- 1Key Laboratory of Prevention and Treatment of Cardiovascular and Cerebrovascular Diseases of Ministry of Education, Gannan Medical University, Ganzhou, China
- 2Department of Pediatric Surgery, First Affiliated Hospital of Gannan Medical University, Ganzhou, China
- 3State Key Laboratory of Marine Environmental Science, Institute of Marine Microbes and Ecospheres, Xiamen University, Xiamen, China
- 4College of Ocean and Earth Sciences, Xiamen University, Xiamen, China
- 5School of Basic Medical Sciences, Gannan Medical University, Ganzhou, China
In recent years, the Alzheimer’s disease (AD) epidemic has become one of the largest global healthcare crises. Besides, the available systemic therapies for AD are still inadequate. Due to the insufficient therapeutic options, new treatment strategies are urgently needed to achieve a satisfactory therapeutic effect. Marine bio-resources have been accepted as one of the most economically viable and sustainable sources with potential applications for drug discovery and development. In this study, a marine cyanobacteria–Synechococcus sp. XM-24 was selected as the object of research, to systematically investigate its therapeutic potential mechanisms for AD. The major active compounds derived from the Synechococcus sp. biomass were identified via pyrolysis-gas chromatography-mass spectrometry (GC-MS), and 22 compounds were identified in this strain. The most abundant chemical compounds was (E)-octadec-11-enoic acid, with the peak area of 30.6%. Follow by tridecanoic acid, 12-methyl- and hexadecanoic acid, with a peak area of 23.26% and 18.23%, respectively. GC-MS analysis also identified indolizine, isoquinoline, 3,4-dihydro- and Phthalazine, 1-methyl-, as well as alkene and alkane from the strain. After the chemical toxicity test, 10 compounds were finally collected to do the further analysis. Then, network pharmacology and molecular docking were adopted to systematically study the potential anti-AD mechanism of these compounds. Based on the analysis, the 10 Synechococcus-derived active compounds could interact with 128 related anti-AD targets. Among them, epidermal growth factor receptor (EGFR), vascular endothelial growth factor A (VEGFA) and mitogen-activated protein kinase 3 (MAPK3) were the major targets. Furthermore, the compounds N-capric acid isopropyl ester, (E)-octadec-11-enoic acid, and 2H-Pyran-2,4(3H)-dione, dihydro-6-methyl- obtained higher degrees in the compounds-intersection targets network analysis, indicating these compounds may play more important role in the process of anti-AD. In addition, Kyoto Encyclopedia of Genes and Genomes (KEGG) enrichment analysis showed that these active compounds exert the anti-AD effects mainly through PI3K-Akt signaling pathway, neuroactive ligand-receptor interaction and ras signaling pathway. Our study identified Synechococcus-derived bioactive compounds have the potential for application to AD by targeting multiple targets and related pathways, which will provide a foundation for future research on applications of marine cyanobacteria in the functional drug industry.
1 Introduction
Recently, cyanobacteria (blue-green algae) have been accepted as one of the economic and viable sources with potential applications in various fields, including food, nutraceutical, therapeutics, pharmaceutical, cosmetics and personal care industries (Bourgade and Stensjö, 2022; Pathania et al., 2022; Bishoyi et al., 2023). They are an extremely diverse group of photosynthetic prokaryotes, owing to their adaptive capacity to tolerate extreme conditions, cyanobacteria are found in almost all the habitats of the Earth, from desert crusts to open ocean (Ferris et al., 1996; Nubel et al., 1999; Garcia-Pichel and Pringault, 2001; Gaysina et al., 2019). A variety of natural ingredients are reported to be found in cyanobacteria, including vitamins, proteins, polysaccharides, lipids, sterols, enzymes as well as other fine chemicals (Dahms et al., 2006; Abed et al., 2009; de Jesus Raposo et al., 2013; Hassan et al., 2022). The metabolic versatility of cyanobacteria lends them to bioengineering applications for sustainable production of various products (Stal, 2007; Xie et al., 2017; Momper et al., 2019; Liu et al., 2021). Especially, cyanobacteria derived bioactive compounds have a development potential in scientific research and great opportunity for drug discovery (Vijayakumar and Menakha, 2015; Sahoo et al., 2021). For example, apratoxin D isolated from cyanobacterium Lyngbya sp. has exhibited powerful cytotoxicity to human lung cancer cells (Gutierrez et al., 2008). Additionally, Symplocamide A, produced by marine cyanobacterium Symploca sp. has shown strong cytotoxicity to neuroblastoma as well as lung cancer cells (Linington et al., 2008). Cyanobacteria are also reported to be a rich source of omega-3 fatty acids, including eicosapentaenoic acid (EPA) and docosahexaenoic acid (DHA), which have the potential to prevent inflammatory and cardiovascular diseases (Sijtsma and de Swaaf, 2004; Udayan et al., 2017; Priyadarsini et al., 2022). Furthermore, cyanobacteria derived compounds could not only be applied directly as drugs, but also be used as potential lead compounds for new drug discovery (Tan L. T. 2007). For example, as potential anticancer drugs, curacin A from cyanobacteria Lyngbya majuscule and dolastatin 10 from cyanobacteria Symploca sp., have been in preclinical and entered into clinical trials, respectively. Moreover, these components also served as drugs leading to the discovery of synthetic analogues, such as TZT-1027, compound 4, LU-103793, and ILX-651, usually holding improved pharmacological and pharmacokinetic properties for the treatment of multiple types of cancers (Uzair et al., 2012). Furthermore, cyanobacteria could also applied in the field of nanotechnology (Mandhata et al., 2022, Bishoyi et al., 2023). Sahoo et al. synthesized silver-nanoparticles (AgNPs) with cyanobacterium Nostoc sphaeroides and explored their antibacterial activity against bacterial strains that could lead urinary tract infection (Sahoo et al., 2021).
In the ocean, most species of picoplanktonic cyanobacteria currently known belong to two genera: Prochlorococcus and Synechococcus (Palenik et al., 2003). They are the most abundant photosynthetic organisms on Earth, numerically dominating most oceanic waters (Scanlan et al., 2009). Compared to Prochlorococcus, Synechococcus has a lower abundant in very oligotrophic waters, but has a broader global distribution, ranging from polar through temperate to tropical environments (Flombaum et al., 2013). For example, in some coastal areas, they could account for 20% of the local primary productivity (Li, 1994; Tai and Palenik, 2009). Due to the great deal of genetic and metabolic versatility within the Synechococcus sp., with strains probably adapted to complex and specific ecological environments (Scanlan et al., 2009; Bourgade and Stensjö, 2022). Besides, due to better adaptation to eutrophic environment, marine Synechococcus sp. is relatively easy to culture in the laboratory (Ahlgren and Rocap, 2006). Compared to Prochlorococcus, Synechococcus require less care and exhibit a faster growth rate, making them great candidates as bioproducers (Partensky et al., 1999; Yu J. et al., 2015; Ungerer et al., 2018). It has been reported that marine Synechococcus sp. contain multiple biologically active compounds, which include antibacterial, antifungal, antiviral, and cancer-fighting compounds (Martins et al., 2008; Costa et al., 2013; do Amaral et al., 2020; Srimongkol et al., 2023). Numbers of studies have indicated that they also have antioxidant and anti-inflammatory potential (de Morais et al., 2015; Gómez et al., 2023). For instance, Phycocyanin is a pigment found in blue-green algae, such as Synechococcus sp. (Hsieh-Lo et al., 2019). It has reported has an antioxidant activity and anti-inflammatory properties that works through the inhibition of histamine release (Romay et al., 1998; Bhat and Madyastha, 2001; Tan H. T. et al., 2022). As is well known, oxidative stress and chronic inflammation plays an important role in the progression of neurodegenerative diseases, such as Parkinson’s disease (PD), Alzheimer’s disease (AD), and multiple sclerosis (MS) (Pangestuti and Kim, 2011). As the various biologically potential of Synechococcus-derived active compounds, such as antioxidant and anti-inflammatory, do they also have anti-AD potential? At present, the knowledge on the anti-AD potential of the substances derived from marine Synechococcus sp. is still scarce, as well as the pharmacological mechanism.
AD, one of the major cause of dementia, is a degenerative brain disease characterized by the aggregates of extracellular β-amyloid (Aβ) plaques and intracellular tau protein, leading to neurofibrillary tangles, neuronal loss and cell death (Parihar and Hemnani, 2004; Citron, 2010). AD derived dementia is characterized by impairment in memory, language, problem-solving and other cognitive domains that affect a person’s ability to perform daily life activities (Kim K. et al., 2020). Until now, Acetylcholinesterase inhibitors (AChEIs) are remain the primary therapy for AD patients with mild-to-moderate cognitive impairment (Yu B. et al., 2022). However, these drugs do not have significant disease-modifying effects, but only provide symptomatic relief (Yeo-Teh and Tang, 2023). Hence, an efficient strategy is urgently needed for AD therapy, and a novel strategy is needed for anti-AD drugs discovery. Due to the diversity of species and metabolism, as well as unique advantages such as efficient photosynthesis and carbon sequestration, active compounds derived from microalgae provide us with new directions (Chen et al., 2022, Pathaniaet al. 2022). For example, a newly developed anti-AD drug‒sodium oligomannurarate, also called GV-971, was derived from marine algae, which can eventually accelerate Aβ clearance (Wang X. et al., 2019). Recently, network pharmacology strategy has proven to be powerful in explaining the molecular mechanisms of natural medicine, such as traditional Chinese medicine and marine natural products, which has complicated ingredients and multiple targets (Hopkins, 2007; Wang S. et al., 2022; Tikhonova et al., 2023). In addition, it could also serve as the basis for the novel drugs discovery. For example, Zeng et al. had used network pharmacology approach to uncover the key ingredients in Ginkgo Folium, as well as their anti-AD mechanisms (Zeng et al., 2021a).
In this study, we identified the organic compounds derived from marine cyanobacteria Synechococcus sp. XM-24 strain via pyrolysis-gas chromatography-mass spectrometry (GC-MS). Then, we evaluated the anti-AD potential of these compounds by applying network pharmacology strategy and molecular docking analysis. The study flow chart is shown in Figure 1. It is hoped that our study will allow further insights into the exploration of active compounds derived from marine cyanobacteria.
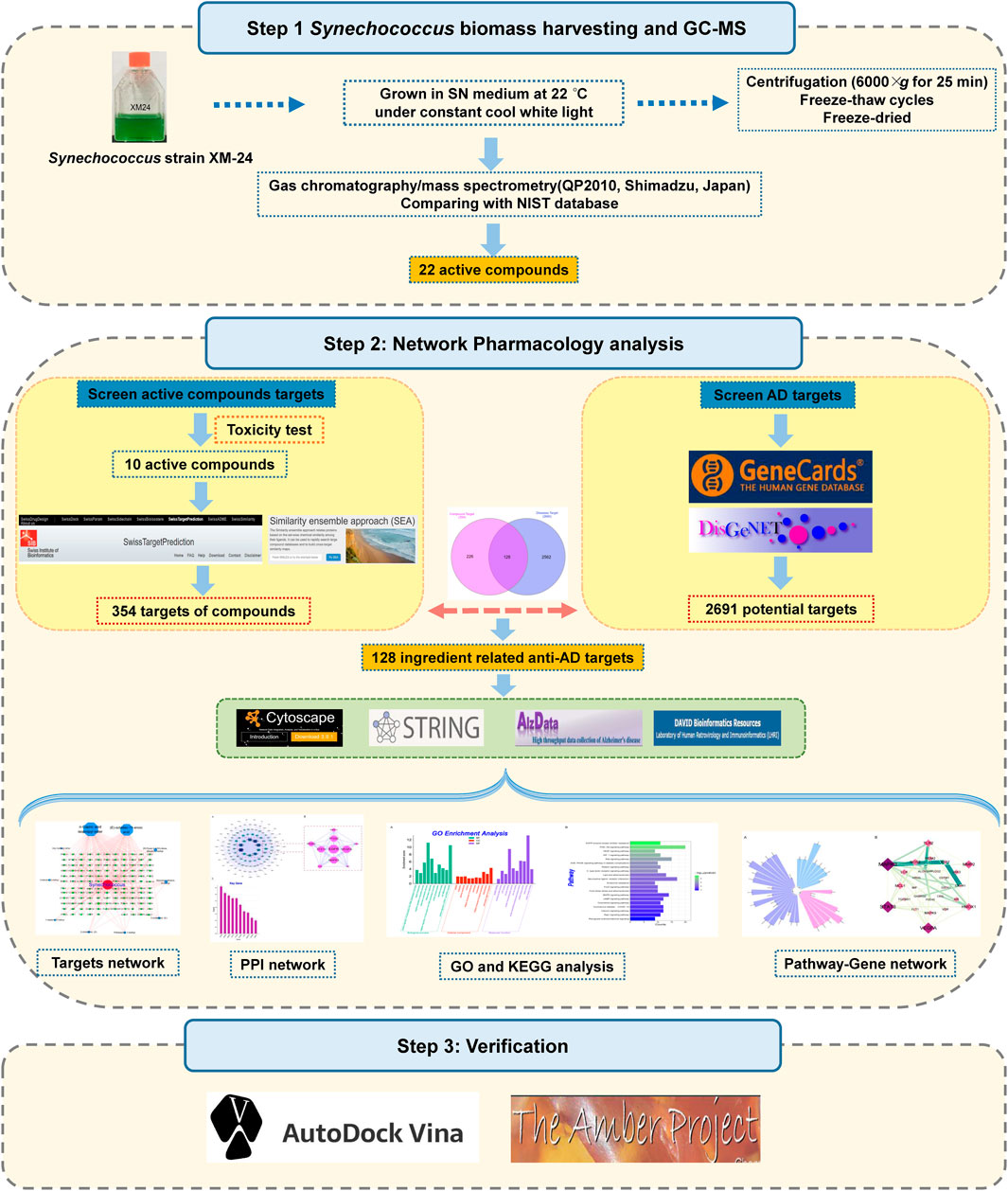
FIGURE 1. Flow chart of a network pharmacology-based strategy to investigate marine Synechococcus sp. derived compounds for application to Alzheimer’s disease.
