- Department of Medicine, Aab Cardiovascular Research Institute, University of Rochester School of Medicine and Dentistry, Rochester, NY, United States
In the post-genomic, big data era, our understanding of vascular diseases has been deepened by multiple state-of-the-art “–omics” approaches, including genomics, epigenomics, transcriptomics, proteomics, lipidomics and metabolomics. Genome-wide transcriptomic profiling, such as gene microarray and RNA-sequencing, emerges as powerful research tools in systems medicine and revolutionizes transcriptomic analysis of the pathological mechanisms and therapeutics of vascular diseases. In this article, I will highlight the workflow of transcriptomic profiling, outline basic bioinformatics analysis, and summarize recent gene profiling studies performed in vascular cells as well as in human and mice diseased samples. Further mining of these public repository datasets will shed new light on our understanding of the cellular basis of vascular diseases and offer novel potential targets for therapeutic intervention.
Introduction
According to a recent disease statistic report released by American Heart Association (AHA), cardiovascular diseases (CVD) remain the leading cause of death in America (Benjamin et al., 2017). The treatment of CVD also imposed a huge economic burden on the healthcare system (Benjamin et al., 2017). Deep understanding of the mechanism of CVD is a valuable approach for devising effective novel cardiovascular therapeutics.
With increasing number of transcriptomic studies (including microarray and RNA-sequencing) performed in cultured cells as well as in experimental mice or patients with CVD, we now have the capability to understand the influence of therapeutic intervention or gene perturbation on CVD outcome at genome-wide levels which were inaccessible in the past. However, the value of these transcriptomic data was always underestimated since most of the deposited data are not released to public until manuscripts are published. Therefore, it is critical to make large-scale efforts to mine, validate, and integrate the underlying information streams arising from various transcriptomics studies (Musunuru et al., 2017). To meet the increasing need of precision medicine, AHA has recently established the Institute for Precision Cardiovascular Medicine1, offering a new category of data-mining grants focused on harmonizing and mining CVD-based data for cardiovascular therapeutics. Therefore, in this article, I will summarize the workflow of transcriptomic profiling, basic bioinformatics analysis, and those profiling studies performed in vascular cells as well as human and mice diseased samples, aiming to provide a direct resource gallery in systems vascular medicine. Obviously, further mining of these publicly available datasets will provide a useful resource for understanding the cellular basis of atherosclerotic vascular diseases.
Overview of Transcriptomic Analysis
For analyzing a small number of gene transcripts, quantitative real-time PCR or pathway-focused (such as pathways of angiogenesis or endothelial cell biology) gene expression analysis using PCR arrays (such as RT2 Profiler PCR Arrays from Qiagen) can be used. In order to understand genome-wide influence of different conditions on CVD outcome, DNA microarray and RNA-sequencing (RNA-seq) are frequently used. Traditional transcriptomic analysis was mostly performed by using DNA microarray, which employs dye (Cy3, Cy5) hybridization-based technology to analyze differential gene expression pattern under certain conditions (such as gene knockout, or drug/stimuli treatment), although microarray has several technical limitations (de Franciscis et al., 2016; Haase et al., 2016). Recently, with the advent of next-generation sequencing technology, transcriptomic analysis has transitioned to RNA-seq (Wang et al., 2009), to quantify the amount of transcripts including protein-coding genes (mRNA), splice variants, as well as long non-coding RNA transcripts (lncRNA) in biological samples at genome-wide level (Mortazavi et al., 2008). Comparatively speaking, RNA-seq has the capability to identify more differentially expressed genes in various cell types than gene microarray (Wang et al., 2009; Zhang et al., 2014). In addition, there are also some commercial lncRNA array services available, such as Arraystar LncRNA Expression Arrays2 which systematically profile lncRNAs together with protein-coding mRNAs. A typical workflow of transcriptomic analysis involves several steps: (1) sample preparation; (2) RNA isolation by TRIzol or other commercial kits; (3) high-quality RNA submitted to Core facility or commercial companies for RNA-seq; or reverse transcription to cDNA for hybridization-based microarray analysis (Figure 1). To visualize the result of data analysis, gene expression values from both transcriptomic analyses can be represented as heat maps, listing the most significantly changed genes in assays. Downstream analysis of microarray and RNA-seq are quite similar, include gene ontology (GO) enrichment and pathway analysis as well as functionally classification of gene annotation (Yue and Reisdorf, 2005).
Advantages and Limitations of Transcriptome Profiling Technologies
Currently, microarrays remain a widely used approach for transcriptome studies due to its relatively low cost (readily affordable by many researchers) and ease to process large numbers of samples (Zhao et al., 2014). However, microarray has several limitations, most of which arise from probe and hybridization-related issues (probe performance and non-specific hybridization etc), such as high background level, difficult to detect very lowly expressed transcripts, and novel transcripts as well as splice variants (Draghici et al., 2006; Zhao et al., 2014). In contrast, RNA-seq has obvious advantages in these aspects (Russo et al., 2003; Wang et al., 2009; Zhao et al., 2014; Zhang et al., 2015) (Table 1): (1) Ability to detect novel transcripts; (2) Wider dynamic range of detection; (3) High signal-to-noise ratio; (4) High reproducibility and low variation. However, performing RNA-seq-based experiments is more expensive than microarray-based experiments, and requires extensive technical and bioinformatic expertise in data analysis (Zhao et al., 2014). The cost issue would potentially limit its application in experimental and clinical medicine. Moreover, a typical RNA-seq data in various formats was at the scale of GB depending on the number of samples tested. This presents a potential challenge for RAW data storage, processing, and analysis (Draghici et al., 2006). Fortunately, with recent technological advances, the costs for performing sequencing have declined; thus, RNA-seq is becoming more affordable than usual to users. Also various data depositing platforms (such as Gene Expression Ominbus and ArrayExpress) have emerged, and these platforms significantly solved the storage issue of large-scale RNA-seq RAW data. Readers are referred to references (Russo et al., 2003; Draghici et al., 2006; Wang et al., 2009; Zhao et al., 2014; Zhang et al., 2015) for details of the advantages and limitations of RNA-seq and microarray technology.
