- 1 Renaissance Computing Institute, University of North Carolina at Chapel Hill, Chapel Hill, NC, USA
- 2 Frank Porter Graham Institute, University of North Carolina at Chapel Hill, Chapel Hill, NC, USA
The success of research in the field of maternal–infant health, or in any scientific field, relies on the adoption of best practices for data and knowledge management. Prior work by our group and others has identified evidence-based solutions to many of the data management challenges that exist, including cost–effective practices for ensuring high-quality data entry and proper construction and maintenance of data standards and ontologies. Quality assurance practices for data entry and processing are necessary to ensure that data are not denigrated during processing, but the use of these practices has not been widely adopted in the fields of psychology and biology. Furthermore, collaborative research is becoming more common. Collaborative research often involves multiple laboratories, different scientific disciplines, numerous data sources, large data sets, and data sets from public and commercial sources. These factors present new challenges for data and knowledge management. Data security and privacy concerns are increased as data may be accessed by investigators affiliated with different institutions. Collaborative groups must address the challenges associated with federating data access between the data-collecting sites and a centralized data management site. The merging of ontologies between different data sets can become formidable, especially in fields with evolving ontologies. The increased use of automated data acquisition can yield more data, but it can also increase the risk of introducing error or systematic biases into data. In addition, the integration of data collected from different assay types often requires the development of new tools to analyze the data. All of these challenges act to increase the costs and time spent on data management for a given project, and they increase the likelihood of decreasing the quality of the data. In this paper, we review these issues and discuss theoretical and practical approaches for addressing these issues.
The Data Management Challenge for Collaborative Research
As highlighted in a recent field guide by the National Institutes of Health (NIH), entitled “Collaboration and Team Science: A Field Guide” (Bennett et al., 2010), and as noted in recent publications (Wuchty et al., 2007; Stokols et al., 2008), the NIH and the scientific community have shifted their focus over the past 10 years from research projects conducted by individual investigators or laboratories to research collaborations among teams of investigators and laboratories. This shift in focus is evident in NIH actions such as the 2006 formation of the Clinical and Translational Science Awards Consortium1, which is designed to promote translational research among investigative teams, the 2006 revision of the NIH Tenure Review Committee, which added “team science” to review criteria, and the 2007 creation of grants involving multiple Principal Investigators. While collaborative research is not new, the NIH focus on translational research has promoted “consortium-oriented” collaborative research in which multiple, independent research laboratories share funding to support research on a broad scientific question of relevance to, and requiring the expertise of, each laboratory. We are involved in two such research collaborations designed to delineate the impact of drug use on health behaviors and to define the mechanisms responsible for these effects. The data management practices for the first collaboration involving the Frank Porter Graham Institute at UNC has previously been presented as a case study (Burchinal and Neebe, 2006). Our collaborative research projects rely on the synthesis of data generated from multiple sources, such as functional and structural neurobiological assays, behavioral tests, genetic analyses, infant vocalizations, and immunological assays. While consortiums like ours have the potential to yield insight into significant scientific problems, they also present significant challenges in the synthesis of different research methodologies and data types. In this paper, we look specifically at the data management challenges faced by research collaborations, we examine the complexities involved in the integration of data across research sites, and we review practices and technologies that we have found to be effective for data management and integration in collaborative research.
Figure 1 provides a high-level generalization of the data management challenges faced by multi-site research collaborations. Importantly, multi-site collaborations include a data coordinating site that manages all project data and serves as a focal point for the integration of data for data exploration and analysis. The data coordinating site is often an administrative core in large consortiums or an individual laboratory in small collaborations. In multi-site research collaborations, different laboratories generate data through their own specialized research activities, and these laboratories are often involved in more than one research collaboration. Laboratories generally develop, over many years, individualized standard operating procedures for the production, description, and analysis of data generated from that laboratory. The standard operating procedures are typically tailored to each laboratory’s research expertise and include methodological approaches for data production and dissemination, annotation capture, and quality assurance procedures. The ability of laboratories to alter their standard operating procedures for different research collaborations is limited because of the resultant disruption in laboratory activities and loss of time (and hence, money). In addition, different laboratories often adopt data-usage policies that may be institution-specific and that may vary from the policies established for the collaboration.
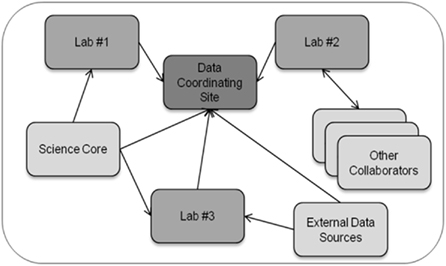
Figure 1. Schematic of data exchange in a multi-site research collaboration. In a multi-site research collaboration, data coining from and to individual laboratories and scientific cores (e.g., metabolomic, proteomics, imaging cores) must be managed and integrated along with the annotation describing the data.
