- 1Louisiana State University Health Sciences Center School of Medicine, Stanley S. Scott Cancer Center, New Orleans, LA, United States
- 2Behavioral and Community Health Sciences Department, Louisiana State University Health Sciences Center School of Public Health, New Orleans, LA, United States
- 3Epidemiology Department, Louisiana State University Health Sciences Center School of Public Health, New Orleans, LA, United States
- 4Department of Genetics, Louisiana State University Health Sciences Center School of Medicine, Stanley S. Scott Cancer Center, New Orleans, LA, United States
- 5Biostatistics Department, Louisiana State University Health Sciences Center School of Public Health, New Orleans, LA, United States
Purpose: Over the past three decades, Hepatocellular Carcinoma (HCC) is one of few cancers for which incidence has increased in the United States (US). It is likely social determinants at the population level are driving this increase. We designed a population-based study to explore whether social determinants at the neighborhood level are geographically associated with HCC incidence in Louisiana by examining the association of HCC incidence with neighborhood concentrated disadvantage.
Methods: Primary HCC cases diagnosed from 2008 to 2012 identified from the Louisiana Tumor Registry were geocoded to census tract of residence at the time of diagnosis. Neighborhood concentrated disadvantage index (CDI) for each census tract was calculated according to the PhenX Toolkit data protocol based on population and socioeconomic measures from the US Census. The incidence of HCC was modeled using multilevel binomial regression with individuals nested within neighborhoods.
Results: The study included 1,418 HCC cases. Incidence of HCC was greater among males than females and among black than white. In multilevel models controlling for age, race, and sex, neighborhood CDI was positively associated with the incidence of HCC. A one standard deviation increase in CDI was associated with a 22% increase in HCC risk [Risk Ratio (RR) = 1.22; 95% CI (1.15, 1.31)]. Adjusting for contextual effects of an individual's neighborhood reduced the disparity in HCC incidence.
Conclusion: Neighborhood concentrated disadvantage, a robust measure of an adverse social environment, was found to be a geographically associated with HCC incidence. Differential exposure to neighborhoods characterized by concentrated disadvantage partially explained the racial disparity in HCC for Louisiana. Our results suggest that increasing rates of HCC, and existing racial disparities for the disease, are partially explained by measures of an adverse social environment.
Introduction
Over the past three decades hepatocellular carcinoma (HCC) is one of the few cancers for which incidence has increased in the United States (US) (1, 2). This is alarming because HCC has been recognized by the US Congress as a recalcitrant cancer of which the 5 year survival is less than 50%1. Consequently, it is projected that by 2030 cancers of the liver and bile duct will be the third leading cause of cancer deaths in the US (3). The increase has been primarily attributed to three risk factors leading to hepatic dysfunction: (1) Hepatitis C virus (HCV) infection; (2) obesity-related metabolic dysfunction leading to Non Alcoholic Fatty Liver Disease (NAFLD); and (3) alcohol-use disorders (AUD). While HCV infection represents the greatest individual risk among the three, what is driving the epidemic at the population level is more complex. Due to the high prevalence of metabolic syndrome (20%) and obesity (35%) in the general population, the population attributable fraction (PAF) for NAFLD is estimated at 32%, followed by HCV infection at 20.5% and AUD at 13.4% (4, 5). Consequently, it may be that the continued increase in HCC will be driven by the rising rates of obesity related metabolic disorders leading to NAFLD (6, 7).
Studies that have modeled and monitored the trend in HCC incidence suggest the peak in HCC will occur soon (8, 9). However, there is concern that these projected trends are primarily based on the risk of HCC associated with HCV infection and therefore too optimistic (6, 10, 11). Over the past decade, the incidence in HCV infection has been decreasing due to a cohort born between 1945 and 1964 that have had high rates of HCV infection, which is not evidenced in the birth cohorts born before 1945 or after 1964 (12). On the other hand, rates of HCC due to NAFLD are increasing dramatically both in the US and internationally due to the decades old obesity pandemic (11, 13, 14). The current study examines the role of a neighborhood risk factor, concentrated disadvantage, associated with both intravenous (IV) drug use risk and obesity risk to determine whether residence in a disadvantaged neighborhood explains both HCC incidence overall and disparities in HCC incidence by race. The study uses data from the Surveillance Epidemiology and End Results (SEER), Louisiana Tumor Registry for 2008–2012. It is hoped that by identifying modifiable factors in neighborhood environments effective intervention can be initiated to target the rising incidence of HCC in Louisiana.
Materials and Methods
HCC Case Ascertainment
This study involved a secondary analysis of data from the Louisiana Tumor Registry (LTR) and US Census. LTR is a member of the of National Cancer Institute's (NCI) Surveillance, Epidemiology and End Results (SEER) Program, as well as a member of the Centers for Disease Control and Prevention's (CDC) National Program of Cancer Registries. Primary cases of HCC diagnosed from January 2008 to December 2012 were identified by International Classification of Diseases for Oncology, Third Edition (ICDO-3) site code C220 and histology codes 8170-8175 and 8180. The Louisiana State University Health Sciences Center-New Orleans Human Research Protection Program and Institutional Review Board reviewed and approved this research project.
Incident cases of HCC among adults 35 years and older were defined as individuals diagnosed with at least one case of primary invasive HCC during the study period. In-situ tumors were not included. Age was categorized into three age groups (35–49, 50–64, and 65 and older) (8). With this age grouping, individuals in the 50–64 age group for this study period (2008–2012) generally fall into the 1945–1965 birth cohort that experienced higher rates of HCV compared to birth cohorts before 1945 and after 1965. Sex was defined as male or female. Race was defined as black or white; we did not include other races in the study due to their small numbers. Thus, the total number of possible individual level demographic risk factor combinations for the study was 12.
