- 1Key Laboratory of Molecular Biophysics of the Ministry of Education, Hubei Key Laboratory of Bioinformatics and Molecular-imaging, Center of AI Biology, Department of Bioinformatics and Systems Biology, College of Life Science and Technology, Huazhong University of Science and Technology, Wuhan, Hubei, China
- 2Dovetree Synbio Company Limited, Shenyang, China
Traditional Chinese Medicine (TCM) is extensively utilized in clinical practice due to its therapeutic and preventative treatments for various diseases. With the development of high-throughput sequencing and systems biology, TCM research was transformed from traditional experiment-based approaches to a combination of experiment-based and omics-based approaches. Numerous academics have explored the therapeutic mechanism of TCM formula by omics approaches, shifting TCM research from the “one-target, one-drug” to “multi-targets, multi-components” paradigm, which has greatly boosted the digitalization and internationalization of TCM. In this review, we concentrated on multi-omics approaches in principles and applications to gain a better understanding of TCM formulas against various diseases from several aspects. We first summarized frequently used TCM quality assessment methods, and suggested that incorporating both chemical and biological ingredients analytical methods could lead to a more comprehensive assessment of TCM. Secondly, we emphasized the significance of multi-omics approaches in deciphering the therapeutic mechanism of TCM formulas. Thirdly, we focused on TCM network analysis, which plays a vital role in TCM-diseases interaction, and serves for new drug discovery. Finally, as an essential source for storing multi-omics data, we evaluated and compared several TCM databases in terms of completeness and reliability. In summary, multi-omics approaches have infiltrated many aspects of TCM research. With the accumulation of omics data and data-mining resources, deeper understandings of the therapeutic mechanism of TCM have been acquired or will be gained in the future.
Introduction
Traditional Chinese Medicine (TCM) has a long history of treating a variety of diseases around the world (Lan et al., 2015; Xiang et al., 2019; Runfeng et al., 2020). The most critical concerns of TCM are the quality of TCM formulas, as well as the effectiveness of TCM formulas, both of which have been extensively studied by researchers and physicians (Jia et al., 2017; Xin et al., 2018a; Xin et al., 2018b; Bai et al., 2019; Xiang et al., 2019; Ding et al., 2020; Liu et al., 2020; Chandrasekara et al., 2021). Western medications are typically prescribed individually for a specific effect with a single component. In contrast, classical TCM formulas, following TCM theory and the principle of “King, Vassal, Assistant, and Delivery servant,” are comprehensive systems with “multiple ingredients, multiple targets, and multiple pathways” to exert the synergistic effect in the prevention and treatment of diseases (Yi and Chang, 2004; Wang and Zhang, 2017). Their complexity in terms of components (especially for these structurally similar components), metabolites, and bioactivities, also hampered research into the therapeutic mechanism of TCM in treating various diseases. With the development of high-throughput sequencing technology, system biology-driven omics approaches meet the needs of TCM research, which could be applied to decoding complex components, targets, and drug-disease interactions (Subramanian et al., 2020). The multi-omics approaches refer to a series of research methods based on high-throughput analysis in modern biological research systems, which mainly include genomics, transcriptomics, metagenomics, metabolomics, epigenomics, and proteomics (Olivier et al., 2019; Subramanian et al., 2020). From the perspective of omics approaches, TCM could be considered as a combination of small molecules. Thus, a single TCM could treat multiple diseases by combining the small molecules with their respective gene/protein targets. After thousands of years of therapeutic practice in China, researchers have found a vast number of TCM formulas that are utilized for treating numerous diseases based on multi-omics approaches and clinical experiments, contributing to new drug discovery and therapeutic mechanisms (Buriani et al., 2012; Olivier et al., 2019; Guo et al., 2020), as well as advancing TCM towards precision medicine (Olivier et al., 2019).
While TCM has been extensively used in the treatment of various kinds of acute and chronic diseases worldwide (Bai et al., 2015; Kim et al., 2010; Miryala et al., 2018; Yang M. and Lao), several limitations have hindered the development of TCM research, including TCM formula quality control, multi-omics approaches on TCM analysis, and data resources for TCM research. Firstly, the quality control of TCM formulas is highly contentious (Yao et al., 2022). Thus, much attention should be paid to its ingredients and production process to ensure its safety and efficacy. Only if the ingredients of TCM formula are safe and lawful, TCM could exert its power in clinics worldwide, laying the groundwork for further studying the therapeutic mechanism, bioactive compounds, targets, and drug discovery to benefit humans. Moreover, TCM research is primarily experience-based and experiment-based methods, in which the small molecules of TCM and the specific targets for the treatment of diseases have not been thoroughly investigated, which impedes the modernization of TCM. Furthermore, the regulating principles of TCM formulas in human body behind the treatment of various diseases remain largely unclear.
Luckily, with the development of sequencing technology and system biology-driven omics approaches, TCM research has also advanced (Zhang et al., 2019b). The mechanisms of TCM and side-effects of TCM formulas were further deciphered at the molecular level (Buriani et al., 2012; Cai et al., 2018; Subramanian et al., 2020), leading to new therapeutic directions (Yang and Lao, 2019; Guo et al., 2020). These efforts are potentially accelerating TCM towards modernization (Qiu, 2007), and enhancing the applicability of the TCM formulas for personalized treatment (Aronson and Rehm, 2015; Jameson and Longo, 2015; Miryala et al., 2018). The concept of network pharmacology analysis is proposed to ascertain which bioactive compounds and targets are effective in treating various diseases. This approach entails deciphering a “compound-protein/gene-disease” network for TCM formulas and elucidating the regulatory principles of small molecules in a high-throughput manner. Furthermore, TCM-associated databases have been developed to meet the needs of TCM research, serving as a repository for TCM formula, herbal ingredients, bioactive compounds, targets, and TCM-disease interactions, and feedback for more comprehensive analysis of the network pharmacology analysis and omics research. These efforts would accelerate the internationalization and digitization of TCM formulas. However, to digitalize all TCM-related resources and fully excavate these resources for deeper understanding of TCM materials and formulas, the help of bioinformaticians would be important. In contrast, bioinformaticians need to better understand TCM research (Gómez-López et al., 2019).
In this review, we have reviewed and summarized the recent progress of multi-omics approaches in deciphering the mechanism of TCM against various diseases (Figure 1). We first discussed the currently widely used quality control methods of the TCM formula. Chemical and biological ingredients (Figure 1A) are both indispensable components for TCM, and we could assess TCM quality by detecting these ingredients using fingerprint-based chemical methods and DNA-sequencing-based biological methods (Figure 1B). Secondly, we emphasized the importance of integrating multi-omics in decoding the mechanism of TCM formulas in treating various diseases (Figure 1C). Thirdly, we also discussed the interaction of bioactive compounds and disease targets from the perspective of network analysis (Figure 1D). Finally, we compared different TCM databases based on their basic properties and search results (Figure 1E). These initiatives may help unravel the “black box” of the therapeutic mechanisms of the TCM formula, provide the best practices of contemporary bioinformatics analysis for TCM formulas, as well as contribute to the TCM toward digitalization, internationalization, and precision medicine. Of course, to translate these advances and fully excavate these resources, the routine clinical practice needs a bioinformatician with good training in clinics, which will help multi-omics approaches and datasets be of importance in better understanding the TCM towards precision therapeutics.
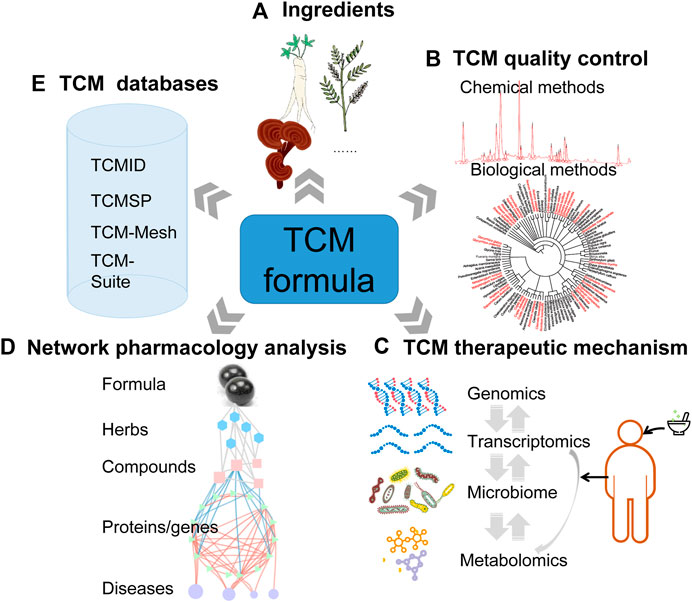
FIGURE 1. Systems biology-driven omics approaches have boosted TCM research from multiple perspectives. (A) Prescribed ingredients of TCM formula recorded in Chinese Pharmacopoeia (2020 edition). (B) The TCM quality control (fingerprint-based chemical ingredients analysis methods and genomics-related biological ingredients analysis methods). (C) Multi-omics approaches including genomics, transcriptomics, microbiome, and metabolomics could be utilized for investigating the therapeutic mechanism of the TCM formula for treating various diseases. (D) Network pharmacology analysis for screening the bioactive compounds and potential targets for TCM formulas in treating various diseases. (E) The databases for TCM research. The current databases serve as a link across TCM formulas, ingredients, bioactive compounds, targets (proteins/genes), and various diseases.
Omics approaches for TCM formula quality control: Biological and chemical ingredient analysis
Omics approaches, including fingerprint-based chemical ingredients analytical methods and DNA-sequencing-based biological ingredients analytical methods (related to genomics approaches), are essential for the quality assessment of TCM formulas. With the popularity of TCM, its quality control methods have become an important research topic to ensure its safety and effectiveness and thus promote the modernization and internationalization of TCM (Cheng et al., 2014; Baing et al., 2019; Yao et al., 2022). Although standards for the quality control of botanical drugs, processed products, TCM extracts, and TCM formulas have been documented in the Chinese Pharmacopoeia (ChP, 2020 edition) (Xu et al., 2021), the methods recorded in ChP are capable of discriminating substances from morphological, chemical, and physical characteristics, they are not precise enough to distinguish the ingredients from genetically related or hybrid species (Cheng et al., 2014).
The methods for chemical ingredient analysis
Currently, the most extensively utilized methods for TCM quality control are fingerprint-based methods (Cheng et al., 2014; Bai et al., 2019), such as thin-layer chromatographic scanning (TLCS), high-performance liquid chromatography (HPLC), gas chromatography (GC), and mass spectrometry (MS), which are focused on the bioactive compounds of the TCM formula. Among them, HPLC is characterized by high separation efficiency, high selectivity, high detection sensitivity, and rapid analysis, which is broadly applicated and usually coupled with other chromatographic methods in assessing the chemical ingredients of TCM formula (Heinisch and Rocca, 2009). For example, liquid chromatography-mass spectrometry (LC-MS)-based metabolic method has been utilized to detect the bioactive compounds of Kaixin Powder (KXP) in treating mentally depressed diseases (Zhu et al., 2010). They have found several bioactive compounds: ginsenosides, 3,6′-disinapoyl sucrose, α/β-asarone, and pachymic acid for Ginseng Radix et Rhizoma, Polygalae Radix, Acori Tatarinowii Rhizoma, and Poria in KXP based on LC-MS, respectively (Zhu et al., 2010). Apart from these five compounds, Wang et al. (2021), have firstly found two novel bioactive compounds of KXP (dehydrotrametenolic and dehydrotumulosic acids) from KXP using HPLC-DAD and HPLC-QTOF-MS/MS. These efforts have also resulted in the widespread use of chromatographic methods for TCM formula quality assessment (Liu et al., 2016b; Fan et al., 2021; Zeng et al., 2021).
