- 1Department of Hepatobiliary Surgery, The Affiliated Hospital of Southwest Medical University, Luzhou, China
- 2Department of General Surgery, Changhai Hospital of The Second Military Medical University, Shanghai, China
- 3Department of Clinical Medicine, Southwest Medical University, Luzhou, China
- 4Department of Ultrasound, Seventh People’s Hospital of Shanghai University of Traditional Chinese Medicine, Shanghai, China
- 5Department of Hepatobiliary Surgery, Zigong Fourth People’s Hospital, Zigong, China
- 6Department of Gastroenterology, Zigong Third People’s Hospital, Zigong, China
- 7School of Computer Science and Engineering, University of Electronic Science and Technology of China, Chengdu, China
Objective: The aim of this study was to assess the diagnostic ability of artificial intelligence (AI) in the detection of early upper gastrointestinal cancer (EUGIC) using endoscopic images.
Methods: Databases were searched for studies on AI-assisted diagnosis of EUGIC using endoscopic images. The pooled area under the curve (AUC), sensitivity, specificity, positive likelihood ratio (PLR), negative likelihood ratio (NLR), and diagnostic odds ratio (DOR) with 95% confidence interval (CI) were calculated.
Results: Overall, 34 studies were included in our final analysis. Among the 17 image-based studies investigating early esophageal cancer (EEC) detection, the pooled AUC, sensitivity, specificity, PLR, NLR, and DOR were 0.98, 0.95 (95% CI, 0.95–0.96), 0.95 (95% CI, 0.94–0.95), 10.76 (95% CI, 7.33–15.79), 0.07 (95% CI, 0.04–0.11), and 173.93 (95% CI, 81.79–369.83), respectively. Among the seven patient-based studies investigating EEC detection, the pooled AUC, sensitivity, specificity, PLR, NLR, and DOR were 0.98, 0.94 (95% CI, 0.91–0.96), 0.90 (95% CI, 0.88–0.92), 6.14 (95% CI, 2.06–18.30), 0.07 (95% CI, 0.04–0.11), and 69.13 (95% CI, 14.73–324.45), respectively. Among the 15 image-based studies investigating early gastric cancer (EGC) detection, the pooled AUC, sensitivity, specificity, PLR, NLR, and DOR were 0.94, 0.87 (95% CI, 0.87–0.88), 0.88 (95% CI, 0.87–0.88), 7.20 (95% CI, 4.32–12.00), 0.14 (95% CI, 0.09–0.23), and 48.77 (95% CI, 24.98–95.19), respectively.
Conclusions: On the basis of our meta-analysis, AI exhibited high accuracy in diagnosis of EUGIC.
Systematic Review Registration: https://www.crd.york.ac.uk/PROSPERO/, identifier PROSPERO (CRD42021270443).
Introduction
Upper gastrointestinal cancer (UGIC) is among the most common malignancies and causes of cancerrelated deaths worldwide, which presents a major challenge for health-care system (1). A majority of UGIC patients are detected at a late stage and have a poor prognosis. In contrast, with early detection, the 5-year overall survival can be more than 90% (2, 3). Thus, the early detection of UGIC is essential to improve patient prognosis.
Endoscopy remains the most optimal approach of UGIC detection (4, 5). However, endoscopic features of early upper gastrointestinal cancer (EUGIC) lesions are subtle and easily missed. Moreover, diagnostic accuracy depends on the expertise of endoscopists (2). One report revealed that EUGIC misdiagnosis can be high regardless of the number of patients, developed or underdeveloped locations, or in countries performing a remarkably high volume of endoscopies (6).
Artificial intelligence (AI) is gaining much popularity in the field of medicine, including gastrointestinal endoscopy (7–11). Owing to its good pattern recognition ability, AI is a promising candidate for detection of upper gastrointestinal lesions (12, 13). However, the data on AI-assisted EUGIC diagnosis are still lacking. Hence, we conducted this study to assess the diagnostic accuracy of AI in the detection of EUGIC using endoscopic images.
Methods
This systematic review and meta-analysis was reported in line with PRISMA guidelines and was registered with the international prospective register of systematic reviews PROSPERO (CRD42021270443).
Search Strategy and Study Selection
Two authors (FK and JD) separately searched electronic databases (PubMed, Medline, Embase, Web of Science, Cochrane library, and Google scholar) from the date of establishment until November 2021 using the following pre-specified search terms: “endoscopy”, “endoscopic”, “early gastric cancer”, “early esophageal cancer”, “early esophageal squamous cell carcinoma”, “early Barrett’s neoplasia”, “early esophageal adenocarcinoma”, “artificial intelligence”, “AI”, “machine learning”, “deep learning”, “artificial neural network”, “support vector machine”, “naive bayes”, and “classification tree”. Potentially relevant studies (based on title and abstract) were then read completely to ensure eligibility in the meta-analysis. In addition, we also reviewed the reference lists of relevant studies to search for eligible studies.
Study Eligibility Criteria
Studies meeting the following criteria were included in the meta-analysis: (1) studies that evaluated AI diagnostic performance for EUGIC using endoscopic images; (2) true positive (TP), false positive (FP), false negative (FN), and true negative (TN) values could be extracted directly or calculated from the original studies. The following studies were excluded from our meta-analysis: (1) reviews, (2) meta-analyses, and (3) comments or protocols. We followed a strict exclusion policy that any study meeting one of the abovementioned exclusion criteria was excluded.
Data Extraction
Two authors (MZJ and XDL) separately extracted data from the included studies, namely, author, publication year, study design, imaging type, AI model, sample size, TP, FP, FN, and TN. TP, FP, FN, and TN were extracted with the histology as the reference standard. Intramucosal carcinoma, T1 cancer, and Barrett’s esophagus (BE) with high-grade dysplasia were considered as positive. Normal tissue, BE without high-grade dysplasia, and non-cancerous lesions were considered as negative. The authors of the studies were contacted for missing information, if necessary. Discrepancies were decided through discussion.
Methodological Quality Assessment
Two authors (XDL and XCL) evaluated the quality and potential bias risk of eligible studies based on the Quality Assessment of Diagnostic Accuracy Studies (QUADAS-2) (14). Disagreements were resolved through discussion. The QUADAS-2 tool was composed of four domains: “patient selection”, “index test”, “reference standard”, and “flow and timing”. In addition, the “patient selection”, “index test”, and “reference standard” were further tested for “applicability”. Each domain was then stratified into high, low, or unclear bias risk.
Statistical Analysis
Statistical analysis was performed using the Meta-Disc software (version 14). To assess AI performance in EUGIC diagnosis, the pooled sensitivity, specificity, positive likelihood ratio (PLR), negative likelihood ratio (NLR), and diagnostic odds ratio (DOR) with 95% confidence interval (CI) were computed. In addition, we plotted a summary receiver operating characteristic (SROC) curve. The area under the curve (AUC) was computed to predict precision in diagnosis. We evaluated AI diagnostic performance based on images (image-based analysis) and patients (patient-based analysis). The forest plot was constructed. The inconsistency index (I2) test determined presence or absence of heterogeneity among studies using sensitivity (15). A fixed-effects model was used if the I2 value < 50%; otherwise, a random-effects model was selected. The Spearman correlation coefficient (SCC) between sensitivity and false positive rate was calculated, and a value > 0.6 indicated a threshold effect.
