- 1School of Pharmacy and Pharmaceutical Science, Shandong First Medical University and Shandong Academy of Medical Sciences, Jinan, China
- 2School of Pharmaceutical Science, Shanxi Medical University, Taiyuan, China
- 3Taiyuan Maternity and Child Health Care Hospital, Taiyuan, China
- 4College of Pharmacy, Shandong University of Traditional Chinese Medicine, Jinan, China
Background: Gardenia Fructus (GF), a traditional Chinese medicine of Gardenia Ellis in Rubiaceae family, has the potential to clear heat and purge fire and has been widely used to treat multiple infection-related diseases. However, the quality markers (Q-Markers) of GF have not been revealed comprehensively.
Methods: In this experiment, the transgenic zebrafish lines, Tg (l-fabp:EGFP) and Tg (lyz:EGFP), were used to evaluate two main kinds of traditional efficacies of GF, hepatoprotective and anti-inflammatory effects. All the GF samples from different production areas were tested their anti-liver injury and anti-inflammantory activities. High-performance liquid chromatography-quadrupole time-of-flight mass spectrometry method (HPLC-Q-TOF/MS) was employed for herbal metabonomic analysis of GF samples. Gray correlation analysis (GCA) was utilized to screen out the components closely associated with the activities. Finally, the zebrafish model was applied to verify the bioactivity of the crucial components to determine the Q-Markers of GF.
Results: The zebrafish models were established by inducing with hydrogen peroxide or copper sulfate and applied to quickly evaluate the hepatoprotective effect and inflammation of GF samples. 27 potentially active components for liver protection and 21 potentially active components with anti-inflammatory properties were identified by herbal metabolomic analysis based on HPLC-Q-TOF/MS. The GCA result showed that five of the 27 components were highly correlated with liver protection, 15 of the 21 components were highly correlated with anti-inflammatory activity. Among them, geniposide and crocin-1 were confirmed their bioactivities on zebrafish experiment to be responsible for the protective effects of GF against liver injury, and genipin-1-β-D-gentiobioside, quinic acid, gardenoside, d-glucuronic acid, l-malic acid, mannitol, rutin, and chlorogenic acid were confirmed to be responsible for the anti-inflammatory effects. Finally, according to the screening principles of Q-Markers, genipin-1-β-D-gentiobioside, geniposide, and gardenoside were preliminarily identified to be the Q-Markers of GF.
Conclusion: This study established an effective research strategy of “Omics Discrimination-Grey Correlation-Biological Verification,” which enabled the rapid identification of key pharmacological components of GF. These markers have provided a scientific basis for constructing a modern quality evaluation system for GF.
Introduction
Gardenia Fructus, the dried fruit of Gardenia jasminoides Ellis in Rubiaceae family, has been recorded in the Shennong Bencaojing treatize of the Han Dynasty (BC 202–AD 220) in China. GF has the potential to clear Heat and purge Fire (Chen et al., 2020). Furthermore, GF plays a significant clinical role in the prevention and treatment of chronic diseases such as liver and gallbladder diseases (Fan et al., 2020) and metabolic diseases (L. Wang et al., 2020). Besides, GF has been listed in medicine food homology in China, and is also a common traditional medicinal food for the health industry. However, the existing quality standard system of traditional Chinese medicine is far from the requirements of the actual market circulation and authentic variety evaluation (X.Wu et al., 2018; C. Liu, Guo. DA and L. Liu et al., 2018). Hence, studying the quality evaluation chemical makers is crucial for ascertaining the intrinsic quality of GF.
Quality markers (Q-Markers) is a new concept of scientific quality evaluation of TCM proposed by the Chinese academician Changxiao Liu in 2016. Q-markers is a chemical substance inherent or formed during processing and preparation of Chinese medicine materials and TCM products (such as Chinese herbal pieces, Chinese herbs decoction, extraction of TCM, Chinese patent medicine preparation). It is closely related to the functional properties of TCM, and is used as a marker substance reflecting the safety and effectiveness of TCM for quality control (C. X. Liu et al., 2016), and also forms the basis of quality evaluation (Ren et al., 2020). In this research, we hope to explore the Q-Markers of GF based on this theory, which can lay the foundation for the establishment of scientific quality control methods for GF.
For the quality evaluation of TCM, the use of Q-Markers is an internationally accepted method to reflect the efficacy and quality by assessing a limited number of chemical components. However, it is not easy to confirm these key chemical components (L. Xu et al., 2018). The routine research methods are usually hard to track the unknown active chemical components, or vast samples produced from separation and separation process (X. Liu et al., 2021). Therefore, more and more researchers are attempting to explore and develop efficient identification methods for key medicinal components. Metabolomics is an important branch of systematic biology, which has high resolution and extremely low detection limit. This approach can simultaneously detect integral components in TCM (Jiang et al., 2016). However, due to the large number of chromatographic peaks and small sample size, it is not sufficient to perform most of the general pharmacological tests. Therefore, we endeavored to propose a more effective method to improve the accuracy of chemical components extracted from the complex chemical system of TCM.
At present, models of liver protection and inflammation based on rodents (rats and mice) are commonly used for evaluating the efficacy of TCM (Chiang et al., 2020; C. Y.; Li et al., 2016; W. K.; Li et al., 2019). However, a large amount of experimental samples volume is required for in vivo models, which is not easy to be achieved for sample extracts of TCM. Moreover, it is difficult to realize the evaluation of the monomer components in the later stage. Zebrafish (Danio rerio), a tropical freshwater fish of the Cyprinidae family, is a vertebrate model and is often used to study human diseases (Lieschke and Currie, 2007). When compared with mice and other commonly used in vivo animal models, zebrafish has the advantages of small size, large number of offspring, high transparency, easy breeding, low feeding cost, and the ease of carrying out large-scale research (Lieschke and Currie, 2007). The function of liver cells and histopathological changes in zebrafish are very similar to humans. The molecular mechanism of the development is also consistent with that of mammals (Goessling and Sadler, 2015). Zebrafish is also widely used to study the immune response to the acute phase of infection, the interaction between pathogens and the host, and the real-time migration of injured immune cells (Torraca and Mostowy, 2018). Therefore, zebrafish was chosen as an experimental model in this study. Although the limited activities could hardly represent the efficacy of GF, we believe that these two activities reflect main parts of the efficacy of GF. What’s more, the zebrafish experiment supplied considerable convenience to verify the activities of the selected monomer components in vivo and validate the screening results (Y. P. Shi et al., 2020).
Finally, we proposed a new integrated research strategy, namely the “Omics Discrimination-Grey Correlation-Biological Verification” by using herbal metabolomics, zebrafish model, and other technologies. Exploration of the pharmacodynamic markers closely related to the efficacy of GF provides an important basis for constructing a quality evaluation system with Q-Markers as the core.
Materials and Methods
Chemicals, Drugs, and Reagents
Hydrogen peroxide (30%, CAS:7722-84-1, Lot#20160908), ethanol (analytical grade 64-17-5), and formic acid (analytical grade, CAS:64-18-6) were acquired from Sinopharm Chemical Reagent Co., Ltd. (Shanghai, China); Acetonitrile (HPLC grade, CAS:75-05-8) was purchased from Oceanpak (Goteborg, Sweden). Tricaine (CAS:886-86-2) were purchased from Sigma-Aldrich Co.; dimethyl sulfoxide (DMSO, CAS:67-68-5, Lot#39075) was purchased from Sangon Biotech Co.; Ibuprofen (CAS:15687-27-1, Lot#VM9V-S7BU); cupric sulfate (CAS:7758-99-8); Ultrapure water was supplied by Watsons Ltd. (Hong Kong, China); Genipin-1-β-D-gentiobioside (CAS:29307-60-6, Lot#P20N8F48431), geniposide (CAS:24512-63-8, Lot#C1722062), crocin-1 (CAS:42553-65-1, Lot# P01N8F45675), quinic acid (CAS:77-95-2, Lot#K29D9B77039), gardenoside (CAS:54835-76-6, Lot#P160A10F95425), d-glucuronic acid (CAS:556-12-3, Lot# K14M10S82777), l-Malic acid (CAS:97-67-6, Lot#S24O10I101046), mannitol (CAS:87-78-5, Lot#SM0513GB13), rutin (CAS:153-18-4, Lot#T10J10Z90356), chlorogenic acid (CAS:327-97-9, Lot#Y22M8K36544), Shanzhiside (CAS:29836-27-9, Lot#P30J9F64637), d-glucaric acid (CAS:87-73-0, Lot#P23B15G75287) were provided by Shanghai Yuanye Bio-Technology Co., Ltd. The purity of all the chemicals were greater than 98%.
The sources and the time of collection of dried ripe fruits of GF were indicated (Table 1). All GF samples were identified as the dried fruits of Gardenia jasminoides Ellis belonging to the Rubiaceae family by Dr Liwen Han, Associate Professor, Shandong First Medical University.
Zebrafish Maintenance and Embryo Collection
The transgenic line zebrafish, Tg (l-fabp:EGFP) and Tg (lyz:EGFP), were provided by Shandong Province Engineering Research Center of Zebrafish Models for Human Diseases and Drug Screening (Biology Institute of Shandong Academy of Sciences). The animals were cultivated in a professional breeding system (Shanghai Haisheng Biotech Co., Ltd.) and maintained under a 14 h/10 h light/dark cycle at a constant temperature of 28 ± 0.5°C. The zebrafish were fed with fairy shrimp twice a day at 9:00 and 16:00 h. Adult male and female zebrafish were placed in a ratio of 2:2 on both sides of a partition board in a mating tank at night; the divider was removed at 8:00 h the next day. The zebrafish was stimulated by light to mate and lay eggs naturally. The embryos were collected within 1 h of spawning and rinsed thrice with fresh water. The clean embryos were transferred to Petri dishes containing fish water (5 mM NaCl, 0.17 mM KCl, 0.4 mM CaCl2, and 0.16 mM MgSO4) and incubated at 28.5°C for subsequent experiments (Avdesh. A et al., 2012). The animal study was reviewed and approved by Ethics Committee of Shandong First Medical University, Shandong First Medical University & Shandong Academy of Medical Sciences.
