- 1INSERM U1129, CEA, Paris Descartes University, Paris, France
- 2UNIACT, Institut Joliot, DRF, Neurospin, CEA, Paris Saclay University, Gif-sur-Yvette, France
- 3Department of Pediatric and Adolescent Oncology, Gustave Roussy, Villejuif, France
- 4Radiation Oncology Department, Gustave Roussy Cancer Campus, Villejuif, France
- 5INSERM, U1030, Villejuif, France
- 6Paris Sud University, Paris-Saclay University, Villejuif, France
- 7Gustave Roussy, Paris-Saclay University, Department of Medical Physics, Villejuif, France
- 8INSERM U992 Unicog CEA, Neurospin, Paris Descartes University, Paris, France
- 9Institut Joliot, Neurospin, CEA, Paris-Saclay University, Gif-sur-Yvette, France
- 10CSI Department for Children with Acquired Brain Injury, Hopitaux de Saint Maurice, Saint-Maurice, France
- 11Paris Sud University, Orsay, France
Pediatric posterior fossa tumor (PFT) survivors who have been treated with cranial radiation therapy often suffer from cognitive impairments that might relate to IQ decline. Radiotherapy (RT) distinctly affects brain regions involved in different cognitive functions. However, the relative contribution of regional irradiation to the different cognitive impairments still remains unclear. We investigated the relationships between the changes in different cognitive scores and radiation dose distribution in 30 children treated for a PFT. Our exploratory analysis was based on a principal component analysis (PCA) and an ordinary least square regression approach. The use of a PCA was an innovative way to cluster correlated irradiated regions due to similar radiation therapy protocols across patients. Our results suggest an association between working memory decline and a high dose (equivalent uniform dose, EUD) delivered to the orbitofrontal regions, whereas the decline of processing speed seemed more related to EUD in the temporal lobes and posterior fossa. To identify regional effects of RT on cognitive functions may help to propose a rehabilitation program adapted to the risk of cognitive impairment.
Introduction
Posterior fossa tumors (PFTs) account for two-thirds of all pediatric brain tumors (1). The most common malignant PFT is medulloblastoma (40%), followed by ependymoma (10%) (2). As a result of improved treatment, event-free survival has significantly increased (3). However, these children suffer from varied cognitive impairments, the most frequently described being decreased sustained attention, working memory, and information processing speed (4). This latter impairment seems to appear first in PFT patients treated with cranio-spinal irradiation (CSI) (4). These cognitive impairments might relate to the decline of global intellectual functioning (full scale IQ, FSIQ) reported to be between two and four points per year (5–9). Several factors have been shown to predict cognitive impairments in PFT patients. Radiotherapy (RT) has been considered to be the major one, especially in young children (6, 8). Three RT-associated risk factors have been highlighted as predictors of cognitive impairments: (i) CSI (6, 7, 10), (ii) the volume receiving the boost [i.e., to the posterior fossa (PF)] (11), and (iii) the dose per fraction (12, 13). Grill et al. (10) observed that PFT survivors with low CSI (25 Gy) showed better cognitive outcomes than those receiving high CSI (36 Gy). Nonetheless, the reduction of CSI dose (14) did not prevent IQ decline (9). An alternative way to decrease cognitive impairments has been to reduce the volume of the PF irradiated, in addition to the reduced CSI. While the PF received the highest dose, the boost dose also contributed to higher doses in other regions such as the temporal lobes, the brainstem, and the hypothalamus (11). Moxon-Emre et al. (15) showed that medulloblastoma survivors for whom the CSI was reduced, and the boost volume was reduced from the entire PF to the tumor bed, had preserved IQ over time. Nonetheless, medulloblastoma survivors treated via either a CSI dose reduction or a diminution of PF volume irradiated (tumor bed boost) still experienced a decline of IQ.
Recent studies reported a higher contribution of specific brain regions to the development of RT-induced cognitive decline. Jalali et al. (16) observed that more than 43.2 Gy to >13% of the left temporal lobe was predicting IQ decline in patient treated for a benign tumor with stereotactic conformal RT. Merchant et al. (6) assessed the impact on IQ change of different mean dose values in distinct regions (whole brain, temporal lobe, hippocampus, infratentorial, and supratentorial spaces) in patients treated for a medulloblastoma, and suggested that supratentorial space was the most sensitive across the brain. Using a neurocognitive questionnaire, Armstrong et al. (17) pointed out a strong association between maximum radiation dose to the temporal lobe and poor performance in Task efficiency (i.e., attention and processing speed) and Organization. These subscores were measured as given by the Childhood Cancer Survivor Study Neurocognitive Questionnaire. While these studies did not identify a relationship between radiation dose of PF and changes in cognitive scores, such associations have been reported in children with ependymoma (18).
Despite marked progress, the regional effect of RT on cognitive impairment still remains unclear. So far, research on this question has been mainly carried out on either single (19) or large (6) brain regions, limiting the analysis to specific anatomical structures. In this study, we implemented a whole brain analysis; to investigate the relation between regional biological dose and changes over time of different cognitive scores (IQ, processing speed, and working memory) in 30 patients treated for a PFT. The use of a principal component analysis (PCA) was an innovative way to cluster correlated irradiated regions due to similar radiation therapy protocols across patients. We aimed to describe the relationships between regional radiation dose and declines in specific cognitive functions.
Patients and Methods
Patient’s Characteristics
Inclusion criteria were (1) PFT patients treated at Gustave Roussy Cancer Campus between 2000 and 2014; (2) 17 years of age or under at diagnosis (3) multiple (>2) IQ assessments after treatment onset (4); for the PFT patients treated with radiation therapy, the computed tomography (CT) scan, T1-weighted MRI, and dosimetric maps had to be available. Thirty patients (14 males and 16 females) matched these criteria. Information was gathered from medical files about the history of the illness (i.e., age at diagnosis) and the type of treatment (i.e., surgery, chemotherapy, and radiation therapy protocol). The underlying malignancy of the 30 patients studied was medulloblastoma, ependymoma, astrocytoma, and embryonal tumor in 25, 3, 1, and 1 patients, respectively. Twenty patients had a localized disease and 10 had a metastatic disease. Complete tumor resection was achieved in 20 PFT. Post-operative complications occurred in 10 patients. No patient relapsed between the two evaluations, but the patient with an astrocytoma whose relapse before the first evaluation, was treated with chemotherapy alone. The mean age at diagnosis was 4.62 years (SD = 3.05; [0.49; 12.24]). The mean delay between treatment and the last assessment was 4.60 years (SD = 4.60; [1.28; 14.24]). Pre-operative hydrocephalus was present in 19 patients (63%). Seventeen patients were treated with RT alone (N = 7) or RT and chemotherapy (N = 10). The remaining patients were treated with chemotherapy alone and were used as controls. All patients with RT received a CSI and a boost in the PF and were treated with three-dimensional conformal radiation therapy (Table 1). This study was approved by an ethical committee (CPP no. 14973, Gustave Roussy, Villejuif, France).