Geocoding Cases and Determining Disadvantage
Individual HCC cases were geocoded to 2010 US census tracts using the Automated Geospatial Geocoding Interface Environment system, which was developed through a partnership between the North American Association of Central Cancer Registries (NAACCR), Texas A&M University, and the NCI as a single, uniform geocoding platform for open use by cancer registries2 Cases were geocoded to census tracts by street address at time of diagnosis, with 95% success rate. At-risk population for census tracts was determined by 2010 US Decennial Census data (15, 16).
Neighborhood concentrated disadvantage index (CDI) scores were calculated based on the PhenX Toolkit protocol. The PhenX Toolkit is a product from the collaboration between the Research Triangle Institute and the National Human Genome Research Institute to develop consensus measures for phenotypes and exposures3 CDI is a construct that operationalizes urban theory regarding the overconcentration of blacks, children and female-headed families in economically disadvantaged neighborhoods (17). We derived CDI using American Community Survey (ACS) 2008–2012 Five-year estimates for census tracts4 Tracts were scored through a principle components analysis of 6 measures (given as percentages): (1) individuals below the federal poverty line, (2) households receiving public assistance income, (3) female-headed households, (4) individuals that are unemployed, (5) individuals that are below the age of 18, and (6) individuals that are black. Factor scores for study census tracts follow a standard normal distribution with a mean of zero and standard deviation of 1.
Census Tract Exclusions
According to the US Census, there were 1,148 census tracts in Louisiana in 2010. Standard US census tracts typically have between 2,500 and 8,000 residents and are relatively homogenous with respect to population characteristics, economic status, and living conditions5 We excluded 19 Louisiana census tracts with zero population in 2010 as well as 8 non-standard tracts with a population less than 500 people. Because census tracts were designed to contain relatively homogenous populations, we did not feel it was appropriate to merge the population for these tracts with neighboring tracts. We excluded a single census tract that encompasses Orleans Parish Prison. The prison had a population of 3,059 in 2010. No incident cases of HCC were omitted based on these tract exclusions. After these exclusions, individuals from 1,120 Louisiana census tracts remained eligible for the study. We further restricted the study area to urban parishes (counties), as we have used census tracts to define “neighborhoods” and this unit best represents neighborhoods for urban areas only. Of 64 Louisiana parishes (counties), 47 were classified as urban according to US Office of Management and Budget's definition of a metropolitan statistical area as “one or more adjacent counties or county equivalents that have at least one urban core area of at least 50,000 population, plus adjacent territory that has a high degree of social and economic integration with the core as measured by commuting ties”6 1,038 out of the 1,120 previously identified census tracts were in urban parishes (counties). Urban tracts contained 93% of eligible population and 94% of the HCC cases identified.
Population at Risk Aggregation
Based on the study design, cases of HCC were aggregated for each of the 12 demographic risk combinations in 1,038 study census tracts, which yielded 12,456 possible data points or “cells.” The population at risk for age, sex, and race specific cells within each census tract was determined from 2010 US census population counts, multiplied by 5 to represent person-years at risk. For each data cell, cancer cases and population-years were used to construct a binomial random variable, where the response was given as the number of incident HCC cases over the person-years at. A total of 196 data cells had no at risk population (person-years) and did not contribute to the analysis. There was a single incident case of HCC recorded for these cells but due to a lack of population at risk, the case was not included in the analysis.
Statistical Analysis
Multiple multilevel generalized linear regression models were used to model the incidence of HCC in Louisiana. The multilevel data structure consisted of individuals (level 1) nested within census tracts (level 2). The number of incident HCC cases over the population years at risk constituted a binomial random response. Models used a log link to estimate adjusted risk ratios for the study population. Correlation among individuals within the same tract was modeled with the use of a random intercept for each tract. All statistical analyses were performed with SAS version 9.4 (SAS Institute, Cary, NC). Multilevel generalized linear models were executed with the Glimmix Procedure, using maximum likelihood estimation based on adaptive quadrature rule. Model fit was assessed through the Pearson Chi-Square goodness of fit statistic (18).
An initial model was used to estimate individual demographic trends in HCC incidence and assess if there was clustering of cases at the neighborhood level. This model contained fixed effects for individual level demographic variables, age, sex and race. We also included effect modifiers (interactions) for the 50–64 age group to account for distinct patterns of HCC incidence in the HCV cohort. Model 1 was used to determine neighborhood variation in HCC incidence after accounting for the composition of individuals within census tracts. A second model included CDI in order to evaluate whether neighborhood disadvantage explained census tract variation in incidence and if CDI contributed to existing racial disparities. Model 3 included an effect modifier (interaction) for the effects of CDI in the 50–64 age group. A p-value smaller than 0.05 for two sided statistical tests was considered statistically significant.
Results
Study population characteristics are presented as Table 1. The study included 2,057,053 Louisiana residents, of which 53% were female and 71% were white. There was a notable difference in neighborhood disadvantage for the population by race, with black residents disproportionately represented in more disadvantaged areas. The disparity in neighborhood living environment was evidenced by a greater mean CDI score for blacks [mean = 0.54, standard deviation (SD) = 9.60] compared to whites (mean = −0.49, SD = 9.33).
We identified 1,418 incident cases of HCC in the study population that met the study criteria (Figure 1). Trends in HCC incidence by age group showed that HCC risk increased with advancing age, with the exception of a peak observed for ages 50–69, particularly among black males (Figure 2). Although we are only considering HCC, these trends are consistent with national trends found for liver and bile duct cancer during this time (2).
