- Biology Department, University of New Brunswick, Fredericton, NB, Canada
The main challenges in developing effective anti-cancer therapies stem from the highly complex and heterogeneous nature of cancer, including the presence of multiple genetically-encoded and environmentally-induced cancer cell phenotypes within an individual. This diversity can make the development of successful treatments difficult as different phenotypes can have different responses to the same treatment. The lack of model-systems that can be used to simultaneously test the effect of therapies on multiple distinct phenotypic states further contributes to this problem. To mitigate these challenges, we suggest that in vitro model-systems that consist of several genetically-related but phenotypically distinct populations can be used as proxies for the several phenotypes (including adherent and circulating tumor cells) present in a patient with advanced disease. As proof of concept, we have developed such a model and showed that different phenotypes had different responses to the same challenge (i.e., a change in extracellular pH) both in terms of sensitivity and phenotypic plasticity. We suggest that similar model-systems could be developed and used when designing novel therapeutic strategies, to address the potential impact of phenotypic heterogeneity and plasticity of cancer on the development of successful therapies. Specifically, the effect of a therapy should be considered on more than one cancer cell phenotype (to increase its effectiveness), and both cell viability as well as changes in phenotypic state (to address potential plastic responses) should be evaluated. Although we are aware of the limitations of in vitro systems, we believe that the use of established cell lines that express multiple phenotypes can provide invaluable insights into the complex interplay between therapies and cancer's heterogeneous and plastic nature.
Introduction
Cancer is a complex disease that manifests itself in a multitude of ways, partly due to its diverse genetic and phenotypic nature—both among and within individuals. This diversity can also pose significant challenges to cancer therapies (1–5). For instance, high levels of genetic heterogeneity are known to be associated with more aggressive cancers and resistance to drugs, and distinct phenotypes have been shown to have different responses to therapies and even cooperate to increase their survival or to drive invasion (2, 5–7). Given its genetic, phenotypic, and behavioral complexity, evolutionary and ecological theories are now being applied to understand the processes that affect cancer initiation, progression and dissemination (1, 8–10). Specifically, cancer is seen as part of a complex ecosystem (the body) in which the survival and reproduction of the distinct cancer cell phenotypes can be affected by their local environments (e.g., location in the tumor), changes in the conditions of their specific habitats (tissues), or new selective pressures (such as therapies) (11, 12).
The Problem
The dynamic interactions between the diverse and continuously evolving genotypes and their fluctuating environments can add difficulty to developing successful therapies. Increased levels of genetic diversity can allow selection for clones with phenotypes that confer adaptive advantage in specific settings, including the presence of various drugs. This unescapable evolutionary aspect of cancer hinders the development of successful therapies as drug resistance is always expected to evolve (13–15). Consequently, an increased body of research has been devoted to understanding the degree of genetic heterogeneity in tumors and predicting patterns of mutation and selection in response to therapies (7, 16). However, specific microenvironmental signals can also alter the phenotype directly by affecting gene expression patterns and inducing alternative phenotypes that are better fit to the new challenges. Phenotypic plasticity is most relevant during metastasis and dissemination stages, when cells undergo transitions from epithelial to mesenchymal phenotypes (and back), and express distinct cell behaviors [e.g., adherent or invasive cells, circulating tumor cells (CTC), or disseminated cells] specific to different progression stages (localized or advanced disease) and cell fates (epithelial, mesenchymal, cancer stem cells) (17, 18). Such cell plasticity has a strong impact on therapies as phenotypic changes and the presence of multiple phenotypes can drastically reduce the effectiveness of treatments (19–21). For instance, shifts in the proportion of epithelial and mesenchymal cells among CTCs have been found to accompany cycles of response to therapy and disease progression in breast cancer (21). Similarly, epithelial-to-mesenchymal transition (EMT) has been shown to mediate resistance to the drug Docetaxel in prostate cancer (22). CTCs, and especially CTC clusters, are also generally more resistant to chemotherapy, possibly due to lack of proliferation and their enrichment in mesenchymal cells (23–25) or cell detachment per se (by mechanisms independent of EMT) (26). Consequently, drugs and therapies directed at targeting specific phenotypes, or blocking cellular plasticity are now being considered (3, 4, 27).
Despite the diversity of genetically-encoded and environmentally-induced cancer cell phenotypes within an individual, most in vitro studies directed at developing new therapeutic strategies generally employ established cell lines with an adherent phenotype [either as monolayers or 3D cancer spheroids (26, 28)]. Nevertheless, efforts are being made toward using additional phenotypes. For instance, patient-derived CTCs have been used to establish in vitro cultures for personalized drug sensitivity tests (29, 30); however, such systems might not fully capture the behavior of real CTCs or CTC clusters (31) and cannot address the range of responses that other phenotypes in a patient might express. More importantly, most studies addressing the effect of drugs and micro-environmental changes generally consider only responses in terms of cell survival and proliferation, without considering possible changes in the phenotypic state of cancer cells (32). This situation is partly due to the lack of model-systems that can be used to simultaneously investigate the response of different cancer cell phenotypes specific to different progression stages, especially the dispersal stage involving CTCs.