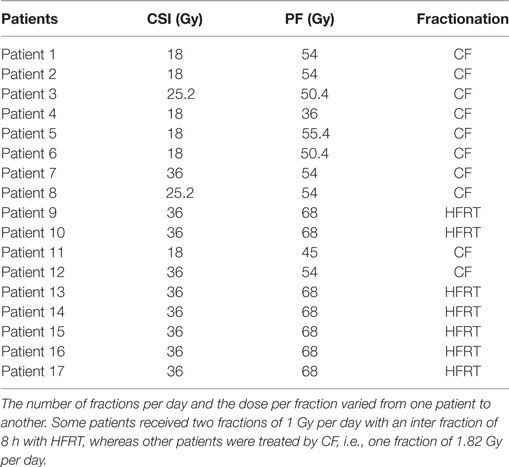
Table 1. Absorbed dose and type of fractionation [conformational fractionation (CF) vs. hyperfractionated radiotherapy (HFRT)] prescribed to the cranio-spinal irradiation (CSI) and posterior fossa (PF) for the 17 patients.
Neuropsychological Assessments
Three cognitive indices were estimated from age appropriate Wechsler Intelligence Scale (20, 21): FSIQ in all patients and the processing speed index (PSI) and working memory index (WMI) when available. Neuropsychological assessments were done by formally trained neuropsychologists from the pediatric department. Because of age or time constraints, not all participants were administered all the tests. Thus, the numbers of patients assessed varied across cognitive scores [N (ΔFSIQ) = 30, N (ΔPSI) = 23, N (ΔWMI) = 14]. Patients were evaluated at variable time points after treatment onset. Thus, the delay (T) between two neuropsychological assessments varied from one patient to the other (Table 2). The change in cognitive scores (ΔFSIQ, ΔPSI, and ΔWMI) of each patient was calculated from the difference between the first and last scores (ΔT). We did not consider intermediate scores. Changes in cognitive scores (ΔFSIQ, ΔWMI, and ΔPSI) were compared using two-tailed Student’s t-tests.
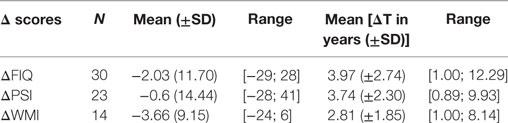
Table 2. Changes in the three measured cognitive scores [Delta (Δ)] with the corresponding number of evaluated patients: mean score change (±SD, range) and mean test interval ΔT (±SD, range).
Neuroimaging Data
To study regional dose effects on changes in cognitive scores, 3D-T1 MRI, CT scan, and absorbed dose maps of patients treated with RT (n = 17) were collected and processed to create individual dose distribution maps into selected brain regions of interest (ROI) covering the whole brain.
Image Collection
3D T1-MR images were acquired on a 3-T scanner using a 32-channel head coil (General Electric, Milwankee, MN, USA). In this clinical retrospective study, two types of T1-weighted images were collected: 3D T1-weighted sagittal slices (matrices: 256 mm × 256 mm, pixel size: 0.5 mm, slice thickness: 1 mm, FOV: 240 mm) and 3D-T1 weighted axial slices (matrices: 224 × 288, pixel size: 0.5 mm, slice thickness: 1 mm).
Computed tomography scans were acquired on a SIEMENS Sensation Open scanner located in Gustave Roussy RT department (matrices: 512 mm × 512 mm, pixel size: 0.8 mm, slice thickness: 3 mm). Radiation dose maps (RD maps) were computed with the ISOgray™ Treatment Planning System (DOSIsoft, version 4.1, Cachan, France). The Clarkson–Cunningham model was used for dose calculation. Dose maps resolution was 3 mm × 3 mm × 1 mm.
Image Analysis
Image Preprocessing
We designed a five-step preprocessing pipeline to identify anatomical ROI on dose maps (Figure 1).
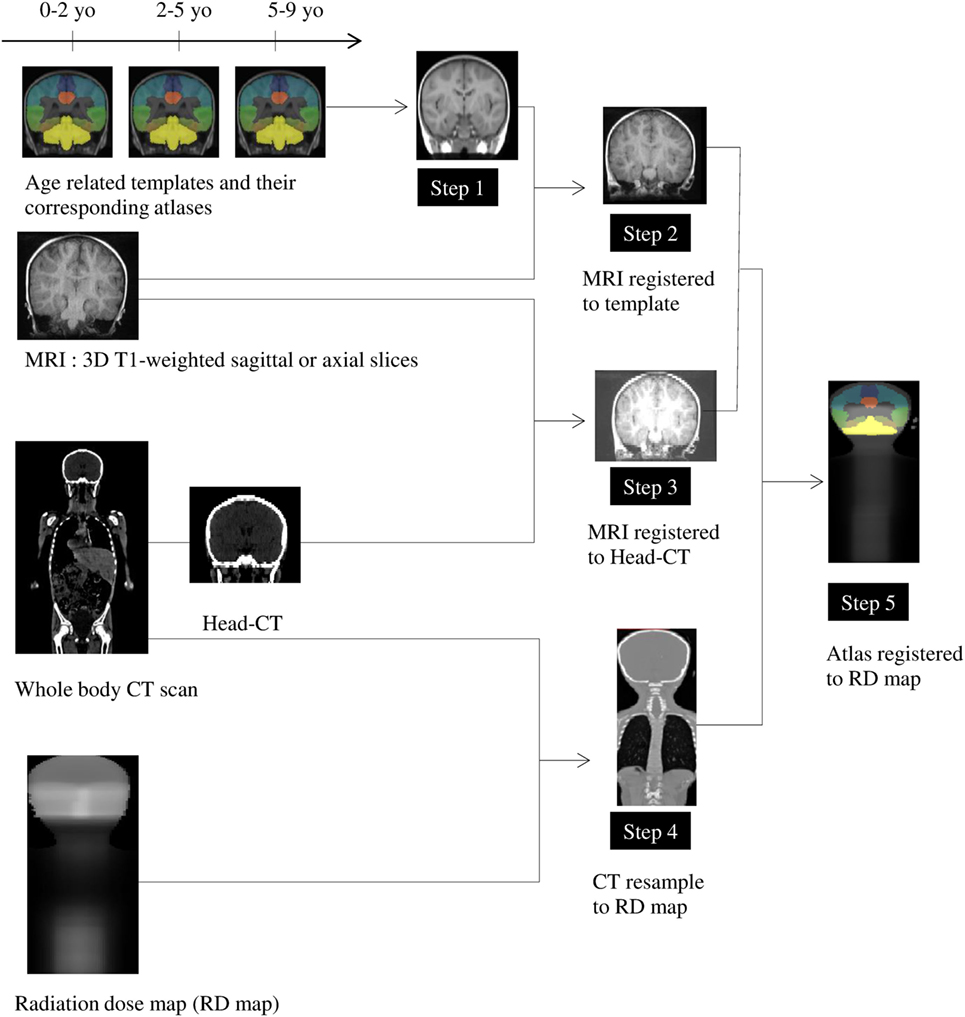
Figure 1. Preprocessing pipeline (see Patients and Methods). Step 1: Selection of age appropriate templates. Step 2: Registration of the selected template on individual patient 3DT1 image. Step 3: Registration of individual 3DT1 image to the corresponding individual CT. Step 4: Down-sampling of CT to match the corresponding radiation dose map. Step 5: Registration of the selected template on the individual dose map coordinate system.
