- Department of Psychiatry, Virginia Institute for Psychiatric and Behavioral Genetics, Virginia Commonwealth University, Richmond, VA, USA
There has been a surge of interest in recent years in incorporating genetic components into on-going longitudinal, developmental studies and related psychological studies. While this represents an exciting new direction in developmental science, much of the research on genetic topics in developmental science does not reflect the most current practice in genetics. This is likely due, in part, to the rapidly changing landscape of the field of genetics, and the difficulty this presents for developmental scientists who are trying to learn this new area. In this review, we present an overview of the paradigm shifts that have occurred in genetics and we introduce the reader to basic genetic methodologies. We present our view of the current stage of research ongoing at the intersection of genetics and social science, and we provide recommendations for how we could do better. We also address a number of issues that social scientists face as they integrate genetics into their projects, including choice of a study design (candidate gene versus genome-wide association versus sequencing), different methods of DNA collection, and special considerations involved in the analysis of genotypic data. Through this review, we hope to equip social scientists with a deeper understanding of the many considerations that go into genetics research, in an effort to foster more meaningful cross-disciplinary initiatives.
Introduction
Recent years have been witness to a surge of interest in incorporating genetic components into on-going longitudinal developmental projects and related psychological studies. This has likely been a product of many factors: Genotyping costs have fallen rapidly, and numerous commercial options for obtaining genotypes are available. It is increasingly easy to obtain DNA, with options such as cheek swab and saliva sample kits being commercially marketed and low burden on participants. Further, there has been rapid growth in the field of genetics. The revolutions and advances that the field has experienced in recent years have brought genetics to the forefront of science. Media attention to these advances, including the highly publicized mapping of the human genome, has raised public awareness about the importance of genetics. Genetics has been at the center of major NIH funding initiatives, and the current Director of NIH, Francis Collins, is the former Director of the Human Genome Project.
Developmental science has not been immune to this revolution. In contrast to the field of ethology, which has a rich tradition of examining of the genetic underpinnings of innate animal behavior (i.e., instincts), the study of genetic influences on human behavior was originally limited to the fledgling field of behavior genetics, which met with great resistance for arguing that behaviors widely “known” to have environmental etiologies (e.g., schizophrenia was caused by bad parenting) were under genetic influence. But genetic influences were not measured in these early behavior genetic studies, rather, they were inferred using family, twin, and adoption designs, by testing for similarity across different types of relatives with different degrees of genetic and environmental overlap. This meant that to study genetic influences on behavior required special study designs and methodologies not widely employed by the broader psychological or developmental community. All this has changed now that it is possible to genotype specific genes and incorporate this information into any on-going study. This has made genetics accessible to mainstream developmental science. This molecular advance occurred in concert with growing recognition by social scientists of the importance of genetic predispositions. As behavior genetic studies increasingly acknowledged the complexity of genetic influences on behavior – that genes are not destiny; that genetic effects are dynamic, changing across development and in conjunction with the environment – there was a newfound consilience between genetics and traditional developmental science. These practical and theoretical shifts have contributed to an exponential increase of studies that incorporate genetic components. Consider that a search for “genetics” in PsychNet yields 26,192 hits for the years 2000–2010, as compared to just 7575 hits for 1990–1999, a more than three-fold increase! In fact, the >26,000 publications from the past decade account for nearly 60% of the total number of hits for “genetics” listed in the entirety of PsychNet.
While obtaining DNA and producing genotypes is now easy, doing the best and most current genetics research is not. Much of the research on genetic topics in developmental science does not reflect the latest developments or most current practice in genetics. This is likely a product of many factors. Many PIs on developmental projects do not have formal training in genetics. In addition, the techniques and practical methodology in genetics are in a period of particularly rapid change and it is difficult to stay abreast of what is considered state of the art. This is true even for specialists, so it is a formidable challenge for individuals who are new to the area, and even within the field of genetics there are differences of opinion as to what strategies are best in a given situation.
In this paper, we provide a guide for how to do “good genetics research.” The authors on this paper come from a diversity of backgrounds, with Danielle M. Dick trained in clinical psychology (behavior genetics) and statistical genetics, Shawn J. Latendresse trained in child development and statistics, and Brien Riley trained in molecular genetics; yet we all have interest in understanding how genetic and environmental influences impact behavioral outcomes, and we work together at an interdisciplinary institute that takes advantage of complementary expertise. We recognize that with the rapid pace at which genetics advances, this review is likely to become dated. However, we hope that our presentation of many of the basic tenets of genetics will give the reader an appreciation of the complex issues that surround studying genetic influences on behavior. We note that we refer alternately to “developmental scientists” or “psychologists” as our target audience for this review, but in actuality the information is relevant to any social scientist with interest in adding a genetic component to an on-going project.
This paper is organized into a variety of sections. We start by presenting an introduction to the paradigm shifts that have occurred in genetics in the past decade, in an effort to provide some historical context of the field. We introduce the reader to the basic methodologies of both linkage and association and the advantages and disadvantages of each. This is not intended to be an exhaustive or thorough coverage of these methodologies, but rather a very basic overview of different strategies. More exhaustive books on these topics exist (Neale et al., 2008). We present our view of the current state of research ongoing at the intersection of genetics and social science, and we provide recommendations for how we could do better. We also address a number of issues that social scientists face as they integrate genetics into their projects, including choice of a study design (candidate gene versus genome-wide association versus sequencing), different methods of DNA collection, and special considerations involved in the analysis of genotypic data. Through this review, we hope to equip social scientists with a deeper understanding of the many considerations that go into genetics research, in an effort to foster more meaningful cross-disciplinary initiatives.
Basic Genetic Methodologies
Molecular genetic studies depend critically on genetic markers, sites in the human genome where the underlying DNA sequence varies between individuals. Genetic markers include sites where different numbers of repeated bases distinguish individuals and the allele is actually of different length (e.g., ACACAC versus ACACACAC), single bases which differ between individuals (e.g., a C on some chromosomes and a T on others), among many kinds of variation. Each different version of sequence at these sites is called an allele, and what molecular genetics generally seeks to do is to identify sites where alleles (and hence positions in the genome) co-occur with disease (or other trait measurement).
Linkage
The paradigm that originally dominated human gene finding efforts was that of linkage analyses. Linkage studies ascertained families with multiple members affected with the disorder of interest. Highly polymorphic genetic markers (those having many alleles) were genotyped across the genome. Usually around 400 of these highly variable markers were genotyped across the genome. The basic technique compared whether affected individuals in a family were more likely than expected by chance to carry the same version of that genetic marker. If so, this suggested that there was a gene (or genes) in that chromosomal region that was related to susceptibility for the disorder. Ming Tsuang, a senior leader in the field of psychiatric genetics, once gave the analogy that gene finding is like hunting for a criminal. You start out with the entire world (genome) to search in order to find your criminal (gene). Finding linkage to a particular chromosomal region is like someone giving you a zip code in which your criminal resides – you still do not know exactly where he is; you still have a lot of houses to search (individual genes in the linkage region) before you find him (the susceptibility gene). But when you started out with the world, narrowing it down to a zipcode is an exciting step forward in the search!
We review this methodology here for sake of completeness, as it dominated the field of genetics for many years. It is now recognized that linkage analyses were underpowered to detect common alleles with small individual effects on the outcome of interest. Although rare alleles with larger effects do seem likely to contribute to some common diseases, common alleles of small effect are generally believed to constitute the genetic influences on most complex behavioral outcomes. However, the use of linkage evidence in conjunction with other kinds of genetic evidence has proven to be a useful strategy in gene identification in some projects (Dick and Bierut, 2006). There are situations where linkage analyses may be more powerful than association analyses (discussed below), such as when many different alleles (e.g., individual disease-predisposing or causal mutations) are present in a single gene. In addition, linkage analyses may have some utility for finding the rare variants mentioned above. It remains to be seen whether multiply affected families have substantially different kinds of genetic risks than unselected cases from the population.
Association
Association analyses are the methods most commonly employed in gene identification efforts today, and the methodology that has been widely incorporated into developmental research. Association analyses are straightforward to understand; they basically test whether a particular genetic variant is more likely to be found in affected individuals than in unaffected individuals (in a case–control design; Figure 1), or more likely to be transmitted to affected children (in family-based designs; though these are increasingly less common since population stratification concerns have been alleviated by genome-wide data, as described below). Association relies on genotyping genetic markers, in the same way that linkage analyses did. The major difference between linkage and association methods is that linkage is a within-family statistic, whereas association is a between-family statistic. In linkage, this meant that the same marker had to be shared across affected members within a family, but it could be a different marker from one family to the next. Association analyses, on the other hand, require that it is the same marker that is shared across all affected individuals in the sample. Linkage analyses only point to a general chromosomal region that may be involved in the outcome, whereas association analyses more narrowly implicate a specific gene or very small region.