Database Search
Traditionally, transcriptomic data were included as supplementary information in published scientific literature. Nowadays, to meet the need of open data and data sharing, most of the transcriptomic profiling data were deposited in ArrayExpress3 and NCBI Gene Expression Ominbus (GEO)4. In this study, I will summarize part of the datasets that has been deposited in GEO database with supported publication records.
Basic Bioinformatics Analysis of Published Datasets
Currently, there are many softwares or websites that can help researchers analyze the data obtained from microarray and RNA-seq when uploading gene ID list. I summarize here some of the softwares and websites in Table 2. Basic bioinformatics analysis of transcriptomic data include the following (Yue and Reisdorf, 2005):
(1) Gene Ontology (GO) analysis. One of the main uses of the GO is to perform enrichment analysis of target gene sets. For example, given a set of genes that are up-regulated under certain conditions, an enrichment analysis will find which GO terms are over-represented (or under-represented) using annotations for that gene set. There are mainly three types of GO analysis, i.e., biological process, molecular function and cellular component.
(2) Pathway analysis: After transcriptomic studies, we may find many genes that are differentially expressed under certain conditions. To summarize the specific pathways that mediated by those genes, two most commonly used pathway analysis-PANTHER and KEGG2016 are frequently used.
(3) Venn diagram analysis of overlapping genes. If multiple RNA-seq or gene arrays were performed, finding the overlapping genes can be quickly achieved by using the venn diagram to show common genes (for example, overlapping genes upregulated by vector-based overexpression or pharmacological agonists, but downregulated by siRNA treatment or pharmacological inhibitor treatment). This would help define a common transcriptional program directed by target gene or therapeutic intervention.
Mining Transcriptomic Profiling Data
Due to the fact that gene microarray or RNA-seq generates big data that cannot be presented in regular format, therefore, it is critical to mine the data/information deposited in publicly available databases and perform related analysis.
Analytical Steps
(1) Enter GEO accession number in GEO database
(2) Click “analyze with GEO2R,” which allows users to identify differentially expressed genes across various experimental conditions.
(3) Define sample groups and assign all samples (technical/biological replicates) in each group to minimize technical variation and improve reproducibility as instructed5.
(4) Perform the test and analyze top 250 differentially expressed genes or all whole gene sets. Adjusted p-value (after multiple-test correction) and log FC (fold change) are two important parameters for mining the data.
An Example to Mine Gene Profiling Data
To exemplify the utility of data-mining, two transcriptomic studies GSE17939 (Ohnesorge et al., 2010) and GSE25145 (Clark et al., 2011), utilizing retrovirus-mediated overexpression of constitutively active mutant of MEK5 (MEK5-CA) in human primary endothelial cells was compared to study the transcriptiome of ERK5 activation. For simplicity, top 250 differentially expressed gene signature were mapped using venn diagram. As can be seen from Figure 2, transduction with MEK5-CA retrovirus, significantly altered 27 common genes in human umbilical vein endothelial cells and human dermal microvascular endothelial cells. Among the 27 genes, well-known downstream genes ensuing ERK5 activation, such as KLF2, KLF4, THBD, and TEK were identified. Venn diagram analysis also showed that MEK5-CA overexpression upregulates novel transcripts such as PLA1A and LINC00520, indicating both transcripts are potential MEK5 downstream effectors which may regulate endothelial function.
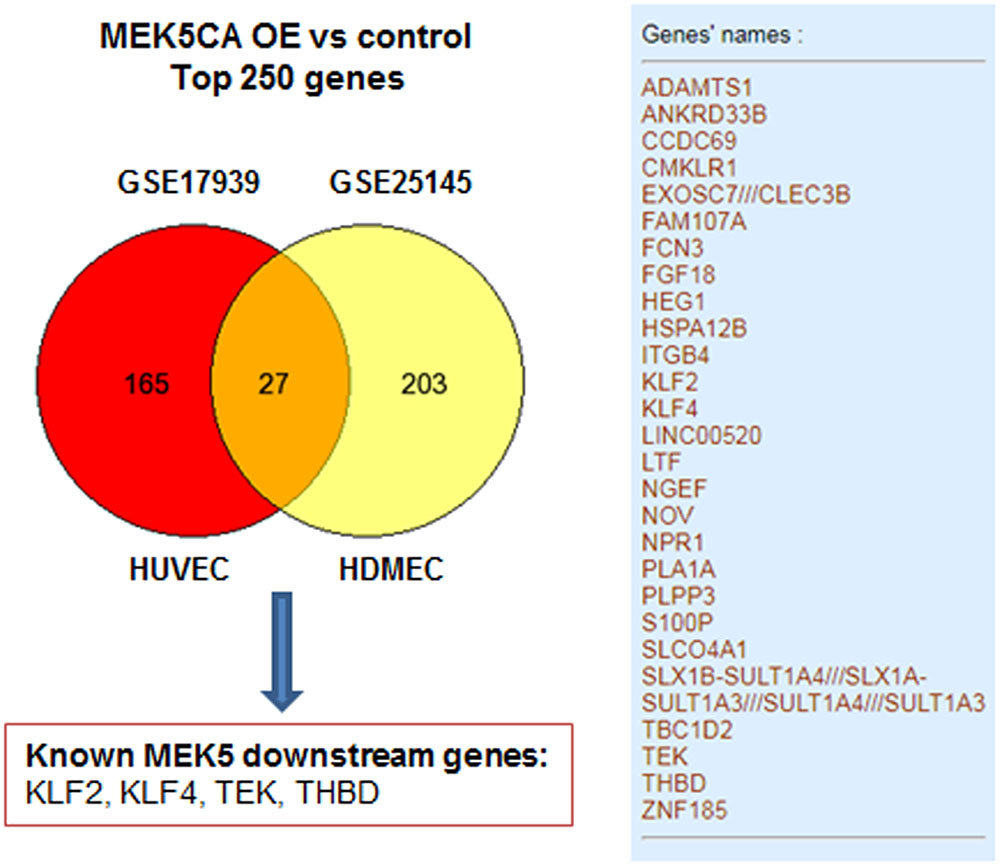
FIGURE 2. An example of mining existing data from public repository GSE17939 (Ohnesorge et al., 2010) and GSE25145 (Clark et al., 2011). A full list of 27 common genes revealed by both datasets was presented in the right panel. Total number of genes was less than 250 which is due to missing annotations in both datasets. MEK5CA OE, overexpression of constitutively active MEK5 mutant; HUVEC, human umbilical vein endothelial cells; HEMEC, Human dermal microvascular endothelial cells.