Step 1: We chose three MRI templates specific to ages 0–2, 2–5, and 5–9 years (151 × 192 × 152 voxels, 1 mm × 1 mm × 1 mm voxel size) and three corresponding anatomical atlases (151 × 192 × 152 voxels, 1 mm × 1 mm × 1 mm) from the Neurodevelopmental MRI DataBase (22). The atlases contained 56 ROIs extracted from the LPBA40 atlas (23) that were adapted to selected ages thanks to label propagation and decision fusion methods (24). For each child, we selected both the atlas and associated template according to the age at which the child received radiation therapy, to be as close as possible to the individual anatomy, which varies significantly during development (22, 25). Since the atlases did not included some particular regions (i.e., corpus callosum, a part of internal capsule, and ventricles), we created a supplementary label that encompassed these regions, resulting in 57 ROIs.
Step 2: The selected template was warped to individual patient 3DT1 image using a non-linear registration tool [Advanced Normalization Tools, SyN (26), and ANTS (27)].
Step 3: Individual MR images were also registered to the corresponding individual CT scan by applying a linear transformation with FSL [FLIRT (28)].
Step 4: Each CT scan was then down sampled to match the corresponding RD map voxel sampling.
Step 5: Finally, we combined the computed transformations into a single concatenated transformation from the template space to the individual dose map coordinate system. This enabled us to perform statistical radiation dose analyses over the group in each ROI extracted from the template.
Individual registrations have been assessed qualitatively by two experimenters independently and by consensus. From this check, four subjects were excluded from the study. In the majority of cases, registrations have been adjusted manually to optimize intersubject comparisons.
Data Analysis
We designed a four-step analysis pipeline to determine the associations between both clinical variables and ROI dose distribution with changes in cognitive scores (Figure 2).
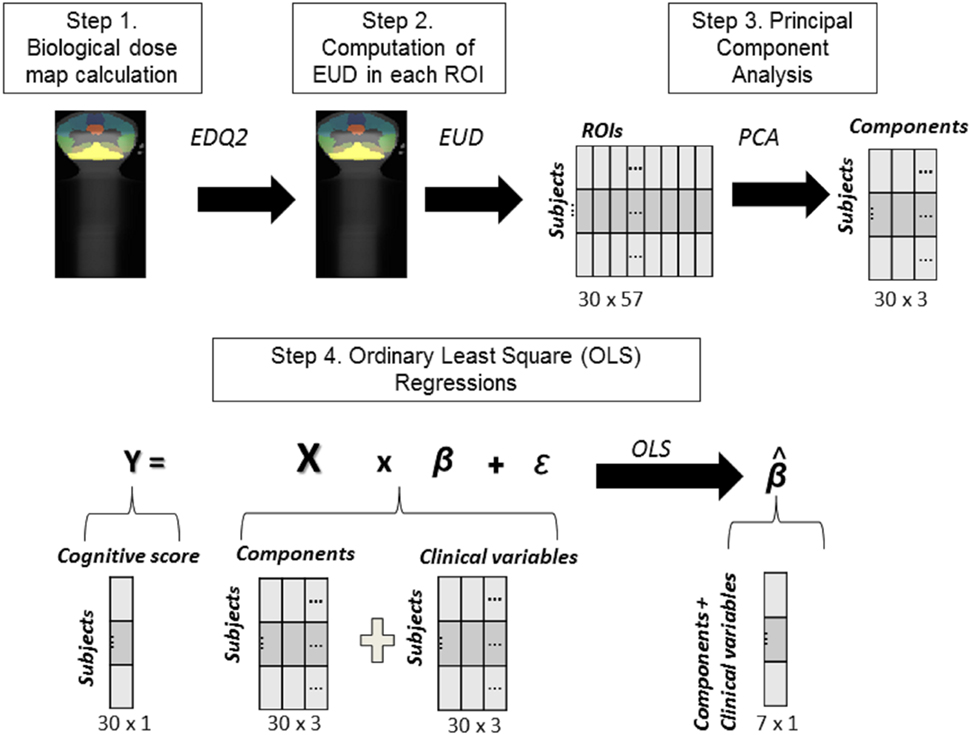
Figure 2. Steps of the analysis. Step 1: Equating dose maps across patients: EQD2 computation. Step 2: Calculation of dose index in each ROI: equivalent uniform dose computation. Step 3: Principal component analysis approach. Step 4: Highlighting the respective contribution of clinical variables and PC-EUD on clinical scores changes using ordinary least square regression.
Equating Dose Maps across Patients: EQD2 Computation
Step 1: Given the differences in fractionation parameters (dose per fraction and number of fractions per day varied from one patient to another), even at equal total doses, the biological effectiveness of these two types of irradiation will be different (Figure 2, step 1). However, using the linear quadratic model (29), it is possible to calculate the total dose equivalent in terms of biological effects for two different fractionations (dose per fraction, time interval between two fractions) and a given tissue (EDQ2). Using this equation, all treatments are thus reduced to biological dose equivalent to treatments performed with fractions of 2 Gy, which is the standard fractionation scheme. Therefore, we corrected the dose of all fractionation types in a uniform way by calculating in each voxel the equivalent dose with the EQD2 formula (30) (Eq. 1; Figure 2, step 1). The EQD2 was calculated taking into account a function (Hm) depending on the number of equally spaced fractions per day; the dose per fraction (d) and the sensitivity of the tissue (α/β). D (the total delivered dose in Gy) and d varied across patients. Based on the current literature, α/β was fixed to 2 and T1/2 to 3 h (31):
Calculation of Dose Index: Equivalent Uniform Dose (EUD) Computation
Step 2: After calculating the biological dose map of each patient, for all subjects and ROIs we computed the EUD that accounts for heterogeneity of dose distribution, as follows (Eq. 2) (Figure 2, step 2):
Equivalent uniform dose corresponds to the value of a homogeneous dose that would cause the same clinical effect than the corresponding heterogeneous dose distribution (30). k was fixed at 5 according to the work of Emami et al. (32). We standardized EUD across the 17 subjects for each of the 57 ROIs.