Thus, a key challenge for the collaboration in general and for the data coordinating site more specifically is to ensure that data management practices throughout the collaboration are adequate for data integration and analysis despite the inability of the data coordinating site to change individual laboratory practices. Data management practices also must remain adequate throughout the natural evolution of the research collaboration as new findings lead to adjustments in the research process. The increased size of data set due to new technologies, such as next-generation genetic sequencers, present both logistical and security issues due to the large size of individual data files and the need to co-locate data files with adequate computational capabilities and data storage facilities to allow for processing and analysis of the data. An additional key challenge is that the synthesis of data entails the integration of numerous data types, and a single laboratory typically does not have direct experience with the many data types that arise in multi-site research collaborations. For instance, in our collaboration on the effect of disruptions in the mother–infant bond as a result of maternal drug use, data types include fluorescence measurements in specific brain regions derived from immunohistochemistry, measurements derived from functional magnetic resonance imaging (fMRI) in specific brain regions, sound vocalizations from infants, and behavioral responses of mothers to the infant vocalizations. By developing the linkages between such diverse data sets, the data coordinating site can enable investigators to more readily retrieve, visualize, and compare results for selected experimental conditions across all measurement types.
Identifying Best Practices for Data Management
High-quality data management practices focus on reducing the amount of error introduced during the multiple stages of the data lifecycle, including data collection, cleaning, scoring, processing, storage, archiving, and analysis, re-analysis, or secondary analysis. The need for quality practices is paramount to good research. For example, we have detected data management-related error rates of 5–10% when data are entered only once and error rates of over 10% when research assistants score and enter developmental test data in projects that depended on their laboratory for data collection and scoring before turning to our data center for data entry and processing. In our study, the implementation of high-quality practices within the data coordinating site dramatically reduced error rates from all sources to less than 1% (Burchinal and Neebe, 2006). The NIH now acknowledges the need for high standards for data management and requires data-sharing plans for all projects and professional data management for large projects (Coulehan and Wells, 2005). We review key points regarding evidence-based practices that we have found to be cost-efficient and associated with a reduction in errors in multi-site research collaborations.
Identification (ID) System
A consistent and comprehensive ID system must be formulated that uniquely identifies each study subject (e.g., human subject, animal subject, or biospecimen). This entails the creation of a unique ID for each subject in each study site at multiple time points for longitudinal studies and for each treatment group for clinical trials or other studies involving treatment or intervention groups. The ID numbers should provide unique identification across nested factors such as time, family members, clinics, or treatment groups. Along with the ID number, a list of important information on the study subjects such as gender or birth date should be established – these data are often stored as the master file. The master file provides annotation for the study subjects, allowing the data coordinating site to validate data entry by different data collectors and for data collected over time.
Variable System
A well-described system for naming and annotating variables that are used across experiments is necessary to establish; this includes the creation of conventions for naming variables and the establishment of checks for inconsistencies and errors related to variable values. Variable names should be unique across all datasets. When practical, systematic variables names can include information about the variables themselves such as the protocols that were used to capture the variable. Annotation should be associated with each variable and should provide details about the measurement captured by the variable, the valid values for the variable, the type of variable (e.g., binary, ordinal), and the methodology used to capture the measurement. The systematic nomenclature and annotation of variables reduce errors by clearly documenting each variable and facilitating the transfer of best data management practices to new members of the research team.
Several challenges exist in the development of a variable system for use in a multi-site collaboration. In a previous collaboration focused on tissue and cell engineering, we found that the inclusion of a staff member with training in both biology and ontologies was invaluable in reducing errors. During the course of that study, we also were able to categorize the issues that arose over a 5-year span, which we present below. (Note that the word “term” is used interchangeably to mean either “variable name” or “variable value.”)
1. Use of vague terms: terms such as “Dex” or “PepMix10” are inexact, are difficult to map between labs, and lose meaning over time.
2. Use of synonyms: the use of synonyms such as “niacinamide” and “vitamin B” leads to failures in the integration of data.
3. Use of similar terms: terms such as “VEGF” and “VEGF-D” refer to different entities, but are similar enough that researchers often mistakenly use one term instead of the other. This problem, as well as the following one, is one that is readily handled by a staff member with expertise in both biology and ontologies.