Preparation of Sample Solutions
All GF samples were ground to a powder form and sifted through a 60-mesh screen. Then, the powder was extracted twice using 70% ethanol with a material-to-liquid ratio of 1:8 for 1 h. The supernatant of each sample was collected after centrifugation at 5,000 r/min for 10 min. The extracted GF samples were obtained by rotary evaporation and drying at 60°C. Anesthetic (tricaine) solution of 1 g L−1 concentration was prepared with distilled water; 30% hydrogen peroxide was diluted 60 times with ultrapure water to make a solution of 5 ml L−1 concentration; CuSO4.5H2O was made into a mother liquor of 5 g L−1 concentration with distilled water; Ibuprofen was dissolved in dimethyl sulfoxide (DMSO) to prepare a solution of 4 g L−1 concentration; gardenoside, genipin-1-β-D-gentiobioside, crocin-1, quinic acid, d-glucuronic acid, l-malic acid, mannitol, rutin, chlorogenic acid, shanzhiside, and d-glucaric acid were each prepared into stock solutions of 100 g L−1 concentration with DMSO. Each GF sample (for HPLC-Q-TOF/MS analysis) was made a solution of 1 g L−1 concentration with acetonitrile water (5:95, v/v). Gardenoside, genipin-1-β-D-gentiobioside, crocin-1, quinic acid, d-glucuronic acid, l-malic acid, mannitol, rutin, and chlorogenic acid were accurately weighted and dissolved in 25 ml of acetonitrile water (5:95, v/v) through a microporous filter membrane to prepare sample solutions of the required concentration for the experiments one by one.
Liver Protection and Anti-Inflammatory Experiments in Zebrafish
Experimental Design
Zebrafish larvae that were 3 days post-fertilization (dpf) were taken in a Petri dish. Larvae of normal and consistent development were selected and placed in a 6-well microplate, with 20 larvae per well. The animals was divided into liver protection group and anti-inflammatory group. All groups were pre-protected for 6 h. The control group (Con) was given only 0.5% DMSO. The indicated concentration gradient of the drug group was set as shown in the results section. In the liver protection experiment, all groups (excluding the control group) were treated with H2O2 at a concentration of 25 μL L−1 for 2 days to induce liver injury (Ma. J et al., 2020). The inflammation model of zebrafish was established by using CuSO4 at a concentration of 4 mg L−1 for 1 h (Gui et al., 2020). The positive group was treated with ibuprofen at a concentration of 4 mg L−1.
Image Capture and Statistical Analysis
Ten zebrafish larvae were randomly selected from each group for photographic observation. In the liver protection experiment, the fluorescence of liver was observed using a fluorescence microscopy. The images were collected and recorded for each zebrafish larva. Fluorescence intensity of the liver (% of control) was measured using Image Pro Plus 5.1 (Media Cybernetios Inc., Rockville, MD, United States). In the anti-inflammatory experiment, abnormalities and the distribution of the neutrophils were observed using a fluorescence microscope. The neutrophils migrating to the lateral neuromast and above were statistically analyzed by GraphPad Prism 6.01 (Graphpad Software Inc., La Jolla, CA, United States). One-way analysis of variance way was used to determine the statistical significance of the differences among the groups, and the experimental data were expressed as mean ± standard error (SE). The difference was considered to be significant when p was <0.05, and the difference was considered to be extremely significant when p was <0.01 (##p < 0.01 is vs. the control group; *p < 0.05 and **p < 0.01 are vs. model group).
Herbal Metabolomic Analysis
HPLC-Q-TOF/MS Analysis of GF
HPLC-Q-TOF/MS consists of a liquid chromatography system (Agilent 1,200 Infinity Series) with a binary pump, degassing device, automatic sampler, and ultraviolet detector as well as a Q-TOF mass spectrometer (Agilent 6,520 series) to examine the GF samples. An Eclipse XDB-C18 column (4.6 × 250 mm; 5 μm; Agilent) was used as the chromatographic column. The chromatographic conditions were as follows: the mobile phase was composed of formic acid–water (0.1:100, v/v) (A) and acetonitrile (B). The gradient elution program was 7–20% B for 0–10 min, 20–30% B for 10–13 min, 30% B for 13–17 min, 30%–7% B for 17–18 min, and 7% B for 18–25 min at a flow rate of 1 ml/min. The sample injection volume was 20 μL. The temperature of the column was set at 25°C. The detection wave was set at 238 nm. The MS conditions were as follows: Electrospray ionization was in the negative ion mode, split ratio was 2:1, scan range m/z was 100–1,100 Da, nebulizer pressure was two psig, gas temperature was 350°C, drying gas flow rate was 12 L/min, and capillary voltage was 3500 V. The raw data of all GF samples were analyzed with Agilent MassHunter Workstation and R software (R Development Core Team, 2011).
Data Analysis
Principal component analysis (PCA) was used to describe the natural distribution and aggregation states of the samples (Nie et al., 2020). Orthogonal partial least squares-discriminant analysis (OPLS-DA) was employed to distinguish the different kinds of compounds from each other (Chung et al., 2019; B. M.; Huang et al., 2018). S-plots provided visible OPLS-DA for model explanation (Feng et al., 2018); A permutations test (PT) evaluated the credibility of the OPLS-DA model (Bocca et al., 2021) using the software SIMCA-P14.1.
Gray Correlation Analysis
Gray correlation analysis (GCA) was used to examine the correlation between two groups of variations (P. Li et al., 2020). In this study, the signal intensities of the different components present in the 9 GF samples (S1-S9) obtained from different producing areas were measured. GCA was applied to investigate the spectral-effect relationships between signal intensity and biological activities related to liver injury and inflammation in the zebrafish.
Specifically,
ρ is the resolution coefficient ranging from 0 to 1, usually 0.5.
The fluorescence intensity of the liver and the number of neutrophils spreading to the lateral neuromast of the zebrafish in the 9 GF samples were set as the sequence of system behavior characteristics. The signal intensities of different component variations of the 9 GF samples were set as the sequence of system associated factors. Owing to the different dimensions of the two datasets, dimensionless processing was performed on the original data to eliminate the adverse effects of unit inconsistencies. Especially, for dimensionless processing, each data point was divided by the average of the corresponding sequence to calibrate and calculate the correlation coefficients (M. Liu et al., 2020).
Result
Biological Activities of the GF Samples on the Zebrafish
The zebrafish model of liver injury was produced using H2O2 at a concentration of 25 mgL−1 for 2 days. As shown in Figure 1A, the fluorescence intensity decreased in the liver of zebrafish in the H2O2 model group when compared with those in the control group. However, the fluorescence intensity in the zebrafish liver was significantly improved in the GF groups of S1, S3, S6, S8, and S9 (the dose received by each group was 500 mg L−1) compared to the H2O2 model group (Figure 1B). The results signify that the liver injury was relieved in the above GF groups. However, the 4 GF groups of S2, S4, S5, and S7 did not differ from the model group in a statistically significant manner. The two groups with the largest difference were S7 (Jiujiang, Jiangxi) and S8 (Fuding, Fujian), with liver fluorescence intensities of 62.0 and 97.0%, respectively.
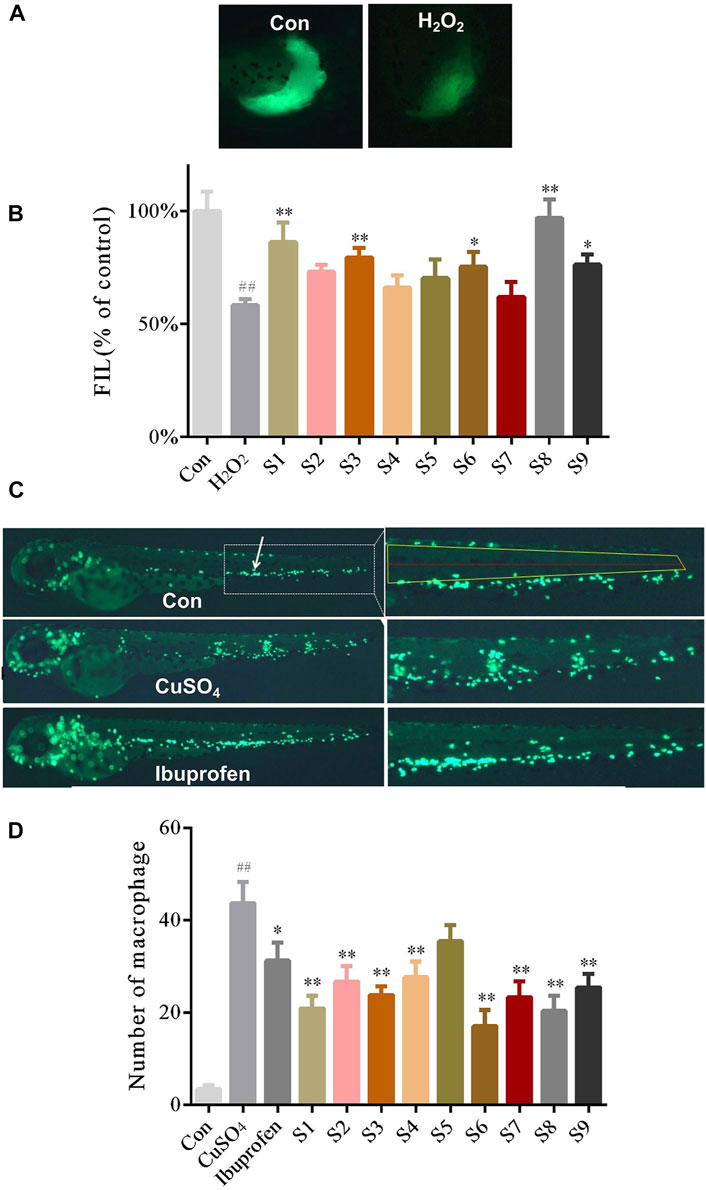
FIGURE 1. The protective effect of nine samples of GF (S1-S9) collected from different areas on liver injury and inflammation (A) Phenotypic micrograph of the transgenic zebrafish with fluorescence label in the liver (B) Statistical results of the fluorescence intensity of the liver in zebrafish until five dpf in all groups. n = 10 (C) Distribution phenotypic micrograph of neutrophils (white arrows) in zebrafish and enlarged drawing of neutrophils spreading to the lateral neuromast of the tail (D) Statistical results of the number of neutrophils diffusion in zebrafish in all groups, n = 10.
