- 1Department of Oncology, The First Affiliated Hospital of Wenzhou Medical University, Wenzhou, China
- 2Department of Head and Neck Surgery, Tumor Hospital of Ganzhou, Ganzhou, China
- 3Department of Thoracic Surgery, The First Affiliated Hospital of Nanjing Medical University, Nanjing, China
- 4Department of Oncology, The First Affiliated Hospital of Nanjing Medical University, Nanjing, China
Background: Few studies have addressed the role of immune-related genes in the survival and prognosis of different esophageal cancer (EC) sub-types. We established two new prognostic model indexes by bioinformatics analysis to select patients with esophageal squamous cell carcinoma (ESCC) and esophageal adenocarcinoma (EAC) who may benefit from immunotherapy.
Methods: Based on TCGA and ImmPort data sets, we screened immune genes differentially expressed between tumor and normal tissues in ESCC and EAC and analyzed the relationship between these genes and patient survival outcomes. We established the risk score models of immune-related genes in ESCC and EAC by multivariate COX regression analysis.
Results: We identified 12 and 11 immune-related differentially expressed genes associated with the clinical prognosis of ESCC and EAC respectively, based on which two prognostic risk score models of the two EC sub-types were constructed. It was found that the survival probability of patients with high scores was significantly lower than that of patients with low scores (p < 0.001). BMP1, EGFR, S100A12, HLA-B, TNFSF18, IL1B, MAPT and OXTR were significantly related to sex, TNM stage or survival outcomes of ESCC or EAC patients (p < 0.05). In addition, the risk score of ESCC was significantly correlated with the level of B cell infiltration in immune cells (p < 0.05).
Conclusions: The prognosis-related immune gene model indexes described herein prove to be useful prognostic biomarkers of the two EC sub-types in that they may provide a reference direction for looking for the beneficiaries of immunotherapy for EC patients.
Introduction
Esophageal cancer (EC) is the seventh most common cancer and the sixth leading cause of cancer death worldwide, with a 5-year survival rate of lower than 20%, seriously affecting human health (1, 2). In 2017, the number of global new EC cases and deaths is 473000 and 436000, respectively (3). It is estimated that there will be about 18440 diagnosed EC cases in 2020 causing about 16170 deaths in the United States (4). The treatment of EC mainly focuses on preoperative chemotherapy or postoperative radiotherapy and chemotherapy. However, about 50% EC patients respond unsatisfactorily to the treatment due to resistance of cancer cells to the chemotherapeutic drugs (5). Esophageal squamous cell carcinoma(ESCC) and esophageal adenocarcinoma (EAC) are two pathological types of EC characterized by different distribution, etiology and risk factors (6, 7). In addition, they vary in molecular characteristics and undergo different changes in their specific genes (8).
In recent years, immunotherapy has emerged as a new treatment for various malignant tumors including EC, knowing that it plays a role in immunosuppression in the tumor micro-environment. Several clinical trials have shown that immune checkpoint inhibitors (ICI) nivolumab and Pembrolizumab can be regarded as new standard second-line treatment strategies for EC (9–14). ICIs include anti-PD1, anti-PD-L1, and anti-CTLA-4 (15). However, only a small number of patients can clinically benefit from ICI treatment because of drug resistance, and some patients even deteriorate sharply after immunotherapy. Therefore, it is necessary to identify biomarkers that can accurately predict patients who could benefit from immunotherapy for the sake of providing individualized treatment (9).
At present, microsatellite instability (MSI), PD-L1, tumor mutation burden (TMB), DNA mismatch repair deficiency (dMMR) and TILs factors are the only factors that are confirmed to be able to predict the efficacy of ICI response (16, 17). One study identified that immune-related genes (ABL1, ATF2, ATG5, C6, CD38, HMGB1, ICOSLG, IL12RB2, and PLAU) were significantly associated with overall survival (OS) of ESCC patients (18). With the emergence of open data sets of gene expression, some studies established immune gene prognostic markers for predicting the survival of EC patients (19). A large-scale multicenter retrospective study analyzed and established immune markers based on four genes (SERPINE1, MMP12, PLAUR and Eps8) in predicting pathological complete remission of neoadjuvant radiotherapy and chemotherapy in EC patients, which is believed to lay a foundation for the combination of neoadjuvant radiotherapy and chemotherapy with immunotherapy (20). The study of Lu et al. showed that CD103+CD8+TIL displayed the tissue-resident memory T cell phenotype and showed high expression of immune checkpoint (PD-1, TIM-3) in ESCC. After blocking anti-PD-1, CD103+CD8+TIL induced strong anti-tumor immunity (21). To help find individual immunotherapy targets, some recent studies established comprehensive prognostic indicators of lung squamous cell carcinoma, colorectal cancer and other tumors based on immune genome map analysis (22–29). However, the prognostic value of immune-related genes in ESCC and EAC as two pathological sub-types of EC has not been fully elucidated.
Using TCGA and ImmPort database, we combined the patient’s clinical information with an immune-related genomic map, and found immune-related genes that were significantly related to prognosis with ESCC and EAC respectively. Based on these genes, we constructed individual immune prognostic index models for EC patients, hoping that they could lay a foundation for promoting individualized immunotherapy of EC.
Materials and Methods
Data Collection and Processing
The process of this study is shown in Figure 1. We used the TCGA database (https://cancergenome.nih.gov/) to obtain immune-related gene transcriptome data, clinical data, and follow-up data of EC patients. In the ImmPort data portal (https://www.immport.org/), the list of immune-related genes was derived.
Screening of Differentially Expressed Genes
We used the edgeR package to find differentially expressed genes, using |logFC|>1 and FDR < 0.05 as the screening criteria.
Identification of Differentially Expressed Immune-Related genes
We crossed the above differentially expressed genes with immune genes and used the edgeR software package of R software (http://bioconductor.org/Packages/edger/) and Wilcoxon test analysis to obtain the immune differentially expressed genes related to EC.
TF Analysis and Construction of the Regulatory Network of TF-Immune-Related Genes
To explore the regulatory mechanism of immune-related genes related to survival, we searched the Cistrome Cancer database (http://cistrome.org), downloaded tumor-related transcription factors(TFs), and extracted differentially expressed TFs related to clinical prognosis. We constructed an interaction network between these TFs and immune differential genes related to prognosis to explore the mechanism of TF in regulating these genes.
Analysis of Survival-Associated Immune-Related Genes
The patients were randomly divided into two groups: training group and testing group. Using the R software survival software package, we carried out univariate Cox analysis in training group to explore the immune-related genes related to survival of EC patients by integrating the differential immunity-related genes with the survival data of the EC patients.
Development of the Immune Gene Prognosis Model in Training Group
Using multivariate Cox regression, we established an evaluation model of prognostic risk indexes based on EC immune-related genes and calculated the risk indexes using the following equation in training group: Risk score= coefficient of multivariate Cox regression(a) × gene expression level(a) + coefficient of multivariate Cox regression(b) × gene expression level(b) + … + coefficient of multivariate Cox regression(n) × gene expression level(n).
Analysis of the Survival Differences Between High- and Low-Risk Patients
Based on the median score of differential immune gene risk scores related to survival, the patients in training group were divided into high- and low-risk groups. Survival curves were mapped out to explore differences in survival prognosis between the two groups. The survival ROC R software package was used to draw the receiver operating characteristic (ROC) and calculated the area under the AUC curve of the patients to judge the true positive rate. Using the Survminer software package of R software, survival prognosis of the patients in the two groups was assessed.
Validation of the Immune Gene Prognosis Model in Testing Group and Entire Group
We verified the reliability of the prognostic score formula in the testing group and the entire group. We used the formula in the training group to calculate the risk score of each patient in the testing group and the entire group, and divided them into high-risk group and low-risk group respectively. Similarly, the ROC curves of the testing group and the entire group were drawn to evaluate the prognostic value of the model.
Clinical Relevance of the Clinical Characteristics
Using the calculated risk score, the clinical correlation between survival-related immune genes and the patient clinical data including sex and tumor stage was evaluated. Using the ggpubr software package, the clinical correlation between the survival-related immune genes and the clinical data including gender and tumor stage was explored.
Evaluation of Immune Cell Infiltration and the Tumor Microenvironment
The data of immune cell infiltration in EC patients were extracted from the Timer database (https://cistrome.shinyapps.io/timer/) to explore whether the risk score was related. The immune cells included B cells, CD4+T cells, CD8+T cells, neutrophils, dendritic cells, and macrophages.
Statistical Analysis
Differences in immune genes were analyzed using R software (version 3.6.1). The expression of immune-related genes and their relationships with survival were analyzed using one-way ANOVA. The classified variables were determined by the χ 2 test, and the variables with p < 0.05 were subjected to multivariate analysis. Univariate and multivariate Cox regression analyses were used to explain clinicopathological features and risk scores on prognosis. The survival ROC R package was used to calculate the ROC curve and evaluate the accuracy of the immune-related gene prediction index. Differences in clinical parameters were analyzed by independent t-test. p < 0.05 was considered statistically significant. The Kaplan-Meier curve was established by R software survival and Survminer software package. Bilateral Log Rank test and Kaplan-Meier method were used to explore the survival of EC patients in high- and low-risk groups.
Results
Identification of Differentially Expressed Immune-Related Genes
Differentially expressed genes were screened between EC and normal tissues from the TCGA database. It was found that 5942 genes were differentially expressed in ESCC, including 4283 up-regulated and 1659 down-regulated genes. Using the same method, 3026 upregulated genes and 625 down-regulated genes were screened in EAC. By crossing these genes with immune genes, we obtained 372 immune-related differential genes in ESCC, of which 257 were up-regulated and 115 were down-regulated (Figures 2A, B). In EAC, 232 immune-related differential genes were upregulated and 37 were down-regulated (Figures 2C, D).