The methods for biological ingredient analysis
On the other hand, biological ingredients are also indispensable components for TCM formulas, which could be identified through DNA barcoding-based approach. Out of the plenty of DNA barcodes (Table 1), ITS2, trnL, and psbA-trnH are the most frequently used herbal DNA barcodes, while COI is a well-known standard DNA barcode for animal materials detection (Coghlan et al., 2012; Li et al., 2018; Zhang et al., 2019a). Numerous studies have also used these representative barcodes to assess the biological ingredients of TCM formulas by detecting their prescribed species using high-throughput sequencing, which has detected 44.4%–100% of the prescribed ingredients of TCM formulas, suggesting the high universality, sensitivity, and specificity of this approach (Cheng et al., 2014; Jia et al., 2017; Joseph and Pe’er, 2021; Li et al., 2018; Xin et al., 2018a; Yao et al., 2022). In addition, DNA barcoding could also be applied to detect whether the prescribed ingredients are replaced by similar ingredients, such as the Arisaematis Rhizoma is replaced by Pinellia pedatisecta in the Ruyi Jinhuang Powder (Li et al., 2018). This approach could also be applied in auditing whether TCM formulas contain derivatives of protected species, especially for these endangered, trade-restricted species of plants and animals (Coghlan et al., 2012; Chen et al., 2015), which could promote the protection of wild species. Taken together, the DNA-barcoding approach innovatively identifies the prescribed ingredients of TCM at molecular level. It breaks away from the traditional morphological identification methods that heavily rely on long-term experience or experiment results, providing the “gene identity card” for TCM materials, and contributing to the standardization of the TCM industry.
However, there are also some limitations and challenges in fully utilizing the methods for TCM quality assessment. Firstly, the methods for chemical ingredients analysis were unable to discriminate the ingredients with comparable or unambiguous peaks (Wolfender et al., 2003). Secondly, fixating on chemical ingredients overlooks the biological characteristics that are also indispensable components of TCM formula (Cheng et al., 2014; Bai et al., 2015; Bai et al., 2019). Thirdly, though DNA barcoding-based approaches, the prescribed ingredients (recorded in ChP) of TCM formula could be detected (Table 1), they are unable to detect all prescribed ingredients of TCM formula. For example, trnL is incompatible with detecting fungal ingredients in TCM formulas (Cheng et al., 2014), and rbcL is a well-characterized barcode in GenBank, but this barcode is limited in identifying species from the same genera (Newmaster et al., 2006; Besse et al., 2021). Thus, more DNA barcode candidates should be considered for TCM biological ingredients assessment. Fourthly, the referenced prescribed species in the GeneBank are not enough for species identification. Further studies should take more databases into consideration, such as the DDBJ, EMBL, and PDB databases (Coghlan et al., 2012), TCM-suite (Yang et al., 2022), as well as tcmbarcode (Chen et al., 2014), and other databases. Finally, the genomics-based DNA barcoding approach could not detect the mineral ingredients. At the same time, the fingerprint-based chemical methods could overcome this drawback, and thus combining the methods for chemical ingredients analysis and the biological ingredients analysis could yield a more comprehensive and reliable assessment for TCM. Collectively, these efforts will benefit the safety issues of TCM formulas and accelerate the digitalized management process and internationalization of the TCM industry.
Omics techniques for understanding the therapeutic mechanism of TCM in treating various diseases
Omics approaches could be used to explain the complicated therapeutic mechanism of TCM against diseases from multiple levels (Xin et al., 2019; Guo et al., 2020), which could help us comprehend the relationship between TCM and human health. In the recent 20 years, high-throughput sequencing techniques have evolved at a breakneck pace. As omics technologies become mature, the multi-omics approaches are indispensable strategies for further understanding the therapeutic mechanism of TCM formulas (Buriani et al., 2012; Aronson and Rehm, 2015). In this review, we have summarized the omics approaches in TCM research, such as the prevalent chronic and metabolic diseases (i.e., diabetes), cancer (a leading cause of death worldwide, such as colorectal cancer, breast cancer, and lung cancer) (Fan et al., 2017; Lehmann et al., 2021; Sun et al., 2021), and infectious diseases (i.e., COVID-19, a global public health event) (Runfeng et al., 2020; Wu et al., 2021b; Chen et al., 2021), as well as the corresponding TCM formulas as examples to illustrate the critical role of multi-omics approaches for elucidating the therapeutic mechanism of TCM formulas in treating diseases (Figure 2).
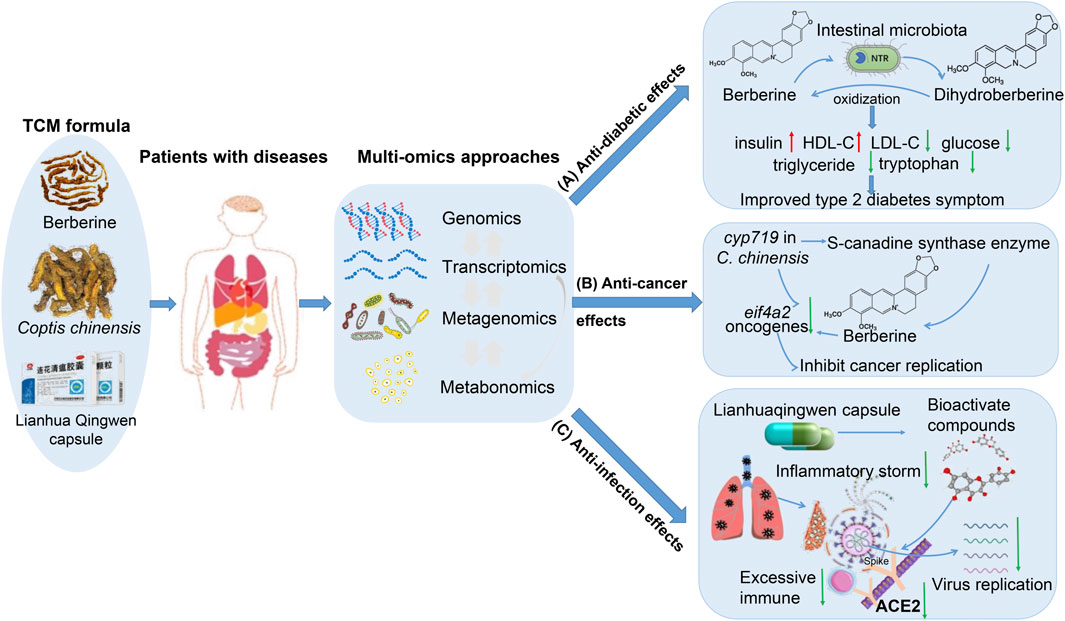
FIGURE 2. Multi-omics approaches are applicable for investigating the therapeutic mechanism of TCM in treating diseases. In this review, we used berberine, C. chinensis Franch. (Ranunculaceae), and Lianhua qingwen capuse as examples to illustrate the critical role of multi-omics approaches in elucidating the therapeutic mechanism of TCM. (A) Multi-omics analysis for berberine in treating type 2 diabetes mellitus. In this case study, intestinal microbes converted berberine as host absorbable molecule dihydroberberine to improve diabetes. NTR: nitroreductase. (B) Multi-omics analysis of C. chinensis Franch. (Ranunculaceae) for anti-cancer effect. Through multi-omics analysis, the functional genes (i.e., cyp719) and bioactive compounds (i.e., berberine) of C. chinensis Franch. (Ranunculaceae) have suppressed the eukaryotic translation initiation factor 4A isoform 2 (eif4a2) expression, and thus blocked cancer replication. (C) Multi-omics analysis for the potential therapeutic mechanism of Lianhua Qingwen capsule in COVID-19 patients. Through multi-omics analysis, the bioactive compounds of Lianhua Qingwen capsule could inhibit the combination of the viral target Spike and human target ACE2, thus preventing the viruses’ invasion and attachment to host cells and inhibiting the virus replication and pro-inflammatory cytokine levels (i.e., IL6, TNF-α), and chemokine levels, and thereby improving the symptoms of COVID-19 patients.
Intestinal microbiota-mediated anti-diabetic effects of berberine
Berberine as an extract of TCM formula from Berberis, Coptis, and Hydrastis (Habtemariam, 2020), is an excellent treatment for chronic and metabolic disorders diseases (Lan et al., 2015; Heindel et al., 2017). Its anti-diabetic function has been demonstrated by plenty of studies. Through metabolomics analysis, the researchers have found that berberine has improved glycometabolism (Dong et al., 2016; Yao et al., 2020) (Figure 2A). Zhang et al. (2010), used transcriptomics, proteomics, and found berberine increased the expression of InsR messenger RNA and protein in various human cells. They lowered the metabolites such as glycaemic levels, hemoglobin A, triglyceride levels, and increased insulin levels in type 2 diabetic (T2D) patients. As known, berberine exhibits poor oral bioavailability (Liu Chang-Shun et al., 2016a). While the intestinal microbes, which contain a specific enzyme called nitroreductase (NTR), are reported as the mediators that could convert berberine into absorbable molecule dihydroberberine (five times more absorption compared to berberine) for host, and then oxidize them back to berberine after absorption in the intestine (Chen et al., 2016; Feng et al., 2018) (Figure 2A). Zhang et al. (2020b), have used metagenomics and metabolomics data from the berberine treatment T2D patients, and found berberine reduced the glycaemic level by inhibiting deoxycholic acid species biotransformation by Ruminococcus bromii. Thus, the interactions between intestinal microbes and bioactive compounds of TCM formulas highlight the critical role of TCM and intestinal microbes in human health (Zhang et al., 2020a), implying that the intestinal microbes might be considered as potential targets for designing the drug delivery system.
Multi-omics analysis for understanding the anti-cancer effects of C. chinensis Franch. (Ranunculaceae)
Based on the genomics approach (Figure 2B), Chen et al., have used genomics approaches to analyze the genome of the C. chinensis Franch. (Ranunculaceae) and revealed the diversification of protoberberine-type alkaloids (Liu et al., 2021). They also discovered that the gene cyp719 is involved in berberine biosynthesis through encoding the enzyme called (S)-canadine synthase. Through metabolome and transcriptome analysis, researchers have found the bioactive compounds of C. chinensis Franch. (Ranunculaceae) such as berberine could downregulate the expression of eukaryotic translation initiation factor 4A isoform 2 (eif4a2) gene (Lv et al., 2016; Chou et al., 2017; Wang et al., 2019) (Figure 2B), which indirectly emphasized the important role of cyp719 gene of C. chinensis Franch. (Ranunculaceae) in anti-cancer effect. While eif4a2 gene is considered a novel target for cancer therapy and anti-cancer drug discovery (Lv et al., 2016; Chou et al., 2017; Wang et al., 2019), its inhibition prevents the cooperation between eif4a2 and oncogenes (Lindqvist and Pelletier, 2009), and thus effectively inhibits the replication of cancer and tumor (Lindqvist and Pelletier, 2009; Slaine et al., 2017).
Multi-omics analysis for understanding the potential anti-infection effects of Lianhua Qingwen capsule
TCM formula may exert comprehensive influences, enhancing the host’s ability to resist viral infection (Yuan and Lin, 2000; Jiang, 2005) (Figure 2C). Among numerous infectious diseases, COVID-19 is a well-known global public health event, which brings trouble to our daily life and huge burden worldwide. Researchers have found numerous TCM formulas that may achieve therapeutic outcomes for COVID-19 patients through multiple pathological pathways and TCM-disease targets across stages (Ren et al., 2020; Wu et al., 2021b). Chai et al., have analyzed the alteration of genetics, DNA methylation, and RNA expression for samples from COVID-19 patients, and found that during viral infection, angiotensin-converting enzyme 2 (ACE2) is identified as a membrane-binding receptor to the fusion and invasion process of the COVID-19 and host cells (Chai et al., 2020). And the COVID-19’ S protein (also called Spike protein; Figure 2C) could recognize and combine with the human cell protein ACE2, then use the host genetics to replicate new COVID-19 viruses (Hoffmann et al., 2020). These novel COVID-19 viruses were released and disseminated in the bloodstream. They then bind the ACE2 of other cells in COVID-19 patients, disrupting their equilibrium, and threatening other organs, such as the intestine, kidney, and heart of COVID-19 patients (Leung et al., 2020). Thus, the potential therapeutic effect of the TCM formula is to find the bioactive compounds to bind to host receptors such as ACE2, to prevent or inhibit the invasion/attachment of the virus to host cells (Figure 2C).
