- 1Department of Psychiatry, Melbourne Neuropsychiatry Centre, The University of Melbourne, Melbourne, VIC, Australia
- 2Section of Psychiatry, Department of Clinical Neuroscience, Karolinska Institutet, Stockholm, Sweden
- 3Department of Clinical Science, Intervention and Technology (CLINTEC), Division of Medical Imaging and Technology, Karolinska Institutet, Stockholm, Sweden
- 4Monash Clinical and Imaging Neuroscience, School of Psychology and Psychiatry, Monash University, Clayton, VIC, Australia
- 5School of Psychological Sciences, Monash University, Melbourne, VIC, Australia
- 6Department of Medicine Huddinge, Karolinska Institutet, Stockholm, Sweden
- 7Primary Care Research Unit, Department of General Practice, The University of Melbourne, Melbourne, VIC, Australia
- 8Centre for Family Medicine (CeFAM), Department of Neurobiology, Care Sciences and Society, Karolinska Institutet, Stockholm, Sweden
- 9Department of Medical Physics, Karolinska University Hospital Huddinge, Stockholm, Sweden
- 10Department of Radiology, Karolinska University Hospital, Stockholm, Sweden
- 11Department of Energy and Engineering, Swedish University of Agricultural Sciences, Uppsala, Sweden
Psychomotor disturbances are a classic feature of major depressive disorders. These can manifest as lack of facial expressions and decreased speech production, reduced body posture and mobility, and slowed voluntary movement. The neural correlates of psychomotor disturbances in depression are poorly understood but it has been suggested that outputs from the cingulate motor area (CMA) to striatal motor regions, including the putamen, could be involved. We used functional and structural magnetic resonance imaging to conduct a region-of-interest analysis to test the hypotheses that neural activation patterns related to motor production and gray matter volumes in the CMA would be different between depressed subjects displaying psychomotor disturbances (n = 13) and matched healthy controls (n = 13). In addition, we conducted a psychophysiological interaction analysis to assess the functional coupling related to self-paced finger-tapping between the caudal CMA and the posterior putamen in patients compared to controls. We found a cluster of increased neural activation, adjacent to a cluster of decreased gray matter volume in the caudal CMA in patients compared to controls. The functional coupling between the left caudal CMA and the left putamen during finger-tapping task performance was additionally decreased in patients compared to controls. In addition, the strength of the functional coupling between the left caudal CMA and the left putamen was negatively correlated with the severity of psychomotor disturbances in the patient group. In conclusion, we found converging evidence for involvement of the caudal CMA and putamen in the generation of psychomotor disturbances in depression.
Introduction
Psychomotor disturbance is a cardinal feature of major depressive disorder. The severity of psychomotor disruption is clinically associated with depression severity and predicts response to certain pharmacological treatments (1–4). Psychomotor disturbances transcend illness phase and manifest as changes in interactiveness and spontaneity, alongside reductions in facial expression, body mobility, postural tone, speed of movement, and speech (5–7). Investigations of the neural correlates underpinning psychomotor disturbances remain sparse; indeed, the motor system has been relatively neglected in brain imaging studies of psychiatric disorders in general (8). However, available evidence points to metabolic deficits, neurochemical changes, and altered structural connections and functional connectivity involving large-scale brain networks that connect frontal cortical regions and subcortical (esp. basal ganglia) areas (9–24). Interactions between the striatum, frontal motor regions, and prefrontal association cortices are known to be critical to the initiation and regulation of motor output and cognitive processing (25, 26). However, the localization and relevance of brain abnormalities in these fronto-striatal systems to psychomotor disturbances observed in depression remains largely unknown.
One important target for investigation of psychomotor disturbances is the cingulate cortex. Converging evidence supporting the involvement of the cingulate cortex in the clinical expression of major depressive disorder comes through experimental studies using interventions, such as sadness induction (27), cognitive behavioral therapy (28), pharmacological probes, such as antidepressants and dopamine-modulating treatments (29, 30), or neuromodulation such as electroconvulsive therapy or transcranial magnetic stimulation (31–35). Additionally, secondary cingulate cortex lesions can also lead to a neuropsychiatric condition known as akinetic mutism that involves severe alterations of volition, psychomotor slowing, and apathy – clinical features that resemble severe major depressive disorder (36, 37).
The cingulate cortex has been suggested to integrate volition, affect, and behavior. It is located on the midline rim of the corpus callosum and is generally divided anatomically into four distinct subregions (38, 39). One of these subregions is the midcingulate region, which contains the cingulate motor area (CMA) (40, 41). The CMA is a cortical midline structure, located in the posterior frontal lobe, superior to the corpus callosum, and inferior to the supplementary motor area. The CMA has been implicated in motor behaviors with affective incentives (42) and receives neural signals from affective limbic regions, frontal executive regions, and motor regions (37, 43). A caudal subregion of the CMA has long been implicated in the execution of simple motor tasks, but only recently has its somatotopy and functional organization been determined with more spatially precise brain imaging (44–47). Functional connectivity and retrograde tracings in primates suggest inputs from caudal CMA to lateral putamen in general, and to rostral ventral putamen in particular, overlap with inputs from primary motor areas (48, 49).
There is currently no empirically validated functional anatomical model of psychomotor disturbances in major depressive disorder. However, within the anatomical framework of large-scale brain networks, Vogt proposed one such pathophysiological model in which a loss of neurons in the cortical output layer (layer V) of the cingulate cortex specifically attenuates the output from the CMA to subcortical motor regions, resulting in a paucity of internally guided movement and speech (50). Clinically, this neural disruption could be reflected in the altered response to external events and slowed movements that characterize psychomotor disturbances observed in major depressive disorder.
Using the framework of Vogt’s theory of movement paucity in major depressive disorder, we hypothesized that psychomotor disturbances in major depressive disorder rely on structural and functional abnormalities in a network encompassing the CMA and striatal motor regions. We predict that participants with major depressive disorder show modifications of functional activation in left CMA and left posterior putamen during a task involving the generation of rapid right-lateralized finger movements (i.e., self-paced finger-tapping). We also anticipate that these functional alterations are accompanied by modifications of gray matter volume in both the left CMA and left putamen. Finally, the severity of both functional and structural abnormalities is predicted to correlate with the degree of observed psychomotor disturbances.
Materials and Methods
Study Sample
The Karolinska University Hospital and Stockholm City Council Ethics Committee approved the study protocol. Each subject gave oral and written informed consent to participate in this study. Thirteen patients with a bipolar I diagnosis (n = 9), bipolar II diagnosis (n = 1), or unipolar depression diagnosis (n = 3) were recruited from The Affective Disorders Unit at Psychiatry Southwest at Karolinska University Hospital in Huddinge, Sweden. All patients were experiencing a current episode of depression with a duration > 1 month and featuring psychomotor disturbances. No patient fulfilled the diagnostic criteria for concurrent mania, hypomania, or rapid cycling disorder. Thirteen healthy controls without psychiatric diagnoses were recruited. Clinical diagnoses were confirmed using a computerized version of the Structured Clinical Interview for DSM Disorders (51). All participants were right handed, had no history of neurologic illness, and had normal or corrected-to-normal visual acuity (52). All patients were on medication (Table 1), and all controls were drug free. Depression severity was rated by Benny Liberg and Mats Adler with the Montgomery Åsberg Depression Rating Scale (53) and psychomotor disturbance was rated with the CORE scale (54). Retrospective assessment of patient files did not reveal the presence of extrapyramidal symptoms or signs in medicated patients.
