- 1Department of Radiology, The Second Affiliated Hospital, Zhejiang University School of Medicine, Hangzhou, China
- 2Department of Radiology, Jiaxing Hospital of Traditional Chinese Medicine, Jiaxing, China
- 3Department of Radiology, Sir Run Run Shaw Hospital, Zhejiang University School of Medicine, Hangzhou, China
- 4Department of Radiology, The Second Affiliated Hospital, Zhejiang Chinese Medical University, Hangzhou, China
Background: Xanthogranulomatous cholecystitis (XGC) is a rare benign chronic inflammatory disease of the gallbladder that is sometimes indistinguishable from gallbladder cancer (GBC), thereby affecting the decision of the choice of treatment. Thus, this study aimed to analyse the radiological characteristics of XGC and GBC to establish a diagnostic prediction model for differential diagnosis and clinical decision-making.
Methods: We investigated radiological characteristics confirmed by the RandomForest and Logistic regression to establish computed tomography (CT), magnetic resonance imaging (MRI), CT/MRI models and diagnostic prediction model, and performed receiver operating characteristic curve (ROC) analysis to prove the effectiveness of the diagnostic prediction model.
Results: Based on the optimal features confirmed by the RandomForest method, the mean area under the curve (AUC) of the ROC of the CT and MRI models was 0.817 (mean accuracy = 0.837) and 0.839 (mean accuracy = 0.842), respectively, whereas the CT/MRI model had a considerable predictive performance with the mean AUC of 0.897 (mean accuracy = 0.906). The diagnostic prediction model established for the convenience of clinical application was similar to the CT/MRI model with the mean AUC and accuracy of 0.888 and 0.898, respectively, indicating a preferable diagnostic efficiency in distinguishing XGC from GBC.
Conclusions: The diagnostic prediction model showed good diagnostic accuracy for the preoperative discrimination of XGC and GBC, which might aid in clinical decision-making.
Introduction
Xanthogranulomatous cholecystitis (XGC) is a rare and chronic inflammatory lesion of the gallbladder characterised by special types of granulomas and foamy cells (1). Epidemiological data from previous studies showed that the incidence of XGC was 1.3%–1.9% and that in India was 8.8%, with little difference in sex among regions (2). Goodman and Ishak described XGC as a special pathological entity in 1981 (3). Although XGC is benign, it is locally aggressive and may affect nearby organs such as the liver, duodenum, colon, and common bile duct (4). It may be misdiagnosed as gallbladder cancer (GBC) or may coexist with GBC because the inflammatory process involved resembles that of GBC in a macroscopic view.
The clinical manifestations and biological examination of XGC are nonspecific and similar to acute or chronic cholecystitis (5). Several studies have reported some imaging features that highly suggest XGC, including diffuse thickening of the gallbladder wall, continuous mucosal lines, intramural hypoattenuating nodules, and the presence of gallstones in the background of chronic gallbladder disease (6, 7). Approaches based on image analysis to guide the most appropriate treatment decisions have been intensively studied (8), and the applications of artificial intelligence (9), machine learning (10), and deep learning (11) in radiology images have been increasingly explored. Imaging tools such as computed tomography (CT) and magnetic resonance imaging (MRI) are generally utilised for clinical evaluation of gallbladder diseases, so analysis of their unique radiological features through machine learning may be a better method to distinguish XGC from GBC (12).
Laparoscopic cholecystectomy is the current gold standard for the treatment of benign gallbladder diseases. However, the high conversion rate of laparoscopic cholecystectomy to open cholecystectomy in XGC is related to the difficulty in distinguishing GBC (13). Moreover, surgeons may perform improper surgery owing to the misdiagnosis of GBC (14). In this study, we retrospectively analysed 60 cases of XGC and 93 cases of GBC confirmed by histopathology to establish a diagnostic prediction model for XGC and GBC based on CT and MRI features to help clinical decision-making on the appropriate treatment method for patients with XGC.
Methods
Patients
The flow chart of patient screening is presented in Figure 1. Patients with an inflammatory lesion originating in the gallbladder from the Second Affiliated Hospital of Zhejiang University School of Medicine from 2011 to 2021 and pathologically confirmed as XGC or GBC were included in the study population. These patients had satisfactory CT and MRI data and did not receive chemotherapy or radiotherapy. However, owing to limited data and poor imaging quality, some patients were excluded. The final study cohort comprised 60 patients with XGC and 93 patients with GBC. Our institutional review board approved this retrospective study and waived the requirement for patient consent.