Lianhua Qingwen capsule is a classic patent TCM, which consists of 13 ingredients, including Forsythia suspensa (Thunb.) Vahl (Oleaceae; 255 g), Lonicera japonica Thunb. (Caprifoliaceae; 255 g), Prunus armeniaca L. (Rosaceae; 85 g), Ephedra sinica Stapf (Ephedraceae; 85 g), Isatis indigotica Fort. (Cruciferae; 255 g), Dryopteris crassirhizoma Nakai (Polypodiaceae; 255 g), Rheum palmatum L. (Polygonaceae; 51 g), Houttuynia cordata Thunb. (Saururaceae; 255 g), Pogostemon cablin (Blanco) Benth. (Blanco; 85 g), Rhodiola crenulata (Hook.f. and Thomson) H. Ohba (Crassulaceae; 85 g), Mentha haplocalyx Briq. (Lamiaceae; 7.5 g), Gypsum Fibrosum (255 g), Glycyrrhiza uralensis Fisch. ex DC. (Fabaceae; 85 g) (Xiao et al., 2020). This TCM is an innovative drug with potential therapeutic effects on respiratory diseases (Hu et al., 2022). Li et al., have assessed the antiviral effectiveness of Lianhua Qingwen capsule against SARS-CoV-2 in Vero E6 cells and human hepatocellular carcinoma cells using cytopathic effect inhibition assay, plaque reduction assay, and real-time quantitative PCR analysis (Runfeng et al., 2020). They have found that TCM formula Lianhua Qingwen could block the replication and expression of proinflammatory cytokines (i.e., IL6, TNF-α). and chemokine (i.e., CXCL-10, IP-10) caused by COVID-19, and thus considerably alleviating the symptoms and the development of the COVID-19 in patients (Runfeng et al., 2020; Wu et al., 2021b) (Figure 2C). Chen et al. found 132 bioactive metabolites in Lianhua Qingwen capsule in treating COVID-19 (Chen et al., 2021). Among them, five compounds (forsythoside I, forsythoside A, rhein, neochlorogenic acid, and its isomers) were further identified with high inhibitory effects on ACE2 receptor of host cells, implying that they could inhibit the invasion of the virus to the host cells, and thus improved the symptoms of COVID-19 patients (Chen et al., 2021). Collectively, these efforts provided insight into the molecular mechanisms of therapeutic effects of Lianhua Qingwen capsule in treating COVID-19, thereby confirming the applicability and vital significance of the TCM formula in infectious illness treatment.
Multi-omics approaches for TCM network analysis research
One of the most successful applications of omics approaches is network pharmacology analysis. TCM formulas typically incorporate various ingredients and multi-targets for synergistic anti-disease effects (Figure 3), which coincides with the concept of network pharmacology analysis (Zhang et al., 2019b; Yang et al., 2022). Network pharmacology analysis is presented to clarify which compounds of TCM formula exert effects in treating diseases and which targets could be exploited for drug design (Boezio et al., 2017; Wu et al., 2018; Zhang et al., 2019b), which has developed into a powerful method for leveraging the “ingredients-compounds-proteins/genes-diseases” network for TCM research (Zhang et al., 2019b; Yang et al., 2022). Multi-omics such as transcriptomics, genomics, epigenomics, and proteomics could be used to determine the ingredients, proteins, and/or genes of TCM formulas, whereas electrochemical method, HPLC, real-time qPCR, and other quantified methods can be used to determine and quantify the content of bioactive compounds (Wolfender et al., 2003; Zhu et al., 2010; Wang et al., 2021). While network pharmacology analysis could facilitate the integration of ingredients, bioactive compounds, and proteins/genes into a network, providing an effective resolution for exploring the TCM-disease interaction (Zhang et al., 2019b).
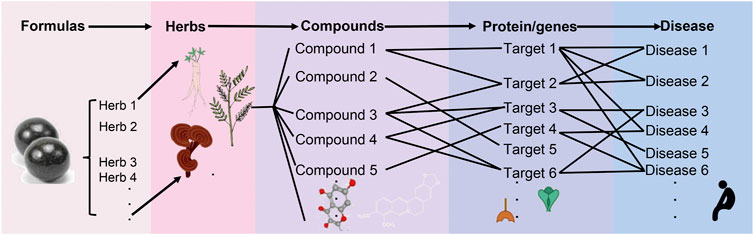
FIGURE 3. TCM network pharmacology analysis is proposed for leveraging the “ingredients-compounds-proteins/genes-diseases” network for TCM research. A TCM formula is made according to the Chinese Pharmacopoeia, herbal ingredients were the major prescribed ingredients in most TCM formulas. While based on network pharmacology analysis, chromatography, and high-throughput sequencing technology, we could decode the relationship between TCM formulas-species ingredients-compounds-targets-diseases. By looking at the bioactive compounds in the prescribed ingredients, we could learn more about the herb, the bioactive compounds, the target proteins, and the genes in treating diseases. This would help us understand how TCM works to treat different diseases.
Network pharmacology analysis in decoding the roles of LDW in treating type 2 diabetes
T2D is a chronic metabolic disease that affects approximately affecting nearly 425 million people around global, leading to poor health status and massive economic outputs (Lin et al., 2015; Gan et al., 2019), causing substantial damage to the blood vessels, heart, and brain with long-time hyperglycemic status (Adeshara et al., 2016; Areosa Sastre et al., 2017). It was reported that Liuwei Dihuang Wan (LDW) is a patented TCM formula for treating T2D (Cheng et al., 2014; He et al., 2019). Based on the bioactive compounds recorded in TCMSP (30,069 bioactive compounds; http://sm.nwsuaf.edu.cn/lsp/tcmsp.php), BATMAN (25,210 bioactive compounds; http://bionet.ncpsb.org/batman-tcm/), and TCM Database@Taiwan (351 bioactive compounds; http://tcm.cmu.edu.tw/) databases, He et al. (2019), used the network pharmacology analysis and found 45 active compounds of LDW ingredients, which exert their pharmacological effects on T2D. As known, people with T2D were identified to have higher levels of inflammatory cytokines, such as IL-1B and IL-6, and lower levels of IL-10 (Kologrivova et al., 2014; Fathy et al., 2019), and the inflammatory cytokines disorder were also reported as biomarkers for early T2D diagnosis (Senthilkumar et al., 2018; Fathy et al., 2019). While the bioactive compounds of LDW, such as beta-sitosterol, isofucosterol, quercetin, are enriched in anti-inflammatory, anti-oxidative stress responses pathway by interacting with the target genes (i.e., akt1, ptgs2), which could decrease the inflammatory and remove oxidative stress and reactive oxygen species (Chasseaud, 1979; Sheehan et al., 2001), thus improved the symptoms in T2D patients. These findings imply that LDW may play an important role in treating T2D through the bioactive compounds interacting with the key or central genes in the development of T2D.
Network pharmacology analysis in decoding the roles of TCM in treating the cardiovascular and cerebrovascular diseases
Cardiovascular and cerebrovascular disease (CCVD) is a term that refers to an illness that affects the heart, brain, or blood vessels (Tao et al., 2013; Wang et al., 2020). According to the WHO, this disease contributes to 32% of fatalities worldwide [https://www.who.int/news-room/fact-sheets/detail/cardiovascular-diseases-(cvds)]. Through network pharmacology analysis, Wang et al. (2020) have found and experimentally verified superoxide dismutase, glutathione peroxidase, malondialdehyde, and nitric oxide synthase of Safflower and Salvia as promising therapeutic targets for treating cerebral and myocardial infarction in. Safflower can decrease the cerebral infarction rate, cerebral cell edema, glial cell proliferation, and inflammatory cell infiltration, as well as increase the content of superoxide dismutase and glutathione peroxidase, and decrease the content of malondialdehyde and nitric oxide synthase (Wang et al., 2020). Moreover, Tao et al. (2013), incorporated the chemical compounds including the chemical structure and pharmacological information, as well as biological functional data of TCM formula Radix Curcumae, and found 58 bioactive compounds and 32 potential targets for the prevention and/or treatment of CCVD. In summary, network pharmacology analysis sheds fresh light on the TCM-disease interactions, providing potential therapeutic targets for drug discovery.
Network pharmacology analysis in decoding the roles of TCM in treating cancer
With the advancement of multi-omics approaches, many TCM formulas have been mined for cancer prevention and treatment (Fan et al., 2017; Xiang et al., 2019; Lehmann et al., 2021; Sun et al., 2021). In this review, we took CRC as an example to elucidate the omics approaches underlying the mechanisms of TCM against cancer. CRC is diagnosed as the third major malignant tumor worldwide, which contributes to the fourth leading cause of cancer death (Arnold et al., 2017). Using network analysis to integrate multiple-omics data, researchers have found that the bioactive compounds in the TCM formula Huang Lian-Gan Jiang (Gingerenone C, Obaculactone, and Isogingerenone B) could interact with the main targets of CRC (i.e., PDE5A, TGFBR2, HRAS) to regulate the signaling pathways (i.e., DAP12, NGF, AKT signaling pathway), contributing to therapeutic effects for CRC patients (Gong et al., 2019). Among these targets, FGFR4 has been confirmed with the ability to inhabit CRC cell proliferation, promote apoptosis, and disrupt the cell cycle, which could be a novel target for drug design and new therapeutic direction (Jiang et al., 2017). In addition, Shaoyao decoction was verified to increase the survival rate and decrease the occurrence of colonic neoplasms in the mice model (Lin et al., 2014). Moreover, through network pharmacology analysis, the bioactivate compounds Zuojinwan: quercetin, baicalein, and wogonin combined with the target genes akt1, jun, and thus activate the important pathway such as PI3K-Akt signaling pathway to induce apoptosis to exert anti-cancer effects in CRC patients (Huang et al., 2020). Relevant scientific research also demonstrated that the Ampelopsis ethanolic extract could inhibit stat3 and Src phosphorylation, and downregulate the expression of their target genes, such as bcl-xL, mmp-2 in CRC patients (Sun et al., 2021). These curative or preventative effects of TCM for various diseases have been demonstrated in rodent or human studies (Sun et al., 2021). The candidate bioactive compounds and targets screened by network pharmacology analysis could guide new drug discovery and therapeutic direction.
Databases and analytical resources for TCM research
The TCM-associated databases are repositories for storing omics data, including ingredients, compounds, genes, targets, and diseases. Based on a comprehensive screening analysis of TCM-associated databases, the network pharmacology analysis has further deepened the therapeutic effects of TCM. Numerous efforts have also been put into the establishment of TCM-associated databases. In this section, we present the most frequently used databases, and then use the well-studied ingredients to assess the properties of these TCM-associated databases.
Current TCM-associated database for TCM research
Currently, numerous TCM databases have emerged in response to the need for different aspects of TCM-disease studies (Mendez et al., 2019; Fang et al., 2021). In this review, we processed the development of the most frequently used TCM databases and their updated versions during our evaluation (Figure 4).