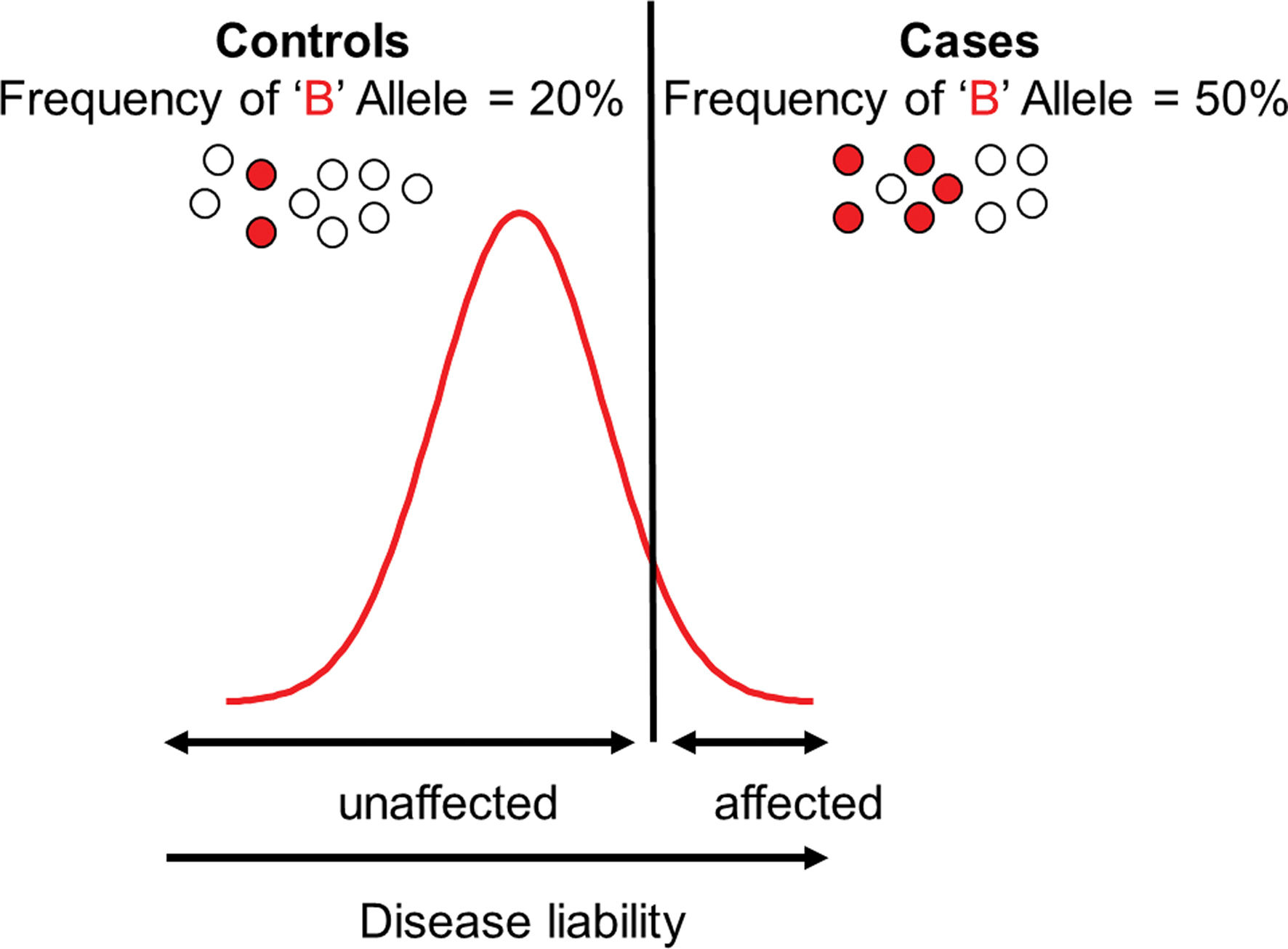
Figure 1. Allele frequency differences between cases and controls as defined by the liability threshold model. The white circles refer to the null allele, and the red circles refer to the risk (‘B’) allele. The cases are above the threshold for disease on the liability distribution and are more likely to carry the risk allele than the controls.
There is another important distinction that must be made in association analyses, that of direct versus indirect association. This distinction is critical and, in the authors’ experience, often lost or ignored in studies incorporating genetic components in fields outside genetics. Direct association arises because the genetic variant that is associated with the disease/outcome has a functional consequence and is directly involved in susceptibility or outcome. Indirect association arises when a genetic variant is associated with disease/outcome because it is very close to the causal variant, but the associated variant is not itself causal in any way. Indirect association results from a phenomenon called linkage disequilibrium (LD). This refers to the fact that an individual’s genotype at any one location is not independent of what their genotype will be at nearby locations in the genome. In other words, genetic variants that are nearby are often correlated. To the degree that two variants are correlated, knowing one’s genotype at one location also tells you something, albeit probabilistic, about what their genotype will be at the other location. This is because any time a mutation (a new variant in the human genome) occurs, it occurs on a specific chromosome background. Thus, at the time it occurs the new variant is perfectly correlated with all the other variants that exist on that chromosome. As the chromosome containing the mutation is transmitted to offspring across several generations, recombination will cause alleles at marker loci that are further from the mutation to be interchanged. This is because genetic variants that are further apart are more likely to be broken up due to recombination events. Genetic variants that are closer together are far less likely to have a recombination occur between them, leading to a higher correlation between the alleles at those nearby marker locations. Accordingly, the associated genetic background surrounding the mutation will become progressively smaller (Figure 2). In general, the closer the marker is to the novel variant, the stronger the LD will be (Jorde, 1995), although there is not a perfect correspondence between distance and correlation. Some regions of the genome are more likely to have recombination events (these are called “hotspots”), leading to lower levels of LD between nearby markers. Accordingly, some stretches of the genome have high LD occurring over long distances, whereas other regions of the genome have very low LD.
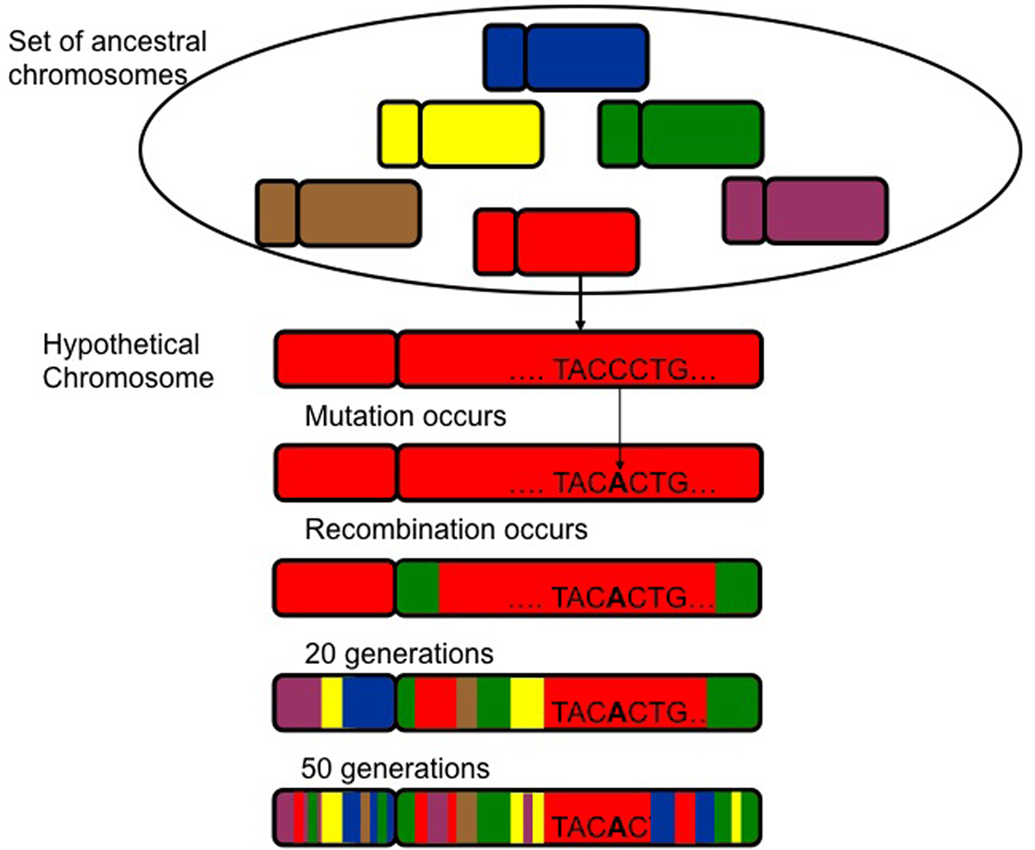
Figure 2. The preservation of linkage disequilibrium (LD) through mutation and subsequent recombination in the human genome. At the time the mutation occurs it is in LD with the genetic background of the chromosome. Recombination changes the genetic background as variation is introduced until only the DNA sequence very near the mutation remains in LD.