Transcriptomic Profiling in Cell, Animal Experiments and Human Patients
Transcriptomic comparisons would facilitate the identification of differentially expressed transcripts between human diseased and control samples, in different vascular cell types (endothelial cells, monocytes/macrophages, and smooth muscle cells), or in response to different pharmacological/genetic/environmental perturbations (Musunuru et al., 2017). Three common types of transcriptome profiling in vascular biology are summarized as below (Tables 3–5):
Human Diseased Samples vs. Controls
Comparing different expression profiles of genes in normal (disease-free, or mild disease, or adjacent non-disease regions) and pathological tissues in the majority of cases can represent both a cause and a consequence of the disease. Given the fate of atherosclerotic plaques can be divided into stabilized (asymptotic) and vulnerable plaques (symptomic), and most acute cardiovascular events are caused by the rupture of vulnerable plaques (Jackson, 2011), thus mining of these data will yield valuable information regarding key genes that regulate plaque stability.
Mice Diseased Samples vs. Controls as well as Cardiovascular Drugs Treatment vs. Control Treatment
Compared with human samples with vascular diseases, mice samples are easier to be obtained by diet/chemical induction. In this regard, ApoE-/- and LDLr-/- mice were two of the most frequently used mouse strains for transcriptomic analysis in vascular diseases, atherosclerosis and abdominal aorta aneurysms (AAAs) in particular (Emini Veseli et al., 2017). In addition, mice are very useful in evaluating vasculoprotective drugs. Comparing differential gene expression among aortas from hyperlipidemic mice treated with drugs or vehicle control could yield important mechanistic insights into drugs’ vasculoprotective actions and mechanisms.
Since hyperlipidemia represents a key risk factor that drives multiple cardiometabolic diseases including atherosclerosis, Novák et al. (2015) have recently reviewed miRNAs in cholesterol, fatty acid metabolism and atherosclerosis. This review highlights the complexity and importance of gene regulation by miRNA in the context of vascular diseases. However, the quest for disease-associated miRNA and target genes has been hampered by research tools, and fortunately, this difficulty can be tackled by computational prediction, followed by target validation (Lagana, 2015).
Gene Profiling Studies in Vascular Cells
Endothelial dysfunction is the underlying cause for multiple cardiometabolic diseases (Fang et al., 2017). However, endothelial dysfunction can be prevented by lipid-lowering statins, laminar flow, as well as naturally occuring phytochemicals. For example, recently, Maleszewska et al. (2016) has conducted RNA-seq analysis to interrogate the transcriptome of endothelial cells in response to fluid shear stress. This deep transcriptomic analysis of endothelial cells in the context of atheroprotective shear stress, together with other recently published transcriptomic profiling data (Qiao et al., 2016), constitute useful resources to further explore functions of mechanosensitive genes and lncRNAs in endothelial cell biology. There are also many deposited GEO datasets evaluating the effects of disease-associated stimuli (such as angiotensin II and oxidized LDL)/disease-modifying drugs (such as statins) on smooth muscle cells function (proliferation and migration) and macrophage function (inflammation, lipid loading, and polarization). In the GEO database, mining of these data will advance our understanding of the patho-mechanisms of atherosclerosis, which would potentially lead to novel anti-atherosclerotic therapy.
Discussion and Conclusion
Genome-wide transcriptomic analysis by microarray and RNA-seq emerge as powerful tools for translational research. Serval studies have conducted microarray and RNA-seq in parallel to compare the similarity and difference in transcriptome profiling of target cells/tissues and found that there is a high concordance between two technologies, suggesting the general suitability and reliability of both technologies (Bottomly et al., 2011; Sirbu et al., 2012; Zhao et al., 2014). Both technologies greatly transformed our traditional method of research into “discovery”-based method for mechanistic studies, allowing us to readily evaluate the cell-type and stimulus (or small-molecule drug)-specific regulation of gene expression. From these studies, readers can also mine data according to web-based instructions, and develop a working hypothesis to test whether gene X is involved in the development and progression of vascular diseases. Due to the complex nature of transcriptional regulation, the level of specific transcripts is determined by both transcriptional machinery and environment (such as diet, nutrient etc) (Musunuru et al., 2017). Together with recently emergence of single cell RNA-seq (Linnarsson and Teichmann, 2016), these biotechnological advances will provide powerful toolboxes for understanding the vascular transcriptome and represent an import step toward precision cardiovascular medicine.
Overall, RNA-seq is a high-throughput, and “discovery-based” approach for investigating transcriptome of different samples. It is more sensitive in detecting very lowly expressed genes or extremely highly expressed genes, therefore, offers a wider dynamic range than traditional microarray (Zhao et al., 2014). It is replacing microarray and thus becoming the predominant tool for transcriptome studies in basic, translational and clinical research (Musunuru et al., 2017). Noteworthy, RNA-seq is also a powerful tool for investigating non-coding RNA, lncRNAs in particular [such as SMILR (Ballantyne et al., 2016), MANTIS (Leisegang et al., 2017), LincRNA-p21 (Wu et al., 2014), Lnc-Ang362 (Leung et al., 2013), MYOSLID (Zhao et al., 2016), RNCR3 (Shan et al., 2016)], regulates many facets of vascular biology (reviewed in Li et al., 2016; Poller et al., 2017), demystifying the “dark” genome of vascular diseases. These lncRNA emerge as important players and therapeutic targets in vascular diseases. However, we have to bear in mind that: different variables of experimental conditions (such as cell types, treatment time, and animal models) pose a challenge to make quantifiable conclusions from published datasets. At least, by mining and comparing different datasets from different studies, we can gain a general comprehension on specific genes that are commonly regulated by the same treatment/disease.