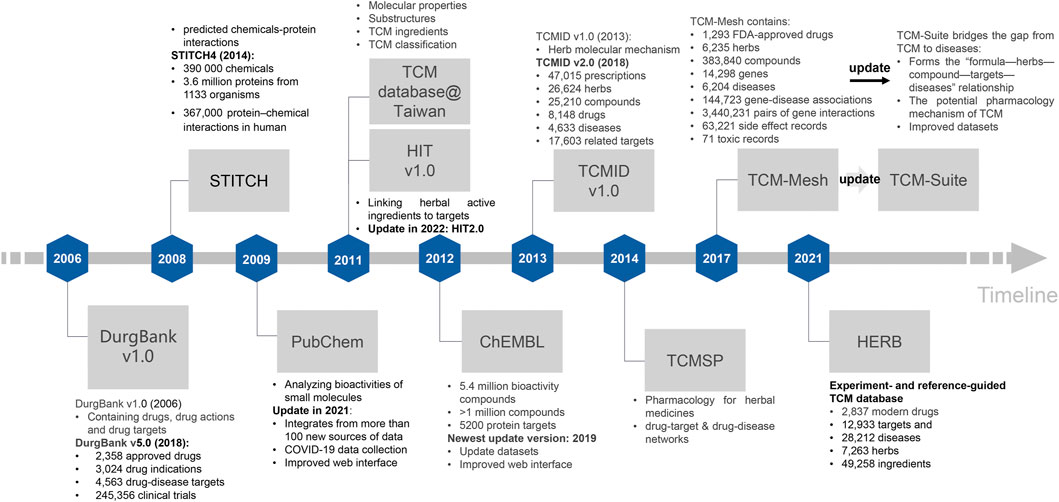
FIGURE 4. The development of commonly used TCM-disease databases along the timeline. We described the TCM databases based on their initial and most recent updated versions, respectively, which highlighted the primary composition, function, and characteristics of these databases along the timeline. The updated version of a database is highlighted in bold font of the description.
DurgBank (http://www.drugbank.ca) was initially reported in 2006, and has been updated continuously over the past years (Wishart David S. et al., 2006). The most recent version is DurgBank 5.0, which comprises 2,358 FDA-approved small molecular pharmaceuticals and 4,964 experimental drugs, as well as 7,129 unique drug targets and other drug-associated informantion (Wishart et al., 2018) (Figure 4). STITCH is primarily concerned with decoding chemical-protein interactions (Kuhn et al., 2008), its most recent version STITCH4 has 390,000 chemicals and 3.6 million proteins from 1,133 organisms, and 367,000 protein–chemical interactions in human (Kuhn et al., 2014). PubChem (http://pubchem.ncbi.nlm.nih.gov) is used to search for the 2D and 3D bioactivities of small molecules (Wang et al., 2009), which was updated in 2021. The updated version of PubChem has integrated more than 100 Corona Virus Disease 2019 (COVID-19) datasets, such as chemical, physical property, and patent associated information, and supports various types of structure search (such as identity, similarity, substructure, and superstructure searches) via its web interfaces (Kim et al., 2021) (Figure 4). TCM Database@Taiwan (http://tcm.cmu.edu.tw/) is one of the largest non-commercial TCM databases for drug screening that launched in 2011 and contains over 20,000 pure compounds derived from 453 TCM ingredients (Chen, 2011). HIT (Herb Ingredients’ Targets, http://lifecenter.sgst.cn/hit/) is a database that connects herbal active ingredients to targets (Ye et al., 2011), which is updated in 2022 (HIT2.0). HIT2.0 has 10,031 compound-target activity pairs with quality indicators between 2,208 targets and 1,237 ingredients from more than 1,250 reputable herbal materials (Yan et al., 2022). ChEMBL (https://www.ebi.ac.uk/chembldb) contains 5.4 million bioactivity compounds for more than 1 million compounds and 5,200 protein targets (Mendez et al., 2019), and the most recent upgraded version is in 2019 with the improved dataset and web interfaces (Mendez et al., 2019). TCMID database (Traditional Chinese Medicine Integrated Database, http://www.megabionet.org/tcmid/) integrates the molecular mechanism of herbal materials (Xue et al., 2013). Its updated version TCMID2.0 is more comprehensive and contains 18,203 herbal ingredients, 15 prescriptions, 82 associated targets, 1,356 drugs, 842 diseases, and 170 herbal materials associated with mass spectrometry spectra (Huang et al., 2018). TCMSP (http://sm.nwsuaf.edu.cn/lsp/tcmsp.php) has considered the pharmacodynamics and pharmacokinetics for systemic pharmacology research, such as the bioactive compounds and compound-related targets screening (Ru et al., 2014), which includes 499 herbal materials with 29,384 ingredients, 3,311 targets, and 837 associated diseases in ChP, as well as 12 important absorptions, distribution, metabolism, and excretion related properties (Ru et al., 2014). TCM-Mesh (http://mesh.tcm.microbioinformatics.org/) was established based on the concept of the “TCM formula-compounds-proteins/genes-diseases” network to elucidate the regulatory mechanisms governing tiny molecules in TCM formula, as well as recording their side-effects (Zhang et al., 2017). Since pharmacotranscriptomics has become a powerful method for assessing the therapeutic efficacy of drugs, and identifying novel drug targets, HERB (high-throughput experiment- and reference-guided database of TCM, http://herb.ac.cn/) is built based on manually curated 1,241 gene targets and 494 modern diseases for 473 herbal materials, linking with 12,933 targets and 28,212 diseases to 7,263 herbs and 49,258 ingredients (Fang et al., 2021). To solve the problem of lacking holistic and systematical analysis of TCM formula, the newest database named TCM-suite (http://tcm-suite.aimicrobiome.cn/) was built (Yang et al., 2022). This database is a holistic pipeline that connects TCM biological and chemical ingredient identification and downstream network pharmacology analysis. It establishes a detailed “TCM formula-ingredients-compounds-proteins/genes-diseases” relationship. It enables users to identify components of a TCM formula and investigate its potential pharmacology mechanism and side effects simultaneously. These databases with different usages have provided the opportunity to explore the potential pharmacology mechanism of TCM formula at the molecular level in the treatment of numerous diseases.
Comparing the properties of frequently used TCM database
The frequently used TCM databases also have different focuses and comprehensiveness. In this work, the databases (Google Scholar citations: as of April 2022: TCMSP: 1748, TCMID: 580, TCM-Mesh: 114), as well as TCM-Suite (http://tcm-suite.aimicrobiome.cn/) were chosen for comparison of their properties (Table 2). By comparing the results from different databases, we found that although the bioactive compounds recorded in the TCM-Suite database were fewer than those in other TCM-associated databases, the data of compound-target associations and target-disease associations were more abundant in this database. Additionally, we have discovered TCM-Suite database is more comprehensive in terms of the compound-target associations and target-disease associations. Most of the ingredients, with fewer bioactive compounds being collected in this database, were matched with more hits in bioactive compound-target associations and target-disease associations. The fewer bioactive compounds in TCM-Suite are probably because the TCM-Suite database removes the ambiguous bioactive compounds of TCM formulas.
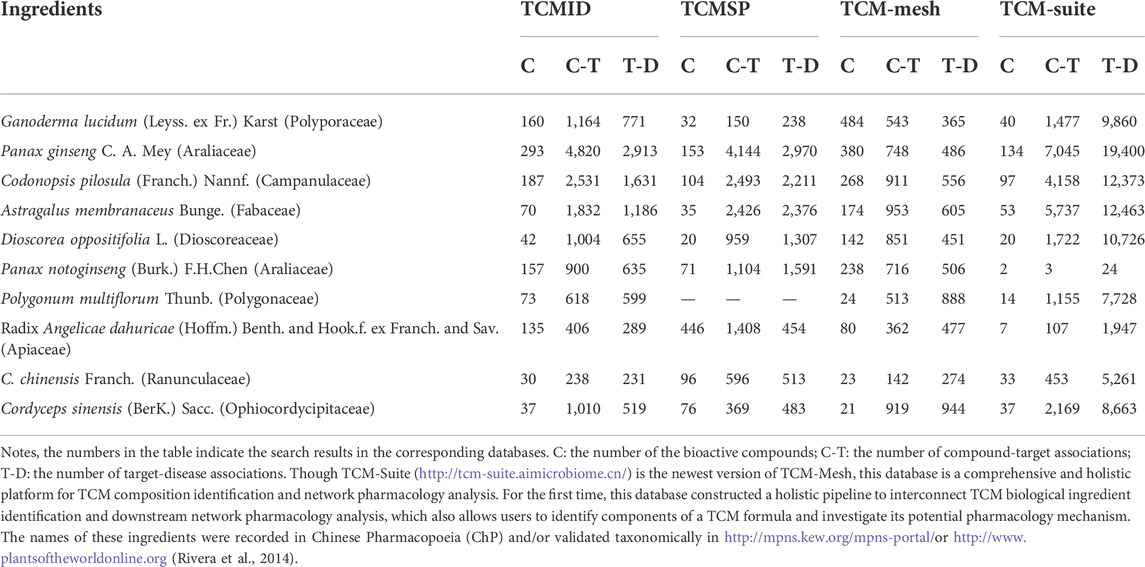
TABLE 2. Comparing the properties of four representative TCM databases based on the selected ingredients reported in the previous study (Zhang R. et al., 2019b).
Discussion
While numerous works have evaluated the multi-omics approaches in TCM research, this is the first systematic and comprehensive review of omics approaches in TCM research from a holistic standpoint. In this review, we have summarized the recent progress of multi-omics approaches in deciphering the mechanism of TCM against various diseases as compared to the previous research and discussed the advantages and limitations, as well as the future direction of multi-omics applied to the field of TCM. With the development of systems biology-driven omics analysis, TCM research has profoundly advanced (Wu et al., 2019; Wu al., 2021a; Gao et al., 2021), which means that TCM studies have already undergone a paradigm shift from the traditional biochemical and molecular analysis approaches to the data-driven omics analysis approaches (Figure 5). Analytical chemistry and biology approaches have pushed forward the development of TCM research and laid the groundwork for applying omics approaches (Zeng et al., 2019). In contrast, the omics approaches have the potential to significantly supplement the traditional analysis approach with more precise and comprehensive approaches, which has undoubtedly made a significant contribution to deciphering the mechanism of TCM from various aspects (bioactive compounds, TCM-drug targets, pharmacodynamic effects, and therapeutic mechanisms). This accomplishment has also sparked various anxieties and concerns for TCM, given the volume of heterogeneous data on issues that may be solved using omics data for the TCM research areas (Figure 5).
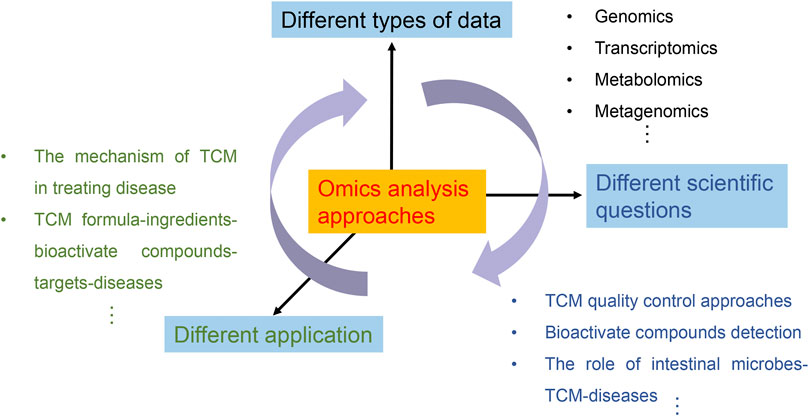
FIGURE 5. TCM research in the omics age has utilized different types of omics data to address different scientific questions, and meet the needs of different applications. With the development of sequencing technology, different types of data including genomics, transcriptomics, metabolomics, and metagenomics data could be gathered to address various scientific topics. The molecular mechanism of TCM formula in treating various diseases is gradually exposed by omics analysis approaches, and the relationship of TCM bioactive compounds-targets-diseases is constructed, providing suggestions for drug-target design. These scientific questions in turn contribute to the practical value of TCM materials, and the different applications will also create different types of data. This bidirectional interaction reinforces the clinical use of TCM and drives its modernization.