Image Acquisition
Multidetector-row CT (SOMATOM Definition Flash; Siemens Healthcare) was used to perform abdominal CT examinations. All patients underwent a plain scan under the fasting state initially and then received an injection of 100-mL contrast agent at 3-4 mL/s into the antecubital vein for enhanced scan. The arterial, portal vein, and equilibrium phases were obtained at 25, 60, and 100 s after the injection of the contrast agent.
A 3.0-T magnet (Signa or Discovery 750; GE Healthcare) with an eight-channel torso-phased array coil was used to obtain axial T1-weighted images (T1WI), T2-weighted images (T2WI), contrast-enhanced images and diffusion-weighted imaging (DWI) of all patients. Dynamic breath-hold imaging acquisitions were performed at 25–32, 45–52, 75–82, and 135–142 s after contrast enhancement to obtain the arterial, portal venous, equilibrium, and delayed phases. The imaging parameters of MRI were as follows: repetition time/echo time (TR/TE) of 205/3.2msec (or 3.9/1.8msec) and 6000/102.5msec (or 12000/86.3msec); the matrix of 256x256, the standard field of view of 4mm; the slice thickness of 4mm with no interslice gap. The parameters of DWI were as follows: TR/TE=1300/60.6msec or 6000/52.5 mec; water selective excitation for fat suppression; 5 mm thickness; matrix size of 128x128; field of view of 36x36cm; the number of shots of 6; slice thickness/gap of 5 mm/1.0 mm; 20 axial slices; scan time of 144s; b value of 0 and 600 s/mm2.
Image Analysis
The original images were evaluated separately by two experienced abdominal radiologists who were blinded to patients’ pathological results. The variables of CT imaging were as follows: gallstones (no, gallbladder stones, intrahepatic bile duct stones, and extrahepatic bile duct stones), bile duct dilation (no, intrahepatic bile duct, extrahepatic bile duct, and intra and extrahepatic bile ducts), gallbladder cavity (normal, atrophy, and dilation), gallbladder morphology (regular and irregular), gallbladder wall thickness (≤10 and ≥10 mm), gallbladder wall thickening pattern (focal and diffuse), CT strengthening method (persistent, delayed, and other), intramural nodules (no, focal ≤20%, and diffuse >20%), intramural fat, mucosal lines (continuous, partly continuous, and disrupted), abnormal enhancement of adjacent liver parenchyma, liver/gallbladder interface (clear and blurred), fat around the gallbladder (clear and blurred), liver involvement, involvement of adjacent tissues outside the liver, peripheral lymph nodes (no, ≤10 mm, and >10 mm).
The variables of MRI were as follows: T1WI signal of the thickened cyst wall (high, equal, low, and mix), T2WI signal of the thickened cyst wall (high, equal, low, and mixed), MRI strengthening method (persistent, delayed, and other), T1WI signal of intramural nodules (no, high, equal, and low), T1WI signal of intramural nodules (no, high, equal, and low), lipid signal, bile signal, DWI (high, slightly high, and equal), apparent diffusion coefficient (ADC) (equal and low), and mucosal enhancement (no, obvious, and mild).
Model Construction and Validation
The general technical workflow is presented in Figure 1. CT, MRI, and CT/MRI RandomForest (RF) classifiers were built based on the extracted CT and MRI image features. The “randomForest” package of R software (ver. 3.6.3; R Foundation for Statistical Computing, Vienna, Austria) was used to run the RF classifiers. Ten-fold cross-validation was performed to adjust the parameters of the classifiers and to determine the best diagnostic model during the training process. Univariate analysis was performed to evaluate the selected radiological features and their association with the above model. Finally, the most predictive parameters were used to construct the optimal diagnosis model using the RF method for distinguishing XGC from GBC.
Statistical Analysis
SPSS ver. 26.0 (IBM Corp., Armonk, NY, USA), and R software (ver. 3.6.3; R Foundation for Statistical Computing, Vienna, Austria) were used to process all data. Continuous variables are presented as median with standard deviation and categorical variables as the number with a percentage. The same variables between the two groups were compared using the Mann–Whitney U-test for continuous non-normally distributed variables and chi-square or Fisher’s exact test for categorical variables. Variables that were statistically significant in the univariate analysis were obtained using ridge regression analysis to minimise multicollinearity, followed by the Logistic regression model. The models constructed using RF were validated using 10-fold cross-validation. Statistical significance was defined with a two-sided p-value of <0.05.
