- UKM Medical Molecular Biology Institute, Universiti Kebangsaan Malaysia, Cheras, Malaysia
Endometrial cancer (EC) is among the most common gynecological cancers affecting women worldwide. Despite the early detection and rather high overall survival rate, around 20% of the cases recur with poor prognosis. The Next Generation Sequencing (NGS) technology, also known as massively parallel sequencing, symbolizes a high-throughput, fast, sensitive and accurate way to study the molecular landscape of a cancer and this has indeed revolutionized endometrial cancer research. Understanding the potential, advantages, and limitations of NGS will be crucial for the healthcare providers and scientists in providing the genome-driven care in this era of precision medicine and pharmacogenomics. This mini review aimed to compile and critically summarize the recent findings contributed by NGS technology pertaining to EC. Importantly, we also discussed the potential of this technology for fundamental discovery research, individualized therapy, screening of at-risk individual and early diagnosis.
Overview of Endometrial Cancer
Endometrial cancer (EC) ranks as the sixth most frequent cancers among women worldwide with around 320, 000 reported cases and 76, 000 deaths (International Agency for Research on Cancer [Iarc], 2014). This cancer is normally detected early with a relatively high overall survival rate (Sgo Clinical Practice Endometrial Cancer Working Group et al., 2014). However, nearly one fifth of the cases have poor prognosis with a median survival of about 1 year (Obel et al., 2006; Salvesen et al., 2012). Unopposed estrogen therapy, estrogen producing tumors, tamoxifen, obesity, nulliparity, diabetes mellitus, and early onset of menstruation are among the risk factors associated with EC (Brinton et al., 2005).
Bokhman (1983) was the first to propose the pathogenetic dualistic model of two different types of endometrial carcinoma, named as type I and type II. Type I endometrioid endometrial cancer (EEC) is driven by estrogen and represents most of sporadic cases (Bansal et al., 2009). EEC typically occurs in premenopausal and younger postmenopausal women who often diagnosed with low-grade well-differentiated tumor thus carrying a better prognosis (Soliman et al., 2005; Garg and Soslow, 2014). On the contrary, the type II non-endometrioid endometrial carcinoma (NEEC) accounts for only 10–20% of sporadic endometrial carcinoma with no underlying estrogen exposure (Doll et al., 2008). NEEC is commonly diagnosed in older postmenopausal women, who typically present with advanced-stage disease and poor prognosis (Amant et al., 2005). It is also associated with high mortality and reduced survival rates (Hamilton et al., 2006; Mendivil et al., 2009). This classification is imperfect as the minority of EC characteristic of both groups may overlap because of heterogeneity of this disease, especially the high grade EEC (Murali et al., 2014). Characterization of molecular landscapes will give insights into tumor classification, which may influence treatment recommendations and provides prospects for precision medicine. Therefore, there is a need to explore the molecular landscape of EC treatment using the next generation sequencing (NGS) approaches.
Lynch Syndrome (LS) is a hereditary cancer syndrome caused by germline alterations in the DNA mismatch repair (MMR) genes (Tafe et al., 2014). Those with LS will have an increased risk of colon cancer. Despite being overlooked in association with LS, individuals with this syndrome also has 20–60% risk of developing EC (Tafe et al., 2014). The mutation frequencies of MMR genes are: 50–66% in MSH2, 24–40% in MLH1, 10–13% in MSH6 and <5% in PMS2 (Wang et al., 2013).
Overview of Next Generation Sequencing (NGS) Technology
The emergence of NGS three decades after Sanger sequencing represents the potential to dramatically revolutionize biomedical research by enabling the high throughput comprehensive analysis of genomes and transcriptomes at an inexpensive scale (Shendure and Ji, 2008). Compared with Sanger sequencing, NGS technologies offer extraordinarily high throughput capacity which reduces cost per base, time and has enabled the discovery of both common and rare variants with a much deeper sequencing read coverage (Koboldt et al., 2013). NGS is also a versatile technology which enables various applications including whole genome sequencing (WGS) for model and non-model organisms (Ng and Kirkness, 2010), whole exome sequencing (Rabbani et al., 2014), targeted resequencing (Meldrum et al., 2011) as well as analysis of coding and non-coding RNA expression, alternative splicing and discovery of novel non-coding RNAs (Wang et al., 2009). In addition, while 30× coverage for WGS is considered as the standard (Rehm et al., 2013), low-pass WGS with coverage less than 10× had also been used to assess structural variation (Cancer Genome Atlas Research Network et al., 2013).
Over the past 4 years, NGS technologies were applied in EC research (Table 1). NGS has promoted and improved the detection of key types of molecular alterations such as single nucleotide substitutions, small insertions, and deletions, copy number alterations, structural variations and novel transcripts. New drug targets were identified thus providing the oncologists with various potential options in treating EC patients (Figure 1). Here, we provide an overview of the use of NGS for subtype classification, identification of potential diagnostic biomarkers and screening of therapeutic targets for personalized treatment of EC.