Zebrafish models of inflammation were established with CuSO4 at a concentration of 4 mgL−1 for 1 h. As shown in Figure 1C, the right panel shows the magnification of the white-dashed box, whereas the yellow box represents the neutrophils diffusion region and the red line represents the lateral neuromast, at 32× magnification. the fluorescent spots near the lateral neuromast of the zebrafish tail were higher in the CuSO4 model group when compared with the control group. The number of fluorescent spots near the lateral neuromast of the zebrafish tail were significantly lowered after treatment with ibuprofen. The above results suggest that the inflammatory model of the zebrafish can be used to study the anti-inflammatory efficacy of GF. Neutrophils spreading close to the lateral neuromast of the zebrafish tail were remarkably inhibited in the GF groups of S1,S2, S3, S4,S6, S7, S8, and S9 (the dose received by each group was 500 mg L−1) when compared with the CuSO4 model group (Figure 1D). The average number of fluorescent spots near the lateral neuromast in the zebrafish tail in the S4 (Panan, Zhejiang) and S6 (Jichun, Hubei) groups were 27 and 17, respectively, and they exhibited the most significant statistical difference among all the GF treatment groups.
Comparison of Activity in GF Samples Collected From Specific Locations
The protective effects of the GF samples S7 and S8 on liver injury were compared and verified at different concentrations (Figure 2B). The S4 and S6 GF samples were also evaluated using the zebrafish inflammatory model to identify the differences in their anti-inflammatory efficacies (Figures 3A,B).
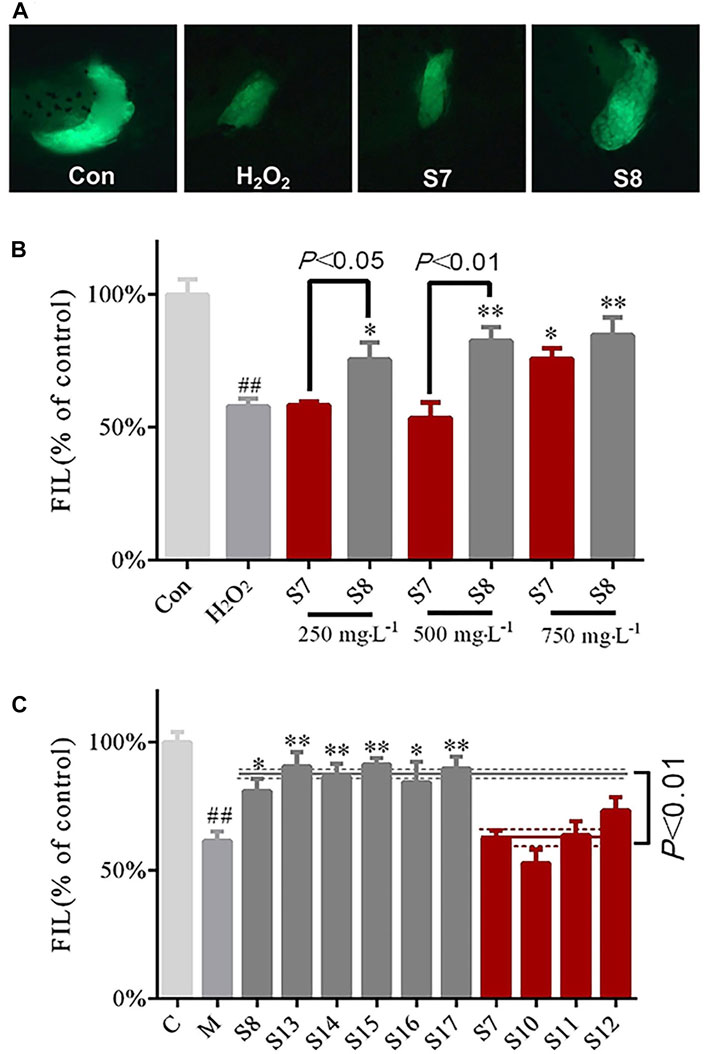
FIGURE 2. Comparison of the protective effects of GF (S7 and S8) collected from specific locations on liver injury (A) Phenotypic micrograph of the transgenic zebrafish with fluorescence label in the liver (B) Comparative analysis of protective effects of S7 and S8 with different doses on liver injury (C) Comparison of multiple batches of protective effects of GF from two areas, Fuding and Jiujiang, on the liver. n = 10.
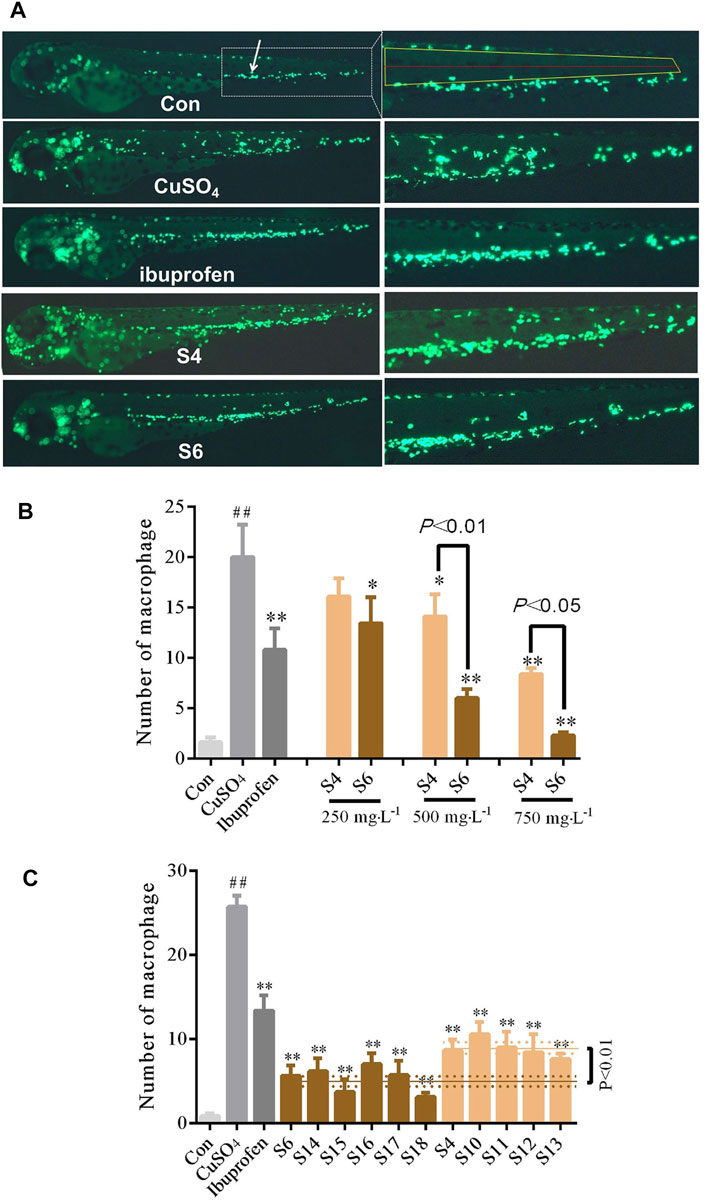
FIGURE 3. Comparison of the anti-inflammatory activities of GF (S4 and S6) collected from specific locations (A) Distribution phenotypic micrograph of neutrophils in zebrafish and enlarged drawing of neutrophils spreading to the lateral neuromast of the tail. at ×32 magnification (B) Comparative analysis of the effects of inflammation inhibition in zebrafish treated with S4 and S6 of different concentrations (C) Multiple batches’ comparison of anti-inflammatory effects of GF from two locations: Panan and Jichun. n = 10.
The results showed that S7 GF differed statistically from the H2O2 model group at a concentration of 750 mgL−1; S8 GF differed statistically significantly from the H2O2 model group at concentrations of 250, 500 and 750 mgL−1. There were significant differences (p < 0.05) between S7 and S8 samples at concentrations of 250 and 500 mgL−1 (Figure 2B). When compared with the H2O2 model group, the six batches of GF from Fuding (S8, S13–S17) were statistically different, which indicates their protective efficacy against liver injury. The six batches of GF groups from Fuding and the four batches of GF groups from Jiujiang (S7, S10–S12) had significant differences (p < 0.01) (Figure 2C). These data further demonstrate that differences in the location of GF may be the main factor for the differences in the protective effects against liver injury.