4. Use of homonyms: oftentimes, different scientific subfields use the same term but with different meanings. For example, the term “CD34” could mean a gene, a cell surface protein, an antibody, or a type of immune cell, depending on the laboratory’s scientific focus.
5. Complex constraints on variable values: valid values for variables are often based on evolving standards. In this case, the implementation of quality assurance checks to ensure that the values are consistent with standards becomes difficult and often requires the removal of the quality assurance checks, which could introduce error. An example is the use of list boxes on a graphical user interface that holds valid values for a variable.
6. Failure to use standard keywords: the use of non-standard terms (when standard terms exists) leads to problems with data integration when merging data sets.
7. Incorrect use of variables: we identified in several cases in which researchers would use a variable to record information if tracking of the information was important to the researcher, but the desired variable was not part of the overall study or the variable system.
8. Failure to provide variable values: researchers who aren’t trained in the need for variable values typically do not provide such values.
Data Provenance and Management through Structured Data Storage
The data system must enable the reproducibility of the results of all analyses of the data, i.e., the data system must provide for the provenance of the results. In practice, provenance is hard to achieve and is costly (Rajendra and Frew, 2005; Yogesh et al., 2006). To address this issue, we suggest the use of a file-based directory structure as this facilitates provenance, is easy to establish, and is cost-efficient to maintain. We suggest separate subdirectories for projects, programs, datasets, and documentation. For our study on the development of language, for example, we had a directory labeled “Langstudy” with subdirectories for analysis and data management. Within the analysis subdirectory, we included separate sub-subdirectories for analyses specific to a given presentation or manuscript. The analysis sub-subdirectories contained all survey programs, memorandums, and other forms of documentation related to analysis. Within the data management subdirectory, we included sub-subdirectories for each data collection effort. Within both the analysis and the data management subdirectories, we included sub-subdirectories for survey programs, data, documentation, and print. The program sub-subdirectory contained all computer programs used to enter, score, and update the data sets. The data sub-subdirectory contained all data files. The documentation sub-subdirectory contained all communication with the project staff regarding data collected for each study instrument, lists of errors in the data, and instructions on how to correct those errors. The print sub-subdirectory contains copies of the output from all software programs used to process the data. The use of file-based directories ensures that all data files can be traced accurately from data collection through data analysis to published manuscript or presentation. Requirements such as data backup and security can be addressed with existing file-based tools. For instance, access to data can be controlled with Unix-based access control lists or Windows Group Policies.
Quality Assurance
While specific quality assurance practices will vary depending on the details of how the data are captured and processed, quality assurance practices should be put in place to validate data correctness, i.e., to ensure that all data values are within the appropriate ranges, that IDs are present, and that duplicate IDs do not exist. Quality assurance practices also should be in place to ensure that the transfer and integration of data within the data management system are reliable, correct, and efficient. It is important to document all quality assurance practices. The implementation of sound quality assurance practices can be quite complex, and fully realized quality assurance approaches such as those practiced using the approaches set forth by six sigma (Stamatis, 2004) or Good Manufacturing Practices/Good Laboratory Practices (Carson and Dent, 2007) are typically beyond the resources of NIH-funded collaborative research. However, several simple, inexpensive quality assurance practices can be effective. For example, the use of a second person to double-check all scoring of assessment tools and all data entry greatly improves data quality. Similarly, when new computer programs are created to automate data processing, a software code review by a second person (or the development team) can aid in identifying quality concerns with the software. All developed software should include software unit tests that demonstrate that the software performs correctly across expected use cases. In addition, quality risk reviews with team members can ensure that problems with data collection and processing are identified early on. These reviews can be structured as brain-storming exercises using a “Cause-and-Effect” diagram (Ishikawa and Loftus, 1990) to capture first the effects of any concerns (e.g., incorrect values in a survey item), to identify the possible causes of any concerns (e.g., errors in data capture software), and to assess the likely risk that each cause is present (e.g., low if software has been validated in other studies). The advantages of this approach are that it is easy to perform and the documentation of risk allows for the prioritization of concerns.
Tracking
A tracking system should be established that allows the project team to follow the progress (or lack thereof) of data collection across project activities. The typical tracking system involves a computerized “to do” list of data processing tasks that are checked-off as they are completed. The tracking system should also record the presence of data quality issues and the actions that were taken to address each issue. Open source and commercial project and ticket tracking systems can be used for tracking if the development of a customized solution is not feasible. An example is the Confluence/Jira tools that are often used for tracking software projects and can be customized for quality tracking.
