- Department of Pharmacy, School of Chemistry, National Autonomous University of Mexico, Mexico City, Mexico
Naturally occurring small molecules include a large variety of natural products from different sources that have confirmed activity against epigenetic targets. In this work we review chemoinformatic, molecular modeling, and other computational approaches that have been used to uncover natural products as inhibitors of DNA methyltransferases, a major family of epigenetic targets with therapeutic interest. Examples of computational approaches surveyed in this work are docking, similarity-based virtual screening, and pharmacophore modeling. It is also discussed the chemoinformatic-guided exploration of the chemical space of naturally occurring compounds as epigenetic modulators which may have significant implications in epigenetic drug discovery and nutriepigenetics.
Section 1: Introduction
Epigenetics has been defined as a change in phenotype without an underlying change in genotype (Berger et al., 2009). In the 1940s Waddington suggested the term “epigenetics” trying to describe “the interactions of genes with their environment, which brings the phenotype into being” (Waddington, 2012). Alterations in epigenetic modifications have been related to several diseases including cancer, diabetes, neurodegenerative disorders, and immune-mediated diseases (Dueñas-González et al., 2016; Tough et al., 2016; Hwang et al., 2017; Lu et al., 2018). Moreover, epigenetic targets are also attractive for the treatment of antiparasitic infections (Sacconnay et al., 2014).
In epigenetic drug discovery, epigenetic targets have been classified into three main groups (Ganesan, 2018). “Writers” are enzymes that catalyze the addition of a functional group to a protein or nucleic acid; “readers” are macromolecules that function as recognition units that can distinguish a native macromolecule vs. the modified one; and “erasers” that are enzymes that aid in the removal of chemical modifications introduced by the writers. Thus far, several targets from these three major families have reached different stages of drug discovery, ranging from lead discovery, preclinical development, clinical trials and approval. Currently, there are seven compounds approved for clinical use (Ganesan, 2018).
DNA methyltransferases (DNMTs) are a family of “writer” enzymes responsible for DNA methylation that is the addition of a methyl group to the carbon atom number five (C5) of cytosine. As surveyed in this work, since DNA methylation has an essential role for cell differentiation and development, alterations in the function of DNMTs have been associated with cancer (Castillo-Aguilera et al., 2017) and other diseases (Lyko, 2017).
Several natural products have been identified as inhibitors of epigenetic targets including DNMTs. Most of these compounds have been uncovered fortuitously. However, there are recent efforts to screen systematically natural products as DNMT inhibitors. The vastness of the chemical space of natural products led to the hypothesis that many more active compounds could potentially been identified. Indeed, it has been estimated that more than 95% of the biodiversity in nature remains to be explored to identify potential bioactive molecules (Ho et al., 2018).
The aim of this work is to discuss a broad range of computational methods to identify novel inhibitors of DNMTs from natural products. The manuscript also discusses the chemical space of natural products as inhibitors of DNMTs. The manuscript is organized into nine sections. After this introduction, Section 2 reviews briefly the structure of DNMTs including different isoforms. The next section covers major aspects of the function of DNMTs including the mechanism of methylation. Section 4 reviews currently known inhibitors of DNMTs from natural sources including food chemicals. Section 5 discusses the epigenetic relevant chemical space of natural products comparing the chemical space of DNMT inhibitors from natural sources vs. other compounds. The next section reviews computational strategies that are used to identify natural compounds as potential epi-hits or epi-leads targeting DNMTs. Sections 7 and 8 presents Summary conclusions and Perspectives, respectively.
Section 2: Structure of Dnmts
The human genome encodes DNMT1, DNMT2, DNMT3A, DNMT3B, and DNMT3L. While DNMT1, DNMT3A, and DNMT3B have catalytic activity, DNMT2 and DNMT3L do not (Lyko, 2017). DNMT1 is a maintenance methyltransferase, responsible for duplicating the pattern of DNA methylation during replication. DNMT1 is essential for proper mammalian development and it has been proposed as the most interesting target for experimental cancer therapies (Dueñas-González et al., 2016). DNMT3A and DNMT3B are de novo methyltransferases. Human DNMT1 has 1616 amino acids whose structure can be divided into an N-terminal regulatory domain and a C-terminal catalytic domain (Jeltsch, 2002; Jurkowska et al., 2011). The N-terminal domain contains a replication foci-targeting domain, a DNA-binding CXXC domain, and a pair of bromo-adjacent homology domains. The C-terminal catalytic domain has 10 amino acid motifs. The cofactor and substrate binding sites in the C-terminal catalytic domain are comprised of motif I and X and motif IV, VI, and VIII, respectively (Lan et al., 2010). The target recognition domain which is maintained by motif IX and involved in DNA recognition, is not conserved between the DNMT family. Figure 1A shows a three-dimensional (3D) model of a DNMT1 (PDB ID: 4WXX) (Zhang et al., 2015). Figure 1B shows a schematic diagram of human of DNMT1, 2, 3A, 3B, and L.
