- 1Department of Psychiatry and Behavioral Sciences, Vanderbilt University Medical Center, Nashville, TN, United States
- 2Department of Physical Medicine and Rehabilitation, Vanderbilt University Medical Center, Nashville, TN, United States
- 3Center for Biomedical Ethics and Society, Vanderbilt University Medical Center, Nashville, TN, United States
- 4Law School, Vanderbilt University, Nashville, TN, United States
- 5Department of Biomedical Informatics, Vanderbilt University Medical Center, Nashville, TN, United States
- 6Department of Medicine, Vanderbilt University Medical Center, Nashville, TN, United States
In the United States, suicide increased by 24% in the past 20 years, and suicide risk identification at point-of-care remains a cornerstone of the effort to curb this epidemic (1). As risk identification is difficult because of symptom under-reporting, timing, or lack of screening, healthcare systems rely increasingly on risk scoring and now artificial intelligence (AI) to assess risk. AI remains the science of solving problems and accomplishing tasks, through automated or computational means, that normally require human intelligence. This science is decades-old and includes traditional predictive statistics and machine learning. Only in the last few years has it been applied rigorously in suicide risk prediction and prevention. Applying AI in this context raises significant ethical concern, particularly in balancing beneficence and respecting personal autonomy. To navigate the ethical issues raised by suicide risk prediction, we provide recommendations in three areas—communication, consent, and controls—for both providers and researchers (2).
In the United States, suicide increased by 24% in the past 20 years, and suicide risk identification at point-of-care remains a cornerstone of the effort to curb this epidemic (1). As risk identification is difficult because of symptom under-reporting, timing, or lack of screening, healthcare systems rely increasingly on risk scoring and now applied artificial intelligence (AI) to assess risk. AI remains the science of solving problems and accomplishing tasks, through automated or computational means, that normally require human intelligence. Applied AI specifically focuses on enabling high-functioning systems to replicate human intelligence for a dedicated purpose, such as risk prediction (3). This science is decades-old and includes traditional predictive statistics and machine learning. Only in the last few years has it been applied rigorously in suicide risk prediction and prevention. These approaches raise significant ethical challenges. AI has the potential to prompt recommendations for suicidal patients including monitoring, evaluation, and intervention. At the most extreme, involuntary hospitalization for those at highest risk brings the goal of preserving life directly in conflict with the immediate liberty interests of the patient. To navigate the ethical issues raised by suicide risk prediction, we provide recommendations in three areas—communication, consent, and controls—for both providers and researchers (2).
Predicting suicide risk remains a key challenge in suicide prevention, with risk misclassification having serious consequences–both “false negatives” who go on to self-harm without being identified and “false positives” who are monitored, screened, or treated unnecessarily, although the frequency and severity of these harms is not well-known.
Until now, suicide screening occurred during interpersonal interaction using clinician judgment. The use of AI has advanced our ability to predict suicide risk across all ages and in diverse populations (4–7). For example, recent models predict suicide attempt risk with excellent accuracy (>90%) and good precision (>80%). Novel applications of predictive models can both reinforce known risk factors that might be missed and identify potentially novel risk factors at the same time. For instance, insomnia has been demonstrated as an important risk factor of suicidality and in preceding the use of violent methods in particular (8–10). Our research in both adults and adolescents identified surrogates for sleep disorder with the use of natural language processing in the context of AI (6, 11). We noted documentation of melatonin use even in the absence of documented sleep disorder as a strong risk factor of suicide attempts. Similar surrogates of psychosis, depression, and more well-known risk factors have been identified in this way.
In addition to reinforcing known research, AI might identify novel risk and protective factors in those at increased risk of suicide. In a large cohort of patients with fibromyalgia, polysomatic symptoms such as frequent clinical encounters for “weakness,” “fatigue,” and “dizziness” correlated strongly with increased suicidality. Further analyses showed that high rates of outpatient engagement were significant protective factors that also might suggest a prevention strategy. All of these patterns were identified through validated AI, and none had been shown prior to our knowledge (12).