Revision Control of Data
During data collection, we recommend the creation of a series of permanent data sets and the use of version numbers to keep track of revisions. The first permanent data set is created when the data are generated. Subsequent permanent data sets are created when new data are added or changes are made to the data in the original data set, and the new data sets are assigned names that indicate that they are revisions of the previous data set. This stage involves the processing of data for correctness, and the master file and variable naming system can aid in this task. For longitudinal studies, for instance, the master file may contain detailed demographic data on subjects, and those data should match the demographic data captured in follow-up studies. All failures and warnings indicative of a mismatch of the data should be tracked, and remediation should be taken to address the issue. The project’s tracking system should capture what changes were made as part of the remediation effort, the team member who made the changes, the date when the changes were made, and the reason why the changes were necessary. Proper tracking of the details related to any changes in the data set provides an explanation for why the data in a revised data set differ from those the original data set. With each revision of the data set, a new version is created and named, and older versions are maintained for reference. Finally, a log can be maintained by the project team that documents all changes and decisions regarding the data.
Analysis Concerns
Permanent data sets for specific analyses should be created only when data are completely entered, cleaned, and frozen. It is often tempting to create an “analysis” data set to begin analyzing the results and to include all of the data – typically from multiple data sets – in one analysis data set. While an analysis data set may make it easier to run an analysis program, a concern is that the project team might make corrections to the data or add new data to the data sets without updating the analysis data set. The creation of analysis data sets can therefore result in the analysis of data that do not include all possible subjects or do not reflect corrections. We recommend an alternative approach in which a single program is used to represent all manipulations needed to create the analyzed data; this program is then run each time an analysis is conducted. The use of a single program to extract data, recode data, and delete ineligible cases has several advantages over the use of an analysis data set. First, any updates to the data sets will be maintained in all analyses because the program is run using the most recent version of the data set. Second, this approach will provide complete documentation about all of the decisions made regarding which subjects were included in the analyses, how the variables were re-coded, and which summary variables were created.
Documentation
The creation of comprehensive documentation for a project is one of the most valuable roles that professional data management provides for a research team. As noted in NIH and FDA guidelines (U. S. Food and Drug Administration, 2003; Coulehan and Wells, 2005), professional data management should result in data that can be traced from collection through analysis in a manner in which all changes to the data and all decisions regarding the data are apparent. We have been able to achieve data provenance through rigorous documentation and the structured storage approach discussed above. Documentation should be created to describe each step of the research process, and the documentation should be available in both electronic and paper forms. Decisions regarding the management of data sets should be documented electronically, both within the data sets and within separate files maintained within the database. All variables should be labeled in each data set in a systematic manner that conveys information about each variable, even after data sets are merged. Codebooks or annotation forms should be created to describe each study, to map variable names onto the data that were collected, and to document decisions made during data-keying and processing. We have found that these codebooks are invaluable for providing quick access to data collection forms and information about the instrument, and they also facilitate the publication process. In addition to our electronic documentation and codebooks, we include a notebook or set of notebooks for each project, which includes the research proposal, all versions of the data collection instruments, scoring instructions, a codebook for each instrument or data set, and paper copies of all communications, including error reports and remediation efforts.
Identifying Approaches for Cross-Collaboration Data Integration and Sharing
The practical matter of integrating data from multiple laboratories may seem trivial at first consideration, but in practice, integration presents many challenges. The research practices adopted by a collaborative team can affect the quality of the data, the efficiency at which the collaboration operates, and the ability to enforce policies. For example, content management systems (CMS) are often used to facilitate the uploading of data from laboratories, but CMS typically do not have good capabilities for handling data provenance in instances, for example, when a laboratory uploads a new version of a data set. On a practical level, when the logistics of a research project are poorly coordinated, the likelihood that a laboratory continues to actively participate in a project declines as investigators become frustrated and focus their time on other projects.
The approach often taken for the coordination and integration of data is to pick a familiar, but not necessarily an ideal, technology for data management and to refine it as needed. For instance, many collaborations use an existing, web-based CMS such as MS SharePoint or Joomla! because information technology (IT) specialists are often familiar with such tools. We advocate for an engineered approach in which each laboratory’s needs for data sharing and integration are ascertained and used to determine the technical approaches. Table 1 lists the various factors that should be considered in gathering technological requirements.
After the project team has carefully reviewed the factors listed above, the team will be in a position to identify the best technical approaches to take to share and integrate data across the collaborative team. We broadly classify the technical approaches below.