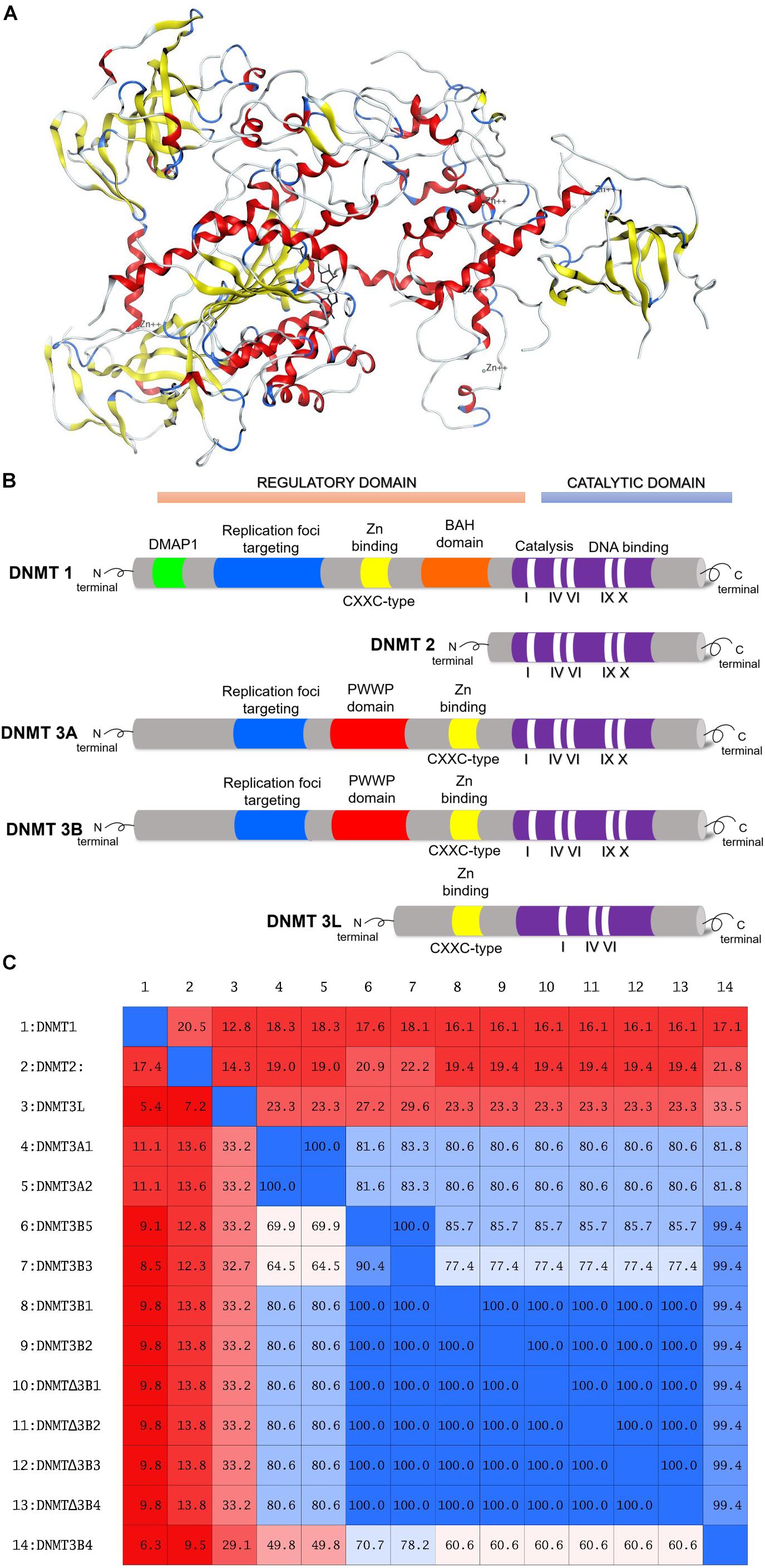
FIGURE 1. (A) Three-dimensional model of DNMT1, amino acid residues 351–1600. Figure rendered from the Protein Data Bank PDB ID: 4WXX. (B) Schematic diagram of the structure of human DNMT1, DNMT2, DNMT3A, DNMT3B, and DNMT3L. (C) Identity matrix of the catalytic site of 14 DNMTs isoforms. Note that there is a significant difference in the sequence of DNMT1, DNMT2, and DNMT3L.
Section 2.1: Isoforms
Two isoforms of DNMT3A have been identified, DNMT3A1 and DNMT3A2. At the N-terminal domain both isoforms have a PWWP (Pro-Trp-Trp-Pro) and an ADD (ATRX-DNMT3-DNMT3L) domains (Jurkowska et al., 2011). The C-terminal domain is identical in the two isoforms (Choi et al., 2011).
There are more than 30 isoforms of DNMT3B, however, only DNMT3B1 and DNMT3B2 are catalytically active (Ostler et al., 2007). Similar to DNMT3A, DNMT3B1, and DNMT3B2 have a PWWP and ADD domains at the N-terminal region (Lyko, 2017). The rest of the isoforms are not catalytically active. Some of these such as DNMT3B3, DNMT3B4, and DNMT3B7 are overexpressed in many tumor cell lines (Gordon et al., 2013). ΔDNMT3B has seven isoforms and lacks 200 amino acids from the N-terminal region of DNMT3B (Wang et al., 2006). ΔDNMT3B1–4 possess catalytic activity whereas ΔDNMT3B5–7 lacks the catalytic domain (Wang et al., 2006). ΔDNMT3B is mainly expressed in non-small cell lung cancer (Wang et al., 2006; Ostler et al., 2007). Figure 1C shows the identity matrix of 14 DNMTs isoforms. The identity matrix indicates that the amino acid sequence at the catalytic site of DNMT3A1 and DNMT3A2 isoforms is identical. In the same manner, the amino acid sequence at the C-terminal domain of the catalytically active isoforms DNMT3B1, DNMT3B2, and ΔDNMT3B1–4 are identical. DNMT1, DNMT2, and DNMT3L show a significant difference in the sequence of the catalytic site with respect to the rest of the isoforms. Therefore, it can be anticipated that is possible to identify or design selective inhibitors for these isoforms.
Section 3: Function and Mechanism of Dnmts
As outlined in Section 2, cytosine-5 DNMTs catalyze the addition of methylation marks to genomic DNA. All DNMTs have a related catalytic mechanism that is featured by the formation of a covalent adduct intermediate between the enzyme and the substrate base. All DNMTs use S-adenosyl-L-methionine (SAM) as the methyl group donor (Vilkaitis et al., 2001; Du et al., 2016). DNMT forms a complex with DNA and the cytosine which will be methylated flips out from the DNA (Klimasauskas et al., 1994). A conserved cysteine performs a nucleophilic attack to the six-position of the target cytosine yielding a covalent intermediate. The five-position of the cytosine is activated and conducts a nucleophilic attack on the cofactor SAM to form the 5-methyl covalent adduct and S-adenosyl-L-homocysteine (SAH). The attack on the six-position is aided by a transient protonation of the cytosine ring at the endocyclic nitrogen atom N3, which can be stabilized by a glutamate and arginine residues. The covalent complex between the methylated base and the DNA is resolved by deprotonation at the five-position to generate the methylated cytosine and the free enzyme.
Section 4: Known Inhibitors of Dnmts From Natural Sources
Thus far more than 500 compounds have been tested as inhibitors of DNMTs. The structural diversity and coverage in chemical space has been analyzed using chemoinformatic methods (Fernandez-de Gortari and Medina-Franco, 2015). The chemical space of DNMT inhibitors has been compared with inhibitors of other epigenetic targets (Naveja and Medina-Franco, 2018). Furthermore, the structure-activity relationships (SAR) of DNMT inhibitors using the concept of activity landscape has been documented (Naveja and Medina-Franco, 2015).
DNA methyltransferase inhibitors have been obtained from a broad number of different strategies including organic synthesis, virtual, and high-throughput screening (Medina-Franco et al., 2015). Organic synthesis has been employed in several instances for lead optimization (Castellano et al., 2008; Kabro et al., 2013; Davide et al., 2016). Natural products and food chemicals have also been a major source of active compounds. Natural products that are known to act as DNMT inhibitors or demethylating agents have been extensively reviewed by Zwergel et al. (2016). These natural products are of the type polyphenols, flavonoids, anthraquinones, and other classes. Some of the first natural products described were curcumin, (-)-epigallocatechin-3-gallate (EGCG), mahanine, genistein, and quercetin. Other natural products that have described as inhibitors of DNMT or demethylating agents are silibinin, luteolin, kazinol Q, laccaic acid, hypericin, boswellic acid, and lycopene. Figure 2 shows the chemical structure of representative DNMT inhibitors with emphasis on compounds from natural origin.