The results demonstrated that S4 GF differed statistically significantly at concentrations of 500 and 750 mgL−1 from the CuSO4 model group; S6 GF was statistically significantly different at concentrations of 250, 500, and 750 mgL−1. There were statistical differences between S4 and S6 samples at concentrations of 500 and 750 mgL−1 (Figure 3B). When compared with the CuSO4 group, the five batches of GF from Panan (S4, S18-S21) and the six batches of GF from Jichun (S6, S22–S26) significantly inhibited the inflammation. There were statistically significant differences (p < 0.01) in samples obtained from these two locations (Figure 3C). This finding suggests that there are great variations in the biological activities of the GF samples sourced from different regions regardless of the time of collection.
Discovery of the Bioactive Compounds in GF by Herbal Metabolomic Analysis
Ten batches of GF collected from Fuding and Jiujiang and 11 batches of GF collected from Panan and Jichun were analyzed using HPLC-Q-TOF/MS to obtain the original MS data. After being processed by MassHunter, the data were imported into R software to extract information on ion fragments. Finally, 1,056 component ion fragments were acquired from GF of Fuding and Jiujiang in the negative ion mode for further multivariate statistical analysis. In the same way, 926 component ion fragments were gained from GF of Panan and Jichun.
In the PCA scatter plot of 10 GF samples from Fuding and Jiujiang in liver protection experiment (Figure 4A), the confidence interval was 95%, significance level was 0.05, and the first and second principal components were 53.1 and 15.3%, respectively. The results signified that GF in the two groups were clearly separated by the coordinate axis t [1]; PCA analysis was performed on the 926 common ion fragments obtained from the 11 GF batches from Panan and Jichun in the above-mentioned anti-inflammatory experiments. PCA plots (Figure 4E) were constructed directly using the first principal component (34.2%) and the second principal component 2 (23%), which showed that the GF samples from Panan and Jichun were clearly separated. The findings indicated that the chemical compositions of the two were significantly different.
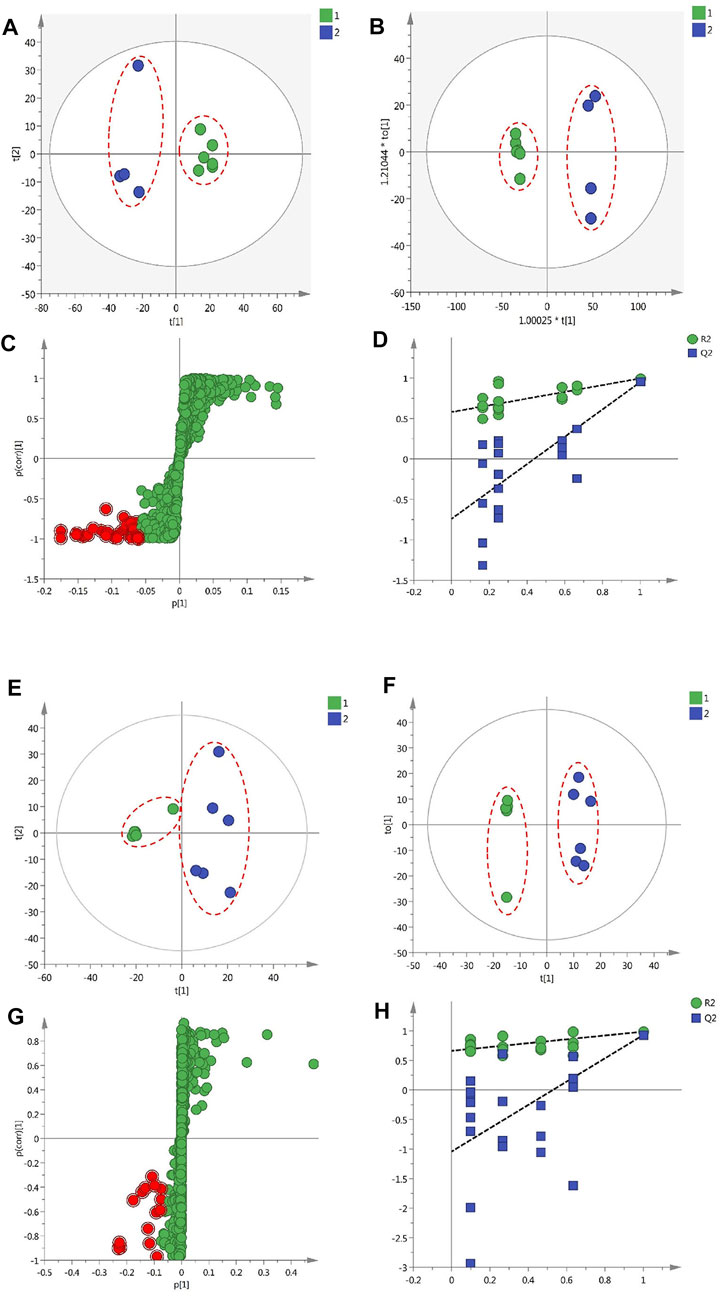
FIGURE 4. The results of multivariate statistical analysis of metabolomic data of protective effects of GF on liver injury and inflammation (A) A PCA scatter plot of 10 GF samples (B) The OPLA-DA scatter plot of the 10 samples (C) The S-plots for the OPLA-DA in the experiments of liver protection (D) PT test of the OPLA-DA model in experiments of liver protection (E) The PCA scatter plot of 11 GF samples (F) The OPLA-DA scatter plot of 11 samples (G) S-plots for the OPLA-DA in anti-inflammatory experiments (H) PT test of the OPLA-DA model in anti-inflammatory experiments.
Intra-group differences cannot be ignored and random errors cannot be eliminated by PCA, which is an unsupervized analysis method. The technique ignores the changing regulations and overall characteristics of the data, which is not conducive for the discovery of inter-group differences and metabolite differences (Hayashi et al., 2019). Therefore, this study used the supervised OPLS-DA model (Han et al., 2018) to further analyze the 1,056 and 926 common ion fragments obtained from 10 batches and 11 batches of GF, respectively. The OPLS-DA model can maximize the differences between the two groups of samples to determine the differences in the components between the two groups (F. Wang et al., 2019). From Figures 4B,F, it can be seen that the two groups of GF samples in the OPLS-DA model were clearly distinguished from each other, and the values of R2Y (cum) and Q2 (cum) for the models were 0.996 and 0.951 and 0.889 and 0.929, respectively. These results indicate that the OPLA-DA model has good applicability and predictability. The results of PT were calculated to be [R2= (0.0, 0.85), Q2 = (0.0, −0.47)] and [R2= (0.0, 0.98), Q2 = (0.0, −1.69)]. In addition, all the Q2 values on the left were less than the highest points on the right, and the intersection point of the blue regression line and ordinate of the Q2 value was less than zero (X. Zhang et al., 2019) (Figures 4D,H). This finding proves that the OPLS-DA model has good reliability. VIP (variables important in the projection) value >2, p < 0.05, and absolute value of the correlation coefficient |pcorr| > 0.58 were used to screen and determine the potentially important fragment ions. The results were represented using S-plots of the OPLA-DA model in the statistical analysis (Figures 4C,G). The red dots represent the filtered compounds. The protective effects of a total of 27 potentially important fragment ions against liver injury were acquired by filtering, and 21 fragment ions of potential anti-inflammatory activity components were identified (Table 2).
Results of GCA
GCA was used to analyze the spectral and effect relationships among the 27 fragment ions obtained from previous metabonomic analysis. The protective effects of GF sourced from the nine producing areas against liver injury and the 21 fragment ions and anti-inflammatory efficacies of GF were also examined. The gray relational degrees were calculated and sorted order respectively to verify the potential active ingredient (degree >0.7) (Table 3).