Shared Space
Perhaps the simplest approach is the use of a shared storage area that is accessible by all members of the collaborative team. This space can be a shared network folder on a file system, an ftp site, a DropBox folder2, or even documents stored in Google Docs3. This approach has the benefit of convenience for collaborators and low maintenance costs. This approach has disadvantages, however, in that it lacks good mechanisms for enforcing policy and security concerns. This approach also provides limited support for the actual integration of data sets or the automation of processes such as quality assurance checks; often, this type of support is provided through custom software or scripts.
Content Management Systems
A CMS such as Microsoft SharePoint4, Joomla!5, or Drupal6 can be configured easily by IT staff with minimal IT experience, especially if one uses virtual appliances with the system pre-installed. The CMS typically offer convenient and familiar interfaces for laboratories, particularly those with limited experience in collaborative research. In general, the CMS are easy to customize, and junior IT staff can usually customize a CMS; however, the customizations can be unwieldy to maintain over time.
Digital Archive
Digital archive systems such as the open source DSpace7 from MIT are aimed at building collections of digital media. As such, these systems often provide for many collaborative needs, including data organization, data federation, metadata support, data provenance, and data security. While digital archive software can be used for research collaborations, support for the detection and tracking of quality assurance issues and for the automated processing of scientific data must be accomplished by an IT specialist with strong programming skills. While configuring and maintaining the system are not difficult, they require more time with a digital archive system than with a shared space or a CMS.
Vertical Data Management Systems
A number of vertical data management systems, including open source versions, have been developed, and these are aimed at specific types of scientific data. For instance, the MIDAS (Kitware8) and Xnat9 systems were developed for the management of neural imaging data, whereas the MADAM system (TM410) was developed for the management of microarray data. The advantages of these systems are that they are optimized for dealing with specific types of data, they can provide data visualization and analysis capabilities, they use structured storage of the data (which facilitates queries), and they include quality checks on the data. The big disadvantage of these systems in collaborative research is that the data management core must set-up and run multiple software systems, each with different approaches for handling issues related to security, provenance, and metadata. Also, these systems rarely facilitate the federation of data.
Library Information Management Systems
Library information management systems (LIMS) provide both centralized and federated approaches to manage a broad range of laboratory data such as biospecimen tracking and reagent training within a single system. Commercially available LIMS include very powerful capabilities for a range of applications, including data integration, quality assurance tracking, data provenance, automation of workflows, and electronic notebooks. These systems are very expensive, however, and they take time to customize, often requiring consultations or contractual agreements with the vendor. Unfortunately, there are very few open source LIMS, and the ones that exist provide very few of the benefits that the commercial versions do and are difficult to customize.
Federated Systems
Federated data systems allow for the integration of data that are located on different computer resources that are geographically separated, without moving the data to a centralized location. The open source Teiid system11 from the JBoss Community is an exemplar of this type of technology. The Teiid system provides feature-rich, cross-site, query, and security mechanisms with a rich graphical user interface for designing virtual databases that pull data from remote sites on-demand and for designing administrative consoles for the management of the system. The system can be extended by software developers to automate processes and to provide useful add-ons such as integration into a CMS. The Teiid system comes with multiple adaptors to read from databases, flat files, MS Excel spreadsheets, and others. Effective use of a federated system requires an IT specialist with programming experience. A disadvantage of federated systems is that federation requires that laboratories provide a mechanism to access the data on their systems, or they need to submit their data to an accessible location, and some laboratories are hesitant to provide this or otherwise incapable.
Distributed Data Systems
Distributed data systems share some capabilities with federated systems; however, we distinguish them here by goal (and this is an arguable distinction), in that federated systems are geared toward a single, integrated view of distributed data (e.g., a virtual database), whereas distributed systems are aimed at providing common access to distributed data (e.g., a distributed file system with data management capabilities built-in). A distributed system, like the iRODS data grid12, provides a unified approach to access data at different locations and in different storage formats, including flat files or relational databases, with a distributed rule-engine that allows the administrator to enforce data management policies, including security, automation, and replication, across the collaborative team. Distributed systems have the advantage of providing centralized control while allowing data to remain distributed. These approaches, however, typically require an IT specialist with strong programming skills.
Hybrid Systems
Hybrid combinations of the approaches mentioned above are worth consideration. For example, a federated system such as Teiid that integrates data from vertical data management systems such as MIDAS or MADAM can provide both vertical-oriented capabilities with federation across data types and laboratories. Likewise, a CMS on top of a datagrid such as iRODS (see text footnote 12) provides both familiar web-based tools with a robust system for policy management. A disadvantage of hybrid systems is that there is a myriad of possibilities that can be confusing to sort out; however, the choice of technology can be facilitated by determining which of the above factors is important and how each factor can be addressed.