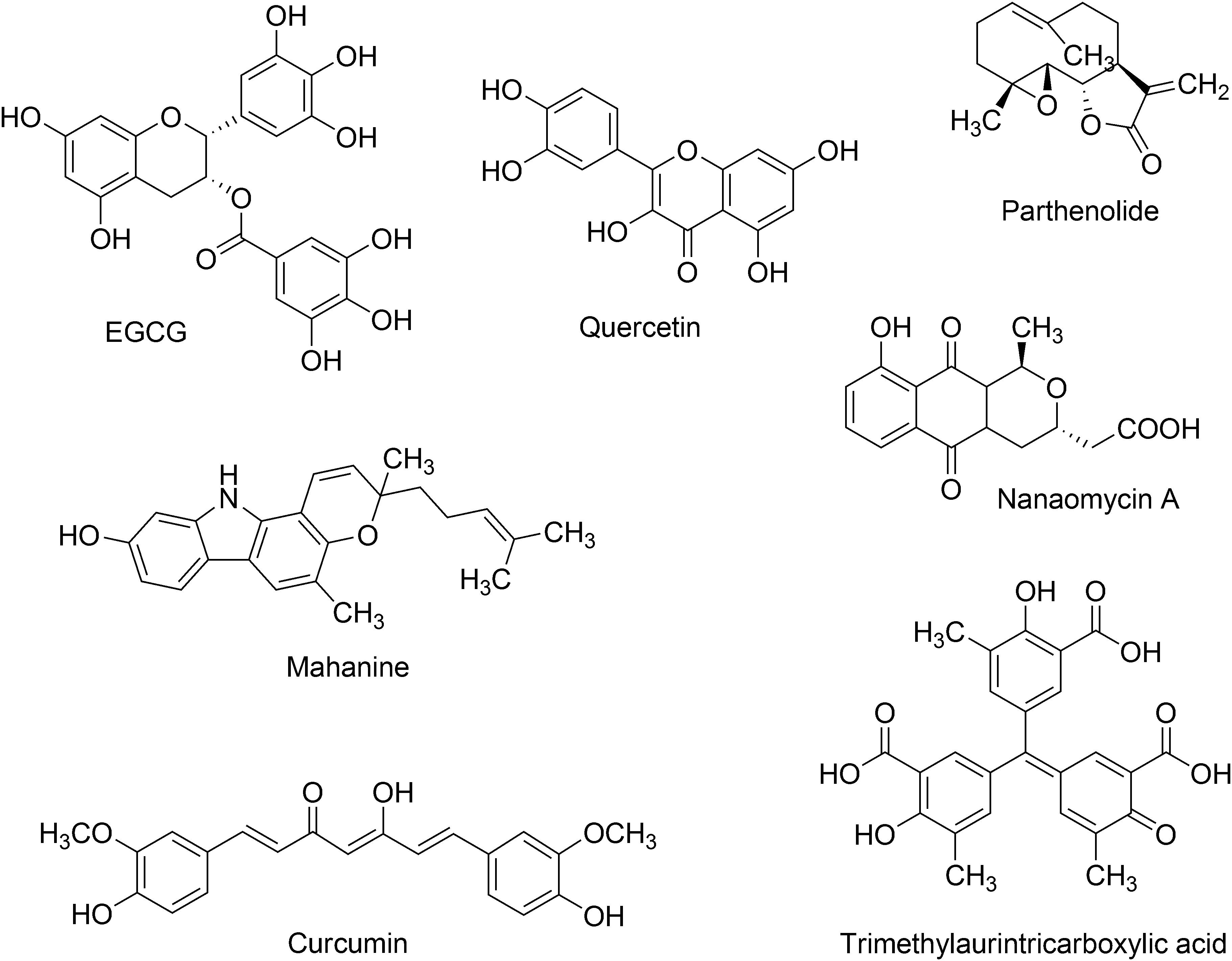
FIGURE 2. Chemical structures of representative inhibitors of DNMTs from natural sources. These natural products are extensively reviewed by Zwergel et al. (2016).
The bioactivity profile and potency in enzymatic and/or cell-based assays of these natural products have been discussed in detail by Zwergel et al. (2016). Of note, it will be valuable if all natural products could have been screened under the same conditions. For few natural products the selectivity has been characterized being nanaomycin A an exception (vide infra). Indeed, for about eight natural products the IC50 has been measured in enzymatic based assays. Despite the fact that the potency of the natural products with DNMTs is not very high in enzymatic-based assays, e.g., IC50 between 0.5 and 10 μM, several natural products have shown promising activity in cell-based assays. Notably, natural products have distinct chemical scaffolds that could be used as a starting point in lead optimization efforts. Moreover, quercetin in combination with green tea extract has advanced into phase I clinical trials for the treatment of prostate cancer.
Most of the natural products with demethylating activity or ability to inhibit DNA methyltransferases in enzymatic assays have been identified fortuitously. However, as discussed in this work, there are efforts toward the identification of bioactive demethylating agents using systematic approaches such a virtual screening. Indeed, the natural product nanaomycin A (Figure 2) was identified from a virtual screening campaign initially focused on the identification of inhibitors of DNMT1. The quinone-based antibiotic isolated from Streptomyces showed antiproliferative effects in three human tumor cell lines, HCT116, A549, and HL60 after 72 h of treatment. Moreover, nanaomycin A showed reduced global methylation levels in all three cell lines when tested at concentrations ranging from 0.5 to 5 μM. Nanaomycin A reactivated the transcription of the RASSF1A tumor suppressor gene inducing its expression up to 18-fold at 5 μM, higher than the reference drug 5-azacytidine (sixfold at 25 μM). In an enzymatic inhibitory assay, nanaomycin A was selective toward DNMT3B with an IC50 = 0.50 μM.
Section 4.1: Natural Products and Food Chemicals
It is remarkable that several natural products are used as dietary sources such as curcumin, caffeic acid and chlorogenic acid found in Coffea arabica, genistein found in soybean, quercetin found in fruits, vegetables, and beverages. Of course, there is a large overlap between the chemical space of food chemicals and natural products (Naveja et al., 2018). This has given rise to systematically screen food chemical databases for potential regulators of epigenetic targets.
Section 5: Epigenetic Relevant Chemical Space of Natural Products: Focus on Dnmt Inhibitors
In drug discovery it is generally accepted that a major benefit of natural products vs. purely synthetic organic molecules is, overall, the feasibility of the former to exert a biological activity and increased chemical diversity (Ho et al., 2018). The chemical space of natural products is vast and its molecular diversity has been quantified over the past few years (López-Vallejo et al., 2012; Olmedo et al., 2017; Shang et al., 2018). A major contribution to these studies has been the increasing availability of natural products collections in the public domain (Medina-Franco, 2015). Examples of major compound collections are the Traditional Chinese Medicine (Chen, 2011), natural products from Brazil – NuBBE (Pilon et al., 2017), AfroDb (Ntie-Kang et al., 2013) or collections available for screening in a medium to high-throughput screening mode. The large importance of natural products in drug discovery has boosted the development of open access applications to mine these rich repositories. Few examples are ChemGPS-NP, TCMAnalyzer, and other resources described elsewhere (Rosen et al., 2009; Chen et al., 2017; Gonzalez-Medina et al., 2017; Liu et al., 2018).
