- 1Department of Psychiatry, University of New Mexico, Albuquerque, NM, United States
- 2Department of Behavioral Sciences, University of New Mexico, Albuquerque, NM, United States
- 3Department of Neurosciences, University of New Mexico, Albuquerque, NM, United States
- 4Mind Research Network, Albuquerque, NM, United States
- 5Department of Neurosurgery, University of New Mexico, Albuquerque, NM, United States
- 6Department of Mathematics and Statistics, University of New Mexico, Albuquerque, NM, United States
- 7The New Mexico VA Health Care System, Albuquerque, NM, United States
- 8Department of Electrical Engineering, University of New Mexico, Albuquerque, NM, United States
- 9Department of Psychology, Georgia State University, Atlanta, GA, United States
Background: The proton magnetic resonance spectroscopy (1H-MRS) signals from glutamate (or the combined glutamate and glutamine signal—Glx) have been found to be greater in various brain regions in people with schizophrenia. Recently, the Psychiatric Genetics Consortium reported that several common single-nucleotide polymorphisms (SNPs) in glutamate-related genes confer increased risk of schizophrenia. Here, we examined the relationship between presence of these risk polymorphisms and brain Glx levels in schizophrenia.
Methods: 1H-MRS imaging data from an axial, supraventricular tissue slab were acquired in 56 schizophrenia patients and 67 healthy subjects. Glx was measured in gray matter (GM) and white matter (WM) regions. The genetic data included six polymorphisms genotyped across an Illumina 5M SNP array. Only three of six glutamate as well as calcium-related SNPs were available for examination. These included three glutamate-related polymorphisms (rs10520163 in CLCN3, rs12704290 in GRM3, and rs12325245 in SLC38A7), and three calcium signaling polymorphisms (rs1339227 in RIMS1, rs7893279 in CACNB2, and rs2007044 in CACNA1C). Summary risk scores for the three glutamate and the three calcium polymorphisms were calculated.
Results: Glx levels in GM positively correlated with glutamate-related genetic risk score but only in younger (≤36 years) schizophrenia patients (p = 0.01). Glx levels did not correlate with calcium risk scores. Glx was higher in the schizophrenia group compared to levels in controls in GM and WM regardless of age (p < 0.001).
Conclusion: Elevations in brain Glx are in part, related to common allelic variants of glutamate-related genes known to increase the risk for schizophrenia. Since the glutamate risk scores did not differ between groups, some other genetic or environmental factors likely interact with the variability in glutamate-related risk SNPs to contribute to an increase in brain Glx early in the illness.
Introduction
Higher brain glutamate, glutamine, or glutamate plus glutamine (Glx) measured with proton magnetic resonance spectroscopy (1H-MRS) have been reported in schizophrenia, more consistently in subcortical regions (1). Though striatal elevations decrease with antipsychotic treatment (2), higher levels are reported in subjects at-risk for psychosis, as well as in never-medicated and chronic schizophrenia groups (1). This suggests that the elevations persist in medicated patients, although perhaps to a less severe extent and may be a trait variable (1). Consistent with this, in the largest sample to date (schizophrenia = 104, healthy control = 97), we reported higher Glx in medial frontal and parietal cortical regions, in clinically stable medicated schizophrenia patients (3). The N-methyl-d-aspartate receptor hypofunction model is a potential mechanism to account for increased glutamate metabolites in schizophrenia. It postulates dysfunction of these receptors in gamma-amino-butyric acid interneurons. Presumably, this results in disinhibition of pyramidal neurons and a paradoxical increase in presynaptic glutamate release across multiple cortical and subcortical fields. Though several genes have been proposed (4), the mechanisms accounting for increased glutamate in schizophrenia remain unknown.
Two studies have examined specific relationships of putative schizophrenia-related genes and brain glutamate levels in patients with the illness, with negative findings (5, 6). Recently, the Psychiatric Genetics Consortium [PGC (7)], the most comprehensive genome-wide association study to date, reported that 6 of 108 loci found to confer risk for schizophrenia involve genes clearly implicated in brain glutamate function (several other identified loci are likely to affect glutamate metabolism and synaptic function indirectly). However, there have been no investigations examining the impact of polymorphisms in these genes on brain glutamate concentrations in people with schizophrenia.
In the present study, we examined the relationships between three of the six glutamate-related risk-conferring single-nucleotide polymorphisms (SNPs) identified by the PGC and brain glutamate levels in schizophrenia and healthy control subjects (the other three SNPs could not be measured with the platform used). To evaluate the specificity of the relationship, we also examined correlations with calcium signaling SNPs also found to confer risk for schizophrenia by the PGC. A subgroup of subjects from our recent 1H-MRS study (3) for whom a saliva sample for genomics was collected was included. Because these common SNPs alleles clearly confer risk for the illness, are in genes directly involved in brain glutamate function (7), and Glx is abnormally increased in schizophrenia (1, 3), we hypothesized that schizophrenia subjects with a higher score of risk-conferring glutamate-related SNPs would have higher Glx brain levels. Because we previously reported increased Glx in both gray matter (GM) and white matter (WM) of medial frontal and parietal regions in schizophrenia subjects (3), we examined both tissue types without specific predictions.
