically significant difference between the 12 non-responders and the 9 responders in the expression of the two reference genes actin (t-test p < 0.001, Wilcoxon p 0.046) and HPRT (t-test p 0.005, Wilcoxon p 0.056).
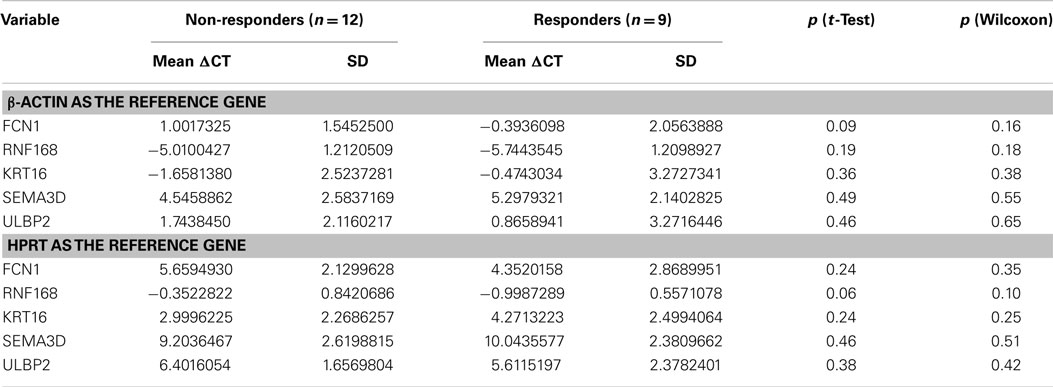
Table 8. mRNA signatures for response to chemotherapy (validation set): differential expression of the genes found in the signatures between responders and non-responders.
As no gene could be validated, we repeated the same microarray procedure for selecting variables in the 21 patients included in the validation set and tested by RT-qPCR. From the 41,093 probes, 25,693 allowed detection of a signal in at least all of the patients in one group (response/non-response). When applying t-tests (asymptotic p-value) for comparing mRNAs expression according to response, after adjusting for multiplicity testing (FDR < 0.05), no gene could be retained. We further tested both predictive and prognostic signature. None of them retained their statistical significance in the validation group, with p-values of 0.20 (signature for response) and 0.27 (signature for survival), respectively.
As an exploratory analysis, we also looked at genes differentially expressed between responders and non-responders, in common between the derivation and validation groups. For this purpose, we performed asymptotic t-tests without FDR application. Three thousand nine hundred ninety-four and 4597 genes were retained respectively in the derivation and validation sets, of which 402 were in common in both sets. Only 153 of them are regulated in the same direction, but only 10 probes had a differential expression between responders and non-responders at an FDR uncorrected p-value <0.01 in both sets and only one with a FC >2 in both sets. They are KCTD1, ALDH3B2, IQCB1, CHCHD6, RMND5A, NRP1 (transcript variant 3), RPS6KA5, C17orf69, NRP1 (transcript variant 1), and IQCB2P.
miRNA Analyses
Derivation group
Constructing signatures predicting response to cisplatin–vinorelbine and prognostic for survival. The results concerning the development of predictive and prognostic signatures based on tumoral miRNAs in the derivation group have been previously published (9). Briefly, miRNAs analyses were performed in 38 patients of whom one was not assessable for response. Sixteen objective responses (43%) were observed. Of the 756 miRNAs, 396 had a CT > 32 in all patients and were excluded from the analysis. Thirty-eight miRNAs were differentially expressed between responders and non-responders with a p-value < 0.05. If we applied the BH method for controlling the FDR for multiple testing, none of them could be retained. However, without FDR selection, a two miRNAs signature was designed: −4*hsa-miR-149 + 3*hsa-miR-375 with a score above −6 predicting response with an area under the ROC curve of 0.90 and 88% sensitivity, 81% specificity, 78% positive, and 89% negative predictive values.