Image acquisition
Participants performed finger-tapping while in the MRI-scanner, following instructions on a computer screen viewed through a head-coil mounted mirror. Before the experiment started, participants were shown how to perform finger-tapping, defined as a thumb-index finger opposition of the right hand. Participants were asked to tap as quickly as possible. The experiment had an ON/OFF design consisting of 20 s of finger-tapping followed by 20 s of rest. During the first second of each ON-period, the instruction “tap” was presented, and the screen then turned black for 19 s. Rest periods were indicated by a fixation-cross presented for 20 s. This stimulus cycle was repeated seven times. The total functional scanning time was 280 s.
A Siemens Avanto 1.5-T scanner was used to acquire blood oxygen level dependent (BOLD) sensitive T2*-weighted echo planar images. Each echo planar image comprised 22 axial slices with a resolution of 3.75 mm × 3.75 mm × 5 mm and an interslice interval of 1 mm. Volumes were acquired with a repetition time (TR) of 2.5 s, an echo time (TE) of 30 ms, a field-of-view of 64 mm × 64 mm, and a flip angle of 90°. The first six (dummy) volumes of each run were discarded to allow for T1 equilibration effects. A total of 112 volumes were acquired. After the functional scans had been collected, a T1-weighted anatomical image [magnetization prepared rapid acquisition gradient echo (MP-RAGE)], 128 slices; TR, 2400 ms; TE, 3.44 ms; with a voxel size of 1.3 mm × 1.3 mm × 1.3 mm] was acquired for all subjects. To rule out radiological signs of pathology, a consultant in neuroradiology (Maria Kristoffersen-Wiberg) assessed the anatomical scans of each subject.
Region-of-interest masks
We used the software GingerALE 2.3 to define the left caudal CMA mask. GingerALE allows for meta-analysis of human brain imaging studies using published co-ordinates in standard space (55). Co-ordinates were derived from a study that mapped the functional anatomy of the CMA at the single-subject level (44). Input foci data included co-ordinates in the left hemisphere that represented neural activation in the CMA during motor execution using the right hand. Significant clusters in the whole-brain analysis were determined by a (corrected using a Monte-Carlo approach) cluster significance threshold of p ≤ 0.05. The resulting ROI mask is illustrated in Figure 3. The ROI mask for the putamen region connecting to the caudal motor cortex was derived from the Oxford Imanova Striatal Connectivity Atlas (seven-regions) supplied with FSL (56).
Analysis of functional activations
Imaging data were analyzed using the FSL 5.0.2 software suite [The Oxford Centre for Functional MRI of the Brain (FMRIB), Oxford University, United Kingdom]. Data processing was carried out using the fMRI Expert Analysis Tool version 5.98. Rigid-body head motion correction was first performed (57). We subsequently de-noised the data using the multivariate ICA-based classifier FIX (58, 59), based on a conservative threshold of five components. Non-brain tissue was then removed (60) and the functional data were smoothed using a Gaussian kernel set to a full-width half-maximum (FWHM) of 6 mm. To account for time differences in slice acquisition, we performed slice-timing correction using Fourier-space time series phase shifting. In addition, we normalized the grand-mean intensity of the entire four-dimensional (4D) dataset by a single multiplicative factor and filtered out physiological noise using a high-pass temporal filter set to a period of 100 s. Registration of the data to standard anatomical space was undertaken with the high-resolution structural (T1) scan using boundary-based registration with BBR, and affine linear registration with FLIRT (61). Estimated transformations were subsequently applied to the co-registered functional data. The time series of each subject was modeled using a general linear model (GLM) containing a single predictor representing the on–off time-course of the experiment, convolved with a hemodynamic response function (gamma). Parameter estimates (PEs) were calculated for all brain voxels. Correction for local autocorrelation in the time series was undertaken using FILM (62).
The subject-specific contrast (COPE) images of the finger-tapping effect were then entered into a second-level group analysis. As our patient sample had a different sex distribution than our control group, we added age and sex as covariates in the regression model to remove their potential confounding effects. We also created a 4D covariate image of gray matter using the feat_gm_prepare script. The 4D image output containing gray matter partial volume information was inserted as voxel-dependent EVs in the GLM model for each subject (63). The higher level analysis was carried out using FMRIB’s local analysis of mixed effects stage 1 and stage 2 (64–66). Z (Gaussianized T/F) statistic images in the ROI analysis were thresholded using clusters determined by Z ≥ 2.3 and a (corrected using Gaussian random field theory) cluster significance threshold of p ≤ 0.05.
Analysis of functional connectivity
We performed a psychophysiological interaction (PPI) analysis to determine group differences in task-dependent alterations of functional coupling between the left caudal CMA and left putamen regions connected to caudal cortical motor regions. Analysis was performed in SPM8 using the preprocessed first-level data described above (preprocessed in FSL). A PPI analysis determines how the statistical dependency between the time courses of neural activation in a region-of-interest (ROI) and a targeted brain region depends on a task context (67). We extracted the BOLD time series data from each subject within a 5 mm sphere centered at the peak of the task-related group activation difference within the left CMA (see Results). At the first level of analysis, a GLM consisting of three predictors was specified: the left CMA time series data as a physiological regressor, the task-based model as psychological regressor, and the interaction term (the PPI) formed by their crossproduct. We entered first-level COPE images representing the interaction term into a second-level random effects analysis of group differences in a region of the left putamen connected to caudal cortical motor areas involved in movement. Inference was undertaken using two-sample T-tests restricted to a mask of the left putamen and corrected for multiple comparisons (p ≤ 0.05) based on minimum cluster-extent thresholds estimated using the AlphaSim permutation procedure (REST toolbox; http://pub.restfmri.net). Simulations were run using an uncorrected voxel-level threshold of p ≤ 0.05, across 1000 permutations, resulting in a minimum required cluster-threshold of 14 voxels (p ≤ 0.05, corrected at the mask level).
Analysis of gray matter volume
Paul Klauser and Benny Liberg inspected every image to assess the presence of artifacts or gross anatomical abnormalities that could impact image preprocessing. We estimated gray matter volume using voxel-based morphometry (VBM) implemented in SPM8 (http://www.fil.ion.ucl.ac.uk/spm/software/spm8/). Each participant’s T1-weighted anatomical scan was segmented into gray, white, and cerebrospinal fluid compartments using the VBM8 toolbox (http://dbm.neuro.uni-jena.de/vbm) set to default parameters. Native-space gray matter images were then spatially normalized to the DARTEL template in MNI standard space created from 550 healthy control subjects from the IXI-database (http://www.brain-development.org). For the generation of gray matter volumes, Jacobian determinants were used to modulate gray voxel intensities with non-linear warping only in order to preserve original gray matter volumes while discarding initial differences in brain sizes. The images were then smoothed with a 6 mm full-width-half-maximum Gaussian kernel prior to statistical analysis. A GLM was used to test for group differences in gray matter volume at each voxel within the CMA ROI mask, as implemented in Randomise (http://fsl.fmrib.ox.ac.uk/fsl/randomise). All results were corrected for multiple comparison type I error at the ROI mask level using a non-parametric cluster size-based procedure. We set the voxelwise cluster-forming threshold to T ≥ 2.5. Then, a clusterwise p-value corrected at the ROI level was calculated from a permutation test (10,000 permutations). Age and gender were entered as covariates in the GLM.
Correlation analyses
Calculation of Spearman’s Rho correlation coefficients between CORE ratings, imaging metrics, and neurobiological indices were assessed to determine associations between clinical phenomenology and brain abnormalities determined with different imaging modalities.
Results
Functional Activation
In our group, comparison of task-related neural activation in the caudal CMA during self-paced finger-tapping, we found that patients activated the left caudal CMA more than healthy controls (cluster size: 356 voxels/2848 mm3; ZCMA = 3.01; p = 0.003, corrected at the cluster level; peak voxel: x = −6, y = −10, z = 38; Figure 1; Table 2).