Materials and Methods
Subjects
Patients with schizophrenia were recruited from the University of New Mexico Hospitals and the Albuquerque Veterans Affairs Medical Center. Inclusion criteria were (1) DSM IV TR diagnosis of schizophrenia made through consensus by two research psychiatrists using the information from a structured interview (SCID DSM IV TR, Patient Version), medical records, and family informants and (2) clinically stable on the same antipsychotic medications for at least 4 weeks. Exclusion criteria were (1) diagnosis of neurological disorder, (2) current substance use disorder (except for nicotine), (3) metallic implants, (4) claustrophobia, and (5) other than Caucasian ancestry. Healthy controls were additionally excluded for any of the following: (1) any current DSM IV TR axis I disorder [determined with SCID DSM IV TR Non-Patient Version; (except current nicotine) or any past history of a disorder (except for substance use)] and (2) a first degree relative with a psychotic disorder. The local internal review board approved the study. Subjects provided written informed consent and were paid for their participation.
Clinical Assessments
Patients were assessed for psychopathology with the Positive and Negative Syndrome Scale (8), the Simpson Angus Scale [SAS (9)] for parkinsonism, the Barnes Akathisia Rating Scale (10), and the Abnormal Involuntary Movement Scale (11). Assessments were completed within 1 week of scanning.
Magnetic Resonance Studies
Acquisition
Scanning was performed on a 3-T scanner (VB-17; 12 channel head-coil). Subjects were told to try to remain awake during the acquisition but no task was implemented. T1-weighted images were collected with 3D-MPRAGE for voxel tissue segmentation (TR/TE/TI 1,500/3.87/700 ms, flip angle 10°, field-of-view = 256 mm × 256 mm, 1-mm thick slice). 1H-MRS imaging was performed with a phase-encoded version of a point-resolved spectroscopy sequence, to allow the simultaneous acquisition of multiple voxels as described previously (12). Acquisitions were obtained both with and without water presaturation. The following parameters were used: TE = 40 ms, TR = 1,500 ms, slice thickness = 15 mm, FOV = 220 mm × 220 mm, circular k-space sampling (radius = 12), Cartesian k-space size = 32 × 32 after zero filling, k-space Hamming filter with 50% width and number of averages = 1, total scan time = 582 s. The nominal voxel size was 0.71 cm3 but the effective voxel volume is estimated to be 2.4 cm3, taking into account the k-space sampling and filtering. The 1H-MRSI volume of interest was prescribed from an axial T2-weighted image to lie immediately above the lateral ventricles and parallel to the anterior–posterior commissure axis, and included portions of the cingulate gyrus and the medial frontal and parietal lobes (Figure 1A). To minimize the chemical shift artifact, the transmitter was set to the frequency of the NAA methyl-peak during the acquisition of the metabolite spectra and to the frequency of the water-peak during the acquisition of the unsuppressed water spectra.
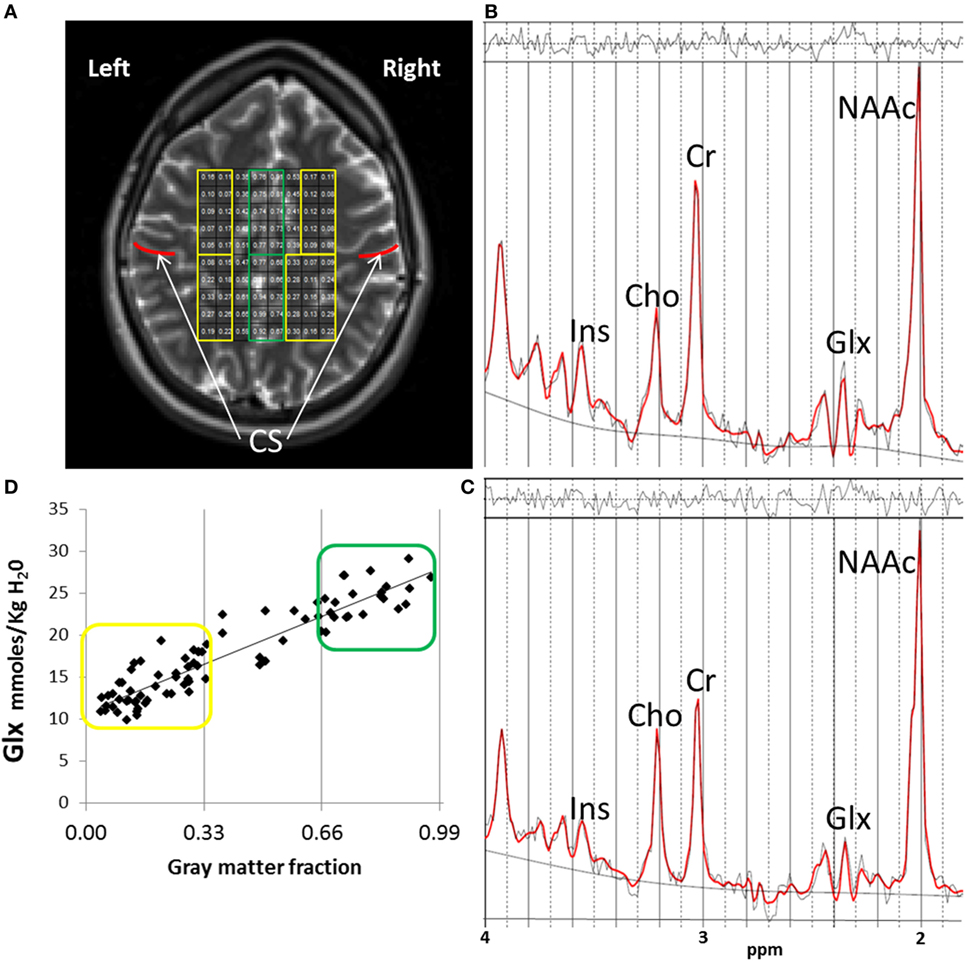
Figure 1. 1H-MRSI methods. (A) 1H-MRSI axial supraventricular slab placement with highlighted predominantly white matter (WM) (in yellow) and gray matter (GM) (in green) voxels. Although a field-of-view of 32 × 32 is acquired, the volume of interest analyzed in all subjects and presented here, is a rectangular subset that fits within the oval of the brain, minus the most outer rows and columns to reduce chemical shift voxel displacement artifact. Regions anterior to the central sulcus (CS, in red) are frontal. Regions posterior to the CS are parietal. (B) Example of one fitted spectrum (red line) from a predominantly GM voxel. Peak areas for glutamate + glutamine (Glx), N-acetyl-aspartate compounds (NAAc), total-creatine (Cr = Phosphocreatine + Creatine), myo-inositol (Ins), and total-choline (Cho = glycerophosphocholine + phosphocholine) are labeled. Top irregular line represents the residual signal after fitting. Lower continuous line represents the baseline used for fitting with LC Model. (C) Example of one fitted spectrum from a predominantly WM voxel. (D) Distribution of Glx values corresponding to the individual voxel’s GM fraction (GM/GM + WM) for the 1H-MRSI axial supraventricular slab from (A). In yellow are predominantly WM and in green predominantly GM Glx values.