To challenge the signature, we analyzed the prognostic value of miRNAs for overall survival among 38 patients, 25 being dead at the time of analysis. After stepwise selection, four miRNAs were included in a prognostic score. The signature was as follows: 3*hsa-miR-200c − 9*hsa-miR-29c − 2*hsa-miR-424 − 2*hsa-miR-124. With a cut-off of −52, the signature distinguished patients with good (n = 18) and poor (n = 20) prognosis with respective median survival of 47.3 months (95% CI 29.8–52.4) and 15.5 months (95% CI 9.1–22.8) (p < 0.001; hazard ratio 21.1, 95% CI 4.7–94.9). The same signature discriminated patients with good progression-free survival (median 19.8 months; 95% CI 15.3–33.8) from the others (median 9.1 months; 95% CI 6.3–15.5) (p < 0.001; hazard ratio 3.8, 95% CI 1.7–8.7).
Validation group
miRNAs analyses were performed on 22 patients included in the validation set (Table 2). The difference between responders and non-responders, in mean expression of the two miRNAs from the predictive signature, was not statistically significant (miR-149, p = 0.07 and miR-375, p = 0.11) (Table 9). Sensitivity, specificity, positive, and negative predictive values of the signature were 33% (3/9), 77% (10/13), 50% (3/6), and 63% (10/16), respectively. Among the four miRNAs included in the prognostic signature, only miR424 retained a borderline statistical significance for survival (HR 0.66, 95% CI 0.44–1.00, p = 0.05). The respective HR value for the three other miRNAs were for miR200c 0.95 (95% CI 0.53–1.70, p = 0.85), for miR29c 1.00 (95% CI 0.43–2.34, p = 0.99) and for miR124a 0.86 (95% CI 0.48–1.52, p = 0.59). The 4-miR signature did not anymore distinguish patients with poor and good prognosis.
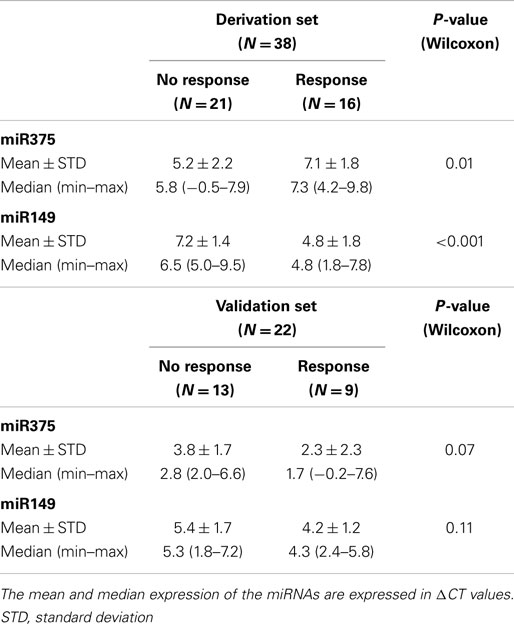
Table 9. Comparison between responders and non-responders of the expression of the two miRNAs contained in the predictive signature, in the derivation and the validation sets.
We further looked at expression levels of the six selected miRNAs involved in the predictive and prognostic signatures. Statistically significant differences in the respective ΔCT values were observed when comparing derivation and validation sets (Table 10).
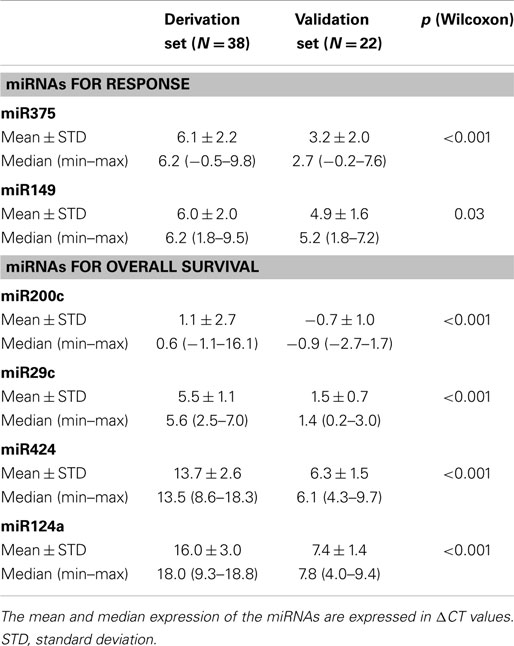
Table 10. miRNA signatures for prediction of response to chemotherapy and of survival (validation set): differential expression according to the ΔCT values between the derivation and validation sets.