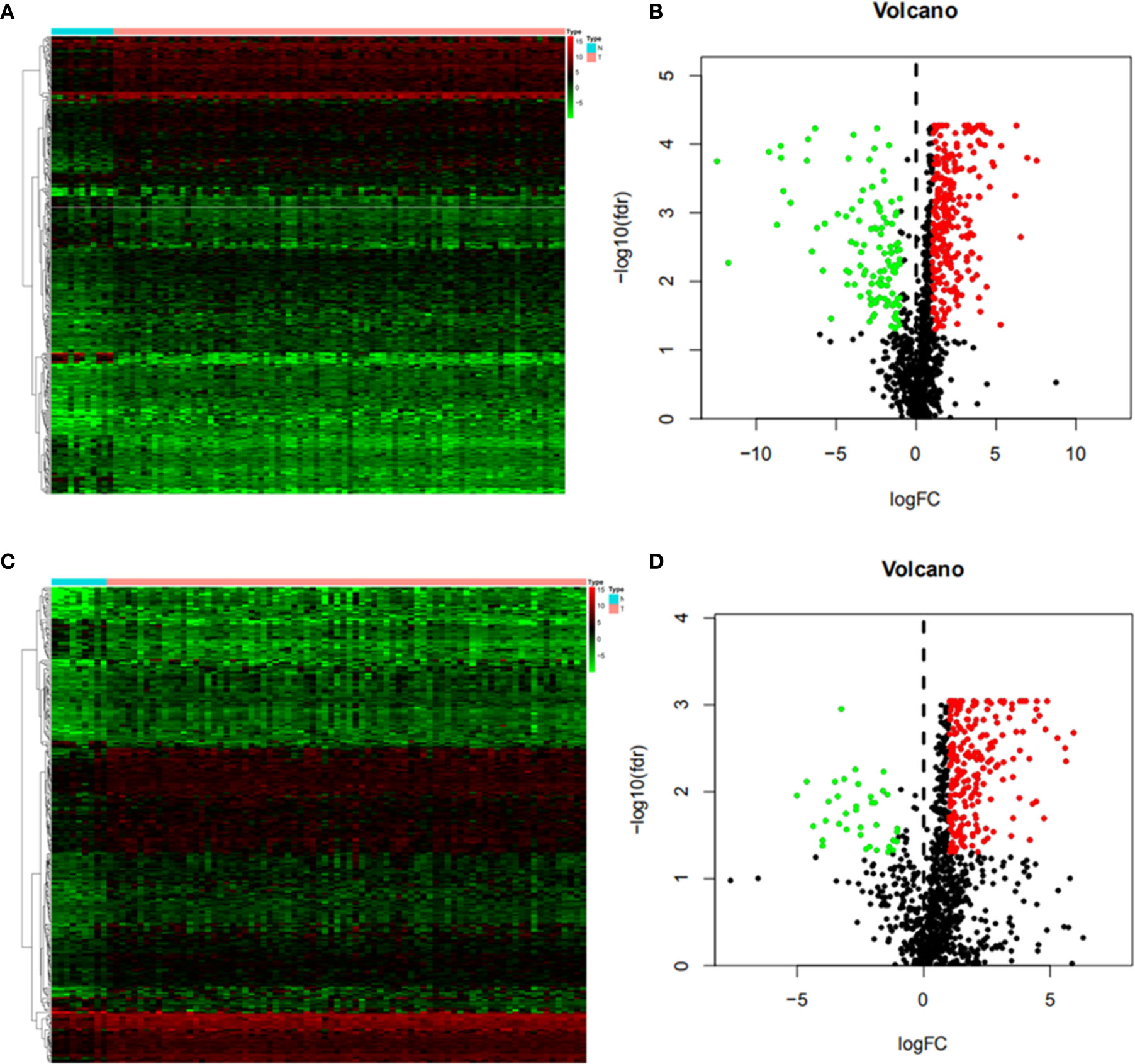
Figure 2 Differential immune genes (A) Heat map of esophageal squamous cell carcinoma(ESCC); (B) Volcanic map of ESCC; (C) Heat map of esophageal adenocarcinoma (EAC); (D) Volcanic map of EAC. The Abscissa of the heat map shows the normal tissue (blue) and esophageal cancer tissue (red), and the ordinate shows the genes. In the volcano map, the blue green and black colors indicate upregulated genes, down-regulated genes and genes with no significant difference respectively.
TF Analysis and Construction of the TF-Immune-Related Gene Regulatory Network
We downloaded 83 and 49 different TFs between the two EC subtypes and normal tissues in the Cistrome database, and found that 65 were upregulated and 18 were down-regulated in ESCC (Figures 3A, B), and 43 were upregulated and 6 were down-regulated in EAC (Figures 3C, D) respectively.
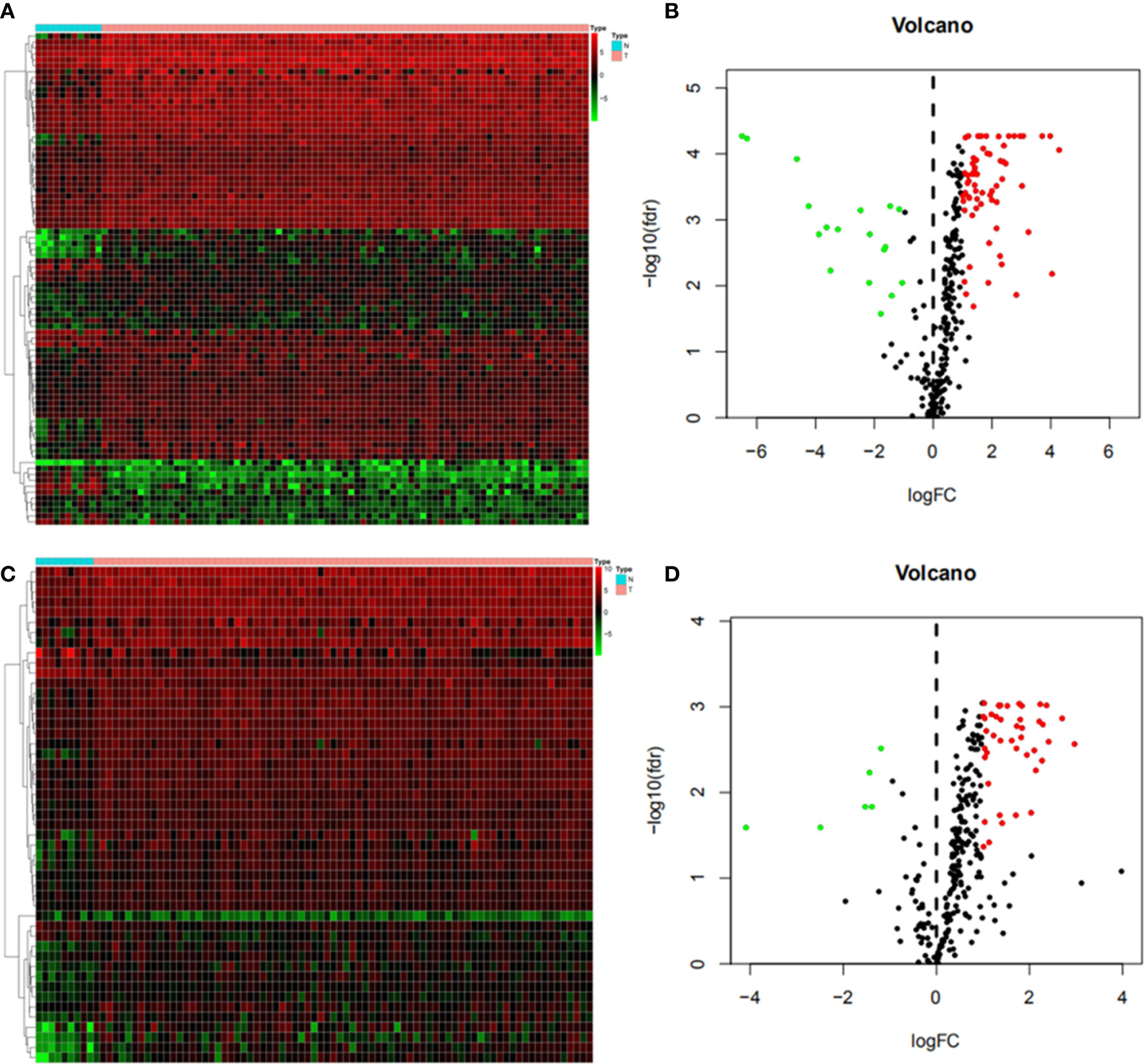
Figure 3 Differential gene expression of immune-related transcription factors (A) Heat map of esophageal squamous cell carcinoma (ESCC); (B) Volcanic map of ESCC; (C) Heat map of esophageal adenocarcinoma (EAC); (D) Volcanic map of EAC.
To understand the regulatory mechanism of these immune differential genes related to prognosis, we developed a regulatory network of TF-immune gene interaction based on these differential TFs and our previously screened immune differential genes, finding that BMP4, CD14, PSME2, HLA-B, IGF2, CKLF, FABP9, IL1F10, TSLP, and OSM were high-risk immune genes while NR2F2, EGFR, and BMP1 were low-risk immune genes in ESCC (Figure 4A). CACYBP, MAPT, CST4, PSMD11, IL17A, PLAU, OXTR, FABP2, CSF2, and TNFSF18 were high-risk immune genes in EAC (Figure 4B).
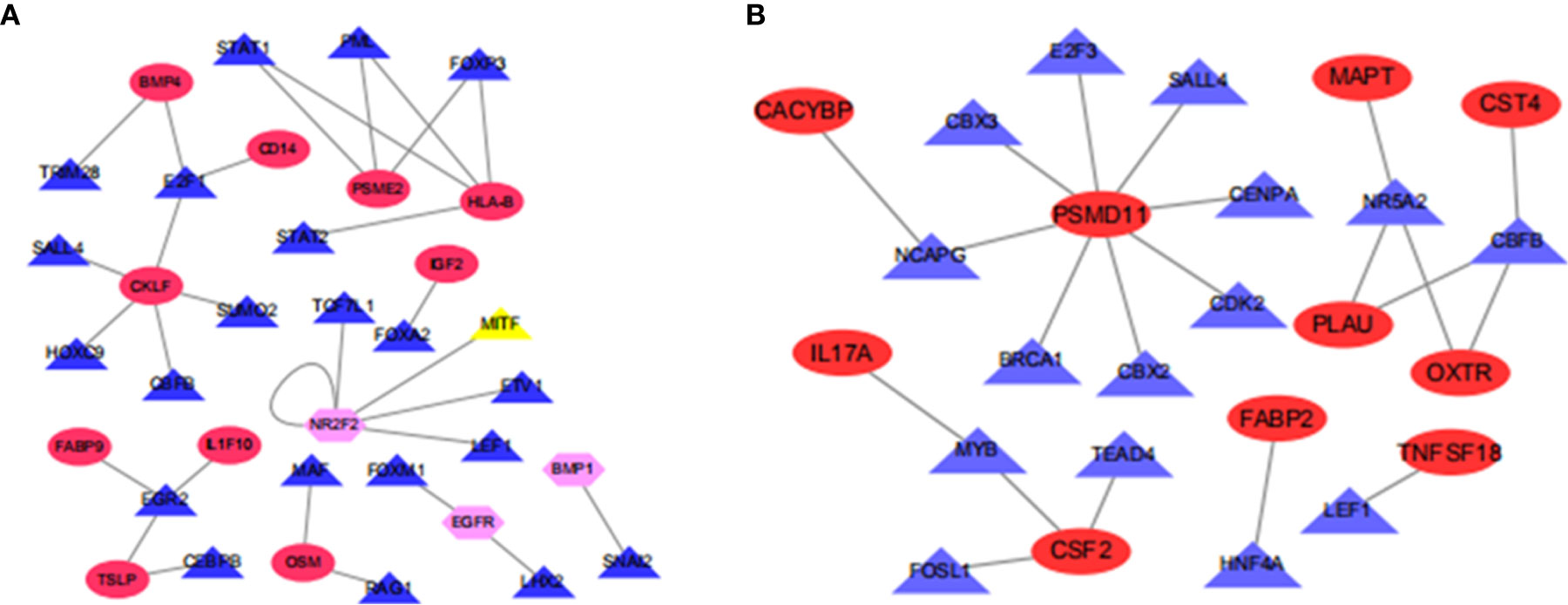
Figure 4 Map of transcriptional factors regulating the immune gene network. (A) Esophageal squamous cell carcinoma; (B) Esophageal adenocarcinoma.