The chemical space of natural products from different sources has been compared to several other collections including the chemical space of drugs approved for clinical use and synthetic compounds (Olmedo et al., 2017; Shang et al., 2018). These studies demonstrate that the chemical space of natural products is vast, that there is a notable overlap with the chemical space of drugs, and that natural products cover novel regions of the chemical space. The overlap with the chemical space of approved drugs is not that surprising since there are a large percentage of drugs from natural origin. Figure 3 shows a visual representation of the chemical space of 15 representative DNMT inhibitors from natural sources vs. 4103 compounds from a commercial vendor library of natural products, 206 fungi metabolites, and 6253 marine natural products (Krishna et al., 2017). The visual representation was generated with principal component analysis of six physicochemical properties of pharmaceutical relevance, namely molecular weight (MW), topological surface area (TPSA), number of hydrogen bond donors and acceptors (HBD/HBA), number of rotatable bonds (RB), and octanol/water partition coefficient (logP). The first two principal components capture about 90% of the total variance. The visual representation of the chemical space in this figure indicates that marine natural products (data points in blue) cover a broader area of the chemical space followed by natural products in the vendor collection (orange) and by fungi metabolites (green). DNMT inhibitors from natural origin (purple) are, in general, inside the subspace of the DNMT1 inhibitors (red). This visualization of the chemical space indicates that there would be expected to identify more DNMT1 inhibitors in the marine and vendor collections, as well as in the data set of fungi metabolites.
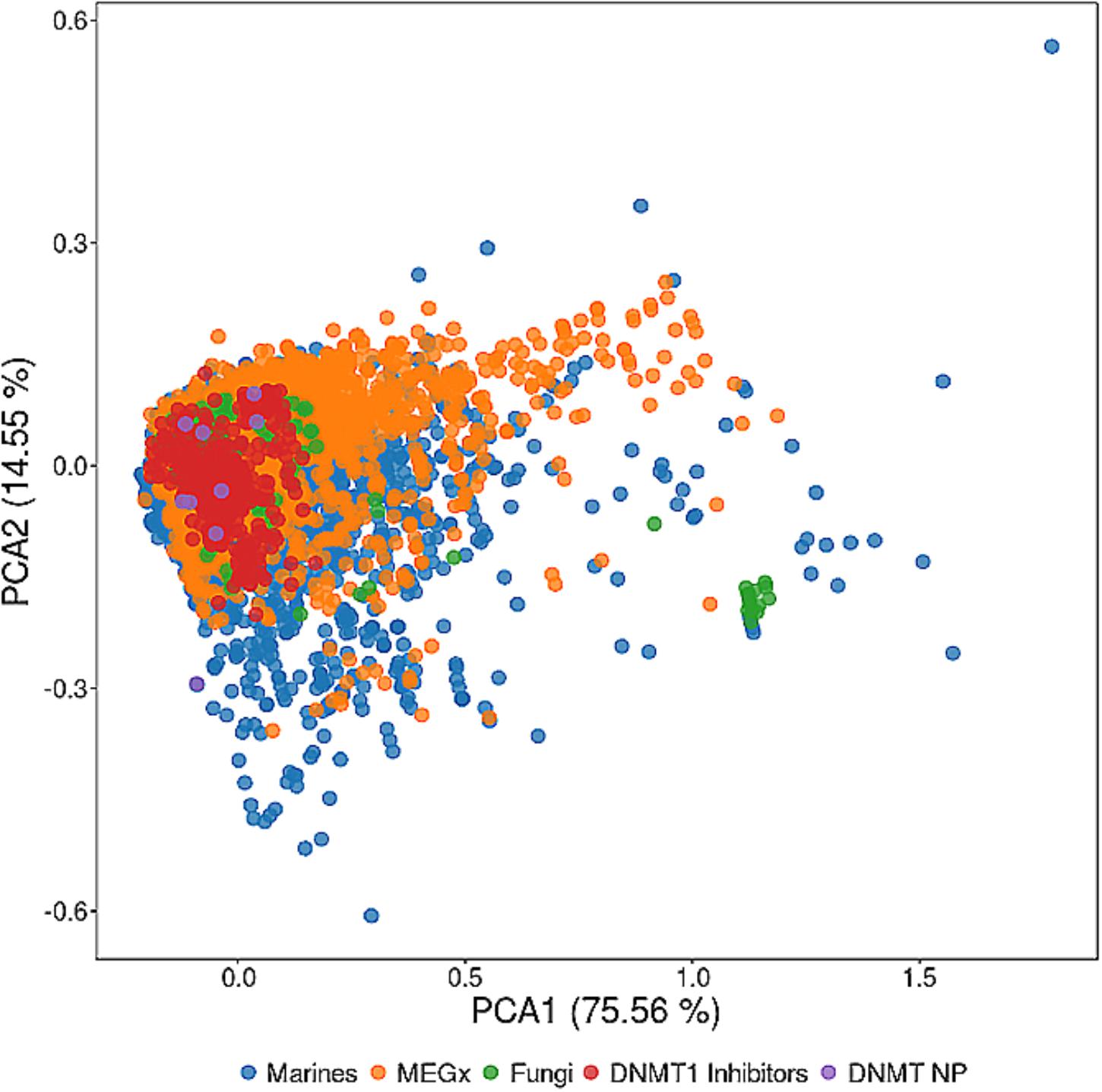
FIGURE 3. Visualization and comparison of the chemical space of DNMT inhibitors from natural sources (DNMT NP) vs. DNMT1 inhibitors and different natural products data sets. The visual representation of the chemical space was based on principal component analysis of six physicochemical properties of pharmaceutical interest. The percentage of variance is shown on each axis of the plot.
Section 6: Opportunities for Searching for Natural Products as Dnmt Inhibitors
Most of the DNMT inhibitors from natural sources have been identified by serendipity. As discussed in Section 5, the chemical space of natural products and food chemicals can be explored in a systematic manner using computational approaches. A classical and general approach is using virtual screening. The main aim of virtual screening is filtering compound data sets to select a reduced number of compounds with increased probability to show biological activity. Virtual screening has proven to be useful to identify hit compounds (Clark, 2008; Lavecchia and Di Giovanni, 2013). Table 1 summarizes representative case studies where virtual screening has led to the identification of active compounds with novel scaffolds. In other studies, virtual screening has uncovered potential active compounds but experimental validation still needs to be conducted. Examples of these studies are further discussed in the following sections.
There are several published studies of virtual screening of natural products to identify DNMT inhibitors and/or demethylating agents. In an early work, Medina-Franco et al. (2011) reported the screening of a lead-like subset of natural products available in ZINC. Authors of that work implemented a multistep virtual screening approach selecting consensus hits identified from three different docking programs. One computational hit showed DNMT1 activity in a previous study. Other candidate compounds were identified for later experimental validation (Medina-Franco et al., 2011).
In a separate work, Maldonado-Rojas et al. (2015) developed a QSAR model based on linear discriminant analysis to screen 800 natural products. Hits selected were further docked with two crystallographic structures of human DNMT employing two docking programs. Six consensus hits were identified as potential inhibitors (Maldonado-Rojas et al., 2015).