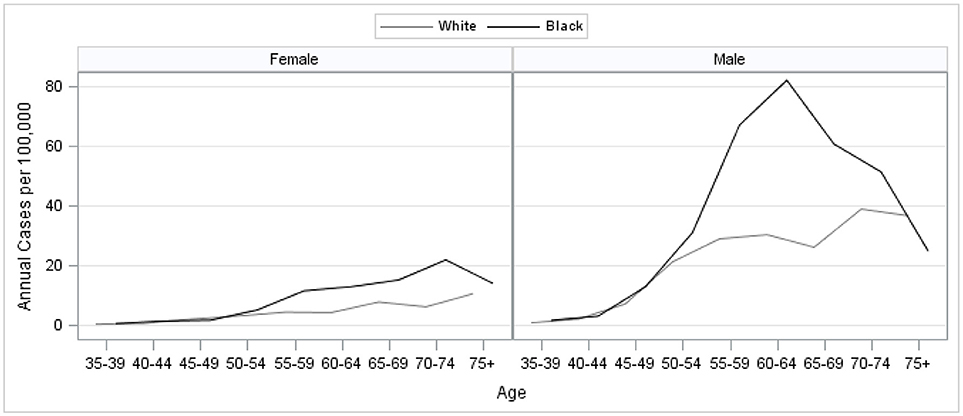
Figure 2. Age, sex, and race-specific HCC incidence, urban parishes (counties) of Louisiana 2008–2012.
Multivariable multilevel model parameter estimates are provided as Table 2. There was significant neighborhood variation in HCC incidence in the first model, which controlled for demographic composition (p = 0.0158). Neighborhood variation in HCC incidence was reduced after adjusting for the effects of neighborhood CDI (Models 2 and 3). Estimated relative risk (RR) and corresponding 95% confidence intervals (CI) are provided as Table 3. Results from Model 1 indicated that men were 3.72 times as likely to develop the disease compared to women (RR = 3.72; 95% CI: 3.13–4.43 and that risk was 1.53 times greater in black residents compared to whites (RR = 1.53; 95% CI: 1.29–1.80). Relative risk for the 50–64 age group, which contains the US HCV birth cohort, differed significantly from other age groups; men in this cohort had 6.50 times the risk of women (RR = 6.50; 95% CI: 5.31–7.96) and black residents had 2.18 times the risk of white residents (RR = 2.18; 95% CI: 1.89–2.52). Neighborhood CDI was significantly associated with HCC, with increased risk exhibited in more disadvantaged areas (Model 2). CDI is a sample-based index, where scores from a sample have a mean of 0 and a standard deviation of 1. Therefore, a single unit increase in CDI represents a one standard deviation increase in neighborhood disadvantage. Results from the second model indicate a single unit increase in CDI was associated with 22% relative increase in HCC risk (RR = 1.22, 95% CI: 1.15–1.31). Further, there was a significant interaction between CDI and age, where the effects of CDI were greater among the 50–64 age group (Model 3). In our final model, the adjusted risk ratio for a single unit increase in CDI was estimated to be 1.31 (RR = 1.31; 95% CI: 1.20–1.43) for the 50–64 age group while it was 1.12 (RR = 1.12; 95% CI: 1.01–1.23) among other age groups. Controlling for differential effects of neighborhood CDI on HCC incidence reduced the estimated relative risk for blacks to 1.37 (95% CI: 1.13–1.67). In the 50–64 age group, the estimated relative risk for blacks was reduced to 1.64 (95% CI: 1.37–1.95). The excess racial disparity for the 50–64 age group was no longer statistically significant from the other ages, as indicated by the overlap in the confidence intervals for the two estimates in this model. Estimated trends in HCC incidence from the final model are presented in Figure 3, where we observe the most profound effects of CDI in black males aged 50–64.
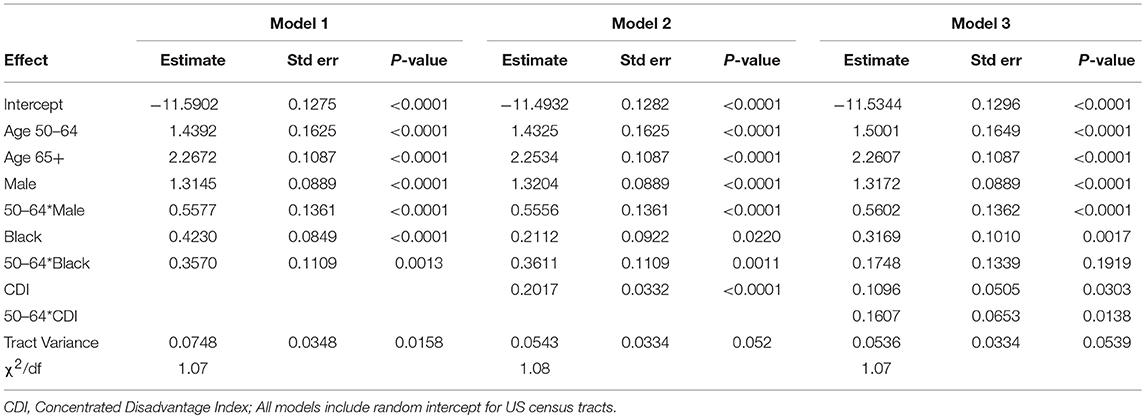
Table 2. Parameter estimates from multilevel binomial regression models of Hepatocellular Carcinoma incidence in urban parishes (counties) of Louisiana, 2008–2012.