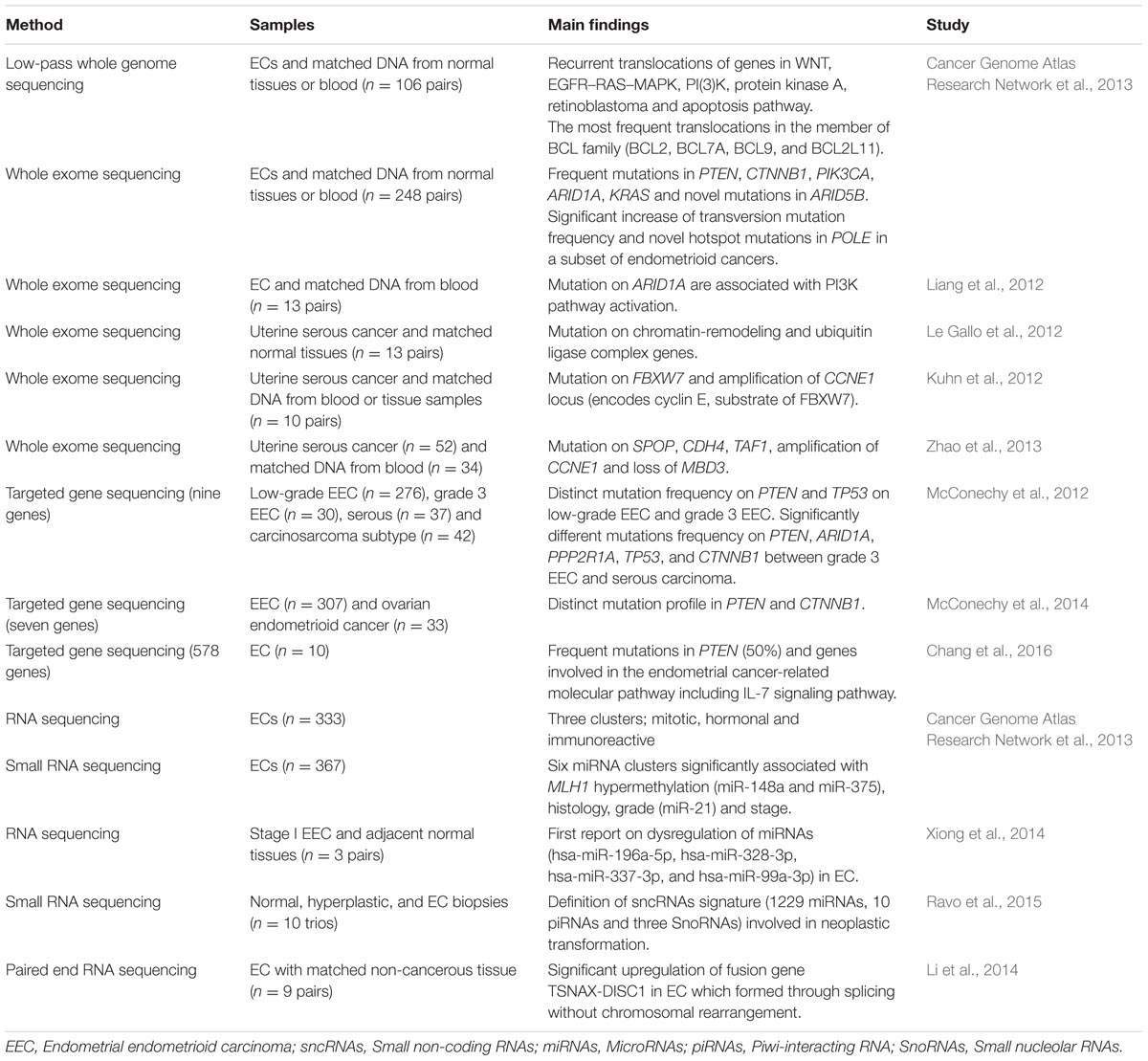
TABLE 1. Main findings contributed by Next Generation Sequencing (NGS) in endometrial cancers (ECs).
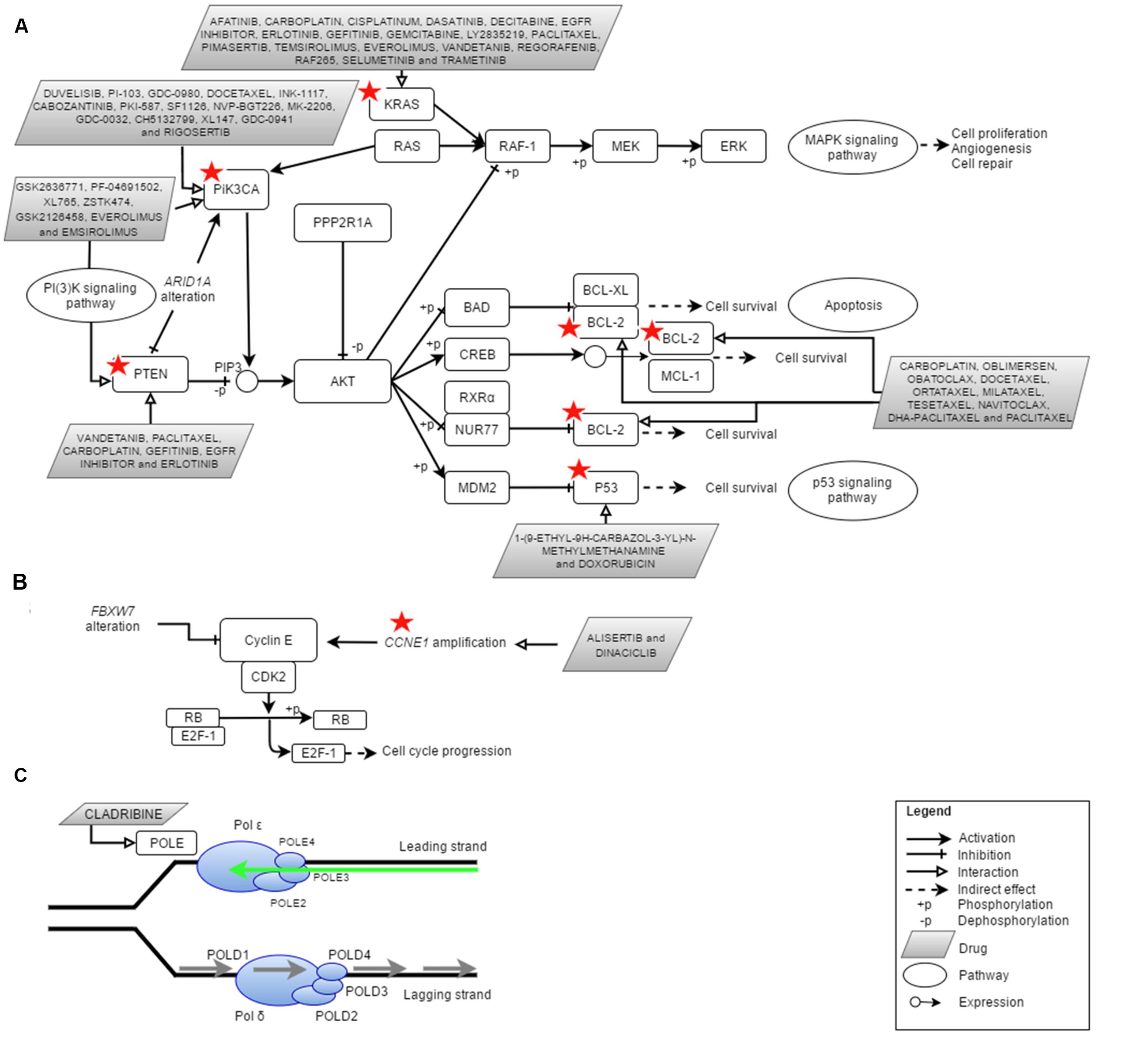
FIGURE 1. Cellular pathways currently being targeted in the treatment of endometrial cancer (EC) and the potential actionable genes in EC identified via Next Generation Sequencing (NGS). Red star depicted genes with clinically cancer-relevant drug interactions as defined by DGIdb (Wagner et al., 2016). (A) PI(3)K, MAPK, p53 and apoptosis pathway involving PIK3CA, PTEN, PPP2R1A, KRAS, P53, and BCL2, (B) cell cycle pathway involving FBXW7 and CCNE1 as well as (C) DNA replication pathway in eukaryote which involves POLE (DNA polymerase 𝜀). Figures were adapted from Kanehisa et al. (2016) and Seshagiri (2013). Adapted by permission from Macmillan Publishers Ltd: [NATURE GENETICS] (Seshagiri), copyright (2013).
Whole genome sequencing provides a comprehensive view of the cancer genome including all types of somatic/germline mutations, nucleotide substitutions, small insertions and deletions, copy number variations, chromosomal rearrangements, as well as analysis of the non-coding regions (Meyerson et al., 2010). Since WGS is still expensive, laborious and produces a massive amount of data for analysis and interpretation, whole exome sequencing (WES) which concentrates only on the protein-coding exon of human genome is a preferred alternative (Rabbani et al., 2014).
However, despite being high throughput, most of the information obtained from WGS and WES are functionally unclear and the genetic alterations could be just possible passenger mutations with unknown clinical significance (Meldrum et al., 2011). Besides, WGS and WES are unsuitable for clinical application because of relatively lower read depth and this later limit the identification of low-allelic-fraction single nucleotide polymorphisms (SNPs) which is important for early diagnosis, prevention of drug resistance and residual tumor detection (Xu et al., 2014). Therefore, deep sequencing of targeted gene provides better alternative in clinical setting due to several advantages including higher coverage for increased analytical sensitivity and specificity, generate feasible and interpretable data, significant cost reduction, and shorter turnaround time (Xu et al., 2014). Targeted gene sequencing also has proven useful for a large number of applications including studying disease-relevant gene subsets, diagnostic testing for hereditary disorders and therapeutic decision-making for somatic cancers (Rehm, 2013).
Since last decade, EC transcriptomes have been dominantly investigated using hybridization-based microarray techniques (Maxwell et al., 2005; Cohn et al., 2010; Ratner et al., 2010). The advent of NGS has also revolutionized cancer transcriptomics studies. RNA and miRNA sequencing have rapidly emerged as powerful tools for genome wide expression profiling and offer several advantages over conventional microarray.