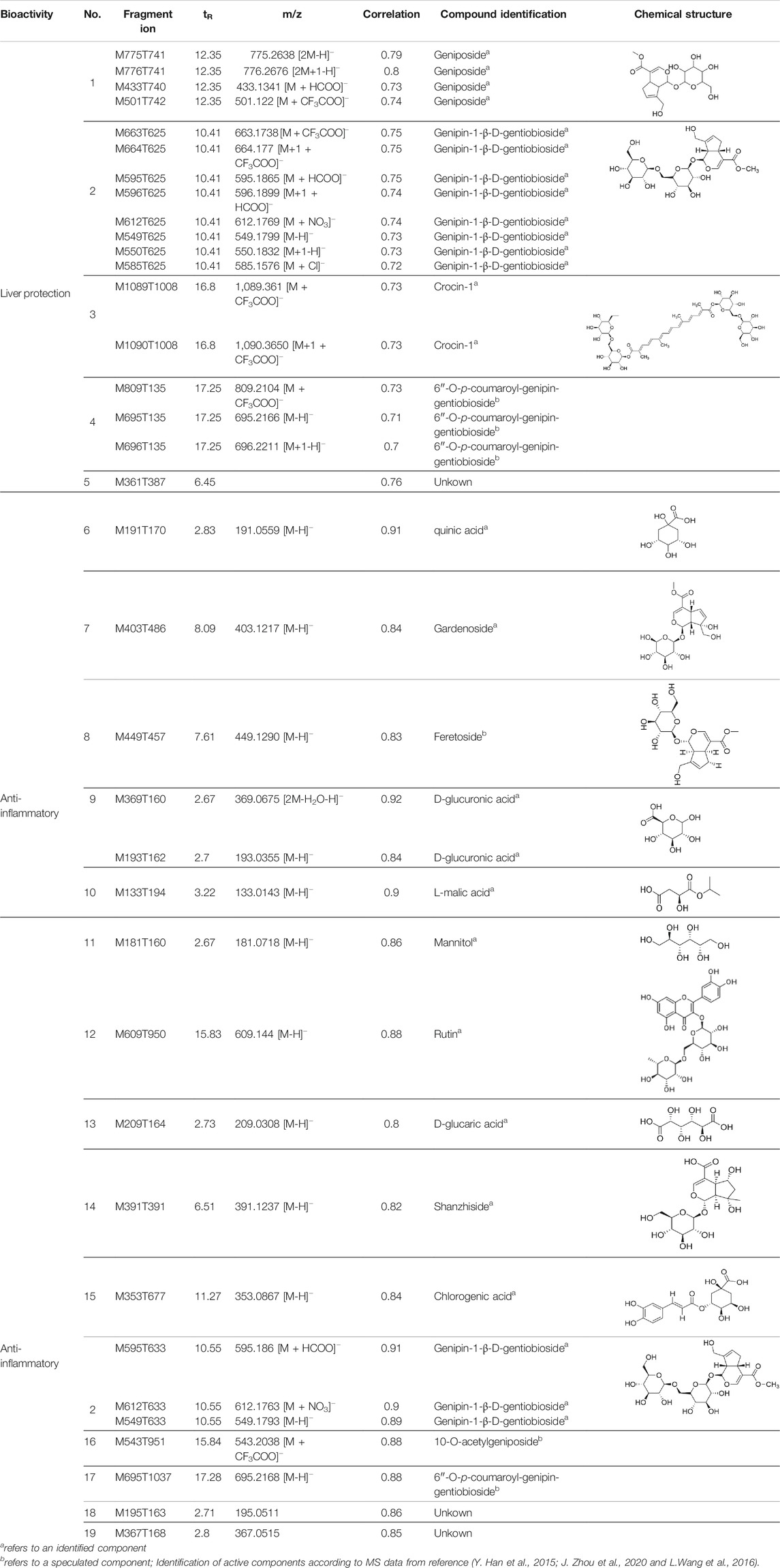
TABLE 3. Components of liver protection and anti-inflammatory effects of GF with gray relational degree>0.75 and component information.
Identification of Active Components
Chemical information related to the GF components is provided in Table 3. The mass numbers of four components related to liver protection and 15 components related to anti-inflammatory effect were analyzed qualitatively. After quality measured by MS compared with the MS database of GF chemical composition, possible compounds were identified. Based on the retention time and m/z data from the total ion chromatograms (TICs) of GF compounds, geniposide (1), genipin-1-β-D-gentiobioside (2), and crocin-1 3) were identified as the pharmacodynamic compounds offering liver protection. In addition, component 4 was presumed to be 6″-O-p-coumaroyl-genipin-gentiobioside, and component 5 was not recognized (Figure 5A); The anti-inflammatory components were finally identified as quinic acid (6), gardenoside (7), d-glucuronic acid (9), l-malic acid (10), mannitol (11), Rutin (12), d-glucaric acid (13), shanzhiside (14), chlorogenic acid (15), and genipin-1-β-D-gentioside (2); Furthermore, due to the large error of component 3, it could only be inferred as feretoside. Components 16 and 17 were presumed to be 10-O-acetylgeniposide and 6″-O-p-coumaroyl-genipin-gentiobioside, and components 18 and 19 were not confirmed (Figure 5B).
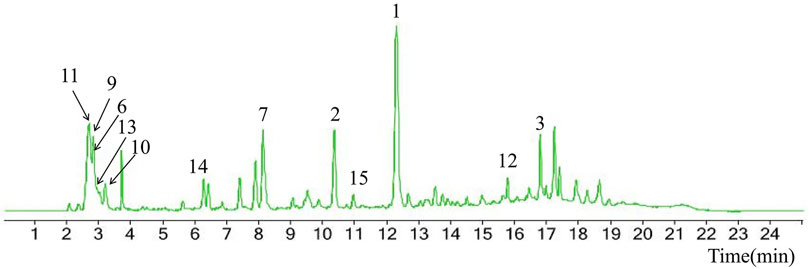
FIGURE 5. TICs of GF compounds at a negative ion mode. The total ion chrtograms (TICs) of GF extract, 1, 2, three on behalf of geniposide, genipin-1-β-D-gentiobioside, crocin-1, respectively; 6,7 and 9–15 represent quinic acid, gardenoside, d-glucuronic acid, l-malic acid, mannitol, rutin, d-glucaric acid, shanzhiside, chlorogenic acid, and genipin-1-β-D-gentioside, respectively.
Confirmation of the Identified Bioactive Compounds
The zebrafish model of liver injury was applied to verify whether the above three identified components (geniposide, genipin-1-β-D-gentiobioside and crocin-1) can be used as chemical markers for the protective effects of GF on the liver. The results suggested that the group administrated with genipin-1-β-D-gentioside did not statistically differ from the H2O2 model group; hence, the compound did not exhibit a protective effect. The groups administrated with geniposide at concentrations of 400 mgL−1 and crocin-1 at concentrations of 50 and 100 mgL−1 were statistically different from the H2O2 model group, which proved that the two components offered liver protection (Figure 6A). The zebrafish model of inflammation was applied to verify whether the 10 identified chemical components can be used as anti-inflammatory markers. The results showed that when compared with the model group, shanzhiside and d-glucaric acid did not inhibit inflammation. Thus, the two components cannot be used as anti-inflammatory markers. On the other hand, quinic acid and l-malic acid both differed statistically significantly at concentrations of 25, 50, and 100 mgL−1 from the CuSO4 model group; Rutin was statistically significantly different at concentrations of 125, 250, and 500 mgL−1; There were significant differences (p < 0.01) between d-glucuronic acid and the CuSO4 model group at concentrations of 50 and 100 mgL−1; Gardenoside, mannitol, chlorogenic acid and genipin-1-β-D-gentiobioside at concentrations of 250 and 500 mgL−1 inhibited inflammation to varying degrees (Figure 6B).
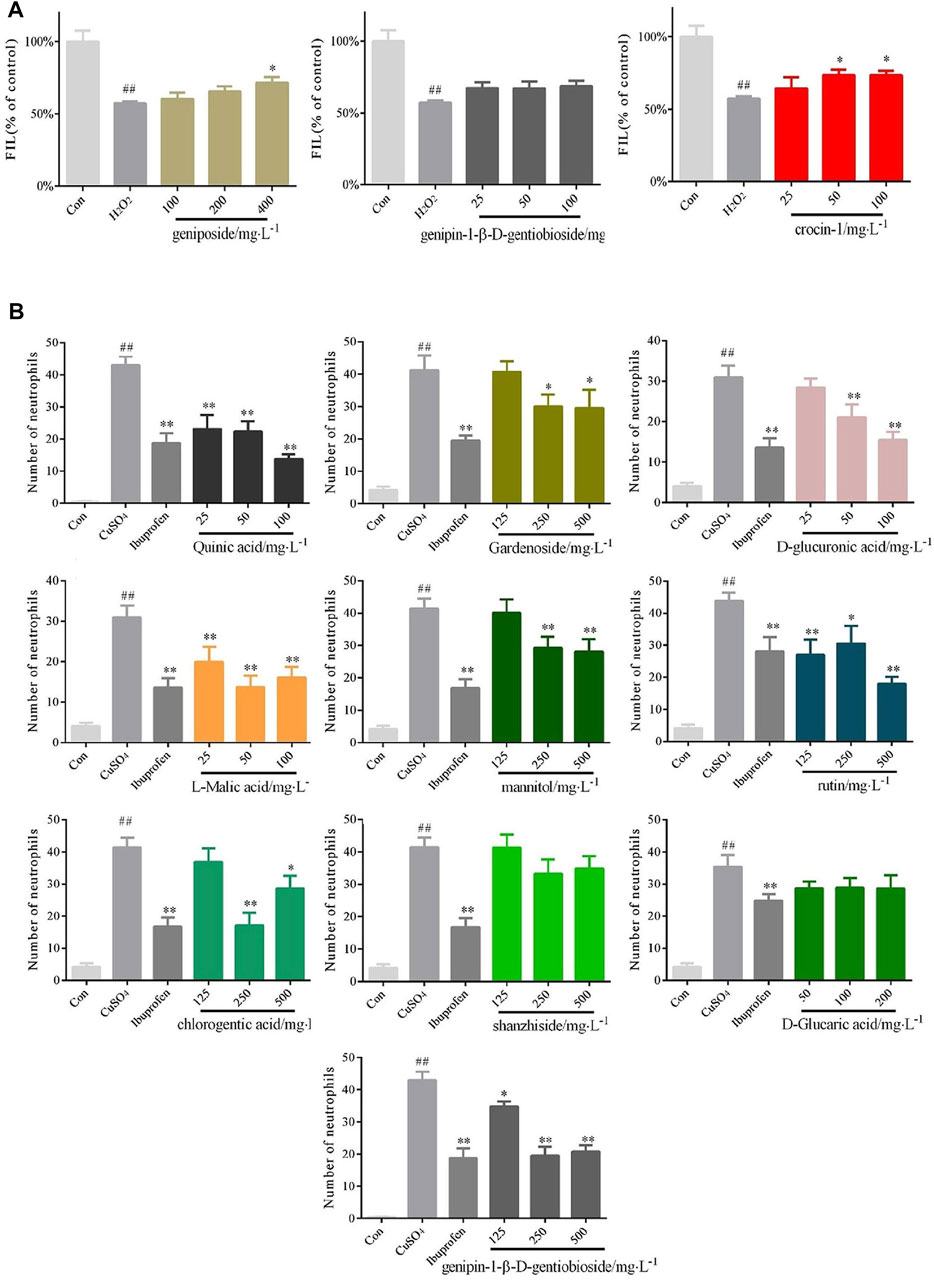
FIGURE 6. Confirmation of the protective effects of the identified bioactive compounds of GF on liver injury and inflammation (A) Quantified statistical results of protective effects of geniposide, genipin-1- β-D-gentiobioside, and crocin-1 with different doses on liver injury. n = 10 (B) Quantified statistical results of anti-inflammatory effects of quinic acid, gardenoside, d-glucuronic acid, l-malic acid, mannitol, rutin, chlorogenic acid, shanzhiside, d-glucaric acid, and genipin-1-β-D-gentioside with different doses. n = 10.