Taking into Account the Spatial Correlation of Radiation Doses across ROIs: PCA Approach
Step 3: Because of the radiation therapy protocol (i.e., CSI and boost in the PF with three-dimensional conformal radiation therapy), EUD was highly correlated across brain regions (Figure 2, step 3). Therefore, it was not possible to assess the effect of irradiation on cognitive scores in each region with an ordinary least square regression, as regression weights would be highly unstable. Thus, we ran a PCA, a data-driven method that clusters correlated variables into common factors named principal components (PCs). In this approach, highly correlated variables share higher weights within each factor/component, but components are uncorrelated. The PCA enabled us (1) to obtain uncorrelated components representative of the radiation dose distribution variability across subjects, each component revealing a brain network with a particular radiation pattern and (2) to reduce the number of variables in our model, as sample size was limited. We performed a PCA taking the ROIs normalized EUD as variables (Figure 2, step 3). Then, we selected the n < 57 PCs accounting for 90% of the variance (33). Due to the high correlation between regions, we recovered only three components (PCs). To figure out the spatial contribution of the ROIs on each PC-EUD, we computed the correlations between EUD in each ROI and each PC-EUD, and projected the correlation coefficients onto a glass brain.
Highlighting the Respective Contribution of Clinical Variables and EUD-PCs on Clinical Score Changes
Step 4: We then considered the computed PCs-EUD and the clinical variables (chemotherapy, time since diagnosis, age at diagnosis, and ΔT) in a least square regression (Figure 2, step 4). We first checked for multicollinearity that could induce a biased estimation and a loss of power (34), using the variance inflation factor, which summarizes how an independent variable is explained by other variables. We removed regressors with a variance inflation factor >10 (35). In each regression, we examined t-scores to determine which variable had the most important effect on the cognitive scores of these 30 patients.
All analyses and plots were computed using the Python libraries, Nilearn, Scikit Learn, and Statsmodels (36, 37).
Results
Neuropsychological Performance
At time of first neuropsychological assessment, the mean estimated IQ over the whole population was 87.5 (SD = 18.4; [45–130]). A declining performance over time was observed in 67, 64, and 48% of the patients for ΔFSIQ, ΔWMI, and ΔPSI, respectively. The remaining patients showed either preserved or better performance over time. However, there were no statistically significant differences between cognitive scores [ΔFSIQ vs. ΔWMI: t(32) = −0.64, p = 0.52; ΔPSI vs. ΔWMI: t(35) = −0.81, p = 0.42; ΔFSIQ vs. ΔPSI: t(51) = −0.37, p = 0.70] (Table 2). Moreover, ANOVAs were conducted to compare the three treatment groups (chemotherapy alone vs. RT and chemotherapy vs. RT alone) on their cognitive scores (ΔFSIQ, ΔWMI, and ΔPSI). There was no statistically significant difference between treatment groups in ΔFSIQ [F(2,30) = 2.36; p = 0.11; RT alone: M = −10.5 (±9.11), chemotherapy alone: M = 1.57 (±11.28), RT and chemotherapy: M = −2.0 (±11.03)]; and ΔWMI [F(2,14) = 1.17; p = 0.34; RT alone: M = −10 (±9.90), chemotherapy alone: M = −0.29 (±5.90), RT and chemotherapy: M = −4.60 (±10.15)], and ΔPSI [F(2,24) = 2.28; p = 0.12; RT alone: M = −10 (±9.90), chemotherapy alone: M = −0.29 (±5.90), RT and chemotherapy: M = −4.60 (±10.15)].
PCs Extracted from EUD of Anatomical ROIs
PC1-EUD, which explained 67% of the variance of original data, was strongly correlated (>0.50) with the dose (EUD) in all regions, especially in the supratentorial space. PC2-EUD explained 19% of the variance and was positively correlated with 16 regions in the PF, inferior occipital and temporal regions (see Table S1 in Supplementary Material). Meanwhile, three regions in the left superior occipital and parietal regions correlated negatively and moderately (>0.40) with PC2-EUD (see Table S1 in Supplementary Material). PC3-EUD explained 5% of the variance and had a moderate positive correlation (>0.40) with the EUD in the left orbitofrontal area. By contrast, the precuneus and the right cuneus negatively correlated with PC3-EUD. Values of all correlation coefficients are shown in Table S2 in Supplementary Material.
Effects of Clinical Variables and EUD Components on Cognitive Score Changes
Our final regression models included the three PCs-EUD, chemotherapy, age at diagnosis, and delay between assessments (ΔT). Indeed, in all models, time since diagnosis (variance inflation factor >10) was highly correlated with ΔT (variance inflation factor >10), while it was not the case between PCs (variance inflation factor <10), chemotherapy (variance inflation factor <10), and age at diagnosis (variance inflation factor <10). We thus removed time since diagnosis from the analysis and checked that all remaining variance inflation factor indices were below 10.
Clinical Variables and Cognitive Score Changes
ΔFSIQ was significantly negatively affected by age at diagnosis and interval between assessments (ΔT), and positively influenced by chemotherapy. These variables had no significant effect on the other scores (Table 3).

Table 3. Effects on changes of cognitive scores (ΔFSIQ, ΔPSI, and ΔWMI) of the clinical variables and the components of the principal component analysis, according to our models (see Patients and Methods).
EUD Components and Cognitive Score Changes
ΔWMI was clearly negatively associated with both PC1-EUD and PC3-EUD and marginally by PC2-EUD (Table 3) PC3-EUD had the highest effect on ΔWMI, followed by PC1-EUD and PC2-EUD (Figure 3). The decline of WMI was first associated with an increase of EUD in left orbitofrontal area (PC3-EUD) and then with an increase of EUD in all regions, especially in the supratentorial space (PC1-EUD). By contrast, an EUD increase in the precuneus and right cuneus was positively associated with ΔWMI (Figure 3).