Whole Genome and Whole Exome Sequencing
To date, there is only one publication in EC by The Cancer Genome Atlas (TCGA) group which utilized WGS to characterize the chromosomal aberration in 106 ECs (Cancer Genome Atlas Research Network et al., 2013). Recurrent translocations of genes in important cancer pathways including WNT, EGFR–RAS–MAPK, PI3K, protein kinase A, retinoblastoma and apoptosis were identified (Cancer Genome Atlas Research Network et al., 2013). The most frequent translocations were discovered in a member of the BCL family which were BCL2, BCL7A, BCL9, and BCL2L11 (Cancer Genome Atlas Research Network et al., 2013). This large-scale consortium also performedWES on 248 ECs and identified novel POLE hotspot mutations (Pro286Arg and Val411Leu) in 13 of the 17 ultra-mutated samples (Cancer Genome Atlas Research Network et al., 2013). In addition, PTEN, PIK3R1, PIK3CA, FBXW7, and KRAS were found to be significantly mutated. This was the largest genomic characterization of EC so far and their findings propose a reclassification that might assist in personalized treatment for patients with aggressive phenotype.
So far there are four published EC studies utilizing WES (Table 1). Liang et al. (2012) was the first to perform WES in 13 ECs with matched normal DNA. In combination with functional genomics, the authors identified 12 potential driver genes including 10 tumor suppressor genes (ARID1A, INHBA, KMO, TTLL5, GRM8, IGFBP3, AKTIP, PHKA2, TRPS1, and WNT11) and two oncogenes (ERBB3 and RPS6KC1). Concentrating on ARID1A, mutation profiles were integrated with functional proteomics in additional 222 EC samples, demonstrating the role of ARID1A as a novel regulator of PI3K pathway activity (Liang et al., 2012).
While Liang et al. (2012) characterized the exomes of ECs without stratification of grade and histological subtypes, another group (Le Gallo et al., 2012) focused on the rare but aggressive serous carcinoma subtype of EC which has high recurrence rate, poor survival and more likely to present with metastatic disease due to resistance to chemotherapy (Hamilton et al., 2006). Le Gallo et al. (2012) performed WES on 13 matched pairs of serous ECs and normal tissues. In addition to confirming the existence of alterations in TP53, PIK3CA and PPP2R1A, they also identified high frequency of somatic alterations in novel genes involved in serous ECs which were FBXW7, CHD4, SPOP, MAP3K4, ABCC9, and CYP4X1 (Le Gallo et al., 2012). Chromatin remodeling and ubiquitin ligase complex pathways were found to be the most perturbed pathways which have been associated with frequent alterations in set of 11 chromatin remodeling genes including CHD4 gene and component of ubiquitin ligase complex (FBXW7 and SPOP gene), respectively (Le Gallo et al., 2012).
In the same year, Kuhn et al. (2012) performed WES on 10 uterine serous carcinomas with matched normal blood or tissue samples. In addition to confirming the previous discovery by Le Gallo et al. (2012), they also identified high mutation frequencies of FBXW7 and genes known to be associated with serous EC including TP53, PIK3CA, and PPP2R1A. DNA copy number analysis revealed concurrent frequent genomic amplification of the CCNE1 with FBXW7 mutations, suggesting that these genes are involved in same signaling pathway (Kuhn et al., 2012). They also proposed the role of endometrial intraepithelial carcinoma as a precursor to serous carcinoma whereby nine cases of serous carcinoma with an associated serous endometrial intraepithelial carcinoma had concordant PIK3CA, PP2R1A, and TP53 mutations (Kuhn et al., 2012). Taken together, the authors presented molecular genetic alterations involving the p53, cyclin E-FBXW7 and PI3K pathways as the major mechanisms in the uterine serous carcinoma progression.
The WES findings from both rather underpowered studies by Kuhn et al. (2012) and Le Gallo et al. (2012) were later supported by Zhao et al. (2013) using five times more samples. Fifty two uterine serous cancer patients of which 34 had matched normal tissue samples were subjected to WES (Zhao et al., 2013). In addition to earlier published studies which identified frequent alterations in TP53, PIK3CA, PPPR1A, and FBXW7, Zhao et al. (2013) also discovered high frequency mutations of the SPOP gene which targets the protein for ubiquitination. Interestingly, this study also reported alterations in TF1 gene, an element of the core TFIID transcriptional machinery which may result in overexpression of cyclin D and subsequently promote cell cycle progression and proliferation (Zhao et al., 2013). Frequent TP53 deletions and amplifications of chromosome segments containing PIK3CA and CCNE1 are known to be targets of FBXW7 (Kuhn et al., 2012). This study also discovered overexpression of ERBB2 which act as an upstream regulator of PIK3CA/AKT/mTOR signaling pathway and loss of MBD3 gene function, a member of the NuRD-chromatin-modification complex (Zhao et al., 2013). To summarize, all of these studies which employed WGS and WES reported specific pathways that are frequently mutated in ECs including DNA damage, chromatin remodeling, cell cycle, and cell proliferation pathways.