Discussion
According to ancient Chinese medical book’s record, the traditional efficacy of GF can be classified into two main categories: one is clearing Heat and the other is purging Fire. According to modern pharmacology, the effect of “purging Fire” is closely related to liver and gallbladder systems. At present, GF is mainly used to treat two types of diseases: 1. dampness–heat jaundice (J. Y. Li et al., 2017) such as epidemic or iteric hepatitis, jaundice, fever, and short red urine caused by stagnation of hepatochlic hygropyrexia; 2. eye redness, swelling, and pain (Chen et al., 2020), caused by hepatobiliary fire attack and other eye discomforts. These two kinds of diseases are related to liver injury. Modern research has confirmed that decoction and alcohol extract of GF have choleretic effect, promote bile secretion, and reduce the bilirubin in the blood to promote its rapid excretion (Tian et al., 2017). Seo (Seo et al., 2017) found that genipin, the main component of GF, ameliorates galactosamine/lipopolysaccharide (LPS)-induced hepatocellular damage by suppressing the signal transduction of necroptosis-mediated inflammasome to exert hepatoprotective effects. Therefore, we considered that the protective effect of GF against liver injury in the zebrafish model can be applied as one of the evaluation indexes of the efficacy of GF. On the other hand, the effectof “clearing Heat” of GF is closely related to its anti-inflammatory properties (Q. Wang et al., 2018). GF is mainly used to treat inflammation such as heat toxin sores and eye redness, swelling, and pain. Besides GF suppresses Streptococcus hemolyticus, dermatophytes, and viral replication (Kim et al., 2020). Inflammatory response caused by bacterial and fungal infections is the chief manifestation of infectious diseases. By exploring the molecular mechanism of geniposide in the mouse macrophages inflammation model caused by LPS, Shi et al. (Q. Shi et al., 2014) determined that geniposide could inhibit the release of inflammatory factors such as tumor necrosis factor-alpha (TNF-α) and interleukin-6 (IL-6). Besides, geniposide can alter the level of mRNA and the key enzymes cyclooxygenase-2 and inducible isoform of nitric oxide synthase in the inflammatory response. Therefore, it was believed that clearing heat and detoxifying, the main clinical effects of GF, had a relatively close relationship with anti-inflammatory properties. Hence, the anti-inflammatory model of zebrafish was selected to characterize the heat-clearing effect of GF.
The genome of zebrafish has been proved a high homology with that of humans (MacRae and Peterson, 2015). In this work, the zebrafish model of liver injury induced H2O2 was applied to evaluate the protective effects of GF on the liver. As a strong oxidant, H2O2 can cause apoptosis outside the cell and promote the production of hydroxyl free radicals, thereby leading to cell death induced by the lipid peroxidation of the cell membrane (B. Huang et al., 2018). In this study, zebrafish was treated with 25 μL L−1 hydrogen peroxide for 2 days. There was a significant difference between the H2O2 group and the control group, which proved that the liver injury model of zebrafish was successfully established and could be used for the discovery of hepatoprotective pharmacodynamic markers of GF. The defense system of zebrafish is highly conservative and has a complete response system similar to that of humans, including the migration and phagocytosis of the inflammatory cells (Robertson et al., 2016). Inflammation caused by CuSO4 in zebrafish belongs to the category of drug-induced reaction among the various inflammatory factors. Zhang et al. (Y. Zhang et al., 2020). utilized the inflammation model of zebrafish induced by CuSO4 to study the anti-inflammatory mechanism of isoniazid. Transgenic zebrafish with fluorescence-labeled neutrophils used in this study demonstrated the phenotypic process of inflammation. Several neutrophils spread close to the lateral neuromast in the zebrafish after treatment with CuSO4. After zebrafish larvae was given 4 mg L−1 copper sulfate for 1 h, there was a significant difference between the CuSO4 group and the control group, which proved that the zebrafish inflammation model was successfully established and could be used for the discovery of anti-inflammatory pharmacodynamic markers of GF.
In this work, representative samples of GF from different parts of China were collected to study the relationship between the composition of GF and its bioactivity. We conceived and established a new strategy of “omics discrimination-grey correlation-biological verification”. In this strategy, herbal metabolomics was used for high-resolution omics detection and analysis. The chemometrics method of gray correlation was employed to establish the correlation between the components and the activity. The zebrafish model was utilized to evaluate the activity of batches of TCM samples and verify the activity of the monomer components. The difficulty of bioactivity association of trace components of TCM was solved by this strategy. When compared with the existing techniques, the speed of research was greatly accelerated and the steps were simplified, which had obvious advantages.
The conventional view is that only the components which can enter the bloodstream is likely true pharmacodynamic substances of TCM. So whether with the potential of entering the bloodstream can be considered as an index for judging them as Q-Marker. Present studies have shown that at least 35 extracted components were detected in the bloodstream when GF was administered to animals (X. Zhang et al., 2018). Among the 10 components (geniposide, genipin-1-β-D-gentiobioside, crocin-1, quinic acid, gardenoside, d-glucuronic acid, l-malic acid, mannitol, rutin, and chlorogenic acid) examined for protective effects against liver injury and inflammation in this zebrafish experiment. Among them, geniposide, genipin-1-β-D-gentiobioside, crocin-1, gardenoside, rutin, and chlorogenic acid were found to significantly migrate to the blood (R. Zhang et al., 2020).
In this research, geniposide and crocin-1 were discovered as hepatoprotective pharmacodynamic markers of GF. On the other hand, quinic acid, gardenoside, d-glucuronic acid, l-malic acid, mannitol, rutin, chlorogenic acid, shanzhiside, d-glucaric acid, and genipin-1-β-D-gentioside were also confirmed as anti-inflammatory pharmacodynamic markers of GF. Some studies have reported that geniposide has significant hepatoprotective effects and gardenoside has anti-inflammatory effects. Zhang et al. (T. Zhang et al., 2016). found that geniposide can alter the abnormal liver metabolism caused by ethanol exposure, thereby alleviating the disturbance of amino acid metabolism and oxidative stress. Wang et al. (J. Wang et al., 2016). stated that geniposide can protect mice from liver injury caused by tripterygium glycosides as it alleviates oxidative stress and inflammation and promotes tissue repair and regeneration. Liang et al. (Liang et al., 2015) indirectly clarified that gardenoside may inhibit the production of inflammatory cytokines TNF-α, IL-1β, and IL-6 while exploring the effects of gardenoside on the steatosis of HepG2 cells induced by free fatty acids. Interestingly, we found that genipin-1-β-D-gentiobioside has a strong anti-inflammatory effect, which has not been previously reported in the literature. This effect warrants in-depth study to gather more evidence for the heat-reducing effect of GF. The iridoid of GF derived from Gardenia Ellis belonging to the Rubiaceae family is considered to be a representative component and a crucial chemical marker. Gardenoside, geniposide and genipin-1-β-D-gentioside are the main components of iridoids. Among them geniposide is the highest content. Quinic acid, d-glucuronic acid, l-malic acid, mannitol and chlorogenic acid belong to organic esters of GF, among which chlorogenic acid is its representative component. Rutin is the only flavonoid with anti-inflammatory activity in the results of this study (W. Li et al., 2021; Xiao et al., 2017). Therefore, based on the factors such as pharmacodynamic correlation, herbal specificity, and measurability, we believe that geniposide, genipin-1-β-D-gentiobioside, and gardenoside have good representativeness and can reflect the intrinsic quality of GF. Hence, they were identified to be the quality markers of GF.
Conclusion
In summary, we have devised a new strategy to study the Q-Markers of TCM with hepatoprotective and anti-inflammatory effects. In this research, through the effective integration of herbal metabolomics, zebrafish models, chemometrics, and other technologies, we have quickly identified “markers” that can reflect the intrinsic quality of TCM. We have also conducted research on the key pharmacodynamic components of GF and have proposed a combination of Q-Markers of GF, which provides the scientific basis for quality evaluation, resource utilization, and standard establishment of GF.
Data Availability Statement
The raw data supporting the conclusion of this article will be made available by the authors, without undue reservation, to any qualified researcher.
Ethics Statement
The animal study was reviewed and approved by the Ethics Committee of Shandong First Medical University, Shandong First Medical University and Shandong Academy of Medical Sciences.
Author Contributions
RD and YS performed the research, RD and QT analyzed the data and wrote the paper. SC, YZ, ZD, XW and QY were supportive during the experiment. LH designed and performed the study.