Spectral Fitting
Data were automatically preprocessed and fitted using LC Model [Version 6.1 (13)] with a simulated basis-set for the sequence parameters which included the following metabolites: alanine, aspartate, creatine, phosphocreatine, gamma-amino-butyric acid, glutamine, glutamate, glycerophosphocholine, phosphocholine, myo-inositol, lactate, N-acetyl-aspartate, N-acetyl-aspartylglutamate, scyllo-inositol, and guanidine. Lipids and macromolecule contributions were fitted using the default simulated intensities of LC Model, which included soft constraints for peak position and line width and prior probabilities of the ratios of macromolecule and lipid peaks. Spectra were fitted in the range between 1.8 and 4.2 ppm and scaled to the non-suppressed water intensity (12). The SD of the fit of the Glx signals, provided by LC Model (related to the Cramer–Rao lower bounds and a measure of the confidence of the fit) was used to exclude data with low confidence. Only data with an LC Model SD ≤ 20% were further analyzed (13) (Figures 1B,C).
Partial-Volume–Relaxation Correction
The Glx results from LC Model were corrected as described previously and are reported in units of molality (moles/kg tissue water) (14). Briefly, the Glx signals were corrected for partial-volume and relaxation effects using GM, WM, and cerebrospinal fluid (CSF) maps from segmented T1-weighted images with SPM5. Water densities and relaxation times in each tissue or CSF compartment were obtained from the literature, for this correction (14). Our group has previously documented the test–retest reliability of these methods (12). As previously discussed (3), voxels were further classified based on their GM fraction as [100 × GM/(GM + WM) as “predominantly” GM (>66%) or “predominantly” WM < 34%; Figure 1D]. Finally, because glutamate and glutamine concentrations vary depending on tissue type and most voxels contained various proportions of GM and WM (15), we also used the GM fraction of each voxel as a covariate in the statistical analyzes (see below).
Genetics
SNP Selection and Analyzes
Our aim was to study the six SNPs identified in the PGC (7) involved in glutamatergic function and the six SNPs involved in calcium signaling (Table 1). The genetic data originated from each subject’s saliva samples processed via 5M Illumina HumanOmni5-Quad SNP array (Illumina, www.illumina.com) and we used Illumina Genome Studio Genotyping Module to optimize call rates. Genome wide scan data from each participant underwent quality assurance testing before inclusion in the analysis. Requirements per SNP included a minor allele frequency greater than 5%, Hardy–Weinberg equilibrium (p < 10−6), and data for over 90% of participants in the sample. Three individuals missing over 10% of the total SNPs found in the platform were excluded. Only three of the glutamate-related and three of the calcium signaling SNPs were available for 125 subjects.
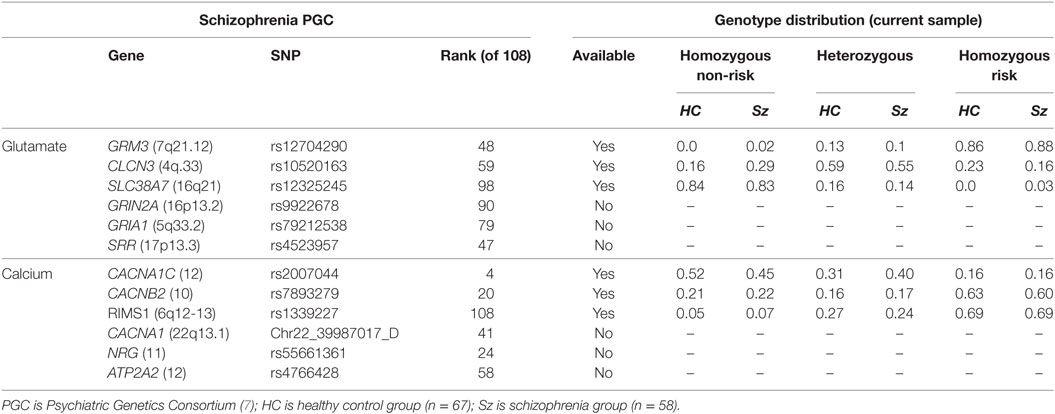
Table 1. Genotype distribution of the available glutamate-related and calcium signaling risk single-nucleotide polymorphisms (SNPs) from the PGC study in schizophrenia and healthy control subjects.