In this review, we have evaluated the TCM formulas from four aspects to address several anxieties and concerns about TCM. Firstly, the inconsistent quality control methods make TCM disputable (Yao Q. et al., 2022). Based on the advancement of sequencing and chromatographic technologies, researchers have combined chemical methods (i.e., HPLC, TLC) (Wolfender et al., 2003; Zhu et al., 2010; Wang Binbin et al., 2021) and biological methods (DNA fragments: ITS2, trnL, trnH-psbA, and COI) (Vences et al., 2005; Jia et al., 2017; Xin et al., 2018a; Xin Tianyi et al., 2018b; Zhang et al., 2019a; Chandrasekara et al., 2021) for quality control of TCM formula through detecting their prescribed ingredients. These initiatives have also yielded significant results in terms of evaluating the quality control of TCM formulas18-20, which would push forward the digital management of TCM. These advancements are inseparable from the database with the storage of referenced materials and the omics approaches, which ensure the legality and therapeutic efficiency of TCM formulas (Newmaster et al., 2006; Chen et al., 2014; Cheng et al., 2014; Guo et al., 2020; Subramanian et al., 2020; Besse et al., 2021).
Secondly, omics analysis approaches would provide clues for clinical applications of TCM formulas, thereby deepening our understanding of the therapeutic mechanism of TCM formulas. Numerous forms of data for TCM research have been analyzed to understand the therapeutic effects of TCM formulas on human diseases (Wang et al., 2015; Dong et al., 2016; Subramanian et al., 2020). Because the majority of the TCM formulas are administered orally, which typically come into contact with intestinal microbes and are transformed into host absorbable molecules under microbes, allowing for optimal utilization of TCM formulas (Feng et al., 2015; Chen Feng et al., 2016; Feng et al., 2018). Through the omics approach, TCM formulas have also been applied in the treatment of certain diseases, and show outstanding anti-diseases, such as cancer (Xiang et al., 2019; Liu et al., 2020), COVID-19 (Fan et al., 2020; Leung et al., 2020), and chronic diseases (Deng, 2010; Dong et al., 2016; Yang et al., 2019; Guo et al., 2020). All of these findings, which are based on omics datasets and approaches, have allowed for the identification of the mechanism underlying significant therapeutic effects (Feng et al., 2015; Liu et al., 2016a; Chen et al., 2016; Feng et al., 2018; Zhang et al., 2020a).
Thirdly, with its notion of integrity, comprehensiveness, and systematic approach, network analysis offers new strategies and approaches for researching TCM formulas, as it may transform TCM from an experience-based to an evidence-based medical system. From a network view based on different types of omics datasets and approaches, we can decode the molecular mechanism of the complicated TCM formulas (Tao et al., 2013; Li et al., 2014). That is, the relationship between TCM formulas and diseases could be divided into prescribed ingredients, bioactive compounds of prescribed ingredients, compounds-targets, and diseases-targets interactions (Zhang et al., 2017), which confirms the advantages of multi-compound, multi-target and multi-pathway characteristics of TCM formula and providing a reliable solution for systemic analyzing the clinical efficacy of TCM formula (Luo et al., 2020). These efforts will benefit target discovery, bioactive compound screening, toxicity evaluation, and the mechanism elucidation of TCM. These efforts may deepen our understanding of how TCM formulas could treat diseases. Based on these advantages, future applications of multi-omics approach in TCM research could be focused on drug mining, drug design, drug re-purposing, and therapeutic mechanisms investigation to make contributions to the modernization of TCM. Network pharmacology analysis is a systems biology-based approach, which selects specific signal nodes for multi-target drug molecule design to uncover the molecular mechanisms underlying the pharmacodynamic effects (Luo et al., 2020). This analytical approach largely depends on the high volume of data, while the current resources including medicines, genes, proteins, etc. are not exhaustive (Yang et al., 2022). It cannot be assured that the databases on TCM, bioactive compounds of TCM, and TCM-related gene targets are accurate and comprehensive. Moreover, no suitable calculation methods have been developed to calculate the correlations in network analysis. Network pharmacology analysis employs computer network screening for target selection, and these correlations were calculated without considering the distribution character of TCM data. Hence, influenced by cascade amplification, the number of disease targets and medication target proteins is gradually expanding, and the interpretation of data cannot keep up with the growth of data. Additionally, the network pharmacology analysis in TCM, such as new targets, or drug mechanism discovery, is still qualitative, and the scientific and mechanism discovery of TCM through network analysis still needs critical and extensive clinical trials. Furthermore, the dose-efficacy relationship between the TCM formula and disease remains further explored and quantified. Last but not least, human disease is dynamic changes, so the course of disease development and treatment efficacy process should be considered in further studies.
Fourthly, the TCM-associated databases provide plenty of TCM-related data, which were created and are continuously updated with the demand for TCM research. We proposed the TCM-Suite database (http://tcm-suite.aimicrobiome.cn/) (Yang et al., 2022), which overcomes the limitations of the currently available databases for network analysis and other omics analysis, and serves as a bridge for integrating the prescribed ingredients of TCM formulas, the bioactive compounds of prescribed ingredients, compounds-targets, and disease-targets as a holistic concept. This database establishes the foundation for the systemic investigation of the therapeutic effects from a network analysis perspective, facilitating the clinical application value and drug development. Given the importance of intestinal microbes in assisting the host’s absorption of bioactive compounds (Feng et al., 2015; Chen et al., 2016; Feng et al., 2018), further database developments should take the interaction between intestinal microbes, TCM formulas, and human symptoms into consideration for building up more complete and exhaustive databases, making the TCM research more concise and convenient. With the need for TCM research, various TCM-related databases have emerged, but the query of these databases is quite different. For example, the herbal material “C. chinensis Franch.” could be accessed by searching “huang lian” or “Coptidis” in TCMID (Huang et al., 2018), “huanglian” in TCMSP (Ru et al., 2014), whereas in the TCM-Suite database (Yang et al., 2022), this herbal material could be accessed by searching “huang lian” or “C. chinensis.” And thus, a platform considering the various official name/ID or a standard platform for TCM research is needed, which could make the databases more concise and available, enabling the researchers to make full use of the resources.
Collectively, systems biology and data-driven omics analysis approaches, including genomics, proteomics, metagenomics, transcriptomics, and metabolomics, play an indispensable role in decoding the interactions of TCM and diseases, which could comprehensively and deeply decode the therapeutic mechanisms of the TCM formula. These efforts have hastened the translation of TCM from fundamental research to a broad spectrum of applications, particularly in clinical applications.
However, apart from the aforementioned limitations for each part of TCM analyses, there are also several limitations of the multi-omics approach applied to TCM. Consider network analyses is still at a very preliminary level of evidence and might often be used misleadingly. Firstly, the high dimensionality, high complexity, and high volume of heterogeneous data make it difficult for TCM analysis. Secondly, there is a lack of data resources for in-depth interpretation of TCM preparations: from identifying herbal ingredients and their bioactive compounds to understanding how small molecules in herbal formulas interact with genes and proteins in the human body. And the high chemical complexity of TCM makes it challenging to characterize their bioactive compounds and investigate systemic actions in humans. Thirdly, the limited knowledge of biochemistry has constrained our further understanding of TCM pharmacological and toxicological effects. Fourthly, even under the idea of “homology of medicine and food” (Hou and Jiang, 2013; Gong et al., 2020), there is limited knowledge about the medicinal value and mechanism of medicinal and edible plants and their effects on human health. A deeper understanding of these medical homologous plants will help people adjust their health according to their daily diet. Fifthly, even though multi-omics have been extensively used in TCM research and clinical practices, more digital information, including protein structure information, biological images, as well as electronic medical records, could be integrated for better understanding of the TCM-disease relationships. Finally, the application of omics approaches in new drug discovery still has a long way to go, pharmacodynamic effects of the new drugs should perform with critical clinical trials. In the future research, it will be useful to highlight the current pitfalls in these approaches and how they can be overcome.
To achieve this, a bioinformatician with good clinical training should act as a bridge to translate multi-omics approaches and datasets into routine clinical practice to assist with clinical diagnosis. We believe these efforts are beneficial for the process of TCM towards modernization, internationalization, and digitalization in the treatment of a variety of complicated diseases.
Conclusions
In this review, we have summarized the recent progress of multi-omics approaches in deciphering the mechanism of TCM against various diseases as compared to the previous research and discussed the advantages and limitations, as well as the future direction of multi-omics applied to the field of TCM. With the development of systems biology-driven omics analysis. And we suggested combing the chemical and biological ingredients analytical methods to get a more objective and comprehensive assessment of TCM quality. Moreover, with the help of bioinformaticians, omics analysis approaches would accelerate the unveiling of the mystery of TCM, and move it towards internationalization. Besides, assisted by network analysis, the link between TCM and diseases could be visualized from ingredients to compounds to targets, which could enhance our understanding of the relationship between TCM and diseases. Furthermore, with heterogeneous TCM data, databases serve as a repository for these data, which promote the modernization and internationalization of TCM and potentially provide critical technological support for clinical diagnosis, drug development, and precision medicine. We believe under the guidance of bioinformaticians, these efforts are advantageous for the TCM process including modernization, internationalization, and digitalization.
Author contributions
KN and HB designed the study. XZ performed the review. KN, HB, RY, XZ, PY, DZ, and QY revised and proofread the manuscript. All the authors have read and approved the final manuscript.
Funding
This work was partially supported by the National Science Foundation of China grant (Grant Numbers. 81774008, 81573702, 32071465, 31871334, and 31671374), the Ministry of Science and Technology’s national key research and development program grant (Grant Number. 2018YFC0910502).
Conflict of interest
Author RY was employed by Dovetree Synbio Company Limited.
The remaining authors declare that the research was conducted in the absence of any commercial or financial relationships that could be construed as a potential conflict of interest.
Publisher’s note
All claims expressed in this article are solely those of the authors and do not necessarily represent those of their affiliated organizations, or those of the publisher, the editors and the reviewers. Any product that may be evaluated in this article, or claim that may be made by its manufacturer, is not guaranteed or endorsed by the publisher.