2 Materials and methods
2.1 Synechococcus culture and biomass harvesting
Marine Synechococcus sp. XM-24 was used in this study. Synechococcus sp. XM-24 was isolated from the coastal region near Xiamen Island, China, and belonged to clade CB5 in subcluster 5.2 (Zheng Q. et al., 2018). The strain were grown in the SN medium at 22°C under constant cool white light. The light intensity was 20–30 μE m−2 s−1. Synechococcus cells were collected via centrifugation (6000×g for 25 min) at the exponential phase (∼15 days). To harvest Synechococcus-derived active compounds, the Synechococcus sp. XM-24 biomass was distributed in falcon-tube, and cells were subjected to freeze‒thaw cycles, then freeze‒dried, and kept frozen (–20°C) until further processing.
2.2 GC‒MS analysis
The pyrolysis-gas chromatography-mass spectrometry (GC‒MS) was applied to analysis the chemical composition of Synechococcus sp. XM-24 biomass as described by Yao Zhang et al. (Zhang et al., 2016). Synechococcus sp. cells were moved into a quartz tube. Then, the biomass were moistened with tetramethylammonium hydroxide solution (100μL, 25% in methanol). The sample was pyrolyzed using a single point pyrolyzer (Frontier Laboratories Ltd., Japan) at 550°C, interfaced directly to a GC‒MS (QP 2010, Shimadzu, Japan). About the stability of the temperature, the room temperature changed by 1°C, the change of the in-cylinder temperature was less than 0.01°C. The products were automatically injected into the gas chromatograph. Helium (99.999%) was used as a carrier gas. The flow rate was 1.8 ml min−1. The column temperature was set at 40°C for 3 min, and increased to 300°C at a rate of 10°C min−1 for 15 min. The mass spectrometer was operated at an ionization energy of 70 eV (EI). The mass range for detection was 25–500 m/z with a cycle time of 1s. Then, National Institute of Standards and Technology Mass Spectral Library database (NIST) was used for compound identification by comparing the obtained mass spectra with the database.
2.3 Potential targets of Synechococcus-derived active compounds
The chemical toxicity test was performed through PubChem databases (https://pubchem.ncbi.nlm.nih.gov/search/search.cgi), and all toxic compounds were eliminated. Then, the Swiss Target Prediction (https://www.swisstargetprediction.ch/) (Daina et al., 2019) and Search Server (SEA) (https://sea.bkslab.org/) (Keiser et al., 2007) were used to identify the potential targets of all these Synechococcus-derived active compounds.
2.4 Potential targets database of Anti-AD
GeneCards (https://www.genecards.org/) (Rebhan et al., 1998) and DisGeNET (https://www.disgenet.org/) (Pinero et al., 2017) were employed to screen potential genes, by setting “Alzheimer’s disease” and “anti-Alzheimer’s disease” as the keyword. Then, after further processing and removing the duplicate targets, the final targets related to anti-AD were collected. In addition, both of collected compound targets and anti-AD targets were imported into the InteractiVenn online platform (http://www.interactivenn.net/) to draw a Venn graph. Venn diagrams were employed to determine the intersection targets between Synechococcus-derived active compounds and anti-AD.
2.5 Active compound‒intersection target network construction
Cytoscape software was used to build a visualization network graph of active compound-intersection targets. Firstly, the obtained main active compounds and the intersection targets were imported into the Cytoscape 3.9.1 software. A visualization network diagram was generated from this software. Then, the Network Analyer plugin in Cytoscape 3.9.1 was used to analyze the topology parameters of this network. Based on degree, betweenness, and closeness, hub nodes were identified (Yu B. et al., 2022).
2.6 Protein-protein interaction network construction
The STRING database (https://string-db.org/) was used to construct a protein–protein interaction (PPI) network for the activities of Synechococcus-derived active compounds against AD. In this study, PPI can help us understand the functions and relationships of key target proteins (Athanasios et al., 2017). The STRING database can collect and integrate data about known and predicted protein‒protein associations, including both direct and indirect interactions, from many organisms (Szklarczyk et al., 2017). On STRING website, the main targets were imported into the “Multiple proteins” option, and “Homo sapiens” was selected in the organism query. And other parameters was established as the “default” criterion. Then, the visualized PPI network map was established using Cytoscape software (Shannon et al., 2003).
2.7 GO functional analysis and KEGG enrichment pathway analysis
To further explore the pathways of the anti-AD, the intersection targets were entered in the DAVID database (https://david.ncifcrf.gov/) (Huang da et al., 2009). Gene Ontology (GO) enrichment analysis is composed of biological process (BP), cellular component (CC), and molecular function (MF). Kyoto Encyclopedia of Genes and Genomes (KEGG) is a knowledge database which could be used to describe the interaction between different molecules and reveal known metabolic pathways (Chen J. et al., 2015). These two analysis helped to figure out the function of the core targets and the critical pathways of Synechococcus-derived active compounds against AD. The intersection targets data were imported into the DAVID database, and GO and KEGG analyses were performed on these intersection targets. Additionally, KEGG pathway enrichment analysis was also performed using ClueGO plug-in in Cytoscape software.
2.8 Bioinformatic analysis of intersection targets correlated with Aβ and Tau pathology
To study the pathological correlation between Synechococcus-derived ingredients with Aβ and tau in the treatment of AD, the intersection targets were imported into the AlzData database (http://www.alzdata.org/index.html) to perform the correlation analysis. The results were visualized through the Hiplot online platform (https://hiplot.com.cn/cloud-tool/drawing-tool/list). For convenience observation, the analysis were magnified by 100 times. The targets related to Aβ, tau and both of Aβ and tau were constructed PPI network using STRING database and Cytoscape software, to identify the core targets most correlated with Aβ and tau.
2.9 Molecular docking
The AutoDock software Vina was used to molecular docking simulation, revealing the binding modes and the strength between Synechococcus-derived active compounds and their protein targets (Morris and Lim-Wilby, 2008). The two-dimensional structures of the main active compounds of Synechococcus sp. XM-24 were collected through PubChem database. The 3D coordinates of KDR (PDB ID, 5EW3; resolution, 2.5 Å) and BIRC5 (PDB ID, 4AOI; resolution, 1.9 Å) were downloaded from the Protein Data Bank (http://www.rcsb.org/pdb/home/home.do) (Burley et al., 2021), and the chemical structures of the main active compounds were obtained from the TCMSP platform. The active pockets in the 3D structure of the proteins were identified by AutoDock Vina Tools, after removing the water molecules and small molecule ligands water molecules from the protein, and adding hydrogen. Then, using the prepared protein models and ligand structures, molecular docking was conducted through the AutoDock Vina software. The lower the score of the docking results, the stronger the binding ability. The molecular docking results were displayed in two-dimensional (2D) and three-dimensional (3D) graphics.
2.10 Molecular dynamics simulation
Proteins and compounds were parameterized using AMBER ff19SB and GAFF force fields, respectively (Wang et al., 2006; Tian et al., 2020). The system was solvated using an OPC water model and a truncated octahedral water box with a cutoff of 10 Å. Periodic boundary conditions (PBCs) were applied, and the system was neutralized. Non-bonded van der Waals interactions were calculated using the Lennard‒Jones 12–6 potential with a cutoff of 10 Å, while long-range electrostatic forces were treated using the PME algorithm. The SHAKE algorithm was used to constrain covalent bonds involving hydrogen atoms (Ryckaert et al., 1977). To eliminate steric clashes, heavy atoms were initially subjected to a constraint force of 10 kcal/(mol·Å) and underwent energy minimization using a combination of 2,500 steps of steepest descent and 2,500 steps of conjugate gradients. The system was then relaxed through 10,000 steps of steepest descent and 10,000 steps of conjugate gradient energy minimization. Subsequently, a 20 ps NVT simulation was performed to gradually heat the system to 300 K. This was followed by two equilibration phases: a 200 ps restrained heavy atoms NPT simulation and a 500 ps unrestrained NVT simulation. The temperature was controlled at 300 K using a Berendsen thermostat, and the pressure was maintained at 1 atm using a Monte Carlo barostat with coupling constants and relaxation times set as 1 ps. Finally, a 100 ns NVT simulation was carried out with a time step of 2 fs. RMSD and RMSF analyses were conducted using the CPPTRAJ module (Roe and Cheatham, 2013).
3 Results
3.1 GC-MS analysis
GC-MS analysis identified twenty-two chemical compounds from Synechococcus sp. XM-24 biomass. The identification of Synechococcus sp derived compounds is based on the mass spectra, including the retention time and peak area, and comparing with NIST database. All substances information are shown in Supplementary Figure S1 and Supplementary Table S1 in the Supplementary Material. The major types of compounds are fatty acids, alkene, alkane and esters. The most abundant chemical compound from Synechococcus sp. XM-24 was (E)-octadec-11-enoic acid, with the peak area of 30.6%. The second most abundant compound was tridecanoic acid, 12-methyl-, with a peak area of 23.26%. Follow by hexadecanoic acid, with a peak area of 18.23%. These three compounds together accounted for 72.09% of the peak area of the GC-MS. GC-MS analysis also identified indolizine, isoquinoline, 3,4-dihydro- and phthalazine, 1-methyl-, from the strain XM-24. In contrast, their proportions were not very high.
3.2 Potential targets of the main compounds derived from Synechococcus sp
We performed a chemical toxicity test on the twenty-two compounds though PubChem databases. The result showed that some components have cell toxicity and neurotoxicity, such as isoquinoline, 3,4-dihydro-, benzenepropionitrile, indolizin and so on. We did not do further analysis of these compounds in this study. Finally, ten compounds were collected as candidates to do the further analysis (Table 1). Then, by searching multiple online databases, including Swiss Target Prediction and SEA, 354 potential targets of the ten compounds were identified.
3.3 Anti-AD targets of main compounds derived from Synechococcus sp
A total of 2,297 and 853 potential anti-AD targets were obtained by searching the GeneCards and DisGeNET databases, respectively. After removing duplicate targets, 2,691 potential anti-AD targets were finally collected. By employing the InteractiVenn online platform, the 354 predicted targets of the Synechococcus-derived active compounds and the 2,691 AD-related disease targets were used to construct the Venn diagram (Figure 2). The results showed that a total of 218 intersection targets of active compounds with potential anti-AD effects were obtained. These intersection targets were used to do the subsequent analysis.
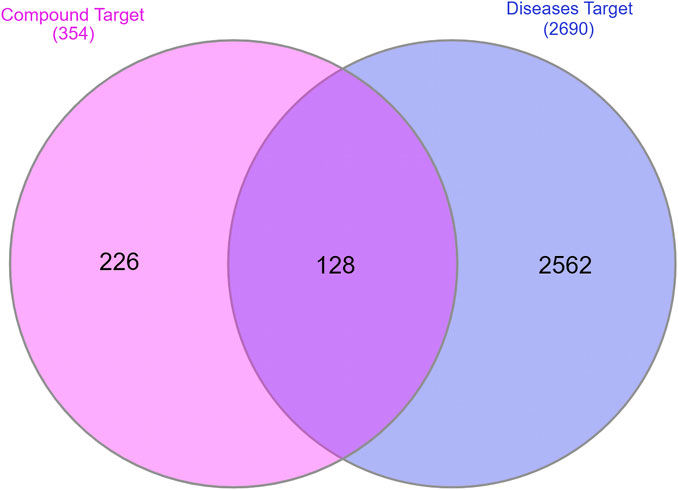
FIGURE 2. The intersecting targets between potential and AD-related targets. Pink: potential targets of active compounds; blue: targets of AD; purple: intersecting targets.