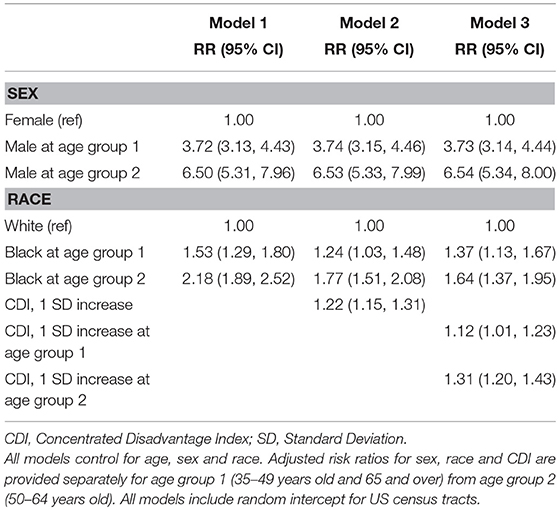
Table 3. Adjusted risk ratios (RR) and 95% confidence intervals (CI) from multilevel binomial regression models of Hepatocellular Carcinoma incidence in urban parishes (counties) of Louisiana, 2008–2012.
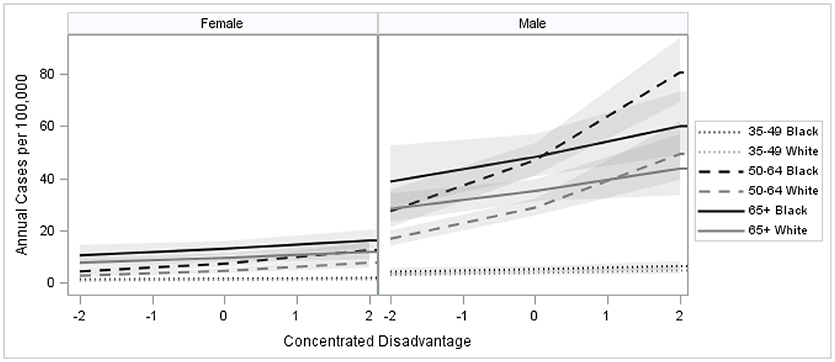
Figure 3. Age, sex, and race-specific predicted HCC risk by neighborhood concentrated disadvantage index, urban parishes (counties) of Louisiana 2008–2012.
Discussion
The current study assessed individual demographic and neighborhood level risk in HCC incidence. We found that neighborhood concentrated disadvantage (CDI), a robust measure of an adverse social and physical environment, was strongly associated with increased HCC risk, with 22% relative increase in risk associated with a single standard deviation increase in disadvantage. These results parallel those from an investigation using data from the NIH-AARP study, which found an association between area socioeconomic deprivation and increased risk of HCC incidence (19). We found that disparities in HCC by race and sex were significantly greater in the 50-64 age group. This age group includes the majority of a 1945–1965 birth cohort with elevated rates of HCV. Thus, disparities measured through population age-adjusted HCC incidence rates have been apparently influenced by HCV risk in this birth cohort. For our study population, there was differential exposure to neighborhood concentrated disadvantage by race, and we found that adjusting for the contextual effects of CDI reduced the observed racial disparities in HCC for all age groups. Additionally, our results show the effects of CDI on HCC were more pronounced in the 50-64 age group that correlates with the US HCV cohort compared to other ages (RR = 1.31 vs. 1.12), which is consistent with the established role of HCV infections as a key mechanism in HCC risk.
Given the observed geographic association between neighborhood disadvantage and HCC incidence, which apparently accounted for some of the racial disparity in HCC incidence, it is worth discussing how neighborhood disadvantage relates to each of the major risk factors for HCC. Not surprisingly, there is literature linking each of these risk factors to neighborhood disadvantage.
IV drug use has been the main driver of HCV in the US (20, 21). Disadvantaged neighborhoods have long been linked to IV drug use. Galea and colleagues indicate neighborhood disadvantage is a central component in their framework of contextual determinants of IV drug use (22). Neighborhood disadvantage has been linked to younger age of initiation, greater injection frequency, cessation failure, and unsafe syringe use among IV drug users (23–27). While we found the effect of neighborhood disadvantage was greater in the 50–64 year old age group, corresponding to the birth cohort at greatest risk of IV drug use and therefore HCV risk, it should be noted that CDI was still strongly associated with HCC risk in other age groups. This is consistent with the epidemiology that indicates obesity related NAFLD has the largest population attributable fraction for HCC.
It is widely conceded that local obesogenic environments are responsible for the obesity epidemic (28). Given the dramatic increase in obesity rates over the past decades, it is the role of these local obesogenic environments that is most concerning with regard to increasing HCC incidence. Disadvantaged populations are widely acknowledged to live in more obesogenic environments. In fact, there are a number of reviews suggesting that the disparities in obesity related outcomes like NAFLD are due to these neighborhood effects that disproportionately affect poor and minority populations (29–36). It is interesting to note that although neighborhood environments are implicated, the specific physical or social conditions responsible for the increased obesity risk has yet to be agreed upon (37, 38). Consequently, attempts to link specific elements of neighborhood obesogenic environments to HCC incidence beyond neighborhood disadvantage would represent a significant contribution to this literature.
Finally, alcohol abuse also tends to be associated with disadvantaged neighborhoods. Overconcentration of alcohol outlets at the neighborhood level historically has been linked to alcohol abuse (39–41). In addition, alcohol outlets tend to be disproportionately located in poor and minority neighborhoods despite the fact that higher SES and white race is associated with higher levels of alcohol use (42). However, the fact that levels of alcohol use have been stable over the period does not suggest overconcentration of alcohol outlets at the neighborhood level are driving the increase in HCC incidence.