Analysis of Survival-Associated Immune-Related Genes
ESCC and EAC patients with complete clinical data were randomly divided into training groups and testing groups. In training groups, we combined the above screened immune-related differentially expressed genes with the clinical information and follow-up data of the patients for survival analysis. To obtain the immune-related differential genes related to prognosis, we used univariate analysis of prognosis-related immune genes(p < 0.05). The results revealed that the 16 genes (CTSL, S100A12, SLC40A1, BMP4, FGF19, TNFSF10, CD14, PSME2, HLA-B, APLN, IGF2, CKLF, FABP9, IL1F10, TSLP, and OSM) were high-risk genes, while NR2F2, EGFR and BMP1 were low-risk genes in ESCC (Figure 5A). Besides, there were 23 high-risk genes and 1 low-risk gene in EAC (Figure 5B).
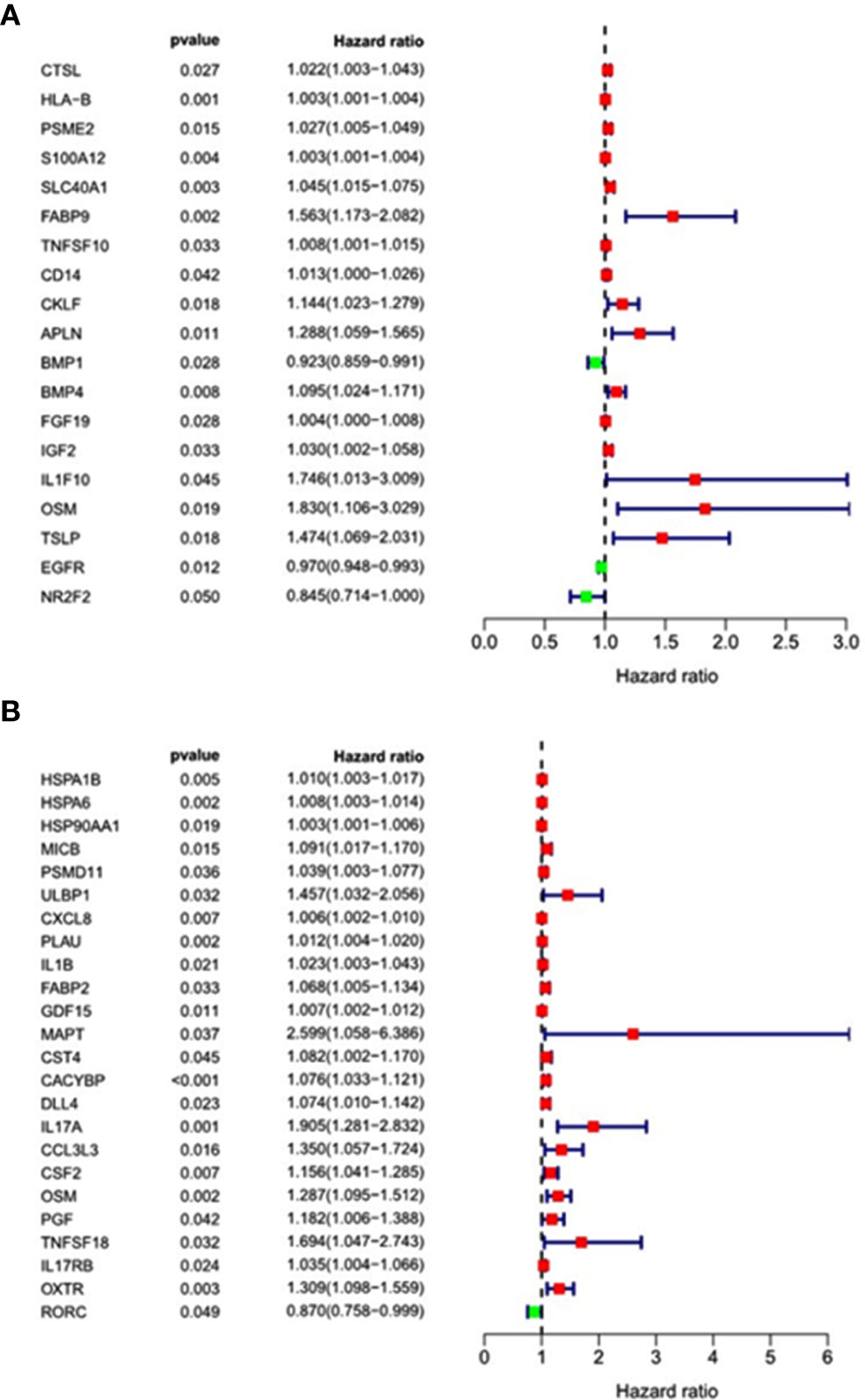
Figure 5 Forest map of univariate analysis of prognosis-related immune genes. (A) Esophageal squamous cell carcinoma; (B) Esophageal adenocarcinoma.
Development of the Immune Gene Prognosis Model in Training Group
Based on the immune differential genes related to prognosis and the survival data of patients, we used multivariate COX regression analysis to construct a prognosis model of immune-related genes. Univariate and multivariate COX regression analyses showed that 12 and 11 genes were included in our prognostic model for ESCC and EAC, respectively. The formula for calculating the risk score is as follows: Risk score of ESCC = 0.006486756 × expression level of HLA-B + 0.003511031 × expression level of S100A12 - 0.134223077 × expression level of SLC40A1 + 0.785407491 × expression level of FABP9 - 0.021149907 × expression level of CD14 + 0.238292334 × expression level of APLN - 0.226841694 × expression level of BMP1 + 0.315827065 × expression level of BMP4 + 0.00809996 × expression level of FGF19 + 0.118629975 × expression level of IGF2 + 1.008924188 × expression level of OSM - 0.01772927 × expression level of EGFR (Table 1A). Risk score of EAC = - 1.230594393 × expression level of ULBP1 + 0.060399108 × expression level of IL1B + 0.163713621 × expression level of FABP2 + 2.31747042 × expression level of MAPT + 0.139658347 × expression level of CST4 + 0.132029819 × expression level of CACYBP + 0.156437833 × expression level of DLL4 + 1.690819322 × expression level of IL17A - 0.428632286 × expression level of PGF + 0.943049137 × expression level of TNFSF18 + 0.568394224 × expression level of OXTR (Table 1B).
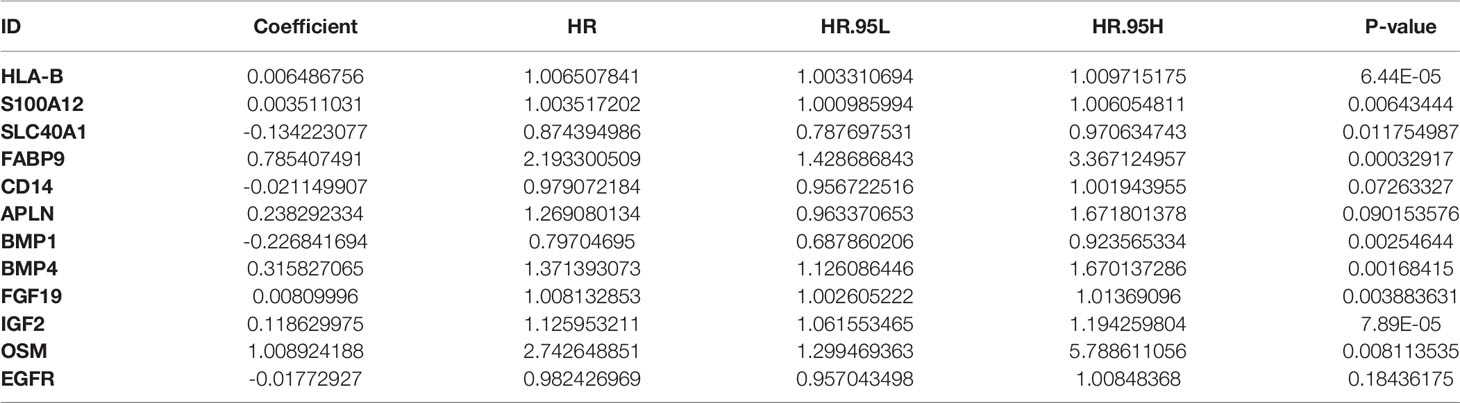
Table 1A The coefficients and HR values of the immune gene prognostic model of esophageal squamous cell carcinoma.
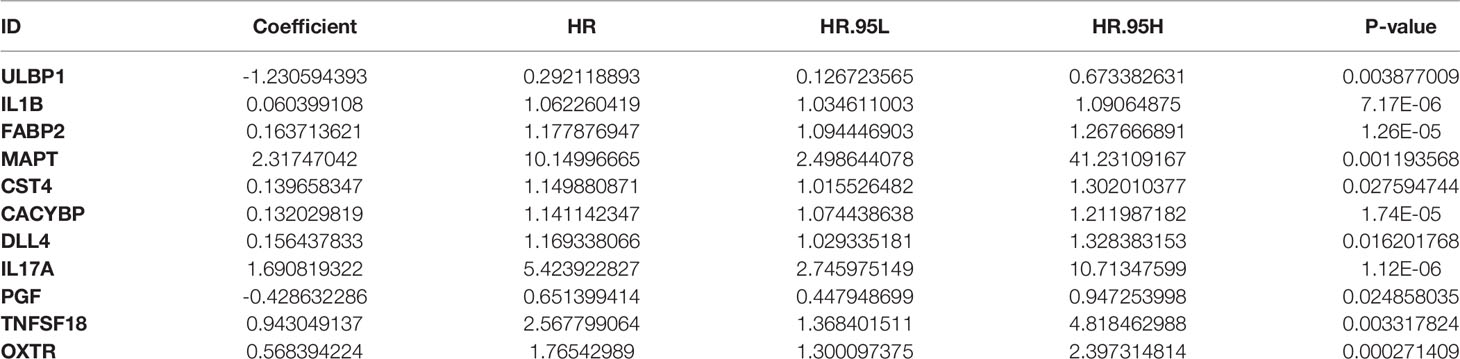
Table 1B The coefficients and HR values of the immune gene prognostic model of esophageal adenocarcinoma.
