- Tianjin Key Laboratory for Modern Drug Delivery & High-Efficiency, School of Pharmaceutical Science and Technology, Tianjin University, Tianjin, China
Diabetes mellitus (DM) is a kind of chronic and metabolic disease, which can cause a number of diseases and severe complications. Network pharmacology approach is introduced to study DM, which can combine the drugs, target proteins and disease and form drug-target-disease networks. Network pharmacology has been widely used in the studies of the bioactive compounds and action mechanisms of natural products for the treatment of DM due to the multi-components, multi-targets, and lower side effects. This review provides a balanced and comprehensive summary on network pharmacology from current studies, highlighting different bioactive constituents, related databases and applications in the investigations on the treatment of DM especially type 2. The mechanisms related to type 2 DM, including α-amylase and α-glucosidase inhibitory, targeting β cell dysfunction, AMPK signal pathway and PI3K/Akt signal pathway are summarized and critiqued. It suggests that the network pharmacology approach cannot only provide a new research paradigm for natural products, but also improve the current antidiabetic drug discovery strategies. Furthermore, we put forward the perspectives on the reasonable applications of network pharmacology for the therapy of DM and related drug discovery.
Introduction
Diabetes mellitus (DM) has drawn much attention of researchers due to its increasing mortality and complex complications. According to the International Diabetes Federation, DM has become a major threat to the health and the third biggest killer after cardiovascular diseases and cancer1. Now DM has terrible influences on both high- and low-income countries, with bearing the majority of the burden in low-income countries. According to the latest statistics of WHO, DM directly caused 1.5 million deaths in 2012. There were approximately 422 million (1 in every 11 people) diabetics worldwide in 2014, which was 14-fold more than those in 19802. And the cases will reach 600 million by 2030 based on the developing trend (Kokil et al., 2015).
Diabetes mellitus is a group of chronic and metabolic diseases, in which there are high blood glucose levels over a long period. It is caused by either the body can’t produce enough insulin or the body can’t effectively respond to the produced insulin. Based on different pathogenesis, DM can be categorized into three types. Type 1 diabetes mellitus (T1DM), namely insulin-dependent DM or childhood-onset diabetes, is caused by insulin deficiency, which is related to dysfunction β cells (Enk and Mandelboim, 2014). Type 2 diabetes mellitus (T2DM), namely non-insulin-dependent DM or adult-onset diabetes, is characterized by insulin resistance (IR) and relatively insulin secretion reducing. There is another type of the diabetes, gestational diabetes mellitus (GDM), which is characterized by high blood glucose levels in pregnant women without previously diagnosed diabetes (Keller et al., 2008; Puri and Hebrok, 2012). According to clinical and non-clinical studies, T2DM is the main category accounting for about 90% of diabetes cases, which is also the focus of this review. The risk factors for T2DM are relatively clear including age, obesity, lifestyle, dietary patterns gene-environment interactions and epigenomics, etc. (Herman and Zimmet, 2012; Karter et al., 2013; Maruthur, 2013). It is reported that multiple genes are involved in genetic susceptibility. The pathogenesis of the DM diseases are closely related to the alterations in multiple signal pathways such as JAK-STAT, AMPK, and PI3K (Richard and Stephens, 2011; Samuel and Shulman, 2012). And the changes of microbial environment might affect the DM pathogenesis (Wen et al., 2008). Study of Qin showed that gut microbial dysbiosis and pathogenic bacteria increasing were observed in patients with T2DM (Qin et al., 2012). If left untreated, DM can cause many complications, including kidneys damage, eyes damage, heart disease, stroke, bone fracture risks, and function failure (Jason et al., 2016). So many recent studies are focusing on the anti-diabetic drugs discovery.
A series of medicines, including analogs, sulfonylureas, thiazolidinedione, biguanides, α-glucosidase inhibitors, and DDP-4 inhibitors, have been used as commercial products for therapy of DM (Morral, 2003; Tian N. et al., 2013). However, because of the “one target, one drug” mechanism, they might have similar side effects, such as weight gain, cardiovascular disease, glycopenia, and gastrointestinal effects (Shah and Mudaliar, 2010). Considering the long-term treatment, side effects and high price of the current antidiabetic drugs, there is a huge demand for effective, low-toxic or non-toxic and affordable drugs for DM.
Compared with the synthetic drugs, natural products have played an important role in DM treatment. The treatment benefits are attributed to multi-components and multi-targets to produce combined or synergistic effects. With the rapid development of novel technologies, increasing studies have been focused on antidiabetic natural products. Nowadays, multi-components and multi-targets therapies have been proved to be more effective and less toxicity than traditional single-target drugs (Espinoza-Fonseca, 2006). With the rapid progress of bioinformatics, network pharmacology, integrating network biology and pharmacology, is considered as a promising approach toward more cost-effective drug development (Khan and Khan, 2016). It can reveal the underlying complex relationship between multi-components and multi-targets. Moreover, network pharmacology provides a system-level approach to understand the pathogenesis of diseases, and can be used for lead discovery, target identification and indication prediction, which coincides with the holistic and systematic concepts in traditional Chinese medicine (TCM) theory (Hopkins, 2008; Tang and Aittokallio, 2014). Compared with conventional “one gene, one drug, one disease” mode, network pharmacology focuses on “multi-targets, multi-components” treatment to diseases. And signal network analysis is a highly attractive tool to investigate complex relationships. What’s more, based on network pharmacology, feasible “drug-target-disease” network models can be established through high-throughput screening and bioinformatics (Poornima et al., 2016). It was reported that network pharmacology had various applications, such as herbal ingredient target/disease gene prediction, network balance regulation, elucidation of synergistic ingredient pairs and active ingredient groups (Tao et al., 2013; Zhang X. et al., 2014; Zintzaras et al., 2014). Thus, strategies based on network including network pharmacology and network medicine are increasingly developed and applied. And several databases have been established to assist network pharmacology analysis including PhIN (pharmacology interaction network database) (Wang Z. et al., 2015), C2Maps (computational connectivity maps) (Huang et al., 2012), databases on TCM (Li and Zhang, 2013), CVDHD (The cardiovascular disease herbal database) (Gu et al., 2013b) and anticancer drugs database (Azmi et al., 2010).
In drug discovery, combined multi-components and multi-targets, natural products based on network pharmacology can afford bright perspectives for treating DM in a systematic manner. It is an efficient and time-saving method to find the potential application in other therapeutic categories of drugs by predicting their targets. Therefore, the network pharmacology approach is developed to find effective drugs for the treatment of DM and related metabolic disorders such as obesity and metabolic syndrome. Based on network pharmacology, the antidiabetic compounds were detected from Ge-Gen-Qin-Lian decoction formula, and the mechanisms were elucidated (Li et al., 2014). In the study of Zhang S. et al. (2014), the “drug-target-pathway-disease” network of green tea was established. And neurotrophin signal pathway, p53 signal pathway, and T2DM pathway related to DM were screened by network pharmacology (Zhang S. et al., 2014). It was reported that the network pharmacology analysis was developed to illustrate the action mechanism of Tangminling Pills for T2DM therapy (Gu et al., 2011).
The aim of this review is to provide a summary of network pharmacology studies on the bioactive compounds and action mechanisms of natural products for the treatment of DM. The recent progresses on the different active constituents, databases and applications in the treatment of DM were highlighted. The mechanisms related to T2DM, including α-amylase and α-glucosidase inhibitory, targeting β cell dysfunction, AMPK signal pathway, PI3K/Akt signal pathway and modulation of gut microbiota were summarized. And the perspectives on their reasonable applications for therapy of DM and related drug discovery were put forward.
Network Pharmacology on DM
Databases of Natural Products
Natural products have played significant roles in the leads for drug discovery, which were involved in the development of approximately 64% of all drugs (Newman and Cragg, 2012). Databases of natural products are important for screening in drug discovery. Thus, several databases were established to provide the related information of natural products.