Discussion
Herein, we report mature data of a prospective translational academic study performed in advanced and metastatic NSCLC, homogeneously treated with cisplatin–vinorelbine. In an attempt of searching for biological markers predicting chemosensitivity and prognostic for survival, we were able, in a derivation group, to build predictive and prognostic biological signatures based on mRNA and miRNA analyses by high throughput techniques. These signatures could not be confirmed in an independent validation group of patients treated in the same way. This study is underlying the difficulties in obtaining, in routine practice, adequate biological samples for translational research as well as the limitations for using those signatures derived from high throughput techniques.
Only 10–15% of newly diagnosed NSCLC patients will ultimately be cured (1). For the others, chemotherapy will be given at least once during the course of the disease. Cisplatin-based regimens are the cornerstone of 1st line chemotherapy for stage IV NSCLC as well as the most common combination for adjuvant therapy after surgery in completely resected stages II–III disease and for combined chemoradiotherapy. However, at least in patients treated with palliative intent, response rates are limited to 20–40% while substantial acute and chronic toxicities are encountered. A better selection of patients able to benefit, or not, from chemotherapy could theoretically help in selecting the most effective drug combinations for a particular patient; this is the purpose of customized chemotherapy. Data from randomized trials and series are suggesting that patients with poor performance status are less likely to benefit from chemotherapy than those with a good one (13). Histology is also of importance with adenocarcinomas being more susceptible to pemetrexed than squamous cell carcinomas (14). Nevertheless, there are no reliable individual predictors of chemotherapy effectiveness.
During the last decades, a better understanding of the tumors’ biology allowed in some situations to propose very active treatments when cancer is expressing a specific target. The best examples are oral tyrosine kinase inhibitors for EGFR activating mutations (5) and ALK translocation (6), with response rates around 60%, whatever the line of treatment. Other targeted therapies are currently under consideration but only a limited number of patients will have access to these very effective drugs. For cytotoxic chemotherapy, therapeutic strategies based on a genotypic analysis have been tested in randomized trials and failed to improve the common “same chemotherapy for all” approach (7, 8). Nonetheless, in these two trials, the number of considered genes was limited to ERCC1 or both ERCC1 and RRM1 that seem unlikely representing an extensive view of the biological tumor heterogeneous behavior. The problems in assessing multiple biological markers are related to the sample volume, generally limited to small biopsies in advanced and metastatic diseases, and the time necessary for performing multiple single analyses. This is an advantage for high throughput techniques like microarrays allowing yet on small samples to perform thousands of analyses at the same time. For this reason, we designed this prospective study searching for biological signatures both at the mRNA and miRNA levels.
Biological signatures have previously been published. Based on mRNA analyses, they all are dealing with survival, eventually disease-free survival, mostly in surgical stages but none was designed for searching a predictive marker of chemosensitivity. Also surgical cases are mainly representing the population used for miRNA analyses in which two signatures were tested for chemosensitivity (15, 16).
To date, more than 40 different prognostic signatures for survival, based on mRNA analyses, have been published. The signature that we identified in the derivation group was never published before. This is reflecting the large heterogeneity in the literature. In a review, only 5 genes were overlapping in a total of 327 included in seven signatures, and yet the 5 were not in common into all of them (17). Among published signatures, in most of them, there has never been any attempt to validate them in independent sets at the difference of our study. But when authors tried to do so, they cannot systematically succeed to validate their prognostic signatures (3). Potential explanations are related to heterogeneity in the patients’ and treatment’s characteristics that are frequently poorly or partially reported, to differences in the component of squamous cell and adenocarcinomas, to the statistical tests used and to the limited number of patients included in the trials that probably needs to be extensively increased for this type of translational research (18). A last component associated with a lack of reproducibility of those signatures is the heterogeneity in the platforms whatever considering the type and the number of genes tested. In a comparison of two common platforms, Affymetrix and Illumina, authors showed that the number of selected genes and their nature were not comparable with the two methods while performed on the same samples (19).