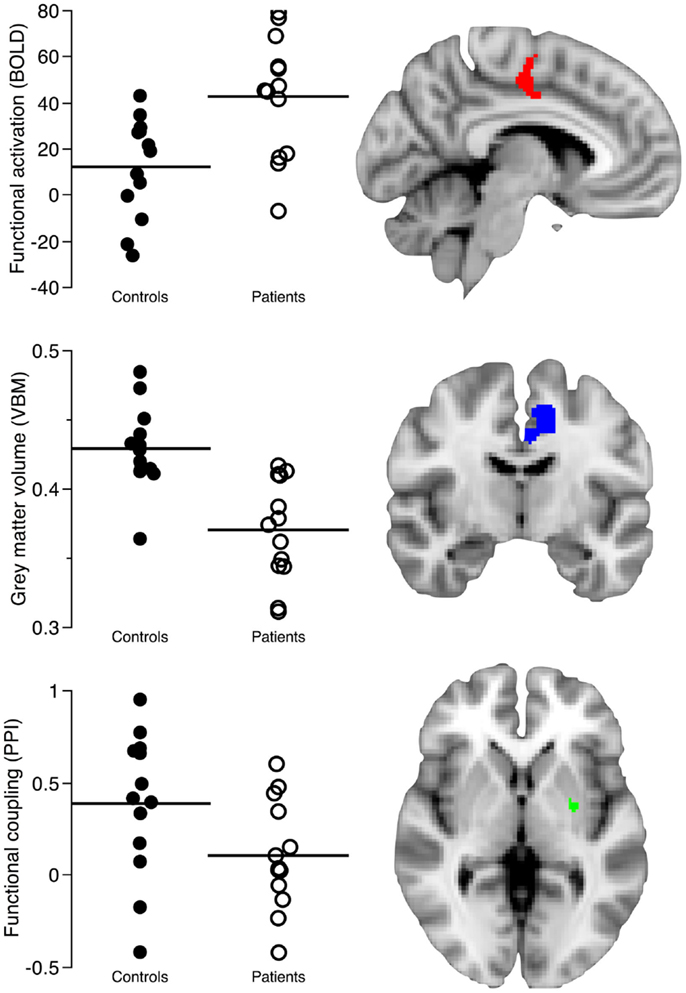
Figure 1. The image shows the structural and functional imaging findings in the left caudal cingulate motor area (CMA) and putamen regions connected to caudal motor regions in the frontal lobe. The first row shows the contrast parameter estimates reflecting how patients activate the caudal cingulate motor regions more than healthy controls (Z > 2.3, p = 0.05, corrected) during self-paced finger-tapping. The second row shows decreased gray matter volume in the left CMA of patients (T > 2.5, p = 0.05, corrected). The third row shows the decreased functional coupling of the left CMA and left posterior putamen regions connected to caudal motor regions in the frontal lobe during self-paced finger-tapping (p = 0.02, corrected). Metrics (mean) from clusters of between-group differences in the left caudal CMA and posterior putamen were retrieved using masks comprising 356 voxels/2848 mm3 from the functional magnetic resonance imaging analysis, 941 voxels/3176 mm3 from the voxel-based morphometry analysis, and 31 voxels/248 mm3 from the psychophysiological interaction analysis.
Functional Connectivity
In our PPI analysis of task-related functional coupling between the caudal CMA and the posterior putamen regions connected to caudal motor regions in the frontal lobe, we found significant group differences (cluster size: 31 voxels/248 mm3, p = 0.02, corrected; peak voxel: x = −26, y = −6, z = 0; Figure 1). We found that neural activation in controls showed a task-related correspondence between the left caudal CMA and left posterior putamen regions connected to caudal motor regions in the frontal lobe, whereas this relationship was absent in the patients.
Gray Matter Volume
In our comparison of left CMA gray matter volume in patients and controls, we observed one cluster of decreased gray matter volume in the patient group that encompassed the left caudal CMA (cluster size: 941 voxels/3176 mm3; TCMA = 6.79; p = 0.01, corrected at the cluster level; peak voxel: x = −9, y = 4.5, z = 33; Figures 1 and 2).
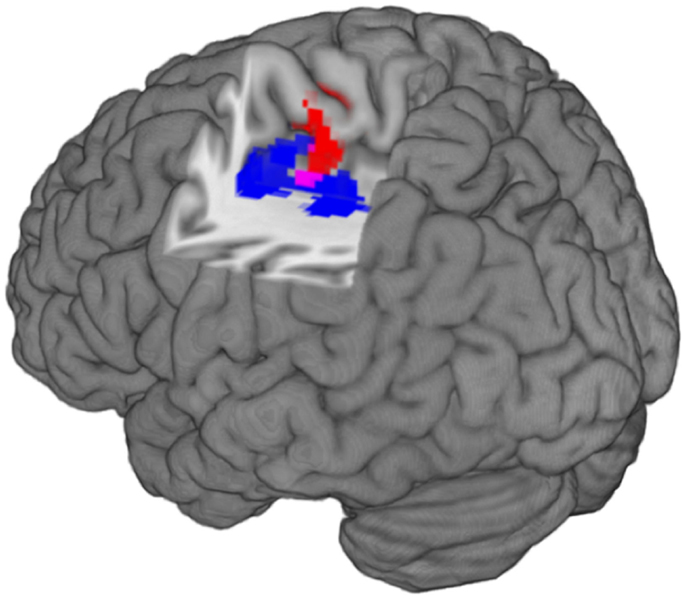
Figure 2. The image shows the localization of structural and functional imaging findings in the left caudal cingulate motor. Left is left in the image. The red cluster represents increased functional activation in patients compared to controls (Z > 2.3, p = 0.05, corrected). The blue cluster represents gray matter volume decreases in patients compared to controls (T > 2.5, p = 0.05, corrected). The purple voxels represent the overlap between function and structural findings.
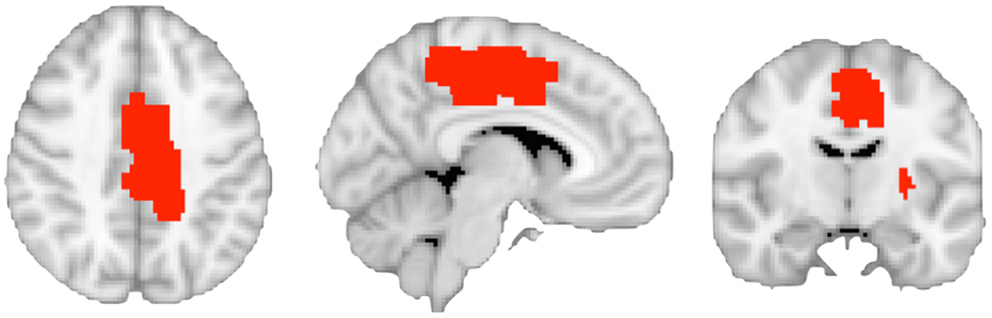
Figure 3. The image shows the binarized region of interest masks defining the left caudal cingulate motor area and the left putamen regions connected to caudal motor regions in the frontal lobe.
Cross-Modality Relationships
We found a statistically significant and negative correlation (rho: −0.57, p = 0.002; Table 3) between mean functional activation (356 voxels/2848 mm3) and gray matter volume (941 voxels/3176 mm3) in the left caudal CMA across both groups. There was also a statistically significant and positive correlation (rho: 0.52, p = 0.006, Table 3) between gray matter volume (941 voxels/3176 mm3) and functional coupling of the left caudal CMA and left posterior putamen regions connected to caudal motor regions in the frontal lobe (31 voxels/248 mm3) across both groups. The spatial overlap of between-group differences in gray matter volume and functional activation is shown in Figure 2.