Targeted Gene Sequencing
Two different studies by McConechy et al. (2012) took on to improve the controversial classification among pathological subtypes endometrial carcinoma (endometrioid, serous, carcinosarcoma, mixed, and clear cell) and between histological endometrioid types of EC and ovarian cancer using a panel of genes related to both cancers. The first study involved targeted enrichment sequencing on a large cohort of 393 EC samples using a nine-gene panel: ARID1A, PPP2R1A, PTEN, PIK3CA, KRAS, CTNNB1, TP53, BRAF and PPP2R5C (McConechy et al., 2012). Both low-grade and grade 3 endometrioid EC demonstrated a similar pattern of high frequency of mutations in PTEN, PIK3CA, ARID1A, KRAS, and CTNNB1 (McConechy et al., 2012). There is a significant increase in TP53 mutation frequency in grade 3 endometrioid EC when compared to low-grade EC (McConechy et al., 2012). ARID1A mutation was significantly associated with concurrent PTEN and PIK3CA mutations in both low-grade endometrioid EC and grade 3 endometrioid ECs, suggesting a cooperative role in endometrioid carcinogenesis (McConechy et al., 2012). However, this phenomenon was not observed in endometrial serous carcinomas which have frequent mutations in TP53 and PPP2R1A but lack mutations in PTEN, ARID1A, and CTNBB1. On the other hand, in carcinosarcoma, two distinct mutation profiles have been identified which were the endometrioid type (PTEN, PIK3CA, ARID1A, and KRAS mutations) and serous type (TP53 and PPP2R1A; McConechy et al., 2012).
Using a panel of seven well-characterized genes in ECs (ARID1A, PTEN, PIK3CA, KRAS, CTNNB1, PPP2R1A, and TP53), McConechy’s research group compared two morphologically similar cancer types, endometrial endometrioid carcinoma (n = 307) and ovarian endometrioid carcinoma (n = 33) using exon capture sequencing (McConechy et al., 2014). Higher frequency of mutations was discovered in low-grade endometrial endometrioid carcinoma (67%) compared to low-grade ovarian endometrioid carcinoma (17%; McConechy et al., 2014). The frequency of CTNNB1 mutation was significantly higher in ovarian endometrioid cancer (53%) when compared to endometrioid EC (28%). However, mutations frequencies in other genes were not significantly different between both cancers. They hypothesized that the different mutation spectrum in both cancers might be because of different exposures to the microenvironment during carcinogenesis, whereby the ovarian endometrioid carcinoma thrives in a highly oxidative environment that promotes tumorigenesis (Kobayashi et al., 2009). Consistent with other previously discussed studies, distinct mutation patterns in PI3K and WNT signaling pathways were identified, suggesting therapeutic opportunities for treatment of EC (McConechy et al., 2014).
The most recently published endometrial research using NGS involved 10 Taiwanese EC patients (Chang et al., 2016). The exomes of 578 cancer-related genes were captured and deep-sequenced using NGS, resulting in average of 500× coverage. This study revealed 120 variants in 99 genes, with 50% of the ECs harboring mutations in the PTEN (Chang et al., 2016). Molecular aberrations in PIK3R1, AKT2 and FOXO1 that could led to putative activation of the IL-7 signaling pathway and which has not been previously linked with EC, are also reported.
Targeted NGS represents a potential to be adopted in clinical laboratory practices and have diagnostic applications for screening of individual at risk for developing EC (Tafe, 2015). To date, there are seven NGS-based assays that include LS-associated genes offered by several reference laboratories including Ambry Genetics (ColoNext; 14 genes), ARUP (Gastrointestinal Hereditary Cancer Panel; 15 genes), Baylor Miraca Genetics Laboratories (High Risk Hereditary Colorectal Cancer Panel; 12 genes), GeneDx (OncoGeneDx: Lynch/Colorectal Cancer High Risk Panel; seven genes), Mayo Medical laboratories (Hereditary Colon Cancer Multi-Gene Panel; 17 genes), Myriad (myRiskTM Hereditary Cancer Panel; 25 genes) and University of Washington (ColoSeqTM; 23 genes; Tafe, 2015). However, the important challenges of NGS include interpreting incidental findings, genetic counseling, and getting informed consent from the patients. These calls for development of clinical practice guidelines for test development, validation, reporting, and reporting of incidental findings (Tafe, 2015).
RNA and miRNA Sequencing
Via RNA sequencing and unsupervised k-means clustering of 333 ECs, Cancer Genome Atlas Research Network et al. (2013) identified three robust clusters which were mitotic, hormonal and immunoreactive subtypes. The mitotic subtype was characterized by TP53 alteration and mostly comprised of serous/mixed histology and endometrioid grade 3 while both of hormonal and immunoreactive subtypes were predominantly comprised of endometrioid grade 1/2 and PTEN mutated patients (Cancer Genome Atlas Research Network et al., 2013). Interestingly, the hormonal subtype exhibited upregulation of hormone related genes (ESR1, PGR and downstream targets) which could make the patients more responsive to hormonal therapy (Cancer Genome Atlas Research Network et al., 2013), implying potential for individualized treatment. In addition, the group performed unsupervised consensus clustering of miRNA expression profiles in 367 ECs and discovered six clusters which some of them were significantly associated with MLH1 hypermethylation (miR-148a and miR-375), histology, grade (miR-21) and stage.
Xiong et al. (2014) simultaneously characterized the transcriptome of both mRNAs and miRNAs using RNA sequencing on three pairs of stage 1 endometrioid EC and adjacent non-cancerous tissue (Xiong et al., 2014). By integrating expression data of mRNAs and miRNAs, they identified a total of 438 target pairs which were inversely correlated including 320 dysregulated genes. Downstream pathway enrichment analysis revealed six differently expressed miRNAs (hsa-let-7c-5p, hsa-miR-196a-5p, hsa-miR-328-3p, hsa-miR-337-3p, and hsa-miR-99a-3p, hsa-miR-181c-3p) targeting 11 differently expressed genes (E2F5, CDKN2A, CCNA2, TP53, BUB1B, CCNE1, CDK1, MCM4, SKP2, CDC6 and TGFB3) in the cell cycle pathway (Xiong et al., 2014).