An in vitro Model-System to Capture Cancer's Heterogeneity
To this end, we suggest that in vitro model-systems that could be used to address the effect of the same microenvironmental change or selective pressure on different cancer cell phenotypes (adherent—such as in tumors, and in suspension—similar to CTCs and CTC clusters) sharing a related genetic background can be developed. Here, we describe the development of such a model. Specifically, we started with a non-small cell lung cancer line (NCI-H2122 [H2122] (ATCC® CRL5985™); ATCC) that was initially derived from a metastatic site (pleural effusion) of a stage 4 adenocarcinoma lung cancer patient. The line grows as a mixture of adherent cells (with round and epithelial morphology) and cells in suspension—both as single cells and grape-like clusters that are loosely adherent or remain in suspension. This cell line (from here on referred to as the ancestral, or ANC line) was used to experimentally evolve two additional lines: one line selected for adherent growth (referred to as the Adherent-Selected or AS line) and one line selected for growth in suspension (referred to as the Suspension-Selected or SS line), by selectively passaging only the adherent or the suspension cell populations, respectively (Figure 1A).
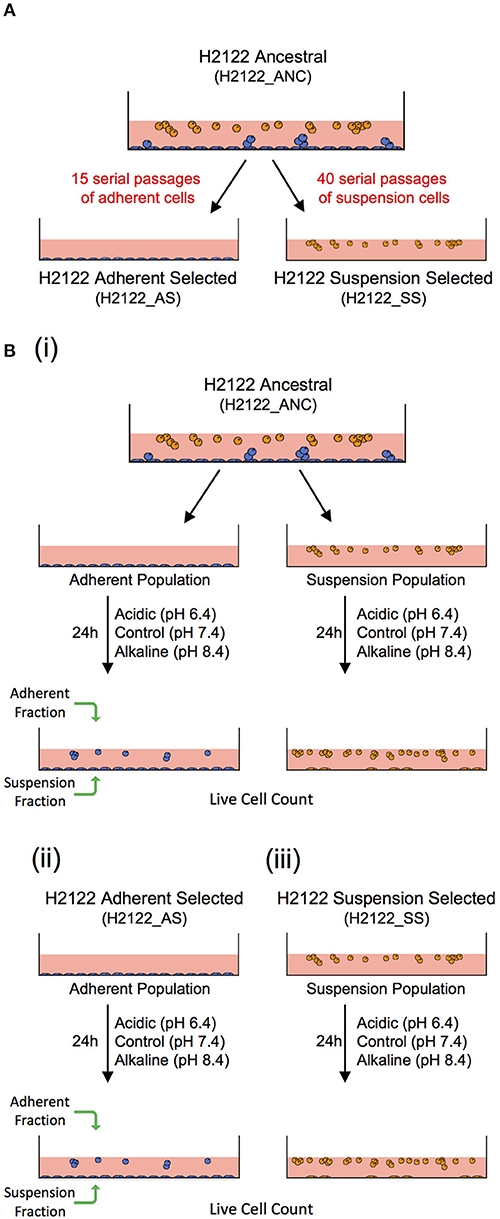
Figure 1. An in vitro model-system to address the effect of the same microenvironmental change on genetically-related but phenotypically distinct cancer cell populations. (A) A non-small cell lung cancer line (H2122_ANC) was used to select for an adherent line (H2122_AS) and a suspension line (H2122_SS) through 15 and 40 serial passages of only the adherent or suspension populations, respectively. (B) Experimental design to address the effect of extracellular acidic and alkaline pH on the ANC_Adherent and ANC_Suspension populations of the (i) ANC line, and the (ii) Adherent Selected (AS) and (iii) Suspension Selected (SS) lines.
These three lines are genetically related but phenotypically distinct, and thus could be used to address the effect of the same microenvironmental challenge on multiple cancer cell phenotypes. Because the ANC line consists of two distinct populations (adherent and suspension) that express phenotypic plasticity (i.e., adherent cells can detach, and cells in suspension can attach), this line could be useful to test the effect of the same challenge on systems that comprise multiple phenotypes, such as during advanced disease. On the other hand, the AS line is strictly adherent (like most established cell lines used in cancer research) and, functionally, can be viewed as analogous to a non-disseminating tumor (i.e., cells do not have the potential to detach). Lastly, as the clusters in both the ANC and SS lines share a series of features with real CTC clusters, including cluster size, morphology, cell-cell connections and the expression of both epithelial and mesenchymal markers (31), these lines can be valuable model-systems to investigate the biology of CTC clusters and test potential therapeutic strategies specifically directed against them.
Proof of Concept
As proof of concept that this model can be used to test the effect of the same challenge on a system comprising genetically-related but phenotypically distinct populations with potential for phenotypic plasticity, we investigated the response of the lines described above to changes in extracellular pH (pHe), in terms of both overall sensitivity and phenotypic plasticity (i.e., the ability of cells to detach and survive in suspension or to attach). Extracellular pH was chosen as the experimental variable because it is known to affect cancer cell behaviors and metastatic potential of tumors in vivo (33); for instance, acidic pHe (around 6.4) promotes metastasis (34), whereas strategies that employ buffers to restore pH to the normal range can suppress this process (35, 36). Recent in vitro studies also showed a reduction in cell adhesion (paralleled by changes in the expression of EMT markers) in two cancer cell lines exposed to acidic pH (6.6) for 48 h (37). Nevertheless, the two pH levels used in this study were chosen arbitrarily as 1 unit below and above the pH of the growth medium, simply as a means to induce measurable non-lethal environmental alterations that can cause clear and quantifiable phenotypic changes (i.e., cell detachment/attachment).