3.4 Active compound-intersection target network
The network analysis results are shown in Figure 3. Finally, 10 Synechococcus-derived active compounds and 218 potential anti-AD targets were imported into the Cytoscape software for construction of visual network. network analysis revealed 139 nodes with 304 edges, with an average node degree of 4.374. Nodes represented the different active compounds and intersection targets. The connections between nodes represent the interactions between active compounds and their targets. As shown in Figure 3, most the active compounds could affected multiple targets, particularly compounds N-capric acid isopropyl ester and (E)-octadec-11-enoic acid. Moreover, the larger the node and the higher the degree value indicated more importance. In our study, compounds N-capric acid isopropyl ester (degree = 74), (E)-octadec-11-enoic acid (degree = 27), 2H-pyran-2,4(3H)-dione, and dihydro-6-methyl-(degree = 9) had larger nodes, indicating that they may play more important roles in the anti-AD process.
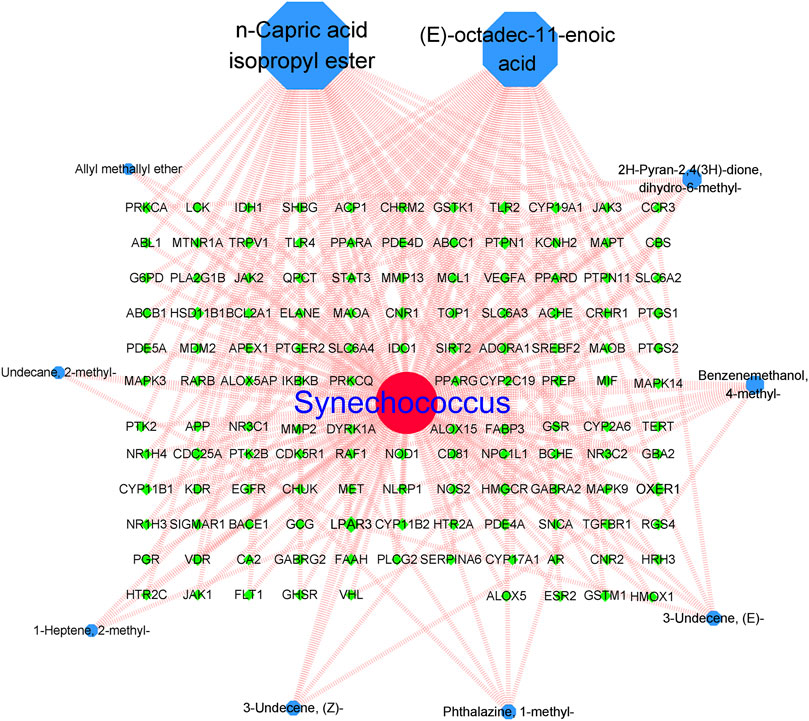
FIGURE 3. Active compounds-intersecting targets network. Blue: active compound nodes; green: targets of AD.
3.5 PPI results
In this part of analysis, 218 intersection targets were imported into the STRING database. The exported data was in TSV format. After hiding the unrelated nodes, the data were imported into the Cytoscape software to bulid the PPI network. The visualized PPI results are shown in Figure 4. The PPI network contained 217 nodes and 853 edges, and the average degree of nodes was 13.433. In Figure 4A, the closer to the center of the concentric circles, the larger the nodes, and the darker the color. It also indicated that the closer to the center of the concentric circles, the more important the nodes. Taking the degree as the screening condition, the higher the degree was the more important of the targets in anti-AD process, as shown in Figures 4B, C. In the figure, epidermal growth factor receptor (EGFR), vascular endothelial growth factor A (VEGFA), mitogen-activated protein kinase 3 (MAPK3), signal transducer and activator of transcription 3 (STAT3) and prostaglandin-endoperoxide synthase 2 (PTGS2) were ranked high in degree value, indicating that they may play an important role in the process of anti-AD.
3.6 GO and KEGG enrichment pathway analysis
In GO analysis, 288 GO enrichment entries were obtained, including 191 BP entries, 41 CC entries, 56 MF entries. In KEGG analysis, 86 KEGG signaling pathways were obtained. The results are shown in Figure 5. Figure 5A showed the GO analysis. For BP terms, the major enriched GO terms were positive regulation of transcription from the RNA polymerase II promoter (GO:0045944, count = 30), signal transduction (GO:0007165, count = 24), negative regulation of the apoptotic process (GO:0043066, count = 20), response to xenobiotic stimulus (GO:0009410, count = 19), and inflammatory response (GO:0006954, count = 19). The CC terms were cytosol (GO:0005829, count = 68), cytoplasm (GO:0005737, count = 67), plasma membrane (GO:0005886, count = 66), nucleus (GO:0005634, count = 54), and integral component of membrane (GO:0016021, count = 49). In MF, genes were mainly enriched in protein binding (GO:0005515, count = 109), identical protein binding (GO:0042802, count = 41), ATP binding (GO:0005524, count = 28), enzyme binding (GO:0019899, count = 25), and protein homodimerization activity (GO:0042803, count = 21). As shown in Figure 6B, the KEGG enrichment analysis revealed that the PI3K-Akt signaling pathway (hsa04151, count = 20), neuroactive ligand-receptor interaction (hsa04080, count = 17) and ras signaling pathway (hsa04014, count = 15), MAPK signaling pathway (hsa04010, count = 14), and Lipid and atherosclerosis (hsa05417, count = 13) were top-ranked, indicating that these pathways play a significant role in the anti-AD process.
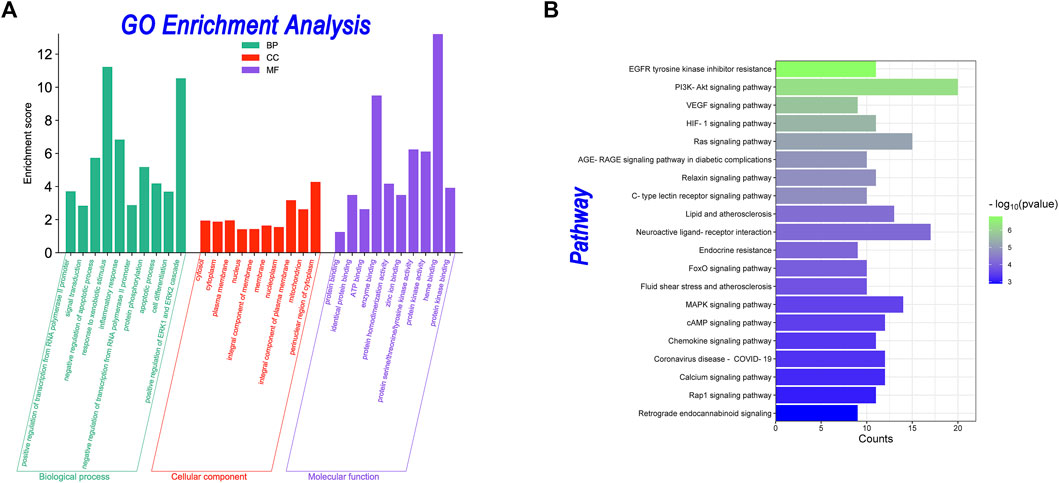
FIGURE 5. GO enrichment and KEGG pathway analyses. (A) Bar plot of GO enrichment analyses. The x-axis indicates the “biological process” in the GO. The y-axis indicates the enrichment score about these terms. (B) Bar plot of KEGG pathway enrichment analyses. The x-axis indicates the counts of the targets in each pathway. The y-axis indicates the main pathways.
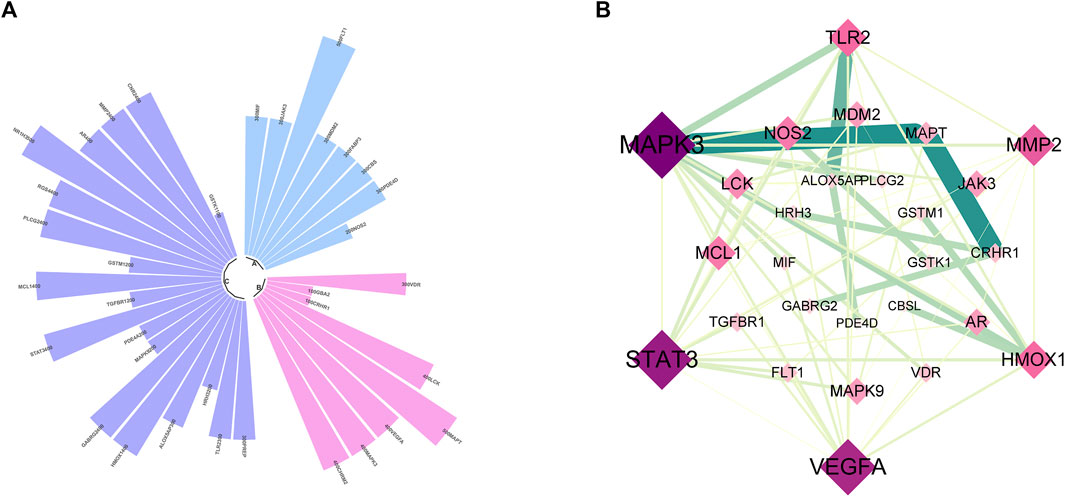
FIGURE 6. Bioinformatics analysis of active compounds targets associated with tau and Aβ pathology. (A) Donut plot. Blue: Aβ-related targets; pink: tau-related targets; purple: tau and Aβ-related targets. (B) PPI network.
3.7 Bioinformatic analysis of intersection targets associated with Aβ and tau pathology
To further explore the relationship between the potential targets of active compounds and the possible mechanisms of anti-AD, the intersection genes were imported into the AlzDate database. The results are shown in Figure 6. Among these targets (Figure 6A), eight were associated with Aβ, 8 were associated with tau, and 14 were associated with both Aβ and Tau. The 30 targets were imported into the STRING database to construct the PPI network, as shown in Figure 6B. A total of 27 nodes and 70 edges were found. After sorting by degree, the core targets included MAPK3 (degree = 16), STAT3 (degree = 14), VEGFA (degree = 13), MMP2 (degree = 9), TLR2 (degree = 8), and HMOX1 (degree = 8), indicating the multi-targeting of Synechococcus sp. against AD.
3.8 Molecular docking
To verify the binding ability between key components and key targets, molecular docking was performed using AutoDock Vina and the results were visualized using the visualization tool. N-capric acid isopropyl ester, (E)-octadec-11-enoic acid and EGFR (8A2D), VEGFA (4QAF) and MAPK3 (4QTB) molecules were docked as shown in Table 2. Among these targets, VEGFA (4QAF) was found to bind to N-capric acid isopropyl ester and (E)-octadec-11-enoic acid with scores of −6.3 and −7.2 kcal/mol, respectively, indicating that pocket VEGFA (4QAF) binds tightly to N-capric acid isopropyl ester, (E)-octadec-11-enoic acid and is stabilized by various interacting forces. Specifically, N-capric acid isopropyl ester potentially interacts with ARG858, ASP855, and PHE856 residues of EGFR (8A2D) through hydrogen bonding (Figure 7A). Among them, the distance between N-capric acid isopropyl ester and ARG858 was 3.0 Å, the distance to ASP855 was 3.2 Å, and the distance to PHE856 was 3.1 Å. N-capric acid isopropyl ester also potentially interacted with EGFR (8A2D) via van der waals, alkyl, and pi-alkyl. And N-capric acid isopropyl ester potentially interacted with VEGFA (4QAF) via van der waals, alkyl, and pi-alkyl. N-capric acid isopropyl ester binds to MAPK3 (4QTB) through the formation of hydrogen bond on TYP53 residue (Figure 7A), the distance between N-capric acid isopropyl ester and TYP53 is 3.1 Å; and the N-capric acid isopropyl ester potentially interacted with MAPK3 (4QTB) via van der waals, alkyl, and pi-alkyl. (E)-octadec-11-enoic acid potentially interacted with the MET793 residue of EGFR (8A2D) through hydrogen bonding (Figure 7B). The distances between (E)-octadec-11-enoic acid and MET793 were 2.9 and 2.2 Å. It also potentially interacted with EGFR (8A2D) via van der Waals, carbon-hydrogen bonding, alkyl, and pi-alkyl. (E)-octadec-11-enoic acid potentially interacted with VEGFA (4QAF) via van der waals, alkyl, and pi-alkyl. (E)-octadec-11-enoic acid bound to MAPK3(4QTB) through the formation of a hydrogen bond at the THR85 residue, which was separated from THR85 by a distance of 2.4 Å; and also potentially interacted with MAPK3(4QTB) via van der waals, carbon-hydrogen bond, alkyl, and pi-alkyl. These results suggest an important binding role between key components and key targets.