Imputation
Any missing genotypes at the six loci of interest were selectively imputed via IMPUTE2 software (v2.3.1) and the 1000 Genomes Phase 3 reference dataset. SNPs rs12704290, rs12325245, and rs1339227 were imputed for all subjects. SNP rs2007044 was imputed for 1 subject. Imputed SNPs demonstrated a 0.9 or greater imputation probability estimate.
Adjustment
For each subject, the number of risk alleles (1 for homozygous non-risk, 2 for heterozygous, and 3 for homozygous risk) was multiplied by the odds ratio (OD) for schizophrenia from the PGC (12) at each SNP. In order to examine the potential effect of ethnicity, we used PLINK to calculate 10 multidimensional scaling (MDS) factors (equivalent to principal components) from the subjects’ SNP array data. The top principal components are generally associated with population structure, in other words ethnicity information. The top MDS factors were entered into the relevant PROC-MIXED model.
Summary Scores
Each SNP OD-adjusted risk value was summed to a total risk score (range 3–9) for glutamate-related SNPs and for calcium signaling SNPs. The direction of effect on Glx brain levels of a risk allele is unknown. However, as proof of concept, the total risk score approach supports the testing of the hypothesis that a higher risk score would positively correlate with the more abnormal (higher) Glx levels in schizophrenia [reported in the literature (1, 3)].
Statistical Analysis
We examined whether the relationships between brain Glx and glutamate-related and calcium signaling genetic risk scores differed across schizophrenia and healthy controls. Because Glx brain levels differ by tissue type and age (15), and there are progressive tissue changes in schizophrenia (16), these variables (age and voxel tissue composition) must be considered in the analyzes. PROC-MIXED (SAS version-9) uses all available data, accounts for correlation between repeated measurements in the same subject (i.e., Glx in many voxels), and can handle missing data more appropriately than other methods (17). Hence, we implemented four repeated-measures PROC-MIXED analyzes: glutamate-related risk score and Glx in GM (1) and in WM (2); as well as calcium signaling risk score and Glx in GM (3) and in WM (4) (p = 0.05/4, Bonferroni-corrected p = 0.0125). Each of these omnibus tests included Glx concentration in all selected voxels as the repeated-measures dependent variable, with the following independent variables: risk score as the within-subject factor, diagnostic-group (schizophrenia, healthy control) as the between-group factor and age as a covariate. In order to facilitate the visualization (by plotting data) of the hypothesized correlations between Glx concentrations (continuous) and risk scores (continuous), age was dichotomized into age-group for the model; we chose a median split of ≤36 years as a neutral cutoff (results did not differ with age dichotomization of <30 or >45).
As in our previous 1H-MRS imaging study (3), we followed a hierarchical, systematic approach to statistical analyzes. To address type-1 errors, only the highest order significant interactions involving diagnostic-group and risk score (the relevant variables of interest) are presented in the Results and followed-up with PROC-MIXED post hoc tests; this protects the post hoc tests for type-I errors (18, 19). In order to control for effects of diagnostic-group differences in spectral fitting [e.g., bias in metabolite values due to worse quality in the ill group as previously reported (3)], we used the Cramer–Rao lower bounds (CRLB = SD/concentration × 100). The CRLB (not the SD reported by LC Model) should be used to account for group differences in spectral quality, since CRLB is independent of concentration (personal communication, Provencher, creator of LC Model). Hence, if the groups differed in CRLBs, GM fraction, relevant demographic or substance use characteristics, these were entered into the model as additional covariates. The potential confound of antipsychotic medication was examined by adding the drug dosage as standardized olanzapine equivalents (20) to the appropriate model in the schizophrenia group. Likewise, the effects of various symptom severity and neurological side-effects measures were examined in each relevant model. All tests were two-tailed and we used Satterthwaite’s correction for unequal variances.
Results
Demographics, Substance Use History, and Quality of Spectral Fitting
Fifty-eight schizophrenia and 67 healthy controls were studied. There were no significant differences between the groups in: age, gender, Hispanic ethnicity, socioeconomic status (SES) of the subject, or SES of the family of origin, smoking status, vascular risk factors, or history of opiate or sedative use disorders (Table 2). Schizophrenia subjects had more frequent lifetime histories of alcohol (p = 0.006), cannabis (p < 0.001), cocaine (p = 0.02), hallucinogens (p = 0.004), and stimulant use disorders (p = 0.05). Also, the schizophrenia group had slightly, but significantly, higher CRLB for Glx (GlxCRLB) in GM (F1,123 = 53.3, p < 0.001) and WM (F1,123 = 36.9, p < 0.001). However, schizophrenia and controls did not differ in GM fractions for GM (p = 0.11) or for WM (p = 0.15). As expected, CSF proportion across voxels was higher in the schizophrenia than the control group (p < 0.001). This difference was addressed by the partial-volume correction method (14).