The existence of LD means that we do not actually have to genotype the causal locus to detect association; we simply have to genotype variation very near the causal locus. In practice, it is exceedingly difficult to determine whether an association signal results due to direct or indirect association, though the default assumption must be that it is indirect. First, most genetic variants (like the alleles of most markers we study) have no obvious functional significance. Only ∼2% of the genome represents genes, and the exons (segments of coding information) are interspersed with often much larger introns (segments within the gene that do not carry coding information) so only ∼1% of the DNA in the genome actually codes for functional molecules. Thus, the chance of a variant impacting the function of a key molecule is small. Second, this difficulty is compounded by the expectation that most of the alleles influencing traits common in the population are common and of relatively small effect (i.e., account for only small quantities of the total trait variance). Such alleles are unlikely to have major effects on the genes in which they lie and thus are unlikely to be obviously functional (unlike the rare mutations that account for rare, strongly genetic diseases like cystic fibrosis or Huntington’s disease). Thus, the absence of obvious functional significance does not mean that a variant can be assumed NOT to impact the trait. Knowing whether an associated variant is causal takes considerable additional testing, and often claims of functionality are controversial and contradictory.
The Current State of Research at the Interface of Genetics and Social Science
So why do developmental scientists need to understand concepts such as recombination and LD? Most developmental scientists will never conduct a linkage study and may never run a genome-wide association studies (GWAS; though the latter is becoming increasingly unlikely due to falling genotype costs, as detailed further in section “What Should We Be Doing?”). Not every scientist interested in genetic influence needs to be engaged in gene finding studies. However, there are still tremendous contributions that developmental scientists can make to understanding how genetic influences impact behavior. Most gene identification efforts continue to focus on adult psychiatric diagnoses, for many justifiable reasons, such as diagnostic reliability across the many sites necessary to obtain large enough numbers of individuals to have power to identify genes of small effect. But because the focus of gene identification is often with a static, distal outcome, this means that developmental scientists have much to offer in terms of further characterizing the risk associated with identified genes, studying how genetic risk unfolds across development, the mediating processes by which risk unfolds, and what environments moderate risk among those carrying genetic susceptibilities. The importance of this line of research cannot be understated, and many projects of this sort are underway. However, the vast majority of extant studies by developmental scientists continue to focus on a small number of purportedly functional polymorphisms from a small handful of genes. The literature is dominated by studies of purportedly functional polymorphisms in the serotonin transporter gene (5HTTLPR), the monoamine oxidase A gene (MAOA-LPR), and the dopaminergic receptor gene DRD2 and adjacent region (TaqI A). It is nearly impossible to believe that, with nearly 4.5 million validated polymorphic positions currently identified in the human genome, those can be the only ones of interest for developmental science! Rather, these polymorphisms rose to prominence based largely on chance – they were polymorphisms discovered early with preliminary data suggesting that they altered function in candidate genes thought to be of interest for relevant behavioral phenotypes. It is certainly not our intention to argue that these genes are not of potential relevance, rather, we argue that with everything we now know about genetics, focusing only on these genes, and only on these widely studied polymorphisms within these genes, does not take advantage of the progress that has occurred in the field of genetics.
What should We be Doing?
Genotyping a single marker in a gene of interest no longer reflects current practice in genetics. The field of genetics has been revolutionized by the discovery and mapping of genetic markers across the human genome. In October of 2002, the International HapMap Project1 was initiated as a collaboration among scientists across multiple countries with the goal of developing a haplotype map of the human genome to describe the common patterns of human DNA sequence variation. With data from the HapMap project, we now know something about the LD structure (i.e., correlation pattern across alleles) for most genes in the human genome (Manolio et al., 2008). Further, there are many polymorphic markers available across most genes of interest. It is possible that multiple polymorphic sites exist in a gene that lead to differential function of that gene and contribute to differential susceptibility to an outcome (McClellan and King, 2010). This is already well-known in Mendelian traits, where >1000 different mutations have been identified in the gene causing cystic fibrosis.
We illustrate the importance of genetic coverage using the example of the Taq1A allele. This polymorphism was originally thought to be in the DRD2 gene, and has an extensive literature of reported associations (and failures to find association) with a number of phenotypes related to substance use, smoking, and a variety of other phenotypes related to impulsivity (Noble, 2000; Dick et al., 2007c). Figure 3 shows a screenshot of output from the program Haploview (Barrett et al., 2005), which uses freely available data from the HapMap project to illustrate the LD structure of the chromosomal region surrounding the DRD2 gene. Along the top of the figure is the base pair position of the chromosomal region, as listed in kilobases (i.e., 1000 nucleotide bases) to give an idea of scale. Directly underneath, the triangles indicate SNPs that were genotyped in the samples on which the Haploview data is based. The SNP highlighted in green is the rs1800497 marker commonly referred to as DRD2 Taq1A. Beneath the SNPs, the genes in the region are listed. The length of the line reflects the length of the gene. One will note that the Taq1A allele is actually located in a small gene next to DRD2 called ANKK1. It is not located in DRD2 despite the large literature making claims about whether DRD2 was involved in many different phenotypes of interest based on genotyping at this marker. Below the genes is the LD plot, where shading indicates the degree of correlation between markers (shown here as the small hash marks at the top of the figure) as measured by D’ (Hedrick and Kumar, 2001), with darker red shading indicating higher correlations; blue or white shading indicates the markers are unlinked or uncorrelated. What stands out is the block-like correlational structure, yielding inverted red triangles (i.e., “blocks”) comprised of groups of SNPs where there is high LD across that group of SNPs and low LD with surrounding SNPs located outside the block. This block-like structure is observed throughout the genome (Gabriel et al., 2002). Knowing the correlational pattern is critical for the selection of markers and the interpretation of genetic association results. For example, DRD2 spans at least two blocks on the attached figure. If different studies genotyped SNPs from different blocks, they could reach different conclusions about whether “DRD2 was associated” with outcome, depending on the location of the marker they chose and where the actual associated SNP was. Further, also note that the LD block that contains Taq1A spans the genes DRD2, ANKK1, and TTC12. This makes it very difficult to know which gene is actually important for an observed association, since the correlational structure means that a signal could originate from any gene in the correlated set (or LD block). More extensive genotyping across these genes, and the other gene located very nearby, NCAM1, has suggested that the association with substance use phenotypes extends to multiple genes in this region This underscores the necessity of understanding genomic structure in order to evaluate the role of hypothesized genes of interest. In the field of genetics, we would never test a single marker in a gene in order to make conclusions about the relevance of that gene for a given genotype. It would be the equivalent of asking a single question about whether a participant got along with his/her mother as a child, and then using the results from that variable to make conclusions about whether the home environment played a role in the outcome of interest. A developmental scientist would rightly argue that such an analysis was at best naïve, and at worst, close to meaningless, and geneticists have ignored much of the literature on genetic associations deriving from the labs of social scientists for similar reasons.