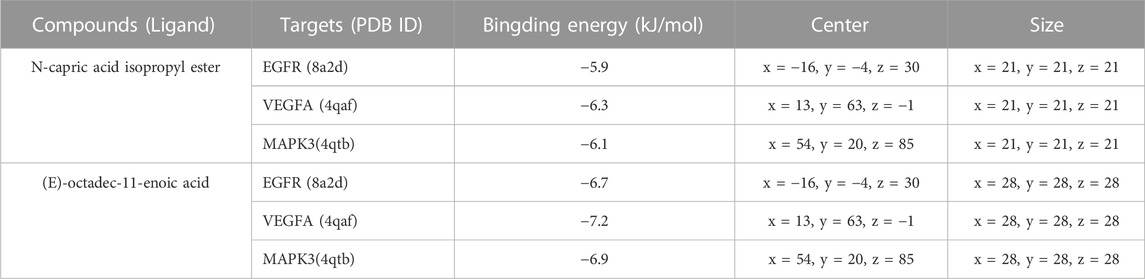
TABLE 2. Molecular docking parameters of N-capric acid isopropyl ester, (E)-octadec-11-enoic acid, and EGFR, VEGFA, and MAPK3.
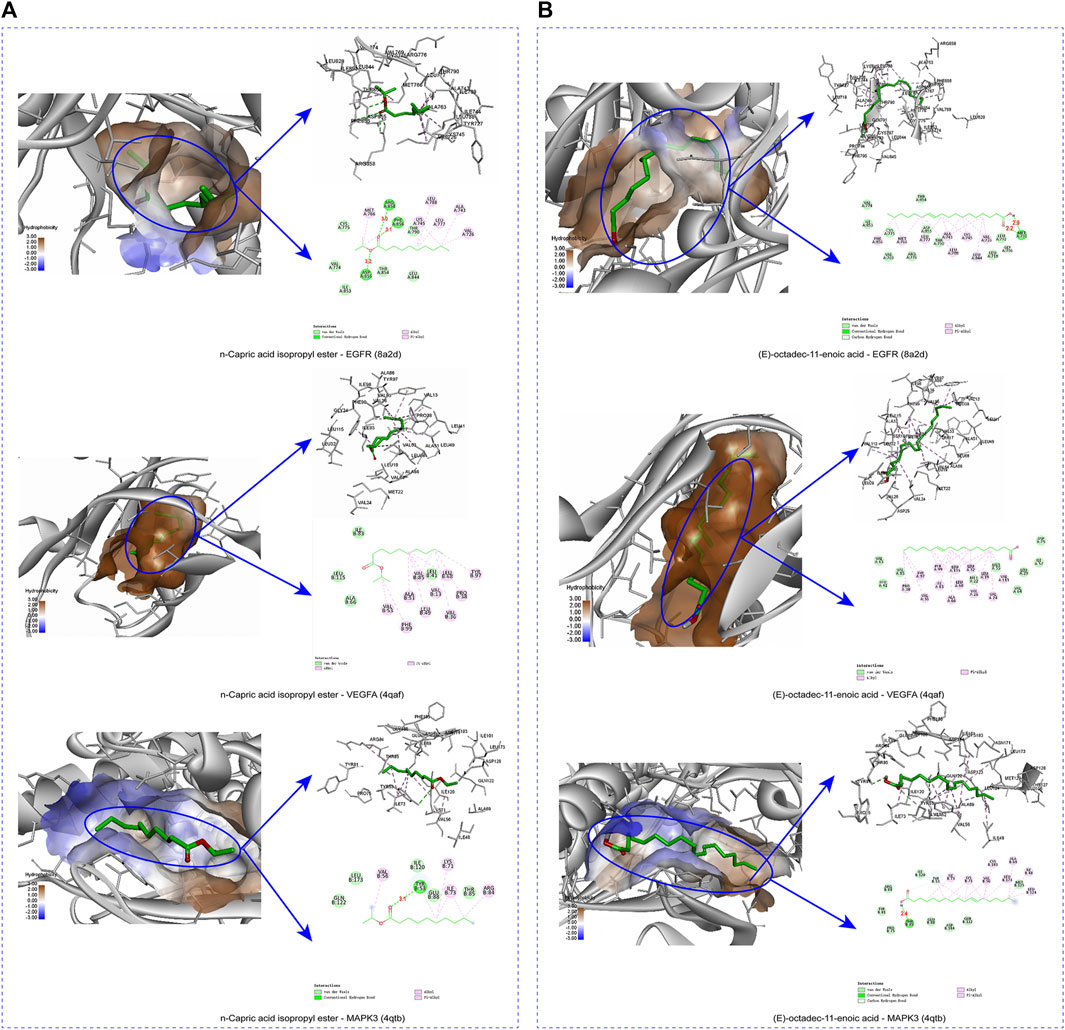
FIGURE 7. The protein-ligand of the docking simulation. (A) 3D and 2D maps of docking N-capric acid isopropyl ester with EGFR, VEGFA, and MAPK3. (B) 3D and 2D maps E-octadec-11-enoic acid of docking with EGFR, VEGFA and MAPK3.
3.9 Molecular dynamics simulation
The stability and binding of many protein‒ligand complexes have been extensively studied using molecular dynamics (MD) simulations. Based on of molecular docking results, molecular dynamics simulations were performed to further evaluate the binding activity of VEGFA (4QAF)-(E)-octadec-11-enoic acid and VEGFA (4QAF)-N-capric acid isopropyl ester. In general, root mean square deviation (RMSD) analysis plays an important role in measuring the stability of proteins and ligands. Low RMSD values indicate a more stable protein-ligand complex. As shown in Figure 8, the RMSD values did not fluctuate significantly, indicating revealed that VEGFA-(E)-octadec-11-enoic acid and VEGFA-N-capric acid isopropyl ester could bind stably to VEGFA.In addition, the RMSF score was used to valuate protein flexibility across amino acid residues. Low RMSF values indicate decreased flexibility, whereas high RMSF values indicate increased flexibility. As shown in Figure 9, both VEGFA-(E)-octadec-11-enoic acid and VEGFA-N-capric acid isopropyl ester showed lower fluctuations in the protein residues represented by root mean square fluctuations. The majorfluctuations were observed near residues 51, 206, 342, and 472, indicating greater residue flexibility in these regions of the protein. As another indicator of stability, the radius of gyration (Rg) was also analyzed. The Rg value indicated that these two protein-ligand complexes did not fluctuate significantly during the MD simulation.At the end of the 100 ns simulation, the Rg values of the complexes were 28.5007Å (Figure 10A) and 28.1664Å (Figure 10B), respectively. In addition, we further explored the H-bond analysis calculated by accompanying molecular dynamics (MD) simulations. Consistent with the molecular docking results, the molecular dynamics simulation results also indicated that there were no hydrogen bonding interactions between the ligand and the target proteins. As shown in the SASA graph (Figure 11), the SASA values of VEGFA-(E)-octadec-11-enoic acid complexes are relatively stable, while the SASA values of VEGFA-N-capric acid isopropyl ester complexes show a slight downward trend from 0 to 100 ns.
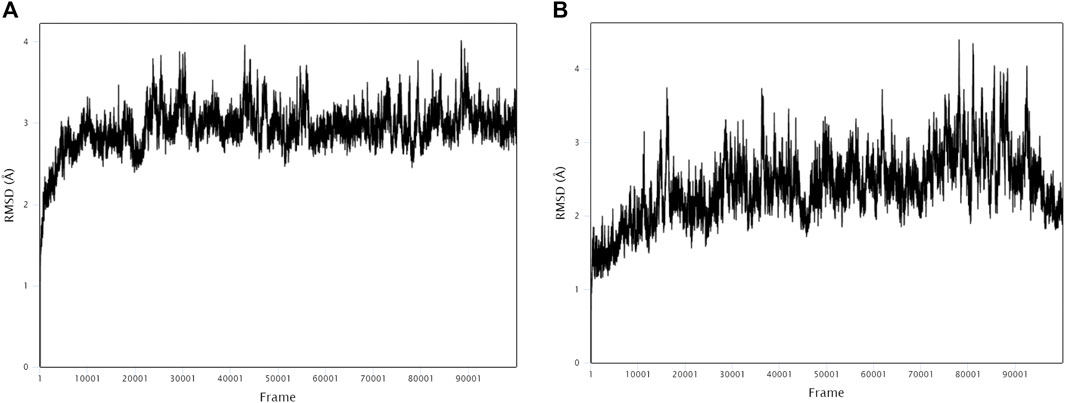
FIGURE 8. MD simulates RMSD trace values of protein-ligand complexes. VEGFA and (A) E-octadec-11-enoic acid, (B) N-capric acid isopropyl ester.
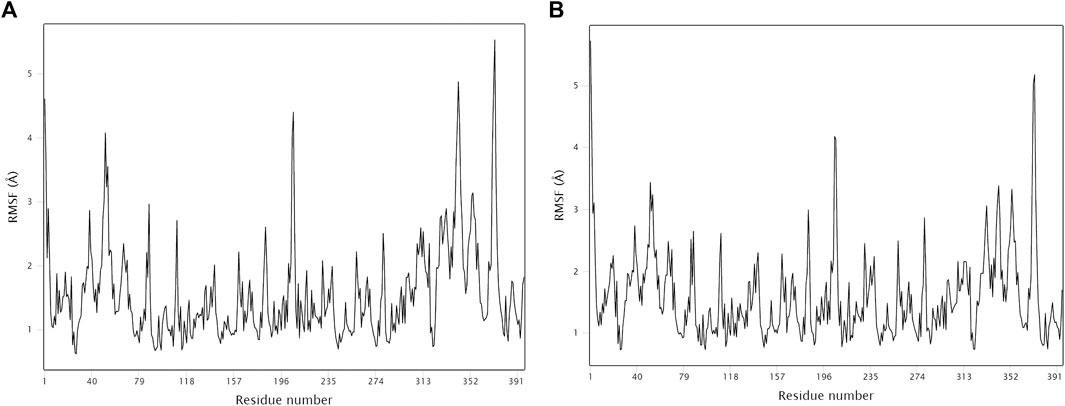
FIGURE 9. MD simulates RMSF trace values of protein-ligand complexes. VEGFA and (A) E-octadec-11-enoic acid. (B) N-capric acid isopropyl ester.
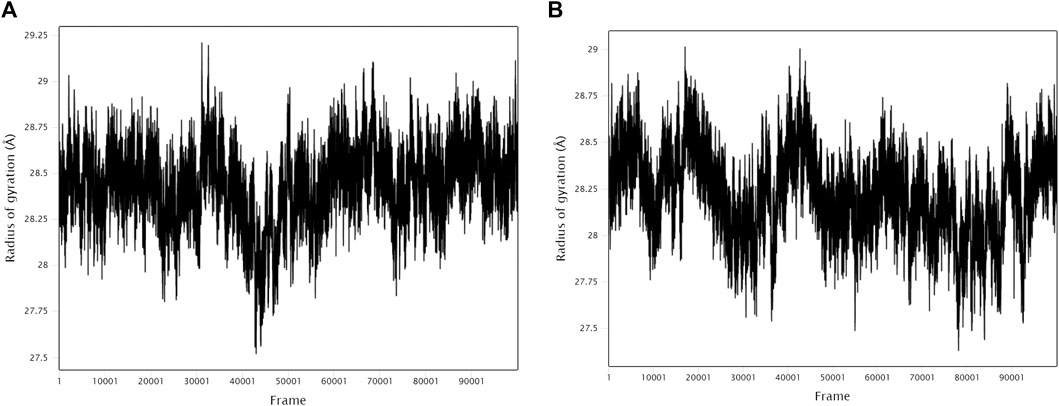
FIGURE 10. Radius of gyration (Rg) of protein-ligand complexes. VEGFA and (A) E-octadec-11-enoic acid, (B) N-capric acid isopropyl ester.
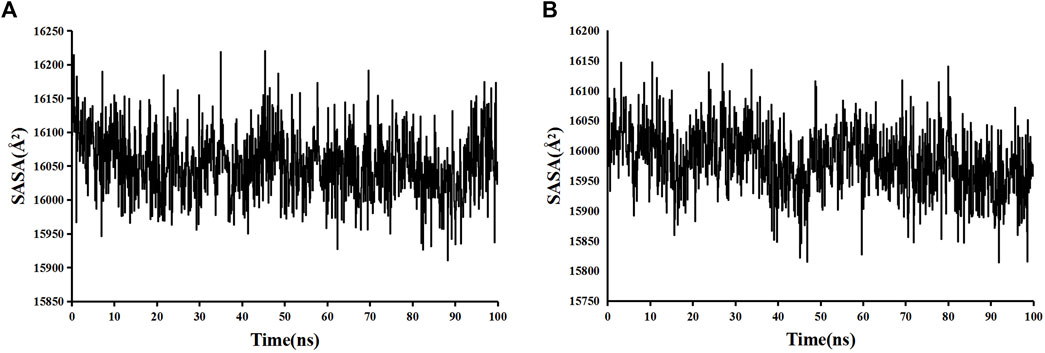
FIGURE 11. Solvent-accessible surface area (SASA) of protein-ligand complexes. VEGFA and (A) E-octadec-11-enoic acid, (B) N-capric acid isopropyl ester.