The invention of new datamining tools/softwares is a good strategy to mine existing data. Recently, a web-based tool, Transcriptomine (Becnel et al., 2017), was developed to mine data on dissecting the effects of genetic or pharmacological interventions on nuclear receptor signaling. We can envisage that deep mining of the “hiden” data in vascular medicine will definitely accelerate biomarker discovery and prompts the identification and functional characterization of novel therapeutic targets (including coding genes and lncRNAs) in vascular diseases including atherosclerosis, AAA, and other rare-occuring vascular diseases. To conclude, mining expression profiling data from bench to bedside represents a cost-efficient new avenue for research of precision cardiovascular medicine.
Author Contributions
SX contributed to the conception, drafting, and revision of the manuscript and approved the final version.
Conflict of Interest Statement
The author declares that the research was conducted in the absence of any commercial or financial relationships that could be construed as a potential conflict of interest.
Footnotes
- ^https://professional.heart.org
- ^http://www.arraystar.com/lncrna-array-service/
- ^https://www.ebi.ac.uk/arrayexpress/
- ^https://www.ncbi.nlm.nih.gov/geo/
- ^https://www.ncbi.nlm.nih.gov/geo/info/geo2r.html
References
Abd Alla, J., El Faramawy, Y., and Quitterer, U. (2013). Microarray gene expression profiling reveals antioxidant-like effects of angiotensin II inhibition in atherosclerosis. Front. Physiol. 4:148. doi: 10.3389/fphys.2013.00148
Abd Alla, J., Graemer, M., Fu, X., and Quitterer, U. (2016). Inhibition of G-protein-coupled receptor kinase 2 prevents the dysfunctional cardiac substrate metabolism in fatty acid synthase transgenic mice. J. Biol. Chem. 291, 2583–2600. doi: 10.1074/jbc.M115.702688
Abd Alla, J., Langer, A., Elzahwy, S. S., Arman-Kalcek, G., Streichert, T., and Quitterer, U. (2010). Angiotensin-converting enzyme inhibition down-regulates the pro-atherogenic chemokine receptor 9 (CCR9)-chemokine ligand 25 (CCL25) axis. J. Biol. Chem. 285, 23496–23505. doi: 10.1074/jbc.M110.117481
Alexander, M. R., Moehle, C. W., Johnson, J. L., Yang, Z., Lee, J. K., Jackson, C. L., et al. (2012). Genetic inactivation of IL-1 signaling enhances atherosclerotic plaque instability and reduces outward vessel remodeling in advanced atherosclerosis in mice. J. Clin. Invest. 122, 70–79. doi: 10.1172/JCI43713
Ashburner, M., Ball, C. A., Blake, J. A., Botstein, D., Butler, H., Cherry, J. M., et al. (2000). Gene ontology: tool for the unification of biology. The Gene Ontology Consortium. Nat. Genet. 25, 25–29. doi: 10.1038/75556
Ayari, H., and Bricca, G. (2013). Identification of two genes potentially associated in iron-heme homeostasis in human carotid plaque using microarray analysis. J. Biosci. 38, 311–315. doi: 10.1007/s12038-013-9310-2
Ballantyne, M. D., Pinel, K., Dakin, R., Vesey, A. T., Diver, L., Mackenzie, R., et al. (2016). Smooth muscle enriched long noncoding RNA (SMILR) regulates cell proliferation. Circulation 133, 2050–2065. doi: 10.1161/CIRCULATIONAHA.115.021019
Bao, M. H., Luo, H. Q., Chen, L. H., Tang, L., Ma, K. F., Xiang, J., et al. (2016). Impact of high fat diet on long non-coding RNAs and messenger RNAs expression in the aortas of ApoE(-/-) mice. Sci. Rep. 6:34161. doi: 10.1038/srep34161
Becnel, L. B., Ochsner, S. A., Darlington, Y. F., McOwiti, A., Kankanamge, W. H., Dehart, M., et al. (2017). Discovering relationships between nuclear receptor signaling pathways, genes, and tissues in Transcriptomine. Sci. Signal. 10, 476. doi: 10.1126/scisignal.aah6275
Benjamin, E. J., Blaha, M. J., Chiuve, S. E., Cushman, M., Das, S. R., Deo, R., et al. (2017). Heart disease and stroke statistics-2017 update: a report from the American Heart Association. Circulation 135, e146–e603. doi: 10.1161/CIR.0000000000000485
Boerma, M., Burton, G. R., Wang, J., Fink, L. M., McGehee, R. E. Jr., and Hauer-Jensen, M. (2006). Comparative expression profiling in primary a and immortalized endothelial cells: changes in gene expression in response to hydroxy methylglutaryl-coenzyme A reductase inhibition. Blood Coagul. Fibrinolysis 17, 173–180. doi: 10.1097/01.mbc.0000220237.99843.a1
Boerma, M., Fu, Q., Wang, J., Loose, D. S., Bartolozzi, A., Ellis, J. L., et al. (2008). Comparative gene expression profiling in three primary human cell lines after treatment with a novel inhibitor of Rho kinase or atorvastatin. Blood Coagul. Fibrinolysis 19, 709–718. doi: 10.1097/MBC.0b013e32830b2891
Bottomly, D., Walter, N. A., Hunter, J. E., Darakjian, P., Kawane, S., Buck, K. J., et al. (2011). Evaluating gene expression in C57BL/6J and DBA/2J mouse striatum using RNA-Seq and microarrays. PLoS ONE 6:e17820. doi: 10.1371/journal.pone.0017820
Briot, A., Civelek, M., Seki, A., Hoi, K., Mack, J. J., Lee, S. D., et al. (2015). Endothelial NOTCH1 is suppressed by circulating lipids and antagonizes inflammation during atherosclerosis. J. Exp. Med. 212, 2147–2163. doi: 10.1084/jem.20150603