Results
Imaging Features
A comparison of CT and MRI features is summarised in Table 1. There were significant differences in the features of gallstones, bile duct dilation, gallbladder cavity, gallbladder morphology, gallbladder wall thickening pattern, strengthening method, intramural nodules, mucosal lines, abnormal enhancement of adjacent liver parenchyma, liver/gallbladder interface, fat around the gallbladder, liver involvement, and peripheral lymph nodes and T2WI signal of the thickened cyst wall, T1WI signal of intramural nodules, T2WI signal of intramural nodules, lipid signal, DWI, ADC, and mucosal enhancement.
RF Model
Based on the characteristics of the collected CT and MRI scans, the RF method was performed to establish three differential diagnosis models of XGC and GBC. In the training process, 10-fold cross-validation was performed 10 times to adjust the parameters of the classifier. Performance evaluation was conducted using the mean AUC. The AUCs of the CT and MRI models were 0.817 and 0.839, respectively, with corresponding accuracies of 0.837 and 0.842. The CT/MRI model showed good discrimination between XGC and GBC with an AUC of 0.897. Additionally, the CT/MRI model showed a high diagnostic performance with an accuracy of 0.906 (Figures 2A, B), thereby confirming the diagnostic importance of radiological features for the recognition of XGC. Furthermore, the Gini index can be used to judge the importance of different variables in the RF model (Figure 2C).
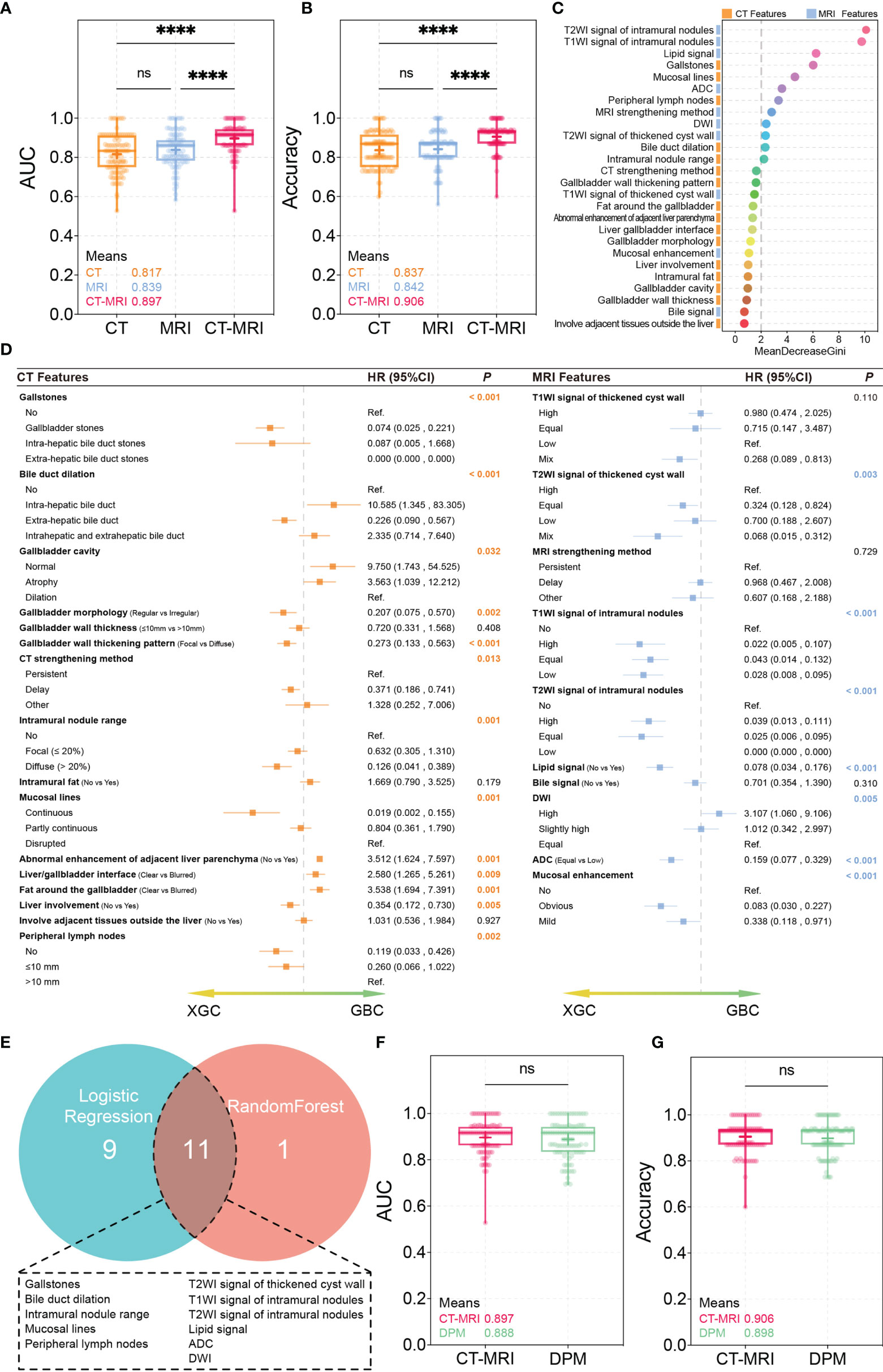
Figure 2 RandomForest and Logistic regression test results. (A, B) AUC and accuracy of the CT, MRI, CT/MRI models. ****P < 0.0001; ns, not significant, Mann-Whitney U Test. (C) The importance of variables measured using the CT/MRI model with the Random Forest Gini Index. (D) Logistic regression for univariate analysis. (E) Correlation of variables obtained using random forest and logistic regression. (F, G) AUC and accuracy of the CT/MRI model and diagnosis prediction model. ns, not significant, Mann-Whitney U Test.
Diagnostic Predictive Model
A total of 20 imaging features showed statistical differences in the univariate analysis through Logistic regression (Figure 2D). To lapply the above diagnostic system conveniently in practice, important imaging features extracted by the two machine learning methods were integrated to further build a new differential diagnosis model. A total of 11 imaging features were retained, including those of gallstones, bile duct dilation, intramural nodules, mucosal lines, and peripheral lymph nodes and T2WI signal of the thickened cyst wall, T1WI signal of intramural nodules, T2WI signal of intramural nodules, lipid signal, ADC, and DWI (Figure 2E).