References
Adeshara, A. K., Diwan, G. A., and Tupe, R. S. (2016). Diabetes and complications: Cellular signaling pathways, current understanding and targeted therapies. Curr. Drug Targets 17, 1309–1328. doi:10.2174/1389450117666151209124007
Areosa Sastre, A., Vernooij, R. W., Gonzalez-Colaco Harmand, M., and Martinez, G. (2017). Effect of the treatment of Type 2 diabetes mellitus on the development of cognitive impairment and dementia. Cochrane Database Syst. Rev. 6, CD003804. doi:10.1002/14651858.CD003804.pub2
Arnold, M., Sierra, M. S., Laversanne, M., Soerjomataram, I., Jemal, A., and Bray, F. (2017). Global patterns and trends in colorectal cancer incidence and mortality. Gut 66, 683–691. doi:10.1136/gutjnl-2015-310912
Aronson, S. J., and Rehm, H. L. (2015). Building the foundation for genomics in precision medicine. Nature 526, 336–342. doi:10.1038/nature15816
Bai, H., Li, X., Li, H., Yang, J., and Ning, K. (2019). Biological ingredient complement chemical ingredient in the assessment of the quality of TCM preparations. Sci. Rep. 9, 5853. doi:10.1038/s41598-019-42341-4
Bai, H., Ning, K., and Wang, C. y. (2015). Biological ingredient analysis of traditional Chinese medicines utilizing metagenomic approach based on high-throughput-sequencing and big-data-mining. Acta Pharm. Sin. B 50, 272–277. doi:10.1038/srep05147
Besse, P., Da Silva, D., and Grisoni, M. (2021). Plant DNA barcoding principles and limits: A case study in the genus vanilla. Methods Mol. Biol. 2222, 131–148. doi:10.1007/978-1-0716-0997-2_8
Boezio, B., Audouze, K., Ducrot, P., and Taboureau, O. (2017). Network-based approaches in pharmacology. Mol. Inf. 36, 1700048. doi:10.1002/minf.201700048
Buriani, A., Garcia-Bermejo, M. L., Bosisio, E., Xu, Q., Li, H., Dong, X., et al. (2012). Omic techniques in systems biology approaches to traditional Chinese medicine research: Present and future. J. Ethnopharmacol. 140, 535–544. doi:10.1016/j.jep.2012.01.055
Cai, F.-F., Zhou, W. J., Wu, R., and Su, S. B. (2018). Systems biology approaches in the study of Chinese herbal formulae. Chin. Med. 13, 65. doi:10.1186/s13020-018-0221-x
Chai, P., Yu, J., Ge, S., Jia, R., and Fan, X. (2020). Genetic alteration, RNA expression, and DNA methylation profiling of coronavirus disease 2019 (COVID-19) receptor ACE2 in malignancies: A pan-cancer analysis. J. Hematol. Oncol. 13, 43. doi:10.1186/s13045-020-00883-5
Chandrasekara, C., Naranpanawa, D. N. U., Bandusekara, B. S., Pushpakumara, D. K. N. G., Wijesundera, D. S. A., and Bandaranayake, P. C. G. (2021). Universal barcoding regions, rbcL, matK and trnH-psbA do not discriminate Cinnamomum species in Sri Lanka. PLoS One 16, e0245592. doi:10.1371/journal.pone.0245592
Chasseaud, L. F. (1979). The role of glutathione and glutathione S-transferases in the metabolism of chemical carcinogens and other electrophilic agents. Adv. Cancer Res. 29, 175–274. doi:10.1016/s0065-230x(08)60848-9
Chen, C. Y. C. (2011). TCM Database@Taiwan: The world's largest traditional Chinese medicine database for drug screening in silico. PLoS One 6, e15939. doi:10.1371/journal.pone.0015939
Chen, F., Wen, Q., Jiang, J., Li, H. L., Tan, Y. F., Li, Y. H., et al. (2016). Could the gut microbiota reconcile the oral bioavailability conundrum of traditional herbs? J. Ethnopharmacol. 179, 253–264. doi:10.1016/j.jep.2015.12.031
Chen, J., Jiang, Z., Li, C., Ping, X., Cui, S., Tang, S., et al. (2015). Identification of ungulates used in a traditional Chinese medicine with DNA barcoding technology. Ecol. Evol. 5, 1818–1825. doi:10.1002/ece3.1457
Chen, S., Pang, X., Song, J., Shi, L., Yao, H., Han, J., et al. (2014). A renaissance in herbal medicine identification: From morphology to DNA. Biotechnol. Adv. 32, 1237–1244. doi:10.1016/j.biotechadv.2014.07.004
Chen, X., Wu, Y., Chen, C., Gu, Y., Zhu, C., Wang, S., et al. (2021). Identifying potential anti-COVID-19 pharmacological components of traditional Chinese medicine Lianhuaqingwen capsule based on human exposure and ACE2 biochromatography screening. Acta Pharm. Sin. B 11, 222–236. doi:10.1016/j.apsb.2020.10.002
Chen, S., Yao, H., Han, J., Liu, C., Song, J., Shi, L., et al. (2010). Validation of the ITS2 region as a novel DNA barcode for identifying medicinal plant species. PLoS One 5, e8613. doi:10.1371/journal.pone.0008613
Cheng, X., Su, X., Chen, X., Zhao, H., Bo, C., Xu, J., et al. (2014). Biological ingredient analysis of traditional Chinese medicine preparation based on high-throughput sequencing: The story for Liuwei Dihuang wan. Sci. Rep. 4, 5147. doi:10.1038/srep05147
Chou, S. T., Hsiang, C. Y., Lo, H. Y., Huang, H. F., Lai, M. T., Hsieh, C. L., et al. (2017). Exploration of anti-cancer effects and mechanisms of Zuo-Jin-Wan and its alkaloid components in vitro and in orthotopic HepG2 xenograft immunocompetent mice. BMC Complement. Altern. Med. 17, 121. doi:10.1186/s12906-017-1586-6
Coghlan, M. L., Haile, J., Houston, J., Murray, D. C., White, N. E., Moolhuijzen, P., et al. (2012). Deep sequencing of plant and animal DNA contained within traditional Chinese medicines reveals legality issues and health safety concerns. PLoS Genet. 8, e1002657. doi:10.1371/journal.pgen.1002657
Deng, Z. (2010). TCM dietotherapy for hypertension. J. Tradit. Chin. Med. 30, 235–236. doi:10.1016/s0254-6272(10)60048-9
Ding, Z., Zhong, R., Yang, Y., Xia, T., Wang, W., Wang, Y., et al. (2020). Systems pharmacology reveals the mechanism of activity of Ge-Gen-Qin-Lian decoction against LPS-induced acute lung injury: A novel strategy for exploring active components and effective mechanism of TCM formulae. Pharmacol. Res. 156, 104759. doi:10.1016/j.phrs.2020.104759
Dong, Y., Chen, Y. T., Yang, Y. X., Zhou, X. J., Dai, S. J., Tong, J. F., et al. (2016). Metabolomics study of type 2 diabetes mellitus and the AntiDiabetic effect of berberine in zucker diabetic fatty rats using uplc-ESI-hdms. Phytother. Res. 30, 823–828. doi:10.1002/ptr.5587
Dunning, L. T., and Savolainen, V. (2010). Broad-scale amplification of matK for DNA barcoding plants, a technical note. Bot. J. Linn. Soc. 164, 1–9. doi:10.1111/j.1095-8339.2010.01071.x
Fan, H. H., Wang, L. Q., Liu, W. L., An, X. P., Liu, Z. D., He, X. Q., et al. (2020). Repurposing of clinically approved drugs for treatment of coronavirus disease 2019 in a 2019-novel coronavirus-related coronavirus model. Chin. Med. J. 133, 1051–1056. doi:10.1097/cm9.0000000000000797
Fan, J., Bao, Y., Meng, X., Wang, S., Li, T., Chang, X., et al. (2017). Mechanism of modulation through PI3K-AKT pathway about Nepeta cataria L.'s extract in non-small cell lung cancer. Oncotarget 8, 31395–31405. doi:10.18632/oncotarget.15608
Fan, W., Zhou, J., Wu, Z., Tan, G., Li, H., Mei, Q., et al. (2021). Analysis of antioxidants in Chrysanthemum indici flos by online gradient extraction and HPLC-FRAP. Anal. Methods 13, 2283–2289. doi:10.1039/d1ay00548k
Fang, S., Dong, L., Liu, L., Guo, J., Zhao, L., Zhang, J., et al. (2021). Herb: A high-throughput experiment- and reference-guided database of traditional Chinese medicine. Nucleic Acids Res. 49, D1197–d1206. doi:10.1093/nar/gkaa1063
Fathy, S. A., Mohamed, M. R., Ali, M. A. M., El-Helaly, A. E., and Alattar, A. T. (2019). Influence of IL-6, IL-10, IFN-γ and TNF-α genetic variants on susceptibility to diabetic kidney disease in type 2 diabetes mellitus patients. Biomarkers 24, 43–55. doi:10.1080/1354750x.2018.1501761
Feng, R., Shou, J. W., Zhao, Z. X., He, C. Y., Ma, C., Huang, M., et al. (2015). Transforming berberine into its intestine-absorbable form by the gut microbiota. Sci. Rep. 5, 12155. doi:10.1038/srep12155
Feng, R., Zhao, Z. X., Ma, S. R., Guo, F., Wang, Y., and Jiang, J. D. (2018). Gut microbiota-regulated pharmacokinetics of berberine and active metabolites in beagle dogs after oral administration. Front. Pharmacol. 9, 214. doi:10.3389/fphar.2018.00214
Gan, W. Z., Ramachandran, V., Lim, C. S. Y., and Koh, R. Y. (2019). Omics-based biomarkers in the diagnosis of diabetes. J. Basic Clin. Physiol. Pharmacol. 31. doi:10.1515/jbcpp-2019-0120
Gao, L., Cao, M., Li, J. Q., Qin, X. M., and Fang, J. S. (2021). Traditional Chinese medicine network pharmacology in cardiovascular precision medicine. Curr. Pharm. Des. 27, 2925–2933. doi:10.2174/1381612826666201112142408
Gómez-López, G., Dopazo, J., Cigudosa, J. C., Valencia, A., and Al-Shahrour, F. (2019). Precision medicine needs pioneering clinical bioinformaticians. Brief. Bioinform. 20, 752–766. doi:10.1093/bib/bbx144
Gong, B., Kao, Y., Zhang, C., Zhao, H., Sun, F., and Gong, Z. (2019). Exploring the pharmacological mechanism of the herb pair "HuangLian-GanJiang" against colorectal cancer based on network pharmacology. Evid. Based. Complement. Altern. Med. 2019, 2735050. doi:10.1155/2019/2735050
Gong, X., Ji, M., Xu, J., Zhang, C., and Li, M. (2020). Hypoglycemic effects of bioactive ingredients from medicine food homology and medicinal health food species used in China. Crit. Rev. Food Sci. Nutr. 60, 2303–2326. doi:10.1080/10408398.2019.1634517
Group, C. P. W. (2009). A DNA barcode for land plants. Proc. Natl. Acad. Sci. U. S. A. 106, 12794–12797. doi:10.1073/pnas.0905845106
Guo, R., Luo, X., Liu, J., Liu, L., Wang, X., and Lu, H. (2020). Omics strategies decipher therapeutic discoveries of traditional Chinese medicine against different diseases at multiple layers molecular-level. Pharmacol. Res. 152, 104627. doi:10.1016/j.phrs.2020.104627
Habtemariam, S. (2020). Berberine pharmacology and the gut microbiota: A hidden therapeutic link. Pharmacol. Res. 155, 104722. doi:10.1016/j.phrs.2020.104722
He, D., Huang, J. H., Zhang, Z. Y., Du, Q., Peng, W. J., Yu, R., et al. (2019). A network pharmacology-based strategy for predicting active ingredients and potential targets of LiuWei DiHuang pill in treating type 2 diabetes mellitus. Drug Des. devel. Ther. 13, 3989–4005. doi:10.2147/DDDT.S216644
Heindel, J. J., Blumberg, B., Cave, M., Machtinger, R., Mantovani, A., Mendez, M. A., et al. (2017). Metabolism disrupting chemicals and metabolic disorders. Reprod. Toxicol. 68, 3–33. doi:10.1016/j.reprotox.2016.10.001
Heinisch, S., and Rocca, J. L. (2009). Sense and nonsense of high-temperature liquid chromatography. J. Chromatogr. A 1216, 642–658. doi:10.1016/j.chroma.2008.11.079
Hoffmann, M., Kleine-Weber, H., Schroeder, S., Kruger, N., Herrler, T., Erichsen, S., et al. (2020). SARS-CoV-2 cell entry depends on ACE2 and TMPRSS2 and is blocked by a clinically proven protease inhibitor. Cell. 181, 271–280. e278. doi:10.1016/j.cell.2020.02.052
Hou, Y., and Jiang, J. G. (2013). Origin and concept of medicine food homology and its application in modern functional foods. Food Funct. 4, 1727–1741. doi:10.1039/c3fo60295h
Hu, C., He, B., Gong, F., Liang, M., Zhao, D., and Zhang, G. (2022). The adverse reactions of Lianhua qingwen capsule/granule compared with conventional drug in clinical application: A meta-analysis. Front. Pharmacol. 13, 764774. doi:10.3389/fphar.2022.764774
Huang, L., Xie, D., Yu, Y., Liu, H., Shi, Y., Shi, T., et al. (2018). Tcmid 2.0: A comprehensive resource for TCM. Nucleic Acids Res. 46, D1117-D1120–d1120. doi:10.1093/nar/gkx1028
Huang, S., Zhang, Z., Li, W., Kong, F., Yi, P., Huang, J., et al. (2020). Network pharmacology-based prediction and verification of the active ingredients and potential targets of zuojinwan for treating colorectal cancer. Drug Des. devel. Ther. 14, 2725–2740. doi:10.2147/dddt.S250991
Jameson, J. L., and Longo, D. L. (2015). Precision medicine-personalized, problematic, and promising. N. Engl. J. Med. 372, 2229–2234. doi:10.1056/NEJMsb1503104
Jia, J., Xu, Z., Xin, T., Shi, L., and Song, J. (2017). Quality control of the traditional patent medicine yimu wan based on SMRT sequencing and DNA barcoding. Front. Plant Sci. 8, 926. doi:10.3389/fpls.2017.00926
Jiang, D., Li, J., Li, J., Wang, M., Han, C., Wang, X., et al. (2017). Combination of FGFR4 inhibitor Blu9931 and 5-fluorouracil effects on the biological characteristics of colorectal cancer cells. Int. J. Oncol. 51, 1611–1620. doi:10.3892/ijo.2017.4143
Jiang, W. Y. (2005). Therapeutic wisdom in traditional Chinese medicine: A perspective from modern science. Discov. Med. 5, 455–461.