Funding
This work was financially supported by the Funding of the Natural Science Foundation of Shandong Province (ZR2019MH037, ZR2019MH051), the Key Project at Central Government Level: The ability establishment of sustainable use for valuable Chinese medicine resources (2060302), Agricultural Science and Technology Fund Project of Shandong Province (No.2019YQ033) and Academic Promotion Program of Shandong First Medical University (No. 2019LJ003).
Conflict of Interest
The authors declare that the research was conducted in the absence of any commercial or financial relationships that could be construed as a potential conflict of interest.
Acknowledgments
We thank Biology Institute of Shandong Academy of Sciences supplied the zebrafish line for us. And thank American Academic Journal Editor (http://www.scimj.com) for editing this manuscript.
References
Avdesh, A., Chen, M., Martin-Iverson, M. T., Mondal, A., Ong, D., Rainey-Smith, S., et al. (2012). Regular Care and Maintenance of a Zebrafish (Danio rerio) Laboratory: an Introduction. JoVE 69, e4196. doi:10.3791/4196
Bocca, C., Le Paih, V., Chao de la Barca, J. M., Kouassy Nzoughet, J., Amati-Bonneau, P., Blanchet, fnm., et al. (2021). A Plasma Metabolomic Signature of Leber Hereditary Optic Neuropathy Showing Taurine and Nicotinamide Deficiencies. Hum. Mol. Genet. 30 (1), 21–29. doi:10.1093/hmg/ddab013
Chen, L., Li, M., Yang, Z., Tao, W., Wang, P., Tian, fnm., et al. (2020). Gardenia Jasminoides Ellis: Ethnopharmacology, Phytochemistry, and Pharmacological and Industrial Applications of an Important Traditional Chinese Medicine. J. Ethnopharmacology 257, 112829. doi:10.1016/j.jep.2020.112829
Chiang, C.-C., Cheng, W.-J., Lin, C.-Y., Lai, K.-H., Ju, S.-C., Lee, fnm., et al. (2020). Kan-Lu-Hsiao-Tu-Tan, a Traditional Chinese Medicine Formula, Inhibits Human Neutrophil Activation and Ameliorates Imiquimod-Induced Psoriasis-like Skin Inflammation. J. Ethnopharmacology 246, 112246. doi:10.1016/j.jep.2019.112246
Chung, I.-M., Kim, J.-K., Han, J.-G., Kong, W.-S., Kim, S.-Y., Yang, Y.-J., et al. (2019). Potential Geo-Discriminative Tools to Trace the Origins of the Dried Slices of Shiitake (Lentinula Edodes) Using Stable Isotope Ratios and OPLS-DA. Food Chem. 295, 505–513. doi:10.1016/j.foodchem.2019.05.143
Fan, X., Lin, L., Cui, B., Zhao, T., Mao, L., Song, fnm., et al. (2020). Therapeutic Potential of Genipin in Various Acute Liver Injury, Fulminant Hepatitis, NAFLD and Other Non-cancer Liver Diseases: More Friend Than Foe. Pharmacol. Res. 159, 104945. doi:10.1016/j.phrs.2020.104945
Feng, S., Gan, L., Yang, C. S., Liu, A. B., Lu, W., Shao, P., et al. (2018). Effects of Stigmasterol and β-Sitosterol on Nonalcoholic Fatty Liver Disease in a Mouse Model: A Lipidomic Analysis. J. Agric. Food Chem. 66 (13), 3417–3425. doi:10.1021/acs.jafc.7b06146
Goessling, W., and Sadler, K. C. (2015). Zebrafish: an Important Tool for Liver Disease Research. Gastroenterology 149 (6), 1361–1377. doi:10.1053/j.gastro.2015.08.034
Gui, Y.-H., Liu, L., Wu, W., Zhang, Y., Jia, Z.-L., Shi, Y.-P., et al. (2020). Discovery of Nitrogenous Sesquiterpene Quinone Derivatives from Sponge Dysidea Septosa with Anti-inflammatory Activity In Vivo Zebrafish Model. Bioorg. Chem. 94, 103435. doi:10.1016/j.bioorg.2019.103435
Han, B., Zhang, Z., Xie, Y., Hu, X., Wang, H., Xia, fnm., et al. (2018). Multi-omics and Temporal Dynamics Profiling Reveal Disruption of central Metabolism inHelicobacter Pylorion Bismuth Treatment. Chem. Sci. 9 (38), 7488–7497. doi:10.1039/c8sc01668b
Han, Y., Wen, J., Zhou, T., and Fan, G. (2015). Chemical Fingerprinting of Gardenia Jasminoides Ellis by HPLC-DAD-ESI-MS Combined with Chemometrics Methods. Food Chem. 188, 648–657. doi:10.1016/j.foodchem.2015.05.039
Hayashi, M., Matsuo, K., Tanabe, K., Ikeda, M., Miyazawa, M., Yasaka, fnm., et al. (2019). Comprehensive Serum Glycopeptide Spectra Analysis (CSGSA): a Potential New Tool for Early Detection of Ovarian Cancer. Cancers 11 (5), 591. doi:10.3390/cancers11050591
Huang, B.-M., Zha, Q.-L., Chen, T.-B., Xiao, S.-Y., Xie, Y., Luo, fnm., et al. (2018). Discovery of Markers for Discriminating the Age of Cultivated Ginseng by Using UHPLC-QTOF/MS Coupled with OPLS-DA. Phytomedicine 45, 8–17. doi:10.1016/j.phymed.2018.03.011
Huang, B., Liang, J.-J., Zhuang, X., Chen, S.-W., Ng, T. K., and Chen, H. (2018). Intravitreal Injection of Hydrogen Peroxide Induces Acute Retinal Degeneration, Apoptosis, and Oxidative Stress in Mice. Oxidative Med. Cell Longevity 2018, 1–10. doi:10.1155/2018/54894762018
Jiang, R., Jiao, Y., and Xu, F. (2016). Chemical Derivatization-Based LC-MS for Metabolomics: Advantages and Challenges. Bioanalysis 8 (18), 1881–1883. doi:10.4155/bio-2016-0192
Kim, J.-H., Kim, K., and Kim, W. (2020). Genipin Inhibits Rotavirus-Induced Diarrhea by Suppressing Viral Replication and Regulating Inflammatory Responses. Sci. Rep. 10 (1), 15836. doi:10.1038/s41598-020-72968-7
Li, C.-Y., Tu, C., Gao, D., Wang, R.-L., Zhang, H.-Z., Niu, fnm., et al. (2016). Metabolomic Study on Idiosyncratic Liver Injury Induced by Different Extracts of Polygonum Multiflorum in Rats Integrated with Pattern Recognition and Enriched Pathways Analysis. Front. Pharmacol. 07, 483. doi:10.3389/fphar.2016.00483
Li, J.-Y., Cao, H.-Y., Sun, L., Sun, R.-F., Wu, C., Bian, Y.-Q., et al. (2017). Therapeutic Mechanism of Yīn-Chén-Hāo Decoction in Hepatic Diseases. Wjg 23 (7), 1125–1138. doi:10.3748/wjg.v23.i7.1125
Li, P., Ji, Y., Wu, Z., and Qu, S.-J. (2020). A New Multi-Attribute Emergency Decision-Making Algorithm Based on Intuitionistic Fuzzy Cross-Entropy and Comprehensive Grey Correlation Analysis. Entropy 22 (7), 768. doi:10.3390/e22070768
Li, W.-K., Wang, G.-F., Wang, T.-M., Li, Y.-Y., Li, Y.-F., Lu, X.-Y., et al. (2019). Protective Effect of Herbal Medicine Huangqi Decoction against Chronic Cholestatic Liver Injury by Inhibiting Bile Acid-Stimulated Inflammation in DDC-Induced Mice. Phytomedicine 62, 152948. doi:10.1016/j.phymed.2019.152948
Li, W., Ren, C., Fei, C., Wang, Y., Xue, Q., Li, fnm., et al. (2021). Analysis of the Chemical Composition Changes of Gardeniae Fructus before and after Processing Based on Ultra‐high‐performance Liquid Chromatography Quadrupole Time‐of‐flight Mass Spectrometry. J. Sep. Sci. 44 (5), 981–991. doi:10.1002/jssc.202000957
Liang, H., Zhang, L., Wang, H., Tang, J., Yang, J., Wu, fnm., et al. (2015). Inhibitory Effect of Gardenoside on Free Fatty Acid-Induced Steatosis in hepG2 Hepatocytes. Ijms 16 (11), 27749–27756. doi:10.3390/ijms161126058
Lieschke, G. J., and Currie, P. D. (2007). Animal Models of Human Disease: Zebrafish Swim into View. Nat. Rev. Genet. 8 (5), 353–367. doi:10.1038/nrg2091
Liu, C., Guo, D.-a., and Liu, L. (2018). Quality Transitivity and Traceability System of Herbal Medicine Products Based on Quality Markers. Phytomedicine 44, 247–257. doi:10.1016/j.phymed.2018.03.006
Liu, C. X., Chen, S. L., Xiao, X. H., Zhang, T. J., Hou, W. B., and Miao, M. L. (2016). A New Concept on Quality Marker of Chinese Materia Medica: Quality Control for Chinese Medicinal Products. Chin. Traditional Herbal Drugs 47 (09), 1443–1457.