Subsequently, we constructed a diagnostic predictive model using these 11 parameters using the RF method. Ten times of 10-fold cross-validation was performed to evaluate the reliability and reproducibility of the diagnostic predictive model. Finally, the average AUC and accuracy of the diagnostic prediction model were 0.888 and 0.898, respectively, which are similar to those of the primary CT/MRI model (Figures 2F, G). Interestingly, the comparison of ROCs verified using the Mann–Whitney U-test showed no statistical difference between the two models, indicating that the diagnostic predictive model made full use of the value of the CT/MRI model. These features were ranked according to their relevance to the differential diagnosis as follows: T2WI signal of intramural nodules, T1WI signal of intramural nodules, lipid signal, gallstones, mucosal lines, ADC, peripheral lymph nodes, DWI, T2WI signal of the thickened cyst wall, bile duct dilation, and intramural nodules.
Discussion
XGC was originally considered a malignant disease process; however, many studies have now confirmed its benign course (15), which is characterised by a focal or diffuse destructive inflammatory process, followed by significant macrophage and foam cell infiltrations and hyperplastic fibrosis. Concerning the pathogenesis of XGC, the most widely accepted theory is that the extravasation of bile through the ruptured Rokitansky-Aschoff sinus or increased internal pressure of the biliary tract causes small mucosal ulcers (16). Extensive inflammatory fibrosis results in the thickening of the gallbladder wall and the formation of multiple yellowish-brown nodules (17). The inflammatory process often spreads to adjacent organs, thereby forming a tumour-like mass around the gallbladder (18). Since XGC is often misdiagnosed as GBC, which leads to unnecessary radical surgical excision, it is important to distinguish between XGC and GBC before surgery.
Although ultrasound is a clinically preferred and ubiquitous imaging method, its specificity for XGC is low (12). Clinically, CT and MRI are widely used to distinguish between XGC and GBC because of their convenience and non-invasiveness (19). We reviewed a large amount of literature in the early stages and incorporated the reported potential radiological features that may distinguish XGC from GBC into the initial model. Using the RF algorithm and Logistic regression method, it was shown that the diagnostic prediction model had a stronger ability to distinguish between XGC and GBC with an AUC of 0.888 and an accuracy of 0.898. This model, constructed with outstanding clinical applicability, summarised 11 radiological features and had high diagnostic performance, thereby confirming the importance of imaging features in the differential diagnosis of XGC and GBC.