Despite the promise of AI in this domain, caveats exist. The high performance metrics above were reported in case-control studies and might not replicate to prospective cohorts. Risk models of rare events like suicide remain prone to low precision, which means many false positives must be screened to identify one true positive (13). Patterns in known and novel risk factors are correlative and not causative without significant and dedicated further study. We do not know how patients and providers will react to this new technology. We do not have broadly-accepted practice standards or guidelines for implementation (14). Institutional policies may not address the particular challenges of using AI in this way. Thus, we risk adopting AI into clinical practice at ethical cost–not just financial cost.
Ethical challenges also pervade suicide research. For example, involuntary commitment threatens the balance between beneficence (preventing suicide) and autonomy (respecting patient choice). Multiple accurate and large-scale prediction models have been recently published in both civilian and military settings (4–7) The emphasis remains on novel prediction, however, and not on the literacy, numeracy, and education necessary to integrate AI technologies (and medical innovation in general) effectively and ethically into practice. Researchers can now integrate predictive technology into studies, which similarly raise ethical concerns (2).
We cannot afford to ignore opportunities to prevent suicide nor tools that might enhance patient safety. Yet we must honor our obligations to do so ethically reflecting time-honored tenets such as respect for persons, beneficence, non-malfeasance, and justice. The primary question remains, how can we incorporate AI into research and care practices for suicide prevention while minimizing impact on individual liberty? Our recommendations follow.
Communication
Public perceptions of data privacy are evolving, with increased reluctance to share personal data. Do patients want AI surveilling their healthcare data? We need public discussion among stakeholders, providers, and patients prior to scaling AI into healthcare systems. In this population, relevant stakeholders include those at high risk of and with histories of suicidality, familial survivors of suicide, their caregivers, their support networks, and those who might direct resources or regulation toward suicide prevention. The benefits of these conversations include: (1) the design of more compassionate and transparent systems; (2) the opportunity to lessen stigma around suicide by encouraging its open discussion in community settings.
Education is crucial in translating AI models into practice. Even health numeracy challenges both providers and patients, so the difficulty of understanding AI and its potential implications is non-trivial. Data scientific curricula specific to engaging smart agents will be required, including culturally-sensitive AI educational materials tailored to all levels of health literacy. A strong precedent exists for specific education of the ethical challenges, unique factors, and potential harms to provider well-being in encountering suicide in practice (14). Furthermore, education for surrogates such as parents in pediatric settings will require additional effort. Patients require active engagement to enhance communication in conversations prompted by “our risk algorithm identified you as at-risk.”
Consent
Healthcare systems are ethically bound to inform both patients and providers (end-users) of AI risk models that these algorithms (1) can be imperfect or wrong; (2) monitor data considered highly sensitive or confidential (e.g., psychiatry notes); (3) might recommend actions that are not immediately apparent; and (4) might prompt intervention without the need to trigger provider action. The need for informed consent in treating suicidal patients has been well-supported throughout the literature and holds true here just as it does throughout healthcare and in other domains impacting individual liberty (14).
Under the most common rubric, imminent risk exists when patients have suicidal intent, plans, and means (14). Providers respond actively to patients' potential for suicide and breach confidentiality if necessary to protect them. Others define imminent risk by foreseeability, or the “reasonable anticipation … of suicide or suicidal behavior in the very near future (p626)” (15). Clinical judgment informed by patient assessment of suicidal potential constitutes a prediction. If AI can produce reliable and valid predictions in this vein, it may be deemed foreseeable risk. Policymakers must balance desirability of action here alongside associated safeguards for privacy and confidentiality.
When foreseeable risk exists, actions promoting patient safety range from safety checks to formal risk assessments. For example, new data from a wearable device may prompt a suicide risk algorithm to alert a provider, the patient, a family member, or the police. These actions all have implications of potential voluntary or involuntary hospitalization and consequences to patient autonomy and privacy.
