- 1Department of Electrical & Computer Engineering, University of Washington, Seattle, WA, United States
- 2Department of Biostatistics, University of Washington, Seattle, WA, United States
- 3Graduate Institute of Biomedical Sciences, China Medical University, Taichung, Taiwan
- 4Department of Psychiatry, Kaohsiung Chang Gung Memorial Hospital, Chang Gung University College of Medicine, Kaohsiung, Taiwan
- 5School of Medicine, Chang Gung University, Taoyuan, Taiwan
- 6Department of Medicine Research, China Medical University Hospital, Taichung, Taiwan
- 7Department of Psychiatry, China Medical University Hospital, Taichung, Taiwan
- 8Brain Disease Research Center, China Medical University Hospital, Taichung, Taiwan
- 9Department of Psychology, College of Medical and Health Sciences, Asia University, Taichung, Taiwan
The D-amino acid oxidase activator (DAOA, also known as G72) gene is a strong schizophrenia susceptibility gene. Higher G72 protein levels have been implicated in patients with schizophrenia. The current study aimed to differentiate patients with schizophrenia from healthy individuals using G72 single nucleotide polymorphisms (SNPs) and G72 protein levels by leveraging computational artificial intelligence and machine learning tools. A total of 149 subjects with 89 patients with schizophrenia and 60 healthy controls were recruited. Two G72 genotypes (including rs1421292 and rs2391191) and G72 protein levels were measured with the peripheral blood. We utilized three machine learning algorithms (including logistic regression, naive Bayes, and C4.5 decision tree) to build the optimal predictive model for distinguishing schizophrenia patients from healthy controls. The naive Bayes model using two factors, including G72 rs1421292 and G72 protein, appeared to be the best model for disease susceptibility (sensitivity = 0.7969, specificity = 0.9372, area under the receiver operating characteristic curve (AUC) = 0.9356). However, a model integrating G72 rs1421292 only slightly increased the discriminative power than a model with G72 protein alone (sensitivity = 0.7941, specificity = 0.9503, AUC = 0.9324). Among the three models with G72 protein alone, the naive Bayes with G72 protein alone had the best specificity (0.9503), while logistic regression with G72 protein alone was the most sensitive (0.8765). The findings remained similar after adjusting for age and gender. This study suggests that G72 protein alone, without incorporating the two G72 SNPs, may have been suitable enough to identify schizophrenia patients. We also recommend applying both naive Bayes and logistic regression models for the best specificity and sensitivity, respectively. Larger-scale studies are warranted to confirm the findings.
Introduction
Schizophrenia is a severe mental disorder characterized by symptoms such as delusions, hallucinations, blunted affect, impaired executive function, reduced motivation, and disorganized communication (1). The prevalence of schizophrenia is around 1% worldwide, and the social and economic costs of schizophrenia are enormous (2, 3). Converging evidence from genome-wide linkage studies, genetic case-control association studies, and genome-wide association studies indicate that several potential candidate genes are associated with schizophrenia (4). More and more genetic studies have employed novel computational tools such as naive Bayes to conduct gene discovery and detect new gene loci associated with schizophrenia (5). Identification of susceptibility genes for schizophrenia will help in early detection and prevention of high-risk individuals, as well as in developing novel therapies (6). The D-amino-acid oxidase activator (DAOA, also named G72) gene is one of the candidate genes.
The G72 gene, located on chromosome 13q3, exists in exclusively four primate species (7). Furthermore, the G72 gene encodes the protein that has been shown to function as a putative activator of D-amino acid oxidase (DAO), located in peroxisomes (7) and a mitochondrial protein (8). In vitro studies also demonstrate that the G72 protein binds to and activates DAO, which is capable of oxidizing D-amino acids such as D-serine, an agonist of the N-methyl-D-aspartate receptor (NMDAR) (7, 9). The agonist activity at NMDAR may have particular relevance to a novel drug target for treatment of schizophrenia (10–16). One hypothesis of schizophrenia is that individuals who overproduce the G72 protein have lower D-amino acid levels and reduced NMDAR activity, predisposing them to schizophrenia (17, 18). A study suggests that the plasma G72 protein levels may be distinctively higher in patients with schizophrenia than healthy individuals (18). Of note, G72 protein levels are very similar between the medicated patients and the drug-free patients, implying that antipsychotic treatment does not influence G72 levels in plasma (18). In addition, G72 transgenic mice studies indicate a role of G72 in modulating behaviors relevant to schizophrenia (19–21). The G72 gene was also reported to predispose to schizophrenia in French Canadian (7), Russian (7), Chinese (22–24), German (25), and Ashkenazi (26) populations in single nucleotide polymorphism (SNP)-based studies.