CT findings included gallstones, mucosal lines, peripheral lymph nodes, bile duct dilation, and intramural nodules. Studies have shown that the development of XGC may be related to gallstones (17, 20), similar to our findings (Figure 3A). Additionally, intramural hypodense nodules, continuous mucosal lines, and absence of intrahepatic bile duct dilatation suggested XGC (Figures 3B, C). Goshima et al. reported that three of the five CT features that meet the XGC characteristics can provide high accuracy in distinguishing XGC from GBC (21), which is consistent with our results. Peripheral lymphadenopathy (>10 mm) was an independent factor in the diagnosis of GBC (Figure 3D), which may help in the correct preoperative diagnosis of XGC. As for the MRI features, the high-signal area of the T2WI corresponded to the area of significant xanthogranulomas (Figure 3E), which is consistent with a previous finding (22). The lipid signal of XGC nodules was owing to tissue cell phagocytosis and accumulation of extravasated bile and bile lipids. Ogawa et al. showed that the positive signal rate with DWI was significantly higher in GBC than in benign gallbladder diseases (23). DWI and ADC may play a significant role in distinguishing XGC from GBC because they are beneficial for distinguishing malignant and benign lesions.
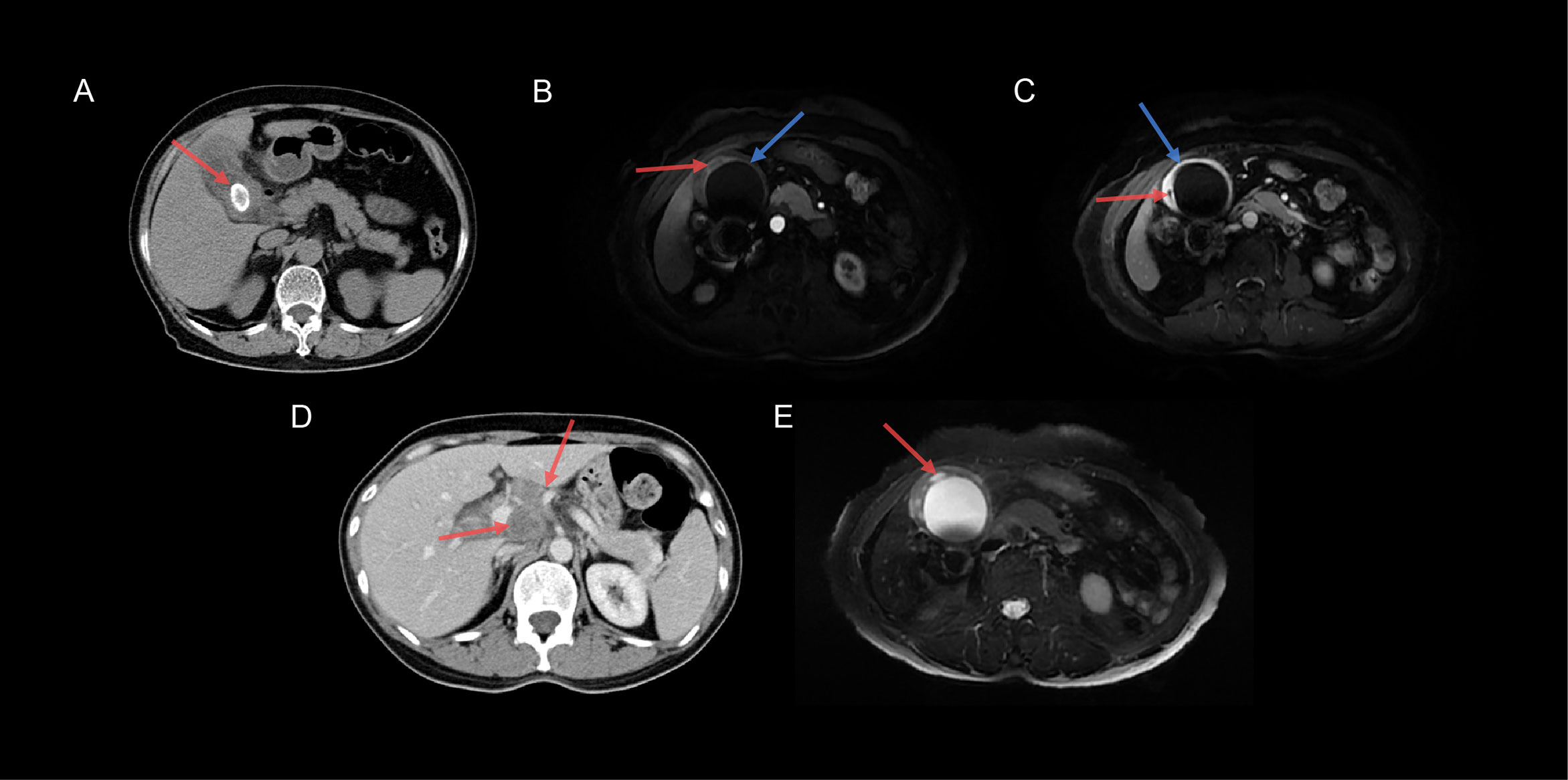
Figure 3 Typical images of XGC and GBC specimens. (A) The CT transverse section revealed cholecystolithiasis (red arrow) in a 46-year-old women with XGC. (B) Axial T1-weighted MRI-enhanced image showed low-intensity nodules (red arrow) in the gallbladder wall, and the continuous mucosal line was significantly enhanced (blue arrow) in the arterial phase. (C) Axial T1-weighted MRI-enhanced image showed that the gallbladder wall was obviously enhanced; however, the intramural nodules were unenhanced (red arrow), and the mucosal line was intact (blue arrow) in the delayed phase. (D) Axial CT image demonstrated two lymph nodes (>10 mm) in the hilar (red arrow) in a 46-year-old women with GBC. (E) Axial T2-weighted MRI-enhanced image demonstrated that the gallbladder wall was apparently thickened with multiple high-intensity nodules (red arrow).
XGC should be operated immediately once diagnosed to prevent complications owing to its invasiveness and destructiveness (24). Compared with open surgery, laparoscopic cholecystectomy has a shorter operating time and shorter postoperative hospital stay (25), which indicates that in some strictly selected cases, laparoscopic surgery is superior to open surgery for XGC treatment (4). Our predictive diagnosis model can distinguish XGC from GBC through adequate preoperative evaluation, helps clinical decision-making, and reduces the incidence of postoperative complications. Despite the high accuracy of the predictive diagnosis model, intraoperative diagnosis is still necessary because XGC may coexist with GBC. The frequency of coexistence of these two