Addressing Usage Policies, Privacy, and Security
In general, the management of data security and confidentiality issues are well known in the research community and are not addressed in detail here. In a collaborative research environment, however, one has to deal with the added complexity that the data coordinating center is responsible for enforcing usage policies and security and privacy concerns related to data originating from multiple laboratories. Depending on the collaboration, this responsibility may become quite complex. For instance, we have been involved in collaborations in which data received from one laboratory required deletion of the data by the data coordinating center after 7 days and data received from another laboratory could only be handled by IT staff that met certain background checks. In isolation, such policies are not hard to deal with; with multiple laboratories with changing and conflicting policies, a well-managed process must be in place to ensure that policies are followed. On the basis of our experience, we believe that this is best achieved when the ability to enforce policies is embedded within the data management technology.
iRODS (see text footnote 12)is an example of a best-of-breed technology in this regard. iRODS allows for separate policies to be implemented as rules and for rules to be applied separately to any data resource within the data system. iRODS also includes a rules engine that automates the execution of policy-governing rules that may have been generated from different groups, thereby allowing laboratories and coordinating sites to generate rules independently. A key point is that iRODS has the ability to execute multiple applied rules, even when those rules have conflicting impacts.
A second issue that we address has only recently received attention within the IT community; this is the concept of “data leakage.” Data-leakage refers to the inappropriate transfer of sensitive data out of a managed-data system. Commercial security vendors such as Symantec, McAfee, and Trend Micro have been developing suites of data-leakage protection technologies that audit and trap data that are moved inappropriately from one computer to another, whether this is done by file copy, email, IM chats, or other means. These technologies are still maturing and are often costly; however, a data coordinating center should consider this technology as part of its overall assessment of risk versus resource allocation. The Renaissance Computing Institute, in collaboration with the North Carolina Translational and Clinical Sciences Institute, has developed the concept of a “Secure Research Workspace” (Owens et al., 2011) as a solution to the data-leakage problem. In the Secure Research Workspace, a combination of computer virtualization and data-leakage technologies are used to provide researchers with an on-demand work environment with provisioned data that cannot be transferred outside of the managed environment, but that allows the researcher to import needed tools and export analysis results as needed.
Application of Integrated Data
The integration of different types of data such as fMRI, sound recordings, and genomic data offers the potential for scientific discovery; however, as noted in Searls (2005), the challenges involved in the integration of different data sources go beyond the challenges involved in bringing the data together, but rather they may involve the development of new methodologies. Data management practices can and should enable such discovery, but the practices depend greatly on the approaches taken by the collaborative research team. Meta-analysis, a statistical method used to combine existing evidence (Hedges and Olkin, 1985), requires the integration of results from data sets that measure the same outcome variables. For instance, the meta-analysis of fMRI data across research studies and laboratories can be performed with voxel-based measurements, anatomical labels, or a combination of laboratory results and coordinates with varying trade-offs (Costafreda, 2009). Recently, these approaches have been applied to the combination of neural imaging and genetics (Mier et al., 2010; Thompson et al., 2010). From the perspective of best data management practices, meta-analysis is similar to other types of analyses; raw data (in this case drawn from published articles) are processed to produce new data sets that are then analyzed using standard programs. As such, the existing best practices – reviewing risk, versioning data sets, and implementing tracking processes – apply and should be used, particularly if the results are likely to be published. For instance, in a meta-analysis of labeled neuroanatomical regions from published fMRI studies, consistent use of variable labels and terminology should be applied across data sets and become part of the data provenance process to ensure that the results are reproducible (Laird et al., 2005).
In contrast to meta-analysis, data exploration is geared at generating new hypotheses or insights that are often not published, but rather lead to the generation of new studies. Key to exploration of different types of data is the generation of a common reference against which the data can be understood. For instance, the PubAnatomy system from Xuan et al. (2010) provides an electronic brain atlas upon which other data such as gene expression can be superimposed onto the anatomical information. This type of system provides great flexibility. For instance, a collaborative project on stress sensitivity might use multiple paradigms to measure the anatomical correlates of stress (e.g., genetic or immunohistological data derived from specific brain regions) and behavioral measures of stress sensitivity (e.g., socialization behavior, physical challenges), and then the collaborative team might use a data exploration system like PubAnatomy to explore the union of the results. This approach, of course, requires making decisions as to how to relate measurements made using different paradigms, and these decisions should be tracked to ensure the reproducibility of the results. Data exploration is much simpler when best data management practices have ensured that the annotation among data sets is consistent, that the data are of high quality, and that the data can be located and retrieved easily.