Super Natural II3 (Banerjee et al., 2015), containing about 326,000 molecules, was a freely available database to find natural products with different incorporating search functions. The database contained approximately 170,000 compounds and provided the following information of the products, including two-dimentional structures, the corresponding structural and physicochemical properties, the predicted toxicity class and the vendor information etc.
NAPRALERT (Loub et al., 1985) was a source database. The database collected the information of natural products by textual-numeric. About 105,000 organism names, 195,000 pharmacological results and 190,000 identified compounds were collected. In addition, it provided a strategy to prophetically identify the classification source of the most promising specific biological activities.
Chemical Entities of Biological Interest (ChEBI)4 (And et al., 2003) was also a freely available dictionary focused on small chemical compounds of either natural products or synthetic products. The database represented more than 12, 000 molecular entities, groups and classes. Some important information was provided such as ChEBI ID, ChEBI names, definition, structural diagrams, formula, synonyms, and registry number.
DrugBank (Wishart et al., 2008) was a database enriched drug data. The 2.0 version of DrugBank presented about 4,900 drug entries, which included about 1,467 FDA-approved drugs. In addition, the database contained common links to nearly all primary bioinformatics and biomedical databases (GenBank, KEGG, PubChem, and PubMed).
Other databases related to natural products were also developed, such as PubChem (Wang et al., 2009), UPND (Universal Natural Products Database) (Gu et al., 2013a), Dictionary of Natural Products (DNPs)5, CHDD (Qiao et al., 2002), Herb BioMap database (China Copyright of Computer Sofware, 2011SR076502), Chinese Natural Product Database (CNPD) (Zheng et al., 2005), Traditional Chinese Medicines Database (TCMD) (He et al., 2001) and ChemBank (Seiler et al., 2008).
All the databases can be useful for the studies involving virtual screening and the design of new compounds from natural compounds. The information about structures and physicochemical properties is useful and it can contribute to future drug development.
Natural Products for DM Therapy
Natural products have played an important role in DM therapy for a long history, especially in Asia, India and Africa. There were massive studies focused on herbal medicines for the development and discovery of antidiabetic medicine. Many kinds of extracts and bioactive constituents were studied on the hypoglycemic effects.
Extracts
Extracts from natural products have been widely prescribed for diabetic treatment worldwide. Some of them were evaluated scientifically and methodically in order to check for their properties (Odhav et al., 2010). Various plant extracts were traditionally used to DM treatment. In a long-term study, Annona muricata aqueous extract was daily administrated to diabetic rats for 28 days, the blood glucose levels and serum creatinine were reduced, and the MDA, AST, ALT activity, and nitrite levels LDL-cholesterol were also reduced (Florence et al., 2014). Khanra et al. (2015) reported that Abroma augusta L. (Malvaceae) leaf extract could be considered as a kind of prophylactic agent against T2DM and its associated kidney and cardiac toxicity (Khanra et al., 2015). Chicory seed extract had the capacity to target hyperglycemia, hyperlipidemia, IR, NAFLD (non-alcoholic fatty liver disease) and NASH (non-alcoholic steatohepatitis) through modulating PPARα/SREBP-1 ratio (Ziamajidi et al., 2013). There were other extracts used for the DM treatment, such as Hypericum perforatum extract (Hasanein and Shahidi, 2011), grape seed and skin extract (Oueslati et al., 2016), and flavonoid rich extract from Sophora tonkinensis Gagnep (Huang et al., 2016). So the herbal extracts can be used for the treatment of T2DM at systematic levels.
Polysaccharides
Polysaccharides are one of the main components of the natural sources and they are composed of more than 10 monosaccharide units linked together by glycosidic bonds. As the main bioactive fractions of natural products, polysaccharides have attracted much more attention during recent years (Zong et al., 2012). As shown in Table 1, polysaccharides from natural products were applied for the DM treatment during the period 2010–2016.
According to our previous study (2015), tea polysaccharides (TPS) were found to increase the body weight and decrease the blood glucose. It had higher potent glucose tolerance. And some biochemical indices were ameliorated. The TC and LDL-c contents were decreased and the TG and HDL-c contents were restored nearly to the normal levels. Furthermore, via upregulating the expressions of PI3K, p-Akt and GLUT4 target proteins in PI3K/Akt signal pathway, TPS could play an effective role in hypoglycemia. It was indicated that TPS might be a promising candidate for T2DM treatment (Li et al., 2015). A water soluble TPS enhanced insulin secretion simulated by glucose through cAMP-PKA pathway by upregulating not only the mRNA transcription but also the expression of PDX-1 protein (Wang H. et al., 2015). Polysaccharides of corn silk (POCS) were evaluated for its antidiabetic effects on streptozotocin (STZ)-induced diabetic rats. The results showed that POCS could not only significantly decrease blood glucose level, but also reduce total cholesterol (TC) and total triglyceride (TG) (Zhao W. et al., 2012). Mulberry leaf polysaccharides were reported to effectively improve the hepatic glucose metabolism and IR to the normal levels. By inhibiting the expression of protein–tyrosine phosphatase 1B, activating the PI3K/AKT signal pathway and relieving oxidative stress, the polysaccharides were proved to be a prophylactic agent for T2DM (Ren et al., 2014). What’s more, polysaccharides from Lachnum calyculiforme (Ye et al., 2011) and polysaccharides from Cynomorium songaricum (Wang et al., 2010) exhibited obvious hypoglycemic effects. The polysaccharides can be applied in the treatment of DM by targeting different signal pathways.
Polyphenols
Antidiabetic polyphenols extracted from natural products in the period 2010–2016 were listed in Table 2. Polyphenols, the secondary metabolites of plants, are mainly responsible for the flavor and color of fruits and other plant products. They are presented in fresh fruits and vegetables and found in various natural beverages such as red wines, tea and cocoa (Quideau et al., 2011). Solayman et al. (2015) reviewed the categories of polyphenols in the DM treatment, including anthocyanin, ellagitannin, luteolin, rosmarinic acids, catechin, resberatrol, rutin, quercetin, diosimin, and myricetin (Solayman et al., 2015). Different polyphenols have different action mechanisms.
It was reported that polyphenols from black soybean seed coat improved hyperglycemia and insulin sensitivity via regulating AMPK signal pathway both in vitro and in vivo, and anthocyanins [cyanidin 3-glucoside (C3G)] and procyanidins (PCs) were the main antidiabetic polyphenols, which could also enhanced glucose uptake (Kurimoto et al., 2013). Polyphenols from Vernonia amygdalina were reported to possess antihyperglycemic effects, most probably via increasing GLUT4 translocation and inhibiting hepatic G6Pase (Ong et al., 2010). EGCG, a kind of green tea polyphenol, could improve endothelial dysfunction and ameliorate metabolic IR in skeletal muscle and liver. And it was reported that EGCG could attenuate the death of β-cells in the db/db mouse, reduce IR, and increase the glucose-induced insulin scretion (Ortsäter et al., 2012). Therefore, the polyphenols could be a promising therapy to treat T2DM, obesity, and cardiovascular, in which there were reciprocal relationships between IR and endothelial dysfunction (Keske et al., 2015).
Other Constituents
Besides polysaccharides and polyphenols, there are other constituents from natural products related to antidiabetic effects. Several reviews provided summaries of the natural constituents for DM therapy, such as terpenoids, tannins, saponins, alkaloids, and lignans (Tundis et al., 2010; Hung et al., 2012; Zhang and Jiang, 2012).
Traditional herbal medicine has been used to treat DM for decades. Recent studies of antidiabetic compounds from 2010 to 2016 were categorized here. According to structure-activity relationship, these action mechanisms of natural products are quite different from those of the currently antidiabetic drugs. Natural products might be the promising sources to provide multi-components with multi-targets and new therapy for DM.