Bu, D. X., Rai, V., Shen, X., Rosario, R., Lu, Y., D’Agati, V., et al. (2010). Activation of the ROCK1 branch of the transforming growth factor-beta pathway contributes to RAGE-dependent acceleration of atherosclerosis in diabetic ApoE-null mice. Circ. Res. 106, 1040–1051. doi: 10.1161/CIRCRESAHA.109.201103
Chen, E. Y., Tan, C. M., Kou, Y., Duan, Q., Wang, Z., Meirelles, G. V., et al. (2013). Enrichr: interactive and collaborative HTML5 gene list enrichment analysis tool. BMC Bioinformatics 14:128. doi: 10.1186/1471-2105-14-128
Clark, P. R., Jensen, T. J., Kluger, M. S., Morelock, M., Hanidu, A., Qi, Z., et al. (2011). MEK5 is activated by shear stress, activates ERK5 and induces KLF4 to modulate TNF responses in human dermal microvascular endothelial cells. Microcirculation 18, 102–117. doi: 10.1111/j.1549-8719.2010.00071.x
Conway, D. E., Williams, M. R., Eskin, S. G., and McIntire, L. V. (2010). Endothelial cell responses to atheroprone flow are driven by two separate flow components: low time-average shear stress and fluid flow reversal. Am. J. Physiol. Heart Circ. Physiol. 298, H367–H374. doi: 10.1152/ajpheart.00565.2009
de Franciscis, S., Metzinger, L., and Serra, R. (2016). The discovery of novel genomic, transcriptomic, and proteomic biomarkers in cardiovascular and peripheral vascular disease: the state of the art. BioMed Res. Int. 2016:7829174. doi: 10.1155/2016/7829174
De Nardo, D., Labzin, L. I., Kono, H., Seki, R., Schmidt, S. V., Beyer, M., et al. (2014). High-density lipoprotein mediates anti-inflammatory reprogramming of macrophages via the transcriptional regulator ATF3. Nat. Immunol. 15, 152–160. doi: 10.1038/ni.2784
Derlindati, E., Dei Cas, A., Montanini, B., Spigoni, V., Curella, V., Aldigeri, R., et al. (2015). Transcriptomic analysis of human polarized macrophages: more than one role of alternative activation? PloS ONE 10:e0119751. doi: 10.1371/journal.pone.0119751
Doring, Y., Manthey, H. D., Drechsler, M., Lievens, D., Megens, R. T., Soehnlein, O., et al. (2012). Auto-antigenic protein-DNA complexes stimulate plasmacytoid dendritic cells to promote atherosclerosis. Circulation 125, 1673–1683. doi: 10.1161/CIRCULATIONAHA.111.046755
Draghici, S., Khatri, P., Eklund, A. C., and Szallasi, Z. (2006). Reliability and reproducibility issues in DNA microarray measurements. Trends Genet. 22, 101–109. doi: 10.1016/j.tig.2005.12.005
Ekstrand, J., Razuvaev, A., Folkersen, L., Roy, J., and Hedin, U. (2010). Tissue factor pathway inhibitor-2 is induced by fluid shear stress in vascular smooth muscle cells and affects cell proliferation and survival. J. Vasc. Surg. 52, 167–175. doi: 10.1016/j.jvs.2010.02.282
Emini Veseli, B., Perrotta, P., De Meyer, G. R. A., Roth, L., Van der Donckt, C., Martinet, W., et al. (2017). Animal models of atherosclerosis. Eur. J. Pharmacol. doi: 10.1016/j.ejphar.2017.05.010 [Epub ahead of print].
Fang, J., Little, P. J., and Xu, S. (2017). Atheroprotective effects and molecular targets of tanshinones derived from herbal medicine danshen. Med. Res. Rev. doi: 10.1002/med.21438 [Epub ahead of print].
Feldmann, R., Geikowski, A., Weidner, C., Witzke, A., Kodelja, V., Schwarz, T., et al. (2013). Foam cell specific LXRalpha ligand. PLoS ONE 8:e57311. doi: 10.1371/journal.pone.0057311
Gleissner, C. A., Shaked, I., Little, K. M., and Ley, K. (2010). CXC chemokine ligand 4 induces a unique transcriptome in monocyte-derived macrophages. J. Immunol. 184, 4810–4818. doi: 10.4049/jimmunol.0901368
Gold, E. S., Ramsey, S. A., Sartain, M. J., Selinummi, J., Podolsky, I., Rodriguez, D. J., et al. (2012). ATF3 protects against atherosclerosis by suppressing 25-hydroxycholesterol-induced lipid body formation. J. Exp. Med. 209, 807–817. doi: 10.1084/jem.20111202
Grootaert, M. O., da Costa Martins, P. A., Bitsch, N., Pintelon, I., De Meyer, G. R., Martinet, W., et al. (2015). Defective autophagy in vascular smooth muscle cells accelerates senescence and promotes neointima formation and atherogenesis. Autophagy 11, 2014–2032. doi: 10.1080/15548627.2015.1096485
Haase, T., Bornigen, D., Muller, C., and Zeller, T. (2016). Systems medicine as an emerging tool for cardiovascular genetics. Front. Cardiovasc. Med. 3:27. doi: 10.3389/fcvm.2016.00027
Hinterseher, I., Erdman, R., Donoso, L. A., Vrabec, T. R., Schworer, C. M., Lillvis, J. H., et al. (2011). Role of complement cascade in abdominal aortic aneurysms. Arterioscler. Thromb. Vasc. Biol. 31, 1653–1660. doi: 10.1161/ATVBAHA.111.227652
Hu, Y. W., Yang, J. Y., Ma, X., Chen, Z. P., Hu, Y. R., Zhao, J. Y., et al. (2014). A lincRNA-DYNLRB2-2/GPR119/GLP-1R/ABCA1-dependent signal transduction pathway is essential for the regulation of cholesterol homeostasis. J. Lipid Res. 55, 681–697. doi: 10.1194/jlr.M044669
Huang, T. S., Wang, K. C., Quon, S., Nguyen, P., Chang, T. Y., Chen, Z., et al. (2017). LINC00341 exerts an anti-inflammatory effect on endothelial cells by repressing VCAM1. Physiol. Genomics 49, 339–345. doi: 10.1152/physiolgenomics.00132.2016
