- 1Department of Psychiatry, University Hospital of Bellvitge-IDIBELL, Barcelona, Spain
- 2Ciber Fisiopatologia Obesidad y Nutrición, Instituto Salud Carlos III, Barcelona, Spain
- 3Department of Clinical Sciences, School of Medicine, University of Barcelona, Barcelona, Spain
- 4Departament de Psicobiologia i Metodologia de les Ciències de la Salut, Universitat Autònoma de Barcelona, Barcelona, Spain
- 5Department of Psychiatry, University of Minnesota Medical School, Minneapolis, MN, United States
- 6Département de Psychoéducation, Université du Québec à Trois-Rivières, Trois-Rivières, QC, Canada
- 7Department of Experimental Psychology, University of Granada, Granada, Spain
- 8Brain, Mind and Behavior Research Center (CIMCYC), University of Granada, Granada, Spain
- 9Ciber de Salud Mental, Instituto Salud Carlos III, Madrid, Spain
- 10Departament d'Infermeria de Salut Pública, Salut Mental i Maternoinfantil, Escola Universitària d'Infermeria, Universitat de Barcelona, Barcelona, Spain
- 11Department of Psychology, University of Alcalá, Alcalá de Henares, Spain
Background: Gambling disorder (GD) is a heterogeneous disorder which has clinical manifestations that vary according to variables in each individual. Considering the importance of the application of specific therapeutic interventions, it is essential to obtain clinical classifications based on differentiated phenotypes for patients diagnosed with GD.
Objectives: To identify gambling profiles in a large clinical sample of n = 2,570 patients seeking treatment for GD.
Methods: An agglomerative hierarchical clustering method defining a combination of the Schwarz Bayesian Information Criterion and log-likelihood was used, considering a large set of variables including sociodemographic, gambling, psychopathological, and personality measures as indicators.
Results: Three-mutually-exclusive groups were obtained. Cluster 1 (n = 908 participants, 35.5%), labeled as “high emotional distress,” included the oldest patients with the longest illness duration, the highest GD severity, and the most severe levels of psychopathology. Cluster 2 (n = 1,555, 60.5%), labeled as “mild emotional distress,” included patients with the lowest levels of GD severity and the lowest levels of psychopathology. Cluster 3 (n = 107, 4.2%), labeled as “moderate emotional distress,” included the youngest patients with the shortest illness duration, the highest level of education and moderate levels of psychopathology.
Conclusion: In this study, the general psychopathological state obtained the highest importance for clustering.
Introduction
Gambling disorder (GD) is defined as a persistent and recurrent maladaptive pattern of gambling behavior associated with impaired functioning in the personal, social, and occupational spheres of one's life (1). Across the globe, GD affects 0.7–6.5% of adults during their lifetime (2). Evidence points to GD as being more likely to occur among males, singles or divorcees, unemployed individuals or with a low income, and with a lower level of education (3). Moreover, GD presents high comorbidity with other mental disorders, mainly substance or alcohol dependence (4) and personality disorders (5).
From an etiological point of view, GD is a multi-causal disorder involving psychosocial and neurobiological risk factors. The interaction between these factors and the specific implication of each can aid in discerning the phenotypes of GD patients. One of the psychosocial risk factors of greatest interest has been psychological or emotional distress, which is related to high levels of general psychopathology as measured by the Symptom Checklist-Revised (SCL-90-R) (6, 7). In this sense, Blaszczynski and Nower (8) pointed out the existence of a subtype of individuals with GD characterized by high emotional vulnerability. These authors hypothesized that emotional vulnerability is a result of different psychosocial factors, such as childhood disturbances, certain personality traits, mood alterations (depression and anxiety), poor coping skills, and substance use. In this context, gambling is viewed as a means of relieving aversive affective states. Posterior evidence has concluded that these “emotionally vulnerable” gamblers exhibit higher psychopathological and gambling severity than other subtypes of gamblers, as well as a higher prevalence of substance abuse and an earlier onset of gambling problems (9–12).