Database of DM
Network pharmacology is a new strategy to find the bioactive constituents as well as the molecular targets and the interactions. Nowadays, there are more studies and knowledge of DM from published literatures and the protein–protein interaction (PPI) databases (Liechti et al., 2010). Several databases such as T1Dbase, T2D-Db, T2DGADB, and T2D@ZJU have integrated the existing information, which contained genetic association studies, gene expression, related signal pathways, and PPI.
T1Dbase was a public website and database related to the molecular genetics and biology of T1DM. T1Dbase integrated the data from assembled genome sequences, derived gene and transcript models, publications linked to genes, T1DM susceptibility regions, genetic linkage and related studies. It provided integrated data and opportunities for researchers to explore the complex pathogenesis of T1DM which was affected by various factors (Hulbert et al., 2007).
T2D-Db collected 330 candidate genes from the Pubmed literatures and provided their corresponding information on almost all known molecular components involved in the pathogenesis of T2DM. Information on candidate genes had been established in this on line database, including SNPs (single nucleotide polymorphism) in candidate genes or candidate regions, GWA (genome wide association studies), pathways, PPI and diseases associated risk factors or complications (Agrawal et al., 2008).
T2DGADB collected 701 publications in T2DM genetic associated research area. It aimed to provide specialized information on the genetic risk factors involved in the development of T2DM. And T2DGADB focused on information related to each SNP association including the populations used, odds ratio and other factors (Lim et al., 2010).
T2D@ZJU summarized three levels of heterogeneous connections related to T2DM, which was searched from pathway databases, PPI databases and literatures. It contained 1,078 T2DM related substances including proteins and its complexes, drugs and the interactions. Compared with T2D-Db and T2DGADB, which focused on the genes associated with T2DM, T2D@ZJU organized the integrated information through network. From the perspective of system biology, it established a basis for the further research of T2DM, which was contributed to the elucidation of the action mechanism and related drug development. Furthermore, this database could also promote the T2DM-related studies of network pharmacology and multi-targets therapeutics (Yang et al., 2013).
The high availability of the DM databases plays a significant role in integrating related complex data. They can be used to predict the target network of the bioactive constituents. After experimental validation of the molecular network, they can provide a new perspective for researchers to better understand the pathogenesis of DM and action mechanisms of associated drugs.
Action Mechanisms Related to T2DM
A large amount of studies have indicated that natural products showed antidiabetic effects via a variety of mechanisms. The present review summarizes and discusses the related antidiabetic mechanisms.
α-Amylase and α-Glucosidase Inhibitory
It has been reported that the inhibition of α-amylase and α-glucosidase could be an important concept for therapy of T2DM. Dietary carbohydrates can be hydrolyzed into oligosaccharide and then into monosaccharide including glucose, which is produced by the main hydrolyzing enzymes such as pancreatic α-amylase and intestinal α-glucosidase. The α-amylase hydrolyzes α-1,4-glycocidic bonds and produced smaller oligosaccharides and disaccharides, while the α-glucosidase hydrolyzes disaccharides to monosaccharide (Apostolidis and Lee, 2010). Therefore, it is an effective approach to manage the blood glucose level via inhibiting the activities of α-amylase and α-glucosidase (Striegel et al., 2015).
Targeting β Cell Dysfunction
The β cell dysfunction and IR are inherently complex with their interrelation for triggering the pathogenesis of DM. It results from inadequate glucose sensing to stimulate insulin secretion. Cytokine-induced inflammation, oxidative stress, and overconsumption of saturated fat and free fatty acids can cause β cell dysfunction (Cerf, 2013). Therefore, preserving β cell function with anti-inflammatory effect and antioxidant effect can maintain glucose homeostasis (Nguyen et al., 2012; Hasnain et al., 2016).
Targeting Signal Pathways
There are various sophisticated signal pathways related to DM that can be therapeutically targeted. The present review provides a summary of signal pathways related to DM (Figure 1), including AMPK signal pathway (Kurimoto et al., 2013), PI3K/Akt signal pathway (Li et al., 2015), mTOR signal pathway (Siegel, 2008), JAK-STAT signal pathway (Gurzov et al., 2016), ROS-ERK-NF-κB signal pathway (Liu et al., 2013), Wnt signal pathway (Chiang et al., 2012), and IGF-1 signal pathway (Siddle, 2011). By targeting these signal pathways, the bioactive constituents can produce multiple effects including glut translocation, glut trafficking, glycogen synthesis, glycolysis, lipolysis, microtubules, gluconeogenesis, lipogenesis, and autophagy.
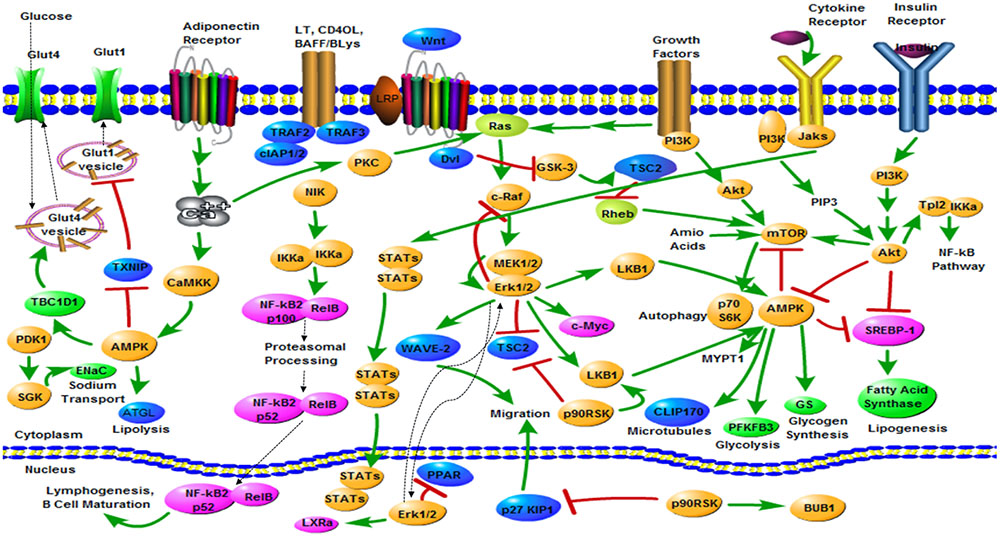
FIGURE 1. Summary of signal pathways related to diabetes mellitus. Pathway diagram keys: Direct stimulatory modification;
Direct inhibitory modification;
Translocation;
Multistep stimulatory modification.
Modulation of Gut Microbiota
The gut microbiota was hypothesized to play a critical role in metabolic diseases, including T2DM (Xu et al., 2015). Ge-Gen-Qin-Lian decoction (GQD) formula, consisted of four herbs: Puerariae Lobatae radix (Ge-Gen), Coptidis rhizoma (Huang-Lian), Scutellariae radix (Huang-Qin), and Glycyrrhizae Radix et Rhizoma Praeparata cum Melle (Gan-Cao), was treated for “Xiaoke,” namely T2DM. GQD treatment conspicuously modulated gut microbial metabolism by degradation of choline into methylamines, together with a decrease in FBG and an expansion of islets in STZ and high-fat-diet-induced diabetic rats (Tian S. et al., 2013). In addition, GQD could modulate the composition of the intestinal microbiota during T2DM clinical treatment, especially enrich the quantity of beneficial bacteria such as Faecalibacterium spp., and it was found that structural alterations in the gut microbiota were associated with the anti-diabetic effects of GQD (Xu et al., 2015). What’s more, in the study of Wang et al. (2016), the quality and quantity of Lactobacillus and Bacteroides genus were significantly increased with the increasing concentration of POCS. The results indicated that POCS could restore the intestinal microbiota balance for the treatment of T2DM. Because most of the herbal drugs were usually orally administrated, the modulation of the herbal drugs on the intestine microbiota has been new mechanistic understanding of the natural products in DM treatment.