Results
Literature Screening and Bias Evaluation
The primary screening uncovered 1,812 eligible studies. Upon removal of duplicates and other studies that were irrelevant to this study (based on title, abstract, and full article), 34 studies (16–49) investigating AI-assisted early esophageal cancer (EEC) and early gastric cancer (EGC) detection were included in the final meta-analysis. Among 34 studies, 18 and 17 studies assessed the diagnostic ability of AI in the detection of EEC (16–33) and EGC (29, 34–49), respectively. An overview of the eligible studies screening process is illustrated in Figure 1. Table 1 presents the characteristics of all eligible studies. Overall, the included studies showed high methodological quality. The quality assessment and risk of bias for each eligible study are summarized in Figure 2.
AI-Assisted EEC Diagnosis Using Endoscopic Images
Meta-Analysis of AI-Assisted EEC Diagnosis Using Endoscopic Images [White-Light Imaging (WLI)/Narrow-Band Imaging (NBI) Images]
Eigtheen studies (16–33) reported the AI-assisted EEC diagnosis performance using endoscopic images. Moreover, 17 and 7 studies reported the AI-assisted EEC diagnosis performance based on per image (16–26, 28–33) and per patient (17, 18, 21, 24, 27, 31, 33), respectively. Among the 17 image-based studies, a total of 13,091 images (4,310 positive vs. 8,781 negative) were identified. Specifically, the positive group composed of the early esophageal squamous cell carcinoma (EESCC), early esophageal adenocarcinoma (EEAC), and EEC images, whereas the negative group consisted of normal, Barrett’s esophagus, and non-cancerous images. In most studies, the AI algorithm type was convolutional neural network (CNN). However, single-shot multibox detector (SSD) (25) and support vector machine (SVM) (29, 31) were also employed. Among the seven patient-based studies, a total of 1,380 patients (316 positive vs. 1,064 negative) were identified. Specifically, EESCC and EEAC constituted the positive group, whereas normal, Barrett’s esophagus, and non-cancerous comprised of the negative group. Most studies used the CNN algorithm. However, SVM was used in one study (31).
In the 17 image-based studies investigating AI-assisted EEC diagnosis, the pooled AUC, sensitivity, specificity, PLR, NLR, and DOR were 0.98, 0.95 (95% CI, 0.95–0.96), 0.95 (95% CI, 0.94–0.95), 10.76 (95% CI, 7.33–15.79), 0.07 (95% CI, 0.04–0.11), and 173.93 (95% CI,81.79–369.83), respectively (Figures 3A–F). In addition, the SCC and p-values were −0.10 and 0.70 (>0.05), respectively, suggesting no significant threshold effect among these studies.
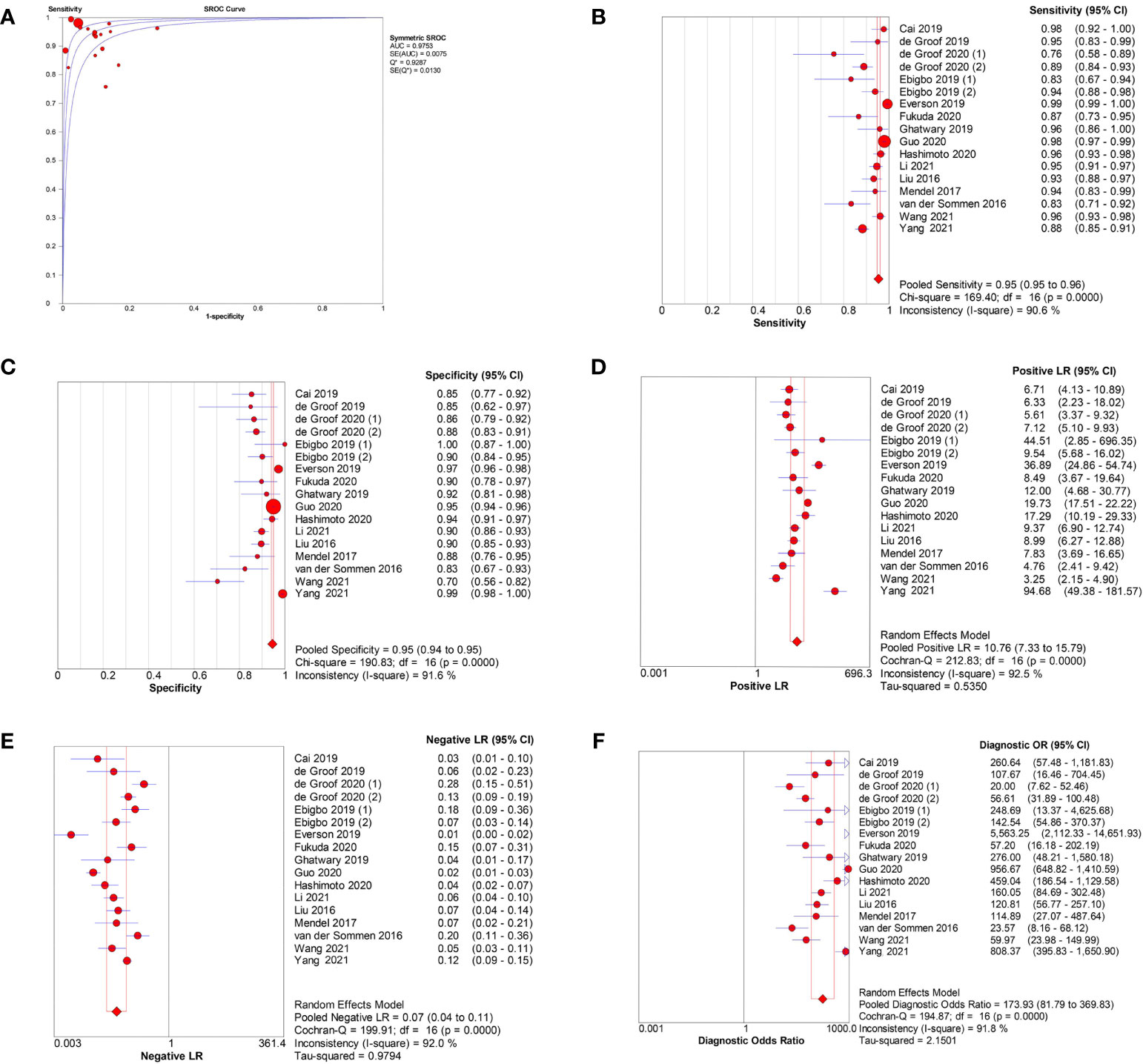
Figure 3 Meta-analysis of AI-assisted EEC diagnosis (image-based analysis). (A) SROC curve. (B) Pooled sensitivity. (C) Pooled specificity. (D) Pooled PLR. (E) Pooled NLR. (F) Pooled DOR.