Clinical Correlations
We found a statistically significant and negative correlation (rho = −0.57; p = 0.04, Table 3) between clinical ratings of psychomotor disturbances (CORE) in patients and functional coupling of the left caudal CMA and left posterior putamen regions connected to caudal motor regions in the frontal lobe (356 voxels/2848 mm3). We did not find a significant correlation (rho = 0.39; p = 0.18) between CORE ratings and functional activation of the left caudal CMA (356 voxels/2848 mm3) or between CORE ratings and gray matter volume in the left caudal CMA (rho = −0.11; p = 0.71; 941 voxels/3176 mm3).
Discussion
In the present study, we used structural and functional neuroimaging to investigate the contribution of the CMA to psychomotor disturbances in major depressive disorder. By combining neuroimaging modalities, we found converging evidence supporting a neural model of psychomotor disturbances that involves a fronto-striatal network contributing to motor execution. Specifically, we found that (1) patients activated the CMA more than healthy controls during right-handed self-paced finger-tapping; (2) functional coupling of the CMA and putamen was absent in patients compared to controls during task performance; (3) patients had decreased gray matter volume in the CMA; (4) clinical ratings of psychomotor disturbance in patients were negatively correlated with functional coupling of the CMA and putamen; and (5) gray matter volume of the CMA region with between-group differences was negatively correlated with functional activation, and positively correlated with functional coupling.
Our study lends experimental support to the involvement of a fronto-striatal network in the emergence of psychomotor disturbances in major depressive disorder. In our study, gray matter volume decreases were associated with decreased functional coupling of the left caudal CMA and left posterior putamen during motor execution of a right-handed self-paced finger-tapping task. The midcingulate region comprising the CMA is known to provide extensive output to skeletomotor areas, including the striatum (41). Changes in the CMA would, therefore, impact upon its glutamatergic inputs to subcortical motor systems and, in turn, lead to impairments in motor behavior, i.e., psychomotor disturbances. Although beyond the scope of the current study, this finding may be relevant to neuron loss in cortical layer V, from which efferent fibers are derived, in accordance with Vogt’s model of motor dysfunction (50).
It is interesting to note the relative position of the clusters resulting from the functional and the structural analysis: few voxels show an overlap between the functional cluster representing increased neural activity and the structural cluster representing decreased gray matter volume in the CMA of patients (Figure 2). The interpretation of the spatial extent of clusters, resulting from cluster-based statistics is known to be problematic, especially when relatively low cluster-forming thresholds are used like in this study (i.e., T > 2.5) (68). Nevertheless, the increase of functional activation in an area that seems to be spared by gray matter volume loss may represent a less affected region or a compensatory mechanism. Compensatory cortical plasticity has been previously described after acute brain lesions but a similar mechanism could also be involved following more chronic alterations of brain networks in the context of mental illness (69).
This study conforms to previous studies that have shown paralimbic hyperactivation in major depressive disorder using both molecular metabolic imaging and functional magnetic resonance imaging with BOLD (70, 71). Hypothetically, a loss of neurons in cingulate layer V suggested by Vogt et al. (50) could arise from excess glutamate signaling with impaired plasticity and neural resilience (72). However, the BOLD signal comprises several neuronal and vascular factors that preclude a specific association of increased activation and hyperglutamatergia (73). Indirect correlational evidence for cingulate hyperglutamatergia may be inferred from the positive correlation of glutamate signaling and spontaneous neural activity of paralimbic medial frontal and posterior cingulate regions: the so-called “default-mode” network (74, 75). Several studies suggest that this network is hyperactivated in major depressive disorder (76–78). In a previous study of the bipolar disorder subgroup of this sample (n = 9), there was an increased activation among depressed patients in the default-mode network during motor execution (15). However, the opposite association between glutamate, functional connectivity, and depression severity has also been shown (79). Thus, we remain cautious regarding definitive interpretations of our findings in the context of the pathophysiology underlying psychomotor disturbances in major depressive disorder.
Our study has limitations. First, this is a ROI study where we had an a priori hypothesis involving selected brain regions in the left hemisphere known to activate during simple right-hand movements. The disadvantage of the ROI approach is that it discerns a comprehensive observation of the full large-scale neuronal networks implicated in these disturbances (80). However, our motivation for choosing the ROI approach was that our hypothesis addressed specific ideas based on previous research on the CMA in major depressive disorder. An advantage of the ROI approach is that it potentially increases the sensitivity of the neuroimaging methods we applied, and previous research suggests that ROI analyses may provide a better statistical control of both type I and type II errors than in whole-brain analyses as the ROI approach is less dependent on correction for multiple comparisons (81). This becomes even more important in smaller samples such as ours. Second, our brain imaging approach is restricted to inference from indirect monitoring of the disease underlying major depressive disorder. Functional imaging studies and anatomical mapping advocate a limbic hyperactivation in major depressive disorder and suggest that more anterior cingulate cortex regions convey limbic signals to the CMA, which in turn suggests that our observed CMA alterations may only be a tertiary marker for a disease that alters upstream nodes in the same neural network (29, 43). The BOLD contrast mechanism itself also highlights the importance of inputs to CMA (82). Third, the CMA and putamen are both rich in ascending midbrain dopamine afferents that contribute to the initiation, preparation, execution, and control of movement (56, 83). Six patients in our sample were treated with antipsychotic medication that affects dopamine transmission in both regions. This could potentially lead to misrepresentative and biased results. However, neuroimaging metrics in these six individuals were distributed across the whole patient sample, and there were no outliers in the patient group with respect to functional activation, gray matter volume, or functional coupling. Fourth, there are many types of motor behavior, and it is possible that finger-tapping does not engage identical fronto-striatal networks in the same way as more elaborate motor behavior. The strength of the finger-tapping task is that it is sensitive to pathology in fronto-striatal neurocircuitry and is considered to measure a defined entity: motor speed (84). Fifth, the BOLD signal in the CMA may be subject to noise due to partial volume effects because of the spatial resolution of our functional scans. This contributes to a possible source of error in our PPI analysis. Finally, given the small sample size of our groups, the GLM may not provide the best fit of our second-level data. However, we analyzed our data at second level using a mixed effects analysis (FMRIB’s Local Analysis of Mixed Effects stage 1 and stage 2) similar to a vast majority of comparable functional imaging studies. In order to confirm the validity of our results, we also re-analyzed data at second level with Randomise for non-parametric inference while using an identical statistical threshold. The spatial extent of those statistically significant results was close to identical regarding the CMA cluster of between-group differences. We would like to reiterate that even if the GLM does not provide the best fit for our second level analysis, the corrected p-value calculated by Randomise is valid, regardless the distribution of our data.
Conclusion
Our data are cross-sectional but implicates the possibility of a chain of events where primary neural hyperactivation in paralimbic regions has secondary effects on gray matter volume in fronto-striatal networks involved in movement. In our study, functional activation was negatively correlated with gray matter volume, which suggests an association between paralimbic hyperactivation and gray matter alterations. The positive correlation between gray matter volume and functional coupling supports the notion of diminished cortical output via layer V to subcortical regions that are involved in motor control. The functional coupling was also negatively correlated with clinical ratings, which suggests a role of decreased functional connectivity within fronto-striatal networks in the emergence of clinical psychomotor disturbances in major depressive disorder. Thus, our results propose an involvement of the CMA in the generation of psychomotor disturbances in a major depressive disorder. Our data also suggest the possibility of elucidating the causality of these events by using an experimental longitudinal study design and analyses of directional effective connectivity within these networks during motor performance.