Glx and Glutamate-Related Risk Scores
Gray Matter
Glx was positively correlated with glutamate risk score but only in the younger schizophrenia group (diagnostic-group × age-group × risk-score: F1,117 = 6.8, p = 0.01; Figure 2). This three-way interaction remained after adjusting for GlxCRLB (actually became more robust, F1,117 = 10.5, p = 0.001), as well as after controlling for histories of alcohol, cannabis, cocaine, stimulant, and hallucinogen use (p’s between 0.002 and 0.003). Also the three-way interaction remained after adding the top 3, 4, or 5 MDS factors to address potential effects of ethnicity (p’s between 0.02 and 0.008). Post hoc PROC-MIXED confirmed that among the younger age-group, the relationships between Glx and risk-score differed between the schizophrenia and control groups (F1,59 = 9.9, p = 0.003). However, among the older age-group, these correlations did not differ between schizophrenia and control groups (F1,58 = 0.55, p = 0.46). Still, when examining the whole schizophrenia group, the associations between Glx and risk score differed between the younger and the older age-groups (F1,54 = 5.9, p = 0.02). Finally, in a test randomizing the dependent variable (Glx) 1,000 times, the observed (6.8) or higher F values occurred in 2 of the 1,000 permutations (p = 0.002). This provides further support that the above findings are unlikely to be the result of chance variations.
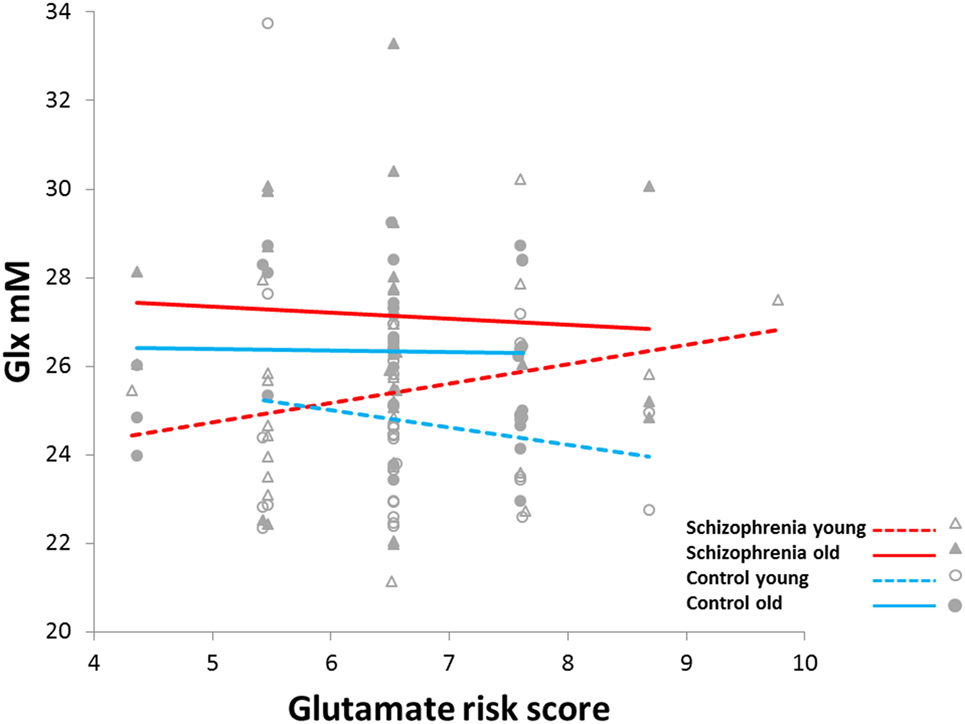
Figure 2. Increased slope of glutamine plus glutamate (Glx) concentration versus glutamate-related risk score in younger schizophrenia (<36 years) compared to younger controls, older schizophrenia (≥36 years), and older controls (F1,117 = 6.8, p = 0.01).
The differences in relationships between Glx and genetic score among the younger and older schizophrenia age-groups remained when accounting for age of onset of psychosis (p = 0.04), positive (p = 0.04) and depressive symptoms (p = 0.03), antipsychotic dose (p = 0.01) and severity of tardive dyskinesia (p = 0.007), parkinsonism (p = 0.02), and akathisia (p = 0.02). However, the differences became a trend when adjusting for negative symptoms score (F1,52 = 3.5, p = 0.07). Finally, severity of negative symptoms did not differ between younger (14.9 ± 4.3) and older (14.2 ± 3.8) schizophrenia patients (p = 0.5).
White Matter
A significant diagnostic-group × age-group × risk-score interaction (F1,117 = 7.7, p = 0.007), disappeared when adjusting for GlxCRLB (F1,117 = 0.2, p = 0.65). There were no other significant interactions or main effects involving diagnostic-group; see Supplementary Material for full statistical model.
Glx and Calcium Signaling Risk Scores
Gray Matter
The only significant effect involving diagnosis was the diagnostic-group × risk-score interaction (F1,119 = 5.0, p = 0.03). However, significance disappeared when adjusting for GlxCRLB (F1,119 = 0.05, p = 0.82).
White Matter
A significant diagnostic-group × age-group × risk-score interaction (F1,117 = 8.4, p = 0.005), disappeared when adjusting for GlxCRLB (F1,117 = 0.2, p = 0.63).
Group Differences for Genetic Risk Scores and Glx Levels
Not surprisingly in this small sample, genetic risk-scores did not differ between the schizophrenia and the healthy controls for glutamate (t123 = 1.02, p = 0.31) or for calcium-related (t123 = 0.02, p = 0.98) SNPs. Glx was higher in the schizophrenia compared to the control group, adjusting for age or tissue type (F1,122 = 26.5, p < 0.001); however, this is not a new finding but merely a statement that the subgroup examined in this report behaves similar to the full sample from the original study (3) in terms of Glx levels.