Hulsen, T., de Vlieg, J., and Alkema, W. (2008). BioVenn - a web application for the comparison and visualization of biological lists using area-proportional Venn diagrams. BMC Genomics 9:488. doi: 10.1186/1471-2164-9-488
Ito, A., Hong, C., Rong, X., Zhu, X., Tarling, E. J., Hedde, P. N., et al. (2015). LXRs link metabolism to inflammation through Abca1-dependent regulation of membrane composition and TLR signaling. eLife 4:e08009. doi: 10.7554/eLife.08009
Jackson, S. P. (2011). Arterial thrombosis–insidious, unpredictable and deadly. Nat. Med. 17, 1423–1436. doi: 10.1038/nm.2515
Kim, T. W., Febbraio, M., Robinet, P., Dugar, B., Greene, D., Cerny, A., et al. (2011). The critical role of IL-1 receptor-associated kinase 4-mediated NF-kappaB activation in modified low-density lipoprotein-induced inflammatory gene expression and atherosclerosis. J. Immunol. 186, 2871–2880. doi: 10.4049/jimmunol.1002242
Kok, M. G., Halliani, A., Moerland, P. D., Meijers, J. C., Creemers, E. E., and Pinto-Sietsma, S. J. (2015). Normalization panels for the reliable quantification of circulating microRNAs by RT-qPCR. FASEB J. 29, 3853–3862. doi: 10.1096/fj.15-271312
Lagana, A. (2015). Computational prediction of microRNA Targets. Adv. Exp. Med. Biol. 887, 231–252. doi: 10.1007/978-3-319-22380-3_12
Lee, K., Santibanez-Koref, M., Polvikoski, T., Birchall, D., Mendelow, A. D., and Keavney, B. (2013). Increased expression of fatty acid binding protein 4 and leptin in resident macrophages characterises atherosclerotic plaque rupture. Atherosclerosis 226, 74–81. doi: 10.1016/j.atherosclerosis.2012.09.037
Leisegang, M. S., Fork, C., Josipovic, I., Richter, F., Preussner, J., Hu, J., et al. (2017). Long noncoding RNA MANTIS facilitates endothelial angiogenic function. Circulation 136, 65–79. doi: 10.1161/CIRCULATIONAHA.116.026991
Lenk, G. M., Tromp, G., Weinsheimer, S., Gatalica, Z., Berguer, R., and Kuivaniemi, H. (2007). Whole genome expression profiling reveals a significant role for immune function in human abdominal aortic aneurysms. BMC Genomics 8:237. doi: 10.1186/1471-2164-8-237
Leung, A., Trac, C., Jin, W., Lanting, L., Akbany, A., Saetrom, P., et al. (2013). Novel long noncoding RNAs are regulated by angiotensin II in vascular smooth muscle cells. Circ. Res. 113, 266–278. doi: 10.1161/CIRCRESAHA.112.300849
Li, H., Zhu, H., and Ge, J. (2016). Long noncoding RNA: recent updates in atherosclerosis. Int. J. Biol. Sci. 12, 898–910. doi: 10.7150/ijbs.14430
Linnarsson, S., and Teichmann, S. A. (2016). Single-cell genomics: coming of age. Genome Biol. 17, 97. doi: 10.1186/s13059-016-0960-x
Liu, L., Liu, Y., Liu, C., Zhang, Z., Du, Y., and Zhao, H. (2016). Analysis of gene expression profile identifies potential biomarkers for atherosclerosis. Mol. Med. Rep. 14, 3052–3058. doi: 10.3892/mmr.2016.5650
Maegdefessel, L., Spin, J. M., Raaz, U., Eken, S. M., Toh, R., Azuma, J., et al. (2014). miR-24 limits aortic vascular inflammation and murine abdominal aneurysm development. Nat. Commun. 5, 5214. doi: 10.1038/ncomms6214
Maere, S., Heymans, K., and Kuiper, M. (2005). BiNGO: a Cytoscape plugin to assess overrepresentation of gene ontology categories in biological networks. Bioinformatics 21, 3448–3449. doi: 10.1093/bioinformatics/bti551
Maleszewska, M., Vanchin, B., Harmsen, M. C., and Krenning, G. (2016). The decrease in histone methyltransferase EZH2 in response to fluid shear stress alters endothelial gene expression and promotes quiescence. Angiogenesis 19, 9–24. doi: 10.1007/s10456-015-9485-2
Mattaliano, M. D., Huard, C., Cao, W., Hill, A. A., Zhong, W., Martinez, R. V., et al. (2009). LOX-1-dependent transcriptional regulation in response to oxidized LDL treatment of human aortic endothelial cells. Am. J. Physiol. Cell Physiol. 296, C1329–C1337. doi: 10.1152/ajpcell.00513.2008
Minta, J., Jungwon Yun, J., and St Bernard, R. (2010). Microarray analysis of ox-LDL (oxidized low-density lipoprotein)-regulated genes in human coronary artery smooth muscle cells. Cell Biol. Int. Rep. 17:e00007. doi: 10.1042/CBR20100006
Mortazavi, A., Williams, B. A., McCue, K., Schaeffer, L., and Wold, B. (2008). Mapping and quantifying mammalian transcriptomes by RNA-Seq. Nat. Methods 5, 621–628. doi: 10.1038/nmeth.1226
Mun, G. I., Lee, S. J., An, S. M., Kim, I. K., and Boo, Y. C. (2009). Differential gene expression in young and senescent endothelial cells under static and laminar shear stress conditions. Free Radic. Biol. Med. 47, 291–299. doi: 10.1016/j.freeradbiomed.2009.04.032
Musunuru, K., Ingelsson, E., Fornage, M., Liu, P., Murphy, A. M., Newby, L. K., et al. (2017). The expressed genome in cardiovascular diseases and stroke: refinement, diagnosis, and prediction: a scientific statement from the American Heart Association. Circ. Cardiovasc. Genet. doi: 10.1161/HCG.0000000000000037 [Epub ahead of print].