lesions was approximately 19.6% in India (26). However, there was no coexistence of XGC and GBC in our study, which is one of the limitations of this study. Owing to the retrospective study design, patients with only a single examination and obvious imaging findings were not included, so there may be an inherent selection bias. On the other hand, there were individual variables that have reported differences; however, they were not significant in our study cohort, indicating that more patients may need to be introduced in future studies.
Conclusion
We established a diagnostic prediction model for XGC using the RF method and logistic regression. The model contains 11 important critical variables, including T2WI signal of intramural nodules, T1WI signal of intramural nodules, lipid signal, gallstones, mucosal lines, ADC, peripheral lymph nodes, DWI, T2WI signal of the thickened cyst wall, bile duct dilation, and intramural nodules. This diagnostic prediction model has exhibited good discrimination between XGC and GBC, thus providing significant assistance in clinical practice.
Data Availability Statement
The raw data supporting the conclusions of this article will be made available by the authors, without undue reservation.
Ethics Statement
The studies involving human participants were reviewed and approved by The Second Affiliated Hospital of Zhejiang University School of Medicine (Approval numbers 20210806). Written informed consent for participation was not required for this study in accordance with the national legislation and the institutional requirements.
Author Contributions
QZ conceived the study and drafted the manuscript. Ri-Sheng Yu and JX helped critically revise the manuscript for important intellectual content. QZ, CL and JZ performed the research. JY, YW and XW helped analyse data. All authors contributed to the article and approved the submitted version.
Funding
This study was supported by grants from the Zhejiang Medical and Health Science and Technology Plan Project (Grant Numbers 2019KY476).
Conflict of Interest
The authors declare that the research was conducted in the absence of any commercial or financial relationships that could be construed as a potential conflict of interest.
Publisher’s Note
All claims expressed in this article are solely those of the authors and do not necessarily represent those of their affiliated organizations, or those of the publisher, the editors and the reviewers. Any product that may be evaluated in this article, or claim that may be made by its manufacturer, is not guaranteed or endorsed by the publisher.
Abbreviations
XGC, xanthogranulomatous cholecystitis; GBC, gallbladder cancer; CT, computed tomography; MRI, magnetic resonance imaging; RF, Random Forest; AUC, the area under the curve; ROC, the receiver operating characteristic curve; T1WI, T1 weighted imaging; T2WI, T1 weighted imaging; DWI, diffusion-weighted magnetic resonance imaging; ADC, apparent diffusion coefficient.