Compared with initial time-consuming laboratory experiments, network pharmacology approaches for well-known pathways with various natural products will be more efficient for antidiabetic drug discovery. Based on combining the network, chemical, pharmacological, biomedical and computational results, we can achieve multi-components and multi-targets therapy for DM.
Applications of Network Pharmacology for DM Therapy
Network pharmacology, as a powerful tool to elucidate complex relationships from a systematic and biological level, has been more and more popular in recent years. It has been applied to various complex chronic diseases, such as cardio-cerebral ischemic diseases (Gu et al., 2013b), cancer (Azmi et al., 2010; Zhang et al., 2012; Poornima et al., 2016), pancreatitis (Hong et al., 2016), gout (Zhao et al., 2015), Alzheimer’s disease and DM and other metabolic disease (Loscalzo, 2011). For DM therapy, network pharmacology has been widely used in the mechanism studies.
According to the study of Liu et al. (2007), a gene network enrichment analysis (GNEA) was used to identify two sets of genes, IS-HD and NR-HD gene sets, which was associated with insulin signal and a network of nuclear receptors. They identified a network of PPI between members from IS-HD and NR-HD gene sets that might facilitate signal. By using GNEA, it was found that insulin-signal process was obviously transcriptionally altered in IR and T2DM. Their results also indicated that integrating high-throughput microarray studies together with PPI networks was an effective method to prove the existing biological processes associated with a complex disorder (Liu et al., 2007).
In the study of Li et al. (2014), the network pharmacology method was used to assist detecting the potential antidiabetic ingredients from the GQD. They collected 287 available chemical components, with 42 of them found in Ge-Gen, 57 of them found in Huang-Qin, 22 of them found in Huang-Lian, and 166 of them found in Gan-Cao. In this study, network target analysis was applied to elucidate the relationships between herbal ingredients and bioactivities. And the network pharmacology method also contributed to better understand the action mechanisms of GQD formula. It was also found that 4-Hydroxymephenytoin, a novel compound from Puerariae Lobatae radix (Ge-Gen), could increase the insulin secretion and improve IR (Li et al., 2014). The network pharmacology approach had been an effective strategy for the modern research on herbal drugs on the DM treatment, and the multi-active compounds could be identified and the multi-targets action mechanisms could be elucidated.
As the second most consumed beverage in the world next to water, Tea (Camellia sinensis L.) had been used as antidiabetic candidate for a long time according to folk remedies (Chen et al., 2007). Tea polyphenols (GTPs) was one of the main components of tea, mainly including EGCG, EGC, ECG, and EC. Different GTPs could act on different targets in the signal network of complex diseases (diabetes, cancer, cardiovascular, etc.), resulting in a synergistic effect, which was in accordance with the theory of network pharmacology. Study of network pharmacology approach on GTPs identified three pathways, p53 signal pathway, neurotrophin signal pathway, and T2DM pathway, which related to DM from all 147 pathways. And they established a diabetes-related pathway by integrating these three pathways (Zhang S. et al., 2014).
In order to find the potential inhibitors from TCM for the T2DM-related targets, Tian S. et al. (2013) developed an integrated approach that combined molecular docking and pharmacophore mapping, and they established the compound-target interaction network. A total of 2,479 non-duplicated compounds from these 32 Chinese herbs of 52 classical TCM formulas were found from two databases. The Bayesian classifiers with satisfactory discrimination capabilities for 15 targets were employed to screen the 2,479 compounds. The results showed that molecular docking or pharmacophore mapping could give satisfactory predictions for most targets. It was proved that some herbal ingredients could directly interact with T2DM related targets, while some ingredients relieved T2DM via antioxidant effects or other supplementary pharmacological effects (Tian S. et al., 2013).
Zhao H.L. et al. (2012) reported that male Zucker diabetic fatty rats were treated by JCU, a kind of TCM, which contained three herbs and berberine. The results showed that JCU could improve hyperglycemia, ameliorate IR, normalize liver enzyme and hepatocyte ballooning degeneration. On three levels including miRNA level, protein level and mRNA level, the study elucidated the action mechanisms of JCU, regulating the critical cytokines on the related pathways including improving the quality and quantity of cell survival, increasing glucose uptake, and ameliorating insulin sensitivity (Zhao H.L. et al., 2012). It in-depth validated that there was of great significance of multi-components TCM to treat DM.
In the work of Gu et al. (2011), the combination of molecular docking and network pharmacology analysis was developed to illustrate the action mechanism of Tangminling Pills for T2DM therapy. The drug–drug network and drug-target network illustrated that more than 100 active compounds of 667 compounds in the formula would target 37 target proteins related to T2DM. That is, they played important roles in the biological network. As well as the critical ingredients in Tangminling Pills were predicted and some of them had been reported in literatures. In addition, several compounds including Rheidin A, Sennoside C, Rheidin C, procyanidin C1, and Dihydrobaicalin were of importance as the antidiabetic candidate due to its pharmacological effects (Gu et al., 2011).
Conclusion and Perspectives
Diabetes mellitus, a complicated metabolic disease, is a currently health problem causing significant mobility and mortality worldwide, which can cause various complications such as kidneys dysfunction, eyes damage, and cardiovascular diseases. Current antidiabetic drugs are still limited due to single-compound, single-target, side-effects, drug tolerance and low efficacy and they can’t cure DM completely. And it is significant that the world populations are heterogeneous and genetic polymorphisms in pharmacologically relevant genes varying across geographical region (Suarez-Kurtz et al., 2014). As a novel perspective, network pharmacology is becoming more and more popular in drug discovery. Compared with the single target of the current antidiabetic drugs, it is an promising approach which can combat the complex problems (Tao et al., 2014). It may also contribute to better explain the low clinical efficacy, side effects and toxicity of the current clinical drugs. At the same time, natural products have vast structural diversity and various bioactivities, which can provide the opportunities to find different lead compounds, different targets for DM. Hence, limited studies result in an urgent need to develop novel network pharmacology approaches to change current theories of drug discovery and to improve our understanding of action mechanisms. Although network pharmacology of natural products is still in its infancy, the appropriate use of network pharmacology approaches may initiate new directions, overcome the disadvantages of current antidiabetic therapies as well as contribute new insights into the discovery of novel antidiabetic drugs. Specific researches can be done to better elucidate the usage of natural products, and explore the possible action mechanisms.
Author Contributions
WL drafted and revised the manuscript. GY, YP, and CW helped in literature survey and manuscript preparation. HC contributed to the conception and design of the work, revised and improved the manuscript. All authors approved on the finally submitted version of the manuscript.
Conflict of Interest Statement
The authors declare that the research was conducted in the absence of any commercial or financial relationships that could be construed as a potential conflict of interest.
Acknowledgments
Our work was financially supported by the grant from the Natural Science Foundation of China (NSFC 31371879) and National High Technology Research and Development Program (“863” Program) of China (Grant No. SS2013AA100207).