It is vital to determine the underlying mechanisms of HCC risk. Successful antiviral treatment has been shown to reduce the progression of liver disease and the development of HCC in HCV patients at all stages of liver disease (43, 44). The effort to address the high rate of HCV is directed at the 1945–1965 birth cohort (45). As this birth cohort ages and antiviral treatment rates improve, obesity related NAFLD will take over as the predominant risk factor for HCC (11). Although several studies have reported significant protective effects of certain medications used to manage chronic metabolic conditions associated with NAFLD (i.e., metformin for diabetes, statins, aspirin) (11, 46), research is needed to identify the neighborhood factors driving the obesity epidemic in order to develop effective preventive strategies targeting disadvantaged neighborhood.
From a physiologic perspective, the link between obesity related NAFLD and HCC is extremely relevant in terms of identifying the neighborhood level risk factor. Epidemiological data suggest the association of about 15% of human cancers with chronic infection and inflammation (47). Inflammation plays a key role in the pathogenesis of chronic liver injury and is considered to be a risk factor for HCC (48). About 90% of incident HCC develops due to chronic liver inflammation, the induction of fibrosis and subsequent cirrhosis (49). Inflammation is involved in cell degeneration, fibrosis, cirrhosis and tumor formation, which are essential stages of HCC development. During HCC initiation, events such as mutations, deletions or overexpression of genes provide mutant cells with a growth and survival advantage (50). However, recent studies demonstrate that these initial genetic alterations or epigenetic changes are not sufficient for a complete neoplastic progression, suggesting that HCC initiation and progression might depend on consistently supportive signals that are provided from an inflammatory microenvironment thus facilitating each step of carcinogenesis (51, 52).
Limitations of this investigation stem from to the cross-sectional nature of the study design. The duration of exposure or the risk associated with neighborhood environment over time is unable to be established, as is temporality between exposure and incident HCC. CDI was measured at time of HCC diagnosis, yet etiologic exposure occurs much earlier in the natural history of this cancer. The study lacks data on individual level risk factors such as HCV infection, NAFLD, and alcohol use that are needed to determine the distribution of underlying clinical risks of this disease. An additional limitation is that we have assessed the effects of neighborhood living environment based on a census-defined spatial unit (tract), which is designed to be relatively homogenous in terms of social characteristics but does lack a subjective definition of “neighborhood.”
The mobility of residents who live within these neighborhoods included in the present study also poses another limitation. As an investigation regarding cancer incidence, it is understood the environmental influence upon the development of HCC began many years before diagnosis. Therefore, the possibility exists that study participants resided within a neighborhood with more disadvantage and then moved into a less disadvantaged neighborhood recorded upon HCC diagnosis. With this, literature describing residential mobility and health have found there is a lack of effect on cross-sectional studies (53). Movers select for neighborhoods of similar health, especially among those with poorer health (54, 55).
The generalizability of this study is also limited when reviewing the racial makeup of the study population. It has been shown Hispanics are experiencing the fastest increase in HCC incidence, with a 35.8% increase between 2003 and 2011 (56). However, only blacks and whites were included in our analyses due to sample size; Louisiana has a very small Hispanic population, with Hispanic individuals encompassing only 3.9% of Louisiana's population of 4.4 million in 20107 In a study utilizing Texas Cancer Registry data, rates of HCC in Hispanics were estimated to be 3–4 times that of non-Hispanic whites (57). While incidence rates of HCC differ by ethnicity, trends in population attributable fraction of incident HCC associated with NAFLD in the Hispanic population are similar to those in non-Hispanic whites (39.3 and 34.8%, respectively). Conversely, blacks show a greater population attributable fraction of HCC associated with HCV (36.1%), with only 14.4% of incident HCC with an etiologic cause of NAFLD (4). The population attributable fraction of incident HCC varies widely by race, and the influence of neighborhood on this variation should be further investigated in the future.
Through employing multilevel analyses this study has identified a statistically significant contribution of neighborhood concentrated disadvantage to racial disparities in HCC in Louisiana. Our results suggest that increasing rates of HCC, and existing racial disparities in the disease, are partially driven by social contexts of adverse living conditions. Our group is continuing to investigate quantifying additional physical and social environment variables to include in subsequent analysis of the neighborhood environment to risk of HCC. Future studies in this area should also investigate whether associations between neighborhood environment and incident HCC are mediated by clinical manifestations of the primary risk factors for HCC.
Author Contributions
DD, CL contributed to the study concept, design, methods, and manuscript development. AG made contributions of intellectual content and manuscript development. SS, RS made contributions of intellectual content. NS, TF revised the article for intellectual content. QY, X-CW contributed to documentation of data and methods and have revised the article for intellectual content. RS made substantial contributions to the study concept, design and intellectual content. All authors have approved the content and agree to be accountable for the work with regard to accuracy and integrity.
Funding
This research was supported by research grants from NIH 5R24MD008121-03, NIMHD U54MD008176, and NIMHD 1R15MD012387.
Conflict of Interest Statement
The authors declare that the research was conducted in the absence of any commercial or financial relationships that could be construed as a potential conflict of interest.
Acknowledgments
This research was supported by the Louisiana State University Health Sciences Center, New Orleans School of Public Health.
Footnotes
1. ^https://www.congress.gov/bill/112th-congress/house-bill/733.
2. ^NAACCR Geocoder https://www.naaccr.org/gis-resources/.
3. ^PhenX Toolkit – Neighborhood Concentrated Disadvantage Index https://www.phenxtoolkit.org/index.php?pageLink=browse.protocoldetails&id=211302.