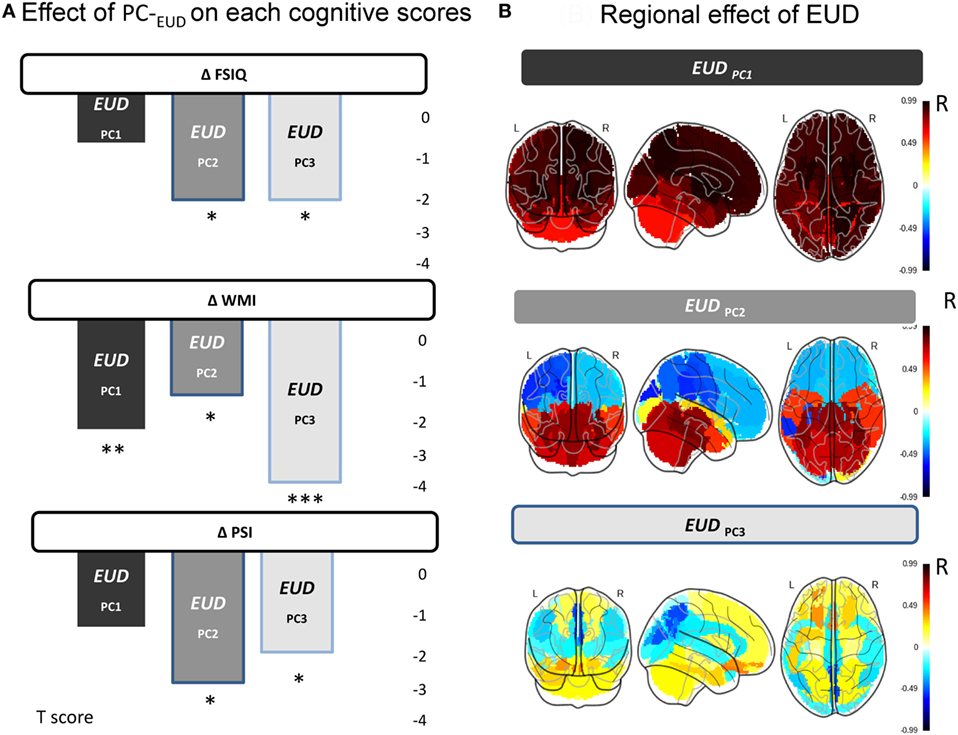
Figure 3. Summary of results: impact of equivalent uniform dose (EUD) principal components on cognitive changes (ΔFSIQ, ΔPSI, and ΔWMI). (A) Effects of EUD components on each cognitive score (ΔFSIQ, ΔPSI, and ΔWMI). The weights of each PC-EUD on the cognitive change are displayed in gray color scale, with significance levels (*p ≤ 0.05: **p ≤ 0.01: ***p ≤ 0.001). (B) Regional effects of EUD. The color scale displays the regional correlation coefficients R between EUD and the PC-EUD in each ROI, i.e., the relative participation of each ROI on each EUD component (with higher positive correlations shown in red, stronger negative correlations in blue).
Only PC2-EUD and PC3-EUD were found to have a negative and significant effect on ΔPSI, with a seemingly higher effect of PC2-EUD than of PC3-EUD, contrarily to ΔWMI (Table 3). The decline of PSI was first associated with an EUD increase in the PF, inferior occipital and temporal regions (PC2-EUD) followed by an increase in the left orbitofrontal area (PC3-EUD). By contrast, PC2-EUD and PC3-EUD were positively associated with ΔPSI in superior occipital and parietal regions (Figure 3).
Finally, PC2-EUD and PC3-EUD had similar and nearly significant negative effects on ΔFSIQ (Table 3) The decline of FSIQ was similarly associated with the increase of EUD in the PF, inferior occipital, temporal regions, and left orbitofrontal areas (PC3-EUD and PC2-EUD). By contrast, EUD in superior occipital and parietal regions was positively associated with ΔFSIQ (PC2-EUD and PC3-EUD) (Figure 3).
Discussion
Our main results suggest different regional associations between radiation dose (EUD) and changes in cognitive scores in patients treated for PFTs. In particular, we highlighted a link between working memory decline and radiation dose in the orbitofrontal region, whereas the decline in processing speed seemed more related to irradiation of the temporal lobes and the PF.
Effect of Clinical Variables on Cognitive Score Changes
Consistently with previous studies (5, 6), the FSIQ decline depended on the delay between the two IQ tests. As shown in previous studies (5, 38), chemotherapy does not seem to have a significant negative impact on PSI and WMI functioning. The surprising positive effect of chemotherapy on FSIQ change might be linked to the positive impact of repeated measurements, also known as the carry over effect (or IQ test–retest) (39). Children acquired expertise concerning neuropsychological task along many neuropsychological tests, improving their performances. Therefore, the change in cognitive scores of each patient calculated from the difference between the first and last scores was positive. A large portion of children with chemotherapy alone showed an IQ improvement which confirms the absence of cognitive effect of chemotherapy (5, 38).
This also explains the unexpected negative effect of age at diagnosis on FSIQ change, as children treated with chemotherapy alone are usually young (below 5 years) at diagnosis.
ROIs EUD and Cognitive Score Changes
All components seem to have specific impacts on changes of the working memory score (WMI). The radiation distribution pattern involving the left orbitofrontal regions (PC3-EUD) had the most negative impact on working memory. Interestingly, this result could be in line with Mabbott et al.?’s findings (40). They observed that working memory performance over time was different according to the tumor location in children treated for a central nervous system germ cell tumor. Patients with pineal tumors showed early, but stable, working memory deficit, whereas patients with suprasellar tumors experienced a significant working memory decline over time. Mabbott et al. suggested the observed decline was related to the radiation field rather than to the tumor location (40). In addition, this observation fits well with the compelling neuroimaging evidence of orbitofrontal implication in tasks relying on working memory [for meta-analysis, see Ref. (41, 42)]. PC1-EUD, however, corresponds to a distributed radiation pattern across the whole brain, suggesting that a global increase of radiation dose (EUD) impacts working memory negatively. From its patterns of spatial radiation distribution, this last component could be interpreted as CSI dose variability across subjects. However, such an overall radiation effect does not allow us to distinguish specifically irradiated brain networks that could be particularly involved in working memory impairment.