On the one hand, problematic or pathological gambling behavior has frequently been associated with certain types of gambling activities. That is, not all gambling activities seem to be addictive to the same degree. Until recently, slot machines were considered the most potentially addictive type of gambling because of their technical and situational characteristics (13). However, the emergence and proliferation of online gambling in the last decade has radically modified the characteristics of gambling activities, by which gambling settings have been shifted to home and work environments (14). Online gambling is considered potentially more addictive because of its unique features, such as anonymity, betting speed, accessibility, attractive design, and marketing, and low cost (15, 16). Indeed, recent evidence has displayed that online gambling increases the likelihood of being a problematic gambler when compared with offline gambling (16). Although online gamblers seem to be an heterogeneous group, their profile is often the following: male, young, single, high levels of education, good economic income, varied gambling preferences, greater severity of gambling behavior (in terms of spending higher amounts), as well as higher rates of alcohol and substance use (17–19). Likewise, it can be expected that specific gambling activities have a higher representation in certain subtypes of gamblers, for instance, online gambling may be more frequent in those subtypes composed of younger gamblers with higher education, with better incomes and the possibility of betting larger amounts of money.
Beyond these results, the question is whether it is possible to formulate the hypothesis that emotional alterations and the type of preferred gambling could explain the existence of more specific subtypes. To the best of our knowledge, no studies have explored the last question in large clinical samples made up of consecutive patients who have requested specific treatment for gambling problems. For that reason, the objective of this study is to explore the existence of distinct GD phenotypes, in a large sample of patients seeking treatment for GD, through clustering analysis, using a wide set of variables including sociodemographic, gambling-related factors, general psychopathological state and personality traits as indicators for the grouping. Based on the available evidence in literature, we hypothesized that different empirical clusters would emerge based on emotional distress. Due to the dramatic change in relation to the proliferation of different types of gambling in this last decade (specifically, the popularization of online gambling) and the change in profiles of people affected by GD, our secondary hypothesis was that one of these clusters would overlap with the emerging profile of the online gambler.
Materials and Methods
Participants
The study sample included n = 2,570 patients treated at the Gambling Disorder Unit at the Department of Psychiatry of Bellvitge University Hospital, in Barcelona, Spain. This public hospital is certified as a tertiary care center for the treatment of psychologically addictive behaviors and it oversees the treatment of complex cases. The catchment area of the hospital includes over two million people in the metropolitan area of Barcelona.
All patients that met diagnostic criteria for GD, between the ages of 18 and 75, were selected for this study. Patients were recruited between January 2005 and November 2015. Exclusion criteria included having an intellectual disability or severe mental disorders (schizophrenia or other psychotic disorders, bipolar disorder, etc.), or meeting criteria for another behavioral addiction apart from GD.
Most participants were men (n = 2,365, 92.0%), born in Spain (n = 2,405, 93.6%), were married or had a partner (n = 1,324, 51.5%) and were employed (n = 1,525, 59.3%). The mean age for the total sample was 41.7 years old (SD = 12.8), the mean age of GD onset was 36.8 years old (SD = 12.9) and the mean duration of GD was 14.0 years (SD = 10.0). The first columns of Table 1 include a description of the study sample.
Instruments
GD Diagnosis and Severity
South Oaks Gambling Screen (SOGS) (20)
This is a self-report 20-item screening questionnaire that discriminates between probable pathological, problem and non-problem gamblers. The Spanish validation used in this work showed excellent internal consistency (α = 0.94) and test-retest reliability (r = 0.98) (21).
Diagnostic Questionnaire for Pathological Gambling According to DSM-IV Criteria (22). Spanish Adaptation by Jiménez-Murcia et al. (23).
This 19-item questionnaire assesses the DSM-IV diagnostic criteria for pathological gambling. It should be noted that with the release of the DSM-5 (1), the term pathological gambling was replaced with GD. Internal consistency of this questionnaire ranged between α = 0.81 for the general population and α = 0.77 for gambling treatment samples. Convergent validity with the SOGS scores was high: r = 0.77 for the general population and r = 0.75 for gambling treatment groups (22). Due to the fact that this questionnaire includes the 8th criterion exploring the presence of illegal acts related to GD, in this study, all patient diagnoses were reassessed and recodified post hoc to avoid the confounding effect of increased GD severity, in patients with a criminal history. Therefore, only patients who met DSM-5 criteria for GD were included in our analysis.
Personality and Psychopathological Status
Temperament and Character Inventory-Revised (TCI-R) (24)
This is a reliable and valid 240-item questionnaire that measures seven personality dimensions: four temperament (novelty seeking, harm avoidance, reward dependence and persistence) and three character dimensions (self-directedness, cooperativeness and self-transcendence). Temperament refers to automatic emotional responses to experiences that are moderately heritable and stable throughout life. In contrast, character refers to self-concepts and individual differences in goals and values. Character is moderately influenced by insight and learning. All items are measured on a 5-point Likert-type scale. A validated Spanish version was used (25). The scales in the Spanish revised version showed adequate internal consistency (Cronbach's α mean value of 0.87). In the study at hand, consistency indices ranged from good (α = 0.70 for novelty seeking) to very good (α = 0.84 for persistence and self-transcendence).