Cultures were maintained in RPMI-1640 medium (ATCC) supplemented with 10% fetal bovine serum (ATCC) and 1% GIBCO™ Penicillin-Streptomycin (10,000 U/ml), from here on referred to as RPMI. Acidic and alkaline media were prepared by adjusting the pH of RPMI (pH 7.4) with 1M HCl (to pH 6.4) and 1M NaOH (to pH 8.4), respectively. For the ANC line, 2.0 × 105 cells were seeded in individual wells of a 12-well plate and grown in regular RPMI overnight, to allow the adherent population to attach to the substrate before the experimental treatments. Next day, the suspension cell population (ANC_Suspension population) was removed from these wells and re-suspended in media at pH 6.4 (acidic), 7.4 (control), or 8.4 (alkaline), and then placed into new wells. The cells adherent to the wells (ANC_Adherent population) were also subjected to the same three media. For the AS cell line, 2.0 × 105 cells per well were seeded in RPMI, allowed to adhere overnight and then the medium was replaced with either control, acidic or alkaline media. For the SS line, 2.0 × 105 cells were seeded in individual wells containing media at each of the three pH levels. Twenty-four hours later, the suspension and adherent cell fractions from each well were collected separately and the number of live cells in each fraction was assessed (Figure 1B). The total numbers of live cells (irrespective of phenotype) in each of the four populations, as well as the numbers of live cells for each fraction/phenotype separately, were compared among the three pH group levels using a one-way ANOVA; Dunnett's multiple comparisons test was used to evaluate significant differences (p < 0.03) between each treatment and the control (GraphPad Prism7).
Different Phenotypes Respond Differently to the Same Set of Challenges
We subjected each of the four cancer cell populations that share a related genetic background but express distinct phenotypes (i.e., ANC_Adherent, ANC_Suspension, AS, and SS) to media of three pH levels—acidic, control, and alkaline, and assessed differences in overall population size and phenotypic state (adherent vs. suspension; Figure 1B). We found that although changes in pH levels did not have a strong effect on the overall size of the populations (with the exception of the acidic treatment of the ANC_Adherent population; Figure 2A; p < 0.001), the acidic and alkaline treatments drastically affected the phenotypic composition (relative to the control pH) of three of the four populations (Figure 2). In the ANC_Adherent population, acidic pH resulted in a higher percentage of suspension cells (3-fold difference, relative to the control), whereas in the alkaline pH treatment, the percentage of suspension cells was half of that in the control (Figure 2A). In the ANC_Suspension population, the adherent fraction was completely lost in the acidic environment, but in the alkaline media the percentage of adherent cells was almost 4-time higher relative to the control (Figure 2B). Lastly, while the AS line was unaffected by either treatment (Figure 2C), the SS line recovered its ability to adhere in the alkaline environment (Figure 2D). Interestingly, in contrast to the epithelial-like morphology expressed by most alkaline-induced adherent cells of the ANC_Suspension population, most SS adherent cells exhibited a round morphology (Figures 2G,H).