More specific brain network radiation patterns are found to influence processing speed. The large impact of PC3-EUD is strongly related to radiation to the temporal lobes and the PF. Previous studies have shown significant associations between radiation dose to the temporal lobe and processing speed impairments (16, 17, 43). The cerebellum has also been shown to play a role in processing speed capabilities (44). Importantly, temporal lobe regions are close to the PF upon which the dose was escalated. Thus, PC3-EUD impact could also reflect the radiation field boost trajectory to the PF across subjects. This would support the hypothesis that the volume receiving the highest dose has the greatest impact on cognitive functions. Accordingly, these findings would support current volume–reduction efforts.
Finally, PC2-EUD and PC3-EUD carry the exact same negative effect on IQ change. As reported earlier, PC2-EUD that includes the temporal lobes and the PF showed the most significant impact on processing speed changes. As for processing speed, previous studies have found associations between radiation dose to the temporal lobe and PF and IQ impairments (16, 18). In the same way, the role on IQ impairment of PC3-EUD, which strongly involves the left orbitofrontal cortex, is somehow expected, as many VBM studies in adults and adolescents have shown a link between IQ and gray matter density in this region (45–47). Alternatively, the equal contribution of these two components on ΔFSIQ might be the expression of an averaging effect as FSIQ is a composite index encompassing both working memory and processing speed.
Higher EUD in the superior occipital and parietal regions did not seem to be associated with lower cognitive scores. We may note that Armstrong et al. (17) did not find any significant association between occipito-parietal radiation dose and cognitive or social problems either. In addition, with the same amount of radiation dose, the parietal lobe white matter was shown to be less affected compared to frontal lobe in medulloblastoma (48, 49). Thus, it would be interesting to test whether the parietal lobe is less susceptible to radiation than other regions.
There are limitations in this study, and results should be interpreted with caution. First, the small size and heterogeneity of the patient population make it difficult to control for other variables that could affect the scores (i.e., hydrocephalus shunt, education, rehabilitation, surgical approach, molecular group, etc.). Moreover, considering only PFT patients prevented us from taking into account several potentially confounding variables such as type and localization of the tumor. However, this was a disadvantage regarding the large spatial correlations between close irradiated regions induced by similar radiation protocol. We could not access separately specific regions that are known to play an important role in working memory [e.g., dorsolateral area (50)] or processing speed [e.g., left middle frontal gyrus (51)]. Second, noise could be induced by intersubject variability of the brain morphology, even if we minimized possible segmentation errors by using atlases specific to age groups. Finally, we have to acknowledge that seven patients (that received hyperfractionated radiotherapy, HFRT) had the same total dose and could be considered as a subgroup that could influence the results (see Figure S1 in Supplementary Material). We recognize the possibility that the HFRT subgroup smaller variance might influence the result in other less crucial ways (see Figure S2 in Supplementary Material).
Conclusion
This study confirms two cases for which there is a relationship between the radiation dose in particular brain areas and specific cognitive decline. The first case shows a correlation between orbitofrontal radiation and working memory decline, whereas the second case portrays a correlation between temporal lobe and PF radiation and slower processing speed. As this study is exploratory, it does aim to provide information regarding brain regions to avoid, but to describe relationships between radiation and cognitive function. The relationship between the cognitive profiles and the irradiation of these regions should be further confirmed in a prospective randomized with both, a bigger cohort and different radiation protocols.
Ethics Statement
This retrospective study was approved by the Comite de Protection des Personnes CPP no. 14973 (Ile de France, France). All patient’s parents gave a written informed consent in accordance with the Declaration of Helsinki.
Author Contributions
All authors carried a substantial contribution to the article and approved the final version of the manuscript. None of them has any conflict of interest. Guarantor of integrity of entire study: EDS, MN, and LH-P. Study concepts and design or data collection or data analysis and interpretation: all authors. Data preprocessing: EDS, CR, AG, and CP. Statistical analysis: EDS and MP-G. Drafting the work or revising it critically for important intellectual content: all authors.
Conflict of Interest Statement
The authors declare that the research was conducted in the absence of any commercial or financial relationships that could be construed as a potential conflict of interest.
The reviewer, JB, and the handling editor declared their shared affiliation, and the handling editor states that the process nevertheless met the standards of a fair and objective review.
Acknowledgments
This study was supported by a PhD fellowship from La Ligue Contre le Cancer. The authors gratefully acknowledge Edouard Duchesnay, Andres Hoyos-Idrobo, Pierre Maroun, Mehdi Rahim and Elvis Dohmatob for their advice regarding their respective domain of expertise. We thank Lucy Airs for her help in English. We also thank Sandrine Lopes Da Silva and Imène Hezam for their help in data collection.
Supplementary Material
The Supplementary Material for this article can be found online at http://journal.frontiersin.org/article/10.3389/fonc.2017.00166/full#supplementary-material.