4. ^American FactFinder https://factfinder.census.gov/faces/nav/jsf/pages/index.xhtml.
5. ^US Census Geographic Areas Reference Manual https://www.census.gov/geo/reference/garm.html.
6. ^2010 Office of Management and Budget (OMB) Standards https://www.census.gov/programs-surveys/metro-micro/about/omb-standards.html.
7. ^2010 US Census https://www.census.gov/2010census/.
References
1. El-Serag HB, Mason AC. Rising incidence of hepatocellular carcinoma in the United States. N Engl J Med. (1999) 340:745–50. doi: 10.1056/NEJM199903113401001
2. Ryerson AB, Eheman CR, Altekruse SF, Ward JW, Jemal A, Sherman RL, et al. Annual Report to the Nation on the Status of Cancer 1975–2012, featuring the increasing incidence of liver cancer. Cancer (2016) 122:1312–37. doi: 10.1002/cncr.29936
3. Rahib L, Smith BD, Aizenberg R, Rosenzweig AB, Fleshman JM, Matrisian LM. Projecting cancer incidence and deaths to 2030: the unexpected burden of thyroid, liver, and pancreas cancers in the United States. Cancer Res. (2014) 74:2913–21. doi: 10.1158/0008-5472.CAN-14-0155
4. Makarova-Rusher OV, Altekruse SF, McNeel TS, Ulahannan S, Duffy AG, Graubard BI, et al. Population attributable fractions of risk factors for hepatocellular carcinoma in the United States. Cancer (2016) 122:1757–65. doi: 10.1002/cncr.29971
5. Welzel TM, Graubard BI, Quraishi S, Zeuzem S, Davila JA, El-Serag HB, et al. Population-attributable fractions of risk factors for hepatocellular carcinoma in the United States. Am J Gastroenterol. (2013) 108:1314–21. doi: 10.1038/ajg.2013.160
6. Beste LA, Leipertz SL, Green PK, Dominitz JA, Ross D, Ioannou GN. Trends in burden of cirrhosis and hepatocellular carcinoma by underlying liver disease in US veterans, 2001-2013. Gastroenterology (2015) 149:1471–82.e5, quiz e17–18. doi: 10.1053/j.gastro.2015.07.056
7. Davis GL, Alter MJ, El-Serag H, Poynard T, Jennings LW. Aging of hepatitis C virus (HCV)-infected persons in the United States: a multiple cohort model of HCV prevalence and disease progression. Gastroenterology (2010) 138:513–21, 521.e1–6. doi: 10.1053/j.gastro.2009.09.067
8. Altekruse SF, Henley SJ, Cucinelli JE, McGlynn KA. Changing hepatocellular carcinoma incidence and liver cancer mortality rates in the United States. Am J Gastroenterol. (2014) 109:542–53. doi: 10.1038/ajg.2014.11
9. Njei B, Rotman Y, Ditah I, Lim JK. Emerging trends in hepatocellular carcinoma incidence and mortality. Hepatology (2015) 61:191–9. doi: 10.1002/hep.27388
10. Baffy G, Brunt EM, Caldwell SH. Hepatocellular carcinoma in non-alcoholic fatty liver disease: an emerging menace. J Hepatol. (2012) 56:1384–91. doi: 10.1016/j.jhep.2011.10.027
11. El-Serag HB, Kanwal F. Epidemiology of hepatocellular carcinoma in the United States: where are we? Where do we go? Hepatology (2014) 60:1767–75. doi: 10.1002/hep.27222
12. Armstrong GL, Wasley A, Simard EP, McQuillan GM, Kuhnert WL, Alter MJ. The prevalence of hepatitis C virus infection in the United States, 1999 through 2002. Ann Intern Med. (2006) 144:705–14. doi: 10.7326/0003-4819-144-10-200605160-00004
13. Bellentani S, Baroni GS, Piscaglia F, Tiribelli C. Natural history of nonalcoholic steatohepatitis–associated hepatocellular carcinoma. Clin Liver Dis. (2016) 8:105–7. doi: 10.1002/cld.582
14. Piscaglia F, Svegliati-Baroni G, Barchetti A, Pecorelli A, Marinelli S, Tiribelli C, et al. Clinical patterns of hepatocellular carcinoma in nonalcoholic fatty liver disease: a multicenter prospective study. Hepatology (2016) 63:827–38. doi: 10.1002/hep.28368
15. Scribner RA, Theall KP, Simonsen NR, Mason KE, Yu Q. Misspecification of the effect of race in fixed effects models of health inequalities. Soc. Sci. Med. (2009) 69:1584–91. doi: 10.1016/j.socscimed.2009.08.010
16. Subramanian SV, Chen JT, Rehkopf DH, Waterman PD, Krieger N. Racial disparities in context: a multilevel analysis of neighborhood variations in poverty and excess mortality among black populations in Massachusetts. Am J Public Health (2005) 95:260–5. doi: 10.2105/AJPH.2003.034132
17. Sampson RJ, Raudenbush SW, Earls F. Neighborhoods and violent crime: a multilevel study of collective efficacy. Science (1997) 277:918–24. doi: 10.1126/science.277.5328.918
18. Blizzard L, Hosmer DW. Parameter estimation and goodness-of-fit in log binomial regression. Biom J. (2006) 48:5–22. doi: 10.1002/bimj.200410165
19. Major JM, Sargent JD, Graubard BI, Carlos HA, Hollenbeck AR, Altekruse SF, et al. Local geographic variation in chronic liver disease and hepatocellular carcinoma: contributions of socioeconomic deprivation, alcohol retail outlets, and lifestyle. Ann Epidemiol. (2014) 24:104–10. doi: 10.1016/j.annepidem.2013.11.006