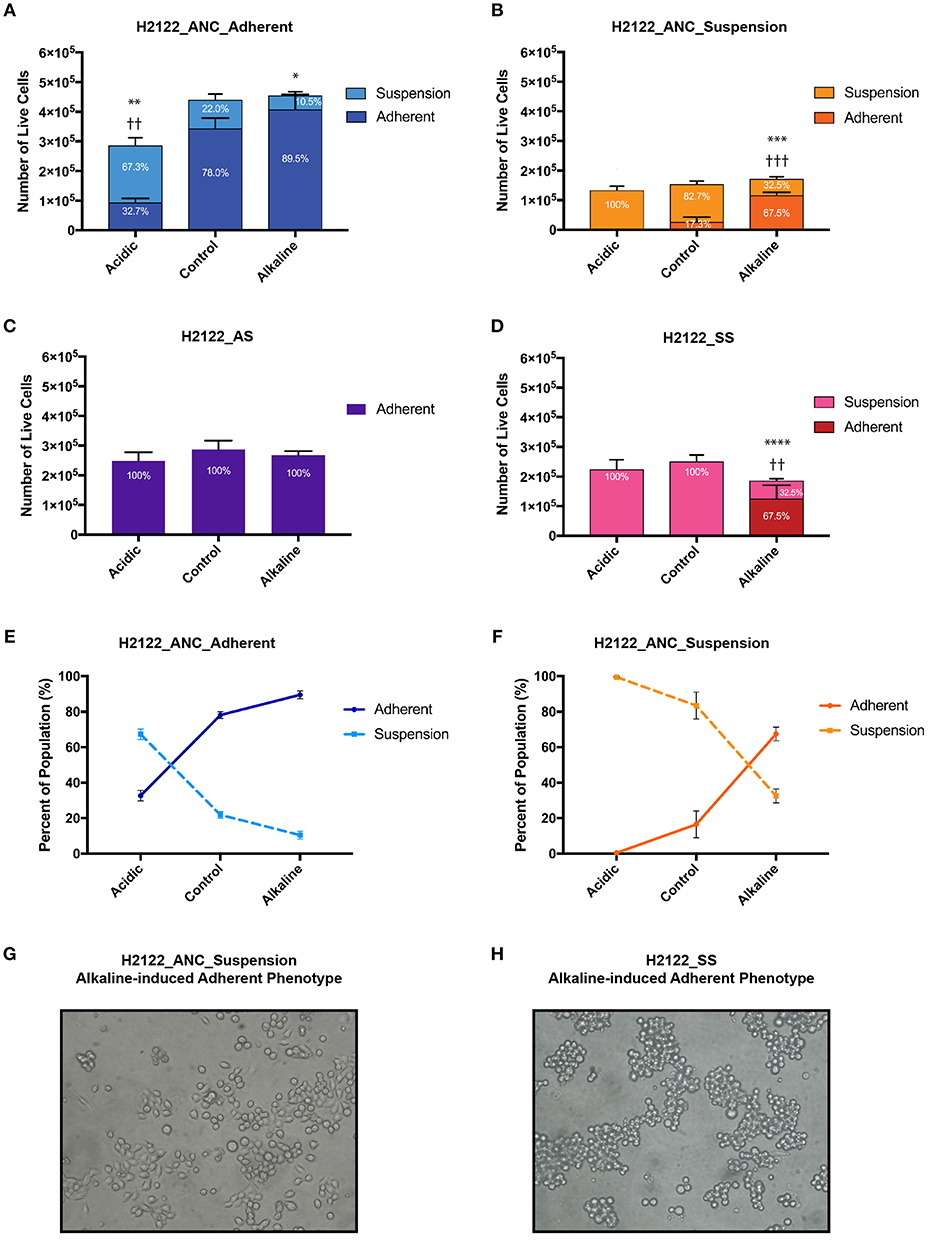
Figure 2. The effect of extracellular pH on the size (as number of live cells) and phenotypic composition (as percentage of live cells in the adherent and suspension cell fractions relative to the entire population) of four genetically-related but phenotypically distinct cancer cell populations subjected for 24 h to acidic (pH 6.4), control (pH 7.4), and alkaline (pH 8.4) media. (A) H2122_ANC_Adherent Population. (B) H2122_ANC_Suspension Population. (C) H2122_AS line. (D) H2122_SS line. (E,F) Changes in the proportion of the suspension and adherent fractions in the Adherent and Suspension populations of the ANC line, as a function of pH [data are from (A,B)]. (H) Attached H2122_SS cells exhibit round morphology following treatment with alkaline media; (G) Attached H2122_ANC_Suspension cells exhibit a mixture of round and epithelial-like morphology following treatment with alkaline media. All treatments were performed in 1.5 ml media, with 3 biological replicates. Viability was assessed using SYTO 9 and Propidium Iodide (PI) (Molecular Probes) and the Countess II FL Automated Cell Counter (ThermoFisher Scientific). SYTO 9 stains DNA in both dead and live cells, while PI is only permeant to necrotic and late apoptotic cells. Because this method could miss early apoptotic cells with intact membranes and apoptotic cells that disintegrated, numbers of dead cells are not reported here. All data were expressed as means ± SD of 3 biological replicates (each with 3 technical replicates). * and † indicate significant differences in the numbers of live cells of the suspension and adherent fractions, respectively, between treatments and control (*p < 0.0332, **/††p < 0.0021, ***/†††p < 0.0002, ****p < 0.0001). Total population sizes did not show statistically significant differences, except in one case (see text).
Generally, the four populations displayed substantial heterogeneity in response to the two pH challenges: the phenotypic composition of the adherent and suspension populations of the ANC line was affected by both acidic and alkaline pHe, the SS was only affected by the alkaline pHe, and the AS appeared unaffected by both treatments. Also, the same challenge had different effects on the four populations. Acidic pH affected the phenotypic composition of both the adherent and suspension populations of the ANC line (and also affected the size of the ANC_Adherent population), but had no effect on the phenotypic composition of the AS and SS populations. On the other hand, alkaline media affected both the adherent and suspension populations of the ANC line as well as the SS line, while the AS line was unaffected. Taken together, these findings argue for the potential benefit of testing the effect of therapies on different phenotypes using systems similar to the one we developed.