4 Discussion
Many studies have demonstrated that the incidence of AD significantly increases with age (Dubois et al., 2021). With the exacerbation of the global aging trend, the number of AD patients is growing rapidly, and the demand for drugs to defeat AD is becoming increasingly urgent (Jeremic et al., 2021). For many years, marine products and their derivatives have been recognized as a rich source of therapeutic agents and structural diversity (Wolfender et al., 2019). Marine Synechococcus is an important genus of oceanic picocyanobacteria (Dore et al., 2022). They are widely distributed in the ocean, from the equator to the poles, showing an flexible adaptability to diverse environment (Callieri, 2017). Marine Synechococcus has many advantages such as easy culture and a high growth rate. Recent studies have shown that Synechococcus contain various active components, such as proteins, pigments, and fatty acids (Liu et al., 2021; Kothari et al., 2023). In particular, unsaturated fatty acids have been proven to have antioxidant, anti-inflammatory and anti-AD-related activities. However, so far, there have been few studies on the application of these components or their combinations in AD treatment. Consequently, network pharmacology, molecular docking, and molecular dynamics simulations were used to investigate the potential targets of Synechococcus sp. XM-24 for the treatment of AD.
Firstly, GC-MS was applied to identify the 22 constituents in the Synechococcus sp. XM-24. Among them, that (E)-octadec-11-enoic acid is the major constituent, while other volatile compounds such as indolizine, isoquinoline, alkene, and alkane, were also been founded. The current literature demonstrates that some compounds could have potential toxicity. Thus, by using multiple databases, we further selected out 10 compounds with higher safety for the further analysis. Subsequently, by constructing a network diagram of active compound-target-signaling pathways, we found that N-capric acid isopropyl ester and (E)-octadec-11-enoic acid had much higher degree values in the network analysis than other compounds. Structurally, these two compounds are medium-chain fatty acid esters and unsaturated fatty acids, respectively. Compound N-capric acid isopropyl ester was reported being found in uraria picta Desv., a terrestrial plant (Mohan et al., 2020). However, its possible medicinal application was not exploring. Moreover, (E)-octadec-11-enoic acid are not commonly found in terrestrial photoautotroph. To date, very few existing literatures reports the anti-AD activity of these two compounds. There are some clues to suggest that these compounds are also promising therapeutic candidates to combat AD. Molecular docking results showed that both N-capric acid isopropyl ester and (E)-octadec-11-enoic acid had certain binding with EGFR, VEGFA, and MAPK3. At the current stage, research on medium-chain fatty acid esters is limited, and they are often used as food additives (Mayser, 2015). However, recent studies have also found that this type of compound has activities such as anti-psychotic and anti-bacterial activity (Koch et al., 2022). Trans-11-octadecenoic acid is a monounsaturated fatty acid that is also present in nuts and sea buckthorn fruit (Arruda et al., 2022). Pharmacological studies have shown that trans-11 vaccenic acid appears to have no health risks related to industrially produced trans fatty acids (Wang X. et al., 2016). In contrast, trans fatty acids are beneficial in the prevention of heart disease, obesity, and cancer (Lim et al., 2014; Wang et al., 2016). In addition, its derivatives have been found to have anti-oxidative stress activity. In vitro cell experiments showed that 10-Oxo-trans-11-octadecenoic acid (KetoC) can protect HepG2 cells from hydrogen peroxide-induced cytotoxicity. KetoC activates the Nrf2-ARE pathway to enhance in vitro and in vivo cellular antioxidant responses, further indicating that KetoC can prevent various diseases caused by oxidative stress (Furumoto et al., 2016). Based on existing studies, there is evidence of complex interactions between oxidative stress and the pathogenesis of Alzheimer’s disease (AD). In AD patients, elevated levels of protein oxidation, lipid peroxidation, and DNA damage products have been detected, suggesting the presence of oxidative damage (Ionescu-Tucker and Cotman, 2021; Afzal et al., 2021). Therefore, the active compounds found in Synechococcus sp. XM-24 hold potential in exerting anti-AD activity through the inhibition of oxidative stress.
Based on the intersection targets analysis, we found that these 10 active compounds mainly play anti-AD effects by acting on 128 core targets. Among them, EGFR, VEGFA, MAPK3, STAT3, and PTGS2 have higher degree values in the network analysis and have been considered the most likely targets to exert anti-AD effects. Moreover, numerous studies have shown that the accumulation of Aβ peptide, which are the main component of amyloid plaques, is the main factor in the pathogenesis of AD (Janus et al., 2000; Tanzi et al., 2004; Guo T. et al., 2020a). In addition, there is a close relationship between the occurrence of tau protein and AD (Mandelkow and Mandelkow, 1998; Kolarova et al., 2012). Therefore, we also analyzed the pathological correlation between these targets to Aβ and tau by using the Alzdatabase. The results showed that 30 targets among these 128 targets were significantly correlated with tau, Aβ, or both. Among them, MAPK3, STAT3, VEGFA, MMP2, and TLR2 had the highest degree values. Furthermore, STAT3, VEGFA, and MAPK3 were also the key targets in the PPI network. Prior studies have shown that the transcription factor Stat3 is a typical inducer of astrocyte proliferation and can be activated in AD model mice and human AD patients (Pekny et al., 2016; Escartin et al., 2021). In vitro and in vivo studies have demonstrated that the accumulation of tau can cause the inactivation of STAT3, which in turn inhibits the expression of N-methyl-d-aspartate receptors (NMDAR), and subsequently caused memory impairment (Wan et al., 2021). In addition, many recent studies have exhibited that the VEGF family plays a significant role in regulating angiogenesis, neurogenesis, and neuron survival (Rosenstein and Krum, 2004; Eichmann and Simons, 2012). The decrease in VEGF-A levels in cerebrospinal fluid is found to have been associated with the higher risk of developing AD and cognitive impairment (Hohman et al., 2015). Moreover, higher concentrations of VEGFA in cerebrospinal fluid were found to be associated with slower rates of hippocampal atrophy and cognitive decline, particularly in AD biomarker-positive adults (Sweeney et al., 2015). Furthermore, MAPK pathway is an important signaling pathway which closely related to cell proliferation, differentiation, and apoptosis (Guo Y. J. et al., 2020b). Through activation of the MAPK signaling pathway, could strongly influence the pathogenesis of AD, such as neuronal apoptosis β-secretase activity and γ-secretase activity (Lee et al., 2008; Kim E. K. and Choi, 2010). Previous studies have shown that stable AD patients treated with acetylcholinesterase inhibitors can further improve cognitive impairment when combined with cilostazol (Tai S. Y. et al., 2017). Based on this, Oguchi T. et al. confirmed through cell experiments that cilostazol can inhibit Aβ-induced SH-SY5Y cell neurotoxicity by inhibiting oxidative stress and the MAPK signaling pathway (Oguchi et al., 2017). According to the research of Tian Qing et al., the traditional Chinese medicine-Uncaria rhynchophylla can exert therapeutic effects on AD by acting on several key targets such as MAPK3 (Zeng et al., 2021b). Based on multiple clinical practice studies conducted in China and Japan, Huang-Lian-Jie-Du decoction (HLJDD) has significant neuroprotective effects. By employing network pharmacology strategies, Zheng et al. confirmed that HLJDD mainly exerts anti-AD effects by acting on multiple potential therapeutic targets, such as VEGFA, AKT1, TNF, YP53 and PTGS2 (Zheng R. et al., 2023). These studies indicate that VEGFA is strongly associated with AD.
In this study, we also conducted GO analysis and KEGG pathway enrichment analysis on potential target genes of active compounds against AD. The results indicated that the PI3K-Akt signaling pathway, Neuroactive ligand-receptor interaction, and Ras signaling pathway were the most significantly enriched pathways. Many studies have proved that the PI3K-Akt pathway can affect various major biological processes including cell proliferation, growth, and survival (Xu et al., 2020; Long et al., 2021). These pathophysiological processes are closely related to the occurrence of AD (Kumar and Bansal, 2022). Hence, the regulation of PI3K-Akt signaling may be helpful for the treatment of AD (Fakhri et al., 2021; Kumar and Bansal, 2022; Khezri et al., 2023). Fuhai Li et al. proposed that PI3K-Akt is a core neuroinflammatory signaling pathway that leads to chronic neurodegeneration (Li F. et al., 2021). Ruolan Li et al. used the clinical clinically used traditional Chinese medicine Yizhiren (AOF) as the research object. In vitro experiments indicated that and demonstrated through cell experiments that AOF can significantly increase the phosphorylation levels of PI3K and Akt proteins, thereby confirming that AOF can exert anti-AD effects by regulating the PI3K/Akt pathway (Li R. et al., 2022). The Neuroactive ligand-receptor interaction is also considered to be an important signaling pathway that associated with AD. According to the analysis of AD brain RNA expression chip dataset in the GEO database, the downregulation of SIRT3 mRNA in the brain tissue of AD patients may promote the progression of AD by affecting this pathway (Song et al., 2020). Zhou et al. found multiple potential AD therapeutic targets closely related to the neuroactive ligand-receptor interaction signaling pathway through the integration of phenotype screening data and multi-pharmacology network research (Zhou et al., 2023). In addition, according to the literature research, intervening in the Rho signaling pathway can effectively regulate the cascade reaction of tau and amyloid-beta in AD (Desale et al., 2021; Kumari et al., 2023). Plant- and marine-derived secondary metabolites are expected to treat multiple neurodegenerative diseases through the Ras/Raf/MAPKs pathway, which provides a new perspective for developing promising new multi-target anti-AD drugs (Gravandi et al., 2023).
As a result, Synechococcus sp. XM-24 highly likely execute anti-AD effects by regulates PI3K-Akt signaling, Neuroactive ligand-receptor interaction and Rho signaling pathway, etc., whereas its active compounds of by interacting with STAT3, VEGFA, and MAPK3. Additionally, we employed molecular docking and molecular dynamics simulations to further investigate the stability and interactions between key compounds and core target proteins. Favorable docking scores demonstrated a strong and efficient binding between these key compounds and core target proteins. Furthermore, during the 70ns MD simulation process, the conformation of the complex remained relatively unchanged, indicating a stable binding between the key active compounds and core target proteins. These results provide strong evidence for the potential therapeutic effects of key active compounds in Synechococcus sp. XM-24 against Alzheimer’s disease.
Moreover, it is also worth mentioning that marine cyanobacteria has advantages different from terrestrial organisms, such as rapid growth rate and easy availability (Rodolfi et al., 2009; Bhalamurugan et al., 2018). There, the application of bio-renewable compounds in cyanobacteria can be a valuable future research direction. Besides, since the growth of cyanobacteria may be affected by many factors, including temperature, nutrients, and accompanying bacteria and so on. By revealing the key anti-AD compounds of Synechococcus sp. XM-24, we can develop an ideal culture condition for better outcomes. In summary, in this study, we efficiently selected the most promising and drug-like small molecules from Synechococcus sp. XM-24 by using GC‒MS technology and network pharmacology research strategies. Then, the molecular virtual docking and molecular dynamic simulation results were confirmed that these active compounds and their combinations are expected to exert anti-AD effects by acting on key targets such as STAT3, VEGFA, and MAPK. In general, our study provides new research strategies and ideas for the study of Synechococcus in the field of anti-AD. However, in future studies, the anti-AD activity of these ingredients and their combinations in vitro and in vivo remains to be experimentally determined to confirm our hypothesis.
5 Conclusion
In this study, GC‒MS, network pharmacology analysis, and molecular docking were used to identify the active compounds, core targets, and signaling pathways of Synechococcus sp. XM-24 to explore its potential mechanisms in the treatment of AD.
A total of 22 active compounds were identified by GC-MS and 10 of them were further analyzed using network pharmacology analysis. The result show that compounds N-capric acid isopropyl ester and (E)-octadec-11-enoic acid obtained higher degrees in the network pharmacology analysis, indicating their higher potential in anti-AD process. Moreover, molecular docking showed favorable intermolecular hydrogen-bond formation between them and three core targets (EGFR, VEGFA, and MAPK3). It is expected that these results could provide a theoretical framework for the application of marine cyanobacteria in the treatment of AD. However, we are of the opinion that further studies are necessary in order to elucidate the specific targets and mechanisms behind them. Marine cyanobacteria was seems to be clearly an essential source of bioactive compounds and will continue to be a source of significant drug leads in the pharmaceutical industry. Furthermore, the application of advanced technology, e.g., multi-omics approach and nanotechnology, will provide us strong supporting on discovering novel active compounds and therapeutic method.