Among the seven patient-based studies investigating AI-assisted EEC diagnosis, the pooled AUC, sensitivity, specificity, PLR, NLR, and DOR were 0.98, 0.94 (95% CI, 0.91–0.96), 0.90 (95% CI, 0.88–0.92), 6.14(95% CI, 2.06–18.30), 0.07 (95% CI, 0.04–0.11), and 69.13 (95% CI, 14.73–324.45), respectively (Figures 4A–F). The SCC and p-values were −0.071 and 0.879 (>0.05), respectively, indicating no significant threshold effect among these studies.
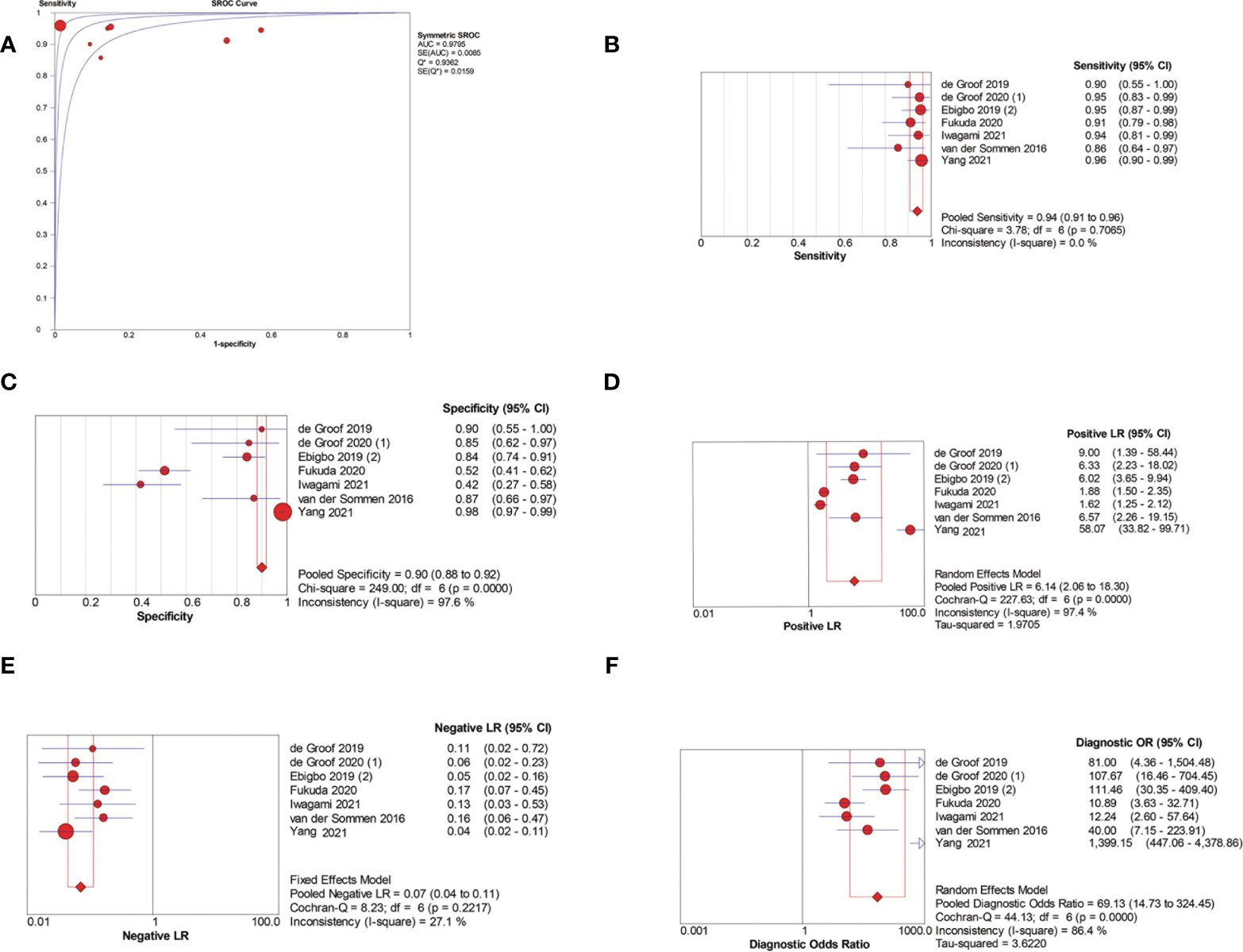
Figure 4 Meta-analysis of AI-assisted EEC diagnosis (patient-based analysis). (A) SROC curve. (B) Pooled sensitivity. (C) Pooled specificity. (D) Pooled PLR. (E) Pooled NLR. (F) Pooled DOR.
AI-Assisted EGC Diagnosis Using Endoscopy Images
Meta-Analysis of AI-Assisted EGC Diagnosis Using Endoscopic Images (WLI/NBI Images)
Seventeen studies (29, 34–49) reported the AI diagnosis performance of EGC using endoscopic images. Fifteen studies (29, 34–38, 40–42, 44–49), one study (39), and one study (43) evaluated the AI diagnosis performance based on per image, per patient, and per lesion, respectively.
Among the 15 image-based studies, a total of 31,423 images (13,367 positive vs. 18,056 negative) were identified. Only the EGC images were categorized in the positive group, whereas the normal and non-cancerous images were categorized in the negative group. A majority of the studies used CNN algorithm. However, the SVM algorithm was also used (29, 37). Among the two patient/lesion-based studies, a total of 414 patients/lesions (103 positive vs. 311 negative) were identified. Only the EGC were placed in the positive group, whereas the gastric ulcers and non-cancerous were placed in the negative group. Both studies utilized CNN algorithm.
Among the 15 image-based EGC detection studies, the pooled AUC, sensitivity, specificity, PLR, NLR, and DOR were 0.94, 0.87 (95% CI, 0.87–0.88), 0.88 (95% CI, 0.87–0.88), 7.20 (95% CI, 4.32–12.00), 0.14 (95% CI, 0.09–0.23), and 48.77 (95% CI, 24.98–95.19), respectively (Figures 5A–F). The SCC and p-values were −0.44 and 0.10 (>0.05), respectively, suggesting no significant threshold effect among these studies.