Phenotypic Plasticity Underlies pH-induced Differences in Phenotypic Composition
Because of the short length of the treatment (24 h) and the doubling time of this cell line (estimated as at least 48 h), the drastic differences in the proportion of phenotypes we observed (in most cases without differences in the overall population size) can only be explained by invoking phenotypic switches. For instance, relative to their controls, in both ANC populations (ANC_Adherent and ANC_Suspension), acidic pH resulted in a higher proportion of cells in suspension (paralleled by a lower proportion of adherent cells; Figure 2E). At least for the ANC_Adherent population (that shows a much larger number of cells in suspension relative to the control), this difference can only be accounted for by increased cell detachment in the acidic environment relative to its control. Similarly, alkaline pH had the opposite effect on both populations—it caused a higher proportion of the adherent phenotype at the expense of the suspension phenotype (Figure 2F). Again, at least for the ANC_Suspension population in the alkaline environment, the higher number of adherent cells (relative to its control) can only be explained by a large number of suspension cells assuming an adherent phenotype. Such pH-induced changes in phenotypic states are further supported by the behavior of the SS line, which is phenotypically homogeneous in the control medium, but becomes heterogenous in the alkaline medium, with a large proportion of cells being adherent (Figure 2D).
Overall, we suggest that this model-system can also be used to gain a better understanding of potential phenotypic switches in response to microenvironmental changes. As the behavior of both ANC populations is consistent with previously known responses to pH in vivo [i.e., acidic tumor environments promote metastasis while neutralization can suppress it; (35)], our findings argue that this line could be useful as a proxy for metastatic stages, in which the phenotypic state of cells (especially with respect to cell detachment and cell survival in circulation) is likely to be affected by changes in their microenvironments. On the other hand, the AS line did not exhibit plasticity, providing a complementary phenotypic state to the ANC line, which can allow investigations into potentially dissimilar responses of genetically-related but phenotypically distinct populations.
Future Directions
The specific goal of this Perspective is 3-fold: (i) to argue that the use of in vitro model-systems that comprise genetically-related but phenotypically distinct cancer cell populations can help capture the phenotypic landscape in a patient with advanced disease, (ii) to highlight the different responses exhibited by different cancer cell populations that are subjected to the same microenvironmental challenge, in terms of both their overall sensitivity and phenotypic state, and (iii) to suggest that these model-systems can be used to both test the effect of therapies on more than one phenotype (to ensure effectiveness and avoid unexpected effects) and design therapies specific for different phenotypes (to improve their effectiveness).
As proof of concept that such lines can be valuable and useful complementary systems for testing and developing new therapies, we used media of two different pH levels and investigated the response of four genetically-related but phenotypically distinct populations. Our data showed clearly that not all phenotypes are sensitive to the same challenge, and that the same challenge can have different effects on both the sensitivity and the phenotypic composition of distinct populations. We believe these findings provide a strong case for the usefulness of such model-systems to investigate the ways in which therapies can affect the range of cancer cell phenotypes likely to be present in the same individual. This would both increase the effectiveness of therapies (as different phenotypes might show different sensitivities to the same treatment) and address potential negative effects (by inducing alternative phenotypes). For instance, the same challenge (e.g., low pHe in our study) that may induce an apparent decrease in the size of a population (as in the ANC_Adherent population; Figure 2A) might have no effect on other phenotypically different populations (the ANC_Suspension population, AS and SS lines; Figures 2B–D). More importantly, although a therapy might result in the decline or stabilization of a cancer population, its phenotypic composition can change in significant ways. For instance, while a therapy may decrease the size of a population (as low pHe did for the ANC_Adherent population), it may also induce the detachment of live cells (Figure 2A; note that the acid-induced suspension fraction proliferated and expressed a mixture of both phenotypes when re-cultured in the three pH media; data not shown). Similarly, a treatment (in our case, alkaline media) that might repress cell detachment (the ANC_Adherent population; Figure 2A) may also promote the adherence of cells already in circulation (the SS line; Figure 2D) and possibly facilitate the colonization stage. Lastly, during systemic therapies, a therapeutic agent can reach different levels in distinct regions of the body (bloodstream vs. tumor); this can have different effects in terms of the cell phenotype induced: at high levels it can promote one phenotype (and inhibit the alternative state) while at low levels can have the opposite effect (Figures 2E,F).
Although here we used pH as a proxy for microenvironmental changes, other factors are likely to have similar differential effects on the sensitivity and phenotypic plasticity of cancer cell populations. For instance, we found that the four cell populations in our model-system have different sensitivities to glucose deprivation, with the SS line being most resistant to glucose deprivation (our unpublished data). Similar findings have been reported for two sublines (with distinct phenotypes) derived from a small cell lung line (NCI-H69); the adherent subline showed increased resistance to etoposide, cyclophosphamide, and gamma radiation when compared to the original line growing in suspension (38, 39). Regarding phenotypic plasticity, we found that glucose deprivation alone or in combination with metformin [an anti-diabetic drug with potential anti-cancer activities; (40)] induces cell detachment in the H2122_ANC and AS lines (our unpublished data). Similar responses have been also reported in two breast cancer lines as co-treatment with 2-deoxy-D-glucose (used to mimic glucose starvation) and metformin resulted in the detachment of viable cells (32).