Joseph, T. A., and Pe'er, I. (2021). An introduction to whole-metagenome shotgun sequencing studies. Methods Mol. Biol. 2243, 107–122. doi:10.1007/978-1-0716-1103-6_6
Kim, H. J., Jee, E. H., Ahn, K. S., Choi, H. S., and Jang, Y. P. (2010). Identification of marker compounds in herbal drugs on TLC with DART-MS. Arch. Pharm. Res. 33, 1355–1359. doi:10.1007/s12272-010-0909-7
Kim, S., Chen, J., Cheng, T., Gindulyte, A., He, J., He, S., et al. (2021). PubChem in 2021: New data content and improved web interfaces. Nucleic Acids Res. 49, D1388–d1395. doi:10.1093/nar/gkaa971
Kologrivova, I. V., Suslova, T. E., Koshel'skaya, O. A., Vinnitskaya, I. V., and Trubacheva, O. A. (2014). System of matrix metalloproteinases and cytokine secretion in type 2 diabetes mellitus and impaired carbohydrate tolerance associated with arterial hypertension. Bull. Exp. Biol. Med. 156, 635–638. doi:10.1007/s10517-014-2413-4
Kolter, A., and Gemeinholzer, B. (2021). Plant DNA barcoding necessitates marker-specific efforts to establish more comprehensive reference databases. Genome 64, 265–298. doi:10.1139/gen-2019-0198
Kuhn, M., Szklarczyk, D., Pletscher-Frankild, S., Blicher, T. H., von Mering, C., Jensen, L. J., et al. (2014). Stitch 4: Integration of protein-chemical interactions with user data. Nucleic Acids Res. 42, D401–D407. doi:10.1093/nar/gkt1207
Kuhn, M., von Mering, C., Campillos, M., Jensen, L. J., and Bork, P. (2008). Stitch: Interaction networks of chemicals and proteins. Nucleic Acids Res. 36, D684–D688. doi:10.1093/nar/gkm795
Lan, J., Zhao, Y., Dong, F., Yan, Z., Zheng, W., Fan, J., et al. (2015). Meta-analysis of the effect and safety of berberine in the treatment of type 2 diabetes mellitus, hyperlipemia and hypertension. J. Ethnopharmacol. 161, 69–81. doi:10.1016/j.jep.2014.09.049
Lehmann, B. D., Colaprico, A., Silva, T. C., Chen, J., Ban, Y., Huang, H., et al. (2021). Multi-omics analysis identifies therapeutic vulnerabilities in triple-negative breast cancer subtypes. Nat. Commun. 12, 6276. doi:10.1038/s41467-021-26502-6
Leung, E. L.-H., Pan, H. D., Huang, Y. F., Fan, X. X., Wang, W. Y., He, F., et al. (2020). The scientific foundation of Chinese herbal medicine against COVID-19. Engineering 6, 1099–1107. doi:10.1016/j.eng.2020.08.009
Li, Q., Sun, Y., Guo, H., Sang, F., Ma, H., Peng, H., et al. (2018). Quality control of the traditional Chinese medicine Ruyi jinhuang powder based on high-throughput sequencing and real-time PCR. Sci. Rep. 8, 8261. doi:10.1038/s41598-018-26520-3
Li, S., Fan, T. P., Jia, W., Lu, A., and Zhang, W. (2014). Network pharmacology in traditional Chinese medicine. Evid. Based. Complement. Altern. Med. 2014, 138460. doi:10.1155/2014/138460
Lin, X., Yi, Z., Diao, J., Shao, M., Zhao, L., Cai, H., et al. (2014). ShaoYao decoction ameliorates colitis-associated colorectal cancer by downregulating proinflammatory cytokines and promoting epithelial-mesenchymal transition. J. Transl. Med. 12, 105. doi:10.1186/1479-5876-12-105
Lin, Y.-J., Ho, T. J., Yeh, Y. C., Cheng, C. F., Shiao, Y. T., Wang, C. B., et al. (2015). Chinese herbal medicine treatment improves the overall survival rate of individuals with hypertension among type 2 diabetes patients and modulates in vitro smooth muscle cell contractility. PLoS One 10, e0145109. doi:10.1371/journal.pone.0145109
Lindqvist, L., and Pelletier, J. (2009). Inhibitors of translation initiation as cancer therapeutics. Future Med. Chem. 1, 1709–1722. doi:10.4155/fmc.09.122
Liu, C.-S., Zheng, Y. R., Zhang, Y. F., and Long, X. Y. (2016a). Research progress on berberine with a special focus on its oral bioavailability. Fitoterapia 109, 274–282. doi:10.1016/j.fitote.2016.02.001
Liu, G. D., Huang, W. Z., Ma, S. P., and Wang, Z. Z. (2016b). Fingerprint of dazhu hongjingtian capsule by HPLC-DAD-QTOF-MS. China J. Chin. Mater Med. 41, 3022–3026. doi:10.4268/cjcmm20161613
Liu, Y., Wang, B., Shu, S., Li, Z., Song, C., Liu, D., et al. (2021). Analysis of the Coptis chinensis genome reveals the diversification of protoberberine-type alkaloids. Nat. Commun. 12, 3276. doi:10.1038/s41467-021-23611-0
Liu, Y., Yang, S., Wang, K., Lu, J., Bao, X., Wang, R., et al. (2020). Cellular senescence and cancer: Focusing on traditional Chinese medicine and natural products. Cell. Prolif. 53, e12894. doi:10.1111/cpr.12894
Luo, K., Chen, S., Chen, K., Song, J., Yao, H., Ma, X., et al. (2010). Assessment of candidate plant DNA barcodes using the Rutaceae family. Sci. China. Life Sci. 53, 701–708. doi:10.1007/s11427-010-4009-1
Luo, T. T., Lu, Y., Yan, S. K., Xiao, X., Rong, X. L., and Guo, J. (2020). Network pharmacology in research of Chinese medicine formula: Methodology, application and prospective. Chin. J. Integr. Med. 26, 72–80. doi:10.1007/s11655-019-3064-0
Lv, X., Li, Y., Tang, C., Zhang, Y., Zhang, J., and Fan, G. (2016). Integration of HPLC-based fingerprint and quantitative analyses for differentiating botanical species and geographical growing origins of Rhizoma coptidis. Pharm. Biol. 54, 3264–3271. doi:10.1080/13880209.2016.1223699
Mendez, D., Gaulton, A., Bento, A. P., Chambers, J., De Veij, M., Felix, E., et al. (2019). ChEMBL: Towards direct deposition of bioassay data. Nucleic Acids Res. 47, D930-D940–d940. doi:10.1093/nar/gky1075
Miryala, S. K., Anbarasu, A., and Ramaiah, S. (2018). Discerning molecular interactions: A comprehensive review on biomolecular interaction databases and network analysis tools. Gene 642, 84–94. doi:10.1016/j.gene.2017.11.028
Newmaster, S. G., Fazekas, A., and Ragupathy, S. (2006). DNA barcoding in land plants: Evaluation of rbcL in a multigene tiered approach. Can. J. Bot. 84, 335–341. doi:10.1139/b06-047
Olivier, M., Asmis, R., Hawkins, G. A., Howard, T. D., and Cox, L. A. (2019). The need for multi-omics biomarker signatures in precision medicine. Int. J. Mol. Sci. 20, E4781. doi:10.3390/ijms20194781
Qiu, J. (2007). China plans to modernize traditional medicine. Nature 446, 590–591. doi:10.1038/446590a
Ren, J. L., Zhang, A. H., and Wang, X. J. (2020). Traditional Chinese medicine for COVID-19 treatment. Pharmacol. Res. 155, 104743. doi:10.1016/j.phrs.2020.104743
Rivera, D., Allkin, R., Obon, C., Alcaraz, F., Verpoorte, R., and Heinrich, M. (2014). What is in a name? The need for accurate scientific nomenclature for plants. J. Ethnopharmacol. 152, 393–402. doi:10.1016/j.jep.2013.12.022
Ru, J., Li, P., Wang, J., Zhou, W., Li, B., Huang, C., et al. (2014). Tcmsp: A database of systems pharmacology for drug discovery from herbal medicines. J. Cheminform. 6, 13. doi:10.1186/1758-2946-6-13
Runfeng, L., Yunlong, H., Jicheng, H., Weiqi, P., Qinhai, M., Yongxia, S., et al. (2020). Lianhuaqingwen exerts anti-viral and anti-inflammatory activity against novel coronavirus (SARS-CoV-2). Pharmacol. Res. 156, 104761. doi:10.1016/j.phrs.2020.104761
Senthilkumar, G. P., Anithalekshmi, M. S., Yasir, M., Parameswaran, S., Packirisamy, R. M., and Bobby, Z. (2018). Role of omentin 1 and IL-6 in type 2 diabetes mellitus patients with diabetic nephropathy. Diabetes Metab. Syndr. 12, 23–26. doi:10.1016/j.dsx.2017.08.005
Sheehan, D., Meade, G., Foley, V. M., and Dowd, C. A. (2001). Structure, function and evolution of glutathione transferases: Implications for classification of non-mammalian members of an ancient enzyme superfamily. Biochem. J. 360, 1–16. doi:10.1042/0264-6021:3600001
Slaine, P. D., Kleer, M., Smith, N. K., Khaperskyy, D. A., and McCormick, C. (2017). Stress granule-inducing eukaryotic translation initiation factor 4A inhibitors block influenza A virus replication. Viruses 9, E388. doi:10.3390/v9120388
Subramanian, I., Verma, S., Kumar, S., Jere, A., and Anamika, K. (2020). Multi-omics data integration, interpretation, and its application. Bioinform. Biol. Insights 14, 1177932219899051. doi:10.1177/1177932219899051
Sun, Q., He, M., Zhang, M., Zeng, S., Chen, L., Zhao, H., et al. (2021). Traditional Chinese medicine and colorectal cancer: Implications for drug discovery. Front. Pharmacol. 12, 685002. doi:10.3389/fphar.2021.685002
Tao, W., Xu, X., Wang, X., Li, B., Wang, Y., Li, Y., et al. (2013). Network pharmacology-based prediction of the active ingredients and potential targets of Chinese herbal Radix Curcumae formula for application to cardiovascular disease. J. Ethnopharmacol. 145, 1–10. doi:10.1016/j.jep.2012.09.051
Vences, M., Thomas, M., Bonett, R. M., and Vieites, D. R. (2005). Deciphering amphibian diversity through DNA barcoding: Chances and challenges. Philos. Trans. R. Soc. Lond. B Biol. Sci. 360, 1859–1868. doi:10.1098/rstb.2005.1717
Wang, B., Feng, X., Liu, S., Qiu, F., Lu, X., and Li, Z. (2021). Comprehensive quality assessment of Kaixin powder by HPLC-DAD quantification and HPLC-QTOF-MS/MS confirmation. ACS omega 6, 11319–11326. doi:10.1021/acsomega.1c00289
Wang, J., Wang, L., Lou, G. H., Zeng, H. R., Hu, J., Huang, Q. W., et al. (2019). Coptidis rhizoma: A comprehensive review of its traditional uses, botany, phytochemistry, pharmacology and toxicology. Pharm. Biol. 57, 193–225. doi:10.1080/13880209.2019.1577466
Wang, W.-L., Xu, S. Y., Ren, Z. G., Tao, L., Jiang, J. W., and Zheng, S. S. (2015). Application of metagenomics in the human gut microbiome. World J. Gastroenterol. 21, 803–814. doi:10.3748/wjg.v21.i3.803
Wang, W. J., and Zhang, T. (2017). Integration of traditional Chinese medicine and Western medicine in the era of precision medicine. J. Integr. Med. 15, 1–7. doi:10.1016/s2095-4964(17)60314-5