A genome wide characterization of small non-coding RNAs (sncRNAs) in EC carcinogenesis was performed on biopsies of normal (n = 10), hyperplastic (n = 6) and tumor Type 1 endometrial tissues (n = 10) using RNA sequencing (Ravo et al., 2015). Significant patterns in sncRNA expression between the sample groups were identified and led to the discovery of sncRNAs signature (129 miRNAs, 10 piRNAs, and three snoRNAs) which is said to be involved in neoplastic transformation (Ravo et al., 2015). Integrated gene expression profiling with the aberrantly expressed sncRNAs signature revealed their involvement in multiple signaling pathway including ERK/MAPK, TGF-β and Wnt/β-catenin in both hyperplastic and neoplastic tissues (Ravo et al., 2015).
Gene fusion refers to an aberrant rearrangement between two genes which encode a new fusion protein, serving as a strong driver mutation in cancer (Mertens et al., 2015). Through pair-end RNA sequencing in nine pairs of EC and matched non-cancerous tissues, a fusion gene called chimeric translin-associated X-disrupted-in-schizophrenia (TSNAX-DISC1) in EC was discovered (Li et al., 2014). This fusion transcript was formed by intragenic splicing and its expression was further validated in 176 paired ECs and matched non-cancerous tissues. Dysregulation of TSNAX is presumed to be associated with cancer and the authors proposed the potential of TSNAX–DISC1 as an EC biomarker (Li et al., 2014).
Conclusion
In this mini review, we compiled and concisely review the literatures using NGS in basic EC research. NGS has indeed revolutionized EC genomics by enabling discovery of the major alterations in the genome which could serve as potential biomarkers for prognosis and drug development. The discovery of these biomarkers by NGS has the potential to accelerate application of genome-guided information into precision medicine and pharmacogenomics. Despite limited publications so far, NGS assays are already actively adopted for routine clinical testing in molecular pathology laboratories for identification of individual at risk of developing EC.
Author Contributions
S-SS and N-SA drafted this manuscript. N-SA and RJ were responsible for idea conception, critical evaluation and manuscript review.
Funding
This study is funded by Universiti Kebangsaan Malaysia Research Grant (Arus Perdana AP-2012-011).
Conflict of Interest Statement
The authors declare that the research was conducted in the absence of any commercial or financial relationships that could be construed as a potential conflict of interest.
References
Amant, F., Moerman, P., Neven, P., Timmerman, D., Van Limbergen, E., and Vergote, I. (2005). Endometrial cancer. Lancet 366, 491–505. doi: 10.1016/S0140-6736(05)67063-8
Bansal, N., Yendluri, V., and Wenham, R. M. (2009). The molecular biology of endometrial cancers and the implications for pathogenesis, classification, and targeted therapies. Cancer Control 16, 8–13.
Bokhman, J. V. (1983). Two pathogenetic types of endometrial carcinoma. Gynecol. Oncol. 15, 10–17. doi: 10.1016/0090-8258(83)90111-7
Brinton, L. A., Lacey, J. V. Jr., and Trimble, E. L. (2005). Hormones and endometrial cancer–new data from the Million Women Study. Lancet 365, 1517–1518. doi: 10.1016/S0140-6736(05)66431-8
Cancer Genome Atlas Research Network, Kandoth, C., Schultz, N., Cherniack, A. D., Akbani, R., Liu, Y., et al. (2013). Integrated genomic characterization of endometrial carcinoma. Nature 497, 67–73. doi: 10.1038/nature12113
Chang, Y. S., Huang, H. D., Yeh, K. T., and Chang, J. G. (2016). Genetic alterations in endometrial cancer by targeted next-generation sequencing. Exp. Mol. Pathol. 100, 8–12. doi: 10.1016/j.yexmp.2015.11.026
Cohn, D. E., Fabbri, M., Valeri, N., Alder, H., Ivanov, I., Liu, C. G., et al. (2010). Comprehensive miRNA profiling of surgically staged endometrial cancer. Am. J. Obstet. Gynecol. 202, 656e1–656e8. doi: 10.1016/j.ajog.2010.02.051
Doll, A., Abal, M., Rigau, M., Monge, M., Gonzalez, M., Demajo, S., et al. (2008). Novel molecular profiles of endometrial cancer–new light through old windows. J. Steroid Biochem. Mol. Biol. 108, 221–229. doi: 10.1016/j.jsbmb.2007.09.020
Garg, K., and Soslow, R. A. (2014). Endometrial carcinoma in women aged 40 years and younger. Arch. Pathol. Lab. Med. 138, 335–342. doi: 10.5858/arpa.2012-0654-RA
Hamilton, C. A., Cheung, M. K., Osann, K., Chen, L., Teng, N. N., Longacre, T. A., et al. (2006). Uterine papillary serous and clear cell carcinomas predict for poorer survival compared to grade 3 endometrioid corpus cancers. Br. J. Cancer 94, 642–646. doi: 10.1038/sj.bjc.6603012
International Agency for Research on Cancer [Iarc]. (2014). World Cancer Report 2014, Chapter 5.12. Geneva: World Health Organization.