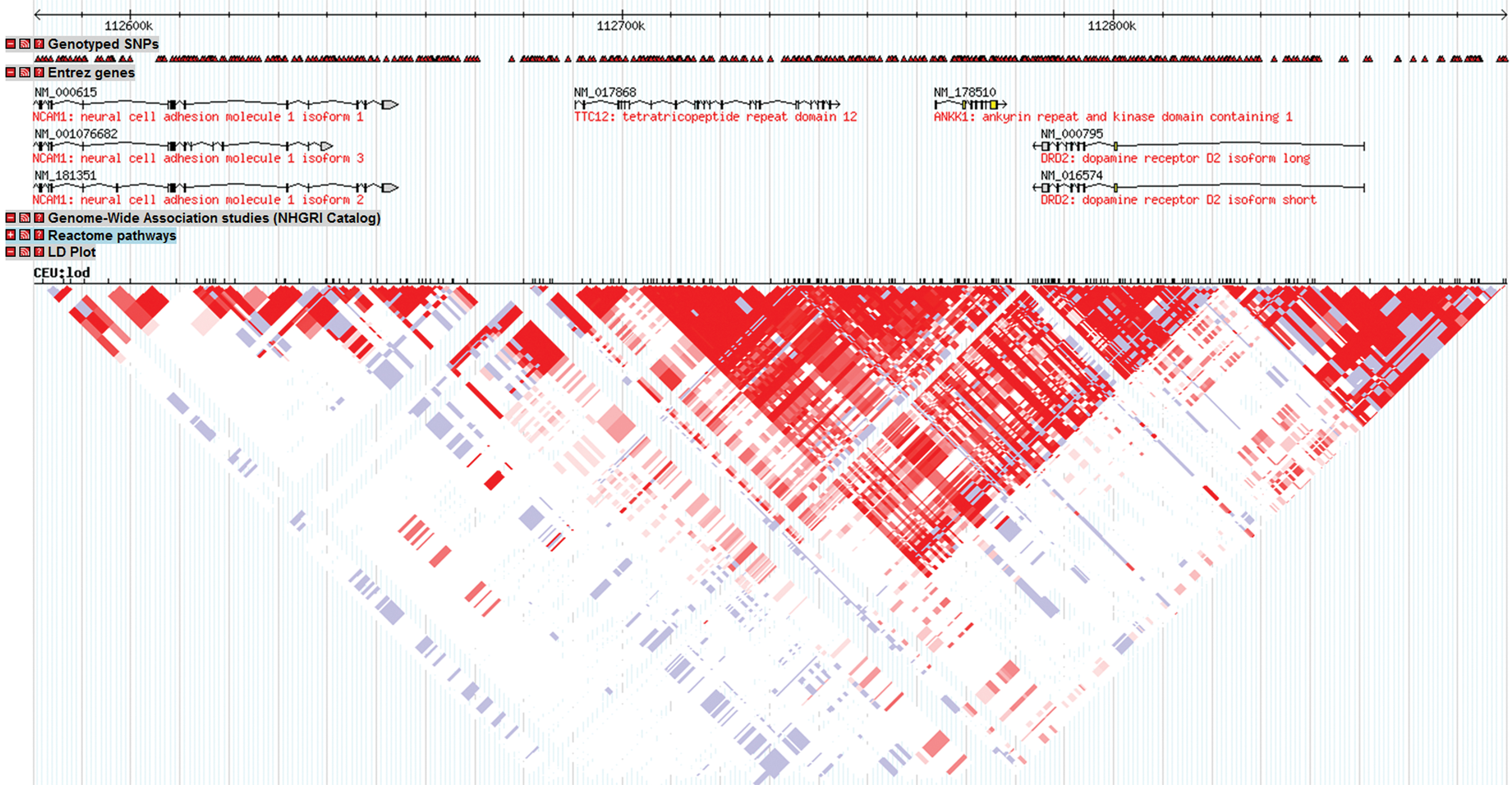
Figure 3. Screenshot from Haploview (Barrett et al., 2005) showing the linkage disequilibrium structure surrounding the DRD2 gene.
In the same way that developmental scientists pay careful attention to the measurement of their outcomes of interest and potential environmental factors of relevance, the same care must be taken in characterizing genes of interest when research programs are expanded in this direction. The genetics research being carried out by developmental scientists should be of the same caliber as that being conducted in other areas of genetics, and it must keep up with the rapid advances going on in that field. Otherwise it will not be taken seriously. This does not mean that all developmental scientists need to be “gene-finders” or to carry out GWAS. But it does mean that anyone involved in this kind of research should understand the complexities of studying genetics and be connected to the latest developments in genetics. Because of the rapid pace at which the field of genetics moves, this necessitates having collaborators who are tied more centrally to the world of genetics, and/or (for the younger generation of social scientists with interest in this area) to obtain focused training in genetics, ideally through a post-doctoral training experience. With ~25,000 genes in the human genome2, thousands of genetic association papers published, and GWAS papers being turned out every day, it impossible to believe that the handful of “usual suspects” are the only genes of interest for developmental outcomes. As large-scale gene finding studies continue to report associations with new and novel genes of interest, these genes too deserve further study by developmental scientist to delineate the trajectories of risk associated with these genes.
Study Design: Candidate Gene? GWAS? Sequencing?
The two primary association strategies currently employed are candidate gene studies and GWAS. Candidate gene studies are driven by theory and focus on a specific gene because it is believed to be involved in the underlying biology associated with the behavior/disorder. The primary drawback to the candidate gene approach is that it is limited by our knowledge of etiological processes, which is often very incomplete when it comes to psychiatric disorders and related behavioral outcomes. Candidate gene research fell out of favor with the advent of GWAS. The primary advantage of GWAS is that this approach is not limited by our knowledge of the underlying biology of behavioral disorders; that is, GWAS is an atheoretical approach and since the whole genome is assayed for effects, there is no selection bias. GWAS have the ability to find novel susceptibility genes that, in turn, can help advance our understanding of the etiology of the trait or disorder. Several different companies now offer GWAS “chips” that have a predefined set of genetic markers that cover genetic variation across the genome. Although the exact markers on the chips vary and companies have used different approaches to select markers, the basic idea is to cover genetic variation across the genome using knowledge about LD patterns (as described above). Through the known LD in the genome, chips with ~1 million markers arrayed on them can track genetic variation down to minor allele frequencies of about 5%, while larger arrays of ~2.5 million markers can track alleles down to about 1% frequency in the population. In this way, there is fairly good coverage of most genes across the genome, as well as intergenic regions (which may contain regulatory elements or have other unknown functions). Another advantage of GWAS is that it provides a built in method to test for genetic background and control for potential population stratification. Population stratification can occur when there are different background allele frequencies and different trait means or prevalences as a function of population membership. In GWAS, having such a large number of markers tested across the genome provides a way to genetically determine ethnic background, so that this information can be used as a covariate in the association analyses, alleviating potential population stratification concerns.
There are caveats to GWAS. The most widely used platforms do not do a good job of covering rare variants (genetic markers with minor allele frequencies less than 1%). In addition, LD patterns are population specific. To address this issue, the HapMap project analyzed DNA from populations with African, Asian, and European ancestry. However, GWAS platforms differ in how well they cover genetic variation for different populations, so this must be considered when analyzing any particular gene (or the genome) because it can affect how good the coverage is in your sample depending on its racial composition. GWAS are also complicated by the fact that multiple testing corrections must be applied when conducting such an incredibly large number of tests (e.g., for 1 million markers across the genome), and there is differing opinion about how best to do that (van den Oord, 2007). This issue will be discussed further in section “Analyzing Genetic Data.”
So which strategy is best? Candidate gene or GWAS? This question is frequently posed by developmental scientists aiming to add genetic components to their studies (or submitting grants to do so for NIH review!). There is no easy answer. Most developmental scientists are not trying to discover new genes, as is generally the goal of GWAS. Thus, a more targeted candidate gene approach that aims to characterize risk processes and/or trajectories associated with identified genes is reasonable. However, we strongly recommend that this targeted approach is not restricted to the “usual suspects,” but rather, draws from the broader literature of genetic findings for the phenotype of relevance, and ideally, includes collaborators from large-scale gene identification projects in relevant fields, in order to take advantage of new findings emerging in the area. Further, with all the knowledge we now possess about LD structure, researchers should use this information to genotype markers that capture genetic variation across the gene of interest, not limit their study to a single marker or polymorphism (except in strongly justified situations, such as where previous studies that have done exhaustive genotyping consistently implicate a particular marker or region of the gene). If a researcher is interested in understanding whether a particular gene is involved in a given outcome, (s)he should do a thorough job of characterizing the genetic variation across that gene. However, issues associated with multiple testing will need to be considered when analyzing the data, as discussed below.
The decision between candidate gene and GWAS is complicated by the logistics of genotyping. Genotyping is far more efficient and low cost when conducted on a large scale. Accordingly, the cost per genotype falls exponentially as the number of genotypes increases. High throughput genotyping using GWAS arrays is 1000 times more cost-efficient than small scale genotyping. Thus, even if a social scientist has no interest in analyzing all 1 million markers across the genome, it may be more cost-efficient to genotype a GWAS chip on all participants, so that genotypes are available across the a priori genes of interest, as well as for future genes of interest that may emerge. This also facilitates collaborations and attempts at replication, since genes of interest from different groups will already be available in the proverbial “GWAS bank.” For this reason, it may be reasonable and cost-efficient to genotype a GWAS chip, even if a more targeted candidate gene strategy is proposed. Currently, GWAS chips cost approximately $200 to purchase and $100 to process, which may still make them out of reach for many social science projects where genotyping is not the primary focus. However, as costs continue to drop, the cost-benefit ratio may shift in favor of running GWAS chips. There are also custom chips available, which may provide an interim compromise of genotyping on a larger scale and covering far more candidate genes in a more cost-efficient manner than genotyping only a few candidates. But this still requires the selection of candidate genes, and does not have the advantage of genotyping across the genome for future collaborative purposes.
Next-Generation Sequencing
There is mounting evidence that some proportion of risk for a variety of common traits is due to rare, possibly more deleterious genetic variation. This is in contrast to the predominant viewpoint of the past several years in which common complex phenotypes were thought to result from fairly common genetic polymorphisms; this was referred to as the common disease – common variant hypothesis. The ability to consider the impact of rare variants has been aided by technological developments. The single most important technological development in studies of genetic disease in recent years is the revolutionary change in the way human sequence data are generated. Whereas genetic techniques were previously limited to genotyping specific markers, these new methodologies make it possible to generate “sequence” data, in other words, the actual sequence of nucleotides that make up an individual’s DNA. Not surprisingly, this approach is fundamentally changing the way genetic disease studies are pursued. Moreover, despite the fact that sequencing an entire genome is still prohibitively expensive (i.e., ~$16,000 per individual), whole genome sequencing is set to become the standard as costs continue to fall with increasing instrument output. In the meantime, intermediate sequencing techniques are being pursued, such as sequencing only the exome, the set of all exons that code for proteins (which constitutes ~34 million bases, or ~1% of the total sequence).