A pilot study (18) modeled disease susceptibility to schizophrenia with plasma G72 protein levels using logistic regression. The current larger-sized study compared three artificial intelligence and machine learning techniques (including logistic regression, naive Bayes, and C4.5 decision tree) in predicting schizophrenia using G72 protein levels plus G72 SNPs. These three artificial intelligence and machine learning algorithms were chosen because they are well-known techniques with distinctively representational models; regression models for logistic regression (27), probabilistic models for naive Bayes (28), and decision tree models for the C4.5 algorithm (29).
Materials and Methods
Study Population
This study was approved by the institutional review board of China Medical University Hospital, Taiwan, and carried out in accordance with the Declaration of Helsinki. Consecutive patients were screened and recruited from the psychiatric treatment programs of China Medical University Hospital, which is a major medical center in Taiwan. The patient population is similar to that of other mental health facilities. After complete description of the study to the subjects, written informed consents were obtained in line with the institutional review board guidelines. The study subjects were partially original to a previous study (18); the same 60 healthy individuals, but with more schizophrenia patients.
In the cohort, both patients and controls were Han Chinese aged 18–50 years, who were physically and neurologically healthy and had normal laboratory assessments (including urine/blood routine and biochemical tests). Both patients and controls were evaluated by the research psychiatrists using the Structured Clinical Interview for DSM-IV (SCID) for diagnosis. All patients had a DSM-IV diagnosis of schizophrenia. Patients with Axis I diagnosis other than schizophrenia, or any Axis II diagnosis were not included. All healthy volunteers were free of any Axis I or II psychiatric disorder. To exclude potential confounding effects, all participants were non-smokers and had no DSM-IV diagnosis of substance (including alcohol) abuse or dependence.
Drug history was ascertained by interviewing the patients and family members or caregivers, contacting other health care providers, and reviewing chart. Healthy controls had no history of exposure to psychotropic agents. Among schizophrenia patients, some patients were psychotropic-free for 3 months or longer and the other patients were stabilized on antipsychotics (risperidone, zotepine, haloperidol, quetiapine, amisulpride, sulpiride, flupentixol, olanzapine, ziprasidone, chlorpromazine, or paliperidone) for at least 3 months (18). The G72 protein level was not correlated with the medications administrated by patients (18).
Laboratory Assessments: Genotyping
DNA was isolated from blood samples using MasterPure DNA purification kit following the manufacturer's instructions (EPICENTRE, Madison, Wisconsin, USA). To extract DNA, we used 200 μl of blood which was further solved in 100 μl of distilled water (30). The extracted DNA was diluted to the concentration of 50 ng/μl determined by the absorbance at 260 nM (ND-1000 UV-Vis spectrophotometer, Thermo Fisher Scientific Inc.). Four standard DNA samples with known genotypes were used for quality control (31).
All SNP genotyping was performed using the Taqman SNP genotyping assay (ABI: Applied Biosystems Inc., Foster City, CA, USA). The primers and probes of SNPs were provided by the ABI Company. The PCR reaction was conducted in 15 μl reaction volume which contained 0.4 μl DNA sample (50 ng), 7.5 μl Master mix (Roche), and 0.4 μl 40x primer pairs and probes. The samples were pre-incubated at 95°C for 10 min to activate the Hot-Start DNA polymerase and to denature DNA, following by 40 amplification cycles of 92°C denaturation for 15 s and 60°C for 60 s. The probe fluorescence signal detection was performed using the ABI Prism 7500 Real-Time PCR System.
Laboratory Assessments: Western Blotting
The plasma G72 protein expression levels were examined by western blotting (18). Ten milliliter of blood was collected into EDTA-containing blood collection tubes by personnel trained in phlebotomy using sterile technique. The blood specimens were processed immediately by centrifugation at 500 g. After centrifugation, plasma was quickly dissected and immediately stored at −80°C until western blotting.
For western blotting, 100 μl plasma was depleted using ProteoPrep® Blue Albumin and IgG Depletion Kit (Sigma). The low-abundant protein fractions were collected to 100 μl. Then, 10 μl of the fractions were mixed with 4X sample buffer (500 mM Tris-HCl (pH 6.8), 16% SDS, 80% glycerol, 400 mM DTT, and 0.08% bromophenol blue) and separated on 12% SDS-PAGE. Proteins in the gels were transferred to 0.45 μm polyvinylidene difluoride (PVDF) membrane (Millipore). The membranes were placed in 5% nonfat dry milk in TBST (20 mM Tris-HCl pH 7.6, 500 mM sodium chloride, 0.1% Tween 20) for 1 h at room temperature, then incubated with goat anti-G72 antibody (G72(N15):sc-46118, Santa Cruz Biotechnology) diluted by 1:1,000 in TBST overnight at 4°C. The membranes were washed for 3 times in TBST and incubated for 2 h with a HRP-linked anti-goat IgG secondary antibody (sc-2030, Santa Cruz Biotechnology) diluted by 1:5,000 in TBST. After 3 washes in TBST, the blots were visualized with an ECL Advance Western Blotting Detection Kit (RPN2135, GE Healthcare). The stained membranes were photographed on ImageQuant LAS 4000 mini (GE Healthcare) and quantified using ImageQuant™ TL 7.0 software (GE Healthcare) by measuring the relative intensity from each band and normalized to the G72 recombinant protein (20 ng) signals. All western blot analyses were repeated for two times.