Virtual screening of synthetic libraries has also been reported to identify active compounds with novel scaffolds and suitable for lead optimization. For instance, Chen et al. (2014) reported a docking-based virtual screening of the commercial screening compound library SPECS with 111,121 molecules (after filtering compounds with undesirable physicochemical properties). Results of that work led to the identification of a compound with a novel scaffold with low micromolar IC50 (10.3 μM). Starting from the computational hit, similarity searching led to the identification of two more potent compounds.
Hassanzadeh et al. (2017) recently reported a pharmacophore-based virtual screening of a compound database with 500 compounds. The pharmacophore was generated using a ligand-based approach by superimposing a group of active nucleoside analogs. Selected hits, which are structurally related to the barbituric acid, were docked into the substrate binding site of DNMT1. One compound was identified with a novel chemical scaffold that inhibits DNMT1 in the low micromolar range (IC50 = 4.1 μM). The compound also showed some selectivity on DNMT1 over DNMT3 enzymes (Hassanzadeh et al., 2017).
Krishna et al. (2017) implemented a virtual screening protocol using several structure- and ligand-based approaches. Methods included a pharmacophore model, a Naïve Bayesian classification model, and ensemble docking. Three out of ten selected compounds from a commercial library of synthetic molecules (e.g., Maybridge with 53,000 small drug-like compounds), showed DNMT1 inhibitory activity at compound concentration of 20 μM. Two of these molecules showed activity at 1 μM (Krishna et al., 2017).
In addition to the studies discussed above and summarized in Table 1, the next subsections discuss other approaches that can be explored. Case studies for each strategy are outlined briefly.
Section 6.1: Similarity-Based Virtual Screening of Natural Products
Similarity searching is a commonly used approach for identifying new hit compounds. Major goals are identifying starting points for later optimization or expand the SAR of analog series. Since similarity searching is fast it can be used to filter large chemical databases and it can be used in combination with other computational approaches such as molecular docking.
Similarity searching involves two major components: a molecular representation and a similarity coefficient. In practice, one of the most common molecular representations are two-dimensional (2D) fingerprints. A fingerprint is generally a string of zeros and ones that indicate the presence or absence of molecular features, respectively. In turn, one of the most common similarity coefficients is Tanimoto’s (Bajusz et al., 2015). Full discussion of molecular representations and similarity coefficients are published elsewhere (Willett et al., 1998; Maggiora et al., 2014).
A novel approach to encode the chemical structures of data sets is the database fingerprint (DBFP) (Fernández-de Gortari et al., 2017). The rationale of DBFP is account for the most structural features encoded in bit positions of an entire data set. In principle, virtually any data set can be represented. For instance, it can be a small or large chemical database of screening compounds or a group of active compounds. DBFP can be used in visual representation of the chemical space (Naveja and Medina-Franco, 2018) and similarity searching (Fernández-de Gortari et al., 2017). More recently, this approach was further refined into the so-called statistical based database fingerprint (SB-DFP). This approach has the same underlying idea and application of DBFP. A key improvement is the approach to account for the most relevant structural features that are derived from a statistical comparison between the structural features of a data set of interest vs. a database of reference.
Section 6.2: Pharmacophore-Based
Thus far, several pharmacophore modeling studies have been conducted for inhibitors of DNMT1. Different approaches and input molecules have been used to develop these models. Most of the pharmacophore models have been employed to virtually screen chemical databases and identify novel hit compounds.
Yoo and Medina-Franco (2011) reported one of the first pharmacophore models for inhibitors of DNMT1. The model was generated based on the docking poses of 14 known inhibitors available at that time. The docking was conducted with a homology model of the catalytic domain of DNMT1. Of note, at the time of that study the crystallographic structure of human DNMT1 was not available. Known DNMT inhibitors used to develop the pharmacophore model included the natural products curcumin, parthenolide, EGCG and mahanine (Yoo and Medina-Franco, 2011). A year later was reported that trimethylaurintricarboxylic acid (Figure 2) showed a good agreement with this structure-based pharmacophore model. This compound is structurally related to 5,5′-methylenedisalicylic acid that has an inhibition of DNMT1 in a low micromolar range (IC50 = 4.79 μM) (Yoo and Medina-Franco, 2012; Yoo et al., 2012).
More recently, as described in the first part of Section 6, Hassanzadeh et al. (2017) developed a pharmacophore model based on a ligand-based approach by 3D superimposition of active nucleoside analogs. That model was used to do virtual screening (vide supra). In the same year, with the aid of the Hypogen module of the software DS4.1, Krishna et al. (2017) developed a ligand-based pharmacophore model using the structures of 20 compounds obtained from the literature. The model was validated with the classification of an external set with known active and inactive compounds. The validated pharmacophore models were employed as part of a combined strategy to identify novel active molecules (Krishna et al., 2017).
Section 6.3: De novo Design
De novo design is a technique currently explored for DNMT inhibitors on a limited basis. Here we briefly outline two promising perspectives related to natural product research. The first one is a strategy that provides a structural diversity classification of natural products scaffolds through generative topographic map algorithm implementation often so-called chemographies. Chemographies allow the visualization of the landscape distribution of the chemical space of natural products and their synthetic mimetic compounds (Miyao et al., 2015). Since chemographies could be generated from pharmacophoric features and molecular descriptors, it would be feasible to do scaffold hopping based on the structures of natural products (Rodrigues et al., 2016). The second approach is based on scaffold simplification that could be adapted to generate fragment-like natural products focused on DNMT inhibitors. This strategy reduces the molecular framework of natural products through the implementation of a scaffold tree algorithm based on rule-based decomposition of ring systems (Bajorath, 2018).
Section 7: Conclusion
Epigenetic targets are attractive to develop therapeutic strategies. DNA methyltransferases are the major enzyme family being one of the first epigenetic targets studied, in particular for the treatment of cancer. However, over the past few years, more therapeutic opportunities related to the modulation of DNMTs are emerging. Therefore, there is a growing interest in the scientific community to identify and develop small molecules that can be used as epi-drugs or epi-probes targeting DNMTs. Virtual screening has become more used in recent years to uncover natural products as inhibitors of DNMTs and/or demethylating agents. To this end, well stablished structure- and ligand-based virtual screening approaches are being used such as automated docking, QSAR and similarity searching. Also, novel chemoinformatic approaches are being developed. Of course, the computational methods should be validated with rigorous experiments in vitro and in vivo experiments to support their application.
Natural products have a well stablished history as inhibitors of DNMTs and demethylating molecules. However, most of the active natural products have been identified by serendipity. The knowledge of the three-dimensional structures of DNMTs in combination with increased in silico approaches and better computational resources are boosting the systematic search of bioactive molecules from natural origin. In addition, the increasing availability of natural product databases facilitates the discovery of epi-drugs and epi-probes targeting DNMTs.