References
1. Zhao F, Lu PX, Yan SX, Wang GF, Yuan J, Zhang SZ, et al. CT and MR Features of Xanthogranulomatous Cholecystitis: An Analysis of Consecutive 49 Cases. Eur J Radiol (2013) 82(9):1391–7. doi: 10.1016/j.ejrad.2013.04.026
2. Hale M, Roberts K, Hodson J, Scott N, Sheridan M, Toogood G. Xanthogranulomatous Cholecystitis: A European and Global Perspective. HPB Off J Int Hepato Pancreato Biliary Assoc (2014) 16(5):448–58. doi: 10.1111/hpb.12152
3. Goodman Z, Ishak K. Xanthogranulomatous Cholecystitis. Am J Surg Pathol (1981) 5(7):653–9. doi: 10.1097/00000478-198110000-00007
4. Cui Y, Zhang H, Zhao E, Cui N, Li Z. Differential Diagnosis and Treatment Options for Xanthogranulomatous Cholecystitis. Med Principles Pract Int J Kuwait University Health Sci Centre (2013) 22(1):18–23. doi: 10.1159/000339659
5. Suzuki H. Specific Radiological Findings, If Present, can Offer High Accuracy for the Differentiation of Xanthogranulomatous Cholecystitis and Gallbladder Cancer. Ann Transl Med (2020) 8(11):662. doi: 10.21037/atm.2020.03.193
6. Truant S, Chater C, Pruvot F-R. Greatly Enlarged Thickened Gallbladder. JAMA Surg (2015) 150(3):267–8. doi: 10.1001/jamasurg.2014.492
7. Feng L, You Z, Gou J, Liao E, Chen L. Xanthogranulomatous Cholecystitis: Experience in 100 Cases. Ann Transl Med (2020) 8(17):1089. doi: 10.21037/atm-20-5836
8. Loukas C, Frountzas M, Schizas D. Patch-Based Classification of Gallbladder Wall Vascularity From Laparoscopic Images Using Deep Learning. Int J Comput Assisted Radiol Surg (2021) 16(1):103–13. doi: 10.1007/s11548-020-02285-x
9. Shoshan Y, Bakalo R, Gilboa-Solomon F, Ratner V, Barkan E, Ozery-Flato M, et al. Artificial Intelligence for Reducing Workload in Breast Cancer Screening With Digital Breast Tomosynthesis. Radiology (2022) p:211105. doi: 10.1148/radiol.211105
10. Reiazi R, Arrowsmith C, Welch M, Abbas-Aghababazadeh F, Eeles C, Tadic T, et al. Prediction of Human Papillomavirus (HPV) Association of Oropharyngeal Cancer (OPC) Using Radiomics: The Impact of the Variation of CT Scanner. Cancers (2021) 13(9). doi: 10.3390/cancers13092269
11. Gao R, Zhao S, Aishanjiang K, Cai H, Wei T, Zhang Y, et al. Deep Learning for Differential Diagnosis of Malignant Hepatic Tumors Based on Multi-Phase Contrast-Enhanced CT and Clinical Data. J Hematol Oncol (2021) 14(1):154. doi: 10.1186/s13045-021-01167-2
12. Bo X, Chen E, Wang J, Nan L, Xin Y, Wang C, et al. Diagnostic Accuracy of Imaging Modalities in Differentiating Xanthogranulomatous Cholecystitis From Gallbladder Cancer. Ann Transl Med (2019) 7(22):627. doi: 10.21037/atm.2019.11.35
13. Makimoto S, Takami T, Hatano K, Kataoka N, Yamaguchi T, Tomita M, et al. Xanthogranulomatous Cholecystitis: A Review of 31 Patients. Surg Endosc (2021) 35(7):3874–80. doi: 10.1007/s00464-020-07828-6
14. Giudicelli X, Rode A, Bancel B, Nguyen A, Mabrut J. Xanthogranulomatous Cholecystitis: Diagnosis and Management. J Visceral Surg (2021) 158(4):326–36. doi: 10.1016/j.jviscsurg.2021.02.004
15. Nacif L, Hessheimer A, Rodríguez Gómez S, Montironi C, Fondevila C. Infiltrative Xanthogranulomatous Cholecystitis Mimicking Aggressive Gallbladder Carcinoma: A Diagnostic and Therapeutic Dilemma. World J Gastroenterol (2017) 23(48):8671–8. doi: 10.3748/wjg.v23.i48.8671
16. Rahman J, Tahir M, Sonawane S. Xanthogranulomatous Cholecystitis: A Diagnostic Challenge for Radiologists, Surgeons, and Pathologists. Cureus (2020) 12(8):e10007. doi: 10.7759/cureus.10007