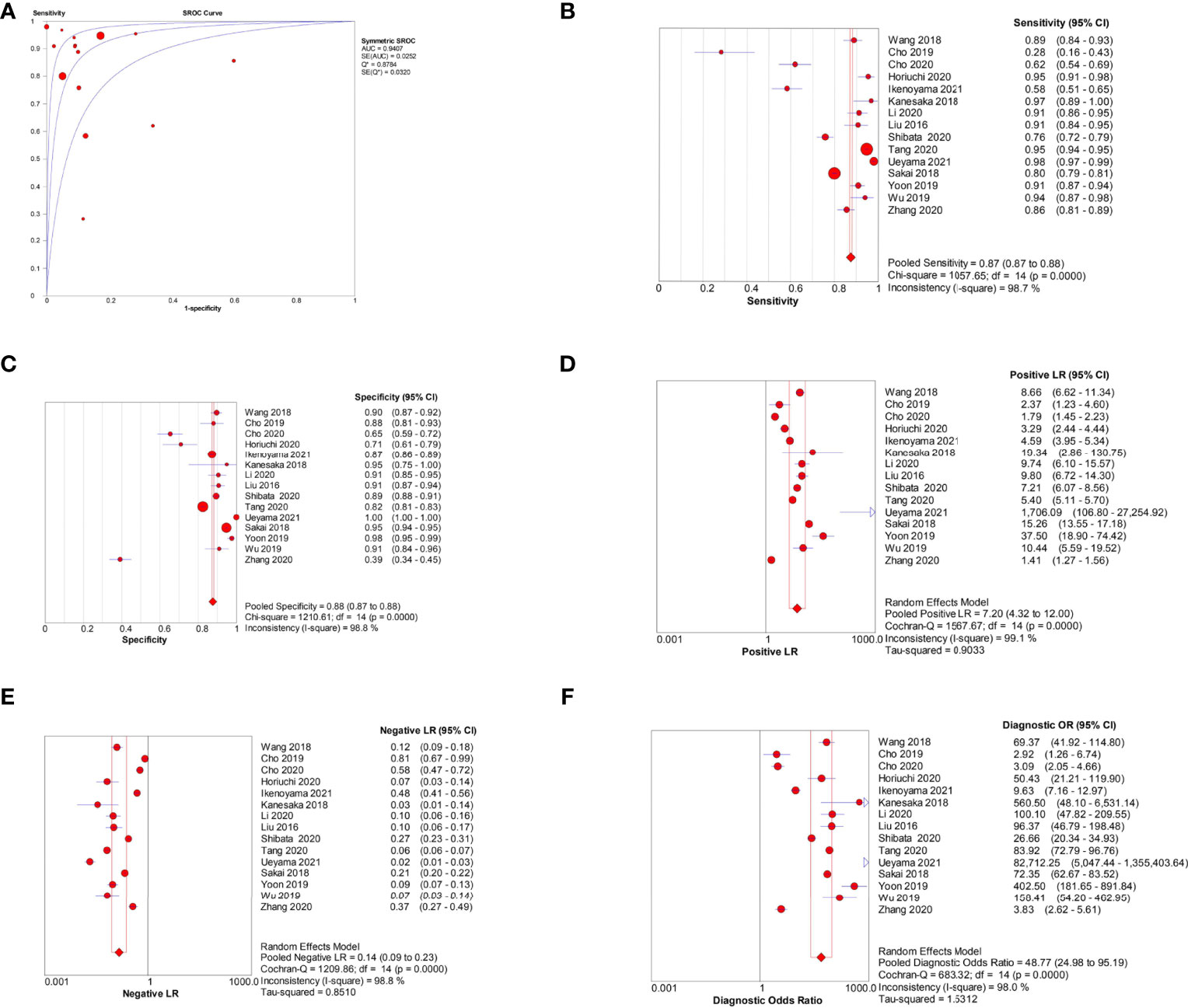
Figure 5 Meta-analysis of AI-assisted EGC diagnosis (image-based analysis). (A) SROC curve. (B) Pooled sensitivity. (C) Pooled specificity. (D) Pooled PLR. (E) Pooled NLR. (F) Pooled DOR.
Only two patient-based studies evaluated AI in the diagnosis of EGC, so meta-analysis was not performed. In Namikawa’s study, the sensitivity and specificity were 0.99 and 0.93, respectively. In Wu’s study, the sensitivity and specificity were 1.00 and 0.8429, respectively.
Subgroup Analysis Based on Imaging Type
To compare the AI diagnostic performance of EEC and EGC detection using WLI and NBI endoscopic images, we performed a subgroup analysis based on imaging type. On the basis of the results of subgroup analysis, the NBI mode showed a better diagnostic performance than the WLI mode. The results are summarized in Table 2.
Meta-Analysis of AI-Assisted EGC Diagnosis Using WLI Endoscopic Images
Fourteen studies (16–22, 25, 28–33) reported the performance of AI-assisted EEC detection using WLI endoscopic images. Among the 14 image-based studies, the pooled AUC, sensitivity, specificity, PLR, NLR, and DOR were 0.97, 0.92 (95% CI, 0.90–0.93), 0.93 (95% CI, 0.91–0.94), 9.11 (95% CI, 6.04-13.75), 0.09 (95% CI, 0.06–0.13), and 136.06 (95% CI, 67.20–275.49), respectively. The SCC and p-values were 0.24 and 0.40 (>0.05), respectively, indicating no significant threshold effect among these studies.
Among the five patient-based studies (17, 18, 21, 31, 33), the pooled AUC, sensitivity, specificity, PLR, NLR, and DOR were 0.95, 0.95 (95% CI, 0.92–0.98), 0.82 (95% CI, 0.74–0.88), 4.7 (95% CI, 3.32–6.65), 0.07 (95% CI, 0.04–0.12), and 86.48 (95% CI, 39.04–191.57), respectively. The SCC and p-values were 0.5 and 0.39 (>0.05), respectively, indicating no significant threshold effect among these studies.
Meta-Analysis of AI-Assisted EEC Diagnosis Using NBI Endoscopic Images
Seven studies (21, 23, 24, 26, 28, 30, 32) reported the AI-assisted EEC detection performance using NBI endoscopic images. Among the seven image-based studies, the pooled AUC, sensitivity, specificity, PLR, NLR, and DOR were 0.99, 0.98 (95% CI, 0.97–0.98), 0.95 (95% CI, 0.95–0.96), 14.00 (95% CI, 6.71–29.20), 0.05 (95% CI, 0.02–0.11), and 363.56 (95% CI, 108.47–1218.26), respectively. The SCC and p-values were −0.04 and 0.94 (>0.05), respectively, indicating no significant threshold effect among these studies. Only two patient-based studies evaluated AI for the diagnosis of EEC, so meta-analysis was not performed. In the study by Ebigbo et al., (21) the sensitivity and specificity were 0.94 and 0.80, respectively. In the study by Fukuda et al. (24), the sensitivity and specificity were 0.91 and 0.52, respectively.
Meta-Analysis of AI-Assisted EGC Diagnosis Using WLI Endoscopic Images
Twelve studies (29, 34, 36, 40, 41, 43–49) reported the AI diagnosis performance of EGC detection using WLI endoscopic images. Eleven studies (29, 34, 36, 40, 41, 44–49) reported the AI diagnosis performance based on per image. In addition, only Wu’s study (43) reported the AI diagnosis performance based on per lesion. Among the 11 image-based EGC detection studies, the pooled AUC, sensitivity, specificity, PLR, NLR, and DOR were 0.92, 0.86 (95% CI, 0.85–0.87), 0.87 (95% CI, 0.87–0.88), 6.12 (95% CI, 3.53–10.63), 0.21 (95% CI, 0.12–0.35), and 29.92 (95% CI, 14.23–62.90). The SCC and p-values were −0.35 and 0.30 (>0.05), respectively, indicating no significant threshold effect among these studies.