Analysis of Survival Differences Between High- and Low-Risk Patients
Taking the median as the demarcation, we divided the patients into a high-risk group and a low-risk group according to the risk score, and found that survival prognosis of the patients in the high-risk group was significantly worse than that in the low-risk group(p < 0.001)(Figures 6A and 7A). Under the ROC curves, the area was 0.835 in ESCC and 0.888 in EAC, showing high true positive rates(Figures 6D and 7D). The number of deaths in the high-risk group was significantly higher than that in the low-risk group, and the survival time was also significantly shorter than that in the low-risk group (Figures 6G and 7G).
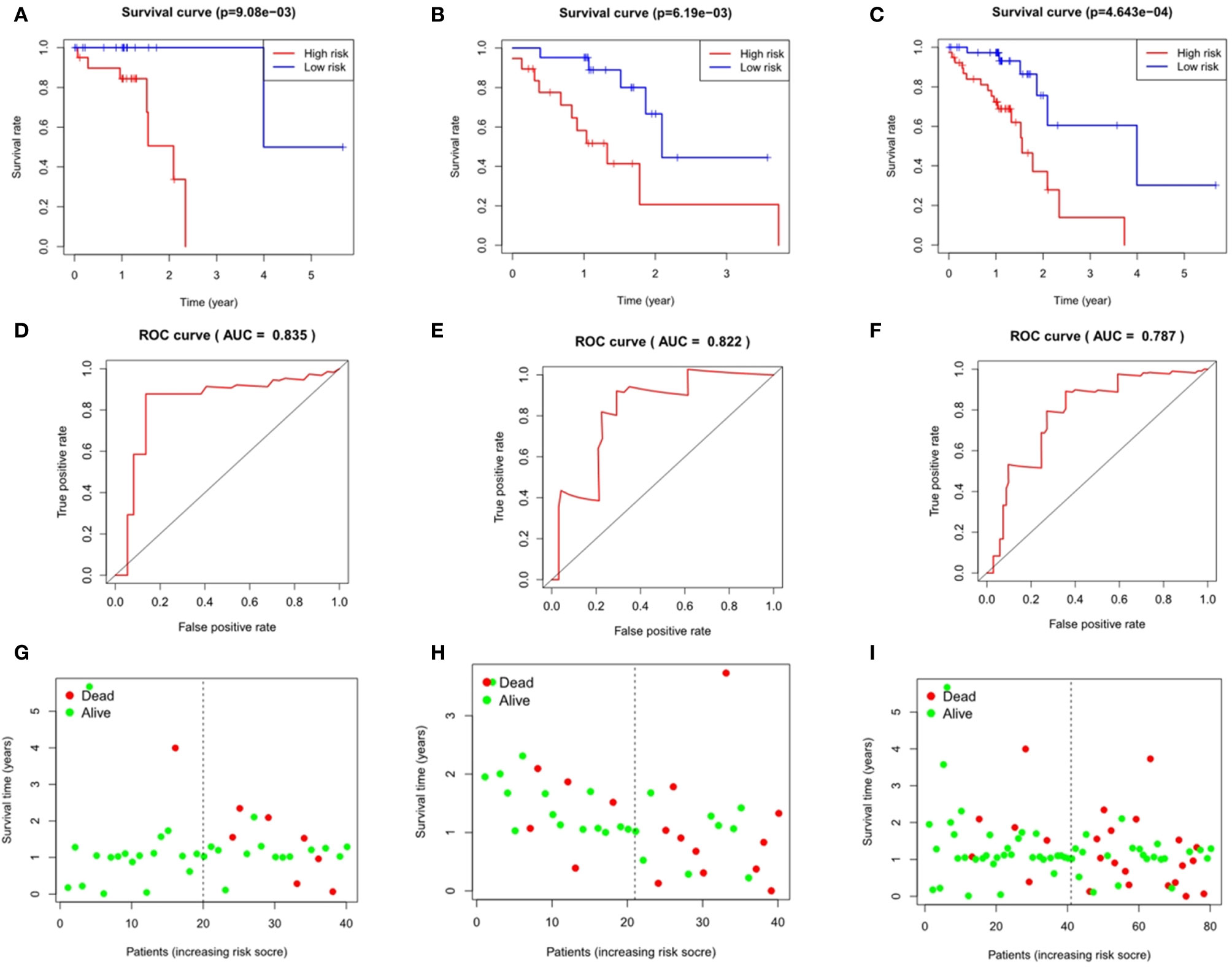
Figure 6 Evaluation and verification of the model in esophageal squamous cell carcinoma. Kaplan-Meier analysis in high and low risk groups in the train group (A), testing group (B) and entire group (C); ROC curve in the train group (D), testing group (E) and entire group (F); survival status in the train group (G), testing group (H) and entire group (I).
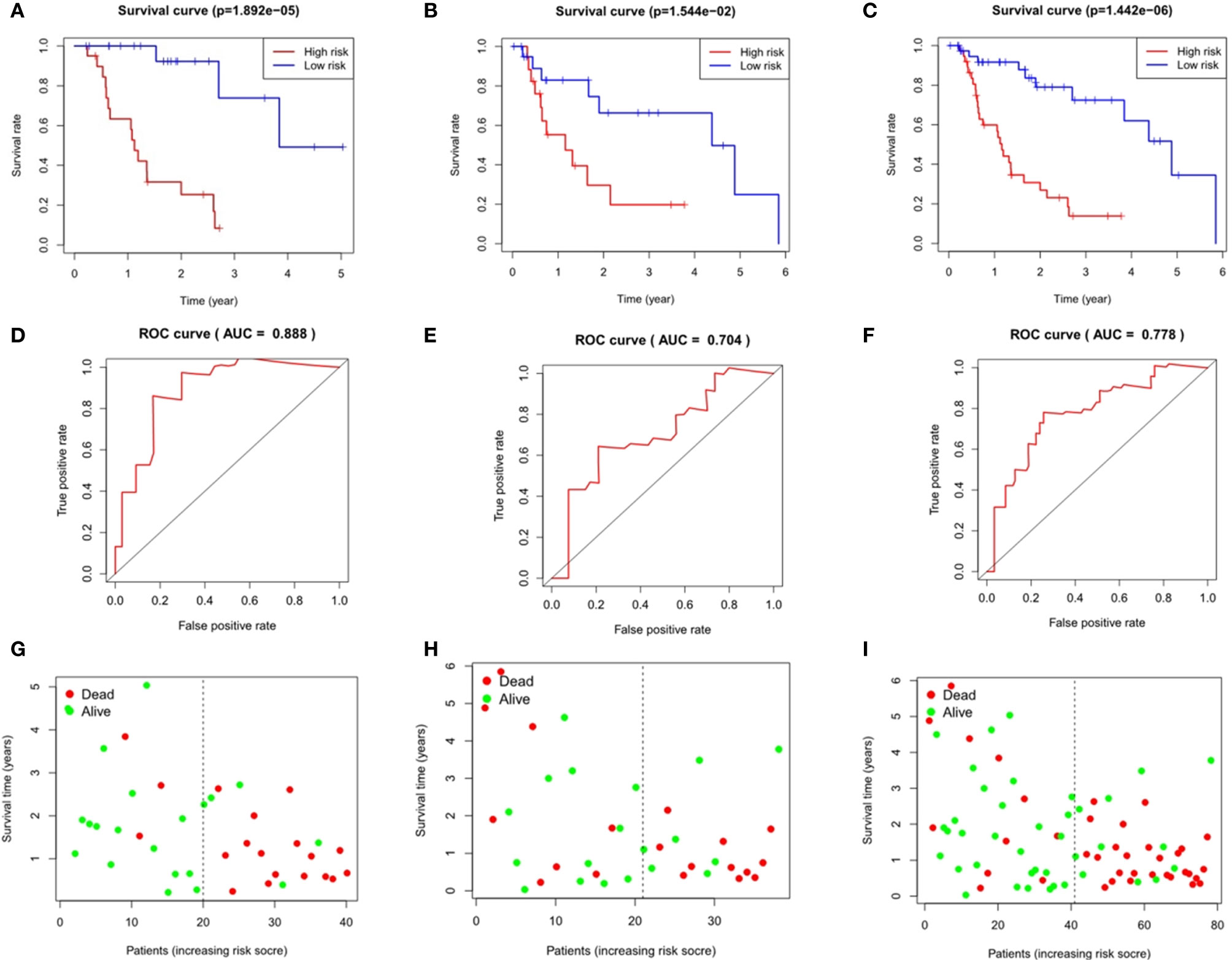
Figure 7 Evaluation and verification of the model in esophageal adenocarcinoma. Kaplan-Meier analysis in high and low risk groups in the train group (A), testing group (B) and entire group (C); ROC curve in the train group (D), testing group (E) and entire group (F); survival status in the train group (G), testing group (H), and entire group (I).
We performed multivariate Cox regression analysis to adjust gender, tumor stage, tumor size, lymph node metastasis status, distant metastasis, and other factors (Figure 8 and Table 2). The analysis chart shows that the risk score could independently predict the prognosis of both ESCC and EAC patients (p < 0.001).