Data availability statement
The datasets presented in this study can be found in online repositories. The names of the repository/repositories and accession number(s) can be found in the article/Supplementary Material.
Author contributions
RX, YW, and QZ: conceptualization. RX, YW, and JC: funding acquisition. FC, YW, WH, and JC: investigation. RX, FC, and YM: methodology and writing-original draft. YW, YM and WH: software. FC, WH, and JC: supervision. YW, FC, YM, and JC: validation. RX, YW, and JC: writing-review and editing. All authors contributed to the article and approved the submitted version.
Funding
The authors declare financial support was received for the research, authorship, and/or publication of this article. The authors acknowledge the Scientific Research Fund of Jiangxi Provincial Education Department (Grant No. GJJ2201438), Open Project of Key Laboratory of Prevention and treatment of cardiovascular and cerebrovascular diseases Ministry of Education (Grant No. XN202030, XN202024, and XN201912), and National Science Foundation of China (Grant No. 21602090) for financial support.
Acknowledgments
We would also like to thank Guangzhou Yinfo Information Technology Co., Ltd. for providing a friendly and versatile web server (https://cloud.yinfotek.com) to aid the molecular dynamics studies.
Conflict of interest
The authors declare that the research was conducted in the absence of any commercial or financial relationships that could be construed as a potential conflict of interest.
Publisher’s note
All claims expressed in this article are solely those of the authors and do not necessarily represent those of their affiliated organizations, or those of the publisher, the editors and the reviewers. Any product that may be evaluated in this article, or claim that may be made by its manufacturer, is not guaranteed or endorsed by the publisher.
Supplementary material
The Supplementary Material for this article can be found online at: https://www.frontiersin.org/articles/10.3389/fphar.2023.1249632/full#supplementary-material
Abbreviations
AChEIs, acetylcholinesterase inhibitors; AD, Alzheimer’s disease; AOF, Yizhiren; BP, biological process; CC, cellular component; EGFR, epidermal growth factor receptor; GC-MS, gas chromatography-mass spectrometry; GO, Gene Ontology; HLJDD, Huang-Lian-Jie-Du decoction; KEGG, Kyoto Encyclopedia of Genes and Genomes; KetoC, 10-Oxo-trans-11-octadecenoic acid; EPA, eicosapentaenoic acid; DHA, docosahexaenoic acid; Aβ, β-amyloid; MAPK3, mitogen-activated protein kinase 3; MF, molecular function; NFT, neurofibrillary tangles; NMDAR, N-methyl-d-aspartate receptors; PTGS2, prostaglandin-endoperoxide synthase 2; PPI, protein–protein interaction; VEGFA, vascular endothelial growth factor A.
References
Abed, R. M., Dobretsov, S., and Sudesh, K. (2009). Applications of cyanobacteria in biotechnology. J. Appl. Microbiol. 106, 1–12. doi:10.1111/j.1365-2672.2008.03918.x
Afzal, M., Sidra, T., and Li, Y. (2021). Mitochondrial dysfunction and oxidative stress in Alzheimer's disease. Front. Aging Neurosci. 13, 748388. doi:10.3389/fnagi.2021.617588
Ahlgren, N. A., and Rocap, G. (2006). Culture isolation and culture-independent clone libraries reveal new marine Synechococcus ecotypes with distinctive light and N physiologies. Appl. Environ. Microbiol. 72, 7193–7204. doi:10.1128/AEM.00358-06
Arruda, H. S., Geraldi, M. V., Cedran, M. F., Bicas, , Lemos, J., Junior, M., et al. (2022). “Bioactive food components activity in mechanistic approach,” in Prebiotics and probiotics. Editors C. B. B. Cazarin, J. L. Bicas, G. M. Pastore, and M. R. Marostica Junior (Academic Press), 55–118.
Athanasios, A., Charalampos, V., Vasileios, T., and Ashraf, G. M. (2017). Protein-protein interaction (PPI) network: recent advances in drug discovery. Curr. Drug Metab. 18, 5–10. doi:10.2174/138920021801170119204832
Bhalamurugan, G. L., Valerie, O., and Mark, L. (2018). Valuable bioproducts obtained from microalgal biomass and their commercial applications: a review. Environ. Eng. Res. 23, 229–241. doi:10.4491/eer.2017.220
Bhat, V. B., and Madyastha, K. M. (2001). Scavenging of peroxynitrite by phycocyanin and phycocyanobilin from Spirulina platensis: protection against oxidative damage to DNA. Biochem. Biophys. Res. Commun. 285, 262–266. doi:10.1006/bbrc.2001.5195
Bishoyi, A. K., Sahoo, C. R., and PadhyNath, R. (2023). Recent progression of cyanobacteria and their pharmaceutical utility: an update. J. Biomol. Struct. Dyn. 41, 4219–4252. doi:10.1080/07391102.2022.2062051
Bourgade, B., and Stensjö, K. (2022). Synthetic biology in marine cyanobacteria: advances and challenges. Front. Microbiol. 13, 994365. doi:10.3389/fmicb.2022.994365
Burley, S. K., Bhikadiya, C., Bi, C., Bittrich, S., Chen, L., Crichlow, G. V., et al. (2021). RCSB Protein Data Bank: powerful new tools for exploring 3D structures of biological macromolecules for basic and applied research and education in fundamental biology, biomedicine, biotechnology, bioengineering and energy sciences. Nucleic Acids Res. 49, D437–D451. doi:10.1093/nar/gkaa1038
Callieri, C. (2017). Synechococcus plasticity under environmental changes. FEMS Microbiol. Lett. 364. doi:10.1093/femsle/fnx229
Chen, C., Tang, T., Shi, Q., Zhou, Z., and Fan, J. (2022). The potential and challenge of microalgae as promising future food sources. Trends Food Sci. Technol. 126, 99–112. doi:10.1016/j.tifs.2022.06.016
Chen, J., Li, C., Zhu, Y., Sun, L., Sun, H., Liu, Y., et al. (2015). Integrating GO and KEGG terms to characterize and predict acute myeloid leukemia-related genes. Hematology 20, 336–342. doi:10.1179/1607845414Y.0000000209
Citron, M. (2010). Alzheimer's disease: strategies for disease modification. Nat. Rev. Drug Discov. 9, 387–398. doi:10.1038/nrd2896
Costa, M., Garcia, M., Costa-Rodrigues, J., Costa, M. S., Ribeiro, M. J., Fernandes, M. H., et al. (2013). Exploring bioactive properties of marine cyanobacteria isolated from the Portuguese coast: high potential as a source of anticancer compounds. Mar. Drugs 12, 98–114. doi:10.3390/md12010098
Dahms, H. U., Ying, X., and Pfeiffer, C. (2006). Antifouling potential of cyanobacteria: a mini-review. Biofouling 22, 317–327. doi:10.1080/08927010600967261
Daina, A., Michielin, O., and Zoete, V. (2019). SwissTargetPrediction: updated data and new features for efficient prediction of protein targets of small molecules. Nucleic Acids Res. 47, W357-W364–W364. doi:10.1093/nar/gkz382
de Jesus Raposo, M. F., de Morais, R. M., and de Morais, A. M. (2013). Health applications of bioactive compounds from marine microalgae. Life Sci. 93, 479–486. doi:10.1016/j.lfs.2013.08.002
de Morais, M. G., Vaz Bda, S., de Morais, E. G., and Costa, J. A. (2015). Biologically active metabolites synthesized by microalgae. Biomed. Res. Int. 2015, 835761. doi:10.1155/2015/835761
Desale, S. E., Chidambaram, H., and Chinnathambi, S. (2021). G-protein coupled receptor, PI3K and Rho signaling pathways regulate the cascades of Tau and amyloid-beta in Alzheimer's disease. Mol. Biomed. 2, 17. doi:10.1186/s43556-021-00036-1
do Amaral, S. C., Santos, A. V., da Cruz Schneider, M. P., da Silva, J. K. R., and Xavier, L. P. (2020). Determination of volatile organic compounds and antibacterial activity of the amazonian cyanobacterium Synechococcus sp. strain GFB01. Molecules 25, 4744. doi:10.3390/molecules25204744
Dore, H., Leconte, J., Guyet, U., Breton, S., Farrant, G. K., Demory, D., et al. (2022). Global phylogeography of marine Synechococcus in coastal areas reveals strong community shifts. mSystems 7, e0065622. doi:10.1128/msystems.00656-22
Dubois, B., Villain, N., Frisoni, G. B., Rabinovici, G. D., Sabbagh, M., Cappa, S., et al. (2021). Clinical diagnosis of Alzheimer's disease: recommendations of the international working group. Lancet Neurol. 20, 484–496. doi:10.1016/S1474-4422(21)00066-1
Eichmann, A., and Simons, M. (2012). VEGF signaling inside vascular endothelial cells and beyond. Curr. Opin. Cell Biol. 24, 188–193. doi:10.1016/j.ceb.2012.02.002
Escartin, C., Galea, E., Lakatos, A., O'Callaghan, J. P., Petzold, G. C., Serrano-Pozo, A., et al. (2021). Reactive astrocyte nomenclature, definitions, and future directions. Nat. Neurosci. 24, 312–325. doi:10.1038/s41593-020-00783-4
Fakhri, S., Iranpanah, A., Gravandi, M. M., Moradi, S. Z., Ranjbari, M., Majnooni, M. B., et al. (2021). Natural products attenuate PI3K/Akt/mTOR signaling pathway: a promising strategy in regulating neurodegeneration. Phytomedicine 91, 153664. doi:10.1016/j.phymed.2021.153664
Ferris, M. J., Muyzer, G., and Ward, D. M. (1996). Denaturing gradient gel electrophoresis profiles of 16S rRNA-defined populations inhabiting a hot spring microbial mat community. Appl. Environ. Microbiol. 62, 340–346. doi:10.1128/aem.62.2.340-346.1996
Flombaum, P., Gallegos, J. L., Gordillo, R. A., Rincon, J., Zabala, L. L., Jiao, N., et al. (2013). Present and future global distributions of the marine Cyanobacteria Prochlorococcus and Synechococcus. Proc. Natl. Acad. Sci. U. S. A. 110, 9824–9829. doi:10.1073/pnas.1307701110
Furumoto, H., Nanthirudjanar, T., Kume, T., Izumi, Y., Park, S. B., Kitamura, N., et al. (2016). 10-Oxo-trans-11-octadecenoic acid generated from linoleic acid by a gut lactic acid bacterium Lactobacillus plantarum is cytoprotective against oxidative stress. Toxicol. Appl. Pharmacol. 296, 1–9. doi:10.1016/j.taap.2016.02.012
Garcia-Pichel, F., and Pringault, O. (2001). Microbiology. Cyanobacteria track water in desert soils. Nature 413, 380–381. doi:10.1038/35096640
Gaysina, L. A., Saraf, A., and Singh, P. (2019). “Cyanobacteria in diverse habitats,” in Cyanobacteria. Editors A. K. Mishra, D. N. Tiwari, and A. N. Rai (Academic Press), 1–28.