Section 8: Perspectives
Natural products inside or outside of the traditional drug-like chemical space represent a large promise to develop novel compounds with DNMT inhibitory activity or demethylating properties. This is because the traditional chemical space is highly represented by small molecules that over the past few years have not been very successful. A notable example in this direction is the reemergence of peptide-based drug discovery. Indeed, linear, cyclic peptides and peptidomimetics are regaining interest in drug discovery (Fosgerau and Hoffmann, 2015; Henninot et al., 2018).
Other promising an emerging avenue are the modulators of protein–protein interactions (PPIs) (Díaz-Eufracio et al., 2018). DNMTs are known to be involved in several PPIs (Díaz-Eufracio et al., 2018). Modulation of such interactions can be conveniently achieved with natural products. This is because PPIs are “difficult targets” not easily addressed by small molecules from the traditional chemical space (Villoutreix et al., 2014). In other words, since PPIs have unique features these can be approached with novel chemical libraries. Natural products collections represent excellent candidates for this purpose.
We foresee an augmented hit and led identification efforts based on natural products combining approaches such as high-throughput screening, structure-, ligand-based in silico screening, structure-based optimization, similarity searching, and scaffold hopping (Schneider et al., 1999). As part of the search for novel and more potent compounds is crucial to consider potential toxicity since toxicity issues play a major part in the lack of success of drug discovery projects.
Disclaimer
A similar version of this manuscript was deposited in a Pre-Print server on July 6, 2018. The reference is: Saldívar-González, F. I.; Gómez-García, A.; Sánchez-Cruz, N.; Ruiz-Rios, J.; Pilón-Jiménez, B. A.; Medina-Franco, J. L. Computational Approaches to Identify Natural Products as Inhibitors of DNA Methyltransferases. Preprints 2018, 2018070116 (doi: 10.20944/preprints201807.0116.v1).
Author Contributions
All authors contributed to methodology and formal analysis. FS-G, JR-R, and BP-J contributed to data curation. AG-G, FS-G, DC-PdL, and JM-F contributed to writing-original draft preparation. AG-G, FS-G, NS-G, and JM-F contributed to writing-review and editing. AG-G, FS-G, and BP-J contributed to visualization. JM-F contributed to project administration.
Funding
This research was funded by Consejo Nacional de Ciencia y Tecnologia (CONACYT, Mexico) grant number 282785, the Programa de Apoyo a Proyectos de Investigación e Innovación Tecnológica (PAPIIT) grant IA203718, and by Programa de Apoyo a Proyectos para la Innovación y Mejoramiento de la Enseñanza (PAPIME) grant PE200118, UNAM.
Conflict of Interest Statement
The authors declare that the research was conducted in the absence of any commercial or financial relationships that could be construed as a potential conflict of interest.
Acknowledgments
FS-G, AG-G, and NS-C acknowledge Consejo Nacional de Ciencia y Tecnologia (CONACyT, Mexico) for the graduate scholarships. DC-PdL and JR-R thanks the Programa de Apoyo a Proyectos para la Innovación y Mejoramiento de la Enseñanza (PAPIME) for the undergraduate scholarship. The authors also thank Chanachai Sae-Lee for providing the sequences used in Figure 1. They also acknowledge all current and past members of the DIFACQUIM research group for their comments and discussions that enriched this manuscript.
References
Bajorath, J. (2018). Improving the utility of molecular scaffolds for medicinal and computational chemistry. Future Med. Chem. 10, 1645–1648. doi: 10.4155/fmc-2018-0106
Bajusz, D., Rácz, A., and Héberger, K. (2015). Why is Tanimoto index an appropriate choice for fingerprint-based similarity calculations? J. Cheminform. 7:20. doi: 10.1186/s13321-015-0069-3
Berger, S. L., Kouzarides, T., Shiekhattar, R., and Shilatifard, A. (2009). An operational definition of epigenetics. Genes Dev. 23, 781–783. doi: 10.1101/gad.1787609
Castellano, S., Kuck, D., Sala, M., Novellino, E., Lyko, F., and Sbardella, G. (2008). Constrained analogues of procaine as novel small molecule inhibitors of DNA methyltransferase-1. J. Med. Chem. 51, 2321–2325. doi: 10.1021/jm7015705
Castillo-Aguilera, O., Depreux, P., Halby, L., Arimondo, P., and Goossens, L. (2017). DNA methylation targeting: the DNMT/HMT crosstalk challenge. Biomolecules 7:3. doi: 10.3390/biom7010003
Chen, C. Y.-C. (2011). TCM Database@Taiwan: the world’s largest traditional Chinese medicine database for drug screening in silico. PLoS One 6:e15939. doi: 10.1371/journal.pone.0015939
Chen, S. J., Wang, Y. L., Zhou, W., Li, S. S., Peng, J. L., Shi, Z., et al. (2014). Identifying novel selective non-nucleoside DNA methyltransferase 1 inhibitors through docking-based virtual screening. J. Med. Chem. 57, 9028–9041. doi: 10.1021/jm501134e
Chen, Y., de Bruyn Kops, C., and Kirchmair, J. (2017). Data resources for the computer-guided discovery of bioactive natural products. J. Chem. Inf. Model. 57, 2099–2111. doi: 10.1021/acs.jcim.7b00341
Choi, S. H., Heo, K., Byun, H.-M., An, W., Lu, W., and Yang, A. S. (2011). Identification of preferential target sites for human DNA methyltransferases. Nucleic Acids Res. 39, 104–118. doi: 10.1093/nar/gkq774
Clark, D. E. (2008). What has virtual screening ever done for drug discovery? Expert Opin. Drug Discov. 3, 841–851. doi: 10.1517/17460440802281978
Davide, G., Sandra, A., Emily, B., Mattia, C., Marta, G., Annalisa, C., et al. (2016). Design and synthesis of N-benzoyl amino acid derivatives as DNA methylation inhibitors. Chem. Biol. Drug Des. 88, 664–676. doi: 10.1111/cbdd.12794
Díaz-Eufracio, B. I., Naveja, J. J., and Medina-Franco, J. L. (2018). Chapter three - protein–protein interaction modulators for epigenetic therapies. Adv. Protein Chem. Struct. Biol. 110, 65–84. doi: 10.1016/bs.apcsb.2017.06.002