We recommend the following risk mitigation steps (Table 1): First, when presenting for treatment, during informed consent, patients with decisional capacity should have the right to “opt-out” of AI monitoring even at the expense of increased system complexity to support this right. How and when to best integrate such consent remain open questions. We have an obligation to respect choice and an individual's right to privacy. Second, we need to study and develop consent forms that account for AI's ability to evolve far more quickly than guidelines can be updated. This concern includes improved consent regarding (1) types, breadth, and depth of data collected and (2) potential risks of AI in practice including those outlined here. Moreover, consent may need to expire to prompt reevaluation of AI–analogous to continuing institutional review board review for ongoing studies to ensure benefits continue to exceed harms.
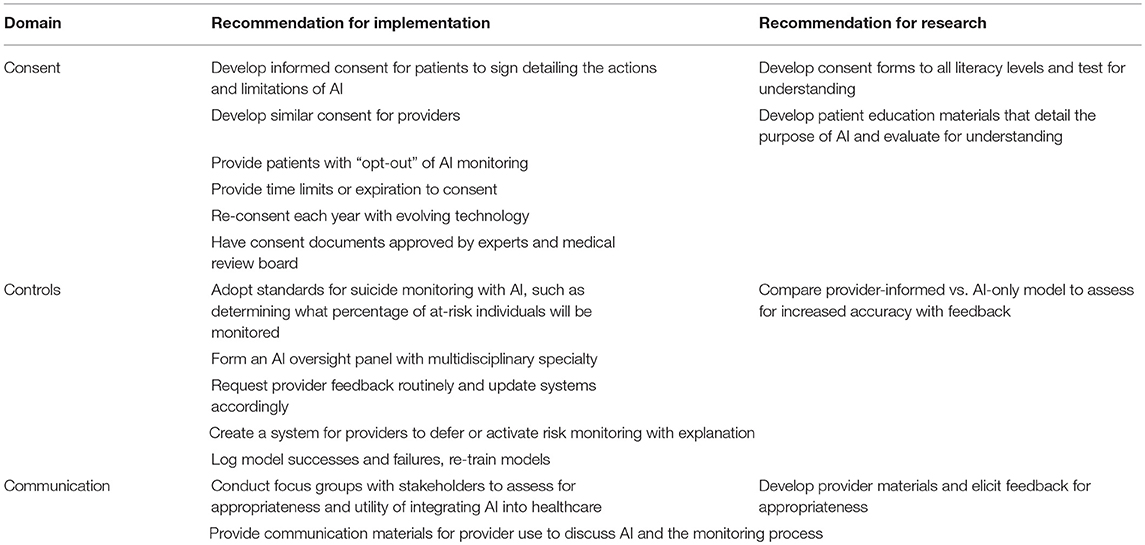
Table 1. Recommendations for risk mitigation applying AI for suicide prevention in healthcare settings.
Controls
Potential benefits of AI-based risk prediction include identifying those at acute risk needing intervention. In large hospital systems, even the highest 1% translates to thousands of individuals monitored. Workflows supporting such alerts do not exist in current practice. Similarly, algorithms can produce aberrant predictions labeling individuals as “high-risk” erroneously due to model mis-specification, data collection glitches, or faults introduced during model or software updates. We have an obligation to evaluate AI tools prospectively throughout their use–not simply for pilot or study trial periods. Further, we must learn from providers before, during, and after implementation of AI tools along with analyzing model successes and failures. To achieve the “learning health system,” implementations need to support feedback loops for providers to correct misclassification from AI and vice-versa (16). To test whether AI yields valid and reliable predictions of suicide risk, prospective evaluation of such AI, e.g., in a pragmatic trial, might directly compare AI predictions with clinician best judgment. Moreover, assessing the additive value of combining both AI predictions and clinical judgment might have important implications for suicide prevention. These opportunities will improve model accuracy and implementation.
As computational models become more sophisticated, they become less easy to interrogate, which has implications for both Communication and Controls. For the latter, this lack of interpretability compounds the potential harms of imperfect models (17). Not only can models miscalculate, this may occur in unexpected or difficult to parse ways. Erroneous predictions threaten provider trust in AI, and could ultimately compromise AI uptake. And the factors leading to errors might be hard to repair. Periodic evaluation of models prospectively will prevent drift in both performance and interpretability.