A more general targeted sequencing approach focuses on genes or regions well-supported (e.g., by GWAS) for involvement in a trait or disease, but is not generally limited to the coding sequence within the target region. Well-supported regions have been observed in a variety of important traits like type 2 diabetes and Crohn’s disease, and to a lesser extent in schizophrenia. In traits for which extensive sequence data have already been generated, excess rare variation has in fact been detected in cases compared to controls, or in individuals at different points on a quantitative trait distribution. This provides support for the idea that there may be very rare genetic variants that affect common phenotypes, like the type social scientists are interested in.
Overall, however, these technologies are in early stages of general application to human diseases, with a number of technical and analytic issues still needing to be worked out. As such, the kinds of variation contributing to individual differences in variables of interest to the social scientist remain poorly understood and sequencing designs are generally not appropriate currently for most such studies, unless there is substantial evidence of the kind detailed above supporting strong genetic effects or strong target regions. But this next generation of genetic techniques are changing the way we think about the many types of genetic variation that can affect behavior, so we include mention of them here to make the social scientist aware where the field of genetics is currently headed.
Other Considerations for the Social Scientist
Different Methods of Collecting DNA
DNA collection methods are distinguished primarily on the basis of cost and sample quality, with a generally inverse relationship between the two. The cheapest way of obtaining DNA samples is to use buccal swabs, brushes or cotton buds that collect cells from the inside of the cheek. These have a long shelf life and can be mailed in large numbers very cheaply. However, they have the distinct disadvantage of yielding the lowest quality, most degraded genomic DNA because the collected cells have been sloughed off by the cheek epithelium and the DNA has been partially degraded by natural processes. Although GWAS using buccal swab samples have been reported, these samples generally are not of the quality needed for the most intensive contemporary applications, like GWAS and sequencing. The enhanced sample quality requirements for these applications argues strongly for the use of higher quality sampling methods.
Collection of DNA from saliva samples is an intermediate cost sampling method ($15–20 per subject) that can still be inexpensively posted to participants. This approach yields high quality DNA suitable for most applications. The saliva samples themselves are stable for at room temperature for 6–12 months. Both buccal swab and saliva sampling have the advantage of being non-invasive which increases participation rates.
Next in terms of rising cost ($100 per participant) and quality is to sample whole blood. The total yield of DNA from one such sample can last a laboratory for many years, even with fairly intensive use (i.e., lots of genotyping). Most critically, blood samples are the only sample source which can, if the additional costs are available, be transformed to yield immortalized cell lines. These cell lines provide a long-term source of DNA, RNA, protein or cell lysates for future work. They also require regular maintenance by skilled staff. Although this final step means the samples from a project will last for as long as they are properly maintained, it is probably not viable for most social scientists unless they have access to either a core facility or a collaborating lab.
Analyzing Genetic Data
Beyond standard quality control protocols administered in the wet lab, genotypic data requires the same sort of pre-analysis cleaning procedures that social scientists would apply to any phenotypic or environmental data. For example, one would want to assess whether willingness to provide a DNA sample is systematically associated with the non-genetic variables of interest (i.e., are the participants who gave DNA different in any way). Likewise, it is important to verify that the values corresponding to each of the alleles reflect viable nucleotide bases; that is, at a locus polymorphic for adenine and guanine, none of the study participants should have a value representing cytosine. In addition to these generic practices, genotypic data should also be screened to determine whether the observed distribution of genotypes differs from that which is expected, given the allelic frequencies observed within a given population – in other words, do the genotypes in your sample deviate from Hardy–Weinberg equilibrium (HWE)? In the simple case of a bi-allelic variant, like a SNP, the expected distribution of genotypes is easily derived via a Punnett square, and HWE can be tested using Pearson’s Chi-square. In contrast, examining polymorphisms with more variants and/or in smaller samples might require use of Fisher’s exact test. Moreover, departures from HWE can result from a variety of population level disturbances that are out of the researchers’ control (assortative mating, selection, mutation, and migration). It should be noted, however, that studies which oversample for specific traits or disorders (e.g., case–control designs) will also, to the extent that they examine genetic variants associated with those characteristics, yield deviations from HWE.
Once the data have been cleaned and deemed ready for use, genotypes can, for all practical purposes, be incorporated into standard analytic frameworks (e.g., regression-based analyses). However, a critical point is that, unlike many variables that are modeled by social scientists, how a genotype is coded infers something about the biological risk (i.e., the underlying genetic model). For example, genetic markers may influence a particular phenotype in a simple linear manner, where each copy of a “risk” allele confers an equivalent cumulative (i.e., additive) effect. In this sense, individuals with two copies of the allele at a given SNP are presumed to have twice the risk of those with single copy, relative to those with 0 copies. Alternately, the influence of genotype might also function in a dominant (one or more copies of the risk allele is sufficient to produce the outcomes, with an equivalent phenotype across those with one or two copies of the risk allele) or recessive (two copies of the risk allele are needed before the phenotype is manifest, with individuals having 0 or 1 copy showing an equivalent phenotype) manner. These genetic models would necessitate a different coding scheme for the marker. Atheoretical approaches like GWAS traditionally model additive genetic influences, such that each copy of a specific variant is assumed to have the same magnitude of effect. In doing so, genotypes (e.g., AA/AG/GG) are coded 0, 1, or 2, with respect to a reference variant (in this case, G). In the absence of biologically plausible hypotheses and/or robust empirical evidence to the contrary, the analysis of candidate genes should generally start with an additive model. It would not be advisable, for instance, to arbitrarily collapse across genotypes solely for the purpose of increasing the statistical power of a model to detect significant associations. However, with the right justification, alternative coding schemes can be introduced. For example, if the literature on a specific SNP strongly suggests that the mere presence of a particular allele confers risk, the genotype may be coded to reflect the dominant influence of that allele (i.e., absence versus presence, irrespective of the number of copies). By contrast, researchers interested in determining the extent to which an allele operates in a non-additive manner could pair the additive term described above with a term differentiating heterozygous from homozygous genotypes, though this model would require an additional degree of freedom. What’s most important to remember is that these decisions should be based on prior research findings, biologically based theory, and in consultation with individuals who have formal training in genetics. Moreover, regardless of how the data get coded, the degree of association between the outcome of interest and a set of genetic variants should roughly map onto the pattern of LD across those variants. That is to say, if you are testing a group of highly correlated SNPs, you would expect a similar association pattern across them. In contrast, if SNPs are located in different LD blocks (i.e., have a low correlation), it would not be concerning if they yielded different association results.
Two remaining points have to do with the aforementioned issues of statistical power and multiple testing. Statistical power should be the foremost consideration of any proposed genetic association study, with sample sizes assembled accordingly. Although power derived from large samples is necessary for the analysis of complete genome-wide data because of the number of individual tests performed, it is also worth noting that many studies of candidate genes are also underpowered to detect effects of the sizes currently expected on the basis of GWAS data from other behavioral or psychiatric phenotypes (allelic odds ratios [OR] in the range of 1.1–1.15). As such, social scientists should be aware that statistical power in genetic association studies is a function of several elements of the research design including, but not limited to, the coding of phenotype (e.g., case–control versus continuous outcome) and genotype (e.g., additive versus dominant or recessive modes of inheritance), minor allele frequency (MAF), type I error rate, and the hypothesis under consideration (i.e., genetic main effect, gene–environment interaction, or even gene–gene interaction). To illustrate, let us consider how several of these factors might influence the sample size required to replicate the effects of a putative SNP with association with major depressive disorder, a phenotype of interest to many social scientists which affects ~6.7% of adults in the U.S. population in a given year (Kessler et al., 2005). We will begin by setting our minimum acceptable thresholds for power at 0.80 and type I error rate at 0.05. Given the dichotomous nature of the phenotype, we will further assume a 1:1 matched case–control design, and start with a baseline model for a genotypic main effect that reflects an additive mode of inheritance, a MAF of 0.1, and an OR of 1.15. To detect this effect under the circumstances described above would require 4261 participants, half cases and half controls. Holding all else constant, hypothesizing a dominant mode of inheritance would require 5037 participants, whereas reducing the frequency of the risk allele by half (i.e., 0.005) would require 8021 participants. Similarly, to be able to detect an effect that is somewhat smaller in magnitude (e.g., OR = 1.1), though still very much in line with those frequently reported in the literature, would require 9296 participants. In contrast, to detect an effect of that same SNP (MAF = 0.1) accounting for 1% of the variability in a continuously distributed indicator of depression within a community sample would only require 781 individuals total. Thus, when estimating power for relatively straightforward designs, we suggest that social scientists utilize some of the free power calculation software that is available on-line (e.g., Quanto3).