The same observations are valid for miRNA signatures. The main sources of heterogeneity are as follow: type of samples, mainly providing from surgery in patients not receiving chemotherapy but in a few cases serum has been used; the number of miRNA involved in the analyses, from a few ones to up to 880; the different techniques, single RT-PCR for very limited number of miRNA to various platforms for high throughput techniques that also are not comparable. Among more than 25 studies assessing the prognostic role of miRNA in NSCLC, the number of studies validated in an independent group of patients and taking into account other prognostic factors in a multivariate analysis was yet more limited (20–23). We found two publications analyzing the predictive role of miRNA for response to chemotherapy. In the first one, a secondary analysis from the adjuvant IALT trial including 639 completely resected NSCLC (16), 7 miRNA were considered for the analysis and the authors did not find any interaction with chemosensitivity. The second was done in a limited group of 34 SCLC patients. A three miRNA signature was associated with objective response to chemotherapy (15). We discussed the discrepancies between our study and the others in a previous publication (9).
The present study is confirming these evidences from the literature. We were able to derive, from a small but homogeneous group of patients, a single biological signature based on mRNA expression predictive of response to the cisplatin–vinorelbine regimen. We internally validated the results obtained with the microarray experiment by RT-qPCR, confirming the tumoral expression associated with the selected mRNAs and their distinction between responders and non-responders. This approach would have given us the possibility to develop a rapid, cheaper test accessible to most pathological laboratories at the difference of expensive and difficult microarrays. However, the final data from the study after attempting a validation in an independent group of patients with the same high throughput techniques was a failure despite the robust methodology of our prospective study. A major point to be primarily considered is that statistics cannot have enough power and reliability for approaching very complex biological analyses involving thousands of genes/proteins. This problem has yet been evoked in the literature when the same group using different statistics on the same samples found two distinct biological prognostic signatures (24, 25).
Our data have main strengths of which the first is the prospective nature of the present study while the majority of the published literature is dealing with retrospective studies using archival tissues. All consecutive patients presenting with suspected lung cancer were proposed to participate. This is limiting the risk of recruitment bias. As this is a prospective study, we avoided the risk of lack of data as regularly reported in retrospective series. Further, our design has foreseen, from the outset, a validation of our data in a distinct group of patients managed and treated in the same way, allowing obtaining a very homogeneous group. The validation group was treated in the same way as the derivation one and presented with similar clinical characteristics able to influence response rate and/or survival as gender, age, performance status, or stage. This was reflected in the similar response rates and survival times with no statistically significant differences (respective p-values of 0.86 and 0.63). The only non-statistically significant difference was linked to histology with a predominance of squamous cell carcinomas in the first group and adenocarcinomas in the second. It was previously pointed out that signatures derived in one histological type may not be applied in another, at least for survival (26, 27). Also, this is not as common to find this type of validation in the previous reported publications. Another strength of our study is underlined by the fact that we repeated the microarray experiment with the same methodology, after the failure of validation using RT-qPCR. Those microarrays as well as the RT-qPCR were commercial tests that can easily be reproducible at the difference of “home-made” techniques. Overall, we could conclude that biological signatures have to be considered for predictive or prognostic purpose only if they are externally validated in a group of patients presenting with the same clinical characteristics, managed and treated in the same ways as in the derivation group.
There are some limitations to our study. The first is the statistical design as there were no data or guidelines allowing us to calculate the adequate number of patients to be recruited. The aim of the study was finding a very efficient test able with a high probability predicting response or non-response to cytotoxic chemotherapy. For this purpose, we proposed different scenarios for which a maximum of 50 patients in the derivation group appears sufficient. Yet if this approach could be unsatisfying, other trials are generally not presenting statistical considerations on the sample size. Previous publications have underlined the risk of misclassifications according to the training-set sizes (28) and probably thousands of samples (18) would be needed to construct list of genes with strong statistical validity and reproducible results. This seems unrealistic when considering the major costs of microarrays and the difficulties in recruiting prospectively patients and samples in translational research in lung cancer. This study can only be achieved with an impressive grant from the first Belgian Cancer Plan. Further, to achieve recruitment of enough patients for transcriptomic analyses, we had to register 300 patients during a 4-year period of whom only 20% can be assessed both for response and transcriptomic analyses, most of them because no adequate tumor sample can be obtained during bronchoscopy. When discussing about the number of patients to be recruited in such trials (18), this will be a major limiting factor for further studies.