Discussion
We found that among younger subjects with schizophrenia, scores in glutamate-related risk-conferring SNPs positively correlated with Glx levels in GM. In older schizophrenia patients, as in the healthy controls regardless of age, there was no such relationship. This pattern of relationships was not found for risk-scores in neuronal calcium signaling SNPs and was not accounted by variance in antipsychotic dose or other common confounds, such as prior substance use histories or the quality of spectral fitting. Finally, Glx levels were higher in the schizophrenia group but the genetic risks scores did not differ from the healthy controls.
Only two other studies have examined the relationship between a glutamate-related gene and in vivo brain glutamate in schizophrenia. Ongur et al. (5) reported that a haplotype of four SNPs within the glutaminase 1 (GLS1) gene was positively associated with the glutamine/glutamate ratio (Gln/Glu) in the parieto-occipital cortex. The sample included a combination of subjects with schizophrenia, bipolar disorder, and healthy controls but there was no difference in the haplotype score versus Gln/Glu correlation across the groups. Gruber et al. (6) found that methionine homozygous carriers for the val66met SNP (rs6265) of the brain-derived neurotrophic factor (BDNF) gene had lower hippocampal glutamate in a combined group of schizophrenia, bipolar disorder, and healthy controls. Again, there were no differences in the associations across groups. However, the PGC did not rank any SNPs in GLS1 or BDNF into the top 108 schizophrenia risk loci (7).
What do these findings tell us about the pathophysiology of schizophrenia? The positive correlation between glutamate-related genes and GM Glx in the younger schizophrenia group is somewhat specific (i.e., not seen with the calcium signaling genes). GRM3 codes for a glutamate receptor predominantly expressed in astrocytes (21); CLCN3 is a voltage-gated chloride channel critical for glutamate reuptake in synaptic vesicles of neurons (22); and SLC38A7 encodes a sodium-coupled l-glutamine transporter expressed in neurons (23). In addition, 1H-MRS visible Glx includes metabolic and neurotransmitter glutamate pools, as well as glutamine, and most glutamine is the product of synaptic glutamate re-uptaked by glial cells (24). Hence, variability in specific common SNPs in these genes, known to confer risk for schizophrenia, can be plausibly related to abnormally increased levels of Glx in GM, though elucidation of the specific mechanisms will require additional experimental approaches. However, the normal range in glutamate risk-scores found in our schizophrenia sample is not sufficient to account for increases in Glx. Hence, some other factors, genetic and/or environmental, must interact with the glutamate genetic risk to increase glutamatergic cortical levels during the early course of the illness. Likewise, this relationship is not apparent in older schizophrenia subjects, with similar glutamate risks scores, also suggests that other factors affect Glx concentration in schizophrenia. Epigenetic factors, like differential methylation of glutamate risk genes during the course of illness, could potentially affect Glx brain levels. In support of this possibility, our recent MRI/genetic/epigenetic preliminary data showed that variation in methylation of PGC gene loci is more robustly related to GM concentration reductions in schizophrenia than the variability in the risk-conferring SNPs themselves (25). Alternatively, other non-specific factors like aging or disease duration may increase Glx levels and obscure a relationship with risk scores in the older subjects.
This study had several strengths, including the assessment of many GM and WM voxels with standardized metrics of quality of spectral fits. As in our recent report (3), controlling for group differences in CRLB can have major effects on the results. However, some limitations must be acknowledged. First, the sample size is small, and replication is necessary. We are not currently aware of a similar 1H-MRS imaging database of supraventricular Glx in schizophrenia with broad SNP characterization for a replication. However, ours is the first proof-of-principle study documenting an association between risk-conferring glutamate-related SNPs and Glx brain levels in schizophrenia. With greater standardization of 1H-MRS protocols, future larger studies would be able to clearly document the extent of specific genetic contributions to glutamatergic dysfunction. Second, glutamate was not resolved from glutamine in this study, and Glx levels do not reflect the rate of glutamatergic metabolism, which would be a more functionally relevant measure. Glutamate and glutamine are present in all brain cell types, so 1H-MRS measurements combine several functional compartments. Hence, interpretation of Glx brain differences is not straight forward. 13C-MRS, though technically demanding and yet to be widely applied in large human samples, could in future studies assess more directly glutamate and glutamine metabolic cycling and their relationship to glutamate-related risk genes in schizophrenia. Third, Glx measurements were acquired without controlling for cognitive state, which can affect glutamate levels (26). Fourth, schizophrenia subjects were treated with antipsychotic medications, agents known to affect brain glutamate levels (2). However, adjustment for antipsychotic dosage within the schizophrenia group, did not cancel the difference in correlations between risk-score and Glx concentrations across the younger and older age-groups. Fourth, only three of the six glutamate and three of the six calcium-related SNPs from PGC were examined due to limited coverage of the Illumina SNP array used. Hence, a complete assessment of the six risk-conferring SNPs could yield different results. Furthermore, it is possible that other risk genes involved in a metabolic pathway that feeds into glutamatergic neurotransmission may also be related to Glx brain concentrations. Fifth, the cortical regions studied were not found to have increased Glx in a recent meta-analysis (1). However, the meta-analysis was published before our recent study, which has by far the largest sample [N = 201 (3)]; still the supplemental data of the meta-analysis showed a small effect size (0.12) for Glx greater in Sz than controls in medial frontal cortex, consistent with our results. Sixth, our criteria for excluding subjects with missing SNP data (>10% of the total SNPs) may be somewhat liberal. Finally, the schizophrenia group had a greater past history of several substance use disorders that could affect Glx levels (27). However, controlling for this history did not eliminate the main findings.