17. Kwon A, Matsui Y, Uemura Y. Surgical Procedures and Histopathologic Findings for Patients With Xanthogranulomatous Cholecystitis. J Am Coll Surgeons (2004) 199(2):204–10. doi: 10.1016/j.jamcollsurg.2004.03.018
18. Garcea G, Rajesh A, Dennison A. Surgical Management of Cystic Lesions in the Liver. ANZ J Surg (2013) 83:516–22. doi: 10.1111/ans.12049
19. Singh VP, Rajesh S, Bihari C, Desai SN, Pargewar SS, Arora A. Xanthogranulomatous Cholecystitis: What Every Radiologist Should Know. World J Radiol (2016) 8(2):183–91. doi: 10.4329/wjr.v8.i2.183
20. Uchiyama K, Ozawa S, Ueno M, Hayami S, Hirono S, Ina S, et al. Xanthogranulomatous Cholecystitis: The Use of Preoperative CT Findings to Differentiate it From Gallbladder Carcinoma. J Hepato-biliary-pancreatic Surg (2009) 16(3):333–8. doi: 10.1007/s00534-009-0067-9
21. Goshima S, Chang S, Wang J, Kanematsu M, Bae K, Federle M. Xanthogranulomatous Cholecystitis: Diagnostic Performance of CT to Differentiate From Gallbladder Cancer. Eur J Radiol (2010) 74(3):e79–83. doi: 10.1016/j.ejrad.2009.04.017
22. Shuto R, Kiyosue H, Komatsu E, Matsumoto S, Kawano K, Kondo Y, et al. CT and MR Imaging Findings of Xanthogranulomatous Cholecystitis: Correlation With Pathologic Findings. Eur Radiol (2004) 14(3):440–6. doi: 10.1007/s00330-003-1931-7
23. Ogawa T, Horaguchi J, Fujita N, Noda Y, Kobayashi G, Ito K, et al. High B-Value Diffusion-Weighted Magnetic Resonance Imaging for Gallbladder Lesions: Differentiation Between Benignity and Malignancy. J Gastroenterol (2012) 47(12):1352–60. doi: 10.1007/s00535-012-0604-1
24. Srinivas G, Sinha S, Ryley N, Houghton P. Perfidious Gallbladders - a Diagnostic Dilemma With Xanthogranulomatous Cholecystitis. Ann R Coll Surgeons Engl (2007) 89(2):168–72. doi: 10.1308/003588407X155833
25. Frountzas M, Schizas D, Liatsou E, Economopoulos K, Nikolaou C, Apostolou K, et al. Presentation and Surgical Management of Xanthogranulomatous Cholecystitis. Hepatobiliary Pancreatic Dis Int HBPD Int (2021) 20(2):117–27. doi: 10.1016/j.hbpd.2021.01.002
Keywords: gallbladder cancer, radiological features, diagnostic prediction model, RandomForest, Xanthogranulomatous cholecystitis (XGC)
Citation: Zhou Q-M, Liu C-X, Zhou J-P, Yu J-N, Wang Y, Wang X-J, Xu J-X and Yu R-S (2022) Machine Learning-Based Radiological Features and Diagnostic Predictive Model of Xanthogranulomatous Cholecystitis. Front. Oncol. 12:792077. doi: 10.3389/fonc.2022.792077
Received: 09 October 2021; Accepted: 31 January 2022;
Published: 24 February 2022.
Edited by:
Qi Liu, Fudan University, ChinaReviewed by:
Faten Limaiem, Hôpital Mongi Slim, TunisiaDimitrios Schizas, National and Kapodistrian University of Athens, Greece
Copyright © 2022 Zhou, Liu, Zhou, Yu, Wang, Wang, Xu and Yu. This is an open-access article distributed under the terms of the Creative Commons Attribution License (CC BY). The use, distribution or reproduction in other forums is permitted, provided the original author(s) and the copyright owner(s) are credited and that the original publication in this journal is cited, in accordance with accepted academic practice. No use, distribution or reproduction is permitted which does not comply with these terms.
*Correspondence: Ri-Sheng Yu, risheng-yu@zju.edu.cn; Jian-Xia Xu, xjx129@163.com