Author Contributions
All authors made substantial contributions to the conception and design of this work. Benny Liberg and Mats Adler collected the original data. Benny Liberg, Paul Klauser, and Ian H. Harding performed the analyses. All authors contributed to the interpretation of data for this work. Benny Liberg, Paul Klauser, and Ian H. Harding drafted the manuscript, which all authors critically revised for important intellectual content. All authors approved the final version of the manuscript. All authors agree to be accountable for all aspects of the work in ensuring that questions related to the accuracy or integrity of any part of the work are appropriately investigated and resolved.
Conflict of Interest Statement
The authors declare that the research was conducted in the absence of any commercial or financial relationships that could be construed as a potential conflict of interest.
Acknowledgments
We thank Kerstin Eriksson at the MRI Unit, Radiology Clinic, Karolinska University Hospital Huddinge for clinical assistance during scanning. We thank Andrew Zalesky for valuable comments on methodology. We also thank staff members of the Section of Affective Disorders, the Southwest Psychiatric Clinic (PSSV), Stockholm County Council, for assistance with recruiting patients. Benny Liberg received funding from Svenska Läkaresällskapet (The Swedish Society of Medicine, SLS-403101), the Southwest Psychiatric Clinic (PSSV), Stockholm County Council, and the Strategic Research Committee, Karolinska Institutet/Stockholm County Council, Sweden. Paul Klauser was supported by the Swiss National Science Foundation and the Swiss Society for Medicine and Biology Scholarships (ID: 148384). Professor Christos Pantelis was supported by a NHMRC Senior Principal Research Fellowship, Australia (ID: 628386).
References
1. Buyukdura JS, McClintock SM, Croarkin PE. Psychomotor retardation in depression: biological underpinnings, measurement, and treatment. Prog Neuropsychopharmacol Biol Psychiatry (2011) 35(2):395–409. doi: 10.1016/j.pnpbp.2010.10.019
Pubmed Abstract | Pubmed Full Text | CrossRef Full Text | Google Scholar
2. Schrijvers D, Hulstijn W, Sabbe BG. Psychomotor symptoms in depression: a diagnostic, pathophysiological and therapeutic tool. J Affect Disord (2008) 109(1–2):1–20. doi:10.1016/j.jad.2007.10.019
Pubmed Abstract | Pubmed Full Text | CrossRef Full Text | Google Scholar
3. Sobin C, Mayer L, Endicott J. The motor agitation and retardation scale: a scale for the assessment of motor abnormalities in depressed patients. J Neuropsychiatry Clin Neurosci (1998) 10(1):85–92.
5. Berk M, Dodd S, Kauer-Sant’anna M, Malhi GS, Bourin M, Kapczinski F, et al. Dopamine dysregulation syndrome: implications for a dopamine hypothesis of bipolar disorder. Acta Psychiatr Scand Suppl (2007) (434):41–9. doi:10.1111/j.1600-0447.2007.01058.x
Pubmed Abstract | Pubmed Full Text | CrossRef Full Text | Google Scholar
6. Malhi GS, Berk M. Does dopamine dysfunction drive depression? Acta Psychiatr Scand Suppl (2007) (433):116–24. doi:10.1111/j.1600-0447.2007.00969.x
Pubmed Abstract | Pubmed Full Text | CrossRef Full Text | Google Scholar
7. Parker G, Hadzi-Pavlovic D, Brodaty H, Boyce P, Mitchell P, Wilhelm K, et al. Psychomotor disturbance in depression: defining the constructs. J Affect Disord (1993) 27(4):255–65. doi:10.1016/0165-0327(93)90049-P
Pubmed Abstract | Pubmed Full Text | CrossRef Full Text | Google Scholar
8. Kupferschmidt DA, Zakzanis KK. Toward a functional neuroanatomical signature of bipolar disorder: quantitative evidence from the neuroimaging literature. Psychiatry Res (2011) 193(2):71–9. doi:10.1016/j.pscychresns.2011.02.011
Pubmed Abstract | Pubmed Full Text | CrossRef Full Text | Google Scholar
9. Bracht T, Federspiel A, Schnell S, Horn H, Hofle O, Wiest R, et al. Cortico-cortical white matter motor pathway microstructure is related to psychomotor retardation in major depressive disorder. PLoS One (2012) 7(12):e52238. doi:10.1371/journal.pone.0052238
Pubmed Abstract | Pubmed Full Text | CrossRef Full Text | Google Scholar
10. Caligiuri MP, Brown GG, Meloy MJ, Eberson SC, Kindermann SS, Frank LR, et al. An fMRI study of affective state and medication on cortical and subcortical brain regions during motor performance in bipolar disorder. Psychiatry Res (2003) 123(3):171–82. doi:10.1016/S0925-4927(03)00075-1
Pubmed Abstract | Pubmed Full Text | CrossRef Full Text | Google Scholar
11. Lohr JB, Caligiuri MP. Abnormalities in motor physiology in bipolar disorder. J Neuropsychiatry Clin Neurosci (2006) 18(3):342–9. doi:10.1176/appi.neuropsych.18.3.342
Pubmed Abstract | Pubmed Full Text | CrossRef Full Text | Google Scholar
12. Exner C, Lange C, Irle E. Impaired implicit learning and reduced pre-supplementary motor cortex size in early-onset major depression with melancholic features. J Affect Disord (2009) 119(1–3):156–62. doi:10.1016/j.jad.2009.03.015
Pubmed Abstract | Pubmed Full Text | CrossRef Full Text | Google Scholar
13. Hickie IB, Naismith SL, Ward PB, Little CL, Pearson M, Scott EM, et al. Psychomotor slowing in older patients with major depression: relationships with blood flow in the caudate nucleus and white matter lesions. Psychiatry Res (2007) 155(3):211–20. doi:10.1016/j.pscychresns.2007.01.006
Pubmed Abstract | Pubmed Full Text | CrossRef Full Text | Google Scholar
14. Liberg B, Adler M, Jonsson T, Landen M, Rahm C, Wahlund LO, et al. The neural correlates of self-paced finger tapping in bipolar depression with motor retardation. Acta Neuropsychiatr (2013) 25(1):43–51. doi:10.1111/j.1601-5215.2012.00659.x
15. Liberg B, Adler M, Jonsson T, Landen M, Rahm C, Wahlund LO, et al. Motor imagery in bipolar depression with slowed movement. J Nerv Ment Dis (2013) 201(10):885–93. doi:10.1097/NMD.0b013e3182a5c2a7
Pubmed Abstract | Pubmed Full Text | CrossRef Full Text | Google Scholar
16. Marchand WR, Lee JN, Suchy Y, Johnson S, Thatcher J, Gale P. Aberrant functional connectivity of cortico-basal ganglia circuits in major depression. Neurosci Lett (2012) 514(1):86–90. doi:10.1016/j.neulet.2012.02.063
Pubmed Abstract | Pubmed Full Text | CrossRef Full Text | Google Scholar
17. Marchand WR, Lee JN, Thatcher GW, Jensen C, Stewart D, Dilda V, et al. A functional MRI study of a paced motor activation task to evaluate frontal-subcortical circuit function in bipolar depression. Psychiatry Res (2007) 155(3):221–30. doi:10.1016/j.pscychresns.2007.03.003
Pubmed Abstract | Pubmed Full Text | CrossRef Full Text | Google Scholar
18. Marchand WR, Lee JN, Thatcher J, Thatcher GW, Jensen C, Starr J. A preliminary longitudinal fMRI study of frontal-subcortical circuits in bipolar disorder using a paced motor activation paradigm. J Affect Disord (2007) 103(1–3):237–41. doi:10.1016/j.jad.2007.01.008
Pubmed Abstract | Pubmed Full Text | CrossRef Full Text | Google Scholar
19. Walther S, Hugli S, Hofle O, Federspiel A, Horn H, Bracht T, et al. Frontal white matter integrity is related to psychomotor retardation in major depression. Neurobiol Dis (2012) 47(1):13–9. doi:10.1016/j.nbd.2012.03.019
Pubmed Abstract | Pubmed Full Text | CrossRef Full Text | Google Scholar
20. Bench CJ, Friston KJ, Brown RG, Frackowiak RS, Dolan RJ. Regional cerebral blood flow in depression measured by positron emission tomography: the relationship with clinical dimensions. Psychol Med (1993) 23(3):579–90. doi:10.1017/S0033291700025368
Pubmed Abstract | Pubmed Full Text | CrossRef Full Text | Google Scholar
21. Dolan RJ, Bench CJ, Liddle PF, Friston KJ, Frith CD, Grasby PM, et al. Dorsolateral prefrontal cortex dysfunction in the major psychoses; symptom or disease specificity? J Neurol Neurosurg Psychiatry (1993) 56(12):1290–4. doi:10.1136/jnnp.56.12.1290
Pubmed Abstract | Pubmed Full Text | CrossRef Full Text | Google Scholar
22. Brody AL, Saxena S, Mandelkern MA, Fairbanks LA, Ho ML, Baxter LR. Brain metabolic changes associated with symptom factor improvement in major depressive disorder. Biol Psychiatry (2001) 50(3):171–8. doi:10.1016/S0006-3223(01)01117-9
Pubmed Abstract | Pubmed Full Text | CrossRef Full Text | Google Scholar
23. Mayberg HS, Lewis PJ, Regenold W, Wagner HN Jr. Paralimbic hypoperfusion in unipolar depression. J Nucl Med (1994) 35(6):929–34.