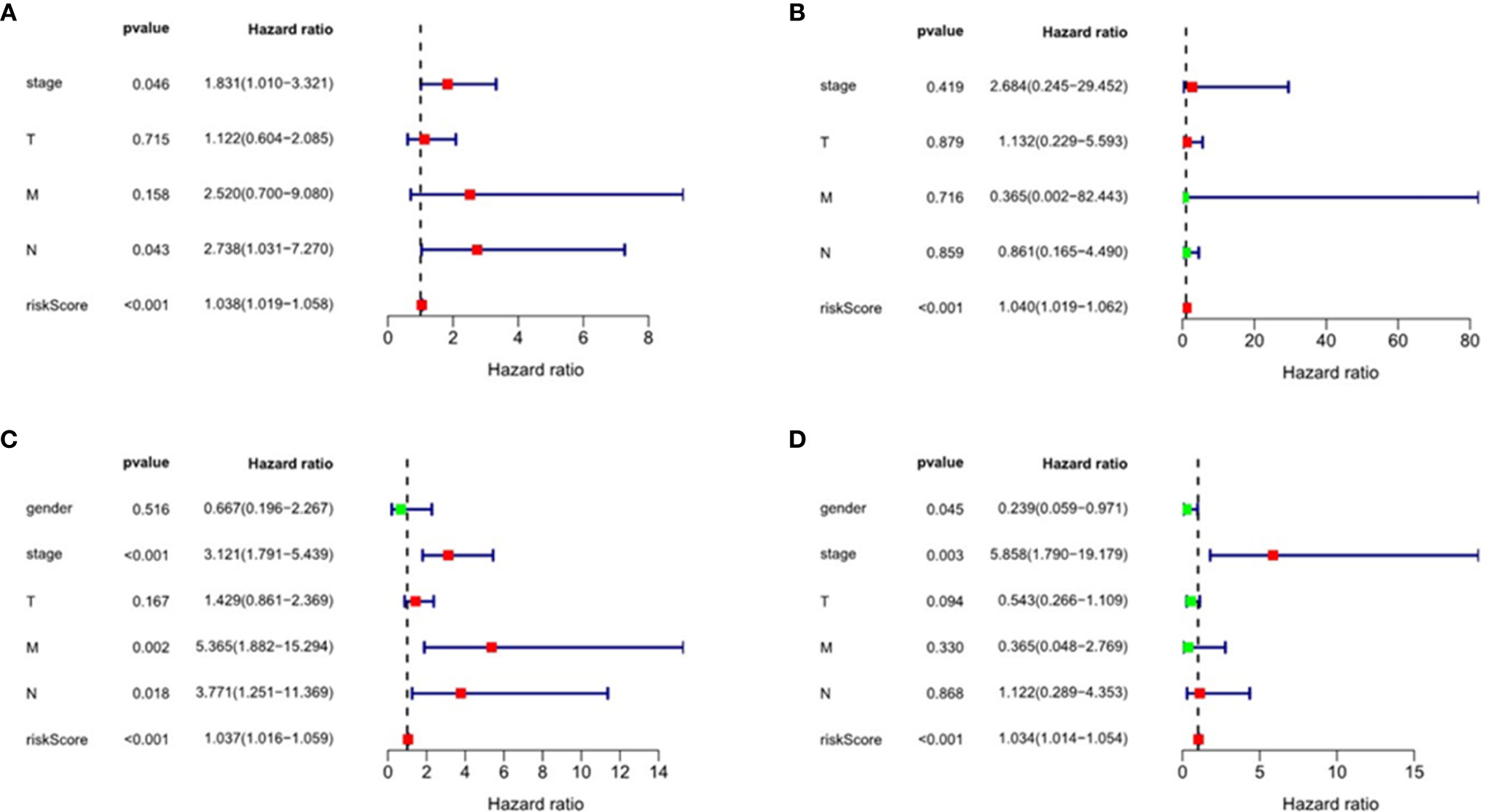
Figure 8 Univariate and multivariate regression analyses of esophageal squamous cell carcinoma(ESCC) and esophageal adenocarcinoma (EAC). Univariate regression analysis: (A) ESCC; (C) EAC. Multivariate regression analysis: (B) ESCC; (D) EAC.
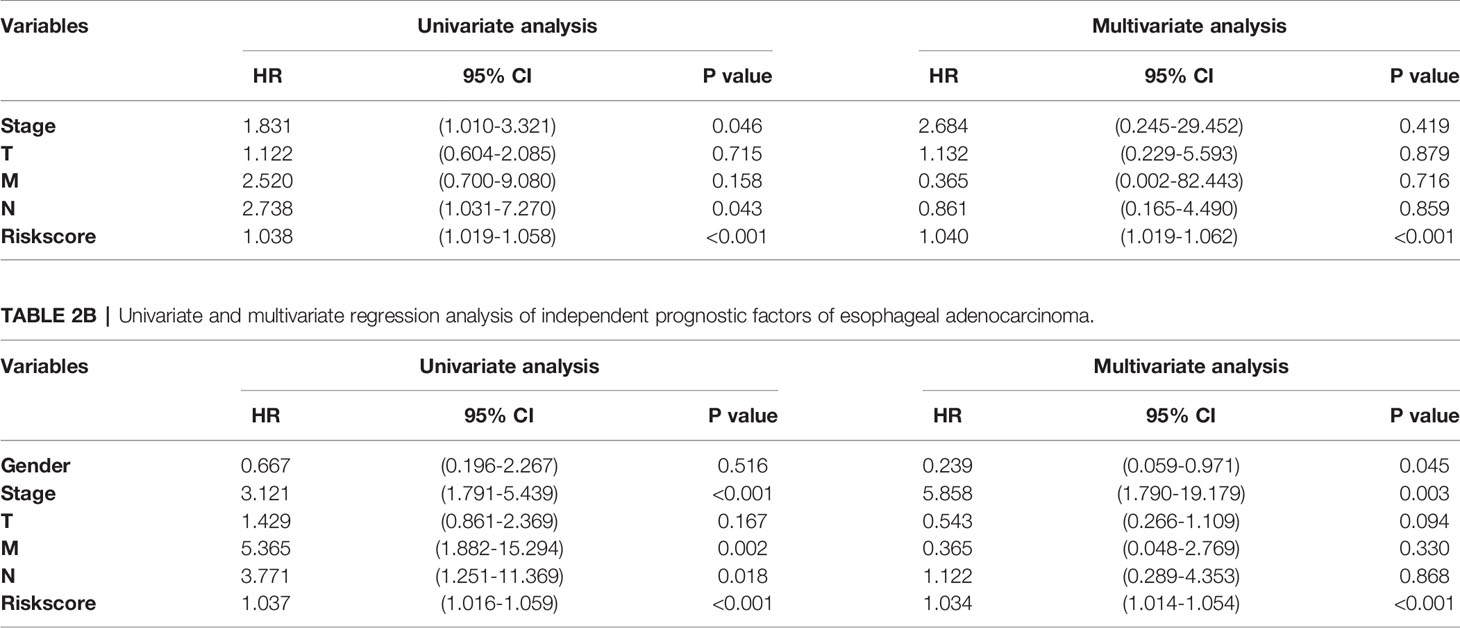
Table 2A Univariate and multivariate regression analysis of independent prognostic factors of esophageal squamous cell carcinoma.
Validation of the Immune Gene Prognosis Model in Testing Group and Entire Group
The area under the ROC curves of the testing group and the entire group of ESCC was 0.822 and 0.787 respectively (Figures 6E, F), and that of EAC was 0.704 and 0.778 respectively (Figures 7E, F), which showed good predictive ability. The Kaplan-Meier curve showed us the difference in survival rate between the high-risk group and the low-risk group. For ESCC, the results showed that the overall survival rate of the high-risk group was significantly lower than that of the low-risk group in both the testing group (p = 6.19e-03, Figure 6B) and the entire group (p = 4.643e-04, Figure 6C). In the case of EAC, we came to the same conclusion(p = 1.544e-02, Figure 7B; p = 1.442e-06, Figure 7C). Figures 6H and 7H show the survival states in the testing cohort and Figures 6I and 7I show that in the entire cohort, in ESCC and EAC, respectively.
Clinical Relevance of the Clinical Characteristics
We also explored the relationship between the risk score, immune-related differential genes and clinical characteristics of the tumors(p < 0.05) (Table 3). The results showed that the expression of immune-related differential gene MAPT, TNFSF18, and OXTR in EAC in males were higher than that in females (Figures 9G, I, J), and vice versa for the expression of IL1B (Figure 9F). The S100A12 gene expression was associated with the tumor stage in ESCC patients (Figure 9C). Its expression in stage 3-4 EC was lower than that in stage 1 and 2. In the T stage, the expression of TNFSF18 in the 3-4 stage was significantly higher than that in the 1-2 stage in EAC (Figure 9H). However, ESCC patients enriched with gene BMP1 and EGFR were often accompanied with a better M stage, devoid of distant metastasis (Figures 9A, D). The study also found a significant positive correlation between our model risk score and the T stage in EAC (Figure 9K). In terms of the survival outcome of ESCC, HLA-B was a risk gene, and its high expression indicated a poor survival outcome (Figure 9B), while the higher the expression level of EGFR, the greater the likelihood of survival (Figure 9E).
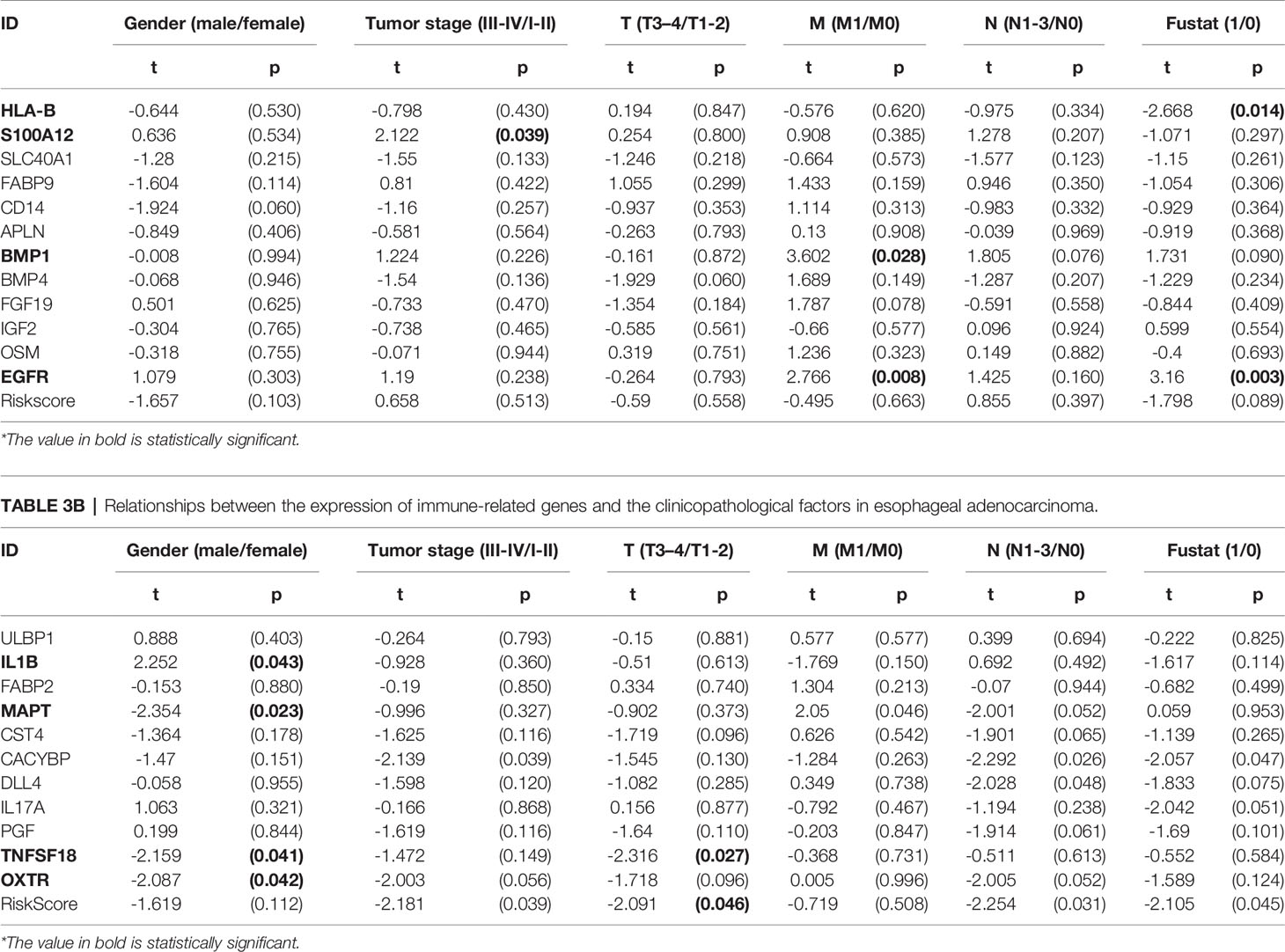
Table 3A Relationships between the expression of immune-related genes and the clinicopathological factors in esophageal squamous cell carcinoma.