References
1. Danjoux CE, Jenkin RD, McLaughlin J, Grimard L, Gaspar LE, Dar AR, et al. Childhood medulloblastoma in Ontario, 1977–1987: population-based results. Med Pediatr Oncol (1996) 26(1):1–9. doi:10.1002/(SICI)1096-911X(199601)26:1<1::AID-MPO1>3.0.CO;2-Q
2. Hanzlik E, Woodrome SE, Abdel-Baki M, Geller TJ, Elbabaa SK. A systematic review of neuropsychological outcomes following posterior fossa tumor surgery in children. Childs Nerv Syst (2015) 31(10):1869–75. doi:10.1007/s00381-015-2867-3
3. Pollack IF, Jakacki RI. Childhood brain tumors: epidemiology, current management and future directions. Nat Rev Neurol (2011) 7(9):495–506. doi:10.1038/nrneurol.2011.110
4. Mabbott DJ, Penkman L, Witol A, Strother D, Bouffet E. Core neurocognitive functions in children treated for posterior fossa tumors. Neuropsychology (2008) 22(2):159–68. doi:10.1037/0894-4105.22.2.159
5. Palmer SL, Gajjar A, Reddick WE, Glass JO, Kun LE, Wu S, et al. Predicting intellectual outcome among children treated with 35–40 Gy craniospinal irradiation for medulloblastoma. Neuropsychology (2003) 17(4):548–55. doi:10.1037/0894-4105.17.4.548
6. Merchant TE, Schreiber JE, Wu S, Lukose R, Xiong X, Gajjar A. Critical combinations of radiation dose and volume predict intelligence quotient and academic achievement scores after craniospinal irradiation in children with medulloblastoma. Int J Radiat Oncol Biol Phys (2014) 90(3):554–61. doi:10.1016/j.ijrobp.2014.06.058
7. Spiegler BJ, Bouffet E, Greenberg ML, Rutka JT, Mabbott DJ. Change in neurocognitive functioning after treatment with cranial radiation in childhood. J Clin Oncol (2004) 22(4):706–13. doi:10.1200/JCO.2004.05.186
8. Mulhern RK, Palmer SL, Merchant TE, Wallace D, Kocak M, Brouwers P, et al. Neurocognitive consequences of risk-adapted therapy for childhood medulloblastoma. J Clin Oncol (2005) 23(24):5511–9. doi:10.1200/JCO.2005.00.703
9. Ris MD, Packer R, Goldwein J, Jones-Wallace D, Boyett JM. Intellectual outcome after reduced-dose radiation therapy plus adjuvant chemotherapy for medulloblastoma: a Children’s Cancer Group study. J Clin Oncol (2001) 19(15):3470–6. doi:10.1200/JCO.2001.19.15.3470
10. Grill J, Renaux VK, Bulteau C, Viguier D, Levy-Piebois C, Sainte-Rose C, et al. Long-term intellectual outcome in children with posterior fossa tumors according to radiation doses and volumes. Int J Radiat Oncol Biol Phys (1999) 45(1):137–45. doi:10.1016/S0360-3016(99)00177-7
11. Merchant TE, Kiehna EN, Li C, Shukla H, Sengupta S, Xiong X, et al. Modeling radiation dosimetry to predict cognitive outcomes in pediatric patients with CNS embryonal tumors including medulloblastoma. Int J Radiat Oncol Biol Phys (2006) 65(1):210–21. doi:10.1016/j.ijrobp.2005.10.038
12. Carrie C, Muracciole X, Gomez F, Habrand JL, Benhassel M, Mege M, et al. Conformal radiotherapy, reduced boost volume, hyperfractionnated radiotherapy and on-line quality control in standard risk medulloblastoma without chemotherapy, results of the French M-SFOP 98 protocol. Int J Radiat Oncol Biol Phys (2003) 57(2):S195. doi:10.1016/S0360-3016(03)00995-7
13. Lawrence YR, Li XA, el Naqa I, Hahn CA, Marks LB, Merchant TE, et al. Radiation dose-volume effects in the brain. Int J Radiat Oncol Biol Phys (2010) 76(3):S20–7. doi:10.1016/j.ijrobp.2009.02.091
14. Packer RJ, Cogen P, Vezina G, Rorke LB. Medulloblastoma: clinical and biologic aspects. Neuro Oncol (1999) 1(3):232–50. doi:10.1215/15228517-1-3-232
15. Moxon-Emre I, Bouffet E, Taylor MD, Laperriere N, Scantlebury N, Law N, et al. Impact of craniospinal dose, boost volume, and neurologic complications on intellectual outcome in patients with medulloblastoma. J Clin Oncol (2014) 32(17):1760–8. doi:10.1200/JCO.2013.52.3290
16. Jalali R, Mallick I, Dutta D, Goswami S, Gupta T, Munshi A, et al. Factors influencing neurocognitive outcomes in young patients with benign and low-grade brain tumors treated with stereotactic conformal radiotherapy. Int J Radiat Oncol Biol Phys (2010) 77(4):974–9. doi:10.1016/j.ijrobp.2009.06.025
17. Armstrong GT, Jain N, Liu W, Merchant TE, Stovall M, Srivastava DK, et al. Region-specific radiotherapy and neuropsychological outcomes in adult survivors of childhood CNS malignancies. Neuro Oncol (2010) 12(11):1173–86. doi:10.1093/neuonc/noq104
18. Merchant TE, Sharma S, Xiong X, Wu S, Conklin H. Effect of cerebellum radiation dosimetry on cognitive outcomes in children with infratentorial ependymoma. Int J Radiat Oncol Biol Phys (2014) 90(3):547–53. doi:10.1016/j.ijrobp.2014.06.043
19. Gondi V, Pugh SL, Tome WA, Caine C, Corn B, Kanner A, et al. Preservation of memory with conformal avoidance of the hippocampal neural stem-cell compartment during whole-brain radiotherapy for brain metastases (RTOG 0933): a phase II multi-institutional trial. J Clin Oncol (2014) 32(34):3810–6. doi:10.1200/JCO.2014.57.2909
20. Wechsler D. The Wechsler Intelligence Scale for Children. 3rd ed. San Antonio, TX: The Psychological Corp (1991).
21. Wechsler D. Wechsler Adult Intelligence Scale. 3rd ed. San Antonio, TX: The Psychological Corp (1997).
22. Richards JE, Sanchez C, Phillips-Meek M, Xie W. A database of age-appropriate average MRI templates. Neuroimage (2016) 124:1254–9. doi:10.1016/j.neuroimage.2015.04.055
23. Shattuck DW, Mirza M, Adisetiyo V, Hojatkashani C, Salamon G, Narr KL, et al. Construction of a 3D probabilistic atlas of human cortical structures. Neuroimage (2008) 39(3):1064–80. doi:10.1016/j.neuroimage.2007.09.031
24. Gousias IS, Rueckert D, Heckemann RA, Dyet LE, Boardman JP, Edwards AD, et al. Automatic segmentation of brain MRIs of 2-year-olds into 83 regions of interest. Neuroimage (2008) 40(2):672–84. doi:10.1016/j.neuroimage.2007.11.034
25. Sanchez CE, Richards JE, Almli CR. Neurodevelopmental MRI brain templates for children from 2 weeks to 4 years of age. Dev Psychobiol (2012) 54(1):77–91. doi:10.1002/dev.20579
26. Klein A, Andersson J, Ardekani BA, Ashburner J, Avants B, Chiang MC, et al. Evaluation of 14 nonlinear deformation algorithms applied to human brain MRI registration. Neuroimage (2009) 46(3):786–802. doi:10.1016/j.neuroimage.2008.12.037
27. Avants BB, Epstein CL, Grossman M, Gee JC. Symmetric diffeomorphic image registration with cross-correlation: evaluating automated labeling of elderly and neurodegenerative brain. Med Image Anal (2008) 12(1):26–41. doi:10.1016/j.media.2007.06.004
28. Jenkinson M, Smith S. A global optimisation method for robust affine registration of brain images. Med Image Anal (2001) 5(2):143–56. doi:10.1016/S1361-8415(01)00036-6
29. Fowler JF. The linear-quadratic formula and progress in fractionated radiotherapy. Br J Radiol (1989) 62(740):679–94. doi:10.1259/0007-1285-62-740-679
30. Niemierko A. Reporting and analyzing dose distributions: a concept of equivalent uniform dose. Med Phys (1997) 24(1):103. doi:10.1118/1.598063
31. Thames HD. An “incomplete-repair” model for survival after fractionated and continuous irradiations. Int J Radiat Biol Relat Stud Phys Chem Med (1985) 47(3):319–39. doi:10.1080/09553008514550461
32. Emami B, Lyman J, Brown A, Coia L, Goitein M, Munzenrider JE, et al. Tolerance of normal tissue to therapeutic irradiation. Int J Radiat Oncol Biol Phys (1991) 21(1):109–22. doi:10.1016/0360-3016(91)90171-Y
33. Jolliffe I. Principal component analysis. Wiley StatsRef: Statistics Reference Online. John Wiley & Sons, Ltd (2014). doi:10.1002/9781118445112.stat06472
34. Yoo W, Mayberry R, Bae S, Singh K, He QP, Lillard JW Jr. A study of effects of multicollinearity in the multivariable analysis. Int J Appl Sci Technol (2014) 4(5):9.