Footnotes
- ^http://www.diabetesatlas.org
- ^http://www.who.int/en/
- ^http://bioinformatics.charite.de/supernatural
- ^http://www.ebi.ac.uk/chebi/
- ^http://dnp.chemnetbase.com
References
Agrawal, S., Dimitrova, N., Nathan, P., Udayakumar, K., Lakshmi, S. S., Sriram, S., et al. (2008). T2D-Db: An integrated platform to study the molecular basis of type 2 diabetes. BMC Genomics 9:320. doi: 10.1186/1471-2164-9-320
Ali, F., Ismail, A., and Kersten, S. (2014). Molecular mechanisms underlying the potential antiobesity-related diseases effect of cocoa polyphenols. Mol. Nutr. Food Res. 58, 33–48. doi: 10.1002/mnfr.201300277
And, M. W., Willett, P., And, W. K., and Noort, P. V. (2003). Evaluation of similarity measures for searching the dictionary of natural products database. J. Chem. Inf. Comp. Sci. 43, 449–457. doi: 10.1021/ci025591m
Apostolidis, E., and Lee, C. M. (2010). In vitro potential of Ascophyllum nodosum phenolic antioxidant-mediated alpha-glucosidase and alpha-amylase inhibition. J. Food Sci. 75, 97–102. doi: 10.1111/j.1750-3841.2010.01544.x
Azmi, A. S., Wang, Z., Philip, P. A., Mohammad, R. M., and Sarkar, F. H. (2010). Proof of concept: network and systems biology approaches aid in the discovery of potent anticancer drug combinations. Mol. Cancer Ther. 9, 3137–3144. doi: 10.1158/1535-7163.MCT-10-0642
Banerjee, P., Erehman, J., Gohlke, B. O., Wilhelm, T., Preissner, R., and Dunkel, M. (2015). Super natural II—a database of natural products. Nucleic Acids Res. 43, 935–939. doi: 10.1093/nar/gku886
Cai, S., Wen, S., Fan, Y., Xuan, G., Xu, G., Xu, T., et al. (2016). Effect of mulberry leaf (Folium Mori) on insulin resistance via IRS-1/PI3K/Glut-4 signalling pathway in type 2 diabetes mellitus rats. Pharm. Biol. 54, 2685–2691. doi: 10.1080/13880209.2016.1178779
Cerf, M. E. (2013). Beta cell dysfunction and insulin resistance. Front. Endocrinol. 4:37. doi: 10.3389/fendo.2013.00037
Chen, H., Zhang, M., Qu, Z., and Xie, B. (2007). Compositional analysis and preliminary toxicological evaluation of a tea polysaccharide conjugate. J. Agric. Food Chem. 55, 2256–2260. doi: 10.1021/jf0632740
Chiang, Y. T., Ip, W., and Jin, T. (2012). The role of the Wnt signaling pathway in incretin hormone production and function. Front. Physiol. 3:273. doi: 10.3389/fphys.2012.00273
Enk, J., and Mandelboim, O. (2014). The role of natural cytotoxicity receptors in various pathologies: emphasis on type I diabetes. Front. Immunol. 5:4. doi: 10.3389/fimmu.2014.00004
Espinoza-Fonseca, L. M. (2006). The benefits of the multi-target approach in drug design and discovery. Bioorgan. Med. Chem. 14, 896–897. doi: 10.1016/j.bmc.2005.09.011
Florence, N. T., Benoit, M. Z., Jonas, K., Alexandra, T., Désiré, D. D. P., Pierre, K., et al. (2014). Antidiabetic and antioxidant effects of Annona muricata (Annonaceae), aqueous extract on streptozotocin-induced diabetic rats. J. Ethnopharmacol. 151, 784–790. doi: 10.1016/j.jep.2013.09.021
Gu, J., Gui, Y., Chen, L., Yuan, G., Lu, H. Z., and Xu, X. (2013a). Use of natural products as chemical library for drug discovery and network pharmacology. PLoS ONE 8:e62839. doi: 10.1371/journal.pone.0062839
Gu, J., Gui, Y., Chen, L., Yuan, G., and Xu, X. (2013b). CVDHD: a cardiovascular disease herbal database for drug discovery and network pharmacology. J. Cheminform. 5, 976–1000. doi: 10.1186/1758-2946-5-51
Gu, J., Zhang, H., Chen, L., Xu, S., Yuan, G., and Xu, X. (2011). Drug-target network and polypharmacology studies of a Traditional Chinese Medicine for type II diabetes mellitus. Comput. Biol. Chem. 35, 293–297. doi: 10.1016/j.compbiolchem.2011.07.003
Gurzov, E. N., Stanley, W. J., Pappas, E. G., Thomas, H. E., and Gough, D. J. (2016). The JAK-STAT pathway in obesity and diabetes. FEBS J. 283, 3002–3015. doi: 10.1111/febs.13709
Hasanein, P., and Shahidi, S. (2011). Effects of Hypericum perforatum extract on diabetes-induced learning and memory impairment in rats. Phytother. Res. 25, 544–549. doi: 10.1002/ptr.3298
Hasnain, S. Z., Prins, J. B., and Mcguckin, M. A. (2016). Oxidative and endoplasmic reticulum stress in β-cell dysfunction in diabetes. J. Mol. Endocrinol. 56, 33–54. doi: 10.1530/JME-15-0232
He, M., Yan, X., Zhou, J., and Xie, G. (2001). Traditional Chinese medicine database and application on the Web. J. Chem. Inf. Comput. Sci. 41, 273–277. doi: 10.1002/chin.200122173
Herman, W. H., and Zimmet, P. (2012). Type 2 diabetes: an epidemic requiring global attention and urgent action. Diabetes Care 35, 943–944. doi: 10.2337/dc12-0298
Hong, X., Wang, G., Qu, J., Xia, S., Tao, X., Qi, B., et al. (2016). Yin-chen-hao tang attenuates severe acute pancreatitis in rat: an experimental verification of in silico network target prediction. Front. Pharmacol. 7:378. doi: 10.3389/fphar.2016.00378
Hopkins, A. L. (2008). Network pharmacology: the next paradigm in drug discovery. Nat. Chem. Biol. 4, 682–690. doi: 10.1038/nchembio.118
Huang, H., Wu, X., Pandey, R., Li, J., Zhao, G., Ibrahim, S., et al. (2012). C2Maps: a network pharmacology database with comprehensive disease-gene-drug connectivity relationships. BMC Genomics 13(Suppl. 6):S17. doi: 10.1186/1471-2164-13-S6-S17
Huang, J., Huang, K., Lan, T., Xie, X., Shen, X., Liu, P., et al. (2013). Curcumin ameliorates diabetic nephropathy by inhibiting the activation of the SphK1-S1P signaling pathway. Mol. Cell. Endocrinol. 365, 231–240. doi: 10.1016/j.mce.2012.10.024
Huang, M., Deng, S., Han, Q., Zhao, P., Zhou, Q., Zheng, S., et al. (2016). Hypoglycemic activity and the potential mechanism of the flavonoid rich extract from Sophora tonkinensis Gagnep. in KK-Ay Mice. Front. Pharmacol. 7:288. doi: 10.3389/fphar.2016.00288
Hulbert, E. M., Smink, L. J., Adlem, E. C., Allen, J. E., Burdick, D. B., Burren, O. S., et al. (2007). T1DBase: integration and presentation of complex data for type 1 diabetes research. Nucleic Acids Res. 35(Suppl. 1), 6338–6338. doi: 10.1093/nar/gkl933
Hung, H. Y., Qian, K., Morris-Natschke, S. L., Hsu, C. S., and Lee, K. H. (2012). Recent discovery of plant-derived anti-diabetic natural products. Nat. Prod. Rep. 29, 580–606. doi: 10.1039/c2np00074a
Jason, K., Swati, P., Rama, Y., Manwaring, H. R., Sandra, P., Srivastava, R. K., et al. (2016). Dietary interventions for type 2 diabetes: how millet comes to help. Front. Plant Sci. 7:1454. doi: 10.3389/fpls.2016.01454
Karter, A. J., Schillinger, D., Adams, A. S., Moffet, H. H., Liu, J., Adler, N. E., et al. (2013). Elevated rates of diabetes in Pacific Islanders and Asian subgroups: the diabetes study of Northern California (DISTANCE). Diabetes Care 36, 569–573. doi: 10.2337/dc12-0722