Machine Learning Algorithms
Machine learning algorithm is a procedure for choosing the best hypothesis from a set of alternatives that fit a set of observations (27, 32). The advantages of machine learning algorithms, including nonlinearity, fault tolerance, and real-time operation, make them suitable for complex applications (33). The current study employed three families of machine learning algorithms, including logistic regression, naive Bayes, and C4.5 decision tree. Logistic regression analysis, the standard method for clinical classification (27), was used as a basis for comparison. The analyses were performed using the Waikato Environment for Knowledge Analysis (WEKA) software (27).
The naive Bayes classifier assumes that the presence or absence of a particular feature is unrelated to the presence or absence of any other feature (27). It calculates the probability that a given instance belongs to a certain class (“schizophrenia“ or “control“ in this study) by using Bayes' theorem.
The C4.5 decision tree is a model which builds decision trees top-down and prunes them using the concept of information entropy (27). The tree is first constructed by finding the root node (SNP or protein level) that is most discriminative for differentiating a disease status from “control.” The best single feature test is decided by the information gain from choosing a feature (SNP or protein level) to split the data into subsets. Here, we used the default parameters of WEKA, such as 0.25 for the confidence factor and 2 for the minimum number of instances per leaf node (34).
Evaluation of the Predictive Performance
The repeated 10-fold cross-validation method was used to investigate the generalization of the predictive models produced by the aforementioned algorithms (34–36). To measure the performance of the predictive models, we used the receiver operating characteristic (ROC) method and calculated the area under curve (AUC) to compare the performances of different predictive models (34, 35). AUC is a better performance metric than accuracy; the higher AUC means the better performance (36).
Statistical Analysis
We analyzed the categorical data using the chi-square test. Differences for continuous variables were compared using the Student's t-test (37). Genotype frequencies were evaluated for Hardy-Weinberg equilibrium using a χ2 goodness-of-fit test. The criterion for significance was set at P < 0.05 for all tests. Data are presented as mean ± standard deviation (SD).
Results
Findings From the Unmatched Sample
The participants were 60 unrelated healthy individuals and 89 schizophrenia patients. As shown in Table 1, there was no significant difference in gender distribution between the two groups. The mean age (37.8 ± 10.5) of schizophrenia patients was older than that of healthy controls (32.8 ± 9.9, P = 0.004). The mean level of G72 protein in the plasma of schizophrenia patients was markedly higher than that of healthy controls (4.057 ± 2.594 ng/μL vs. 1.147 ± 0.574 ng/μL, respectively, P < 0.0001) (Table 1). The genotype frequencies for both rs1421292 and rs2391191 of the G72 gene were in Hardy–Weinberg equilibrium (P = 0.39 and 0.27, respectively).
AUC After Adding rs1421292 Was Only Slightly Better Than That From G72 Protein Alone
Table 2 summarizes the results from the naive Bayes algorithm. We generated five models (Models 1–5) with various combinations of three factors (rs2391191, rs1421292, and G72 protein levels). Among the five models, Model 2 with rs1421292 and G72 protein levels had the best AUC. Its AUC, sensitivity, and specificity were 0.9356, 0.7969, and 0.9372, respectively (Table 2). However, the AUC value after adding rs1421292 was only slightly better than that of G72 protein alone by 0.32% (Model 2 vs. Model 5).
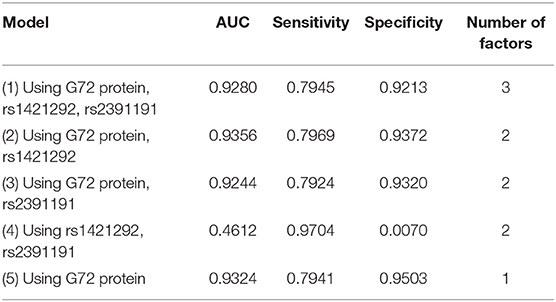
Table 2. Five naive Bayes models for differentiating schizophrenia patients from unmatched healthy individuals.
We then employed the C4.5 decision tree algorithm with the same three factors (Table 3). Among the five models, Model 7 with rs1421292 and G72 protein levels had the best AUC. Its AUC, sensitivity, and specificity were 0.8525, 0.8202, and 0.8843, respectively (Table 3). The AUC value after adding rs1421292 was only slightly better than that of G72 protein alone by 0.19% (Model 7 vs. Model 10).