Novák, J., Olejnickova, V., Tkacova, N., and Santulli, G. (2015). Mechanistic Role of MicroRNAs in coupling lipid metabolism and atherosclerosis. Adv. Exp. Med. Biol. 887, 79–100. doi: 10.1007/978-3-319-22380-3_5
Ohnesorge, N., Viemann, D., Schmidt, N., Czymai, T., Spiering, D., Schmolke, M., et al. (2010). Erk5 activation elicits a vasoprotective endothelial phenotype via induction of Kruppel-like factor 4 (KLF4). J. Biol. Chem. 285, 26199–26210. doi: 10.1074/jbc.M110.103127
Oteng, A. B., Bhattacharya, A., Brodesser, S., Qi, L., Tan, N. S., and Kersten, S. (2017). Feeding Angptl4-/- mice trans fat promotes foam cell formation in mesenteric lymph nodes without leading to ascites. J. Lipid Res. 58, 1100–1113. doi: 10.1194/jlr.M074278
O’Toole, T. E., Abplanalp, W., Li, X., Cooper, N., Conklin, D. J., Haberzettl, P., et al. (2014). Acrolein decreases endothelial cell migration and insulin sensitivity through induction of let-7a. Toxicol. Sci. 140, 271–282. doi: 10.1093/toxsci/kfu087
Pirola, L., Balcerczyk, A., Tothill, R. W., Haviv, I., Kaspi, A., Lunke, S., et al. (2011). Genome-wide analysis distinguishes hyperglycemia regulated epigenetic signatures of primary vascular cells. Genome Res. 21, 1601–1615. doi: 10.1101/gr.116095.110
Poller, W., Dimmeler, S., Heymans, S., Zeller, T., Haas, J., Karakas, M., et al. (2017). Non-coding RNAs in cardiovascular diseases: diagnostic and therapeutic perspectives. Eur. Heart J. doi: 10.1093/eurheartj/ehx165 [Epub ahead of print].
Qiao, C., Meng, F., Jang, I., Jo, H., Chen, Y. E., and Zhang, J. (2016). Deep transcriptomic profiling reveals the similarity between endothelial cells cultured under static and oscillatory shear stress conditions. Physiol. Genomics 48, 660–666. doi: 10.1152/physiolgenomics.00025.2016
Ramsey, S. A., Vengrenyuk, Y., Menon, P., Podolsky, I., Feig, J. E., Aderem, A., et al. (2014). Epigenome-guided analysis of the transcriptome of plaque macrophages during atherosclerosis regression reveals activation of the Wnt signaling pathway. PLoS Genet. 10:e1004828. doi: 10.1371/journal.pgen.1004828
Rayner, K. J., Sheedy, F. J., Esau, C. C., Hussain, F. N., Temel, R. E., Parathath, S., et al. (2011). Antagonism of miR-33 in mice promotes reverse cholesterol transport and regression of atherosclerosis. J. Clin. Invest. 121, 2921–2931. doi: 10.1172/JCI57275
Reschen, M. E., Gaulton, K. J., Lin, D., Soilleux, E. J., Morris, A. J., Smyth, S. S., et al. (2015). Lipid-induced epigenomic changes in human macrophages identify a coronary artery disease-associated variant that regulates PPAP2B Expression through Altered C/EBP-beta binding. PLoS Genet. 11:e1005061. doi: 10.1371/journal.pgen.1005061
Rigassi, L., Barchiesi Bozzolo, F., Lucchinetti, E., Zaugg, M., Fingerle, J., Rosselli, M., et al. (2015). 2-Methoxyestradiol blocks the RhoA/ROCK1 pathway in human aortic smooth muscle cells. Am. J. Physiol. Endocrinol. Metab. 309, E995–E1007. doi: 10.1152/ajpendo.00267.2015
Rush, C., Nyara, M., Moxon, J. V., Trollope, A., Cullen, B., and Golledge, J. (2009). Whole genome expression analysis within the angiotensin II-apolipoprotein E deficient mouse model of abdominal aortic aneurysm. BMC Genomics 10:298. doi: 10.1186/1471-2164-10-298
Russo, G., Zegar, C., and Giordano, A. (2003). Advantages and limitations of microarray technology in human cancer. Oncogene 22, 6497–6507. doi: 10.1038/sj.onc.1206865
Shan, K., Jiang, Q., Wang, X. Q., Wang, Y. N., Yang, H., Yao, M. D., et al. (2016). Role of long non-coding RNA-RNCR3 in atherosclerosis-related vascular dysfunction. Cell Death Dis. 7, e2248. doi: 10.1038/cddis.2016.145
Sirbu, A., Kerr, G., Crane, M., and Ruskin, H. J. (2012). RNA-Seq vs dual- and single-channel microarray data: sensitivity analysis for differential expression and clustering. PLoS ONE 7:e50986. doi: 10.1371/journal.pone.0050986
Spin, J. M., Hsu, M., Azuma, J., Tedesco, M. M., Deng, A., Dyer, J. S., et al. (2011). Transcriptional profiling and network analysis of the murine angiotensin II-induced abdominal aortic aneurysm. Physiol. Genom. 43, 993–1003. doi: 10.1152/physiolgenomics.00044.2011
Tabet, F., Vickers, K. C., Cuesta Torres, L. F., Wiese, C. B., Shoucri, B. M., Lambert, G., et al. (2014). HDL-transferred microRNA-223 regulates ICAM-1 expression in endothelial cells. Nat. Commun. 5, 3292. doi: 10.1038/ncomms4292
Tozawa, H., Kanki, Y., Suehiro, J., Tsutsumi, S., Kohro, T., Wada, Y., et al. (2011). Genome-wide approaches reveal functional interleukin-4-inducible STAT6 binding to the vascular cell adhesion molecule 1 promoter. Mol. Cell. Biol. 31, 2196–2209. doi: 10.1128/MCB.01430-10