Meta-Analysis of AI-Assisted EGC Diagnosis Using NBI Endoscopic Images
Four studies (35, 37, 38, 42) reported the AI diagnosis performance for EGC using endoscopic NBI images based on per image. In addition, no studies reported the AI diagnosis performance based on per lesion or per patient. Among the four image-based EGC detection studies, the pooled AUC, sensitivity, specificity, PLR, NLR, and DOR were 0.99, 0.97 (95% CI, 0.96–0.98), 0.96 (95% CI, 0.95–0.97), 25.92 (95% CI, 1.63–413.31), 0.05 (95% CI, 0.02–0.12), and 523.76 (95% CI, 37.39–7336.36), respectively. The SCC and p-values were −0.8 and 0.2 (>0.05), respectively, suggesting no significant threshold effect among these studies.
Subgroup Analysis Based on Pathologic Type in Esophagus
We also performed a subgroup analysis between early esophageal squamous cell carcinoma (EESCC) and early esophageal adenocarcinoma (EEAC). On the basis of the results of subgroup analysis, AI showed a better diagnostic performance in EESCC than EEAC. The results are summarized in Table 3.
Meta-Analysis of AI-Assisted EESCC Diagnosis Using Endoscopic Images (WLI/NBI Images)
Six studies (16, 23, 24, 26, 28, 33) reported the AI-assisted EESCC diagnosis performance using endoscopic images based on per image. Among the six image-based studies, the pooled AUC, sensitivity, specificity, PLR, NLR, and DOR were 0.99, 0.96 (95% CI, 0.96–0.97), 0.95 (95% CI, 0.95–0.96), 18.21 (95% CI, 10.07–32.93), 0.04 (95% CI, 0.01–0.11), and 491.74 (95% CI, 170.20–1420.71), respectively. The SCC and p-values were −0.20 and 0.70 (>0.05), respectively, indicating no significant threshold effect among these studies. Only two patient-based studies (24, 33) evaluated AI for the diagnosis of EESCC, so meta-analysis was not performed. In the study by Yang et al. (33), the sensitivity and specificity were 0.97 and 0.99, respectively. In the study by Fukuda et al. (24), the sensitivity and specificity were 0.91 and 0.52, respectively.
Meta-Analysis of AI-Assisted EEAC Diagnosis Using Endoscopic Images (WLI/NBI Images)
Ten studies (17–22, 25, 30–32) reported the AI-assisted EEAC diagnosis performance using endoscopic images based on per image. Among the 10 image-based studies, the pooled AUC, sensitivity, specificity, PLR, NLR, and DOR were 0.96, 0.93 (95% CI, 0.91–0.94), 0.89 (95% CI, 0.87–0.91), 7.41 (95% CI, 5.09–10.77), 0.10 (95% CI, 0.06–0.15), and 87.66 (95% CI, 44.40–173.08), respectively. The SCC and p-values were −0.03 and 0.93 (>0.05), respectively, indicating no significant threshold effect among these studies.
Among the five patient-based studies (17, 18, 21, 27, 31), the pooled AUC, sensitivity, specificity, PLR, NLR, and DOR were 0.96, 0.94 (95% CI, 0.89–0.97), 0.75 (95% CI, 0.68–0.81), 4.76 (95% CI, 1.69–13.38), 0.09 (95% CI, 0.05–0.17), and 51.94 (95% CI, 20.89–129.11), respectively. The SCC and p-values were 0.6 and 0.29 (>0.05), respectively, indicating no significant threshold effect among these studies.
Discussion
In this study, we conducted a comprehensive literature search and included all studies that assessed diagnostic performance of AI in EUGIC using endoscopic images. Next, we conducted a meta-analysis to explore the diagnostic performance of AI in EUGIC detection. On the basis of our results, AI demonstrated an excellent diagnostic ability, with high accuracy, sensitivity, specificity, PLR, and DOR, and with low NLR in detecting EUGIC, suggesting the feasibility of AI-assisted EUGIC diagnosis in clinical practice. To the best of our knowledge, this is the first systematic review and meta-analysis that explored the AI-assisted detection of EUGIC based on upper gastrointestinal endoscopic images.
Endoscopy is the primary tool used in the diagnosis of UGIC (50, 51). However, EUGIC lesions manifest as indistinct mucosal alterations under the classic WLI images. Therefore, EUGIC detection is often highly dependent on endoscopist’s experience and expertise (52). Previous studies also revealed that WLI-based EGC diagnosis is possible, but with poor sensitivity or specificity (36, 47, 48). More recently, AI-assisted image recognition makes remarkable breakthroughs in the field of medical imaging diagnosis and is gaining popularity in clinical practice (7–11, 53, 54). Traditional AI algorithms like SVM and decision trees require experts to manually design the image features, before the algorithm extracts the feature from images (53, 55). This results in the detection of only specific lesions, and in case the features are insufficient, satisfactory identification results cannot be obtained. Simultaneously, manual design is highly dependent on the previous knowledge of designers. Thus, it is not feasible to work with large amounts of data. At present, many studies on medical image recognition adopt deep learning algorithm based on CNN. The deep learning can automatically learn the most predictive characteristics from a large image data file with no requirement of previous knowledge and classify these images. In our study, most included studies used the CNN algorithm, so we did not compare the AI diagnostic ability between the different algorithms. Many studies demonstrated excellent AI performance in detecting early esophageal and stomach cancers with the CNN algorithm. Consistent with these studies, in our study, AI exhibited an excellent diagnosis performance for EUGIC with high accuracy, sensitivity, and specificity.
Although several advanced technologies like NBI, confocal laser endomicroscopy, and blue laser imaging have shown great promise in the endoscopic detection of EUGIC, endoscopists still need extensive specialized training and substantial experience to identify early cancer lesions accurately. NBI endoscopy is an optical image-enhanced technology that better visualizes surface structures and blood vessels than does WLI (56). Multiple studies have demonstrated NBI has a high sensitivity in detecting EUGIC (37, 57, 58). To compare the AI diagnostic performance for EUGIC detection using WLI and NBI endoscopic images, we performed a subgroup analysis based on imaging type. On the basis of our results, the NBI imaging mode has a superior diagnostic performance for both EEC and EGC detection, with higher AUC, sensitivity, specificity, PLR and DOR, and lower NLR.
There are limitations to this study. First, most studies were based on the retrospective review of selected images. At the same time, the number of positive images and negative images included in some included studies was significantly different. All retrospective studies were considered at high risk for selection bias, so those studies might overestimate the diagnostic accuracy of AI. Second, few studies compared the diagnostic efficacy between AI and endoscopists, so we could not perform meta-analysis to compared the diagnostic efficacy between AI and endoscopists.