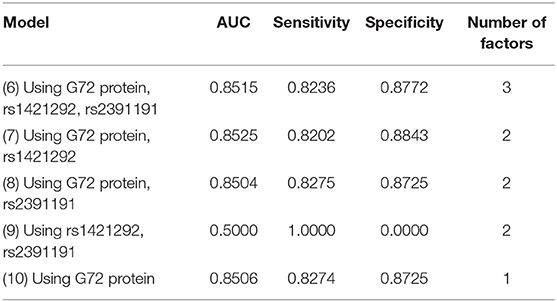
Table 3. Five C4.5 decision tree models for differentiating schizophrenia patients from unmatched healthy individuals.
We finally tested the same factors with logistic regression (Table 4). The AUC, sensitivity, and specificity for the best logistic regression model (Model 12, applying rs1421292, and G72 protein levels) were 0.9272, 0.8576, and 0.8923, respectively. The AUC value only slightly increased by 0.97% after adding rs1421292 (Model 12 vs. Model 15).
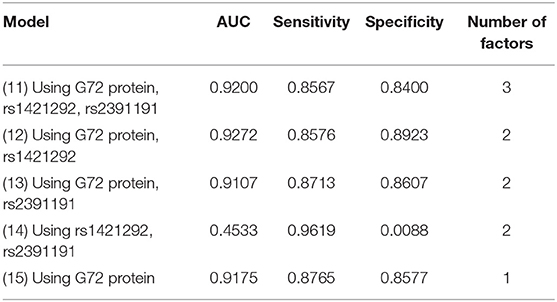
Table 4. Five logistic regression models for differentiating schizophrenia patients from unmatched healthy individuals.
Of the G72 Protein Models, Naive Bayes Was Specific; And Logistic Regression, Sensitive
Among all the 15 models (Models 1–15) with unmatched schizophrenia patients and healthy controls, the naive Bayes (Model 2) with rs1421292 and G72 protein levels had the highest AUC. Of the three models with G72 protein alone (Models 5, 10, and 15), the naive Bayes (Model 5) had the best specificity (0.9503) and logistic regression (Model 15) had the best sensitivity (0.8765).
We further tested the relationship between G72 genotypes and G72 protein levels. The distribution of the two SNPs [for example, the numbers of TT (n = 57), TA (n = 65), and AA (n = 27) carriers in rs1421292] was illustrated in Table 5. As shown in Table 5, the G72 protein levels were marginally higher in the subjects with the TT or TA genotype than the AA homozygotes for rs1421292 (3.051 ± 2.588 vs. 2.137 ± 1.819; P = 0.084). There was no association between genetic variances of rs2391191 and G72 protein levels.
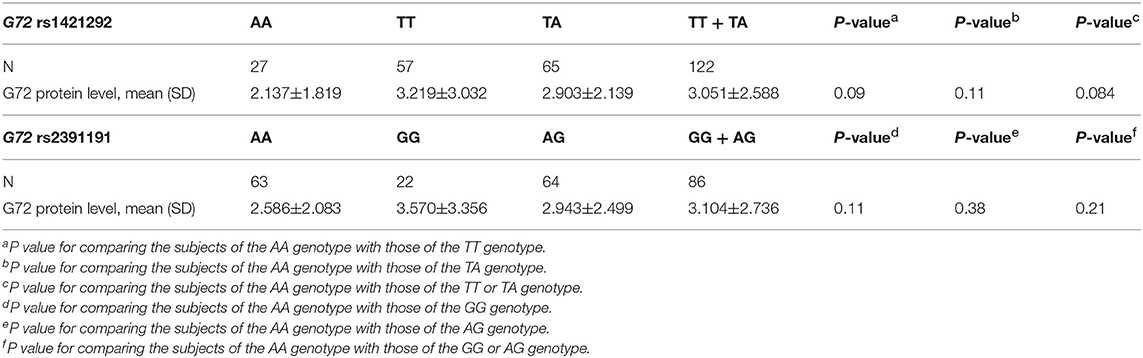
Table 5. Relationship between G72 genotypes and G72 protein level with schizophrenia patients and unmatched healthy individuals.
Findings From the Matched Sample
Next, we selected 66 patients from the schizophrenia group to match better with healthy controls by age. The demographic characteristics of age and gender-matched schizophrenia patients and healthy controls are shown in Table 6. There was no significant difference in gender and age distributions between the two groups. The G72 levels in the plasma of schizophrenia patients were markedly higher than that of the matched healthy controls (4.188 ± 2.772 ng/μL and 1.147 ± 0.574 ng/μL, respectively, P < 0.0001) (Table 6).
The Findings From the Matched Sample Were Similar to Those From the Unmatched Sample
Table 7 shows the analytic results of schizophrenia patients and matched healthy controls. The findings from the matched sample were similar to those from the unmatched sample. Among the three models (Models 16–18) with G72 protein alone, the naive Bayes model (Model 16) performed best in specificity (0.966), and logistic regression (Model 18) had the best sensitivity (0.8483) (Table 7).