In summary we report in younger schizophrenia patients, a positive relationship between GM Glx levels, with a combined score for glutamate-related SNPs found to confer risk for the illness. This relationship is somewhat specific, as it is not present in WM (which also had increased Glx in schizophrenia), or for calcium signaling SNPs (which also confer risk for schizophrenia). The overall findings suggest that though variance in some common SNPs may indeed contribute to the increased cortical glutamate levels in schizophrenia, other genetic and/or environmental mechanisms must also be involved early in the disease. Future studies very early in the illness with greater Glx brain coverage and examining epigenetic factors that modulate the impact of specific risk-conferring SNPs may shed further light on the underlying neurobiology of glutamatergic dysfunction in schizophrenia. Also, modulators of presynaptic glutamate release may be particularly effective for patient subgroups early in the illness and which have dysregulation of central nervous system glutamatergic tone (28).
Ethics Statement
This study was carried out in accordance with the recommendations of UNM-HSC Human Research Review Committee with written informed consent from all subjects. All subjects gave written informed consent in accordance with the Declaration of Helsinki. The protocol was approved by the Human Research Review Committee.
Author Contributions
JB: design, data collection and analyses, and writing of the manuscript. VP, NP, TJ, RJ, CQ, NP-B, JL, JC, JT, VC, and CG: data analyses and writing of the manuscript.
Conflict of Interest Statement
JB received honoraria for advisory board consulting from Otsuka America Pharmaceutical Inc. in 2013. VP, TJ, RJ, NP, CQ, JC, JL, NP-B, VC, JT, and CG reported no biomedical financial interests or potential conflicts of interest.
Acknowledgments
The authors are grateful to Nicholas Lemke and Patrick Gallegos, employees of the UNM Department of Psychiatry and Behavioral Sciences and to Diana South and Cathy Smith, MRN employees, for their contributions with data collection.
Funding
This study was supported by NIMH R01MH084898 to JB, NIMH 2R01MH065304 and VACSR&D IIR-04-212-3 to JC and 1P20RR021938/P20GM103472 and 1R01EB006841 to VC and DHHS/NIH/NCRR 3 UL1 RR031977-02S2.
Supplementary Material
The Supplementary Material for this article can be found online at http://journal.frontiersin.org/article/10.3389/fpsyt.2017.00079/full#supplementary-material.
References
1. Merritt K, Egerton A, Kempton MJ, Taylor MJ, McGuire PK. Nature of glutamate alterations in schizophrenia: a meta-analysis of proton magnetic resonance spectroscopy studies. JAMA Psychiatry (2016) 73(7):665–74. doi: 10.1001/jamapsychiatry.2016.0442
2. de la Fuente-Sandoval C, Leon-Ortiz P, Azcarraga M, Stephano S, Favila R, Diaz-Galvis L, et al. Glutamate levels in the associative striatum before and after 4 weeks of antipsychotic treatment in first-episode psychosis: a longitudinal proton magnetic resonance spectroscopy study. JAMA Psychiatry (2013) 70(10):1057–66. doi:10.1001/jamapsychiatry.2013.289
3. Bustillo JR, Jones T, Chen H, Lemke N, Abbott C, Qualls C, et al. Glutamatergic and neuronal dysfunction in gray and white matter: a spectroscopic imaging study in a large schizophrenia sample. Schizophr Bull (2016) 43(3):611–9. doi:10.1093/schbul/sbw122
4. Harrison PJ, Weinberger DR. Schizophrenia genes, gene expression, and neuropathology: on the matter of their convergence. Mol Psychiatry (2005) 10(1):40–68; image 5. doi:10.1038/sj.mp.4001558
5. Ongur D, Haddad S, Prescot AP, Jensen JE, Siburian R, Cohen BM, et al. Relationship between genetic variation in the glutaminase gene GLS1 and brain glutamine/glutamate ratio measured in vivo. Biol Psychiatry (2011) 70(2):169–74. doi:10.1016/j.biopsych.2011.01.033
6. Gruber O, Hasan A, Scherk H, Wobrock T, Schneider-Axmann T, Ekawardhani S, et al. Association of the brain-derived neurotrophic factor val66met polymorphism with magnetic resonance spectroscopic markers in the human hippocampus: in vivo evidence for effects on the glutamate system. Eur Arch Psychiatry Clin Neurosci (2012) 262(1):23–31. doi:10.1007/s00406-011-0214-6
7. Schizophrenia Working Group of the Psychiatric Genomics Consortium. Biological insights from 108 schizophrenia-associated genetic loci. Nature (2014) 511(7510):421–7. doi:10.1038/nature13595
8. Kay SR, Fiszbein A, Opler LA. The Positive and Negative Syndrome Scale (PANSS) for schizophrenia. Schizophr Bull (1987) 13(2):261–76. doi:10.1093/schbul/13.2.261
9. Simpson GM, Angus JW. A rating scale for extrapyramidal side effects. Acta Psychiatr Scand Suppl (1970) 212:11–9. doi:10.1111/j.1600-0447.1970.tb02066.x
10. Barnes TR. A rating scale for drug-induced akathisia. Br J Psychiatry (1989) 154:672–6. doi:10.1192/bjp.154.5.672