Liu, M., Zhao, L., Han, L., Li, H., Shi, Y., Cui, fnm., et al. (2020). Discovery and Identification of Proangiogenic Chemical Markers from Gastrodiae Rhizoma Based on Zebrafish Model and Metabolomics Approach. Phytochem. Anal. 31 (6), 835–845. doi:10.1002/pca.2949
Liu, X., Yang, J., Yu, H., Zhang, J., Du, J., Wang, fnm., et al. (2021). Chemical Constituents from the Fruits of Cullen Corylifolium (L.) Medik. By the Targeted Separation Mode. Nat. Product. Res. 35 (7), 1071–1076. doi:10.1080/14786419.2019.1638382
Ma, J., Li, M., Kalavagunta, P. K., Li, J., He, Q., Zhang, fnm., et al. (2018). Protective Effects of Cichoric Acid on H2O2-Induced Oxidative Injury in Hepatocytes and Larval Zebrafish Models. Biomed. Pharmacother. 104, 679–685. doi:10.1016/j.biopha.2018.05.081
MacRae, C. A., and Peterson, R. T. (2015). Zebrafish as Tools for Drug Discovery. Nat. Rev. Drug Discov. 14 (10), 721–731. doi:10.1038/nrd4627
Nie, F., Wu, D., Wang, R., and Li, X. (2020). Truncated Robust Principle Component Analysis with a General Optimization Framework. IEEE Trans. Pattern Anal. Mach. Intell., 14, 8. doi:10.1109/tpami.2020.3027968
R Development Core Team (2011). R: A Language and Environment for Statistical Computing. Vienna, Austria: R Foundation for Statistical Computing. 3-900051-07-0.
Ren, J.-l., Zhang, A.-H., Kong, L., Han, Y., Yan, G.-L., Sun, fnm., et al. (2020). Analytical Strategies for the Discovery and Validation of Quality-Markers of Traditional Chinese Medicine. Phytomedicine 67, 153165. doi:10.1016/j.phymed.2019.153165
Robertson, A. L., Ogryzko, N. V., Henry, K. M., Loynes, C. A., Foulkes, M. J., Meloni, fnm., et al. (2016). Identification of Benzopyrone as a Common Structural Feature in Compounds with Anti-inflammatory Activity in a Zebrafish Phenotypic Screen. Dis. Model. Mech. 9 (6), 621–632. doi:10.1242/dmm.024935
Seo, M.-J., Hong, J.-M., Kim, S.-J., and Lee, S.-M. (2017). Genipin Protects D -galactosamine and Lipopolysaccharide-Induced Hepatic Injury through Suppression of the Necroptosis-Mediated Inflammasome Signaling. Eur. J. Pharmacol. 812, 128–137. doi:10.1016/j.ejphar.2017.07.024
Shi, Q., Cao, J., Fang, L., Zhao, H., Liu, Z., Ran, fnm., et al. (2014). Geniposide Suppresses LPS-Induced Nitric Oxide, PGE2 and Inflammatory Cytokine by Downregulating NF-Κb, MAPK and AP-1 Signaling Pathways in Macrophages. Int. Immunopharmacology 20 (2), 298–306. doi:10.1016/j.intimp.2014.04.004
Shi, Y.-p., Zhang, Y.-g., Li, H.-n., Kong, H.-t., Zhang, S.-s., Zhang, X.-m., et al. (2020). Discovery and Identification of Antithrombotic Chemical Markers in Gardenia Fructus by Herbal Metabolomics and Zebrafish Model. J. Ethnopharmacology 253, 112679. doi:10.1016/j.jep.2020.112679
Tian, J., Zhu, J., Yi, Y., Li, C., Zhang, Y., Zhao, fnm., et al. (2017). Dose-related Liver Injury of Geniposide Associated with the Alteration in Bile Acid Synthesis and Transportation. Sci. Rep. 7 (1), 8938. doi:10.1038/s41598-017-09131-2
Torraca, V., and Mostowy, S. (2018). Zebrafish Infection: from Pathogenesis to Cell Biology. Trends Cel Biol. 28 (2), 143–156. doi:10.1016/j.tcb.2017.10.002
Wang, F., Chen, L., Chen, H., Chen, S., and Liu, Y. (2019). Analysis of Flavonoid Metabolites in Citrus Peels (Citrus Reticulata "dahongpao") Using UPLC-ESI-MS/MS. Molecules 24 (15), 2680. doi:10.3390/molecules24152680
Wang, J., Miao, M., Qu, L., Cui, Y., and Zhang, Y. (2016). Protective Effects of Geniposide against Tripterygium Glycosides (TG)-induced Liver Injury and its Mechanisms. J. Toxicol. Sci. 41 (1), 165–173. doi:10.2131/jts.41.165
Wang, L., Liu, S., Zhang, X., Xing, J., Liu, Z., and Song, F. (2016). A Strategy for Identification and Structural Characterization of Compounds from Gardenia Jasminoides by Integrating Macroporous Resin Column Chromatography and Liquid Chromatography-Tandem Mass Spectrometry Combined with Ion-Mobility Spectrometry. J. Chromatogr. A 1452, 47–57. doi:10.1016/j.chroma.2016.05.026
Wang, L., Yang, C., Song, F., Liu, Z., and Liu, S. (2020). Therapeutic Effectiveness of Gardenia Jasminoides on Type 2 Diabetic Rats: Mass Spectrometry-Based Metabolomics Approach. J. Agric. Food Chem. 68 (36), 9673–9682. doi:10.1021/acs.jafc.0c02873
Wang, Q., Su, C. P., Zhang, H. M., Ren, Y. L., Wang, W., and Guo, S. Z. (2018). [Anti-inflammatory Mechanism of Heat-Clearing and Detoxifying Chinese Herbs]. Zhongguo Zhong Yao Za Zhi 43 (18), 3787–3794. doi:10.19540/j.cnki.cjcmm.20180611.012
Wu, X., Zhang, H., Fan, S., Zhang, Y., Yang, Z., Fan, fnm., et al. (2018). Quality Markers Based on Biological Activity: A New Strategy for the Quality Control of Traditional Chinese Medicine. Phytomedicine 44, 103–108. doi:10.1016/j.phymed.2018.01.016
Xiao, W., Li, S., Wang, S., and Ho, C.-T. (2017). Chemistry and Bioactivity of Gardenia Jasminoides. J. Food Drug Anal. 25 (1), 43–61. doi:10.1016/j.jfda.2016.11.005
Xu, L., Zhao, W., Wang, D., and Ma, X. (2018). Chinese Medicine in the Battle against Obesity and Metabolic Diseases. Front. Physiol. 9, 850. doi:10.3389/fphys.2018.00850
Zhang, R., Zhang, J. L., Li, B. T., Hua, C. L., and Liu, R. Q. (2020). Study on the Mechanism of the Components of Gardenia Fructus into Blood against Alzheimer's Disease Based on Network Pharmacology. J. Traditional Chin. Med. China 45 (11), 2601–2610. doi:10.19540/j.cnki.cjcmm.20200204.401
Zhang, T., Zhang, A., Qiu, S., Sun, H., Han, Y., Guan, fnm., et al. (2016). High-throughput Metabolomics Approach Reveals New Mechanistic Insights for Drug Response of Phenotypes of Geniposide towards Alcohol-Induced Liver Injury by Using Liquid Chromatography Coupled to High Resolution Mass Spectrometry. Mol. Biosyst. 13 (1), 73–82. doi:10.1039/c6mb00742b
Zhang, X., Pi, Z., Zheng, Z., Liu, Z., and Song, F. (2018). Comprehensive Investigation of In-Vivo Ingredients and Action Mechanism of Iridoid Extract from Gardeniae Fructus by Liquid Chromatography Combined with Mass Spectrometry, Microdialysis Sampling and Network Pharmacology. J. Chromatogr. B 1076, 70–76. doi:10.1016/j.jchromb.2018.01.023
Zhang, X., Shi, Y., Wang, L., Li, X., Zhang, S., Wang, X., et al. (2019). Metabolomics for Biomarker Discovery in Fermented Black Garlic and Potential Bioprotective Responses against Cardiovascular Diseases. J. Agric. Food Chem. 67 (44), 12191–12198. doi:10.1021/acs.jafc.9b04073
Zhang, Y., Wang, C., Jia, Z.-l., Ma, R.-j., Wang, X.-f., Chen, W.-y., et al. (2020). Isoniazid Promotes the Anti-inflammatory Response in Zebrafish Associated with Regulation of the PPARγ/NF-Κb/ap-1 Pathway. Chemico-Biological Interactions 316, 108928. doi:10.1016/j.cbi.2019.108928
Keywords: gardenia fructus, zebrafish, quality markers, herbal metabolomics, gray correlation analysis, anti-inflammatory, liver protection
Citation: Dong R, Tian Q, Shi Y, Chen S, Zhang Y, Deng Z, Wang X, Yao Q and Han L (2021) An Integrated Strategy for Rapid Discovery and Identification of Quality Markers in Gardenia Fructus Using an Omics Discrimination-Grey Correlation-Biological Verification Method. Front. Pharmacol. 12:705498. doi: 10.3389/fphar.2021.705498
Received: 05 May 2021; Accepted: 14 June 2021;
Published: 24 June 2021.
Edited by:
Luca Rastrelli, University of Salerno, ItalyReviewed by:
Rita Celano, University of Salerno, ItalyNorberto Peporine Lopes, University of São Paulo, Brazil
Copyright © 2021 Dong, Tian, Shi, Chen, Zhang, Deng, Wang, Yao and Han. This is an open-access article distributed under the terms of the Creative Commons Attribution License (CC BY). The use, distribution or reproduction in other forums is permitted, provided the original author(s) and the copyright owner(s) are credited and that the original publication in this journal is cited, in accordance with accepted academic practice. No use, distribution or reproduction is permitted which does not comply with these terms.
*Correspondence: Liwen Han, aGFubGl3ZW5Ac2RmbXUuZWR1LmNu
†These authors share first authorship