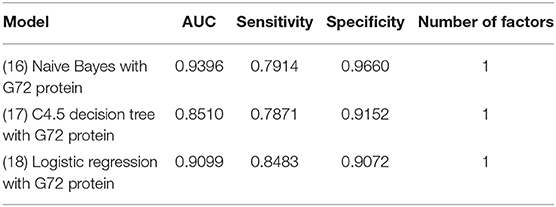
Table 7. The models of naive Bayes, C4.5 decision tree, and Logistic regression for differentiating schizophrenia patients from matched healthy individuals using G72 protein.
Discussion
To our knowledge, this is the first study to examine the relationships between schizophrenia and G72 SNPs plus plasma G72 protein levels. We compared three machine learning algorithms, including logistic regression, naive Bayes, and C4.5 decision tree, in differentiating schizophrenia patients from healthy individuals. The results showed that the naive Bayes with G72 rs1421292 SNP and G72 protein levels (Model 2) performed best among all models (Models 1–15). The combination of G72 rs1421292 SNP and G72 protein levels was also the best model using the C4.5 decision tree (Model 7) and logistic regression (Model 12). These results were consistent with another finding of this study; that is, G72 rs1421292 SNP was marginally associated with G72 protein levels (Table 5). The proposed procedures can be implemented using the publicly available software WEKA (27) and thus can be widely used in genomic studies.
However, the AUC value after adding rs1421292 was only slightly better than that of G72 protein alone by an increase of 0.32% (Model 2 vs. Model 5) and 0.97% (Model 12 vs. Model 15), respectively. Hence, the present study suggests that G72 protein alone may have been feasible enough in AUC. Moreover, among the three models with G72 protein alone, logistic regression performed best in sensitivity, and the naive Bayes model was the most specific. This finding remained similar in the matched sample. We therefore recommend a combination model using logistic regression (for sensitivity) and naive Bayes (for specificity).
The common SNPs, such as rs2391191 and rs1421292, of the G72 gene have received considerable attention. These two SNPs were shown to be associated with schizophrenia (7, 23–25); however, the findings are discordant. The rs2391191 SNP was reported to predispose to schizophrenia in Chinese (23, 24) and German (25) subjects, but not in French Canadian (7), United States (38), Scottish (22), Chinese (22, 39), and Taiwanese (40) populations. On the other hand, the rs1421292 SNP was found to be associated with schizophrenia in French Canadian (7), Russian (7), and German (25) subjects, but not in Japanese sample (41), UK population (42) and a mix of different races (including 84% Caucasian and 9% African American) in the United States (38). Moreover, two recent genome-wide association studies (GWAS) have been conducted to identify susceptible genetic loci affecting schizophrenia in mainly European (43) and Chinese (44) populations, respectively. However, no association of schizophrenia with SNPs in the G72 gene was found in these two large GWAS. Furthermore, by utilizing expression quantitative trait loci (eQTL) analyses, one of these GWAS implicated that there was no genetic risk regulating gene expression of G72 effect in brain or blood when eQTL analyses were used to explain associations with schizophrenia (43). The current study showed that the models which combined rs2391191 and rs1421292 (Models 4, 9, and 14) were not as good as other models (Tables 2–4). Moreover, adding rs2391191 or rs1421292 could not increase the AUC significantly than G72 protein alone. In agreement with several previous studies (7, 22, 38–44), the current study with a small sample size didn't demonstrate an association between the two G72 SNPs and schizophrenia.
In our previous study (18) on G72 protein levels, the severity of disease, the medications administrated by patients, as well as illness duration of the medicated patients did not influence the G72 protein level. In addition, the G72 protein level was significantly associated with schizophrenia in multivariate logistic regression analyses (18). The G72 protein level was also higher in drug-free or medicated schizophrenia patients than in healthy controls (18). The current larger-sized study extended the previous study by combining G72 protein levels with G72 SNPs as well as by leveraging the state-of-the-art artificial intelligence and machine learning algorithms. The current results implicated that the relevance of G72 protein levels to schizophrenia is much more significant than that of SNPs.
This study has several limitations. First, we chose only two SNPs of G72 for the current study because they seem to be two most commonly used SNPs. Whether other SNPs of G72 (25) could contribute more in the models of predicting schizophrenia remains unknown. Second, the findings of the current study came from a single population. More studies are necessary to testify whether the findings could be replicated in non-Taiwanese subjects (45, 46). Third, the small sample size does not allow us to draw definite conclusions (46). In the future, large-scale prospective studies in other ethnicities are warranted to reconfirm the potential of G72 protein level and G72 SNPs as the biomarkers for schizophrenia.