Keller, M. P., Choi, Y., Wang, P., Davis, D. B., Rabaglia, M. E., Oler, A. T., et al. (2008). A gene expression network model of type 2 diabetes links cell cycle regulation in islets with diabetes susceptibility. Genome Res. 18, 706–716. doi: 10.1101/gr.074914.107
Keske, M. A., Ng, H. L., Premilovac, D., Rattigan, S., Kim, J. A., Munir, K., et al. (2015). Vascular and metabolic actions of the green tea polyphenol epigallocatechin gallate. Curr. Med. Chem. 22, 59–69. doi: 10.2174/0929867321666141012174553
Khan, S. N., and Khan, A. U. (2016). Breaking the spell: combating multidrug resistant ‘Superbugs’. Front. Microbiol. 6:1574. doi: 10.3389/fmicb.2016.00174
Khanra, R., Dewanjee, S., Dua, T. K., Sahu, R., Gangopadhyay, M., De, F. V., et al. (2015). Abroma augusta L. (Malvaceae) leaf extract attenuates diabetes induced nephropathy and cardiomyopathy via inhibition of oxidative stress and inflammatory response. J. Transl. Med. 13, 1–14. doi: 10.1186/s12967-014-0364-1
Kim, E. A., Lee, S. H., Lee, J. H., Kang, N., Oh, J. Y., Cha, S., et al. (2016). A marine algal polyphenol, dieckol, attenuates blood glucose levels by Akt pathway in alloxan induced hyperglycemia zebrafish model. RSC Adv. 6, 78570–78575. doi: 10.1039/C6RA12724J
Kokil, G. R., Veedu, R. N., Ramm, G. A., Prins, J. B., and Parekh, H. S. (2015). Type 2 diabetes mellitus: limitations of conventional therapies and intervention with nucleic acid-based therapeutics. Chem. Rev. 115, 4719–4743. doi: 10.1021/cr5002832
Kurimoto, Y., Shibayama, Y., Inoue, S., Soga, M., Takikawa, M., Ito, C., et al. (2013). Black soybean seed coat extract ameliorates hyperglycemia and insulin sensitivity via the activation of AMP-activated protein kinase in diabetic mice. J. Agric. Food Chem. 61, 5558–5564. doi: 10.1021/jf401190y
Li, H., Zhao, L., Zhang, B., Jiang, Y., Wang, X., Guo, Y., et al. (2014). A network pharmacology approach to determine active compounds and action mechanisms of ge-gen-qin-lian decoction for treatment of type 2 diabetes. Evid. Based Compl. Alt. Med. 2014:495840. doi: 10.1155/2014/495840
Li, S., Chen, H., Wang, J., Wang, X., Hu, B., and Lv, F. (2015). Involvement of the PI3K/Akt signal pathway in the hypoglycemic effects of tea polysaccharides on diabetic mice. Int. J. Biol. Macromol. 81, 967–974. doi: 10.1016/j.ijbiomac.2015.09.037
Li, S., and Zhang, B. (2013). Traditional Chinese medicine network pharmacology: theory, methodology and application. Chin. J. Nat. Med. 11, 110–120. doi: 10.1016/S1875-5364(13)60037-0
Liechti, R., Csárdi, G., and Bergmann, S. (2010). EuroDia: a beta-cell gene expression resource. Database 2010, 207–214. doi: 10.1093/database/baq024
Lim, J. E., Hong, K. W., Jin, H. S., Kim, Y. S., Park, H. K., and Oh, B. (2010). Type 2 diabetes genetic association database manually curated for the study design and odds ratio. BMC Med. Inform. Decis. 10:76. doi: 10.1186/1472-6947-10-76
Lin, W., Wang, W., Liao, D., Chen, D., Zhu, P., Cai, G., et al. (2015). Polysaccharides from Enteromorpha prolifera improve glucose metabolism in diabetic rats. J. Diabetes Res. 2015:675201. doi: 10.1155/2015/675201
Liu, M., Liberzon, A., Kong, S. W., Lai, W. R., Park, P. J., Kohane, I. S., et al. (2007). Network-based analysis of affected biological processes in type 2 diabetes models. PLoS Genet. 3:e96. doi: 10.1371/journal.pgen.0030096
Liu, M., Qin, J., Hao, Y., Liu, M., Luo, J., Luo, T., et al. (2013). Astragalus polysaccharide suppresses skeletal muscle myostatin expression in diabetes: involvement of ROS-ERK and NF-κB pathways. Oxid. Med. Cell. Longev. 2013, 782497–782497. doi: 10.1155/2013/782497
Loscalzo, J. (2011). Network medicine: a network-based approach to human disease. Nat. Rev. Genet. 12, 56–68. doi: 10.1038/nrg2918
Loub, W. D., Farnsworth, N. R., Soejarto, D. D., and Quinn, M. L. (1985). NAPRALERT: computer handling of natural product research data. J. Chem. Inf. Comput. Sci. 25, 99–103. doi: 10.1021/ci00046a009
Ma, X., Zhou, F., Chen, Y., Zhang, Y., Hou, L., Cao, X., et al. (2014). A polysaccharide from Grifola frondosa relieves insulin resistance of HepG2 cell by Akt-GSK-3 pathway. Glycoconj. J. 31, 355–363. doi: 10.1007/s10719-014-9526-x
Ma, Y., Mao, D., Geng, L., Wang, Z., and Xu, C. (2013). Production, fractionation, characterization of extracellular polysaccharide from a newly isolated Trametes gibbosa and its hypoglycemic activity. Carbohydr. Polym. 96, 460–465. doi: 10.1016/j.carbpol.2013.04.019
Man, S., Ma, J., Wang, C., Li, Y., Gao, W., and Lu, F. (2016). Chemical composition and hypoglycaemic effect of polyphenol extracts from Litchi chinensis seeds. J. Funct. Foods 22, 313–324. doi: 10.1016/j.jff.2016.01.032
Maruthur, N. M. (2013). The growing prevalence of type 2 diabetes: increased incidence or improved survival? Curr. Diabetes Rep. 13, 786–794. doi: 10.1007/s11892-013-0426-4
Morral, N. (2003). Novel targets and therapeutic strategies for type 2 diabetes. Trends. Endocrin. Met. 14, 169–175. doi: 10.1016/S1043-2760(03)00031-6
Newman, D. J., and Cragg, G. M. (2012). Natural products as sources of new drugs over the 30 years from 1981 to 2010. J. Nat. Prod. 75, 311–335. doi: 10.1021/np200906s
Nguyen, D. V., Shaw, L. C., and Grant, M. B. (2012). Inflammation in the pathogenesis of microvascular complications in diabetes. Front. Endocrinol. 3:170. doi: 10.3389/fendo.2012.00170
Oboh, G., Ademiluyi, A. O., Akinyemi, A. J., Henle, T., Saliu, J. A., and Schwarzenbolz, U. (2012). Inhibitory effect of polyphenol-rich extracts of jute leaf (Corchorus olitorius) on key enzyme linked to type 2 diabetes (α-amylase and α-glucosidase) and hypertension (angiotensin I converting) in vitro. J. Funct. Foods 4, 450–458. doi: 10.1016/j.jff.2012.02.003
Odhav, B., Kandasamy, T., Khumalo, N., and Baijnath, H. (2010). Screening of African traditional vegetables for their alpha-amylase inhibitory effect. J. Med. Plants Res. 4, 1502–1507. doi: 10.5897/JMPR09.090
Ong, K. W., Hsu, A., Song, L., Huang, D., and Tan, B. K. (2010). Polyphenols-rich Vernonia amygdalina shows anti-diabetic effects in streptozotocin-induced diabetic rats. J. Ethnopharmacol. 133, 598–607. doi: 10.1016/j.jep.2010.10.046
Ortsäter, H., Grankvist, N., Wolfram, S., Kuehn, N., and Sjöholm, Å (2012). Diet supplementation with green tea extract epigallocatechin gallate prevents progression to glucose intolerance in db/db mice. Nutr. Metab. 9:11. doi: 10.1186/1743-7075-9-11