24. Meyer JH, McNeely HE, Sagrati S, Boovariwala A, Martin K, Verhoeff NP, et al. Elevated putamen D(2) receptor binding potential in major depression with motor retardation: an [11C]raclopride positron emission tomography study. Am J Psychiatry (2006) 163(9):1594–602. doi:10.1176/appi.ajp.163.9.1594
Pubmed Abstract | Pubmed Full Text | CrossRef Full Text | Google Scholar
25. Frey SH, Fogassi L, Grafton S, Picard N, Rothwell JC, Schweighofer N, et al. Neurological principles and rehabilitation of action disorders: computation, anatomy, and physiology (CAP) model. Neurorehabil Neural Repair (2011) 25(5 Suppl):6S–20S. doi:10.1177/1545968311410940
Pubmed Abstract | Pubmed Full Text | CrossRef Full Text | Google Scholar
26. Rizzolatti G, Luppino G. The cortical motor system. Neuron (2001) 31(6):889–901. doi:10.1016/S0896-6273(01)00423-8
27. Mayberg HS, Liotti M, Brannan SK, McGinnis S, Mahurin RK, Jerabek PA, et al. Reciprocal limbic-cortical function and negative mood: converging PET findings in depression and normal sadness. Am J Psychiatry (1999) 156(5):675–82.
28. McGrath CL, Kelley ME, Holtzheimer PE, Dunlop BW, Craighead WE, Franco AR, et al. Toward a neuroimaging treatment selection biomarker for major depressive disorder. JAMA Psychiatry (2013) 70(8):821–9. doi:10.1001/jamapsychiatry.2013.143
Pubmed Abstract | Pubmed Full Text | CrossRef Full Text | Google Scholar
29. Seminowicz DA, Mayberg HS, McIntosh AR, Goldapple K, Kennedy S, Segal Z, et al. Limbic-frontal circuitry in major depression: a path modeling metanalysis. Neuroimage (2004) 22(1):409–18. doi:10.1016/j.neuroimage.2004.01.015
Pubmed Abstract | Pubmed Full Text | CrossRef Full Text | Google Scholar
30. Austin MP, Mitchell P, Hadzi-Pavlovic D, Hickie I, Parker G, Chan J, et al. Effect of apomorphine on motor and cognitive function in melancholic patients: a preliminary report. Psychiatry Res (2000) 97(2–3):207–15. doi:10.1016/S0165-1781(00)00222-5
Pubmed Abstract | Pubmed Full Text | CrossRef Full Text | Google Scholar
31. Liston C, Chen AC, Zebley BD, Drysdale AT, Gordon R, Leuchter B, et al. Default mode network mechanisms of transcranial magnetic stimulation in depression. Biol Psychiatry (2014) 76(7):517–26. doi:10.1016/j.biopsych.2014.01.023
Pubmed Abstract | Pubmed Full Text | CrossRef Full Text | Google Scholar
32. Ohnishi T, Hayashi T, Okabe S, Nonaka I, Matsuda H, Iida H, et al. Endogenous dopamine release induced by repetitive transcranial magnetic stimulation over the primary motor cortex: an [11C]raclopride positron emission tomography study in anesthetized macaque monkeys. Biol Psychiatry (2004) 55(5):484–9. doi:10.1016/j.biopsych.2003.09.016
33. Ohnishi T, Matsuda H, Imabayashi E, Okabe S, Takano H, Arai N, et al. rCBF changes elicited by rTMS over DLPFC in humans. Suppl Clin Neurophysiol (2004) 57:715–20. doi:10.1016/S1567-424X(09)70412-X
Pubmed Abstract | Pubmed Full Text | CrossRef Full Text | Google Scholar
34. Paillere Martinot ML, Martinot JL, Ringuenet D, Galinowski A, Gallarda T, Bellivier F, et al. Baseline brain metabolism in resistant depression and response to transcranial magnetic stimulation. Neuropsychopharmacology (2011) 36(13):2710–9. doi:10.1038/npp.2011.161
Pubmed Abstract | Pubmed Full Text | CrossRef Full Text | Google Scholar
35. McCormick LM, Yamada T, Yeh M, Brumm MC, Thatcher RW. Antipsychotic effect of electroconvulsive therapy is related to normalization of subgenual cingulate theta activity in psychotic depression. J Psychiatr Res (2009) 43(5):553–60. doi:10.1016/j.jpsychires.2008.08.004
Pubmed Abstract | Pubmed Full Text | CrossRef Full Text | Google Scholar
36. Tengvar C, Johansson B, Sorensen J. Frontal lobe and cingulate cortical metabolic dysfunction in acquired akinetic mutism: a PET study of the interval form of carbon monoxide poisoning. Brain Inj (2004) 18(6):615–25. doi:10.1080/02699050310001622806
Pubmed Abstract | Pubmed Full Text | CrossRef Full Text | Google Scholar
37. Paus T. Primate anterior cingulate cortex: where motor control, drive and cognition interface. Nat Rev Neurosci (2001) 2(6):417–24. doi:10.1038/35077500
Pubmed Abstract | Pubmed Full Text | CrossRef Full Text | Google Scholar
38. Palomero-Gallagher N, Vogt BA, Schleicher A, Mayberg HS, Zilles K. Receptor architecture of human cingulate cortex: evaluation of the four-region neurobiological model. Hum Brain Mapp (2009) 30(8):2336–55. doi:10.1002/hbm.20667
Pubmed Abstract | Pubmed Full Text | CrossRef Full Text | Google Scholar
39. Yu C, Zhou Y, Liu Y, Jiang T, Dong H, Zhang Y, et al. Functional segregation of the human cingulate cortex is confirmed by functional connectivity based neuroanatomical parcellation. Neuroimage (2011) 54(4):2571–81. doi:10.1016/j.neuroimage.2010.11.018
Pubmed Abstract | Pubmed Full Text | CrossRef Full Text | Google Scholar
40. Picard N, Strick PL. Motor areas of the medial wall: a review of their location and functional activation. Cereb Cortex (1996) 6(3):342–53. doi:10.1093/cercor/6.3.342
Pubmed Abstract | Pubmed Full Text | CrossRef Full Text | Google Scholar
41. Vogt BA, Berger GR, Derbyshire SW. Structural and functional dichotomy of human midcingulate cortex. Eur J Neurosci (2003) 18(11):3134–44. doi:10.1111/j.1460-9568.2003.03034.x
Pubmed Abstract | Pubmed Full Text | CrossRef Full Text | Google Scholar
42. Shima K, Tanji J. Role for cingulate motor area cells in voluntary movement selection based on reward. Science (1998) 282(5392):1335–8. doi:10.1126/science.282.5392.1335
Pubmed Abstract | Pubmed Full Text | CrossRef Full Text | Google Scholar
43. Morecraft RJ, Van Hoesen GW. Convergence of limbic input to the cingulate motor cortex in the rhesus monkey. Brain Res Bull (1998) 45(2):209–32. doi:10.1016/S0361-9230(97)00344-4
Pubmed Abstract | Pubmed Full Text | CrossRef Full Text | Google Scholar
44. Amiez C, Petrides M. Neuroimaging evidence of the anatomo-functional organization of the human cingulate motor areas. Cereb Cortex (2014) 24(3):563–78. doi:10.1093/cercor/bhs329
Pubmed Abstract | Pubmed Full Text | CrossRef Full Text | Google Scholar
45. Procyk E, Wilson CR, Stoll FM, Faraut MC, Petrides M, Amiez C. Midcingulate motor map and feedback detection: converging data from humans and monkeys. Cereb Cortex (2014). doi:10.1093/cercor/bhu213
Pubmed Abstract | Pubmed Full Text | CrossRef Full Text | Google Scholar
46. Picard N, Strick PL. Imaging the premotor areas. Curr Opin Neurobiol (2001) 11(6):663–72. doi:10.1016/S0959-4388(01)00266-5
Pubmed Abstract | Pubmed Full Text | CrossRef Full Text | Google Scholar
47. Paus T, Petrides M, Evans AC, Meyer E. Role of the human anterior cingulate cortex in the control of oculomotor, manual, and speech responses: a positron emission tomography study. J Neurophysiol (1993) 70(2):453–69.