Conclusions
In conclusion, this preliminary study tested and compared numerous models using machine learning algorithms for predicting schizophrenia. The findings suggest that the models with G72 protein alone, without adding G72 SNPs, may have good enough power to discriminate patients with schizophrenia from healthy individuals. We also propose a combination of logistic regression and naive Bayes models to build a both sensitive and specific model to predict schizophrenia. Independent replications with larger-scale studies in other racial populations are needed to confirm the role of the G72 SNPs and G72 protein found in the current study.
Author Contributions
EL, C-HL, and H-YL designed the study. EL analyzed the data. EL, C-HL, and H-YL drafted and revised the manuscript. Y-LL, Y-JH, and C-HH conducted the laboratory experiments. All authors provided the final approval of the version to be published.
Funding
This work was supported by National Health Research Institutes, Taiwan (NHRI-EX107-10731NI), Ministry of Science and Technology in Taiwan (MOST 107-2314-B-039−039), Taiwan Ministry of Health and Welfare Clinical Trial, and Research Center of Excellence (MOHW107-TDU-B-212-123004), China Medical University and Hospital (DMR-103-085; CMU107-BC-4).
Conflict of Interest Statement
The authors declare that the research was conducted in the absence of any commercial or financial relationships that could be construed as a potential conflict of interest.
References
1. Saha S, Chant D, Welham J, McGrath J. A systematic review of the prevalence of schizophrenia. PLoS Med. (2005) 2:e141. doi: 10.1371/journal.pmed.0020141
2. Messias EL, Chen CY, Eaton WW. Epidemiology of schizophrenia: review of findings and myths. Psychiatr Clin North Am. (2007) 30:323–38. doi: 10.1016/j.psc.2007.04.007
3. Patel A, Everitt B, Knapp M, Reeder C, Grant D, Ecker C, et al. Schizophrenia patients with cognitive deficits: factors associated with costs. Schizophr Bull (2006) 32:776–85. doi: 10.1093/schbul/sbl013
4. Sullivan PF. How good were candidate gene guesses in schizophrenia genetics? Biol Psychiatry (2017) 82:696–7. doi: 10.1016/j.biopsych.2017.09.004
5. Andreassen OA, Thompson WK, Dale AM. Boosting the power of schizophrenia genetics by leveraging new statistical tools. Schizophr Bull. (2014) 40:13–7. doi: 10.1093/schbul/sbt168
6. Jarskog LF, Miyamoto S, Lieberman JA. Schizophrenia: new pathological insights and therapies. Annu Rev Med. (2007) 58:49–61. doi: 10.1146/annurev.med.58.060904.084114
7. Chumakov I, Blumenfeld M, Guerassimenko O, Cavarec L, Palicio M, Abderrahim H, et al. Genetic and physiological data implicating the new human gene G72 and the gene for D-amino acid oxidase in schizophrenia. Proc Natl Acad Sci USA. (2002) 99:13675–80. doi: 10.1073/pnas.182412499
8. Kvajo M, Dhilla A, Swor DE, Karayiorgou M, Gogos JA. Evidence implicating the candidate schizophrenia/bipolar disorder susceptibility gene G72 in mitochondrial function. Mol Psychiatry (2008) 13:685–96. doi: 10.1038/sj.mp.4002052
9. Sacchi S, Bernasconi M, Martineau M, Mothet JP, Ruzzene M, Pilone MS, et al. pLG72 modulates intracellular D-serine levels through its interaction with D-amino acid oxidase: effect on schizophrenia susceptibility. J Biol Chem. (2008) 283:22244–56. doi: 10.1074/jbc.M709153200
10. Coyle JT, Tsai G, Goff D. Converging evidence of NMDA receptor hypofunction in the pathophysiology of schizophrenia. Ann N Y Acad Sci. (2003) 1003:318–27. doi: 10.1196/annals.1300.020
11. Ermilov M, Gelfin E, Levin R, Lichtenberg P, Hashimoto K, Javitt DC, et al. A pilot double-blind comparison of d-serine and high-dose olanzapine in treatment-resistant patients with schizophrenia. Schizophr Res. (2013) 150:604–5. doi: 10.1016/j.schres.2013.09.018