In conclusion, on the basis of our meta-analysis, AI achieved high accuracy in diagnosis of EUGIC. Further prospective studies comparing the diagnostic performance between AI and endoscopists are warranted.
Data Availability Statement
The original contributions presented in the study are included in the article/supplementary material. Further inquiries can be directed to the corresponding author.
Author Contribution
DL designed the study. FK and JD screened electronic databases. MZ and XDL extracted data from the selected articles. XDL and XCL evaluated eligible study quality and potential bias risk. Statistical analyses were performed by YT and BL. DL wrote the manuscript. SS supervised the study. All authors contributed to the article and approved the submitted version.
Funding
This study is supported by the Key Research and Development Project of Science & Technology Department of Sichuan Province (20ZDYF1129) and the Applied Basic Research Project of Science & Technology Department of Luzhou city (2018-JYJ-45).
Conflict of Interest
The authors declare that the research was conducted in the absence of any commercial or financial relationships that could be construed as a potential conflict of interest.
Publisher’s Note
All claims expressed in this article are solely those of the authors and do not necessarily represent those of their affiliated organizations, or those of the publisher, the editors and the reviewers. Any product that may be evaluated in this article, or claim that may be made by its manufacturer, is not guaranteed or endorsed by the publisher.
References
1. Siegel RL, Miller KD, Jemal A. Cancer Statistics, 2020. CA Cancer J Clin (2020) 70:7–30. doi: 10.3322/caac.21590
2. Veitch AM, Uedo N, Yao K, East JE. Optimizing Early Upper Gastrointestinal Cancer Detection at Endoscopy. Nat Rev Gastroenterol Hepatol (2015) 12:660–7. doi: 10.1038/nrgastro.2015.128
3. Soetikno R, Kaltenbach T, Yeh R, Gotoda T. Endoscopic Mucosal Resection for Early Cancers of the Upper Gastrointestinal Tract. J Clin Oncol (2005) 23:4490–8. doi: 10.1200/JCO.2005.19.935
4. Ono H, Yao K, Fujishiro M, Oda I, Uedo N, Nimura S, et al. Guidelines for Endoscopic Submucosal Dissection and Endoscopic Mucosal Resection for Early Gastric Cancer. Dig Endosc (2016) 28:3–15. doi: 10.1111/den.12518
5. Mannath J, Ragunath K. Role of Endoscopy in Early Oesophageal Cancer. Nat Rev Gastroenterol Hepatol (2016) 13:720–30. doi: 10.1038/nrgastro.2016.148
6. Menon S, Trudgill N. How Commonly is Upper Gastrointestinal Cancer Missed at Endoscopy? A Meta-Analysis. Endosc Int Open (2014) 2:E46–50. doi: 10.1055/s-0034-1365524
7. Mori Y, Kudo SE, Mohmed HEN, Misawa M, Ogata N, Itoh H, et al. Artificial Intelligence and Upper Gastrointestinal Endoscopy: Current Status and Future Perspective. Dig Endosc (2019) 31:378–88. doi: 10.1111/den.13317
8. Hassan C, Spadaccini M, Iannone A, Maselli R, Jovani M, Chandrasekar VT, et al. Performance of Artificial Intelligence in Colonoscopy for Adenoma and Polyp Detection: A Systematic Review and Meta-Analysis. Gastrointest Endosc (2021) 93:77–85.e6. doi: 10.1016/j.gie.2020.06.059
9. Chahal D, Byrne MF. A Primer on Artificial Intelligence and its Application to Endoscopy. Gastrointest Endosc (2020) 92:813–20.e4. doi: 10.1016/j.gie.2020.04.074
10. Esteva A, Kuprel B, Novoa RA, Ko J, Swetter SM, Blau HM, et al. Dermatologist-Level Classification of Skin Cancer With Deep Neural Networks. Nature (2017) 542:115–8. doi: 10.1038/nature21056
11. Liang H, Tsui BY, Ni H, Valentim CCS, Baxter SL, Liu G, et al. Evaluation and Accurate Diagnoses of Pediatric Diseases Using Artificial Intelligence. Nat Med (2019) 25:433–8. doi: 10.1038/s41591-018-0335-9
12. Zhu Y, Wang QC, Xu MD, Zhang Z, Cheng J, Zhong YS, et al. Application of Convolutional Neural Network in the Diagnosis of the Invasion Depth of Gastric Cancer Based on Conventional Endoscopy. Gastrointest Endosc (2019) 89:806–15.e1. doi: 10.1016/j.gie.2018.11.011
13. Ebigbo A, Messmann H. Artificial Intelligence in the Upper GI Tract: The Future is Fast Approaching. Gastrointest Endosc (2021) 93:1342–3. doi: 10.1016/j.gie.2021.01.012
14. Whiting PF, Rutjes AW, Westwood ME, Mallett S, Deeks JJ, Reitsma JB, et al. QUADAS-2: A Revised Tool for the Quality Assessment of Diagnostic Accuracy Studies. Ann Intern Med (2011) 155:529–36. doi: 10.7326/0003-4819-155-8-201110180-00009
15. Higgins JP, Thompson SG, Deeks JJ, Altman DG. Measuring Inconsistency in Meta-Analyses. BMJ (2003) 327:557–60. doi: 10.1136/bmj.327.7414.557
16. Cai SL, Li B, Tan WM, Niu XJ, Yu HH, Yao LQ, et al. Using a Deep Learning System in Endoscopy for Screening of Early Esophageal Squamous Cell Carcinoma (With Video). Gastrointest Endosc (2019) 90:745–53.e2. doi: 10.1016/j.gie.2019.06.044
17. de Groof J, van der Sommen F, van der Putten J, Struyvenberg MR, Zinger S, Curvers WL, et al. The Argos Project: The Development of a Computer-Aided Detection System to Improve Detection of Barrett's Neoplasia on White Light Endoscopy. United Eur Gastroenterol J (2019) 7:538–47. doi: 10.1177/2050640619837443
18. de Groof AJ, Struyvenberg MR, Fockens KN, van derPutten J, van derSommen F, Boers TG, et al. Deep Learning Algorithm Detection of Barrett's Neoplasia With High Accuracy During Live Endoscopic Procedures: A Pilot Study (With Video). Gastrointest Endosc (2020) 91:1242–50. doi: 10.1016/j.gie.2019.12.048