20. Dhanasekaran R, Limaye A, Cabrera R. Hepatocellular carcinoma: current trends in worldwide epidemiology, risk factors, diagnosis, and therapeutics. Hepatic Med Evid Res. (2012) 4:19–37. doi: 10.2147/HMER.S16316
21. Wang CC, Krantz E, Klarquist J, Krows M, McBride L, Scott EP, et al. Acute hepatitis C in a contemporary US cohort: modes of acquisition and factors influencing viral clearance. J Infect Dis. (2007) 196:1474–82. doi: 10.1086/522608
22. Galea S, Ahern J, Vlahov D. Contextual determinants of drug use risk behavior: a theoretic framework. J Urban Health (2003). 80:iii50–8. doi: 10.1093/jurban/jtg082
23. Latkin CA, Williams CT, Wang J, Curry AD. Neighborhood social disorder as a determinant of drug injection behaviors: a structural equation modeling approach. Health Psychol Off J Div Health Psychol Am Psychol Assoc. (2005) 24:96–100. doi: 10.1037/0278-6133.24.1.96
24. Nandi A, Glass TA, Cole SR, Chu H, Galea S, Celentano DD, et al. Neighborhood poverty and injection cessation in a sample of injection drug users. Am J Epidemiol. (2010) 171:391–8. doi: 10.1093/aje/kwp416
25. Fuller CM, Ompad DC, Galea S, Wu Y, Koblin B, Vlahov D. Hepatitis C incidence-a comparison between injection and noninjection drug users in New York City. J Urban Health (2004). 81:20–24. doi: 10.1093/jurban/jth084
26. Schroeder JR, Latkin CA, Hoover DR, Curry AD, Knowlton AR, Celentano DD. Illicit drug use in one's social network and in one's neighborhood predicts individual heroin and cocaine use. Ann Epidemiol. (2001) 11:389–94. doi: 10.1016/S1047-2797(01)00225-3
27. Boardman D, Finch BK, Ellison CG, Williams DR, Jackson JS, Boardman JD, et al. Neighborhood disadvantage, stress, and drug use among adults. J Health Soc Behav. (2001) 42:151–65.
28. Swinburn BA, Sacks G, Hall KD, McPherson K, Finegood DT, Moodie ML, et al. The global obesity pandemic: shaped by global drivers and local environments. Lancet Lond Engl. (2011) 378:804–14. doi: 10.1016/S0140-6736(11)60813-1
29. Black JL, Macinko J. Neighborhoods and obesity. Nutr Rev. (2008) 66:2–20. doi: 10.1111/j.1753-4887.2007.00001.x
30. Ding D, Gebel K. Built environment, physical activity, and obesity: what have we learned from reviewing the literature? Health Place (2012) 18:100–5. doi: 10.1016/j.healthplace.2011.08.021
31. Feng J, Glass TA, Curriero FC, Stewart WF, Schwartz BS. The built environment and obesity: a systematic review of the epidemiologic evidence. Health Place (2010) 16:175–90. doi: 10.1016/j.healthplace.2009.09.008
32. Giskes K, van Lenthe F, Avendano-Pabon M, Brug J. A systematic review of environmental factors and obesogenic dietary intakes among adults: are we getting closer to understanding obesogenic environments? Obes Rev. (2011) 12:e95–106. doi: 10.1111/j.1467-789X.2010.00769.x
33. Lovasi GS, Hutson MA, Guerra M, Neckerman KM. Built Environments and obesity in disadvantaged populations. Epidemiol Rev. (2009) 31:7–20. doi: 10.1093/epirev/mxp005
34. Papas MA, Alberg AJ, Ewing R, Helzlsouer KJ, Gary TL, Klassen AC. The built environment and obesity. Epidemiol. Rev. (2007) 29:129–43. doi: 10.1093/epirev/mxm009
35. Ludwig J, Sanbonmatsu L, Gennetian L, Adam E, Duncan GJ, Katz LF, et al. Neighborhoods, obesity, and diabetes-a randomized social experiment. N Engl J Med. (2011) 365:1509–19. doi: 10.1056/NEJMsa1103216
36. Holstein BE, Currie C, Boyce W, Damsgaard MT, Gobina I, Kökönyei G, et al. Socio-economic inequality in multiple health complaints among adolescents: international comparative study in 37 countries. Int J Public Health (2009) 54(Suppl. 2):260–70. doi: 10.1007/s00038-009-5418-4
37. Cobb LK, Appel LJ, Franco M, Jones-Smith JC, Nur A, Anderson CA. The relationship of the local food environment with obesity: a systematic review of methods, study quality and results. Obes Silver Spring Md. (2015) 23:1331–44. doi: 10.1002/oby.21118
38. Mayne SL, Auchincloss AH, Michael YL. Impact of policy and built environment changes on obesity-related outcomes: a systematic review of naturally-occurring experiments. Obes Rev. (2015) 16:362–75. doi: 10.1111/obr.12269
39. Scribner R. Commentary on Halonen et al. (2013): exposure to alcohol outlets and alcohol consumption-back to square one? Addiction (2013) 108:329–30. doi: 10.1111/add.12038
40. Theall KP, Scribner R, Cohen D, Bluthenthal RN, Schonlau M, Lynch S, et al. The neighborhood alcohol environment and alcohol-related morbidity. Alcohol Alcohol. (2009) 44:491–9. doi: 10.1093/alcalc/agp042