Oueslati, N., Charradi, K., Bedhiafi, T., Limam, F., and Aouani, E. (2016). Protective effect of grape seed and skin extract against diabetes-induced oxidative stress and renal dysfunction in virgin and pregnant rat. Biomed. pharmacother. 83, 584–592. doi: 10.1016/j.biopha.2016.07.024
Poornima, P., Kumar, J. D., Zhao, Q., Blunder, M., and Efferth, T. (2016). Network pharmacology of cancer: from understanding of complex interactomes to the design of multi-target specific therapeutics from nature. Pharmacol. Res. 111, 290–302. doi: 10.1016/j.phrs.2016.06.018
Puri, S., and Hebrok, M. (2012). Diabetic β cells: to be or not to be? Cell 150, 1103–1104. doi: 10.1016/j.cell.2012.08.021
Qiao, X., Hou, T., Zhang, W., Guo, S., and Xu, X. (2002). A 3D structure database of components from Chinese traditional medicinal herbs. J. Chem. Inf. Comput. Sci. 33, 481–489. doi: 10.1002/chin.200233253
Qin, J., Li, Y., Cai, Z., Li, S., Zhu, J., Zhang, F., et al. (2012). A metagenome-wide association study of gut microbiota in type 2 diabetes. Nature 490, 55–60. doi: 10.1038/nature11450
Quideau, S., Deffieux, D., Douatcasassus, C., and Pouységu, L. (2011). Plant polyphenols: chemical properties, biological activities, and synthesis. Angew. Chem. Int. Edit. 50, 586–621. doi: 10.1002/anie.201000044
Ren, C., Zhang, Y., Cui, W., Lu, G., Wang, Y., Gao, H., et al. (2014). A polysaccharide extract of mulberry leaf ameliorates hepatic glucose metabolism and insulin signaling in rats with type 2 diabetes induced by high fat-diet and streptozotocin. Int. J. Biol. Macromol. 72, 951–959. doi: 10.1016/j.ijbiomac.2014.09.060
Richard, A. J., and Stephens, J. M. (2011). Emerging roles of JAK–STAT signaling pathways in adipocytes. Trends Endocrinol. Metab. 22, 325–332. doi: 10.1016/j.tem.2011.03.007
Samuel, V. T., and Shulman, G. I. (2012). Mechanisms for insulin resistance: common threads and missing links. Cell 148, 852–871. doi: 10.1016/j.cell.2012.02.017
Seiler, K. P., George, G. A., Happ, M. P., Bodycombe, N. E., Carrinski, H. A., Norton, S., et al. (2008). ChemBank: a small-molecule screening and cheminformatics resource database. Nucleic Acids Res. 36(Suppl. 1), 351–359. doi: 10.1093/nar/gkm843
Shah, P., and Mudaliar, S. (2010). Pioglitazone: side effect and safety profile. Expert Opin. Drug. Saf. 9, 347–354. doi: 10.1517/14740331003623218
Siddle, K. (2011). Signalling by insulin and IGF receptors: supporting acts and new players. J. Mol. Endocrinol. 47, 1–10. doi: 10.1530/JME-11-0022
Siegel, N. (2008). The mTOR pathway and its role in human genetic diseases. Mutat. Res. 659, 284–292. doi: 10.1016/j.mrrev.2008.06.001
Solayman, M., Ali, Y., Alam, F., Islam, M. A., Alam, N., Khalil, M. I., et al. (2015). Polyphenols: potential future arsenals in the treatment of diabetes. Curr. Pharm. Des. 22, 549–565. doi: 10.2174/1381612822666151125001111
Striegel, L., Kang, B., Pilkenton, S. J., Rychlik, M., and Apostolidis, E. (2015). Effect of black tea and black tea pomace polyphenols on α-glucosidase and α-amylase inhibition, relevant to type 2 diabetes prevention. Front. Nutr. 2:3. doi: 10.3389/fnut.2015.00003
Suarez-Kurtz, G., Vargens, D. D., Santoro, A. B., Hutz, M. H., de Moraes, M. E., Pena, S. D., et al. (2014). Global pharmacogenomics: distribution of CYP3A5 polymorphisms and phenotypes in the Brazilian population. PLoS ONE 9:e83472. doi: 10.1371/journal.pone.0083472
Sun, C., Chen, Y., Li, X., Tai, G., Fan, Y., and Zhou, Y. (2014). Anti-hyperglycemic and anti-oxidative activities of ginseng polysaccharides in STZ-induced diabetic mice. Food Funct. 5, 845–848. doi: 10.1039/c3fo60326a
Tang, H. L., Chen, C., Wang, S. K., and Sun, G. J. (2015). Biochemical analysis and hypoglycemic activity of a polysaccharide isolated from the fruit of Lycium barbarum L. Int. J. Biol. Macromol. 77, 235–242. doi: 10.1016/j.ijbiomac.2015.03.026
Tang, J., and Aittokallio, T. (2014). Network pharmacology strategies toward multi-target anticancer therapies: from computational models to experimental design principles. Curr. Pharm. Des. 20, 23–36. doi: 10.2174/13816128113199990470
Tao, L., Zhu, F., Qin, C., Zhang, C., Xu, F., Tan, C. Y., et al. (2014). Nature’s contribution to today’s pharmacopeia. Nat. Biotechnol. 32, 979–980. doi: 10.1038/nbt.3034
Tao, W., Xu, X., Wang, X., Li, B., Wang, Y., Li, Y., et al. (2013). Network pharmacology-based prediction of the active ingredients and potential targets of Chinese herbal Radix Curcumae formula for application to cardiovascular disease. J. Ethnopharmacol. 145, 1–10. doi: 10.1016/j.jep.2012.09.051
Tian, N., Wang, J., Wang, P., Song, X., Yang, M., and Kong, L. (2013). NMR-based metabonomic study of Chinese medicine Gegen Qinlian Decoction as an effective treatment for type 2 diabetes in rats. Metabolomics 9, 1228–1242. doi: 10.1007/s11306-013-0535-8
Tian, S., Li, Y., Li, D., Xu, X., Wang, J., Zhang, Q., et al. (2013). Modeling compound-target interaction network of traditional Chinese medicines for type II diabetes mellitus: insight for polypharmacology and drug design. J. Chem. Inf. Model. 53, 1787–1803. doi: 10.1021/ci400146u
Tundis, R., Loizzo, M. R., and Menichini, F. (2010). Natural products as alpha-amylase and alpha-glucosidase inhibitors and their hypoglycaemic potential in the treatment of diabetes: an update. Mini Rev. Med. Chem. 10, 315–331. doi: 10.2174/138955710791331007
Wang, C., Yin, Y., Cao, X., and Li, X. (2016). Effects of Maydis stigma polysaccharide on the intestinal microflora in type-2 diabetes. Pharm. Biol. 54, 3086–3092. doi: 10.1080/13880209.2016.1211153
Wang, H., Shi, S., Bao, B., Li, X., and Wang, S. (2015). Structure characterization of an arabinogalactan from green tea and its anti-diabetic effect. Carbohydr. Polym. 124, 98–108. doi: 10.1016/j.carbpol.2015.01.070
Wang, J., Zhang, J., Zhao, B., Wu, Y., Wang, C., and Wang, Y. (2010). Structural features and hypoglycaemic effects of Cynomorium songaricum polysaccharides on STZ-induced rats. Food Chem. 120, 443–451. doi: 10.1016/j.foodchem.2009.10.034
Wang, L. Y., Wang, Y., Xu, D. S., Ruan, K. F., Feng, Y., and Wang, S. (2012). MDG-1, a polysaccharide from Ophiopogon japonicus exerts hypoglycemic effects through the PI3K/Akt pathway in a diabetic KKAy mouse model. J. Ethnopharmacol. 143, 347–354. doi: 10.1016/j.jep.2012.06.050
Wang, Y., Xiao, J., Suzek, T. O., Zhang, J., Wang, J., and Bryant, S. H. (2009). PubChem: a public information system for analyzing bioactivities of small molecules. Nucleic Acids Res. 37(Suppl. 2), 623–633. doi: 10.1093/nar/gkp456