Du, Q., Wang, Z., and Schramm, V. L. (2016). Human DNMT1 transition state structure. Proc. Natl. Acad. Sci. U.S.A. 113, 2916–2921. doi: 10.1073/pnas.1522491113
Dueñas-González, A., Jesús Naveja, J., and Medina-Franco, J. L. (2016). Chapter 1 - Introduction of Epigenetic Targets in Drug Discovery and Current Status of Epi-Drugs and Epi-Probes, in Epi-Informatics. Boston, MA: Academic Press, 1–20. doi: 10.1016/B978-0-12-802808-7.00001-0
Fernández-de Gortari, E., García-Jacas, C. R., Martinez-Mayorga, K., and Medina-Franco, J. L. (2017). Database fingerprint (DFP): an approach to represent molecular databases. J. Cheminform. 9:9. doi: 10.1186/s13321-017-0195-1
Fernandez-de Gortari, E., and Medina-Franco, J. L. (2015). Epigenetic relevant chemical space: a chemoinformatic characterization of inhibitors of DNA methyltransferases. RSC Adv. 5, 87465–87476. doi: 10.1039/C5RA19611F
Fosgerau, K., and Hoffmann, T. (2015). Peptide therapeutics: current status and future directions. Drug Discov. Today 20, 122–128. doi: 10.1016/j.drudis.2014.10.003
Ganesan, A. (2018). Epigenetic drug discovery: a success story for cofactor interference. Philos. Trans. R. Soc. B Biol. Sci. 373:20170069. doi: 10.1098/rstb.2017.0069
Gonzalez-Medina, M., Naveja, J. J., Sanchez-Cruz, N., and Medina-Franco, J. L. (2017). Open chemoinformatic resources to explore the structure, properties and chemical space of molecules. RSC Adv. 7, 54153–54163. doi: 10.1039/C7RA11831G
Gordon, C. A., Hartono, S. R., and Chédin, F. (2013). Inactive DNMT3B splice variants modulate de novo DNA methylation. PLoS One 8:e69486. doi: 10.1371/journal.pone.0069486
Henninot, A., Collins, J. C., and Nuss, J. M. (2018). The current state of peptide drug discovery: back to the future? J. Med. Chem. 61, 1382–1414. doi: 10.1021/acs.jmedchem.7b00318
Ho, T. T., Tran, Q. T. N., and Chai, C. L. L. (2018). The polypharmacology of natural products. Future Med. Chem. 10, 1361–1368. doi: 10.4155/fmc-2017-0294
Hwang, J.-Y., Aromolaran, K. A., and Zukin, R. S. (2017). The emerging field of epigenetics in neurodegeneration and neuroprotection. Nat. Rev. Neurosci. 18, 347–361. doi: 10.1038/nrn.2017.46
Jeltsch, A. (2002). Beyond Watson and crick: DNA methylation and molecular enzymology of DNA methyltransferases. ChemBioChem 3, 274–293. doi: 10.1002/1439-7633(20020402)3:4<274::AID-CBIC274>3.0.CO;2-S
Jurkowska, R. Z., Jurkowski, T. P., and Jeltsch, A. (2011). Structure and function of mammalian DNA methyltransferases. ChemBioChem 12, 206–222. doi: 10.1002/cbic.201000195
Kabro, A., Lachance, H., Marcoux-Archambault, I., Perrier, V., Dore, V., Gros, C., et al. (2013). Preparation of phenylethylbenzamide derivatives as modulators of DNMT3 activity. MedChemComm 4, 1562–1570. doi: 10.1039/c3md00214d
Klimasauskas, S., Kumar, S., Roberts, R. J., and Cheng, X. D. (1994). HHAL methyltransferase flips its target base out of the DNA helix. Cell 76, 357–369. doi: 10.1016/0092-8674(94)90342-5
Krishna, S., Shukla, S., Lakra, A. D., Meeran, S. M., and Siddiqi, M. I. (2017). Identification of potent inhibitors of DNA methyltransferase 1 (DNMT1) through a pharmacophore-based virtual screening approach. J. Mol. Graph. Model. 75, 174–188. doi: 10.1016/j.jmgm.2017.05.014
Lan, J., Hua, S., He, X. N., and Zhang, Y. (2010). DNA methyltransferases and methyl-binding proteins of mammals. Acta Biochim. Biophys. Sin. 42, 243–252. doi: 10.1093/abbs/gmq015
Lavecchia, A., and Di Giovanni, C. (2013). Virtual screening strategies in drug discovery: a critical review. Curr. Med. Chem. 20, 2839–2860. doi: 10.2174/09298673113209990001
Liu, Z., Du, J., Yan, X., Zhong, J., Cui, L., Lin, J., et al. (2018). TCMAnalyzer: a chemo- and bioinformatics web service for analyzing traditional Chinese medicine. J. Chem. Inf. Model. 58, 550–555. doi: 10.1021/acs.jcim.7b00549
López-Vallejo, F., Giulianotti, M. A., Houghten, R. A., and Medina-Franco, J. L. (2012). Expanding the medicinally relevant chemical space with compound libraries. Drug Discov. Today 17, 718–726. doi: 10.1016/j.drudis.2012.04.001
Lu, W., Zhang, R., Jiang, H., Zhang, H., and Luo, C. (2018). Computer-aided drug design in epigenetics. Front. Chem. 6:57. doi: 10.3389/fchem.2018.00057
Lyko, F. (2017). The DNA methyltransferase family: a versatile toolkit for epigenetic regulation. Nat. Rev. Genet. 19, 81–92. doi: 10.1038/nrg.2017.80
Maggiora, G., Vogt, M., Stumpfe, D., and Bajorath, J. (2014). Molecular similarity in medicinal chemistry. J. Med. Chem. 57, 3186–3204. doi: 10.1021/jm401411z
Maldonado-Rojas, W., Olivero-Verbel, J., and Marrero-Ponce, Y. (2015). Computational fishing of new DNA methyltransferase inhibitorsfrom natural products. J. Mol. Graph. Model. 60, 43–54. doi: 10.1016/j.jmgm.2015.04.010
Hassanzadeh, M., Kasymov, R., Mahernia, S., Adib, M., Emperle Michael Dukatz, M., Bashtrykov, P., et al. (2017). Discovery of novel and selective DNA methyltransferase 1 inhibitors by pharmacophore and docking-based virtual screening. ChemistrySelect 2, 8383–8392. doi: 10.1002/slct.201701734
Medina-Franco, J. L. (2015). “Discovery and development of lead compounds from natural sources using computational approaches,” in Evidence-Based Validation of Herbal Medicine, ed. P. Mukherjee (New York, NY: Elsevier), 455–475. doi: 10.1016/B978-0-12-800874-4.00021-0
Medina-Franco, J. L., López-Vallejo, F., Kuck, D., and Lyko, F. (2011). Natural products as DNA methyltransferase inhibitors: a computer-aided discovery approach. Mol. Divers. 15, 293–304. doi: 10.1007/s11030-010-9262-5
Medina-Franco, J. L., Méndez-Lucio, O., Yoo, J., and Dueñas, A. (2015). Discovery and development of DNA methyltransferase inhibitors using in silico approaches. Drug Discov. Today 20, 569–577. doi: 10.1016/j.drudis.2014.12.007
Miyao, T., Reker, D., Schneider, P., Funatsu, K., and Schneider, G. (2015). Chemography of natural product space. Planta Med. 81, 429–435. doi: 10.1055/s-0034-1396322
Naveja, J. J., and Medina-Franco, J. L. (2015). Activity landscape sweeping: insights into the mechanism of inhibition and optimization of DNMT1 inhibitors. RSC Adv. 5, 63882–63895. doi: 10.1039/C5RA12339A