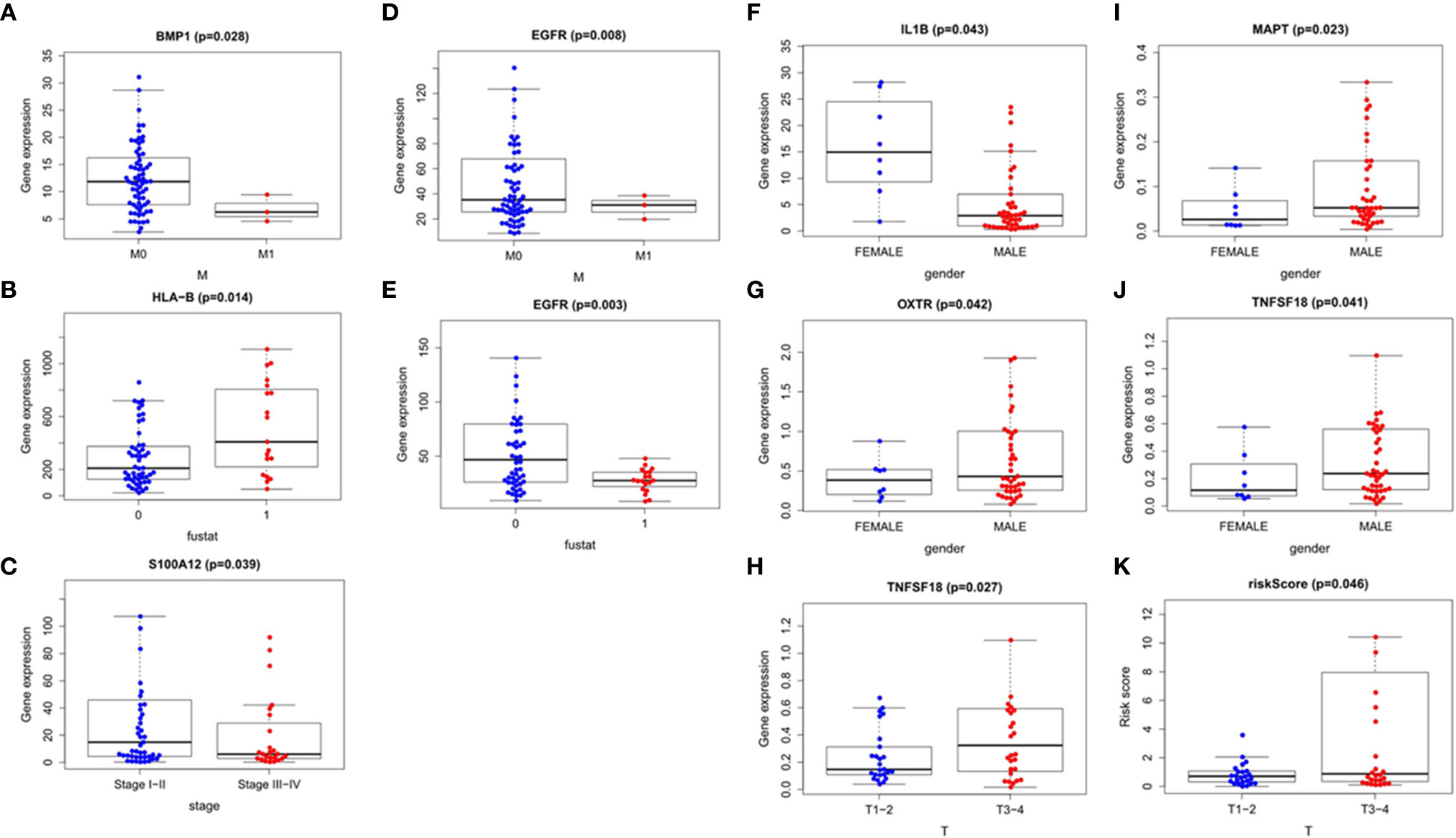
Figure 9 Correlation analysis of the clinical factors. Esophageal squamous cell carcinoma: (A–E) Esophageal adenocarcinoma: (F–K).
Evaluation of Immune Cell Infiltration and the Tumor Microenvironment
We used Timer samples to explore whether the immune genome could indicate the tumor immune microenvironment status in EC patients. We downloaded the immune infiltration level in EC patients, analyzed and visualized the correlation between prognosis-related immune genes and immune cell abundance using TIMER database. The result showed that the level of B cell infiltration was negatively correlated with the score of our immune differential gene evaluation model related to the prognosis of ESCC(p < 0.05)(Figure 10A). We found no other statistical correlation between this prognostic index and six kinds of immune cell infiltration(Figures 10B–L).
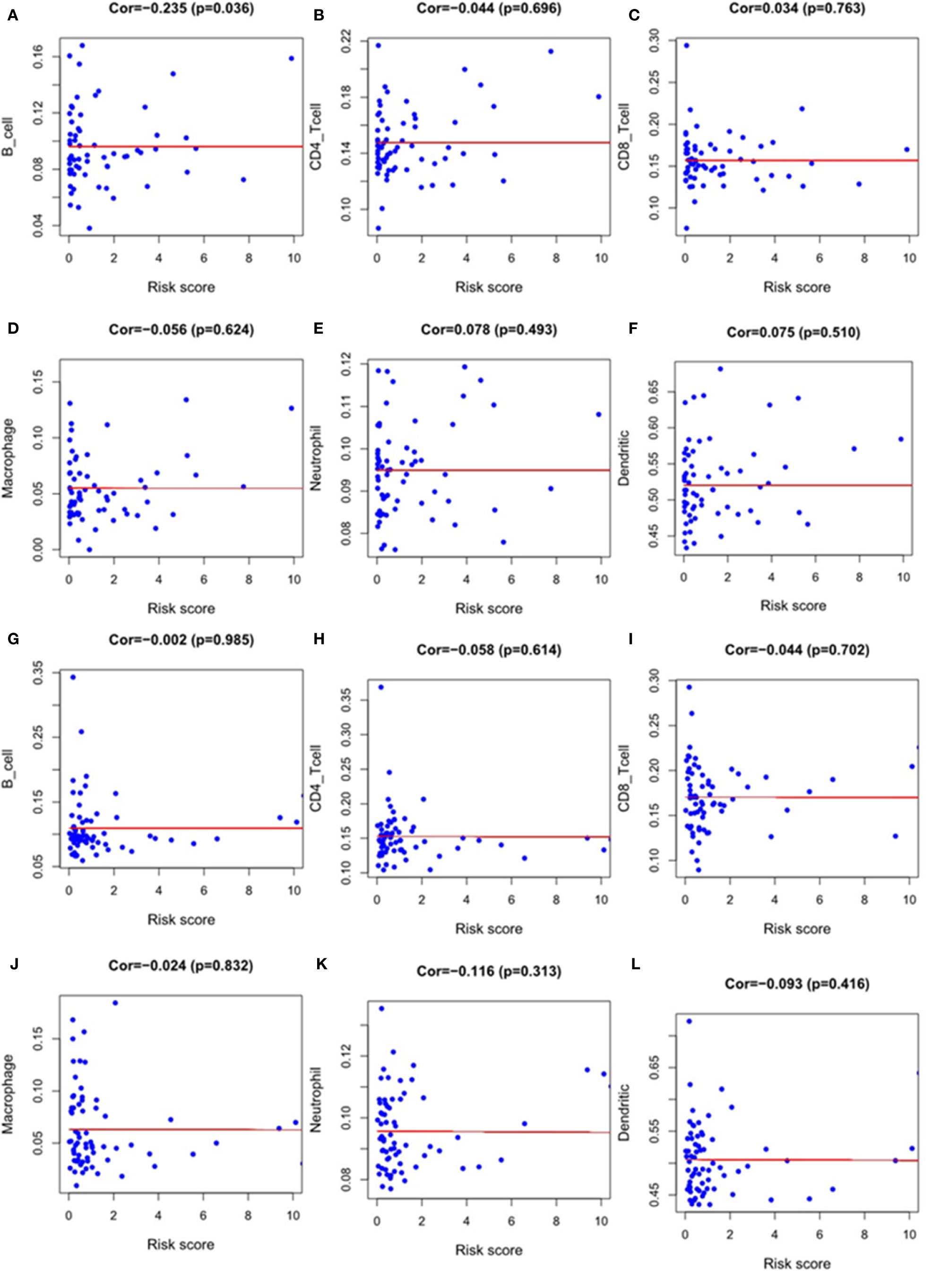
Figure 10 The relationship between the model index and the level of immune cell infiltration, including B-cell, CD4-T cell, CD8-T cell, macrophage, neutrophil and dendritic cell. Esophageal squamous cell carcinoma: (A–F); Esophageal adenocarcinoma: (G–L).
Discussion
In recent years, immunotherapy has become a research hotspot in cancer therapy. It is necessary to explore the indicators to predict the prognosis of patients on immunotherapy for the sake of screening out immunotherapy beneficiaries. So far, we found no clear consensus biomarker to predict the efficacy of immunotherapy for EC. Few studies have classified the results of immune-related genes in EC according to its histological sub-types. In this study, we analyzed the whole genomes of ESCC and EAC in the TCGA database and established the immune gene prognostic models related to the prognosis of ESCC and EAC based on respective 12 and 11 genes. In addition, we explored the clinical significance of these immune-related genes and their correlations with immune cell infiltration with a view to improve the efficacy of immunotherapy and promote the development of individualized immunotherapy for EC patients.
Genome analysis strongly suggests that ESCC and EAC are different tumor entities, and their genetic change profiles are very different (8, 30). A study on the genomic characteristics of EC found that ESCC and EAC had a set of driving genes that were almost mutually exclusive, indicating that their development was independent (31). Our univariate COX regression analysis showed that 19 and 24 differential immune genes were related to the prognosis of ESCC and EAC respectively. Among them, we mainly analyzed four genes (EGFR and BMP1 in ESCC and IL-1B and MAPT in EAC) that were related to gender, tumor stage, lymph node metastasis, distant metastasis, and other clinical features in EC, supposing that they might play essential roles in the prognosis of EC patients.