Wang, Y., Shi, Y., Zou, J., Zhang, X., Liang, Y., Tai, J., et al. (2020). Network pharmacology exploration reveals a common mechanism in the treatment of cardio-cerebrovascular disease with Salvia miltiorrhiza Burge. and Carthamus tinctorius L. BMC Complement. Med. Ther. 20, 351. doi:10.1186/s12906-020-03026-y
Wang, Y., Xiao, J., Suzek, T. O., Zhang, J., Wang, J., and Bryant, S. H. (2009). PubChem: A public information system for analyzing bioactivities of small molecules. Nucleic Acids Res. 37, W623–W633. doi:10.1093/nar/gkp456
Wishart, D. S., Feunang, Y. D., Guo, A. C., Lo, E. J., Marcu, A., Grant, J. R., et al. (2018). DrugBank 5.0: A major update to the DrugBank database for 2018. Nucleic Acids Res. 46, D1074-D1082–d1082. doi:10.1093/nar/gkx1037
Wishart, D. S., Knox, C., Guo, A. C., Shrivastava, S., Hassanali, M., Stothard, P., et al. (2006). DrugBank: A comprehensive resource for in silico drug discovery and exploration. Nucleic Acids Res. 34, D668–D672. doi:10.1093/nar/gkj067
Wolfender, J. L., Ndjoko, K., and Hostettmann, K. (2003). Liquid chromatography with ultraviolet absorbance-mass spectrometric detection and with nuclear magnetic resonance spectroscopy: A powerful combination for the on-line structural investigation of plant metabolites. J. Chromatogr. A 1000, 437–455. doi:10.1016/s0021-9673(03)00303-0
Wu, G. S., Li, H. K., and Zhang, W. D. (2019). Metabolomics and its application in the treatment of coronary heart disease with traditional Chinese medicine. Chin. J. Nat. Med. 17, 321–330. doi:10.1016/s1875-5364(19)30037-8
Wu, G., Zhao, J., Zhao, J., Song, N., Zheng, N., Zeng, Y., et al. (2021a). Exploring biological basis of Syndrome differentiation in coronary heart disease patients with two distinct Syndromes by integrated multi-omics and network pharmacology strategy. Chin. Med. 16, 109. doi:10.1186/s13020-021-00521-3
Wu, X., Li, W., Qin, Z., Xue, L., Huang, G., Luo, Z., et al. (2021b). Traditional Chinese medicine as an adjunctive therapy for mild and common COVID-19: A systematic review and network meta-analysis. Med. Baltim. 100, e27372. doi:10.1097/md.0000000000027372
Wu, Z., Lu, W., Yu, W., Wang, T., Li, W., Liu, G., et al. (2018). Quantitative and systems pharmacology 2. in silico polypharmacology of G protein-coupled receptor ligands via network-based approaches. Pharmacol. Res. 129, 400–413. doi:10.1016/j.phrs.2017.11.005
Xiang, Y., Guo, Z., Zhu, P., Chen, J., and Huang, Y. (2019). Traditional Chinese medicine as a cancer treatment: Modern perspectives of ancient but advanced science. Cancer Med. 8, 1958–1975. doi:10.1002/cam4.2108
Xiao, M., Tian, J., Zhou, Y., Xu, X., Min, X., Lv, Y., et al. (2020). Efficacy of huoxiang zhengqi dropping pills and Lianhua qingwen granules in treatment of COVID-19: A randomized controlled trial. Pharmacol. Res. 161, 105126. doi:10.1016/j.phrs.2020.105126
Xin, T., Su, C., Lin, Y., Wang, S., Xu, Z., and Song, J. (2018a). Precise species detection of traditional Chinese patent medicine by shotgun metagenomic sequencing. Phytomedicine 47, 40–47. doi:10.1016/j.phymed.2018.04.048
Xin, T., Xu, Z., Jia, J., Leon, C., Hu, S., Lin, Y., et al. (2018b). Biomonitoring for traditional herbal medicinal products using DNA metabarcoding and single molecule, real-time sequencing. Acta Pharm. Sin. B 8, 488–497. doi:10.1016/j.apsb.2017.10.001
Xin, T., Zhang, Y., Pu, X., Gao, R., Xu, Z., and Song, J. (2019). Trends in herbgenomics. Sci. China. Life Sci. 62, 288–308. doi:10.1007/s11427-018-9352-7
Xu, X., Xu, H., Shang, Y., Zhu, R., Hong, X., Song, Z., et al. (2021). Development of the general chapters of the Chinese Pharmacopoeia 2020 edition: A review. J. Pharm. Anal. 11, 398–404. doi:10.1016/j.jpha.2021.05.001
Xue, R., Fang, Z., Zhang, M., Yi, Z., Wen, C., and Shi, T. (2013). Tcmid: Traditional Chinese Medicine integrative database for herb molecular mechanism analysis. Nucleic Acids Res. 41, D1089–D1095. doi:10.1093/nar/gks1100
Yan, D., Zheng, G., Wang, C., Chen, Z., Mao, T., Gao, J., et al. (2022). Hit 2.0: An enhanced platform for herbal ingredients' targets. Nucleic Acids Res. 50, D1238–d1243. doi:10.1093/nar/gkab1011
Yang, M., Lao, L., Yang, M., and Lao, L. (2019). Emerging applications of metabolomics in traditional Chinese medicine treating hypertension: Biomarkers, pathways and MoreEmerging applications of metabolomics in traditional Chinese medicine treating hypertension: Biomarkers, pathways and more. Front. Pharmacol. 10, 158. doi:10.3389/fphar.2019.00158
Yang, P. S., Lang, J., Li, H., Lu, J., Lin, H., Tian, G., et al. (2022). TCM-suite: A comprehensive and holistic platform for traditional Chinese medicine component identification and network pharmacology analysis. iMeta e47. doi:10.1002/imt2.47
Yang, X., Han, S., Zhang, X., Sun, Y., Xing, Y., et al. (2019). The role of traditional Chinese medicine in the regulation of oxidative stress in treating coronary heart disease. Oxid. Med. Cell. Longev. 2019, 3231424. doi:10.1155/2019/3231424
Yao, Q., Zhu, X., Han, M., Chen, C., Li, W., Bai, H., et al. (2022). Decoding herbal materials of TCM preparations with the multi-barcode sequencing approach. Sci. Rep. 12, 5988. doi:10.1038/s41598-022-09979-z
Yao, Y., Chen, H., Yan, L., Wang, W., and Wang, D. (2020). Berberine alleviates type 2 diabetic symptoms by altering gut microbiota and reducing aromatic amino acids. Biomed. Pharmacother. 131, 110669. doi:10.1016/j.biopha.2020.110669
Ye, H., Ye, L., Kang, H., Zhang, D., Tao, L., Tang, K., et al. (2011). Hit: Linking herbal active ingredients to targets. Nucleic Acids Res. 39, D1055–D1059. doi:10.1093/nar/gkq1165
Yi, Y. D., and Chang, I. M. (2004). An overview of traditional Chinese herbal formulae and a proposal of a new code system for expressing the formula titles. Evid. Based. Complement. Altern. Med. 1, 125–132. doi:10.1093/ecam/neh019
Yuan, R., and Lin, Y. (2000). Traditional Chinese medicine: An approach to scientific proof and clinical validation. Pharmacol. Ther. 86, 191–198. doi:10.1016/s0163-7258(00)00039-5
Zeng, K. W., Jiang, Y., Wang, J., Ye, M., Li, J., Ai, X. N., et al. (2019). TCM chemical biology-emerging interdiscipline of "TCM chemistry" and "biology. China J. Chin. Mater Med. 44, 849–860. doi:10.19540/j.cnki.cjcmm.20190222.011
Zeng, X., Zheng, Y., Liu, Y., and Su, W. (2021). Chemical composition, quality control, pharmacokinetics, pharmacological properties and clinical applications of fufang danshen tablet: A systematic review. J. Ethnopharmacol. 278, 114310. doi:10.1016/j.jep.2021.114310
Zhang, H., Wei, J., Xue, R., Wu, J. D., Zhao, W., Wang, Z. Z., et al. (2010). Berberine lowers blood glucose in type 2 diabetes mellitus patients through increasing insulin receptor expression. Metabolism. 59, 285–292. doi:10.1016/j.metabol.2009.07.029
Zhang, P., Liu, C., Zheng, X., Wu, L., Liu, Z., Liao, B., et al. (2019a). Full-length multi-barcoding: DNA barcoding from single ingredient to complex mixtures. Genes. 10, E343. doi:10.3390/genes10050343
Zhang, R., Gao, X., Bai, H., and Ning, K. (2020a). Traditional Chinese medicine and gut microbiome: Their respective and concert effects on healthcare. Front. Pharmacol. 11, 538. doi:10.3389/fphar.2020.00538
Zhang, R., Zhu, X., Bai, H., and Ning, K. (2019b). Network pharmacology databases for traditional Chinese medicine: Review and assessment. Front. Pharmacol. 10, 123. doi:10.3389/fphar.2019.00123
Zhang, R. Z., Yu, S. J., Bai, H., and Ning, K. (2017). TCM-Mesh: The database and analytical system for network pharmacology analysis for TCM preparations. Sci. Rep. 7, 2821. doi:10.1038/s41598-017-03039-7
Zhang, Y., Gu, Y., Ren, H., Wang, S., Zhong, H., Zhao, X., et al. (2020b). Gut microbiome-related effects of berberine and probiotics on type 2 diabetes (the PREMOTE study). Nat. Commun. 11, 5015. doi:10.1038/s41467-020-18414-8
Zhu, K. Y., Fu, Q., Xie, H. Q., Xu, S. L., Cheung, A. W. H., Zheng, K. Y. Z., et al. (2010). Quality assessment of a formulated Chinese herbal decoction, kaixinsan, by using rapid resolution liquid chromatography coupled with mass spectrometry: A chemical evaluation of different historical formulae. J. Sep. Sci. 33, 3666–3674. doi:10.1002/jssc.201000498
Keywords: traditional Chinese medicine, multi-omics approaches, quality control, pharmacodynamic effects, network pharmacology analysis, TCM databases
Citation: Zhu X, Yao Q, Yang P, Zhao D, Yang R, Bai H and Ning K (2022) Multi-omics approaches for in-depth understanding of therapeutic mechanism for Traditional Chinese Medicine. Front. Pharmacol. 13:1031051. doi: 10.3389/fphar.2022.1031051
Received: 29 August 2022; Accepted: 15 November 2022;
Published: 25 November 2022.
Edited by:
Yiider Tseng, Shandong University of Traditional Chinese Medicine, ChinaReviewed by:
Xiaofei Zhang, Shaanxi University of Chinese Medicine, ChinaHua Yang, China Pharmaceutical University, China
Copyright © 2022 Zhu, Yao, Yang, Zhao, Yang, Bai and Ning. This is an open-access article distributed under the terms of the Creative Commons Attribution License (CC BY). The use, distribution or reproduction in other forums is permitted, provided the original author(s) and the copyright owner(s) are credited and that the original publication in this journal is cited, in accordance with accepted academic practice. No use, distribution or reproduction is permitted which does not comply with these terms.
*Correspondence: Ronghua Yang, eXJoOTYxODNAMTYzLmNvbQ==; Hong Bai, YmFpaG9uZ0BodXN0LmVkdS5jbg==; Kang Ning, bmluZ2thbmdAaHVzdC5lZHUuY24=
†These authors have contributed equally to this work