Wang, Z., Li, J., Dang, R., Liang, L., and Lin, J. (2015). PhIN: a protein pharmacology interaction network database. CPT Pharm. Syst. Pharm. 4:e00025. doi: 10.1002/psp4.25
Wen, L., Ley, R. E., Volchkov, P. Y., Stranges, P. B., Avanesyan, L., Stonebraker, A. C., et al. (2008). Innate immunity and intestinal microbiota in the development of type 1 diabetes. Nature 455, 1109–1113. doi: 10.1038/nature0733
Wishart, D. S., Knox, C., Guo, A. C., Cheng, D., Shrivastava, S., Tzur, D., et al. (2008). DrugBank: a knowledgebase for drugs, drug actions and drug targets. Nucleic Acids Res. 36(Suppl. 1), 901–906. doi: 10.1093/nar/gkm958
Xiao, Z. Q., Wang, Y. L., Gan, S. R., and Chen, J. C. (2014). Polysaccharides from Liriopes Radix ameliorates hyperglycemia via various potential mechanisms in diabetic rats. J. Sci. Food. Agric. 94, 975–982. doi: 10.1002/jsfa.6347
Xu, J., Lian, F., Zhao, L., Zhao, Y., Chen, X., Zhang, X., et al. (2015). Structural modulation of gut microbiota during alleviation of type 2 diabetes with a Chinese herbal formula. ISME J. 9, 552. doi: 10.1038/ismej.2014.177
Yang, Z., Yang, J., Liu, W., Wu, L., Xing, L., Wang, Y., et al. (2013). T2D@ZJU: a knowledgebase integrating heterogeneous connections associated with type 2 diabetes mellitus. Database 2013, 446–447. doi: 10.1093/database/bat052
Ye, M., Qiu, T., Peng, W., Chen, W. X., Ye, Y. W., and Lin, Y. R. (2011). Purification, characterization and hypoglycemic activity of extracellular polysaccharides from Lachnum calyculiforme. Carbohydr. Polym. 86, 285–290. doi: 10.1016/j.carbpol.2011.04.051
Yin, W., Li, B., Li, X., Yu, F., Cai, Q., Zhang, Z., et al. (2015). Anti-inflammatory effects of grape seed procyanidin B2 on a diabetic pancreas. Food Funct. 6, 3065–3071. doi: 10.1039/c5fo00496a
Zhang, A., Sun, H., Yang, B., and Wang, X. (2012). Retraction note: predicting new molecular targets for rhein using network pharmacology. BMC Syst. Biol. 6:20. doi: 10.1186/1752-0509-6-20
Zhang, S., Lei, S., Li, Q., Wang, X., Li, S., Zhang, Y., et al. (2014). Systematic analysis of the multiple bioactivities of green tea through a network pharmacology approach. Evid. Based Compl. Alt. Med. 2014, 512081–512081. doi: 10.1155/2014/512081
Zhang, X., Gu, J., Cao, L., Li, N., Ma, Y., Su, Z., et al. (2014). Network pharmacology study on the mechanism of traditional Chinese medicine for upper respiratory tract infection. Mol. Biosyst. 10, 2517–2525. doi: 10.1039/c4mb00164h
Zhang, T. T., and Jiang, J. G. (2012). Active ingredients of traditional Chinese medicine in the treatment of diabetes and diabetic complications. Expert Opin. Investig. Drug 21, 1625–1642. doi: 10.1517/13543784.2012.713937
Zhao, F., Li, G., Yang, Y., Shi, L., Xu, L., and Yin, L. (2015). A network pharmacology approach to determine active ingredients and rationality of herb combinations of Modified-Simiaowan for treatment of gout. J. Ethnopharmacol. 117, 1–16. doi: 10.1016/j.jep.2015.03.035
Zhao, H. L., Sui, Y., Qiao, C. F., Yip, K. Y., Leung, R. K., Tsui, S. K., et al. (2012). Sustained antidiabetic effects of a berberine-containing Chinese herbal medicine through regulation of hepatic gene expression. Diabetes Metab. Res. Rev. 61, 933–943. doi: 10.2337/db11-1164
Zhao, W., Yin, Y., Yu, Z., Liu, J., and Chen, F. (2012). Comparison of anti-diabetic effects of polysaccharides from corn silk on normal and hyperglycemia rats. Int. J. Biol. Macromol. 50, 1133–1137. doi: 10.1016/j.ijbiomac.2012.02.004
Zhao, X. H., Qian, L., Yin, D. L., and Zhou, Y. (2013). Hypolipidemic effect of the polysaccharides extracted from pumpkin by cellulase-assisted method on mice. Int. J. Biol. Macromol. 64, 137–138. doi: 10.1016/j.ijbiomac.2013.12.001
Zheng, J., Yang, B., Yu, Y., Chen, Q., Huang, T., and Li, D. (2012). Ganoderma lucidum polysaccharides exert anti-hyperglycemic effect on streptozotocin-induced diabetic rats through affecting β-cells. Comb. Chem. High Throughput Screen. 15, 542–550. doi: 10.2174/138620712801619168
Zheng, S., Luo, X., Chen, G., Zhu, W., Shen, J., Chen, K., et al. (2005). A new rapid and effective chemistry space filter in recognizing a druglike database. J. Chem. Inf. Model. 45, 856–862. doi: 10.1021/ci050031j
Zhou, J., Yan, J., Bai, Z., Li, K., and Huang, K. (2015). Hypoglycemic activity and potential mechanism of a polysaccharide from the loach in streptozotocin-induced diabetic mice. Carbohydr. Polym. 121, 199–206. doi: 10.1016/j.carbpol.2014.12.037
Zhu, K. X., Nie, S. P., Li, C., Gong, D., and Xie, M. Y. (2014). Ganoderma atrum polysaccharide improves aortic relaxation in diabetic rats via PI3K/Akt pathway. Carbohydr. Polym. 103, 520–527. doi: 10.1016/j.carbpol.2013.12.080
Ziamajidi, N., Khaghani, S., Hassanzadeh, G., Vardasbi, S., Ahmadian, S., Nowrouzi, A., et al. (2013). Amelioration by chicory seed extract of diabetes- and oleic acid-induced non-alcoholic fatty liver disease (NAFLD)/non-alcoholic steatohepatitis (NASH) via modulation of PPARα and SREBP-1. Food Chem. Toxicol. 58, 198–209. doi: 10.1016/j.fct.2013.04.018
Zintzaras, E., Miligkos, M., Ziakas, P., Balk, E. M., Mademtzoglou, D., Doxani, C., et al. (2014). Assessment of the relative effectiveness and tolerability of treatments of type 2 diabetes mellitus: a network meta-analysis. Clin. Ther. 36, 1443–1453. doi: 10.1016/j.clinthera.2014.06.035
Keywords: network pharmacology, diabetes mellitus, natural products, database, mechanism
Citation: Li W, Yuan G, Pan Y, Wang C and Chen H (2017) Network Pharmacology Studies on the Bioactive Compounds and Action Mechanisms of Natural Products for the Treatment of Diabetes Mellitus: A Review. Front. Pharmacol. 8:74. doi: 10.3389/fphar.2017.00074
Received: 14 December 2016; Accepted: 06 February 2017;
Published: 23 February 2017.
Edited by:
Jianbo Xiao, University of Macau, MacauReviewed by:
Ake Sjoholm, University of South Alabama, USAAlessandro Martorana, University of Rome Tor Vergata, Italy
Copyright © 2017 Li, Yuan, Pan, Wang and Chen. This is an open-access article distributed under the terms of the Creative Commons Attribution License (CC BY). The use, distribution or reproduction in other forums is permitted, provided the original author(s) or licensor are credited and that the original publication in this journal is cited, in accordance with accepted academic practice. No use, distribution or reproduction is permitted which does not comply with these terms.
*Correspondence: Haixia Chen, Y2hlbmh4QHRqdS5lZHUuY24=