Kanehisa, M., Sato, Y., Kawashima, M., Furumichi, M., and Tanabe, M. (2016). KEGG as a reference resource for gene and protein annotation. Nucleic Acids Res. 44, D457–D462. doi: 10.1093/nar/gkv1070
Kobayashi, H., Kajiwara, H., Kanayama, S., Yamada, Y., Furukawa, N., Noguchi, T., et al. (2009). Molecular pathogenesis of endometriosis-associated clear cell carcinoma of the ovary (Review). Oncol. Rep. 22, 233–240.
Koboldt, D. C., Steinberg, K. M., Larson, D. E., Wilson, R. K., and Mardis, E. R. (2013). The next-generation sequencing revolution and its impact on genomics. Cell 155, 27–38. doi: 10.1016/j.cell.2013.09.006
Kuhn, E., Wu, R. C., Guan, B., Wu, G., Zhang, J., Wang, Y., et al. (2012). Identification of molecular pathway aberrations in uterine serous carcinoma by genome-wide analyses. J. Natl. Cancer Inst. 104, 1503–1513. doi: 10.1093/jnci/djs345
Le Gallo, M., O’Hara, A. J., Rudd, M. L., Urick, M. E., Hansen, N. F., O’Neil, N. J., et al. (2012). Exome sequencing of serous endometrial tumors identifies recurrent somatic mutations in chromatin-remodeling and ubiquitin ligase complex genes. Nat. Genet. 44, 1310–1315. doi: 10.1038/ng.2455
Li, N., Zheng, J., Li, H., Deng, J., Hu, M., Wu, H., et al. (2014). Identification of chimeric TSNAX-DISC1 resulting from intergenic splicing in endometrial carcinoma through high-throughput RNA sequencing. Carcinogenesis 35, 2687–2697. doi: 10.1093/carcin/bgu201
Liang, H., Cheung, L. W., Li, J., Ju, Z., Yu, S., Stemke-Hale, K., et al. (2012). Whole-exome sequencing combined with functional genomics reveals novel candidate driver cancer genes in endometrial cancer. Genome Res. 22, 2120–2129. doi: 10.1101/gr.137596.112
Maxwell, G. L., Chandramouli, G. V. R., Dainty, L., Litzi, T. J., Berchuck, A., Barrette, J. C., et al. (2005). Microarray analysis of endometrial carcinomas and mixed mullerian tumors reveals distinct gene expression profiles associated with different histologic types of uterine cancer. Clin. Cancer Res. 11, 4056–4066. doi: 10.1158/1078-0432.CCR-04-2001
McConechy, M. K., Ding, J., Cheang, M. C., Wiegand, K. C., Senz, J., Tone, A. A., et al. (2012). Use of mutation profiles to refine the classification of endometrial carcinomas. J. Pathol. 228, 20–30. doi: 10.1186/1471-2164-15-244
McConechy, M. K., Ding, J., Senz, J., Yang, W., Melnyk, N., Tone, A. A., et al. (2014). Ovarian and endometrial endometrioid carcinomas have distinct CTNNB1 and PTEN mutation profiles. Mod. Pathol. 27, 128–134. doi: 10.1038/modpathol.2013.107
Meldrum, C., Doyle, M. A., and Tothill, R. W. (2011). Next-generation sequencing for cancer diagnostics: a practical perspective. Clin. Biochem. Rev. 32, 177–195.
Mendivil, A., Schuler, K. M., and Gehrig, P. A. (2009). Non-endometrioid adenocarcinoma of the uterine corpus: a review of selected histological subtypes. Cancer Control 6, 46–52.
Mertens, F., Johansson, B., Fioretos, T., and Mitelman, F. (2015). The emerging complexity of gene fusions in cancer. Nat. Rev. Cancer 5, 371–381. doi: 10.1038/nrc3947
Meyerson, M., Gabriel, S., and Getz, G. (2010). Advances in understanding cancer genomes through second-generation sequencing. Nat. Rev. Genet. 11, 685–696. doi: 10.1038/nrg2841
Murali, R., Soslow, R. A., and Weight, B. (2014). Classification of endometrial carcinoma: more than two types. Lancet Oncol. 15, e268–e278. doi: 10.1016/S1470-2045(13)70591-6
Ng, P. C., and Kirkness, E. F. (2010). Whole genome sequencing. Methods Mol. Biol. 628, 215–226. doi: 10.1007/978-1-60327-367-1_12
Obel, J. C., Friberg, G., and Fleming, G. F. (2006). Chemotherapy in endometrial cancer. Clin. Adv. Hematol. Oncol. 4, 459–468.