41. Halonen JI, Kivimäki M, Virtanen M, Pentti J, Subramanian SV, Kawachi I, et al. Proximity of off-premise alcohol outlets and heavy alcohol consumption: a cohort study. Drug Alcohol Depend. (2013) 132:295–300. doi: 10.1016/j.drugalcdep.2013.02.022
42. LaVeist TA, Wallace JM. Health risk and inequitable distribution of liquor stores in African American neighborhood. Soc Sci Med. (2000) 51:613–7. doi: 10.1016/S0277-9536(00)00004-6
43. Morgan RL, Baack B, Smith BD, Yartel A, Pitasi M, Falck-Ytter Y. Eradication of hepatitis C virus infection and the development of hepatocellular carcinoma: a meta-analysis of observational studies. Ann Intern Med. (2013) 158:329–37. doi: 10.7326/0003-4819-158-5-201303050-00005
44. Volk ML, Tocco R, Saini S, Lok ASF. Public health impact of antiviral therapy for hepatitis C in the United States. Hepatology (2009) 50:1750–5. doi: 10.1002/hep.23220
45. Smith BD, Morgan RL, Beckett GA, Falck-Ytter Y, Holtzman D, Ward JW. Hepatitis C virus testing of persons born during 1945–1965: recommendations from the centers for disease control and prevention. Ann Intern Med. (2012) 157:817–22. doi: 10.7326/0003-4819-157-9-201211060-00529
46. Petrick JL, Sahasrabuddhe VV, Chan AT, Alavanja MC, Beane-Freeman LE, Buring JE, et al. NSAID use and risk of hepatocellular carcinoma and intrahepatic cholangiocarcinoma: the liver cancer pooling project. Cancer Prev Res. (2015) 8:1156–62. doi: 10.1158/1940-6207.CAPR-15-0126
48. Agosti P, Sabbà C, Mazzocca A. Emerging metabolic risk factors in hepatocellular carcinoma and their influence on the liver microenvironment. Biochim Biophys Acta (2018) 1864:607–17. doi: 10.1016/j.bbadis.2017.11.026
49. Llovet JM, Zucman-Rossi J, Pikarsky E, Sangro B, Schwartz M, Sherman M, et al. Hepatocellular carcinoma. Nat Rev Dis Primer (2016) 2:16018. doi: 10.1038/nrdp.2016.18
50. Suhail M, Sohrab SS, Qureshi A, Tarique M, Abdel-Hafiz H, Al-Ghamdi K, et al. Association of HCV mutated proteins and host SNPs in the development of hepatocellular carcinoma. Infect Genet Evol J Mol Epidemiol Evol Genet Infect Dis. (2018) 60:160–72. doi: 10.1016/j.meegid.2018.02.034
51. Baumert TF, Jühling F, Ono A, Hoshida Y. Hepatitis C-related hepatocellular carcinoma in the era of new generation antivirals. BMC Med. (2017) 15:52. doi: 10.1186/s12916-017-0815-7
52. Ghouri YA, Mian I, Rowe JH. Review of hepatocellular carcinoma: epidemiology, etiology, and carcinogenesis. J Carcinog. (2017) 16:1. doi: 10.4103/jcar.JCar_9_16
53. Geronimus AT, Bound J, Ro A. Residential mobility across local areas in the united states and the geographic distribution of the healthy population. Demography (2014) 51:777–809. doi: 10.1007/s13524-014-0299-4
54. Green MA, Subramanian SV, Vickers D, Dorling D. Internal migration, area effects and health: does where you move to impact upon your health? Soc Sci Med. (2015) 136–137:27–34. doi: 10.1016/j.socscimed.2015.05.011
55. Smith KR, Hanson HA, Brown BB, Zick CD, Kowaleski-Jones L, Fan JX. Movers and Stayers: how residential selection contributes to the association between female body mass index and neighbourhood characteristics. Int J Obes. (2016) 40:1384–91. doi: 10.1038/ijo.2016.78
56. Ha J, Yan M, Wong RJ. Racial/ethnic disparities in hepatocellular carcinoma epidemiology. Curr Hepatol Rep. (2017) 16:39–45. doi: 10.1007/s11901-017-0331-4
Keywords: hepatocellular carcinoma, social determinants, neighborhood environment, multilevel analysis, neighborhood concentrated disadvantage
Citation: Danos D, Leonardi C, Gilliland A, Shankar S, Srivastava RK, Simonsen N, Ferguson T, Yu Q, Wu X-C and Scribner R (2018) Increased Risk of Hepatocellular Carcinoma Associated With Neighborhood Concentrated Disadvantage. Front. Oncol. 8:375. doi: 10.3389/fonc.2018.00375
Received: 26 April 2018; Accepted: 21 August 2018;
Published: 11 September 2018.
Edited by:
Patricia Matthews-Juarez, Meharry Medical College, United StatesReviewed by:
Jerry Polesel, Centro di Riferimento Oncologico di Aviano (IRCCS), ItalyJohn F. Gibbs, Seton Hall University, United States
Copyright © 2018 Danos, Leonardi, Gilliland, Shankar, Srivastava, Simonsen, Ferguson, Yu, Wu and Scribner. This is an open-access article distributed under the terms of the Creative Commons Attribution License (CC BY). The use, distribution or reproduction in other forums is permitted, provided the original author(s) and the copyright owner(s) are credited and that the original publication in this journal is cited, in accordance with accepted academic practice. No use, distribution or reproduction is permitted which does not comply with these terms.
*Correspondence: Claudia Leonardi, Y2xlb24xQGxzdWhzYy5lZHU=
Richard Scribner, cnNjcmliQGxzdWhzYy5lZHU=