The model-system we presented here used an established non-small lung cancer cell line that we experimentally evolved into two additional lines. The ANC line is highly heterogeneous, containing a mixture of cells expressing one of two stable phenotypes (strictly adherent or strictly suspension) as well as cells expressing some level of phenotypic plasticity (being able to express both phenotypes). Its heterogeneity and plasticity could be valuable in addressing the effect of therapies on multiple plastic phenotypes. The cell clusters in this line are also heterogeneous. They consist of a mixture of cells expressing epithelial or mesenchymal markers—a feature shared with CTC clusters isolated from cancer patients, and thus could be used in developing therapies directly targeted against CTC clusters (31).
Many other cancer cell lines available from ATCC (of lung, breast, prostate, pancreatic, colon, gastric origin) grow as mixtures of adherent cells and cells in suspension, and thus can be used as proxies for genetically heterogeneous and phenotypically plastic tumors with dispersal potential. Selection experiments similar to the ones we presented here can also be performed on such lines to evolve specific phenotypes. In fact, a small cell lung cell line (NCI-H69) that grows mostly as tightly-packed floating aggregates has been previously used to derive an adherent subline. The suspension and adherent lines were shown to differ in their sensitivity to drugs (discussed above) as well as expression of EMT markers, with the ancestral suspension line being positive for E-cadherin while the derived adherent line was negative for E-cadherin and positive for vimentin (39). Many other established cell lines that grow strictly (or mainly) as cell clusters in suspension are also available from ATCC, and they can be used to identify potential targets that are common to the CTC cluster phenotype (31).
Conclusions
Based on the growing evidence regarding the impact of phenotypic heterogeneity and plasticity on the success of current therapies (discussed in the Introduction) we argue that alternative model-systems that can better capture cancer's heterogeneity and plasticity could be increasingly integrated in the development of new therapeutic strategies, in three important ways. First, since responses to therapies are known to differ among cancer cell phenotypes (with some phenotypes—such as CTCs and CTC clusters being more refractory to standard treatments), model-systems expressing heterogeneous phenotypes could assist in the development of phenotype-specific therapies. Second, changes in phenotypic state should also be evaluated to address potential unforeseen effects. Third, to increase the efficiency of therapies, strategies directed at altering the tumor microenvironment [such as altering pHe; (36, 41)] to prevent phenotypic transitions could be considered; model-systems like the one we developed can help identify such strategies. Although we are aware of the limitations of in vitro models (including the system we presented here), we believe that the use of experimental evolution and established cell lines that express phenotypic plasticity can provide invaluable general insights into the complex responses that therapies can trigger in a cancer patient.
Data Availability
All datasets generated for this study are included in the manuscript.
Author Contributions
EJ designed and performed research, acquired, analyzed and interpreted data, and contributed to the writing of the manuscript. IC designed and performed research, acquired, analyzed and interpreted data, and edited the manuscript. AN designed research, interpreted data, wrote the manuscript, and supervised the study.
Funding
This work was supported by a grant from the Natural Sciences and Engineering Research Council (NSERC) of Canada to AMN.
Conflict of Interest Statement
The authors declare that the research was conducted in the absence of any commercial or financial relationships that could be construed as a potential conflict of interest.
Acknowledgments
We would like to thank Alexander May and Dakota van Dijk for their previous contributions to the development of the lines used in this study, and Alexander May and Ian Robey for comments on the manuscript.
References
1. McGranahan N, Swanton C. Clonal heterogeneity and tumor evolution: past, present, and the future. Cell. (2017) 168:613–28. doi: 10.1016/j.cell.2017.01.018
2. Fisher R, Pusztai L, Swanton C. Cancer heterogeneity: implications for targeted therapeutics. Br J Cancer. (2013) 108:479–85. doi: 10.1038/bjc.2012.581
3. Doherty M, Smigiel J, Junk D, Jackson M. Cancer stem cell plasticity drives therapeutic resistance. Cancers. (2016) 8:8. doi: 10.3390/cancers8010008