48. Habas C. Functional connectivity of the human rostral and caudal cingulate motor areas in the brain resting state at 3T. Neuroradiology (2010) 52(1):47–59. doi:10.1007/s00234-009-0572-1
Pubmed Abstract | Pubmed Full Text | CrossRef Full Text | Google Scholar
49. Takada M, Tokuno H, Hamada I, Inase M, Ito Y, Imanishi M, et al. Organization of inputs from cingulate motor areas to basal ganglia in macaque monkey. Eur J Neurosci (2001) 14(10):1633–50. doi:10.1046/j.0953-816x.2001.01789.x
Pubmed Abstract | Pubmed Full Text | CrossRef Full Text | Google Scholar
50. Vogt BA, Fountolakis KN, Samaras D, Kövari E, Vogt LJ, Hof PR. Cingulate neuropathological substrates of depression. In: Vogt BA, editor. Cingulate Neurobiology and Disease. Oxford: Oxford University Press (2009). p. xxxiv, 829.
51. First MB, Spitzer RL, Gibbon M, Williams JB. Structured Clinical Interview for DSM-IV-TR Axis I Disorders, Research Version, Patient Edition (SCID-I/P). 4th ed. New York: Biometrics Research Department, New York State Psychiatric Institute (2001).
52. Oldfield RC. The assessment and analysis of handedness: the Edinburgh inventory. Neuropsychologia (1971) 9(1):97–113. doi:10.1016/0028-3932(71)90067-4
53. Asberg M, Montgomery SA, Perris C, Schalling D, Sedvall G. A comprehensive psychopathological rating scale. Acta Psychiatr Scand Suppl (1978) (271):5–27. doi:10.1111/j.1600-0447.1978.tb02357.x
54. Hickie I, Mason C, Parker G. Comparative validity of two measures of psychomotor function in patients with severe depression. J Affect Disord (1996) 37(2–3):143–9. doi:10.1016/0165-0327(95)00087-9
Pubmed Abstract | Pubmed Full Text | CrossRef Full Text | Google Scholar
55. Turkeltaub PE, Eden GF, Jones KM, Zeffiro TA. Meta-analysis of the functional neuroanatomy of single-word reading: method and validation. Neuroimage (2002) 16(3 Pt 1):765–80. doi:10.1006/nimg.2002.1131
56. Tziortzi AC, Haber SN, Searle GE, Tsoumpas C, Long CJ, Shotbolt P, et al. Connectivity-based functional analysis of dopamine release in the striatum using diffusion-weighted MRI and positron emission tomography. Cereb Cortex (2014) 24(5):1165–77. doi:10.1093/cercor/bhs397
Pubmed Abstract | Pubmed Full Text | CrossRef Full Text | Google Scholar
57. Jenkinson M, Bannister P, Brady M, Smith S. Improved optimization for the robust and accurate linear registration and motion correction of brain images. Neuroimage (2002) 17(2):825–41. doi:10.1006/nimg.2002.1132
Pubmed Abstract | Pubmed Full Text | CrossRef Full Text | Google Scholar
58. Griffanti L, Salimi-Khorshidi G, Beckmann CF, Auerbach EJ, Douaud G, Sexton CE, et al. ICA-based artefact removal and accelerated fMRI acquisition for improved resting state network imaging. Neuroimage (2014) 95:232–47. doi:10.1016/j.neuroimage.2014.03.034
Pubmed Abstract | Pubmed Full Text | CrossRef Full Text | Google Scholar
59. Salimi-Khorshidi G, Douaud G, Beckmann CF, Glasser MF, Griffanti L, Smith SM. Automatic denoising of functional MRI data: combining independent component analysis and hierarchical fusion of classifiers. Neuroimage (2014) 90:449–68. doi:10.1016/j.neuroimage.2013.11.046
Pubmed Abstract | Pubmed Full Text | CrossRef Full Text | Google Scholar
60. Smith SM. Fast robust automated brain extraction. Hum Brain Mapp (2002) 17(3):143–55. doi:10.1002/hbm.10062
61. Jenkinson M, Smith S. A global optimisation method for robust affine registration of brain images. Med Image Anal (2001) 5(2):143–56. doi:10.1016/S1361-8415(01)00036-6
Pubmed Abstract | Pubmed Full Text | CrossRef Full Text | Google Scholar
62. Woolrich MW, Ripley BD, Brady M, Smith SM. Temporal autocorrelation in univariate linear modeling of FMRI data. Neuroimage (2001) 14(6):1370–86. doi:10.1006/nimg.2001.0931
Pubmed Abstract | Pubmed Full Text | CrossRef Full Text | Google Scholar
63. Oakes TR, Fox AS, Johnstone T, Chung MK, Kalin N, Davidson RJ. Integrating VBM into the general linear model with voxelwise anatomical covariates. Neuroimage (2007) 34(2):500–8. doi:10.1016/j.neuroimage.2006.10.007
Pubmed Abstract | Pubmed Full Text | CrossRef Full Text | Google Scholar
64. Woolrich MW, Behrens TE, Beckmann CF, Jenkinson M, Smith SM. Multilevel linear modelling for FMRI group analysis using Bayesian inference. Neuroimage (2004) 21(4):1732–47. doi:10.1016/j.neuroimage.2003.12.023
Pubmed Abstract | Pubmed Full Text | CrossRef Full Text | Google Scholar
65. Woolrich MW, Jbabdi S, Patenaude B, Chappell M, Makni S, Behrens T, et al. Bayesian analysis of neuroimaging data in FSL. Neuroimage (2009) 45(1 Suppl):S173–86. doi:10.1016/j.neuroimage.2008.10.055
Pubmed Abstract | Pubmed Full Text | CrossRef Full Text | Google Scholar
66. Beckmann CF, Jenkinson M, Smith SM. General multilevel linear modeling for group analysis in FMRI. Neuroimage (2003) 20(2):1052–63. doi:10.1016/S1053-8119(03)00435-X
Pubmed Abstract | Pubmed Full Text | CrossRef Full Text | Google Scholar
67. Friston KJ, Buechel C, Fink GR, Morris J, Rolls E, Dolan RJ. Psychophysiological and modulatory interactions in neuroimaging. Neuroimage (1997) 6(3):218–29. doi:10.1006/nimg.1997.0291
Pubmed Abstract | Pubmed Full Text | CrossRef Full Text | Google Scholar
68. Woo CW, Krishnan A, Wager TD. Cluster-extent based thresholding in fMRI analyses: pitfalls and recommendations. Neuroimage (2014) 91:412–9. doi:10.1016/j.neuroimage.2013.12.058
Pubmed Abstract | Pubmed Full Text | CrossRef Full Text | Google Scholar
69. Nudo RJ. Recovery after brain injury: mechanisms and principles. Front Hum Neurosci (2013) 7:887. doi:10.3389/fnhum.2013.00887