Finally, a central issue in the field of genetic association studies is the lack of consensus on how to deal with multiple, non-independent analyses. Because there is likely to be LD between many of the individual markers examined, a standard Bonferroni correction (which assumes complete independence) could mask the existence of some, if not many important associations. As such, statistical geneticists have developed a number of alternative strategies for taking these dependencies into consideration (see Ziegler et al., 2008 for a recent review). One fairly common approach is to use the existing LD structure to estimate the number of “independent” effects represented by a set of SNPs (Nyholt, 2004). That is, the estimated number of effects is used as the denominator in a modified Bonferroni correction. A software package for this purpose, SNPSpD, is freely available on-line4. Still, since there is no agreed upon gold standard for dealing with multiple testing, it is probably most important for researchers to present sufficient information for a diverse audience to be able to assess the approach taken, and the interpretation proffered.
Additional Challenges and Considerations
This review has largely focused on basic genetic concepts that are critical for social scientists to understand when incorporating genetic components into their research. But we would be remiss to leave the reader with the impression that teaming up with geneticists will provide clear answers about the way forward. The rapidly changing landscape in the field of genetics and constant advances in the tools used for gene finding have raised nearly as many questions as they have provided answers. GWAS have not been wildly successful in psychiatric and behavioral phenotypes, raising question about why this is the case, as other common complex, polygenic disorders have enjoyed greater success (e.g., with GWAS mapping many novel loci for Crohn’s disease and type 2 diabetes; McCarthy et al., 2008). However, even in disorders that have enjoyed more success in mapping risk loci, the variants that have been identified only account for a very small fraction of the heritability. This has been called the “missing heritability problem” (Manolio et al., 2009), and several potential explanations have been put forth for this phenomenon, including the potential importance of rare variants and/or structural variants (genomic changes such as insertions, deletions, inversions, and translocations of stretches of genetic material), neither of which are well captured by current GWAS platforms. In addition, there is the possibility of gene–gene and/or gene–environment interactions. The very small effect sizes associated with identified variants have led to the need for increasingly large consortia and meta-analyses to have reasonable power to detect these small effects; for example, a recent GWAS of body mass index analyzed data from nearly 250,000 individuals (Speliotes et al., 2010)! As studies are combined for these large meta-analyses, the ability to analyze more refined phenotypes is usually compromised, as different assessments often have been used across different studies. One can imagine that the additional layer of trying to find common environmental measures that have been assessed across studies to incorporate gene–environment interaction into these efforts becomes formidable. I often hear social scientists chiding geneticists for “ignoring” the environment; hopefully the reader will now have better appreciation of the daunting challenges that are being faced by geneticists and why incorporating environmental information into gene finding efforts is yet another layer of complexity on an already challenging problem. Of course one could argue that taking into account environmental information might increase the effect size associated with any one locus and reduce the number of participants that are required, but this debate is beyond the scope of this paper. The take home message is that a degree of patience and appreciation for the challenges faced by each respective field is critical, as social scientists begin to enter the world of genetics, and geneticists try to incorporate aspects of social science.
Where Does Epigenetics Fit into this Discussion?
Most of this review has focused on changes to the DNA sequence, such as SNPs and copy number variants. The emerging field of epigenetics assesses the impact of chemical modification of the DNA bases rather than changes to the sequence itself. To better understand this concept, one needs to understand that the expression of a gene is influenced by transcription factors, which bind to specific sequences of DNA. It is through the binding of transcription factors that genes can be turned on or off. Epigenetic mechanisms involve changes to how readily transcription factors can access the DNA. Several different types of epigenetic changes are known to exist that involve different types of chemical changes that can regulate DNA transcription. One epigenetic process that affects transcription binding is DNA methylation. DNA methylation involves the addition of a methyl group (CH3) onto a cytosine (one of the four base pairs that make up DNA). This leads to gene silencing because methylated DNA hinders the binding of transcription factors. A second major regulatory mechanism is related to the configuration of DNA. DNA is wrapped around clusters of histone proteins to form nucleosomes. Together the nucleosomes of DNA and histone are organized into chromatin. When the chromatin is tightly condensed, it is difficult for transcription factors to reach the DNA, and the gene is silenced. In contrast, when the chromatin is opened, the gene can be activated and expressed. Accordingly, modifications to the histone proteins that form the core of the nucleosome can affect the initiation of transcription by affecting how readily transcription factors can access the DNA and bind to their appropriate sequence.
Epigenetics has caused a great deal of excitement in social science, as it provides a potential mechanism for how the environment can “get under the skin.” It provides a biological process by which exposure to environmental events could affect outcome and could lead to long-term enduring changes. Elegant work in animal models suggests that epigenetic changes may be involved in the associations between early environmental manipulations and long-term effects that persist into adulthood, and we refer the interested reader to reviews by Meaney and colleagues on this topic (Meaney, 2010; Zhang and Meaney, 2010). At this time, epigenetic processes are not well-understood. As mentioned previously, the mechanisms by which identified sequence variants in the genome affect outcome are also poorly understood at this time. It is possible that some of these variants may act by altering the likelihood of epigenetic modification, providing an explanation for why some individuals are more influenced by environmental events. Future research is necessary to better understand how these genetic phenomena interrelate.
An Example of Research at the Intersection of Genetics and Social Science
The challenges inherent in studying genetics should not discourage the social scientist from embarking on this area of research, as the potential for moving both fields forward by taking advantage of the knowledge base of each is too great. Our experience with these interdisciplinary collaborations is that they end up being rewarding, educational, and beneficial to all involved – and that they result in exciting research advances! As one example, some years back, the Principle Investigators on the Child Development project (Drs. Jack Bates, Ken Dodge, and Greg Pettit) approached Danielle M. Dick about adding a genetic component to their on-going longitudinal study of >500 children, first assessed as they entered kindergarten, with >20 years of developmental data. The project’s guiding model of developmental process was that children’s biological dispositions, cultural contexts, life experiences, and characteristic social cognitions transactionally combine to influence a variety of behavioral outcomes, making it a natural extension of the project to add genotypic data. The rich, longitudinal assessments of the Child Development Project (CDP) offered special advantages for studying the pathways by which genetic factors influence behavioral development. One of the first genes we genotyped was GABRA2, a gene originally identified as associated with alcohol dependence in the collaborative study of the genetics of alcoholism (Edenberg et al., 2004), the largest gene identification project currently in existence for alcohol dependence, on which Danielle M. Dick is a collaborator. The association with adult alcohol dependence was subsequently replicated in many independent samples from around the world (Enoch, 2008). Based on the twin literature indicating that childhood behavior problems and adult alcohol dependence overlap largely due to shared genetic factors (Slutske et al., 1998), we hypothesized that GABRA2 would be associated with behavior problems at earlier stages of development. We also hypothesized that the association between the gene and behavior problems would be moderated by parental monitoring, based on our work in the Finnish twin studies showing that parental monitoring moderates the importance of genetic effects (Dick et al., 2007b). These hypotheses were supported: GABRA2 was associated with trajectories of externalizing behavior across adolescence, with the genotype previously associated with adult alcohol dependence in COGA associated with persistent, elevated levels of behavior problems across adolescence in CDP. Furthermore, this association was moderated by parental monitoring: the association between the genotype and behavior problems was stronger under conditions of lower parental monitoring, attenuated under higher parental monitoring (Dick et al., 2009). A second set of analyses produced similarly exciting results in the sample. CHRM2 is another gene associated with alcohol dependence in the COGA project (Wang et al., 2004) and subsequently replicated in an independent sample (Luo et al., 2005), We genotyped markers across this gene in CDP, and, parallel to GABRA2, found associations with trajectories of externalizing behavior. Further, we tested whether this association was moderated by peer group antisocial behavior based on previous twin studies demonstrating that genetic influences on substance use and problem behavior are enhanced as individuals are exposed to higher levels of peer antisocial behavior and substance use (Button et al., 2007; Dick et al., 2007a; Harden et al., 2008). Our hypothesis was supported: the association between CHRM2 and externalizing behavior was exacerbated among those exposed to higher levels of peer group antisocial behavior (Latendresse et al., 2011). These studies illustrate how genotyping genes coming out of gene identification projects in longitudinal, developmental samples can help us understand the pathways of risk associated with those genes. The DNAs for the child development project are currently in Brien Riley’s laboratory, making it possible to genotype novel genes coming out of the gene identification projects on which Danielle M. Dick and Brien Riley are involved in this developmental sample. Taking genes that are identified in highly selected, affected populations, and characterizing their risk in community-based samples, across development and in conjunction with experiential and other individual risk and protective factors, will be critical to potentially use genetic information in the future to inform prevention and intervention efforts.