4. Brooks MD, Burness ML, Wicha MS. Therapeutic implications of cellular heterogeneity and plasticity in breast cancer. Cell Stem Cell. (2015) 17:260–71. doi: 10.1016/j.stem.2015.08.014
5. Bhatia S, Frangioni JV, Hoffman RM, Iafrate AJ, Polyak K. The challenges posed by cancer heterogeneity. Nat Biotechnol. (2012) 30:604–10. doi: 10.1038/nbt.2294
6. Chapman A, Fernandez del Ama L, Ferguson J, Kamarashev J, Wellbrock C, Hurlstone A. Heterogeneous tumor subpopulations cooperate to drive invasion. Cell Rep. (2014) 8:688–95. doi: 10.1016/j.celrep.2014.06.045
7. Andor N, Graham TA, Jansen M, Xia LC, Aktipis CA, Petritsch C, et al. Pan-cancer analysis of the extent and consequences of intratumor heterogeneity. Nat Med. (2016) 22:105–13. doi: 10.1038/nm.3984
8. Aktipis CA, Nesse RM. Evolutionary foundations for cancer biology. Evol Appl. (2013) 6:144–59. doi: 10.1111/eva.12034
9. Merlo LMF, Pepper JW, Reid BJ, Maley CC. Cancer as an evolutionary and ecological process. Nat Rev Cancer. (2006) 6:924–35. doi: 10.1038/nrc2013
10. Maley CC, Aktipis A, Graham TA, Sottoriva A, Boddy AM, Janiszewska M, et al. Classifying the evolutionary and ecological features of neoplasms. Nat Rev Cancer. (2017) 17:605–19. doi: 10.1038/nrc.2017.69
11. Amend SR, Roy S, Brown JS, Pienta KJ. Ecological paradigms to understand the dynamics of metastasis. Cancer Lett. (2016) 380:237–42. doi: 10.1016/j.canlet.2015.10.005
12. Lloyd MC, Cunningham JJ, Bui MM, Gillies RJ, Brown JS, Gatenby RA. Darwinian dynamics of intratumoral heterogeneity: not solely random mutations but also variable environmental selection forces. Cancer Res. (2016) 76:3136–44. doi: 10.1158/0008-5472.CAN-15-2962
13. Szakács G, Paterson JK, Ludwig JA, Booth-Genthe C, Gottesman MM. Targeting multidrug resistance in cancer. Nat Rev Drug Discov. (2006) 5:219–34. doi: 10.1038/nrd1984
14. Sun X, Hu B. Mathematical modeling and computational prediction of cancer drug resistance. Brief Bioinform. (2018) 19:1382–99. doi: 10.1093/bib/bbx065
15. Chatterjee N, Bivona TG. Polytherapy and targeted cancer drug resistance. Trends Cancer. (2019) 5:170–82. doi: 10.1016/j.trecan.2019.02.003
16. Hansen E, Woods RJ, Read AF. How to use a chemotherapeutic agent when resistance to it threatens the patient. PLoS Biol. (2017) 15:e2001110. doi: 10.1371/journal.pbio.2001110
17. Lambert AW, Pattabiraman DR, Weinberg RA. Emerging biological principles of metastasis. Cell. (2017) 168:670–91. doi: 10.1016/j.cell.2016.11.037
18. Hamilton G, Hochmair M, Rath B, Klameth L, Zeillinger R. Small cell lung cancer: circulating tumor cells of extended stage patients express a mesenchymal-epithelial transition phenotype. Cell Adhes Migr. (2016) 10:360–7. doi: 10.1080/19336918.2016.1155019
19. Gupta PB, Pastushenko I, Skibinski A, Blanpain C, Kuperwasser C. Phenotypic plasticity: driver of cancer initiation, progression, and therapy resistance. Cell Stem Cell. (2019) 24:65–78. doi: 10.1016/j.stem.2018.11.011
20. Jolly MK, Somarelli JA, Sheth M, Biddle A, Tripathi SC, Armstrong AJ, et al. Hybrid epithelial/mesenchymal phenotypes promote metastasis and therapy resistance across carcinomas. Pharmacol Ther. (2019) 194:161–84. doi: 10.1016/j.pharmthera.2018.09.007
21. Yu M, Bardia A, Wittner BS, Stott SL, Smas ME, Ting DT, et al. Circulating breast tumor cells exhibit dynamic changes in epithelial and mesenchymal composition. Science. (2013) 339:580–4. doi: 10.1126/science.1228522
22. Marín-Aguilera M, Codony-Servat J, Reig Ò, Lozano JJ, Fernández PL, Pereira MV, et al. Epithelial-to-mesenchymal transition mediates docetaxel resistance and high risk of relapse in prostate cancer. Mol Cancer Ther. (2014) 13:1270–84. doi: 10.1158/1535-7163.MCT-13-0775
23. Hou JM, Krebs MG, Lancashire L, Sloane R, Backen A, Swain RK, et al. Clinical significance and molecular characteristics of circulating tumor cells and circulating tumor microemboli in patients with small-cell lung cancer. J Clin Oncol. (2012) 30:525–32. doi: 10.1200/JCO.2010.33.3716
24. Krebs MG, Hou J-M, Sloane R, Lancashire L, Priest L, Nonaka D, et al. Analysis of circulating tumor cells in patients with non-small cell lung cancer using epithelial marker-dependent and -independent approaches. J Thorac Oncol. (2012) 7:306–15. doi: 10.1097/JTO.0b013e31823c5c16
25. Giuliano M, Shaikh A, Lo HC, Arpino G, De Placido S, Zhang XH, et al. Perspective on circulating tumor cell clusters: why it takes a village to metastasize. Cancer Res. (2018) 78:845–52. doi: 10.1158/0008-5472.CAN-17-2748
26. Kaushik V, Yakisich JS, Way LF, Azad N, Iyer AKV. Chemoresistance of cancer floating cells is independent of their ability to form 3D structures: implications for anticancer drug screening. J Cell Physiol. (2019) 234:4445–53. doi: 10.1002/jcp.27239