36. Seabold S, Perktold J. Statsmodels: econometric and statistical modeling with python. Proceedings of the 9th Python in Science Conference. (Vol. 57) (2010). 61 p. Available from: https://conference.scipy.org/proceedings/scipy2010/pdfs/seabold.pdf
37. Abraham A, Pedregosa F, Eickenberg M, Gervais P, Mueller A, Kossaifi J, et al. Machine learning for neuroimaging with scikit-learn. Front Neuroinformatics (2014) 8:14. doi:10.3389/fninf.2014.00014
38. von der Weid N, Mosimann I, Hirt A, Wacker P, Nenadov Beck M, Imbach P, et al. Intellectual outcome in children and adolescents with acute lymphoblastic leukaemia treated with chemotherapy alone: age- and sex-related differences. Eur J Cancer (2003) 39(3):359–65. doi:10.1016/S0959-8049(02)00260-5
39. Kempf-Leonard K, editor. Encyclopedia of Social Measurement. 1st ed. Amsterdam: Elsevier (2004).
40. Mabbott DJ, Monsalves E, Spiegler BJ, Bartels U, Janzen L, Guger S, et al. Longitudinal evaluation of neurocognitive function after treatment for central nervous system germ cell tumors in childhood. Cancer (2011) 117(23):5402–11. doi:10.1002/cncr.26127
41. Wager TD, Smith EE. Neuroimaging studies of working memory. Cogn Affect Behav Neurosci (2003) 3:255–74. doi:10.3758/CABN.3.4.255
42. Owen AM, McMillan KM, Laird AR, Bullmore E. N-back working memory paradigm: a meta-analysis of normative functional neuroimaging studies. Hum Brain Mapp (2005) 25:46–59. doi:10.1002/hbm.20131
43. Redmond KJ, Mahone EM, Terezakis S, Ishaq O, Ford E, McNutt T, et al. Association between radiation dose to neuronal progenitor cell niches and temporal lobes and performance on neuropsychological testing in children: a prospective study. Neuro Oncol (2013) 15(3):360–9. doi:10.1093/neuonc/nos303
44. Eckert MA. Slowing down: age-related neurobiological predictors of processing speed. Front Neurosci (2011) 5:25. doi:10.3389/fnins.2011.00025
45. Shaw P, Greenstein D, Lerch J, Clasen L, Lenroot R, Gogtay N, et al. Intellectual ability and cortical development in children and adolescents. Nature (2006) 440(7084):676–9. doi:10.1038/nature04513
46. Jung RE, Haier RJ. The parieto-frontal integration theory (P-FIT) of intelligence: converging neuroimaging evidence. Behav Brain Sci (2007) 30(02):135. doi:10.1017/S0140525X07001185
47. Narr KL, Woods RP, Thompson PM, Szeszko P, Robinson D, Dimtcheva T, et al. Relationships between IQ and regional cortical gray matter thickness in healthy adults. Cereb Cortex (2007) 17(9):2163–71. doi:10.1093/cercor/bhl125
48. Qiu D, Kwong DL, Chan GC, Leung LH, Khong PL. Diffusion tensor magnetic resonance imaging finding of discrepant fractional anisotropy between the frontal and parietal lobes after whole-brain irradiation in childhood medulloblastoma survivors: reflection of regional white matter radiosensitivity? Int J Radiat Oncol Biol Phys (2007) 69(3):846–51. doi:10.1016/j.ijrobp.2007.04.041
49. Qiu D, Leung LHT, Kwong DLW, Chan GCF, Khong P-L. Mapping radiation dose distribution on the fractional anisotropy map: applications in the assessment of treatment-induced white matter injury. Neuroimage (2006) 31(1):109–15. doi:10.1016/j.neuroimage.2005.12.007
50. Levy R, Goldman-Rakic PS. Segregation of working memory functions within the dorsolateral prefrontal cortex. Exp Brain Res (2000) 133(1):23–32. doi:10.1007/s002210000397
Keywords: pediatric, posterior fossa, radiotherapy, cognitive impairments, radiation effects
Citation: Doger de Speville E, Robert C, Perez-Guevara M, Grigis A, Bolle S, Pinaud C, Dufour C, Beaudré A, Kieffer V, Longaud A, Grill J, Valteau-Couanet D, Deutsch E, Lefkopoulos D, Chiron C, Hertz-Pannier L and Noulhiane M (2017) Relationships between Regional Radiation Doses and Cognitive Decline in Children Treated with Cranio-Spinal Irradiation for Posterior Fossa Tumors. Front. Oncol. 7:166. doi: 10.3389/fonc.2017.00166
Received: 11 May 2017; Accepted: 25 July 2017;
Published: 18 August 2017
Edited by:
Bhadrasain Vikram, National Cancer Institute (NIH), United StatesReviewed by:
John Austin Vargo, West Virginia University Hospitals, United StatesJeff Buchsbaum, National Institutes of Health (NIH), United States
Copyright: © 2017 Doger de Speville, Robert, Perez-Guevara, Grigis, Bolle, Pinaud, Dufour, Beaudré, Kieffer, Longaud, Grill, Valteau-Couanet, Deutsch, Lefkopoulos, Chiron, Hertz-Pannier and Noulhiane. This is an open-access article distributed under the terms of the Creative Commons Attribution License (CC BY). The use, distribution or reproduction in other forums is permitted, provided the original author(s) or licensor are credited and that the original publication in this journal is cited, in accordance with accepted academic practice. No use, distribution or reproduction is permitted which does not comply with these terms.
*Correspondence: Marion Noulhiane, bWFyaW9uLm5vdWxoaWFuZUBwYXJpc2Rlc2NhcnRlcy5mcg==