The overexpression of epidermal growth factor receptor (EGFR) in ESCC often indicates a poor prognosis (32). It was reported that an ESCC-targeted antibody could improve the radiosensitivity of recurrent ESCC with overexpression of EGFR, suggesting an effective treatment (33). In their in vitro and in vivo model study, Hoi and his team found that chemotherapy upregulated the expression of PD-L1 in ESCC by activating the EGFR/ERK pathway, suggesting that anti-PD-L1 immunotherapy combined with conventional chemotherapy could achieve a better therapeutic effect (5). Another study showed a correlation between the expression of PD-L1 and EGFR in ESCC, as well as a negative correlation between them on tumor cells or tumor-infiltrating immune cells in ESCC (34). However, few studies focused on the relationship between EGFR gene expression in EC and the prognosis of patients undergoing immunotherapy. Our study found that EGFR was an immune differential gene in patients with ESCC, and its down-regulation was associated with poor prognosis. The expression of EGFR in patients without distant metastasis was higher than that in patients with distant metastasis, and its high expression often indicated a better survival outcome. Our study identified that BMP1 was also an immune differential gene related to prognosis in ESCC, and a high expression of BMP1 tended to indicate a lower possibility of distant metastasis. It was reported that the up-regulation of BMP1 may indicate the poor prognosis of gastric cancer (35), osteosarcoma (36), renal clear cell carcinoma (37), and other tumors. Nevertheless, the study of BMP1 in EC has not been reported.
Interleukin1β (IL-1β) is considered an essential regulatory factor that promotes tumor progression, metastasis and immunosuppression (38, 39). In addition, IL could be used as a biomarker for the prognosis of non-small cell lung cancer (40). A team study on the relationship between genetic polymorphisms of IL1A and IL1B and thyroid cancer in the Chinese Han population showed that IL1Ars3783521 was a risk factor for thyroid cancer, and rs3136558 and rs1143623 in the IL1B gene suggested susceptibility to the disease in patients older than 48 years (38). Our pilot study showed that IL-1B was a risk immune-related differential gene for EAC, and its expression was generally higher in females than that in males. It was found in the present study that MAPT expression was associated with poor prognosis in EAC, and its expression in men was higher than that in women. Some studies using the TCGA database found that MAPT gene expression was closely related to survival of patients with low-grade gliomas (41). By analyzing the clinical data of patients with breast cancer, Pan et al. concluded that MAPT-AS1 may be a potential therapeutic target for ER-negative breast cancer related to tumor growth, invasion and drug resistance (42). The prognostic role of MAPT in prostate cancer and childhood blastoma were also proposed in some studies (41).
More importantly, we reported herein two prognostic index models of immune-related genes based on the expression levels and corresponding regression coefficients of specific genes in ESCC and EAC. The risk score of EC patients was calculated to provide reference for predicting the efficacy of immunotherapy in EC patients. According to the score, the patients were divided into a high-risk group and a low-risk group. The results showed that the survival prognosis of the high-risk group of ESCC and EAC was significantly worse than that of the low-risk group, and the higher the risk score, the worse the prognosis. Previous studies explored some markers to predict the efficacy of chemotherapy and radiotherapy in EC patients, but there are few biological indicators to evaluate the efficacy of immunotherapy for the EC subtypes. A study of immune-related genes in EC is similar to ours, but it does not reflect the difference of immune-related genes in different pathological types of EC. In this study, we established a prognostic score model based on nine genes (HSPA6, CACYBP, DKK1, EGF, FGF19, GAST, OSM, ANGPTL3 and NR2F2). We also analyzed the clinical associations of these genes and found that DKK1 was associated with worse T stages of EC. The expression of OSM in patients with tumor stage III and IV was reportedly higher than that in patients with stage I and II (43). Xi et al. developed a prognostic model of EC based on the histological grade, tumor location, baseline PET SUV max and lymph node size and found that it could be used to evaluate whether induction chemotherapy before neoadjuvant radiotherapy and chemotherapy could benefit the patients (44). Wang and his team established a model for predicting the postoperative survival of ESCC by using UBE2C and MGP genes, as well as the clinicopathological factors including the tumor staging and grade (45). A study on the genome of ESCC used data from two clinical centers and found that NFE2L2 may be a tumor suppressor in ESCC. However, its mutation was found to be associated with poor prognosis, and hot spot mutations in the SLC35E2 promoter region also indicated a low survival rate (46).
Besides, We also explored the relationship between our model risk score and immune cell infiltration. The tumor immune microenvironment contains a variety of immune cells including dendritic cell, natural killer cell, macrophage, T and B lymphocyte, all of which can affect the efficacy of ICIs (47). However, we only concluded that the immune differential gene risk score related to prognosis was negatively correlated with B cell infiltration in ESCC. In the study of other immune cells, no correlation was found between the infiltration level and the score of the model. A recent study used tissue microarray of ESCC and EAC to explore changes in immune cell infiltration in the two EC subtypes by the immunohistochemical method. Their results showed that CD45RO+ and CD8+ cells were highly expressed in EC, and the level of invasion in ESCC was higher than that in EAC (48). Guo et al. also created immune-related genes in the prognostic index score in EC. Unlike our results, they found no statistically significant correlation between B cell infiltration and the score, while the positive level of dendritic cell and macrophage neutrophil suggested a higher risk score (43), which is inconsistent with our results. Therefore, we analyzed their research and found that sample differences might cause this inconsistency.
Our work had some potential limitations. First, our research data came from the public databases, which may lack detailed clinical treatment plans and follow-up information in some patients. Second, our study is a retrospective analysis, and it is therefore necessary to verify the prognostic role of these immune-related genes in EC in larger-scale studies. In addition, current work lacked clear evidences that patients with specific scores could or could not benefit from immunotherapy. Finally, we failed to clarify the relationship between immunogenomics, proteomics, and metabonomics and explore the immunobiological mechanism of EC at the molecular level.
Conclusions
We identified the immune differential genes of different EC subtypes by using the public databases for genome analysis and established a model index of immune differential genes related to prognosis. The results of the present study may provide a new idea for individualized immunotherapy for EC patients.
Data Availability Statement
The datasets presented in this study can be found in online repositories. The names of the repository/repositories and accession number(s) can be found in the article/supplementary material.
Author Contributions
YZ and TX conceived and designed the study and assisted in writing the manuscript. ZF and RX performed the data analyses and contributed to the writing of the manuscript. ZC, JX, and YG reviewed the manuscript. All authors contributed to the article and approved the submitted version.
Funding
This study was supported by grant from the Natural Science Foundation of Zhejiang Province (No. LY21H280012) and the new-shoot Talents Porgram of Zhejiang province (No. 2021).
Conflict of Interest
The authors declare that the research was conducted in the absence of any commercial or financial relationships that could be construed as a potential conflict of interest.
References
1. Bray F, Ferlay J, Soerjomataram I, Siegel RL, Torre LA, Jemal A. Global cancer statistics 2018: GLOBOCAN estimates of incidence and mortality worldwide for 36 cancers in 185 countries. CA: Cancer J Clin (2018) 68(6):394–424. doi: 10.3322/caac.21492
2. Lagergren J, Smyth E, Cunningham D, Lagergren P. Oesophageal cancer. Lancet (2017) 390(10110):2383–96. doi: 10.1016/S0140-6736(17)31462-9
3. Fitzmaurice C, Abate D, Abbasi N, Abbastabar H, Abd-Allah F, Abdel-Rahman O, et al. Global, regional, and national cancer incidence, mortality, years of life lost, years lived with disability, and disability-adjusted life-years for 29 cancer groups, 1990 to 2017: a systematic analysis for the global burden of disease study. JAMA Oncol (2019) 5(12):1749–68. doi: 10.1001/jamaoncol.2019.2996
4. Siegel RL, Miller KD, Jemal A. Cancer statistics, 2020. CA: Cancer J Clin (2020) 70(1):7–30. doi: 10.3322/caac.21590
5. Ng HY, Li J, Tao L, Lam AK-Y, Chan KW, Ko JMY, et al. Chemotherapeutic treatments increase PD-L1 expression in esophageal squamous cell carcinoma through EGFR/ERK activation. Trans Oncol (2018) 11(6):1323–33. doi: 10.1016/j.tranon.2018.08.005
6. Abnet CC, Arnold M, Wei W-Q. Epidemiology of esophageal squamous cell carcinoma. Gastroenterology (2018) 154(2):360–73. doi: 10.1053/j.gastro.2017.08.023
7. Coleman HG, Xie S-H, Lagergren J. The epidemiology of esophageal adenocarcinoma. Gastroenterology (2018) 154(2):390–405. doi: 10.1053/j.gastro.2017.07.046
8. Network CGAR. Integrated genomic characterization of oesophageal carcinoma. Nature (2017) 541(7636):169–75. doi: 10.1038/nature20805
9. Baba Y, Nomoto D, Okadome K, Ishimoto T, Iwatsuki M, Miyamoto Y, et al. Tumor immune microenvironment and immune checkpoint inhibitors in esophageal squamous cell carcinoma. Cancer Sci (2020) 111(9):3132. doi: 10.1111/cas.14541
10. Kudo T, Hamamoto Y, Kato K, Ura T, Kojima T, Tsushima T, et al. Nivolumab treatment for oesophageal squamous-cell carcinoma: an open-label, multicentre, phase 2 trial. Lancet Oncol (2017) 18(5):631–9. doi: 10.1016/S1470-2045(17)30181-X