27. Sylvester PW. Targeting met mediated epithelial-mesenchymal transition in the treatment of breast cancer. Clin Transl Med. (2014) 3:30. doi: 10.1186/s40169-014-0030-5
28. Zanoni M, Piccinini F, Arienti C, Zamagni A, Santi S, Polico R, et al. 3D tumor spheroid models for in vitro therapeutic screening: a systematic approach to enhance the biological relevance of data obtained. Sci Rep. (2016) 6:19103. doi: 10.1038/srep19103
29. Cayrefourcq L, Mazard T, Joosse S, Solassol J, Ramos J, Assenat E, et al. Establishment and characterization of a cell line from human circulating colon cancer cells. Cancer Res. (2015) 75:892–901. doi: 10.1158/0008-5472.CAN-14-2613
30. Lim J, Salamon H, Chow T, Adams B, Hough J, Ryan CJ, et al. Ex vivo propagation and characterization of circulating tumor cell clusters from late stage prostate cancer patients. J Clin Oncol. (2016) 34:314. doi: 10.1200/jco.2016.34.2_suppl.314
31. May AN, Crawford BD, Nedelcu AM. In vitro model-systems to understand the biology and clinical significance of circulating tumor cell clusters. Front Oncol. (2018) 8:63. doi: 10.3389/fonc.2018.00063
32. Bizjak M, Malavašič P, Dolinar K, Pohar J, Pirkmajer S, Pavlin M. Combined treatment with Metformin and 2-deoxy glucose induces detachment of viable MDA-MB-231 breast cancer cells in vitro. Sci Rep. (2017) 7:1761. doi: 10.1038/s41598-017-01801-5
33. White KA, Grillo-Hill BK, Barber DL. Cancer cell behaviors mediated by dysregulated pH dynamics at a glance. J Cell Sci. (2017) 130:663–9. doi: 10.1242/jcs.195297
34. Rofstad EK, Mathiesen B, Kindem K, Galappathi K. Acidic extracellular pH promotes experimental metastasis of human melanoma cells in athymic nude mice. Cancer Res. (2006) 66:6699–707. doi: 10.1158/0008-5472.CAN-06-0983
35. Robey IF, Baggett BK, Kirkpatrick ND, Roe DJ, Dosescu J, Sloane BF, et al. Bicarbonate increases tumor pH and inhibits spontaneous metastases. Cancer Res. (2009) 69:2260–8. doi: 10.1158/0008-5472.CAN-07-5575
36. Ibrahim-Hashim A, Abrahams D, Enriquez-Navas PM, Luddy K, Gatenby RA, Gillies RJ. Tris-base buffer: a promising new inhibitor for cancer progression and metastasis. Cancer Med. (2017) 6:1720–9. doi: 10.1002/cam4.1032
37. Riemann A, Rauschner M, Gießelmann M, Reime S, Haupt V, Thews O. Extracellular acidosis modulates the expression of Epithelial-Mesenchymal Transition (EMT) markers and adhesion of epithelial and tumor cells. Neoplasia. (2019) 21:450–8. doi: 10.1016/j.neo.2019.03.004
38. Kraus AC, Ferber I, Bachmann S-O, Specht H, Wimmel A, Gross MW, et al. In vitro chemo- and radio-resistance in small cell lung cancer correlates with cell adhesion and constitutive activation of AKT and MAP kinase pathways. Oncogene. (2002) 21:8683–95. doi: 10.1038/sj.onc.1205939
39. Krohn A, Ahrens T, Yalcin A, Plönes T, Wehrle J, Taromi S, et al. Tumor cell heterogeneity in Small Cell Lung Cancer (SCLC): phenotypical and functional differences associated with Epithelial-Mesenchymal Transition (EMT) and DNA methylation changes. PLoS ONE. (2014) 9:e100249. doi: 10.1371/journal.pone.0100249
40. Vancura A, Bu P, Bhagwat M, Zeng J, Vancurova I. Metformin as an anticancer agent. Trends Pharmacol Sci. (2018) 39:867–78. doi: 10.1016/j.tips.2018.07.006
Keywords: extracellular pH, phenotypic plasticity, therapy, H2122, metastasis, experimental evolution, selection, microenvironment
Citation: Jong ED, Chan ICW and Nedelcu AM (2019) A Model-System to Address the Impact of Phenotypic Heterogeneity and Plasticity on the Development of Cancer Therapies. Front. Oncol. 9:842. doi: 10.3389/fonc.2019.00842
Received: 20 February 2019; Accepted: 15 August 2019;
Published: 29 August 2019.
Edited by:
Thomas Daubon, Institut National de la Santé et de la Recherche Médicale (INSERM), FranceReviewed by:
Marco A. Velasco-Velazquez, National Autonomous University of Mexico, MexicoClaudia Matteucci, University of Rome Tor Vergata, Italy
Copyright © 2019 Jong, Chan and Nedelcu. This is an open-access article distributed under the terms of the Creative Commons Attribution License (CC BY). The use, distribution or reproduction in other forums is permitted, provided the original author(s) and the copyright owner(s) are credited and that the original publication in this journal is cited, in accordance with accepted academic practice. No use, distribution or reproduction is permitted which does not comply with these terms.
*Correspondence: Aurora M. Nedelcu, YW5lZGVsY3VAdW5iLmNh
†Present address: Eric D. Jong, Lunenfeld-Tanenbaum Research Institute, Sinai Health System, Toronto, ON, Canada; Institute of Medical Science, University of Toronto, Toronto, ON, Canada
Irina C. W. Chan, Canadian College of Naturopathic Medicine, Toronto, ON, Canada