Data Integration and Very Large Data Sets
In recent years, we have seen an explosion in the amount of scientific data that is being generated, and more specifically, there has been a dramatic increase in the size of data sets that researchers and data coordinating sites have to work with. For example, for one of our NIH-funded projects, we are sequencing whole human genomes to identify linkages between genomic variants and cancer. Our team receives data sets from sequencing facilities that contain approximately 100–400 GB of data per sequenced subject; thus, we require 10–40 TB of disk space for every 100 subjects just to store the data. Another 10 TB of disk space is required to process the data to determine variants in the DNAs and several Terabytes of disk space are required to construct a database for analysis. Very large data sets often require the development of new approaches for the storage, processing, and querying of data. Presently, a Terabye of high-quality data storage costs $1,000 per Terabyte for 3–4 years of support. Therefore, collaborative teams must plan for what data is going to be stored, the storage technologies that will be used to store the data (e.g., tape, slow disk drives, fast disk drives, a combination), and the software approaches that will be used to organize the data. Planning for data store is imperative to efficiently allocate the resources that are available to the research collaboration and the ability of the collaboration to effectively use the data.
An in-depth review of data storage approaches is beyond the scope of this paper; however, the impact of very large data sets on data integration merits attention. Typically, data are integrated either with a relational database management system (RDBMS) or a computer program that integrates individual data sets in the process of analysis. Both of these approaches can scale poorly with a large number of large data sets due to the number of read and write computer operations that are required to process the data. The scaling problem has led to the development of No-SQL database technologies, initially formulated in Google Inc.’s BigTable technology (Chang et al., 2006), that are designed to provide high scalability for processing data sets within the Terabyte to Petabyte scale. While commercial parallel RDBMS systems can arguably deal with data of this size, the commercial systems are often too costly for use in academic projects (see Stonebraker, 2010 for a discussion of the pros and cons of No-SQL technologies). Several open source No-SQL technologies, such as the Apache Hadoop/HBase system13, can address the scaling problem and provide for data integration with very large data sets. The SeqWare system (O’Connor et al., 2011) uses No-SQL technology and can be used effectively to manage the large data sets associated with next-generation genomic sequencing technologies and other technology that generate very large data sets. The authors are currently investigating the integration of SeqWare with a traditional RDBMS system to determine whether this approach provide the flexibility and security offered by RDBMS with the scaling offered by No-SQL technology. A primary disadvantage of using No-SQL approaches, despite its growing adoption by many businesses, is that there is a lack of IT professionals who are adequately trained to use these systems.
Conclusion
Collaborative research projects face the double challenge of ensuring the integrity of research data and the orchestration of data management across multiple laboratories. Sound data management practices are needed to ensure success in addressing these challenges. While high-quality practices require that research staff receive specific training in best practices and sufficient time to implement those practices, the benefits are broad. The practices and technologies reviewed here can help to maintain data integrity and provide comprehensive documentation on how the project was implemented, thereby facilitating the integration of data and enabling cross-collaborative discoveries to be made.
Conflict of Interest Statement
The authors declare that the research was conducted in the absence of any commercial or financial relationships that could be construed as a potential conflict of interest.
Acknowledgments
The work described in this manuscript was supported in part by Award Number P01DA022446 (JMJ) from the National Institute on Drug Abuse. The content is solely the responsibility of the authors and does not necessarily represent the official views of the National Institute on Drug Abuse or the National Institutes of Health. The work was also supported by internal funds from the Renaissance Computing Institute. The authors would like to acknowledge Dr. Karamarie Fecho of Copperline Professional Solutions, LLC, for the review and editing of this manuscript. NICHD P30 HD03110 awarded to J. Piven.
Footnotes
- ^http://www.ctsaweb.org/
- ^http://www.dropbox.com/
- ^http://docs.(google).com
- ^http://sharepoint.microsoft.com
- ^http://www.joomla.org/
- ^http://drupal.org/
- ^http://www.dspace.org/
- ^http://www.kitware.com/products/midas.html
- ^http://www.xnat.org/
- ^http://www.irods.org/
- ^http://www.tm4.org/madam.html
- ^http://www.jboss.org/teiid
- ^http://hadoop.apache.org
References
Bennett, L. M., Gadlin, H., and Levine-Finley, S. (2010). Collaboration and Team Science: A Field Guide. Bethesda, MD: National Institutes of Health.