12. Goff DC. D-cycloserine: an evolving role in learning and neuroplasticity in schizophrenia. Schizophr Bull (2012) 38:936–41. doi: 10.1093/schbul/sbs012
13. Javitt DC. Twenty-five years of glutamate in schizophrenia: are we there yet? Schizophr Bull. (2012) 38:911–3. doi: 10.1093/schbul/sbs100
14. Moghaddam B, Javitt D. From revolution to evolution: the glutamate hypothesis of schizophrenia and its implication for treatment. Neuropsychopharmacology (2012) 37:4–15. doi: 10.1038/npp.2011.181
15. Lane HY, Lin CH, Green MF, Hellemann G, Huang CC, Chen PW, et al. Add-on treatment of benzoate for schizophrenia: a randomized, double-blind, placebo-controlled trial of D-amino acid oxidase inhibitor. JAMA Psychiatry (2013) 70:1267–75. doi: 10.1001/jamapsychiatry.2013.2159
16. Lin CH, Lin CH, Chang YC, Huang YJ, Chen PW, Yang HT, et al. Sodium benzoate, a D-amino acid oxidase inhibitor, added to clozapine for the treatment of schizophrenia: a randomized, double-blind, placebo-controlled trial. Biol Psychiatry (2017) 84:422–32. doi: 10.1016/j.biopsych.2017.12.006
17. Hashimoto K, Fukushima T, Shimizu E, Komatsu N, Watanabe H, Shinoda N, et al. Decreased serum levels of D-serine in patients with schizophrenia: evidence in support of the N-methyl-D-aspartate receptor hypofunction hypothesis of schizophrenia. Arch Gen Psychiatry (2003) 60:572–6. doi: 10.1001/archpsyc.60.6.572
18. Lin CH, Chang HT, Chen YJ, Lin CH, Huang CH, Tun R, et al. Distinctively higher plasma G72 protein levels in patients with schizophrenia than in healthy individuals. Mol Psychiatry (2014) 19:636–7. doi: 10.1038/mp.2013.80
19. Cheng L, Hattori E, Nakajima A, Woehrle NS, Opal MD, Zhang C, et al. Expression of the G72/G30 gene in transgenic mice induces behavioral changes. Mol Psychiatry (2014) 19:175–83. doi: 10.1038/mp.2012.185
20. Otte DM, Bilkei-Gorzo A, Filiou MD, Turck CW, Yilmaz O, Holst MI, et al. Behavioral changes in G72/G30 transgenic mice. Eur Neuropsychopharmacol. (2009) 19:339–48. doi: 10.1016/j.euroneuro.2008.12.009
21. Otte DM, Sommersberg B, Kudin A, Guerrero C, Albayram O, Filiou MD, et al. N-acetyl cysteine treatment rescues cognitive deficits induced by mitochondrial dysfunction in G72/G30 transgenic mice. Neuropsychopharmacology (2011) 36:2233–43. doi: 10.1038/npp.2011.109
22. Ma J, Qin W, Wang XY, Guo TW, Bian L, Duan SW, et al. Further evidence for the association between G72/G30 genes and schizophrenia in two ethnically distinct populations. Mol Psychiatry (2006) 11:479–87. doi: 10.1038/sj.mp.4001788
23. Wang X, He G, Gu N, Yang J, Tang J, Chen Q, et al. Association of G72/G30 with schizophrenia in the Chinese population. Biochem Biophys Res Commun. (2004) 319:1281–6. doi: 10.1016/j.bbrc.2004.05.119
24. Zou F, Li C, Duan S, Zheng Y, Gu N, Feng G, et al. A family-based study of the association between the G72/G30 genes and schizophrenia in the Chinese population. Schizophr Res. (2005) 73:257–61. doi: 10.1016/j.schres.2004.01.015
25. Schumacher J, Jamra RA, Freudenberg J, Becker T, Ohlraun S, Otte AC, et al. Examination of G72 and D-amino-acid oxidase as genetic risk factors for schizophrenia and bipolar affective disorder. Mol Psychiatry (2004) 9:203–7. doi: 10.1038/sj.mp.4001421
26. Korostishevsky M, Kaganovich M, Cholostoy A, Ashkenazi M, Ratner Y, Dahary D, et al. Is the G72/G30 locus associated with schizophrenia? single nucleotide polymorphisms, haplotypes, and gene expression analysis. Biol Psychiatry (2004) 56:169–76. doi: 10.1016/j.biopsych.2004.04.006
27. Witten IHFE. Data Mining: Practical Machine Learning Tools and Techniques. Francisco, CA: Morgan Kaufmann Publishers (2005).