A Note on Applied vs. General Artificial Intelligence
The vast majority of AI integration in healthcare today exemplifies Applied Artificial Intelligence, which is currently being explored in healthcare systems and the focus of our discussion. Much of the attention in the media in fact focuses on the concept of Artificial General Intelligence (AGI)–a concept closer to truly autonomous systems and agents (18). It is conceivable that autonomous AGI might one day be applied in mental health and suicide risk prediction and similarly that this system might consider and draw its own conclusions about mental health surveillance and intervention. Ethical considerations in light of AGI will require a continual and iterative reassessment of principles that might govern such systems. One might imagine such a system participating in the ethical discussion. We reiterate this possibility remains theoretical and likely far off in the timeline of healthcare information technology but feel it deserves mention given the rapid advancement in machine learning that has already changed the biomedical literature.
In healthcare settings, AI is already being implemented at scale to prevent suicide (19, 20). With appropriate communication, consent, and controls, ethical application of AI can succeed as it has in preventing gun violence in Chicago (21). To approach Zero Suicide, novel methods like AI are needed to identify those at risk who would otherwise be missed. Implementation of such technology demands an ethical framework to ensure benefits outweigh risk and to prioritize the welfare of the patient. The potential partnerships between smart humans and smart agents may shine light on the patients at most risk, wherever they are, rather than hoping those patients walk into the light of a routine or emergent healthcare encounter. In suicide, this last hope often comes too late.
Author Contributions
All authors on the manuscript (LM, EC, and CW) were involved in the conceptualization, writing, and revising of this manuscript.
Funding
This manuscript was prepared with support from the Vanderbilt Patient Centered Outcomes Research Education and Training Initiative and the Agency for Healthcare Research and Quality (AHRQ 6K12HS022990-05).
Conflict of Interest Statement
The authors declare that the research was conducted in the absence of any commercial or financial relationships that could be construed as a potential conflict of interest.
References
1. Curtin SC, Warner M, Hedegaard H. Increase in suicide in the United States, 1999–2014. NCHS Data Brief (2016) 1:8. Available online at: https://www.cdc.gov/nchs/products/databriefs/db241.htm
2. Tucker RP, Tackett MJ, Glickman D, Reger MA. Ethical and practical considerations in the use of a predictive model to trigger suicide prevention interventions in healthcare settings. Suicide Life Threat Behav. (2018). 10.1111/sltb.12431. [Epub ahead of print].
3. Young R. Applied vs. Generalized Artificial Intelligence-What's the Difference? (2018). Avaliable online at: https://www.itchronicles.com/technology/applied-generalized-artificial-intelligence (Accessed October 24, 2018).
4. Barak-Corren Y, Castro VM, Javitt S, Hoffnagle AG, Dai Y, Perlis RH, et al. Predicting suicidal behavior from longitudinal electronic health records. Am J Psychiatry (2017) 174:154–62. doi: 10.1176/appi.ajp.2016.16010077
5. Kessler RC, Warner CH, Ivany C, Petukhova MV, Rose S, Bromet EJ, et al. Predicting suicides after psychiatric hospitalization in us army soldiers: the army study to assess risk and resilience in servicemembers (army starrs). JAMA Psychiatry (2015) 72:49–57. doi: 10.1001/jamapsychiatry.2014.1754
6. Walsh CG, Ribeiro JD, Franklin JC. Predicting suicide attempts in adolescents with longitudinal clinical data and machine learning. J Child Psychol Psychiatry (2018) 59:1261–70. doi: 10.1111/jcpp.12916
7. Simon GE, Johnson E, Lawrence JM, Rossom RC, Ahmedani, B, Frances L, Lynch, et al. Predicting suicide attempts and suicide deaths following outpatient visits using electronic health records. Am J Psychiatry (2018) 175:951–60. doi: 10.1176/appi.ajp.2018.17101167
8. Ribeiro JD, Pease JL, Gutierrez PM, Silva C, Bernert RA, Rudd MD, et al. Sleep problems outperform depression and hopelessness as cross-sectional and longitudinal predictors of suicidal ideation and behavior in young adults in the military. J Affect Disord. (2012) 136:743–50. doi: 10.1016/j.jad.2011.09.049
9. Eickhoff E, Yung K, Davis DL, Bishop F, Klam WP, Doan AP. Excessive video game use, sleep deprivation, and poor work performance among U.S. marines treated in a military mental health clinic: a case series. Mil Med. (2015) 180:e839–43. doi: 10.7205/MILMED-D-14-00597
10. Pompili M, Innamorati M, Forte A, Longo L, Mazzetta C, Erbuto D, et al. Insomnia as a predictor of high-lethality suicide attempts. Int J Clin Pract. (2013) 67:1311–6. doi: 10.1111/ijcp.12211
11. Walsh CG, Ribeiro JD, Franklin JC. Predicting risk of suicide attempts over time through machine learning. Clin Psychol Sci. (2017) 5:457–69. doi: 10.1177/2167702617691560
12. McKernan LC, Lenert MC, Crofford LJ, Walsh CG. Outpatient engagement lowers predicted risk of suicide attempts in fibromyalgia. Arthritis Care Res (Hoboken). (2018). doi: 10.1002/acr.23748. [Epub ahead of print].