11. Kato K, Cho BC, Takahashi M, Okada M, Lin C-Y, Chin K, et al. Nivolumab versus chemotherapy in patients with advanced oesophageal squamous cell carcinoma refractory or intolerant to previous chemotherapy (ATTRACTION-3): a multicentre, randomised, open-label, phase 3 trial. Lancet Oncol (2019) 20(11):1506–17. doi: 10.1016/S1470-2045(19)30626-6
12. Doi T, Piha-Paul S, Jalal S, Saraf S, Lunceford J, Koshiji M, et al. Safety and Antitumor Activity of the Anti-Programmed Death-1 Antibody Pembrolizumab in Patients With Advanced Esophageal Carcinoma. ASCO (2018) 36(1):61–7. doi :10.1200/jco.2017.74.9846
13. Shah MA, Kojima T, Hochhauser D, Enzinger P, Raimbourg J, Hollebecque A, et al. Efficacy and safety of pembrolizumab for heavily pretreated patients with advanced, metastatic adenocarcinoma or squamous cell carcinoma of the esophagus: the phase 2 KEYNOTE-180 study. JAMA Oncol (2019) 5(4):546–50. doi: 10.1001/jamaoncol.2018.5441
14. Kojima T, Shah M, Muro K, Francois E, Adenis A, Hsu C, et al. Randomized Phase III KEYNOTE-181 Study of Pembrolizumab Versus Chemotherapy in Advanced Esophageal Cancer. Am Soc Clin Oncol (2019) 38(35):4138–48. doi: 10.1200/jco.20.01888
15. Wang X, Wang F, Zhong M, Yarden Y, Fu L. The biomarkers of hyperprogressive disease in PD-1/PD-L1 blockage therapy. Mol Cancer (2020) 19:1–15. doi: 10.1186/s12943-020-01200-x
16. Greally M, Chou JF, Chatila WK, Margolis M, Capanu M, Hechtman JF, et al. Clinical and molecular predictors of response to immune checkpoint inhibitors in patients with advanced esophagogastric cancer. Clin Cancer Res (2019) 25(20):6160–9. doi: 10.1158/1078-0432.CCR-18-3603
17. Yang H, Wang K, Wang T, Li M, Li B, Li S, et al. The Combination Options and Predictive Biomarkers of PD-1/PD-L1 Inhibitors in Esophageal Cancer. Front Oncol (2020) 10:300:300. doi: 10.3389/fonc.2020.00300
18. Li Y, Lu Z, Che Y, Wang J, Sun S, Huang J, et al. Immune signature profiling identified predictive and prognostic factors for esophageal squamous cell carcinoma. Oncoimmunology (2017) 6(11):e1356147. doi: 10.1080/2162402X.2017.1356147
19. Wang L, Wei Q, Zhang M, Chen L, Li Z, Zhou C, et al. Identification of the prognostic value of immune gene signature and infiltrating immune cells for esophageal cancer patients. Int Immunopharmacol (2020) 87:106795. doi: 10.1016/j.intimp.2020.106795
20. Zhang C, Zhang G, Sun N, Zhang Z, Xue L, Zhang Z, et al. An individualized immune signature of pretreatment biopsies predicts pathological complete response to neoadjuvant chemoradiotherapy and outcomes in patients with esophageal squamous cell carcinoma. Signal Transduct Targeted Ther (2020) 5(1):1–10. doi: 10.1038/s41392-020-00221-8
21. Han L, Gao Q, Zhou X, Shi C, Chen G, Song Y, et al. Characterization of CD103+ CD8+ tissue-resident T cells in esophageal squamous cell carcinoma: may be tumor reactive and resurrected by anti-PD-1 blockade. Cancer Immunol Immunother (2020) 69(8):1493–504. doi: 10.1007/s00262-020-02562-3
22. Qiang W, Dai Y, Sun G, Xing X, Sun X. Development of a prognostic index of colon adenocarcinoma based on immunogenomic landscape analysis. Ann Trans Med (2020) 8(6):284. doi: 10.21037/atm.2020.03.09
23. Gao X, Yang J, Chen Y. Identification of a four immune-related genes signature based on an immunogenomic landscape analysis of clear cell renal cell carcinoma. J Cell Physiol (2020) 235(12):9834–50. doi: 10.1002/jcp.29796
24. Xiao H, Wang B, Xiong H, Guan J, Wang J, Tan T, et al. A novel prognostic index of hepatocellular carcinoma based on immunogenomic landscape analysis. J Cell Physiol (2020) 236(4):2572–91. doi: 10.1002/jcp.30015
25. Lin P, Guo Y, Shi L, Li X, Yang H, He Y, et al. Development of a prognostic index based on an immunogenomic landscape analysis of papillary thyroid cancer. Aging (2019) 11(2):480–500. doi: 10.18632/aging.101754
26. Lin K, Huang J, Luo H, Luo C, Zhu X, Bu F, et al. Development of a prognostic index and screening of potential biomarkers based on immunogenomic landscape analysis of colorectal cancer. Aging (2020) 12(7):5832–57. doi: 10.18632/aging.102979
27. Zhao Y, Pu C, Liu Z. Exploration the Significance of a Novel Immune-Related Gene Signature in Prognosis and Immune Microenvironment of Breast Cancer. Front Oncol (2020) 10:1211:1211. doi: 10.3389/fonc.2020.01211
28. Gu H, Lin L, Zhang C, Yang M, Zhong H, Wei R. The Potential of Five Immune-Related Prognostic Genes to Predict Survival and Response to Immune Checkpoint Inhibitors for Soft Tissue Sarcomas Based on Multi-Omic Study. Front Oncol (2020) 10:1317:1317. doi: 10.3389/fonc.2020.01317
29. Wu M, Li X, Zhang T, Liu Z, Zhao Y. Identification of a Nine-Gene Signature and Establishment of a Prognostic Nomogram Predicting Overall Survival of Pancreatic Cancer. Front Oncol (2019) 9:996:996. doi: 10.3389/fonc.2019.00996
30. Wang K, Johnson A, Ali SM, Klempner SJ, Bekaii-Saab T, Vacirca JL, et al. Comprehensive genomic profiling of advanced esophageal squamous cell carcinomas and esophageal adenocarcinomas reveals similarities and differences. Oncol (2015) 20(10):1132. doi: 10.1634/theoncologist.2015-0156
31. Lin D-C, Dinh HQ, Xie J-J, Mayakonda A, Silva TC, Jiang Y-Y, et al. Identification of distinct mutational patterns and new driver genes in oesophageal squamous cell carcinomas and adenocarcinomas. Gut (2018) 67(10):1769–79. doi: 10.1136/gutjnl-2017-314607
32. Kashyap MK, Abdel-Rahman O. Expression, regulation and targeting of receptor tyrosine kinases in esophageal squamous cell carcinoma. Mol Cancer (2018) 17(1):54. doi: 10.1186/s12943-018-0790-4
33. Yu Y, Guan H, Jiang L, Li X, Xing L, Sun X. Nimotuzumab, an EGFR−targeted antibody, promotes radiosensitivity of recurrent esophageal squamous cell carcinoma. Int J Oncol (2020) 56(4):945–56. doi: 10.3892/ijo.2020.4981
34. Zhang W, Pang Q, Zhang X, Yan C, Wang Q, Yang J, et al. Programmed death-ligand 1 is prognostic factor in esophageal squamous cell carcinoma and is associated with epidermal growth factor receptor. Cancer Sci (2017) 108(4):590–7. doi: 10.1111/cas.13197
35. Hsieh Y-Y, Tung S-Y, Pan H-Y, Yen C-W, Xu H-W, Deng Y-F, et al. Upregulation of bone morphogenetic protein 1 is associated with poor prognosis of late-stage gastric Cancer patients. BMC Cancer (2018) 18(1):508. doi: 10.1186/s12885-018-4383-9
36. Garimella R, Tadikonda P, Tawfik O, Gunewardena S, Rowe P, Van Veldhuizen P. Vitamin D impacts the expression of Runx2 target genes and modulates inflammation, oxidative stress and membrane vesicle biogenesis gene networks in 143B osteosarcoma cells. Int J Mol Sci (2017) 18(3):642. doi: 10.3390/ijms18030642
37. Xiao W, Wang X, Wang T, Xing J. Overexpression of BMP1 reflects poor prognosis in clear cell renal cell carcinoma. Cancer Gene Ther (2019) 27(5):330–40. doi: 10.1038/s41417-019-0107-9
38. Li H, Duan N, Zhang Q, Shao Y. IL1A & IL1B genetic polymorphisms are risk factors for thyroid cancer in a Chinese Han population. Int Immunopharmacol (2019) 76:105869. doi: 10.1016/j.intimp.2019.105869
39. Kaplanov I, Carmi Y, Kornetsky R, Shemesh A, Shurin GV, Shurin MR, et al. Blocking IL-1β reverses the immunosuppression in mouse breast cancer and synergizes with anti–PD-1 for tumor abrogation. Proc Natl Acad Sci (2019) 116(4):1361–9. doi: 10.1073/pnas.1812266115
40. Pérez-Ramírez C, Cañadas-Garre M, Alnatsha A, Molina MÁ, Robles AI, Villar E, et al. Interleukins as new prognostic genetic biomarkers in non-small cell lung cancer. Surg Oncol (2017) 26(3):278–85. doi: 10.1016/j.suronc.2017.05.004
41. Zaman S, Chobrutskiy BI, Sikaria D, Blanck G. MAPT (Tau) expression is a biomarker for an increased rate of survival for low−grade glioma. Oncol Rep (2019) 41(2):1359–66. doi: 10.3892/or.2018.6896
42. Pan Y, Pan Y, Cheng Y, Yang F, Yao Z, Wang O. Knockdown of LncRNA MAPT-AS1 inhibites proliferation and migration and sensitizes cancer cells to paclitaxel by regulating MAPT expression in ER-negative breast cancers. Cell Biosci (2018) 8(1):7. doi: 10.1186/s13578-018-0207-5
43. Guo X, Wang Y, Zhang H, Qin C, Cheng A, Liu J, et al. Identification of the Prognostic Value of Immune-Related Genes in Esophageal Cancer. Front Genet (2020) 11:989:989. doi: 10.3389/fgene.2020.00989
44. Xi M, Liao Z, Deng W, Xu C, Komaki R, Blum M, et al. A prognostic scoring model for the utility of induction chemotherapy prior to neoadjuvant chemoradiotherapy in esophageal cancer. J Thoracic Oncol (2017) 12(6):1001–10. doi: 10.1016/j.jtho.2017.03.017
45. Wang W, Wang Z, Zhao J, Wei M, Zhu X, He Q, et al. A novel molecular and clinical staging model to predict survival for patients with esophageal squamous cell carcinoma. Oncotarget (2016) 7(39):63526. doi: 10.18632/oncotarget.11362
46. Cui Y, Chen H, Xi R, Cui H, Zhao Y, Xu E, et al. Whole-genome sequencing of 508 patients identifies key molecular features associated with poor prognosis in esophageal squamous cell carcinoma. Cell Res (2020) 30(10):902-13. doi: 10.1038/s41422-020-0333-6
47. Wang SH, Lin YS, Xiong X, Wang L, Guo Y, Chen YP, et al. Low-Dose Metformin Reprograms the Tumor Immune Microenvironment in Human Esophageal Cancer: Results of a Phase II Clinical Trial. Clin Cancer Res (2020) 26(18):4921–32. doi: 10.1158/1078-0432.Ccr-20-0113
Keywords: esophageal carcinoma, histological subtype, immune gene, prognostic model index, risk score, bioinformatics, immunotherapy
Citation: Fei Z, Xie R, Chen Z, Xie J, Gu Y, Zhou Y and Xu T (2021) Establishment of a Novel Risk Score System of Immune Genes Associated With Prognosis in Esophageal Carcinoma. Front. Oncol. 11:625271. doi: 10.3389/fonc.2021.625271
Received: 02 November 2020; Accepted: 15 March 2021;
Published: 30 March 2021.
Edited by:
Zhengfei Zhu, Fudan University, ChinaReviewed by:
Qiongyu Hao, Charles R. Drew University of Medicine and Science, United StatesMantang Qiu, Peking University People’s Hospital, China
Copyright © 2021 Fei, Xie, Chen, Xie, Gu, Zhou and Xu. This is an open-access article distributed under the terms of the Creative Commons Attribution License (CC BY). The use, distribution or reproduction in other forums is permitted, provided the original author(s) and the copyright owner(s) are credited and that the original publication in this journal is cited, in accordance with accepted academic practice. No use, distribution or reproduction is permitted which does not comply with these terms.
*Correspondence: Yue Zhou, Y2hpcnVyZ2Vvbnpob3V5dWVAMTYzLmNvbQ==; Tongpeng Xu, dG9uZ3BlbmdfeHVfbmptdUAxNjMuY29t
†These authors have contributed equally to this work