Rabbani, B., Tekin, M., and Mahdieh, N. (2014). The promise of whole-exome sequencing in medical genetics. J. Hum. Genet. 59, 5–15. doi: 10.1038/jhg.2013.114
Ratner, E. S., Tuck, D., Richter, C., Nallur, S., Patel, R. M., Schultz, V., et al. (2010). MicroRNA signatures differentiate uterine cancer tumor subtypes. Gynecol. Oncol. 118, 251–257. doi: 10.1016/j.ygyno.2010.05.010
Ravo, M., Cordella, A., Rinaldi, A., Bruno, G., Alexandrova, E., Saggese, P., et al. (2015). Small non-coding RNA deregulation in endometrial carcinogenesis. Oncotarget. 6, 4677–4691. doi: 10.18632/oncotarget.2911
Rehm, H. L. (2013). Disease-targeted sequencing: a cornerstone in the clinic. Nat. Rev. Genet. 4, 295–300. doi: 10.1038/nrg3463
Rehm, H. L., Bale, S. J., Bayrak-Toydemir, P., Berg, J. S., Brown, K. K., Deignan, J. L., et al. (2013). ACMG clinical laboratory standards for next-generation sequencing. Genet. Med. 15, 733–747. doi: 10.1038/gim.2013.92
Salvesen, H. B., Haldorsen, I. S., and Trovik, J. (2012). Markers for individualised therapy in endometrial carcinoma. Lancet. Oncol. 13, e353–e361. doi: 10.1016/S1470-2045(12)70213-9
Seshagiri, S. (2013). The burden of faulty proofreading in colon cancer. Nat. Genet. 45, 121–122. doi: 10.1038/ng.2540
Sgo Clinical Practice Endometrial Cancer Working Group, Burke, W. M., Orr, J., Leitao, M., Salom, E., Gehrig, P., et al. (2014). Society of gynecologic oncology clinical practice committee. Endometrial cancer: a review and current management strategies: part I. Gynecol. Oncol. 134, 385–392. doi: 10.1016/j.ygyno.2014.05.018
Shendure, J., and Ji, H. (2008). Next-generation DNA sequencing. Nat. Biotechnol. 26, 1135–1145. doi: 10.1038/nbt1486
Soliman, P. T., Oh, J. C., Schmeler, K. M., Sun, C. C., Slomovitz, B. M., Gershenson, D. M., et al. (2005). Risk factors for young premenopausal women with endometrial cancer. Obstet. Gynecol. 105, 575–580. doi: 10.1097/01.AOG.0000154151.14516.f7
Tafe, L. J. (2015). Targeted next-generation sequencing for hereditary cancer syndromes: a focus on lynch syndrome and associated endometrial cancer. J. Mol. Diagn. 17, 472–482. doi: 10.1016/j.jmoldx.2015.06.001
Tafe, L. J., Riggs, E. R., and Tsongalis, G. J. (2014). Lynch syndrome presenting as endometrial cancer. Clin. Chem. 60, 111–121. doi: 10.1373/clinchem.2013.206888
Wagner, A. H., Coffman, A. C., Ainscough, B. J., Spies, N. C., Skidmore, Z. L., Campbell, K. M., et al. (2016). DGIdb 2.0: mining clinically relevant drug-gene interactions. Nucleic Acids Res. 44, D1036–D1044. doi: 10.1093/nar/gkv1165
Wang, Y., Wang, Y., Li, J., Cragun, J., Hatch, K., Chambers, S. K., et al. (2013). Lynch syndrome related endometrial cancer: clinical significance beyond the endometrium. J. Hematol. Oncol. 6, 22. doi: 10.1186/1756-8722-6-22
Wang, Z., Gerstein, M., and Snyder, M. (2009). RNA-Seq: a revolutionary tool for transcriptomics. Nat. Rev. Genet. 101, 57–63. doi: 10.1038/nrg2484
Xiong, H., Li, Q., Liu, S., Wang, F., Xiong, Z., Chen, J., et al. (2014). Integrated microRNA and mRNA Transcriptome sequencing reveals the potential roles of miRNAs in stage I endometrioid endometrial carcinoma. PLoS ONE 9:e110163. doi: 10.1371/journal.pone.0110163
Xu, H., DiCarlo, J., Satya, R. V., Peng, Q., and Wang, Y. (2014). Comparison of somatic mutation calling methods in amplicon and whole exome sequence data. BMC Genomics 15:244. doi: 10.1186/1471-2164-15-244
Keywords: next generation sequencing, endometrial cancer, molecular landscape, precision medicine, screening, early diagnosis
Citation: Suhaimi S-S, Ab Mutalib N-S and Jamal R (2016) Understanding Molecular Landscape of Endometrial Cancer through Next Generation Sequencing: What We Have Learned so Far? Front. Pharmacol. 7:409. doi: 10.3389/fphar.2016.00409
Received: 05 August 2016; Accepted: 14 October 2016;
Published: 01 November 2016.
Edited by:
Massimo Libra, University of Catania, ItalyReviewed by:
Vincenzo Bramanti, Azienda Ospedaliera Ospedali Riuniti “Villa Sofia - Cervello” - Palermo, ItalySilvana Canevari, Istituto Nazionale dei Tumori – Istituto di Ricovero e Cura a Carattere Scientifico, Italy
Copyright © 2016 Suhaimi, Ab Mutalib and Jamal. This is an open-access article distributed under the terms of the Creative Commons Attribution License (CC BY). The use, distribution or reproduction in other forums is permitted, provided the original author(s) or licensor are credited and that the original publication in this journal is cited, in accordance with accepted academic practice. No use, distribution or reproduction is permitted which does not comply with these terms.
*Correspondence: Nurul-Syakima Ab Mutalib, c3lha2ltYUBwcHVrbS51a20uZWR1Lm15 Rahman Jamal, cmFobWFuakBwcHVrbS51a20uZWR1Lm15
†These authors shared first co-authorship.