Gómez, P. I., Mayorga, J., Flaig, D., Castro-Varela, P., Jaupi, A., Ulloa, P. A., et al. (2023). Looking beyond Arthrospira: comparison of antioxidant and anti-inflammatory properties of ten cyanobacteria strains. Algal Res. 74, 103182. doi:10.1016/j.algal.2023.103182
Gravandi, M. M., Abdian, S., Tahvilian, M., Iranpanah, A., Moradi, S. Z., Fakhri, S., et al. (2023). Therapeutic targeting of Ras/Raf/MAPK pathway by natural products: a systematic and mechanistic approach for neurodegeneration. Phytomedicine 115, 154821. doi:10.1016/j.phymed.2023.154821
Guo, T., Zhang, D., Zeng, Y., Huang, T. Y., Xu, H., and Zhao, Y. (2020a). Molecular and cellular mechanisms underlying the pathogenesis of Alzheimer's disease. Mol. Neurodegener. 15, 40. doi:10.1186/s13024-020-00391-7
Guo, Y. J., Pan, W. W., Liu, S. B., Shen, Z. F., Xu, Y., and Hu, L. L. (2020b). ERK/MAPK signalling pathway and tumorigenesis. Exp. Ther. Med. 19, 1997–2007. doi:10.3892/etm.2020.8454
Gutierrez, M., Suyama, T. L., Engene, N., Wingerd, J. S., Matainaho, T., and Gerwick, W. H. (2008). Apratoxin D, a potent cytotoxic cyclodepsipeptide from Papua New Guinea collections of the marine cyanobacteria Lyngbya majuscula and Lyngbya sordida. J. Nat. Prod. 71, 1099–1103. doi:10.1021/np800121a
Hassan, S., Meenatchi, R., Pachillu, K., Bansal, S., Brindangnanam, P., Arockiaraj, J., et al. (2022). Identification and characterization of the novel bioactive compounds from microalgae and cyanobacteria for pharmaceutical and nutraceutical applications. J. Basic Microbiol. 62, 999–1029. doi:10.1002/jobm.202100477
Hohman, T. J., Bell, S. P., and Jefferson, A. L.Alzheimer's Disease Neuroimaging, Initiative (2015). The role of vascular endothelial growth factor in neurodegeneration and cognitive decline: exploring interactions with biomarkers of Alzheimer disease. JAMA Neurol. 72, 520–529. doi:10.1001/jamaneurol.2014.4761
Hopkins, A. L. (2007). Network pharmacology. Nat. Biotechnol. 25, 1110–1111. doi:10.1038/nbt1007-1110
Hsieh-Lo, M. C., Gustavo, O.-B., Alberto, M., and Mojica, L. (2019). Phycocyanin and phycoerythrin: strategies to improve production yield and chemical stability. Algal Res. 42, 101600. doi:10.1016/j.algal.2019.101600
Huang da, W., Sherman, B. T., and Lempicki, R. A. (2009). Systematic and integrative analysis of large gene lists using DAVID bioinformatics resources. Nat. Protoc. 4, 44–57. doi:10.1038/nprot.2008.211
Ionescu-Tucker, A., and Cotman, C. W. (2021). Emerging roles of oxidative stress in brain aging and Alzheimer's disease. Neurobiol. Aging 107, 86–95. doi:10.1016/j.neurobiolaging.2021.07.014
Janus, C., Pearson, J., McLaurin, J., Mathews, P. M., Jiang, Y., Schmidt, S. D., et al. (2000). A beta peptide immunization reduces behavioural impairment and plaques in a model of Alzheimer's disease. Nature 408, 979–982. doi:10.1038/35050110
Jeremic, D., Jimenez-Diaz, L., and Navarro-Lopez, J. D. (2021). Past, present and future of therapeutic strategies against amyloid-beta peptides in Alzheimer's disease: a systematic review. Ageing Res. Rev. 72, 101496. doi:10.1016/j.arr.2021.101496
Keiser, M. J., Roth, B. L., Armbruster, B. N., Ernsberger, P., Irwin, J. J., and Shoichet, B. K. (2007). Relating protein pharmacology by ligand chemistry. Nat. Biotechnol. 25, 197–206. doi:10.1038/nbt1284
Khezri, M. R., Ghasemnejad-Berenji, M., and Moloodsouri, D. (2023). The PI3K/AKT signaling pathway and caspase-3 in Alzheimer's disease: which one is the beginner? J. Alzheimers Dis. 92, 391–393. doi:10.3233/JAD-221157
Kim, E. K., and Choi, E. J. (2010). Pathological roles of MAPK signaling pathways in human diseases. Biochim. Biophys. Acta 1802, 396–405. doi:10.1016/j.bbadis.2009.12.009
Kim, K., Kim, M. J., Kim, D. W., Kim, S. Y., Park, S., and Park, C. B. (2020). Clinically accurate diagnosis of Alzheimer's disease via multiplexed sensing of core biomarkers in human plasma. Nat. Commun. 11, 119. doi:10.1038/s41467-019-13901z
Koch, C., Nordzieke, S., Grieger, J., and Mayser, P. (2022). Medium-chain fatty acid esters are effective even in azole-resistant Malassezia pachydermatis. Mycoses 65, 1188–1193. doi:10.1111/myc.13512
Kolarova, M., Garcia-Sierra, F., Bartos, A., Ricny, J., and Ripova, D. (2012). Structure and pathology of tau protein in Alzheimer disease. Int. J. Alzheimers Dis. 2012, 731526. doi:10.1155/2012/731526
Kothari, R., Singh, H. M., Azam, R., Goria, K., Bharti, A., Singh, A., et al. (2023). Potential avenue of genetic engineered algal derived bioactive compounds: influencing parameters, challenges and future prospects. Phytochem. Rev. 22, 935–968. doi:10.1007/s11101-023-09859-y
Kumar, M., and Bansal, N. (2022). Implications of phosphoinositide 3-kinase-akt (PI3K-Akt) pathway in the pathogenesis of Alzheimer's disease. Mol. Neurobiol. 59, 354–385. doi:10.1007/s12035-021-02611-7
Kumari, S., Dhapola, R., and Reddy, D. H. (2023). Apoptosis in Alzheimer's disease: insight into the signaling pathways and therapeutic avenues. Apoptosis 28, 943–957. doi:10.1007/s10495-023-01848-y
Lee, J. W., Lee, Y. K., Yuk, D. Y., Choi, D. Y., Ban, S. B., Oh, K. W., et al. (2008). Neuro-inflammation induced by lipopolysaccharide causes cognitive impairment through enhancement of beta-amyloid generation. J. Neuroinflammation 5, 37. doi:10.1186/1742-2094-5-37
Li, F., Eteleeb, A., Buchser, W., Wang, G., Xiong, C., Payne, P. R., et al. (2021). Weakly activated core inflammation pathways were identified as a central signaling mechanism contributing to the chronic neurodegeneration in Alzheimer's disease. bioRxiv, 2021.08.30.458295. doi:10.1101/2021.08.30.458295
Li, R., Wang, L., Zhang, Q., Duan, H., Qian, D., Yang, F., et al. (2022). Alpiniae oxyphyllae fructus possesses neuroprotective effects on H(2)O(2) stimulated PC12 cells via regulation of the PI3K/Akt signaling Pathway. Front. Pharmacol. 13, 966348. doi:10.3389/fphar.2022.966348
Li, W. K. W. (1994). Primary production of prochlorophytes, cyanobacteria, and eucaryotic ultraphytoplankton: measurements from flow cytometric sorting. Limnol. Oceanogr. 39, 169–175. doi:10.4319/lo.1994.39.1.0169
Lim, J.-N., Oh, J.-J., Wang, T., Lee, J. S., Kim, S. H., Kim, Y.-J., et al. (2014). trans-11 18:1 vaccenic acid (TVA) has a direct anti-carcinogenic effect on MCF-7 human mammary adenocarcinoma cells. Nutrients 6, 627–636. doi:10.3390/nu6020627
Linington, R. G., Edwards, D. J., Shuman, C. F., McPhail, K. L., Matainaho, T., and Gerwick, W. H. (2008). Symplocamide A, a potent cytotoxin and chymotrypsin inhibitor from the marine Cyanobacterium Symploca sp. J. Nat. Prod. 71, 22–27. doi:10.1021/np070280x
Liu, D., Liberton, M., Hendry, J. I., Aminian-Dehkordi, J., Maranas, C. D., and Pakrasi, H. B. (2021). Engineering biology approaches for food and nutrient production by cyanobacteria. Curr. Opin. Biotechnol. 67, 1–6. doi:10.1016/j.copbio.2020.09.011
Long, H.-Z., Cheng, Y., Zhou, Z.-W., Luo, H. Y, Wen, D. D., and Gao, L.-C. (2021). PI3K/AKT signal pathway: a target of natural products in the prevention and treatment of Alzheimer’s disease and Parkinson’s disease. Front. Pharmacol. 12, 648636. doi:10.3389/fphar.2021.648636
Mandelkow, E. M., and Mandelkow, E. (1998). Tau in Alzheimer's disease. Trends Cell Biol. 8, 425–427. doi:10.1016/s0962-8924(98)01368-3
Mandhata, C. P., Sahoo, C. R., and Padhy, R. N. (2022). Biomedical applications of biosynthesized gold nanoparticles from cyanobacteria: an overview. Biol. Trace Elem. Res. 200, 5307–5327. doi:10.1007/s12011-021-03078-2
Martins, R. F., Ramos, M. F., Herfindal, L., Sousa, J. A., Skaerven, K., and Vasconcelos, V. M. (2008). Antimicrobial and cytotoxic assessment of marine cyanobacteria - synechocystis and Synechococcus. Mar. Drugs. 6, 1–11. doi:10.3390/md6010001
Mayser, P. (2015). Medium chain fatty acid ethyl esters - activation of antimicrobial effects by Malassezia enzymes. Mycoses 58, 215–219. doi:10.1111/myc.12300
Mohan, B., Saxena, H. O., Parihar, S., and Kakkar, A. (2020). Gas chromatography-mass spectrometry (GC-MS) determination of phytoconstituents from ethanolic and aqua-ethanolic root extracts of Uraria picta Desv. (Fabaceae). Pharma Innov. 9, 463–467. doi:10.22271/tpi.2020.v9.i7g.4973
Momper, L., Hu, E., Moore, K. R., Skoog, E. J., Tyler, M., Evans, A. J., et al. (2019). Metabolic versatility in a modern lineage of cyanobacteria from terrestrial hot springs. Free Radic. Biol. Med. 140, 224–232. doi:10.1016/j.freeradbiomed.2019.05.036
Morris, G. M., and Lim-Wilby, M. (2008). Molecular docking. Methods Mol. Biol. 443, 365–382. doi:10.1007/978-1-59745-177-2_19
Nubel, U., Garcia-Pichel, F., Kuhl, M., and Muyzer, G. (1999). Quantifying microbial diversity: morphotypes, 16S rRNA genes, and carotenoids of oxygenic phototrophs in microbial mats. Appl. Environ. Microbiol. 65, 422–430. doi:10.1128/AEM.65.2.422-430.1999
Oguchi, T., Ono, R., Tsuji, M., Shozawa, H., Somei, M., Inagaki, M., et al. (2017). Cilostazol suppresses aβ-induced neurotoxicity in SH-SY5Y cells through inhibition of oxidative stress and MAPK signaling pathway. Front. Aging Neurosci. 9, 337. doi:10.3389/fnagi.2017.00337
Palenik, B., Brahamsha, B., Larimer, F. W., Land, M., Hauser, L., Chain, P., et al. (2003). The genome of a motile marine Synechococcus. Nature 424, 1037–1042. doi:10.1038/nature01943
Pangestuti, R., and Kim, S.-K. (2011). Biological activities and health benefit effects of natural pigments derived from marine algae. J. Funct. Foods 3, 255–266. doi:10.1016/j.jff.2011.07.001
Parihar, M. S., and Hemnani, T. (2004). Alzheimer's disease pathogenesis and therapeutic interventions. J. Clin. Neurosci. 11, 456–467. doi:10.1016/j.jocn.2003.12.007
Partensky, F., Hess, W. R., and Vaulot, D. (1999). Prochlorococcus, a marine photosynthetic prokaryote of global significance. Microbiol. Mol. Biol. Rev. 63, 106–127. doi:10.1128/MMBR.63.1.106-127.1999
Pathania, R., Srivastava, A., Srivastava, S., and Shukla, P. (2022). Metabolic systems biology and multi-omics of cyanobacteria: perspectives and future directions. Bioresour. Technol. 343, 126007. doi:10.1016/j.biortech.2021.126007
Pekny, M., Pekna, M., Messing, A., Steinhauser, C., Lee, J. M., Parpura, V., et al. (2016). Astrocytes: a central element in neurological diseases. Acta Neuropathol. 131, 323–345. doi:10.1007/s00401-015-1513-1
Pinero, J., Bravo, A., Queralt-Rosinach, N., Gutierrez-Sacristan, A., Deu-Pons, J., Centeno, E., et al. (2017). DisGeNET: a comprehensive platform integrating information on human disease-associated genes and variants. Nucleic Acids Res. 45, D833-D839–D839. doi:10.1093/nar/gkw943
Priyadarsini, M., Raichel Nivetha, X., Mathimani, T., Anto, S., Hareesh Krishnan, H., Glivin, G., et al. (2022). Omega-3-fatty acids from algae for health benefits. Mater. Today Proc. 66, 1514–1518. doi:10.1016/j.matpr.2022.07.177
Rebhan, M., Chalifa-Caspi, V., Prilusky, J., and Lancet, D. (1998). GeneCards: a novel functional genomics compendium with automated data mining and query reformulation support. Bioinformatics 14, 656–664. doi:10.1093/bioinformatics/14.8.656
Rodolfi, L., Chini Zittelli, G., Bassi, N., Padovani, G., Biondi, N., Bonini, G., et al. (2009). Microalgae for oil: strain selection, induction of lipid synthesis and outdoor mass cultivation in a low-cost photobioreactor. Biotechnol. Bioeng. 102, 100–112. doi:10.1002/bit.22033
Roe, D. R., and Cheatham, T. E. (2013). PTRAJ and CPPTRAJ: software for processing and analysis of molecular dynamics trajectory data. J. Chem. Theory Comput. 9, 3084–3095. doi:10.1021/ct400341p
Romay, C., Armesto, J., Remirez, D., Gonzalez, R., Ledon, N., and Garcia, I. (1998). Antioxidant and anti-inflammatory properties of C-phycocyanin from blue-green algae. Inflamm. Res. 47, 36–41. doi:10.1007/s000110050256
Rosenstein, J. M., and Krum, J. M. (2004). New roles for VEGF in nervous tissue-beyond blood vessels. Exp. Neurol. 187, 246–253. doi:10.1016/j.expneurol.2004.01.022
Ryckaert, J.-P., Ciccotti, G., and Berendsen, H. J. C. (1977). Numerical integration of the cartesian equations of motion of a system with constraints: molecular dynamics of n-alkanes. J. Comput. Phys. 23, 327–341. doi:10.1016/0021-9991(77)90098-5
Sahoo, C. R., Luke, A. M., Paidesetty, S. K., and PadhyNath, R. (2021). Biogenic synthesis of silver-nanoparticles with the brackish water cyanobacterium Nostoc sphaeroides and assessment of antibacterial activity against urinary tract infecting bacteria. J. Taibah Univ. Sci. 15, 805–813. doi:10.1080/16583655.2021.2005909
Scanlan, D. J., Ostrowski, M., Mazard, S., Dufresne, A., Garczarek, L., Hess, W. R., et al. (2009). Ecological genomics of marine picocyanobacteria. Microbiol. Mol. Biol. Rev. 73, 249–299. doi:10.1128/MMBR.00035-08
Shannon, P., Markiel, A., Ozier, O., Baliga, N. S., Wang, J. T., Ramage, D., et al. (2003). Cytoscape: a software environment for integrated models of biomolecular interaction networks. Genome Res. 13, 2498–2504. doi:10.1101/gr.1239303
Sijtsma, L., and de Swaaf, M. E. (2004). Biotechnological production and applications of the omega-3 polyunsaturated fatty acid docosahexaenoic acid. Appl. Microbiol. Biotechnol. 64, 146–153. doi:10.1007/s00253-003-1525-y
Song, S., Li, B., Jia, Z., and Guo, L. (2020). Sirtuin 3 mRNA expression is downregulated in the brain tissues of Alzheimer's disease patients: a bioinformatic and data mining approach. Med. Sci. Monit. 26, e923547. doi:10.12659/MSM.923547
Srimongkol, P., Songserm, P., Kuptawach, K., Puthong, S., Sangtanoo, P., Thitiprasert, S., et al. (2023). Sulfated polysaccharides derived from marine microalgae, Synechococcus sp. VDW, inhibit the human colon cancer cell line Caco-2 by promoting cell apoptosis via the JNK and p38 MAPK signaling pathway. Algal Res. 69, 102919. doi:10.1016/j.algal.2022.102919
Stal, L. J. (2007). “Cyanobacteria,” in Algae and cyanobacteria in extreme environments. Editor J. Seckbach (Dordrecht: Springer Netherlands), 659–680.