Naveja, J. J., and Medina-Franco, J. L. (2018). Insights from pharmacological similarity of epigenetic targets in epipolypharmacology. Drug Discov. Today 23, 141–150. doi: 10.1016/j.drudis.2017.10.006
Naveja, J. J., Rico-Hidalgo, M. P., and Medina-Franco, J. L. (2018). Analysis of a large food chemical database: chemical space, diversity, and complexity. F1000Res. 7:993. doi: 10.12688/f1000research.15440.2
Ntie-Kang, F., Zofou, D., Babiaka, S. B., Meudom, R., Scharfe, M., Lifongo, L. L., et al. (2013). AfroDb: a select highly potent and diverse natural product library from African medicinal plants. PLoS One 8:e78085. doi: 10.1371/journal.pone.0078085
Olmedo, D. A., González-Medina, M., Gupta, M. P., and Medina-Franco, J. L. (2017). Cheminformatic characterization of natural products from panama. Mol. Divers. 21, 779–789. doi: 10.1007/s11030-017-9781-4
Ostler, K. R., Davis, E. M., Payne, S. L., Gosalia, B. B., Expósito-Céspedes, J., Beau, M. M. L., et al. (2007). Cancer cells express aberrant DNMT3B transcripts encoding truncated proteins. Oncogene 26, 5553–5563. doi: 10.1038/sj.onc.1210351
Pilon, A. C., Valli, M., Dametto, A. C., Pinto, M. E. F., Freire, R. T., Castro-Gamboa, I., et al. (2017). NuBBEDB: an updated database to uncover chemical and biological information from Brazilian biodiversity. Sci. Rep. 7:7215. doi: 10.1038/s41598-017-07451-x
Rodrigues, T., Reker, D., Schneider, P., and Schneider, G. (2016). Counting on natural products for drug design. Nat. Chem. 8, 531–541. doi: 10.1038/nchem.2479
Rosen, J., Lovgren, A., Kogej, T., Muresan, S., Gottfries, J., and Backlund, A. (2009). ChemGPS-NPWeb: chemical space navigation online. J. Comput. Aided Mol. Des. 23, 253–259. doi: 10.1007/s10822-008-9255-y
Sacconnay, L., Angleviel, M., Randazzo, G. M., Queiroz, M. M., Queiroz, E. F., Wolfender, J. L., et al. (2014). Computational studies on sirtuins from Trypanosoma cruzi: structures, conformations and interactions with phytochemicals. PLoS Negl. Trop. Dis. 8:e2689. doi: 10.1371/journal.pntd.0002689
Schneider, G., Neidhart, W., Giller, T., and Schmid, G. (1999). Scaffold-hopping by topological pharmacophore search: a contribution to virtual screening. Angew. Chem. Int. Ed. 38, 2894–2896. doi: 10.1002/(SICI)1521-3773(19991004)38:19<2894::AID-ANIE2894>3.0.CO;2-F
Shang, J., Hu, B., Wang, J., Zhu, F., Kang, Y., Li, D., et al. (2018). Cheminformatic insight into the differences between terrestrial and marine originated natural products. J. Chem. Inf. Model. 58, 1182–1193. doi: 10.1021/acs.jcim.8b00125
Tough, D. F., Tak, P. P., Tarakhovsky, A., and Prinjha, R. K. (2016). Epigenetic drug discovery: breaking through the immune barrier. Nat. Rev. Drug Discov. 15, 835–853. doi: 10.1038/nrd.2016.185
Vilkaitis, G., Merkiene, E., Serva, S., Weinhold, E., and Klimasauskas, S. (2001). The mechanism of DNA cytosine-5 methylation - kinetic and mutational dissection of Hhai methyltransferase. J. Biol. Chem. 276, 20924–20934. doi: 10.1074/jbc.M101429200
Villoutreix, B. O., Kuenemann, M. A., Poyet, J. L., et al. (2014). Drug-like protein–protein interaction modulators: challenges and opportunities for drug discovery and chemical biology. Mol. Inf. 33, 414–437. doi: 10.1002/minf.201400040
Wang, L., Wang, J., Sun, S., Rodriguez, M., Yue, P., Jang, S. J., et al. (2006). A novel DNMT3B subfamily, ΔDNMT3B, is the predominant form of DNMT3B in Non-small cell lung cancer. Int. J. Oncol. 29, 201–207. doi: 10.3892/ijo.29.1.201
Willett, P., Barnard, J., and Downs, G. (1998). Chemical similarity searching. J. Chem. Inf. Comput. Sci. 38, 983–996. doi: 10.1021/ci9800211
Yoo, J., Kim, J. H., Robertson, K. D., and Medina-Franco, J. L. (2012). Molecular modeling of inhibitors of human DNA methyltransferase with a crystal structure: discovery of a novel DNMT1 inhibitor. Adv. Protein Chem. Struct. Biol. 87, 219–247. doi: 10.1016/B978-0-12-398312-1.00008-1
Yoo, J., and Medina-Franco, J. L. (2011). Homology modeling, docking, and structure-based pharmacophore of inhibitors of DNA methyltransferase. J. Comp. Aided Mol. Des. 25, 555–567. doi: 10.1007/s10822-011-9441-1
Yoo, J., and Medina-Franco, J. L. (2012). Trimethylaurintricarboxylic acid inhibits human DNA methyltransferase 1: insights from enzymatic and molecular modeling studies. J. Mol. Model. 18, 1583–1589. doi: 10.1007/s00894-011-1191-4
Zhang, Z.-M., Liu, S., Lin, K., Luo, Y., Perry, J. J., Wang, Y., et al. (2015). Crystal structure of human DNA methyltransferase 1. J. Mol. Biol. 427, 2520–2531. doi: 10.1016/j.jmb.2015.06.001
Keywords: chemical space, chemoinformatics, databases, DNMT inhibitors, drug discovery, molecular modeling, similarity searching, virtual screening
Citation: Saldívar-González FI, Gómez-García A, Chávez-Ponce de León DE, Sánchez-Cruz N, Ruiz-Rios J, Pilón-Jiménez BA and Medina-Franco JL (2018) Inhibitors of DNA Methyltransferases From Natural Sources: A Computational Perspective. Front. Pharmacol. 9:1144. doi: 10.3389/fphar.2018.01144
Received: 08 August 2018; Accepted: 21 September 2018;
Published: 10 October 2018.
Edited by:
Shibashish Giri, University of Leipzig, GermanyReviewed by:
Jaigopal Sharma, Delhi Technological University, IndiaSujata Mohanty, All India Institute of Medical Sciences, India
Rup Lal, University of Delhi, India
Copyright © 2018 Saldívar-González, Gómez-García, Chávez-Ponce de León, Sánchez-Cruz, Ruiz-Rios, Pilón-Jiménez and Medina-Franco. This is an open-access article distributed under the terms of the Creative Commons Attribution License (CC BY). The use, distribution or reproduction in other forums is permitted, provided the original author(s) and the copyright owner(s) are credited and that the original publication in this journal is cited, in accordance with accepted academic practice. No use, distribution or reproduction is permitted which does not comply with these terms.
*Correspondence: José L. Medina-Franco, bWVkaW5hamxAdW5hbS5teA==; am9zZS5tZWRpbmEuZnJhbmNvQGdtYWlsLmNvbQ==