Conclusions
The integration of genetics into developmental projects and other studies conducted by social scientists has great potential to enhance our understanding of the mediating pathways and mechanisms by which genetic susceptibility may (or may not) translate into risk behavior. However, in order to realize this potential, genetics research conducted by developmental, social scientists must reflect the current “state-of-the-science” practices from the field of genetics. Close collaborations between social scientists and geneticists are likely to be necessary to achieve this and to be of mutual benefit to both fields.
Conflict of Interest Statement
The authors declare that the research was conducted in the absence of any commercial or financial relationships that could be construed as a potential conflict of interest.
Acknowledgments
Danielle M. Dick is funded by AA018755, AA018333, AA15416, DA025039, AA008401, AA017828, and a NARSAD Young Investigator Award. Shawn J. Latendresse is supported by AA020333. BR is supported by MH083094, AA011408, and AA017828.
Footnotes
References
Barrett, J. C., Fry, B., Maller, J., and Daly, M. J. (2005). Haploview: analysis and visualization of LD and haplotype maps. Bioinformatics 21, 263–265.
Button, T. M., Corley, R. P., Rhee, S. H., Hewitt, J. K., Young, S. E., and Stallings, M. C. (2007). Delinquent peer affiliation and conduct problems: a twin study. J. Abnorm. Psychol. 116, 554–564.
Dick, D. M., and Bierut, L. (2006). The genetics of alcohol dependence. Curr. Psychiatr. Rep. 8, 151–157.
Dick, D. M., Latendresse, S. J., Lansford, J. E., Budde, J., Goate, A., Dodge, K. A., Pettit, G. S., and Bates, J. E. (2009). The role of GABRA2 in trajectories of externalizing behavior across development and evidence of moderation by parental monitoring. Arch. Gen. Psychiatry 66, 649–657.
Dick, D. M., Pagan, J. L., Viken, R., Purcell, S., Kaprio, J., Pulkkinen, L., and Rose, R. J. (2007a). Changing environmental influences on substance use across development. Twin Res. Hum. Genet. 10, 315–326.
Dick, D. M., Viken, R., Purcell, S., Kaprio, J., Pulkkinen, L., and Rose, R. J. (2007b). Parental monitoring moderates the importance of genetic and environmental influences on adolescent smoking. J. Abnorm. Psychol. 116, 213–218.
Dick, D. M., Wang, J. C., Plunkett, J., Aliev, F., Hinrichs, A., Bertelsen, S., Budde, J. P., Goldstein, E. L., Kaplan, D., Edenberg, H. J., Nurnberger, J., Hesselbrock, V., Schuckit, M., Kuperman, S., Tischfield, J., Porjesz, B., Begleiter, H., Bierut, L. J., and Goate, A. (2007c), Family-based association analyses of alcohol dependence phenotypes across DRD2 and neighboring gene ANKK1. Alcohol Clin.Exp. Res. 31, 1645–1653.
Edenberg, H. J., Dick, D. M., Xuei, X., Tian, H., Almasy, L., Bauer, L. O., Crowe, R. R., Goate, A., Hesselbrock, V., Jones, K., Kwon, J., Li, T. K., Nurnberger, J. I. Jr., O’Connor, S. J., Reich, T., Rice, J., Schuckit, M. A., Porjesz, B., Foroud, T., and Begleiter, H. (2004). Variations in GABRA2, encoding the α2 subunit of the GABA-A receptor are associated with alcohol dependence and with brain oscillations. Am. J. Hum. Genet. 74, 705–714.
Enoch, M. A. (2008). The role of GABA(A) receptors in the development of alcoholism. Pharmacol. Biochem. Behav. 90, 95–104.
Gabriel, S. B., Schaffner, S. F., Nguyen, H., Moore, J. M., Roy, J., Blumenstiel, B., Higgins, J., DeFelice, M., Lochner, A., Faggart, M., Liu-Cordero, S. N., Rotimi, C., Adeyemo, A., Cooper, R., Ward, R., Lander, E. S., Daly, M. J., and Altshuler, D. (2002). The structure of haplotype blocks in the human genome. Science 296, 2225–2229.
Harden, K. P., Hill, J. E., Turkheimer, E., and Emery, R. E. (2008). Gene-environment correlation and interaction in peer effects on adolescent alcohol and tobacco use. Behav. Genet. 38, 339–347.
Hedrick, P., and Kumar, S. (2001). Mutation and linkage disequilibrium in human mtDNA. Eur. J. Human Genet. 9, 969–972.
Kessler, R. C., Chui, W. T., Demler, O., and Walters, E. E. (2005). Prevalence, severity and comorbity of 12-month DSMIV disorders in the national comorbidity survey replication. Arch. Gen. Psychiatry 62, 617–627.
Latendresse, S. J., Bates, J. E., Goodnight, J. A., Lansford, J. E., Budde, J. P., Goate, A., Dodge, K. A., Pettit, G. S., and Dick, D. M. (in press). Differential susceptibility to adolescent externalizing trajectories: examining the interplay between CHRM2 and peer group antisocial behavior. Child Dev.
Luo, X., Kranzler, H. R., Zuo, L., Wang, S., Blumberg, H. P., and Gelernter, J. (2005). CHRM2 gene predisposes to alcohol dependence, drug dependence, and affective disorders: results from an extended case-control structured association study. Hum. Mol. Genet. 14, 2421–2432.
Manolio, T. A., Brooks, L. D., and Collins, F. S. (2008). A HapMap harvest of insights into the genetics of common disease. J. Clin. Invest. 118, 1590–1605.
Manolio, T. A., Collins, F. S., Cox, N. J., Goldstein, D. B., Hindorff, L. A., Hunter, D. J., McCarthy, M. I., Ramos, E. M., Cardon, L. R., Chakravarti, A., Cho, J. H., Guttmacher, A. E., Kong, A., Kruglyak, L., Mardis, E., Rotimi, C. N., Slatkin, M., Valle, D., Whittemore, A. S., Boehnke, M., Clark, A. G., Eichler, E. E., Gibson, G., Haines, J. L., Mackay, T. F., McCarroll, S. A., and Visscher, P. M. (2009). Finding the missing heritability of complex diseases. Nature 461, 747–753.
McCarthy, M. I., Abecasis, G. R., Cardon, L. R., Goldstein, D. B., Little, J., Ioannidis, J. P., and Hirschhorn, J. N. (2008). Genome-wide association studies for complex traits: consensus, uncertainty and challenges. Nat. Rev. Genet. 9, 356–369.
Meaney, M. J. (2010). Epigenetics and the biological definition of gene x environment interactions. Child Dev. 81, 41–79.
Neale, B. M., Ferreira, M. A. R., Medland, S. E., and Posthuma, D. (2008). Statistical Genetics: Gene Mapping Through Linkage and Association. New York: Taylor & Francis.
Noble, E. P. (2000). The DRD2 gene in psychiatric and neurological disorders and its phenotypes. Pharmacogenetics 1, 309–333.
Nyholt, D. R. (2004). A simple correction for multiple testing for single-nucleotide polymorphisms in linkage disequilibrium with each other. Am. J. Hum. Genet. 74, 765–769.
Slutske, W. S., Heath, A. C., Dinwiddle, S. H., Madden, P. A. F., Bucholz, K. K., Dunne, M. P., Statham, D. J., and Martin, N. G. (1998). Common genetic risk factors for conduct disorder and alcohol dependence. J. Abnorm. Psychol. 107, 363–374.