Burchinal, M., and Neebe, E. (2006). Data management: recommended practices. Monogr. Soc. Res. Child Dev. 71, 9–23.
Carson, P., and Dent, N. (2007). Good Clinical, Laboratory and Manufacturing Practices: Techniques for the QA Professional. Cambridge, UK: Royal Society of Chemistry.
Chang, F., Dean, J., Ghemawat, S., Hsieh, W. C., Wallach, D. A., Burrows, M., Chandra, T., Fikes, A., and Gruber, R. E. (2006). “Bigtable: a distributed storage system for structured data,” in OSDI’06: Seventh Symposium on Operating System Design and Implementation, Seattle, WA.
Costafreda, S. G. (2009). Pooling fMRI data: meta-analysis, mega-analysis and multi-center studies. Front. Neuroinform. 3:33. doi: 10.3389/neuro.11.033.2009
Coulehan, M. B., and Wells, J. F. (2005). Guidelines for Responsible Data Management in Scientific Research. Bethesda, MD: U.S. Department of Health and Human Services.
Hedges, L. B., and Olkin, I. (1985). Statistical Methods for Meta-Analysis. Stanford, CA: Academic Press.
Ishikawa, K., and Loftus, J. H. (1990). Introduction to Quality Control. Tokyo: 3A Corporation.Bennett, 448.
Laird, A. R., McMillan, K. M., Lancaster, J. L., Kochunov, P., Turkeltaub, P. E., Pardo, J. V., and Fox, P. T. (2005). A comparison of label-based review and ALE meta analysis in the stroop task. Hum. Brain Mapp. 25, 6–21.
Mier, D., Kirsch, P., and Meyer-Lindenberg, A. (2010). Neural substrates of pleiotropic action of genetic variation in COMT: a meta-analysis. Mol. Psychiatry 15, 918–927.
O’Connor, B. D., Merriman, B., and Nelson, S. F. (2011). SeqWare Query Engine: storing and searching sequence data in the cloud. BMC Bioinformatics 11(Suppl. 12), S2. doi: 10.1186/1471-2105-11-S12-S2
Owens, P., Shoffner, M., Wang, X., Schmitt, C. P., Lamm, B., and Mustafa, J. (2011). Secure Medical Workspace Prototype. Technical Report TR-11-01. Chapel Hill, NC: RENCI.
Rajendra, B., and Frew, J. (2005). Lineage retrieval for scientific data processing: a survey. ACM Comput. Surv. 37, 1–28.
Searls, D. B. (2005). Data integration: challenges for drug discovery. Nat. Rev. Drug Discov. 4, 45–58.
Stamatis, D. H. (2004). Six Sigma Fundamentals: A Complete Guide to the System, Methods, and Tools. New York: Productivity Press.
Stokols, D., Hall, K. L., Taylor, B. K., and Moser, R. P. (2008). The science of team science: overview of the field and introduction to the supplement. Am. J. Prevent. Med. 35, S77–S89.
Thompson, P. M., Martin, N. G., and Wright, M. J. (2010). Imaging genomics. Curr. Opin. Neurol. 23, 368–373.
U. S. Food and Drug Administration. (2003). Storage and Retrieval of Records and Data. Part 58: Good Laboratory Practice for Nonclinical Laboratory Studies. Subpart J: Records and Report. Code of Federal Regulations. Title 21, Section 58.190. Silver Spring, MD: U.S. Food and Drug Administration.
Wuchty, S., Jones, B. F., and Uzzi, B. (2007). The increasing dominance of teams in production of knowledge. Science 316, 1036–1039.
Keywords: data management, collaborative research, data entry, data integration
Citation: Schmitt CP and Burchinal M (2011) Data management practices for collaborative research. Front. Psychiatry 2:47. doi: 10.3389/fpsyt.2011.00047
Received: 20 February 2011; Accepted: 11 July 2011;
Published online: 22 July 2011.
Edited by:
Josephine Johns, University of North Carolina at Chapel Hill, USAReviewed by:
Naveen Ashish, University of California Irvine, USARoger Bakeman, Georgia State University, USA
Copyright: © 2011 Schmitt and Burchinal. This is an open-access article subject to a non-exclusive license between the authors and Frontiers Media SA, which permits use, distribution and reproduction in other forums, provided the original authors and source are credited and other Frontiers conditions are complied with.
*Correspondence: Charles P. Schmitt, Renaissance Computing Institute, University of North Carolina, Suite 540, 100 Europa Drive, Chapel Hill, NC 27517, USA. e-mail:Y3NjaG1pdHRAcmVuY2kub3Jn