Pubmed Abstract | Pubmed Full Text | CrossRef Full Text | Google Scholar
70. Wessa M, Houenou J, Paillere-Martinot ML, Berthoz S, Artiges E, Leboyer M, et al. Fronto-striatal overactivation in euthymic bipolar patients during an emotional go/nogo task. Am J Psychiatry (2007) 164(4):638–46. doi:10.1176/appi.ajp.164.4.638
Pubmed Abstract | Pubmed Full Text | CrossRef Full Text | Google Scholar
71. Agid Y, Buzsaki G, Diamond DM, Frackowiak R, Giedd J, Girault JA, et al. How can drug discovery for psychiatric disorders be improved? Nat Rev Drug Discov (2007) 6(3):189–201. doi:10.1038/nrd2217
Pubmed Abstract | Pubmed Full Text | CrossRef Full Text | Google Scholar
72. Schloesser RJ, Martinowich K, Manji HK. Mood-stabilizing drugs: mechanisms of action. Trends Neurosci (2012) 35(1):36–46. doi:10.1016/j.tins.2011.11.009
Pubmed Abstract | Pubmed Full Text | CrossRef Full Text | Google Scholar
73. Logothetis NK, Pfeuffer J. On the nature of the BOLD fMRI contrast mechanism. Magn Reson Imaging (2004) 22(10):1517–31. doi:10.1016/j.mri.2004.10.018
Pubmed Abstract | Pubmed Full Text | CrossRef Full Text | Google Scholar
74. Hu Y, Chen X, Gu H, Yang Y. Resting-state glutamate and GABA concentrations predict task-induced deactivation in the default mode network. J Neurosci (2013) 33(47):18566–73. doi:10.1523/JNEUROSCI.1973-13.2013
Pubmed Abstract | Pubmed Full Text | CrossRef Full Text | Google Scholar
75. Enzi B, Duncan NW, Kaufmann J, Tempelmann C, Wiebking C, Northoff G. Glutamate modulates resting state activity in the perigenual anterior cingulate cortex – a combined fMRI-MRS study. Neuroscience (2012) 227:102–9. doi:10.1016/j.neuroscience.2012.09.039
Pubmed Abstract | Pubmed Full Text | CrossRef Full Text | Google Scholar
76. Greicius MD, Flores BH, Menon V, Glover GH, Solvason HB, Kenna H, et al. Resting-state functional connectivity in major depression: abnormally increased contributions from subgenual cingulate cortex and thalamus. Biol Psychiatry (2007) 62(5):429–37. doi:10.1016/j.biopsych.2006.09.020
Pubmed Abstract | Pubmed Full Text | CrossRef Full Text | Google Scholar
77. Grimm S, Boesiger P, Beck J, Schuepbach D, Bermpohl F, Walter M, et al. Altered negative BOLD responses in the default-mode network during emotion processing in depressed subjects. Neuropsychopharmacology (2009) 34(4):932–43. doi:10.1038/npp.2008.81
78. Kuhn S, Gallinat J. Resting-state brain activity in schizophrenia and major depression: a quantitative meta-analysis. Schizophr Bull (2013) 39(2):358–65. doi:10.1093/schbul/sbr151
Pubmed Abstract | Pubmed Full Text | CrossRef Full Text | Google Scholar
79. Horn DI, Yu C, Steiner J, Buchmann J, Kaufmann J, Osoba A, et al. Glutamatergic and resting-state functional connectivity correlates of severity in major depression – the role of pregenual anterior cingulate cortex and anterior insula. Front Syst Neurosci (2010) 4:33. doi:10.3389/fnsys.2010.00033
Pubmed Abstract | Pubmed Full Text | CrossRef Full Text | Google Scholar
80. Phillips ML, Swartz HA. A critical appraisal of neuroimaging studies of bipolar disorder: toward a new conceptualization of underlying neural circuitry and a road map for future research. Am J Psychiatry (2014) 171(8):829–43. doi:10.1176/appi.ajp.2014.13081008
Pubmed Abstract | Pubmed Full Text | CrossRef Full Text | Google Scholar
81. Poldrack RA. Region of interest analysis for fMRI. Soc Cogn Affect Neurosci (2007) 2(1):67–70. doi:10.1093/scan/nsm006
82. Logothetis NK, Pauls J, Augath M, Trinath T, Oeltermann A. Neurophysiological investigation of the basis of the fMRI signal. Nature (2001) 412(6843):150–7. doi:10.1038/35084005
83. Allman JM, Hakeem A, Erwin JM, Nimchinsky E, Hof P. The anterior cingulate cortex. The evolution of an interface between emotion and cognition. Ann N Y Acad Sci (2001) 935:107–17. doi:10.1111/j.1749-6632.2001.tb03476.x
Pubmed Abstract | Pubmed Full Text | CrossRef Full Text | Google Scholar
Keywords: major depression, bipolar disorder, psychomotor disturbances, cingulate cortex, cingulate motor area, striatum, putamen
Citation: Liberg B, Klauser P, Harding IH, Adler M, Rahm C, Lundberg J, Masterman T, Wachtler C, Jonsson T, Kristoffersen-Wiberg M, Pantelis C and Wahlund B (2014) Functional and structural alterations in the cingulate motor area relate to decreased fronto-striatal coupling in major depressive disorder with psychomotor disturbances. Front. Psychiatry 5:176. doi: 10.3389/fpsyt.2014.00176
Received: 08 October 2014; Accepted: 21 November 2014;
Published online: 04 December 2014.
Edited by:
Sebastian Walther, University of Bern, SwitzerlandReviewed by:
Andrea Federspiel, University of Bern, SwitzerlandDidier Schrijvers, University of Antwerp, Belgium
Copyright: © 2014 Liberg, Klauser, Harding, Adler, Rahm, Lundberg, Masterman, Wachtler, Jonsson, Kristoffersen-Wiberg, Pantelis and Wahlund. This is an open-access article distributed under the terms of the Creative Commons Attribution License (CC BY). The use, distribution or reproduction in other forums is permitted, provided the original author(s) or licensor are credited and that the original publication in this journal is cited, in accordance with accepted academic practice. No use, distribution or reproduction is permitted which does not comply with these terms.
*Correspondence: Benny Liberg, Department of Psychiatry, Melbourne Neuropsychiatry Centre, Alan Gilbert Building, Level 3, 161 Barry Street, Carlton South, VIC 3053, Australia e-mail:YmVubnkubGliZXJnQGdtYWlsLmNvbQ==