Speliotes, E. K., Willer, C. J., Berndt, S. I., Monda, K. L., Thorleifsson, G., Jackson, A. U., Allen, H. L., Lindgren, C. M., Luan, J., Mägi, R., Randall, J. C., Vedantam, S., Winkler, T. W., Qi, L., Workalemahu, T., Heid, I. M., Steinthorsdottir, V., Stringham, H. M., Weedon, M. N., Wheeler, E., Wood, A. R., Ferreira, T., Weyant, R. J., Segrè, A. V., Estrada, K., Liang, L., Nemesh, J., Park, J. H., Gustafsson, S., Kilpeläinen, T. O., Yang, J., Bouatia-Naji, N., Esko, T., Feitosa, M. F., Kutalik, Z., Mangino, M., Raychaudhuri, S., Scherag, A., Smith, A. V., Welch, R., Zhao, J. H., Aben, K. K., Absher, D. M., Amin, N., Dixon, A. L., Fisher, E., Glazer, N. L., Goddard, M. E., Heard-Costa, N. L., Hoesel, V., Hottenga, J. J., Johansson, A., Johnson, T., Ketkar, S., Lamina, C., Li, S., Moffatt, M. F., Myers, R. H., Narisu, N., Perry, J. R., Peters, M. J., Preuss, M., Ripatti, S., Rivadeneira, F., Sandholt, C., Scott, L. J., Timpson, N. J., Tyrer, J. P., van Wingerden, S., Watanabe, R. M., White, C. C., Wiklund, F., Barlassina, C., Chasman, D. I., Cooper, M. N., Jansson, J. O., Lawrence, R. W., Pellikka, N., Prokopenko, I., Shi, J., Thiering, E., Alavere, H., Alibrandi, M. T., Almgren, P., Arnold, A. M., Aspelund, T., Atwood, L. D., Balkau, B., Balmforth, A. J., Bennett, A. J., Ben-Shlomo, Y., Bergman, R. N., Bergmann, S., Biebermann, H., Blakemore, A. I., Boes, T., Bonnycastle, L. L., Bornstein, S. R., Brown, M. J., Buchanan, T. A., Busonero, F., Campbell, H., Cappuccio, F. P., Cavalcanti-Proença, C., Chen, Y. D., Chen, C. M., Chines, P. S., Clarke, R., Coin, L., Connell, J., Day, I. N., Heijer, M., Duan, J., Ebrahim, S., Elliott, P., Elosua, R., Eiriksdottir, G., Erdos, M. R., Eriksson, J. G., Facheris, M. F., Felix, S. B., Fischer-Posovszky, P., Folsom, A. R., Friedrich, N., Freimer, N. B., Fu, M., Gaget, S., Gejman, P. V., Geus, E. J., Gieger, C., Gjesing, A. P., Goel, A., Goyette, P., Grallert, H., Grässler, J., Greenawalt, D. M., Groves, C. J., Gudnason, V., Guiducci, C., Hartikainen, A. L., Hassanali, N., Hall, A. S., Havulinna, A. S., Hayward, C., Heath, A. C., Hengstenberg, C., Hicks, A. A., Hinney, A., Hofman, A., Homuth, G., Hui, J., Igl, W., Iribarren, C., Isomaa, B., Jacobs, K. B., Jarick, I., Jewell, E., John, U., Jørgensen, T., Jousilahti, P., Jula, A., Kaakinen, M., Kajantie, E., Kaplan, L. M., Kathiresan, S., Kettunen, J., Kinnunen, L., Knowles, J. W., Kolcic, I., König, I. R., Koskinen, S., Kovacs, P., Kuusisto, J., Kraft, P., Kvaløy, K., Laitinen, J., Lantieri, O., Lanzani, C., Launer, L. J., Lecoeur, C., Lehtimäki, T., Lettre, G., Liu, J., Lokki, M. L., Lorentzon, M., Luben, R. N., Ludwig, B. M. A. G. I. C., Manunta, P., Marek, D., Marre, M., Martin, N. G., McArdle, W. L., McCarthy, A., McKnight, B., Meitinger, T., Melander, O., Meyre, D., Midthjell, K., Montgomery, G. W., Morken, M. A., Morris, A. P., Mulic, R., Ngwa, J. S., Nelis, M., Neville, M. J., Nyholt, D. R., O’Donnell, C. J., O’Rahilly, S., Ong, K. K., Oostra, B., Paré, G., Parker, A. N., Perola, M., Pichler, I., Pietiläinen, K. H., Platou, C. G., Polasek, O., Pouta, A., Rafelt, S., Raitakari, O., Rayner, N. W., Ridderstråle, M., Rief, W., Ruokonen, A., Robertson, N. R., Rzehak, P., Salomaa, V., Sanders, A. R., Sandhu, M. S., Sanna, S., Saramies, J., Savolainen, M. J., Scherag, S., Schipf, S., Schreiber, S., Schunkert, H., Silander, K., Sinisalo, J., Siscovick, D. S., Smit, J. H., Soranzo, N., Sovio, U., Stephens, J., Surakka, I., Swift, A. J., Tammesoo, M. L., Tardif, J. C., Teder-Laving, M., Teslovich, T. M., Thompson, J. R., Thomson, B., Tönjes, A., Tuomi, T., van Meurs, J. B., van Ommen, G. J., Vatin, V., Viikari, J., Visvikis-Siest, S., Vitart, V., Vogel, C. I., Voight, B. F., Waite, L. L., Wallaschofski, H., Walters, G. B., Widen, E., Wiegand, S., Wild, S. H., Willemsen, G., Witte, D. R., Witteman, J. C., Xu, J., Zhang, Q., Zgaga, L., Ziegler, A., Zitting, P., Beilby, J. P., Farooqi, I. S., Hebebrand, J., Huikuri, H. V., James, A. L., Kähönen, M., Levinson, D. F., Macciardi, F., Nieminen, M. S., Ohlsson, C., Palmer, L. J., Ridker, P. M., Stumvoll, M., Beckmann, J. S., Boeing, H., Boerwinkle, E., Boomsma, D. I., Caulfield, M. J., Chanock, S. J., Collins, F. S., Cupples, L. A., Smith, G. D., Erdmann, J., Froguel, P., Grönberg, H., Gyllensten, U., Hall, P., Hansen, T., Harris, T. B., Hattersley, A. T., Hayes, R. B., Heinrich, J., Hu, F. B., Hveem, K., Illig, T., Jarvelin, M. R., Kaprio, J., Karpe, F., Khaw, K. T., Kiemeney, L. A., Krude, H., Laakso, M., Lawlor, D. A., Metspalu, A., Munroe, P. B., Ouwehand, W. H., Pedersen, O., Penninx, B. W., Peters, A., Pramstaller, P. P., Quertermous, T., Reinehr, T., Rissanen, A., Rudan, I., Samani, N. J., Schwarz, P. E., Shuldiner, A. R., Spector, T. D., Tuomilehto, J., Uda, M., Uitterlinden, A., Valle, T. T., Wabitsch, M., Waeber, G., Wareham, N. J., Watkins, H., Procardis, Consortium, Wilson, J. F., Wright, A. F., Zillikens, M. C., Chatterjee, N., McCarroll, S. A., Purcell, S., Schadt, E. E., Visscher, P. M., Assimes, T. L., Borecki, I. B., Deloukas, P., Fox, C. S., Groop, L. C., Haritunians, T., Hunter, D. J., Kaplan, R. C., Mohlke, K. L., O’Connell, J. R., Peltonen, L., Schlessinger, D., Strachan, D. P., van Duijn, C. M., Wichmann, H. E., Frayling, T. M., Thorsteinsdottir, U., Abecasis, G. R., Barroso, I., Boehnke, M., Stefansson, K., North, K. E., McCarthy, M. I., Hirschhorn, J. N., Ingelsson, E., and Loos, R. J. (2010). Association analyses of 249,796 individuals reveal 18 new loci associated with body mass index. Nat. Genet. 42, 937–948.
van den Oord, E. J. (2007). Controlling false discoveries in genetic studies. Am. J. Med. Genet. B Neuropsychiatr. Genet. 147B, 637–644.
Wang, J. C., Hinrichs, A. L., Stock, H., Budde, J., Allen, R., Bertelsen, S., Kwon, J. M., Wu, W., Dick, D. M., Rice, J., Jones, K., Nurnberger, J. I. Jr., Tischfield, J., Porjesz, B., Edenberg, H. J., Hesselbrock, V., Crowe, R., Schuckit, M., Begleiter, H., Reich, T., Goate, A. M., and Bierut, L. J. (2004). Evidence of common and specific genetic effects: association of the muscarinic acetylcholine receptor M2 (CHRM2) gene with alcohol dependence and major depressive syndrome. Human Mol. Genet. 13, 1903–1911.
Zhang, T. Y., and Meaney, M. J. (2010). Epigenetics and the environmental regulation of the genome and its function. Annu. Rev. Psychol. 61, 433–439.
Keywords: genetics, association, linkage, advantages and disadvantages, gene–environment interaction, learning genetics
Citation: Dick DM, Latendresse SJ and Riley B (2011) Incorporating genetics into your studies: a guide for social scientists. Front. Psychiatry 2:17. doi: 10.3389/fpsyt.2011.00017
Received: 07 February 2011; Accepted: 09 April 2011;
Published online: 09 May 2011.
Edited by:
Benjamin Hankin, University of Denver, USAReviewed by:
Lisa Badanes, University of Colorado Health Science Center, USAJennifer Lau, Oxford University, UK
Copyright: © 2011 Dick, Latendresse and Riley. This is an open-access article subject to a non-exclusive license between the authors and Frontiers Media SA, which permits use, distribution and reproduction in other forums, provided the original authors and source are credited and other Frontiers conditions are complied with.
*Correspondence: Danielle M. Dick, Department of Psychiatry, Virginia Institute for Psychiatric and Behavioral Genetics, Virginia Commonwealth University, PO Box 980126, Richmond, VA 23298-0126, USA. e-mail:ZGRpY2tAdmN1LmVkdQ==