Another problem is the absence of distinction in our analyses between the tumor and its microenvironment. This could be a factor of discrepancy between the derivation and validation sets that cannot be approached by the case-mix evaluation using conventional clinical variables. According to the technique we used, the whole biopsy was directly lysed in Tripure, including both these components. Microdissection could be an option to avoid this problematic that cannot be done in the present setting at risk of degrading RNA by rapid activation of cell RNAses in those very small biopsies. We previously used successfully this technique of extemporaneous treatment of the biopsy that we were able to reproduce in the present study (29). We can further discuss the interest in the content of the signature. For predictive or prognostic purposes, it may be possible that the genes, by themselves, contained in the signatures have limited importance (30), while this is not the case if the research is dealing with assessment of biological cancer behavior, provided that the signature is reproducible in any situation. We limited the risk of additional contamination by other tissues by restricting the eligible biopsies to tumors accessible by conventional bronchoscopy, avoiding contamination by blood, skin, or muscle in case of percutaneous lung biopsy or inclusion of other tissues depending on metastatic sites (liver, nodes …). For the latter, at the time we designed the study, we did not have data regarding bio-equivalence between primary tumor and metastases, another reason why biopsies from metastatic sites were not considered.
A third limitation is related to the reference genes used for RT-qPCR normalization. In the second set, we decided using two genes, HPRT (as in the derivation set) and β-actin, as we observed for HPRT differential expression between responders and non-responders. This was ultimately the case with β-actin. We confirmed that without normalization for these reference genes, the ones contained in the signatures were not differentially expressed in the validation set (data not shown). Other authors have yet published on the limited sensitivity of various reference genes in lung cancer samples, due to nucleic acid degradation and that “stably expressed reference genes for normalization of gene expression data using RT-qPCR have not been identified” (31).
Based on the literature data and the results of our study, high throughput techniques have probably a limited usefulness for clinical application. According to the efficiency of targeted therapies, we have to evaluate if specific genetic abnormalities could have potential predictive or prognostic implications as suggested by meta-analyses. The evolution of sequencing allowing multiple evaluations of specific targets on the same sample is opening new options for future translational research.
This academic prospective translational study looking at the interest of biological signatures for predicting chemosensitivity and for prognostic purpose in advanced and metastatic NSCLC did not reach its primary objective of validating biological signatures either at mRNA or miRNA levels. According to the available literature, the role of high throughput techniques remains questionable due to their lack of reproducibility. Signatures derived from these analyses should not be anymore considered in absence of external validation in independent groups of patients with identical clinical characteristics and treatments.
Author Contributions
All the authors gave substantial contributions to the conception/design of the study, data acquisition, and interpretation. They all revised the manuscript and approved the final version. They agree to be accountable for all aspects of the work in ensuring that questions related to the accuracy or integrity of any part of the work are appropriately investigated and resolved.
Conflict of Interest Statement
The research was conducted in the absence of any commercial or financial relationships that could be considered as a potential conflict of interest. The study was sponsored by the Belgium National cancer Plan and the FNRS (National Scientific Research fund)-Télévie that have no influence on the design and conduct of the study, nor in the data acquisition and interpretation as well as in the writing of the manuscript.
Acknowledgments
We thank Louise Toussaint for her help in performing the RT-PCR at the Laboratory of Molecular and Cellular Biology, Gembloux.