Sweeney, M. D., Sagare, A. P., and Zlokovic, B. V. (2015). Cerebrospinal fluid biomarkers of neurovascular dysfunction in mild dementia and Alzheimer's disease. J. Cereb. Blood Flow. Metab. 35, 1055–1068. doi:10.1038/jcbfm.2015.76
Szklarczyk, D., Morris, J. H., Cook, H., Kuhn, M., Wyder, S., Simonovic, M., et al. (2017). The STRING database in 2017: quality-controlled protein-protein association networks, made broadly accessible. Nucleic Acids Res. 45, D362-D368–D368. doi:10.1093/nar/gkw937
Tai, S. Y., Chen, C. H., Chien, C. Y., and Yang, Y. H. (2017). Cilostazol as an add-on therapy for patients with Alzheimer's disease in Taiwan: a case control study. BMC Neurol. 17, 40. doi:10.1186/s12883-017-0800-y
Tai, V., and Palenik, B. (2009). Temporal variation of Synechococcus clades at a coastal Pacific Ocean monitoring site. ISME J. 3, 903–915. doi:10.1038/ismej.2009.35
Tan, H. T., Yusoff, F. M., Khaw, Y. S., Noor Mazli, N. A. I., Nazarudin, M. F., Shaharuddin, N. A., et al. (2022). A review on a hidden gem: phycoerythrin from blue-green algae. Mar. Drugs 21, 28. doi:10.3390/md21010028
Tan, L. T. (2007). Bioactive natural products from marine cyanobacteria for drug discovery. Phytochemistry 68, 954–979. doi:10.1016/j.phytochem.2007.01.012
Tanzi, R. E., Moir, R. D., and Wagner, S. L. (2004). Clearance of Alzheimer's Abeta peptide: the many roads to perdition. Neuron 43, 605–608. doi:10.1016/j.neuron.2004.08.024
Tian, C., Kasavajhala, K., Belfon, K. A. A., Raguette, L., Huang, H., Migues, A. N., et al. (2020). ff19SB: amino-acid-specific protein backbone parameters trained against quantum mechanics energy surfaces in solution. J. Chem. Theory Comput. 16, 528–552. doi:10.1021/acs.jctc.9b00591
Tikhonova, M. A., Chang, H. M., and Singh, S. K. (2023). Editorial: experimental and innovative approaches to multi-target treatment of Parkinson's and Alzheimer's diseases - volume II. Front. Neurosci. 17, 1171866. doi:10.3389/fnins.2023.1171866
Udayan, A., Arumugam, M., and Pandey, A. (2017). “Nutraceuticals from algae and cyanobacteria,” in Algal green chemistry. Editors R. P. Rastogi, D. Madamwar, and P. A. Amsterdam (Elsevier), 65–89.
Ungerer, J., Wendt, K. E., Hendry, J. I., Maranas, C. D., and Pakrasi, H. B. (2018). Comparative genomics reveals the molecular determinants of rapid growth of the cyanobacterium Synechococcus elongatus UTEX 2973. Proc. Natl. Acad. Sci. U. S. A. 115, E11761-E11770–E11770. doi:10.1073/pnas.1814912115
Uzair, B., Tabassum, S., Rasheed, M., and Rehman, S. F. (2012). Exploring marine cyanobacteria for lead compounds of pharmaceutical importance. ScientificWorldJournal 2012, 179782. doi:10.1100/2012/179782
Vijayakumar, S., and Menakha, M. (2015). Pharmaceutical applications of cyanobacteria—a review. J. Acute Med. 5, 15–23. doi:10.1016/j.jacme.2015.02.004
Wan, H. L., Hong, X. Y., Zhao, Z. H., Li, T., Zhang, B. G., Liu, Q., et al. (2021). STAT3 ameliorates cognitive deficits via regulation of NMDAR expression in an Alzheimer's disease animal model. doi:10.7150/thno.56541
Wang, J., Wang, W., Kollman, P. A., and Case, D. A. (2006). Automatic atom type and bond type perception in molecular mechanical calculations. J. Mol. Graph. Model. 25, 247–260. doi:10.1016/j.jmgm.2005.12.005
Wang, S., Ma, Y., Huang, Y., Hu, Y., Huang, Y., and Wu, Y. (2022). Potential bioactive compounds and mechanisms of Fibraurea recisa Pierre for the treatment of Alzheimer's disease analyzed by network pharmacology and molecular docking prediction. Front. Aging Neurosci. 14, 1052249. doi:10.3389/fnagi.2022.1052249
Wang, X., Gupta, J., Kerslake, M., Rayat, G., Proctor, S. D., and Chan, C. B. (2016). Trans-11 vaccenic acid improves insulin secretion in models of type 2 diabetes in vivo and in vitro. Mol. Nutr. Food Res. 60, 846–857. doi:10.1002/mnfr.201500783
Wang, X., Sun, G., Feng, T., Zhang, J., Huang, X., Wang, T., et al. (2019). Sodium oligomannate therapeutically remodels gut microbiota and suppresses gut bacterial amino acids-shaped neuroinflammation to inhibit Alzheimer's disease progression. Cell Res. 29, 787–803. doi:10.1038/s41422-019-0216-x
Wolfender, J. L., Litaudon, M., Touboul, D., and Queiroz, E. F. (2019). Innovative omics-based approaches for prioritisation and targeted isolation of natural products - new strategies for drug discovery. Nat. Prod. Rep. 36, 855–868. doi:10.1039/c9np00004f
Xie, M., Wang, W., Zhang, W., Chen, L., and Lu, X. (2017). Versatility of hydrocarbon production in cyanobacteria. Appl. Microbiol. Biotechnol. 101, 905–919. doi:10.1007/s00253-016-8064-9
Xu, F., Na, L., Li, Y., and Chen, L. (2020). Roles of the PI3K/AKT/mTOR signalling pathways in neurodegenerative diseases and tumours. Cell Biosci. 10, 54. doi:10.1186/s13578-020-00416-0
Yeo-Teh, N. S. L., and Tang, B. L. (2023). A review of scientific ethics issues associated with the recently approved drugs for Alzheimer's disease. Sci. Eng. Ethics 29, 2. doi:10.1007/s11948-022-00422-0
Yu, B., Wang, M., Xu, H., Gao, R., Zhu, Y., Ning, H., et al. (2022). Investigating the role of dahuang in hepatoma treatment using network pharmacology, molecular docking, and survival analysis. Biomed. Res. Int. 2022, 5975223. doi:10.1155/2022/5975223
Yu, J., Liberton, M., Cliften, P. F., Head, R. D., Jacobs, J. M., Smith, R. D., et al. (2015). Synechococcus elongatus UTEX 2973, a fast growing cyanobacterial chassis for biosynthesis using light and CO₂. Sci. Rep. 5, 8132. doi:10.1038/srep08132
Zeng, P., Fang, M., Zhao, H., and Guo, J. (2021a). A network pharmacology approach to uncover the key ingredients in Ginkgo Folium and their anti-Alzheimer's disease mechanisms. Aging (Albany NY) 13, 18993–19012. doi:10.18632/aging.203348
Zeng, P., Su, H. F., Ye, C. Y., Qiu, S. W., and Tian, Q. (2021b). Therapeutic mechanism and key alkaloids of uncaria rhynchophylla in Alzheimer's disease from the perspective of pathophysiological processes. Front. Pharmacol. 12, 806984. doi:10.3389/fphar.2021.806984
Zhang, Y., Xiao, W., and Jiao, N. (2016). Linking biochemical properties of particles to particle-attached and free-living bacterial community structure along the particle density gradient from freshwater to open ocean. J. Geophys. Res. Biogeosciences. 121, 2261–2274. doi:10.1002/2016jg003390
Zheng, Q., Wang, Y., Xie, R., Lang, A. S., Liu, Y. T., Lu, J. Y., et al. (2018). Dynamics of heterotrophic bacterial assemblages within Synechococcus cultures. Appl. Environ. Microbiol. 84, e01517-17. doi:10.1128/AEM.01517-17
Zheng, R., Shi, S., Zhang, Q., Yuan, S., Guo, T., Guo, J., et al. (2023). Molecular mechanisms of Huanglian Jiedu decoction in treating Alzheimer's disease by regulating microbiome via network pharmacology and molecular docking analysis. Front. Cell Infect. Microbiol. 13, 1140945. doi:10.3389/fcimb.2023.1140945
Keywords: cyanobacteria, marine Synechococcus sp., gas chromatography-mass spectrometry, volatile organic compounds, Alzheimer’s disease, network pharmacology
Citation: Xie R, Chen F, Ma Y, Hu W, Zheng Q, Cao J and Wu Y (2023) Network pharmacology‒based analysis of marine cyanobacteria derived bioactive compounds for application to Alzheimer’s disease. Front. Pharmacol. 14:1249632. doi: 10.3389/fphar.2023.1249632
Received: 12 July 2023; Accepted: 05 October 2023;
Published: 19 October 2023.
Edited by:
Budheswar Dehury, Regional Medical Research Center (ICMR), IndiaReviewed by:
Chita Ranjan Sahoo, Siksha O Anusandhan University, IndiaAnuj Kumar, Dalhousie University, Canada
Copyright © 2023 Xie, Chen, Ma, Hu, Zheng, Cao and Wu. This is an open-access article distributed under the terms of the Creative Commons Attribution License (CC BY). The use, distribution or reproduction in other forums is permitted, provided the original author(s) and the copyright owner(s) are credited and that the original publication in this journal is cited, in accordance with accepted academic practice. No use, distribution or reproduction is permitted which does not comply with these terms.
*Correspondence: Jinguo Cao, amluZ3VvY2FvQGdtdS5lZHUuY24=; Yi Wu, d3V5aUBnbXUuZWR1LmNu
†These authors have contributed equally to this work