13. Carter G, Milner A, McGill K, Pirkis J, Kapur N, Spittal MJ. Predicting suicidal behaviours using clinical instruments: systematic review and meta-analysis of positive predictive values for risk scales. Br J Psychiatry (2017) 210:387–95. doi: 10.1192/bjp.bp.116.182717
14. Saigle V, Racine E. Ethical challenges faced by healthcare professionals who care for suicidal patients: a scoping review. Monash Bioeth Rev. (2018) 35:50–79. doi: 10.1007/s40592-018-0076-z
15. Hirschfeld RM, Russell JM. Assessment and treatment of suicidal patients. New Eng J Med. (1997) 337:910–15.
16. Simon RI, Hales RE. The American Psychiatric Publishing Textbook of Suicide Assessment and Management. Washington, DC: American Psychiatric Pub. (2012).
17. Institute of Medicine (US) Roundtable on Evidence-Based Medicine, Olsen LA, Aisner D, McGinnis JM, editors. The Learning Healthcare System: Workshop Summary. Washington, DC: National Academies Press (2007).
18. Everitt T, Goertzel B, Potapov A. Artificial General Intelligence. Lecture Notes in Artificial Intelligence. Heidelberg: Springer (2017).
19. Kessler RC, Hwang I, Hoffmire CA, McCarthy JF, Petukhova MV, Rosellini AJ, et al. Developing a practical suicide risk prediction model for targeting high-risk patients in the Veterans health Administration. Int J Methods Psychiatr Res. (2017) 26:1–14 doi: 10.1002/mpr.1575
Keywords: artificial intelligence, suicide, ethics, code of ethics, machine learning
Citation: McKernan LC, Clayton EW and Walsh CG (2018) Protecting Life While Preserving Liberty: Ethical Recommendations for Suicide Prevention With Artificial Intelligence. Front. Psychiatry 9:650. doi: 10.3389/fpsyt.2018.00650
Received: 10 September 2018; Accepted: 16 November 2018;
Published: 03 December 2018.
Edited by:
Matthias Jaeger, Psychiatrie Baselland, SwitzerlandReviewed by:
Gianluca Serafini, Dipartimento di Neuroscienze e Organi di Senso, Ospedale San Martino (IRCCS), ItalyEva-Maria Pichler, Medizinische Fakultät, Universität Zürich, Switzerland
Copyright © 2018 McKernan, Clayton and Walsh. This is an open-access article distributed under the terms of the Creative Commons Attribution License (CC BY). The use, distribution or reproduction in other forums is permitted, provided the original author(s) and the copyright owner(s) are credited and that the original publication in this journal is cited, in accordance with accepted academic practice. No use, distribution or reproduction is permitted which does not comply with these terms.
*Correspondence: Lindsey C. McKernan, TGluZHNleS5tY2tlcm5hbkB2YW5kZXJiaWx0LmVkdQ==