References
1. Goldstraw P, Crowley J, Chansky K, Giroux DJ, Groome PA, Rami-Porta R, et al. The IASLC Lung Cancer Staging Project: proposals for the revision of the TNM stage groupings in the forthcoming (seventh) edition of the TNM Classification of malignant tumours. J Thorac Oncol (2007) 2(8):706–14. doi: 10.1097/JTO.0b013e31812f3c1a
2. Sculier JP, Chansky K, Crowley JJ, VanMeerbeeck J, Goldstraw P. The impact of additional prognostic factors on survival and their relationship with the anatomical extent of disease expressed by the 6th Edition of the TNM classification of malignant tumors and the proposals for the 7th Edition. J Thorac Oncol (2008) 3(5):457–66. doi:10.1097/JTO.0b013e31816de2b8
Pubmed Abstract | Pubmed Full Text | CrossRef Full Text | Google Scholar
3. Hou J, Aerts J, denHamer B, vanIjcken W, denBakker M, Riegman P, et al. Gene expression-based classification of non-small cell lung carcinomas and survival prediction. PLoS One (2010) 5(4):e10312. doi:10.1371/journal.pone.0010312
Pubmed Abstract | Pubmed Full Text | CrossRef Full Text | Google Scholar
4. NSCLC Meta-Analyses Collaborative Group. Chemotherapy in addition to supportive care improves survival in advanced non-small-cell lung cancer: a systematic review and meta-analysis of individual patient data from 16 randomized controlled trials. J Clin Oncol (2008) 26(28):4617–25. doi:10.1200/JCO.2008.17.7162
Pubmed Abstract | Pubmed Full Text | CrossRef Full Text | Google Scholar
5. Gao G, Ren S, Li A, Xu J, Xu Q, Su C, et al. Epidermal growth factor receptor-tyrosine kinase inhibitor therapy is effective as first-line treatment of advanced non-small-cell lung cancer with mutated EGFR: a meta-analysis from six phase III randomized controlled trials. Int J Cancer (2012) 131(5):E822–9. doi:10.1002/ijc.27396
Pubmed Abstract | Pubmed Full Text | CrossRef Full Text | Google Scholar
6. Shaw AT, Kim DW, Nakagawa K, Seto T, Crino L, Ahn MJ, et al. Crizotinib versus chemotherapy in advanced ALK-positive lung cancer. N Engl J Med (2013) 368(25):2385–94. doi:10.1056/NEJMoa1214886
Pubmed Abstract | Pubmed Full Text | CrossRef Full Text | Google Scholar
7. Bepler G, Williams C, Schell MJ, Chen W, Zheng Z, Simon G, et al. Randomized international phase III trial of ERCC1 and RRM1 expression-based chemotherapy versus gemcitabine/carboplatin in advanced non-small-cell lung cancer. J Clin Oncol (2013) 31(19):2404–12. doi:10.1200/JCO.2012.46.9783
Pubmed Abstract | Pubmed Full Text | CrossRef Full Text | Google Scholar
8. Cobo M, Isla D, Massuti B, Montes A, Sanchez JM, Provencio M, et al. Customizing cisplatin based on quantitative excision repair cross-complementing 1 mRNA expression: a phase III trial in non-small-cell lung cancer. J Clin Oncol (2007) 25(19):2747–54. doi:10.1200/JCO.2006.09.7915
Pubmed Abstract | Pubmed Full Text | CrossRef Full Text | Google Scholar
9. Berghmans T, Ameye L, Willems L, Paesmans M, Mascaux C, Lafitte JJ, et al. Identification of microRNA-based signatures for response and survival for non-small cell lung cancer treated with cisplatin-vinorelbine A ELCWP prospective study. Lung Cancer (2013) 82(2):340–5. doi:10.1016/j.lungcan.2013.07.020
Pubmed Abstract | Pubmed Full Text | CrossRef Full Text | Google Scholar
10. Traitement des cancers bronchiques non à petites cellules: maladies avancées (métastatiques). Recommandations de pratique clinique de l’European Lung Cancer Working Party. Rev Med Brux (2007) 28(6):495–511.
12. Hochberg Y, Benjamini Y. More powerful procedures for multiple significance testing. Stat Med (1990) 9(7):811–8. doi:10.1002/sim.4780090710
Pubmed Abstract | Pubmed Full Text | CrossRef Full Text | Google Scholar
13. Berghmans T, Lafitte JJ, Scherpereel A, Paesmans M, Lecomte J, Marco VG, et al. An ELCWP phase III trial comparing ifosfamide and cisplatin regimens in advanced NSCLC. Anticancer Res (2013) 33(12):5477–82.