19. de Groof AJ, Struyvenberg MR, van der Putten J, van derSommen F, Fockens KN, Curvers WL, et al. Deep-Learning System Detects Neoplasia in Patients With Barrett's Esophagus With Higher Accuracy Than Endoscopists in a Multistep Training and Validation Study With Benchmarking. Gastroenterology (2020) 158:915–29.e4. doi: 10.1053/j.gastro.2019.11.030
20. Ebigbo A, Mendel R, Probst A, Manzeneder J, Prinz F, de Souza LA Jr., et al. Real-Time Use of Artificial Intelligence in the Evaluation of Cancer in Barrett's Oesophagus. Gut (2020) 69:615–6. doi: 10.1136/gutjnl-2019-319460
21. Ebigbo A, Mendel R, Probst A, Manzeneder J, Souza LA, Papa JP, et al. Computer-Aided Diagnosis Using Deep Learning in the Evaluation of Early Oesophageal Adenocarcinoma. Gut (2019) 68:1143–5. doi: 10.1136/gutjnl-2018-317573
22. Mendel R, Ebigbo A, Probst A, Messmann H, Palm C. Barrett’s Esophagus Analysis Using Convolutional Neural Networks. In: Bildverarbeitung Für Die Medizin 2017 (2017). (Berlin, Heidelberg: Springer Vieweg). p. 80–5. doi: 10.1007/978-3-662-54345-0_23
23. Everson M, Herrera L, Li W, Luengo IM, Ahmad O, Banks M, et al. Artificial Intelligence for the Real-Time Classification of Intrapapillary Capillary Loop Patterns in the Endoscopic Diagnosis of Early Oesophageal Squamous Cell Carcinoma: A Proof-of-Concept Study. United Eur Gastroenterol J (2019) 7:297–306. doi: 10.1177/2050640618821800
24. Fukuda H, Ishihara R, Kato Y, Matsunaga T, Nishida T, Yamada T, et al. Comparison of Performances of Artificial Intelligence Versus Expert Endoscopists for Real-Time Assisted Diagnosis of Esophageal Squamous Cell Carcinoma (With Video). Gastrointest Endosc (2020) 92:848–55. doi: 10.1016/j.gie.2020.05.043
25. Ghatwary N, Zolgharni M, Ye X. Early Esophageal Adenocarcinoma Detection Using Deep Learning Methods. Int J Comput Assist Radiol Surg (2019) 14:611–21. doi: 10.1007/s11548-019-01914-4
26. Guo L, Xiao X, Wu C, Zeng X, Zhang Y, Du J, et al. Real-Time Automated Diagnosis of Precancerous Lesions and Early Esophageal Squamous Cell Carcinoma Using a Deep Learning Model (With Videos). Gastrointest Endosc (2020) 91:41–51. doi: 10.1016/j.gie.2019.08.018
27. Iwagami H, Ishihara R, Aoyama K, Fukuda H, Shimamoto Y, Kono M, et al. Artificial Intelligence for the Detection of Esophageal and Esophagogastric Junctional Adenocarcinoma. J Gastroenterol Hepatol (2021) 36:131–6. doi: 10.1111/jgh.15136
28. Li B, Cai SL, Tan WM, Li JC, Yalikong A, Feng XS, et al. Comparative Study on Artificial Intelligence Systems for Detecting Early Esophageal Squamous Cell Carcinoma Between Narrow-Band and White-Light Imaging. World J Gastroenterol (2021) 27:281–93. doi: 10.3748/wjg.v27.i3.281
29. Liu DY, Gan T, Rao NN, Xing YW, Zheng J, Li S, et al. Identification of Lesion Images From Gastrointestinal Endoscope Based on Feature Extraction of Combinational Methods With and Without Learning Process. Med Image Anal (2016) 32:281–94. doi: 10.1016/j.media.2016.04.007
30. Hashimoto R, Requa J, Dao T, Ninh A, Tran E, Mai D, et al. Artificial Intelligence Using Convolutional Neural Networks for Real-Time Detection of Early Esophageal Neoplasia in Barrett's Esophagus (With Video). Gastrointest Endosc (2020) 91:1264–71.e1. doi: 10.1016/j.gie.2019.12.049
31. van der Sommen F, Zinger S, Curvers WL, Bisschops R, Pech O, Weusten BL, et al. Computer-Aided Detection of Early Neoplastic Lesions in Barrett's Esophagus. Endoscopy (2016) 48:617–24. doi: 10.1055/s-0042-105284
32. Wang YK, Syu HY, Chen YH, Chung CS, Tseng YS, Ho SY, et al. Endoscopic Images by a Single-Shot Multibox Detector for the Identification of Early Cancerous Lesions in the Esophagus: A Pilot Study. Cancers (Basel) (2021) 13:321. doi: 10.3390/cancers13020321
33. Yang XX, Li Z, Shao XJ, Ji R, Qu JY, Zheng MQ, et al. Real-Time Artificial Intelligence for Endoscopic Diagnosis of Early Esophageal Squamous Cell Cancer (With Video). Dig Endosc (2021) 33:1075–84. doi: 10.1111/den.13908
34. Wang ZJ GJ, Meng QQ, Yang T, Wang ZY, Chen XC, Wang D, et al. Application of Artificial Intelligence for Automatic Detection of Early Gastric Cancer by Training a Deep Learning Model. Chin J Dig Endosc (2018) 35:6. doi: 10.3760/cma.j.issn.1007-5232.2018.08.004
35. Horiuchi Y, Aoyama K, Tokai Y, Hirasawa T, Yoshimizu S, Ishiyama A, et al. Convolutional Neural Network for Differentiating Gastric Cancer From Gastritis Using Magnified Endoscopy With Narrow Band Imaging. Dig Dis Sci (2020) 65:1355–63. doi: 10.1007/s10620-019-05862-6
36. Ikenoyama Y, Hirasawa T, Ishioka M, Namikawa K, Yoshimizu S, Horiuchi Y, et al. Detecting Early Gastric Cancer: Comparison Between the Diagnostic Ability of Convolutional Neural Networks and Endoscopists. Dig Endosc (2021) 33:141–50. doi: 10.1111/den.13688
37. Kanesaka T, Lee TC, Uedo N, Lin KP, Chen HZ, Lee JY, et al. Computer-Aided Diagnosis for Identifying and Delineating Early Gastric Cancers in Magnifying Narrow-Band Imaging. Gastrointest Endosc (2018) 87:1339–44. doi: 10.1016/j.gie.2017.11.029
38. Li L, Chen Y, Shen Z, Zhang X, Sang J, Ding Y, et al. Convolutional Neural Network for the Diagnosis of Early Gastric Cancer Based on Magnifying Narrow Band Imaging. Gastric Cancer (2020) 23:126–32. doi: 10.1007/s10120-019-00992-2
39. Namikawa K, Hirasawa T, Nakano K, Ikenoyama Y, Ishioka M, Shiroma S, et al. Artificial Intelligence-Based Diagnostic System Classifying Gastric Cancers and Ulcers: Comparison Between the Original and Newly Developed Systems. Endoscopy (2020) 52:1077–83. doi: 10.1055/a-1194-8771
40. Shibata T, Teramoto A, Yamada H, Ohmiya N, Saito K, Fujita H. Automated Detection and Segmentation of Early Gastric Cancer From Endoscopic Images Using Mask R-CNN. Appl Sci (2020) 10:3842. doi: 10.3390/app10113842