11. Schooler NR, Kane JM. Research diagnoses for tardive dyskinesia. Arch Gen Psychiatry (1982) 39(4):486–7. doi:10.1001/archpsyc.1982.04290040080014
12. Gasparovic C, Bedrick EJ, Mayer AR, Yeo RA, Chen H, Damaraju E, et al. Test-retest reliability and reproducibility of short-echo-time spectroscopic imaging of human brain at 3T. Magn Reson Med (2011) 66(2):324–32. doi:10.1002/mrm.22858
13. Provencher SW. Automatic quantitation of localized in vivo 1H spectra with LCModel. NMR Biomed (2001) 14(4):260–4. doi:10.1002/nbm.698
14. Gasparovic C, Song T, Devier D, Bockholt HJ, Caprihan A, Mullins PG, et al. Use of tissue water as a concentration reference for proton spectroscopic imaging. Magn Reson Med (2006) 55(6):1219–26. doi:10.1002/mrm.20901
15. Maudsley AA, Domenig C, Govind V, Darkazanli A, Studholme C, Arheart K, et al. Mapping of brain metabolite distributions by volumetric proton MR spectroscopic imaging (MRSI). Magn Reson Med (2009) 61(3):548–59. doi:10.1002/mrm.21875
16. Olabi B, Ellison-Wright I, McIntosh AM, Wood SJ, Bullmore E, Lawrie SM. Are there progressive brain changes in schizophrenia? A meta-analysis of structural magnetic resonance imaging studies. Biol Psychiatry (2011) 70(1):88–96. doi:10.1016/j.biopsych.2011.01.032
17. Gueorguieva R, Krystal JH. Move over ANOVA: progress in analyzing repeated-measures data and its reflection in papers published in the archives of general psychiatry. Arch Gen Psychiatry (2004) 61(3):310–7. doi:10.1001/archpsyc.61.3.310
18. Day B. Statistical Methods in Cancer Research Volume I – The Analysis of Case-Control Studies. Lyon: IARC Publications (1980). 196 p.
20. Gardner DM, Murphy AL, O’Donnell H, Centorrino F, Baldessarini RJ. International consensus study of antipsychotic dosing. Am J Psychiatry (2010) 167(6):686–93. doi:10.1176/appi.ajp.2009.09060802
21. Sun W, McConnell E, Pare JF, Xu Q, Chen M, Peng W, et al. Glutamate-dependent neuroglial calcium signaling differs between young and adult brain. Science (2013) 339(6116):197–200. doi:10.1126/science.1226740
22. Stobrawa SM, Breiderhoff T, Takamori S, Engel D, Schweizer M, Zdebik AA, et al. Disruption of ClC-3, a chloride channel expressed on synaptic vesicles, leads to a loss of the hippocampus. Neuron (2001) 29(1):185–96. doi:10.1016/S0896-6273(01)00189-1
23. Hagglund MG, Sreedharan S, Nilsson VC, Shaik JH, Almkvist IM, Backlin S, et al. Identification of SLC38A7 (SNAT7) protein as a glutamine transporter expressed in neurons. J Biol Chem (2011) 286(23):20500–11. doi:10.1074/jbc.M110.162404
24. Rae CD. A guide to the metabolic pathways and function of metabolites observed in human brain 1H magnetic resonance spectra. Neurochem Res (2014) 39(1):1–36. doi:10.1007/s11064-013-1199-5
25. Lin DLJ, Chen J, Sui J, Du Y, Calhoun VD. Exploration of genetic and epigenetic effects on brain gray matter density in schizophrenia. 12th International Imaging Genetics Conference. Irvine (2016).
26. Apsvalka D, Gadie A, Clemence M, Mullins PG. Event-related dynamics of glutamate and BOLD effects measured using functional magnetic resonance spectroscopy (fMRS) at 3T in a repetition suppression paradigm. Neuroimage (2015) 118:292–300. doi:10.1016/j.neuroimage.2015.06.015
27. Prisciandaro JJ, Schacht JP, Prescot AP, Renshaw PF, Brown TR, Anton RF. Associations between recent heavy drinking and dorsal anterior cingulate N-acetylaspartate and glutamate concentrations in non-treatment-seeking individuals with alcohol dependence. Alcohol Clin Exp Res (2016) 40(3):491–6. doi:10.1111/acer.12977
Keywords: glutamate, genetics, single-nucleotide polymorphisms, spectroscopy, schizophrenia
Citation: Bustillo JR, Patel V, Jones T, Jung R, Payaknait N, Qualls C, Canive JM, Liu J, Perrone-Bizzozero NI, Calhoun VD, Turner JA and Gasparovic C (2017) Risk-Conferring Glutamatergic Genes and Brain Glutamate Plus Glutamine in Schizophrenia. Front. Psychiatry 8:79. doi: 10.3389/fpsyt.2017.00079
Received: 30 January 2017; Accepted: 24 April 2017;
Published: 12 June 2017
Edited by:
Anouk Marsman, Copenhagen University Hospital Hvidovre, DenmarkReviewed by:
Gabriele Ende, Central Institute of Mental Health, GermanyPhilip R. Corlett, Yale University, United States
Copyright: © 2017 Bustillo, Patel, Jones, Jung, Payaknait, Qualls, Canive, Liu, Perrone-Bizzozero, Calhoun, Turner and Gasparovic. This is an open-access article distributed under the terms of the Creative Commons Attribution License (CC BY). The use, distribution or reproduction in other forums is permitted, provided the original author(s) or licensor are credited and that the original publication in this journal is cited, in accordance with accepted academic practice. No use, distribution or reproduction is permitted which does not comply with these terms.
*Correspondence: Juan R. Bustillo, amJ1c3RpbGxvQHNhbHVkLnVubS5lZHU=