14. Treat J, Scagliotti GV, Peng G, Monberg MJ, Obasaju CK, Socinski MA. Comparison of pemetrexed plus cisplatin with other first-line doublets in advanced non-small cell lung cancer (NSCLC): a combined analysis of three phase 3 trials. Lung Cancer (2012) 76(2):222–7. doi:10.1016/j.lungcan.2011.10.021
Pubmed Abstract | Pubmed Full Text | CrossRef Full Text | Google Scholar
15. Ranade AR, Cherba D, Sridhar S, Richardson P, Webb C, Paripati A, et al. MicroRNA 92a-2*: a biomarker predictive for chemoresistance and prognostic for survival in patients with small cell lung cancer. J Thorac Oncol (2010) 5(8):1273–8. doi:10.1097/JTO.0b013e3181dea6be
Pubmed Abstract | Pubmed Full Text | CrossRef Full Text | Google Scholar
16. Voortman J, Goto A, Mendiboure J, Sohn JJ, Schetter AJ, Saito M, et al. MicroRNA expression and clinical outcomes in patients treated with adjuvant chemotherapy after complete resection of non-small cell lung carcinoma. Cancer Res (2010) 70(21):8288–98. doi:10.1158/0008-5472.CAN-10-1348
Pubmed Abstract | Pubmed Full Text | CrossRef Full Text | Google Scholar
17. Roepman P, Jassem J, Smit EF, Muley T, Niklinski J, vandeVelde T, et al. An immune response enriched 72-gene prognostic profile for early-stage non-small-cell lung cancer. Clin Cancer Res (2009) 15(1):284–90. doi:10.1158/1078-0432.CCR-08-1258
Pubmed Abstract | Pubmed Full Text | CrossRef Full Text | Google Scholar
18. Ein-Dor L, Zuk O, Domany E. Thousands of samples are needed to generate a robust gene list for predicting outcome in cancer. Proc Natl Acad Sci U S A (2006) 103(15):5923–8. doi:10.1073/pnas.0601231103
Pubmed Abstract | Pubmed Full Text | CrossRef Full Text | Google Scholar
19. Chang JW, Wei NC, Su HJ, Huang JL, Chen TC, Wu YC, et al. Comparison of genomic signatures of non-small cell lung cancer recurrence between two microarray platforms. Anticancer Res (2012) 32(4):1259–65.
20. Saito M, Schetter AJ, Mollerup S, Kohno T, Skaug V, Bowman ED, et al. The association of microRNA expression with prognosis and progression in early-stage, non-small cell lung adenocarcinoma: a retrospective analysis of three cohorts. Clin Cancer Res (2011) 17(7):1875–82. doi:10.1158/1078-0432.CCR-10-2961
Pubmed Abstract | Pubmed Full Text | CrossRef Full Text | Google Scholar
21. Tan X, Qin W, Zhang L, Hang J, Li B, Zhang C, et al. A 5-microRNA signature for lung squamous cell carcinoma diagnosis and hsa-miR-31 for prognosis. Clin Cancer Res (2011) 17(21):6802–11. doi:10.1158/1078-0432.CCR-11-0419
Pubmed Abstract | Pubmed Full Text | CrossRef Full Text | Google Scholar
22. Yanaihara N, Caplen N, Bowman E, Seike M, Kumamoto K, Yi M, et al. Unique microRNA molecular profiles in lung cancer diagnosis and prognosis. Cancer Cell (2006) 9(3):189–98. doi:10.1016/j.ccr.2006.01.025
Pubmed Abstract | Pubmed Full Text | CrossRef Full Text | Google Scholar
23. Yu SL, Chen HY, Chang GC, Chen CY, Chen HW, Singh S, et al. MicroRNA signature predicts survival and relapse in lung cancer. Cancer Cell (2008) 13(1):48–57. doi:10.1016/j.ccr.2007.12.008
Pubmed Abstract | Pubmed Full Text | CrossRef Full Text | Google Scholar
24. Boutros PC, Lau SK, Pintilie M, Liu N, Shepherd FA, Der SD, et al. Prognostic gene signatures for non-small-cell lung cancer. Proc Natl Acad Sci U S A (2009) 106(8):2824–8. doi:10.1073/pnas.0809444106
Pubmed Abstract | Pubmed Full Text | CrossRef Full Text | Google Scholar
25. Lau SK, Boutros PC, Pintilie M, Blackhall FH, Zhu CQ, Strumpf D, et al. Three-gene prognostic classifier for early-stage non small-cell lung cancer. J Clin Oncol (2007) 25(35):5562–9. doi:10.1200/JCO.2007.12.0352
Pubmed Abstract | Pubmed Full Text | CrossRef Full Text | Google Scholar