41. Tang D, Wang L, Ling T, Lv Y, Ni MH, Zhan Q, et al. Development and Validation of a Real-Time Artificial Intelligence-Assisted System for Detecting Early Gastric Cancer: A Multicentre Retrospective Diagnostic Study. EBioMedicine (2020) 62:103146. doi: 10.1016/j.ebiom.2020.103146
42. Ueyama H, Kato Y, Akazawa Y, Yatagai N, Komori H, Takeda T, et al. Application of Artificial Intelligence Using a Convolutional Neural Network for Diagnosis of Early Gastric Cancer Based on Magnifying Endoscopy With Narrow-Band Imaging. J Gastroenterol Hepatol (2021) 36:482–9. doi: 10.1111/jgh.15190
43. Wu L, He X, Liu M, Xie HP, An P, Zhang J, et al. Evaluation of the Effects of an Artificial Intelligence System on Endoscopy Quality and Preliminary Testing of its Performance in Detecting Early Gastric Cancer: A Randomized Controlled Trial. Endoscopy (2021) 53:1199–207. doi: 10.1055/a-1350-5583
44. Sakai ST Y, Hori K, Nishimura M, Ikematsu H, Yano T, Yokota H. Automatic Detection of Early Gastric Cancer in Endoscopic Images Using a Transferring Convolutional Neural Network. 40th Annu Int Conf IEEE Eng Med Biol Soc (2018), 2018: 4138–41. doi: 10.1109/EMBC.2018.8513274
45. Yoon HJ, Kim S, Kim JH, Keum JS, Oh SI, Jo J, et al. A Lesion-Based Convolutional Neural Network Improves Endoscopic Detection and Depth Prediction of Early Gastric Cancer. J Clin Med (2019) 8:1310. doi: 10.3390/jcm8091310
46. Wu L, Zhou W, Wan X, Zhang J, Shen L, Hu S, et al. A Deep Neural Network Improves Endoscopic Detection of Early Gastric Cancer Without Blind Spots. Endoscopy (2019) 51:522–31. doi: 10.1055/a-0855-3532
47. Zhang L, Zhang Y, Wang L, Wang J, Liu Y. Diagnosis of Gastric Lesions Through a Deep Convolutional Neural Network. Dig Endosc (2020) 33:788–96. doi: 10.1111/den.13844
48. Cho BJ, Bang CS, Park SW, Yang YJ, Seo SI, Lim H, et al. Automated Classification of Gastric Neoplasms in Endoscopic Images Using a Convolutional Neural Network. Endoscopy (2019) 51:1121–9. doi: 10.1055/a-0981-6133
49. Cho BJ, Bang CS, Lee JJ, Seo CW, Kim JH. Prediction of Submucosal Invasion for Gastric Neoplasms in Endoscopic Images Using Deep-Learning. J Clin Med (2020) 9:1858. doi: 10.3390/jcm9061858
50. Hamashima C. Update Version of the Japanese Guidelines for Gastric Cancer Screening. Jpn J Clin Oncol (2018) 48:673–83. doi: 10.1093/jjco/hyy077
51. di Pietro M, Canto MI, Fitzgerald RC. Endoscopic Management of Early Adenocarcinoma and Squamous Cell Carcinoma of the Esophagus: Screening, Diagnosis, and Therapy. Gastroenterology (2018) 154:421–36. doi: 10.1053/j.gastro.2017.07.041
52. Yamazato T, Oyama T, Yoshida T, Baba Y, Yamanouchi K, Ishii Y, et al. Two Years' Intensive Training in Endoscopic Diagnosis Facilitates Detection of Early Gastric Cancer. Intern Med (2012) 51:1461–5. doi: 10.2169/internalmedicine.51.7414
53. Orrù G, Pettersson-Yeo W, Marquand AF, Sartori G, Mechelli A. Using Support Vector Machine to Identify Imaging Biomarkers of Neurological and Psychiatric Disease: A Critical Review. Neurosci Biobehav Rev (2012) 36:1140–52. doi: 10.1016/j.neubiorev.2012.01.004
54. Tang Y, Zheng Y, Chen X, Wang W, Guo Q, Shu J, et al. Identifying Periampullary Regions in MRI Images Using Deep Learning. Front Oncol (2021) 11:674579. doi: 10.3389/fonc.2021.674579
55. Erickson BJ, Korfiatis P, Akkus Z, Kline TL. Machine Learning for Medical Imaging. Radiographics (2017) 37:505–15. doi: 10.1148/rg.2017160130
56. Zhang Q, Wang F, Chen ZY, Wang Z, Zhi FC, Liu SD, et al. Comparison of the Diagnostic Efficacy of White Light Endoscopy and Magnifying Endoscopy With Narrow Band Imaging for Early Gastric Cancer: A Meta-Analysis. Gastric Cancer (2016) 19:543–52. doi: 10.1007/s10120-015-0500-5
57. Zhao YY, Xue DX, Wang YL, Zhang R, Sun B, Cai YP, et al. Computer-Assisted Diagnosis of Early Esophageal Squamous Cell Carcinoma Using Narrow-Band Imaging Magnifying Endoscopy. Endoscopy (2019) 51:333–41. doi: 10.1055/a-0756-8754
58. Horiuchi Y, Hirasawa T, Ishizuka N, Tokai Y, Namikawa K, Yoshimizu S, et al. Performance of a Computer-Aided Diagnosis System in Diagnosing Early Gastric Cancer Using Magnifying Endoscopy Videos With Narrow-Band Imaging (With Videos). Gastrointest Endosc (2020) 92:856–65.e1. doi: 10.1016/j.gie.2020.04.079
Keywords: artificial intelligence, upper gastrointestinal tract, early detection of cancer, endoscopy, systematic review
Citation: Luo D, Kuang F, Du J, Zhou M, Liu X, Luo X, Tang Y, Li B and Su S (2022) Artificial Intelligence–Assisted Endoscopic Diagnosis of Early Upper Gastrointestinal Cancer: A Systematic Review and Meta-Analysis. Front. Oncol. 12:855175. doi: 10.3389/fonc.2022.855175
Received: 14 January 2022; Accepted: 28 April 2022;
Published: 10 June 2022.
Edited by:
William Karnes, University of California, Irvine, United StatesReviewed by:
Bogdan Silviu Ungureanu, University of Medicine and Pharmacy of Craiova, RomaniaPeter Klare, Agatharied Hospital GmbH, Germany
Copyright © 2022 Luo, Kuang, Du, Zhou, Liu, Luo, Tang, Li and Su. This is an open-access article distributed under the terms of the Creative Commons Attribution License (CC BY). The use, distribution or reproduction in other forums is permitted, provided the original author(s) and the copyright owner(s) are credited and that the original publication in this journal is cited, in accordance with accepted academic practice. No use, distribution or reproduction is permitted which does not comply with these terms.
*Correspondence: Song Su, MTM4ODI3Nzg1NTRAMTYzLmNvbQ==
†These authors have contributed equally to this work