Tuomisto, T. T., Lumivuori, H., Kansanen, E., Hakkinen, S. K., Turunen, M. P., van Thienen, J. V., et al. (2008). Simvastatin has an anti-inflammatory effect on macrophages via upregulation of an atheroprotective transcription factor, Kruppel-like factor 2. Cardiovasc. Res. 78, 175–184. doi: 10.1093/cvr/cvn007
Van Assche, T., Hendrickx, J., Crauwels, H. M., Guns, P. J., Martinet, W., Fransen, P., et al. (2011). Transcription profiles of aortic smooth muscle cells from atherosclerosis-prone and -resistant regions in young apolipoprotein E-deficient mice before plaque development. J. Vasc. Res. 48, 31–42. doi: 10.1159/000317398
Van Campenhout, A., Moran, C. S., Parr, A., Clancy, P., Rush, C., Jakubowski, H., et al. (2009). Role of homocysteine in aortic calcification and osteogenic cell differentiation. Atherosclerosis 202, 557–566. doi: 10.1016/j.atherosclerosis.2008.05.031
Verschuren, L., Radonjic, M., Wielinga, P. Y., Kelder, T., Kooistra, T., van Ommen, B., et al. (2012). Systems biology analysis unravels the complementary action of combined rosuvastatin and ezetimibe therapy. Pharmacogenet. Genomics 22, 837–845. doi: 10.1097/FPC.0b013e328359d274
Wang, Z., Gerstein, M., and Snyder, M. (2009). RNA-Seq: a revolutionary tool for transcriptomics. Nat. Rev. Genet. 10, 57–63. doi: 10.1038/nrg2484
White, S. J., Hayes, E. M., Lehoux, S., Jeremy, J. Y., Horrevoets, A. J., and Newby, A. C. (2011). Characterization of the differential response of endothelial cells exposed to normal and elevated laminar shear stress. J. Cell. Physiol. 226, 2841–2848. doi: 10.1002/jcp.22629
Wolf, S. C., Sauter, G., Jobst, J., Kempf, V. A., Risler, T., and Brehm, B. R. (2008). Major differences in gene expression in human coronary smooth muscle cells after nebivolol or metoprolol treatment. Int. J. Cardiol. 125, 4–10. doi: 10.1016/j.ijcard.2007.02.045
Woods, C. G., Fu, J., Xue, P., Hou, Y., Pluta, L. J., Yang, L., et al. (2009). Dose-dependent transitions in Nrf2-mediated adaptive response and related stress responses to hypochlorous acid in mouse macrophages. Toxicol. Appl. Pharmacol. 238, 27–36. doi: 10.1016/j.taap.2009.04.007
Wu, G., Cai, J., Han, Y., Chen, J., Huang, Z. P., Chen, C., et al. (2014). LincRNA-p21 regulates neointima formation, vascular smooth muscle cell proliferation, apoptosis, and atherosclerosis by enhancing p53 activity. Circulation 130, 1452–1465. doi: 10.1161/CIRCULATIONAHA.114.011675
Yue, L., and Reisdorf, W. C. (2005). Pathway and ontology analysis: emerging approaches connecting transcriptome data and clinical endpoints. Curr. Mol. Med. 5, 11–21. doi: 10.2174/1566524053152906
Zhang, W., Yu, Y., Hertwig, F., Thierry-Mieg, J., Zhang, W., Thierry-Mieg, D., et al. (2015). Comparison of RNA-seq and microarray-based models for clinical endpoint prediction. Genome Biol. 16, 133. doi: 10.1186/s13059-015-0694-1
Zhang, Y., Chen, K., Sloan, S. A., Bennett, M. L., Scholze, A. R., O’Keeffe, S., et al. (2014). An RNA-sequencing transcriptome and splicing database of glia, neurons, and vascular cells of the cerebral cortex. J. Neurosci. 34, 11929–11947. doi: 10.1523/JNEUROSCI.1860-14.2014
Zhao, J., Zhang, W., Lin, M., Wu, W., Jiang, P., Tou, E., et al. (2016). MYOSLID is a novel serum response factor-dependent long noncoding RNA that amplifies the vascular smooth muscle differentiation program. Arterioscler. Thromb. Vasc. Biol. 36, 2088–2099. doi: 10.1161/ATVBAHA.116.307879
Keywords: transcriptome, microarray, RNA-sequencing, vascular medicine, gene profiling, long non-coding RNA
Citation: Xu S (2017) Transcriptome Profiling in Systems Vascular Medicine. Front. Pharmacol. 8:563. doi: 10.3389/fphar.2017.00563
Received: 10 July 2017; Accepted: 08 August 2017;
Published: 25 August 2017.
Edited by:
Weien Yuan, Shanghai Jiao Tong University, ChinaReviewed by:
Liborio Stuppia, Università degli Studi “G. d’Annunzio" Chieti – Pescara, ItalyGaetano Santulli, Columbia University, United States
Kristen J. Bubb, University of Sydney, Australia
Copyright © 2017 Xu. This is an open-access article distributed under the terms of the Creative Commons Attribution License (CC BY). The use, distribution or reproduction in other forums is permitted, provided the original author(s) or licensor are credited and that the original publication in this journal is cited, in accordance with accepted academic practice. No use, distribution or reproduction is permitted which does not comply with these terms.
*Correspondence: Suowen Xu, suowen.xu@gmail.com; suowen_xu@urmc.rochester.edu