26. Sun Z, Wigle DA, Yang P. Non-overlapping and non-cell-type-specific gene expression signatures predict lung cancer survival. J Clin Oncol (2008) 26(6):877–83. doi:10.1200/JCO.2007.13.1516
Pubmed Abstract | Pubmed Full Text | CrossRef Full Text | Google Scholar
27. Zhu CQ, Strumpf D, Li CY, Li Q, Liu N, Der S, et al. Prognostic gene expression signature for squamous cell carcinoma of lung. Clin Cancer Res (2010) 16(20):5038–47. doi:10.1158/1078-0432.CCR-10-0612
Pubmed Abstract | Pubmed Full Text | CrossRef Full Text | Google Scholar
28. Michiels S, Koscielny S, Hill C. Prediction of cancer outcome with microarrays: a multiple random validation strategy. Lancet (2005) 365(9458):488–92. doi:10.1016/S0140-6736(05)17866-0
Pubmed Abstract | Pubmed Full Text | CrossRef Full Text | Google Scholar
29. Mascaux C, Laes JF, Anthoine G, Haller A, Ninane V, Burny A, et al. Evolution of microRNA expression during human bronchial squamous carcinogenesis. Eur Respir J (2009) 33(2):352–9. doi:10.1183/09031936.00084108
Pubmed Abstract | Pubmed Full Text | CrossRef Full Text | Google Scholar
30. Lu Y, Lemon W, Liu PY, Yi Y, Morrison C, Yang P, et al. A gene expression signature predicts survival of patients with stage I non-small cell lung cancer. PLoS Med (2006) 3(12):e467. doi:10.1371/journal.pmed.0030467
Pubmed Abstract | Pubmed Full Text | CrossRef Full Text | Google Scholar
31. Soes S, Sorensen BS, Alsner J, Overgaard J, Hager H, Hansen LL, et al. Identification of accurate reference genes for RT-qPCR analysis of formalin-fixed paraffin-embedded tissue from primary non-small cell lung cancers and brain and lymph node metastases. Lung Cancer (2013) 81(2):180–6. doi:10.1016/j.lungcan.2013.04.007
Pubmed Abstract | Pubmed Full Text | CrossRef Full Text | Google Scholar
Keywords: non-small cell lung cancer, mRNA, miRNA, chemotherapy, survival
Citation: Berghmans T, Ameye L, Lafitte J-J, Colinet B, Cortot A, CsToth I, Holbrechts S, Lecomte J, Mascaux C, Meert A-P, Paesmans M, Richez M, Scherpereel A, Tulippe C, Willems L, Dernies T, Leclercq N and Sculier J-P (2015) Prospective validation obtained in a similar group of patients and with similar high throughput biological tests failed to confirm signatures for prediction of response to chemotherapy and survival in advanced NSCLC: a prospective study from the European Lung Cancer Working Party. Front. Oncol. 4:386. doi: 10.3389/fonc.2014.00386
Received: 17 October 2014; Paper pending published: 17 November 2014;
Accepted: 24 December 2014; Published online: 28 January 2015.
Edited by:
Frank Griesinger, University of Oldenburg, GermanyReviewed by:
Sacha I. Rothschild, University Hospital Basel, SwitzerlandRabab Mohamed Gaafar, National Cancer Institute, Egypt
Copyright: © 2015 Berghmans, Ameye, Lafitte, Colinet, Cortot, CsToth, Holbrechts, Lecomte, Mascaux, Meert, Paesmans, Richez, Scherpereel, Tulippe, Willems, Dernies, Leclercq and Sculier. This is an open-access article distributed under the terms of the Creative Commons Attribution License (CC BY). The use, distribution or reproduction in other forums is permitted, provided the original author(s) or licensor are credited and that the original publication in this journal is cited, in accordance with accepted academic practice. No use, distribution or reproduction is permitted which does not comply with these terms.
*Correspondence: Jean-Paul Sculier, Department of Intensive Care and Oncological Emergencies and Thoracic Oncology Clinic, Institut Jules Bordet, Université Libre de Bruxelles (ULB), Rue Héger-Bordet 1, Brussels 1000, Belgium e-mail:c2N1bGllckBib3JkZXQuYmU=