28. Lee KE, Sha N, Dougherty ER, Vannucci M, Mallick BK. Gene selection: a Bayesian variable selection approach. Bioinformatics (2003) 19:90–7. doi: 10.1093/bioinformatics/19.1.90
29. Hewett R, Kijsanayothin P. Tumor classification ranking from microarray data. BMC Genomics (2008) 9(Suppl 2):S21. doi: 10.1186/1471-2164-9-S2-S21
30. Hsiao TJ, Lin E. The Pro12Ala polymorphism in the peroxisome proliferator-activated receptor gamma (PPARG) gene in relation to obesity and metabolic phenotypes in a Taiwanese population. Endocrine (2015) 48:786–93. doi: 10.1007/s12020-014-0407-7
31. Hsiao TJ, Lin E. The ENPP1 K121Q polymorphism is associated with type 2 diabetes and related metabolic phenotypes in a Taiwanese population. Mol Cell Endocrinol. (2016) 433:20–5. doi: 10.1016/j.mce.2016.05.020
32. Lin E, Kuo PH, Liu YL, Yu YW, Yang AC, Tsai SJ. A deep learning approach for predicting antidepressant response in major depression using clinical and genetic biomarkers. Front Psychiatry (2018) 9:290. doi: 10.3389/fpsyt.2018.00290
33. Lin E, Lane HY. Machine learning and systems genomics approaches for multi-omics data. Biomark Res. (2017) 5:2. doi: 10.1186/s40364-017-0082-y
34. Huang LC, Hsu SY, Lin E. A comparison of classification methods for predicting Chronic Fatigue Syndrome based on genetic data. J Transl Med. (2009) 7:81. doi: 10.1186/1479-5876-7-81
35. Lin E, Hwang Y. A support vector machine approach to assess drug efficacy of interferon-α and ribavirin combination therapy. Mol Diagn Ther. (2008) 12:219–23. doi: 10.1007/BF03256287
36. Linden A. Measuring diagnostic and predictive accuracy in disease management: an introduction to receiver operating characteristic (ROC) analysis. J Eval Clin Pract. (2006) 12:132–9. doi: 10.1111/j.1365-2753.2005.00598.x
37. Lin E, Kuo PH, Liu YL, Yang AC, Tsai SJ. Transforming growth factor-beta signaling pathway-associated genes SMAD2 and TGFBR2 are implicated in metabolic syndrome in a Taiwanese population. Sci Rep. (2017) 7:13589. doi: 10.1038/s41598-017-14025-4
38. Mulle JG, Chowdari KV, Nimgaonkar V, Chakravarti A. No evidence for association to the G72/G30 locus in an independent sample of schizophrenia families. Mol Psychiatry (2005) 10:431–3. doi: 10.1038/sj.mp.4001619
39. Yue W, Kang G, Zhang Y, Qu M, Tang F, Han Y, et al. Association of DAOA polymorphisms with schizophrenia and clinical symptoms or therapeutic effects. Neurosci Lett. (2007) 416:96–100. doi: 10.1016/j.neulet.2007.01.056
40. Liu YL, Fann CS, Liu CM, Chang CC, Wu JY, Hung SI, et al. No association of G72 and D-amino acid oxidase genes with schizophrenia. Schizophr Res. (2006) 87:15–20. doi: 10.1016/j.schres.2006.06.020
41. Ohi K, Hashimoto R, Yasuda Y, Yoshida T, Takahashi H, Iike N, et al. Association study of the G72 gene with schizophrenia in a Japanese population: a multicenter study. Schizophr Res. (2009) 109:80–5. doi: 10.1016/j.schres.2009.01.019
42. Bass NJ, Datta SR, McQuillin A, Puri V, Choudhury K, Thirumalai S, et al. Evidence for the association of the DAOA (G72) gene with schizophrenia and bipolar disorder but not for the association of the DAO gene with schizophrenia. Behav Brain Funct. (2009) 5:28. doi: 10.1186/1744-9081-5-28
43. Schizophrenia Working Group of the Psychiatric Genomics C. Biological insights from 108 schizophrenia-associated genetic loci. Nature (2014) 511:421–7. doi: 10.1038/nature13595
44. Li Z, Chen J, Yu H, He L, Xu Y, Zhang D, et al. Genome-wide association analysis identifies 30 new susceptibility loci for schizophrenia. Nat Genet. (2017) 49:1576–83. doi: 10.1038/ng.3973
45. Lin E, Kuo PH, Liu YL, Yang AC, Kao CF, Tsai SJ. Association and interaction of APOA5, BUD13, CETP, LIPA and health-related behavior with metabolic syndrome in a Taiwanese population. Sci Rep. (2016) 6:36830. doi: 10.1038/srep36830
Keywords: artificial intelligence, D-amino acid oxidase activator, G72, machine learning algorithm, schizophrenia, single nucleotide polymorphism
Citation: Lin E, Lin C-H, Lai Y-L, Huang C-H, Huang Y-J and Lane H-Y (2018) Combination of G72 Genetic Variation and G72 Protein Level to Detect Schizophrenia: Machine Learning Approaches. Front. Psychiatry 9:566. doi: 10.3389/fpsyt.2018.00566
Received: 16 August 2018; Accepted: 18 October 2018;
Published: 06 November 2018.
Edited by:
Chad A. Bousman, University of Calgary, CanadaReviewed by:
Jingyu Liu, Mind Research Network (MRN), United StatesTianmei Si, Peking University Sixth Hospital, China
Copyright © 2018 Lin, Lin, Lai, Huang, Huang and Lane. This is an open-access article distributed under the terms of the Creative Commons Attribution License (CC BY). The use, distribution or reproduction in other forums is permitted, provided the original author(s) and the copyright owner(s) are credited and that the original publication in this journal is cited, in accordance with accepted academic practice. No use, distribution or reproduction is permitted which does not comply with these terms.
*Correspondence: Hsien-Yuan Lane, aHlsYW5lQGdtYWlsLmNvbQ==
†These authors have contributed equally to this work