Symptom Checklist-Revised (SCL-90-R) (26)
This questionnaire is a measure of emotional distress and evaluates a broad range of psychological problems and psychopathological symptoms. This questionnaire contains 90 items and assesses nine primary symptom dimensions: somatization, obsession-compulsion, interpersonal sensitivity, depression, anxiety, hostility, phobic anxiety, paranoid ideation, and psychoticism. It also includes three global indices: (1) a global severity index (GSI), designed to measure overall psychological distress; (2) a positive symptom distress index (PSDI), to measure the intensity of the symptoms; and (3) a positive symptom total (PST), which reflects self-reported symptoms. A validated Spanish version was used (27). The Spanish validation scale obtained good psychometrical indexes, with a mean internal consistency of 0.75 (Cronbach's alpha). In the study sample, consistency indices were in the very good (α = 0.83 for hostility) to excellent range (α = 0.98 for the global indexes), with exception of paranoia (α = 0.74, good).
Other Sociodemographic and Clinical Variables
Additional demographic, clinical, and social/family variables related to gambling were measured using a semi-structured, face-to-face clinical interview described elsewhere (28). Some of the GD variables covered included the age of GD onset, the mean and maximum amount of monetary spending in gambling episodes, and accumulated gambling-related debts. Moreover, GD severity was assessed according to five different variables: total number of DSM-5 criteria, SOGS-total score, number of gambling activities, mean bets per gambling episode, and cumulative debts.
Table 1 contains the α-values obtained in the sample for all the scales in this work. These coefficients ranged between good to excellent.
Procedure
The present study was carried out in accordance with the latest version of the Declaration of Helsinki. The University Hospital of Bellvitge Ethics Committee of Clinical Research approved the study (ref. 307/06), and signed informed consent was obtained from all participants. Experienced psychologists and psychiatrists with more than 15 years of clinical experience in the field of addictive disorders conducted the two face-to-face clinical interviews. All the measures used in this study correspond to the assessment carried out prior to the beginning of treatment.
Statistical Analysis
Statistical analysis was carried out with SPSS 20 for Windows. Empirical clusters were explored through the TwoStep-Clustering Component, entering origin (Spanish vs. immigrant), sex, education level, civil status, employment status, age, age of GD onset, duration of the gambling problem, GD severity and gambling related variables (SOGS-total score, number of gambling activities, mean bets per gambling episode and cumulative debts), comorbid psychopathological state (SCL-90R scales), substances use-abuse (tobacco, alcohol and other drugs) and personality traits (TCI-R scales) as indicators. The TwoStep-Clustering system constitutes a scalable algorithm designed to handle large datasets including both continuous and categorical variables. During the first step, subjects are pre-clustered into many small sub-clusters according to a sequential clustering approach and during the second step the resulting sub-clusters are considered as inputs and grouped into the desired number of clusters according to the agglomerative hierarchical clustering method. By default, the TwoStep algorithm uses a combination of the Schwarz Bayesian Information Criterion and log-likelihood distance in determining the final number of clusters, choosing a solution with a reasonably large ratio of Schwarz Bayesian Information Criterion changes and a large ratio of distance measures. The log-likelihood measure is computed by using the normal density for continuous variables and the multinomial probability mass function for categorical variables. In this study, we compared the automatic number of clusters selected by the TwoStep Clustering procedure as finalistic candidate solutions, and two additional models: the auto-determined number of clusters minus one, and the auto-determined number plus one. The final chosen model was based on (29) criteria: (a) the highest cohesion and separation index; (b) adequate number of individuals in each group (to allow for meaningful comparisons); and (c) the best clinical interpretability.
The comparison between the derived empirical clusters for the study variables (sociodemographic, clinical, and personality measures) was carried out with chi-square tests (χ2) for categorical factors and analysis of variance (ANOVA) for quantitative measures. Finner's correction (a familywise error rate stepwise procedure which offers more powerful test than the classical Bonferroni correction) controlled for inflation in Type-I error due to multiple statistical comparisons (30).
Results
Cluster Composition and Comparison Between Groups
The three-cluster solution was selected as being the most optimal in the sample of n = 2,570 GD patients who met inclusion criteria. This structure was auto-determined as the most appropriate by the TwoStep-Clustering procedure. The Silhouette's index [a validity measure of consistency within clusters, which can be interpreted as the level of cohesion/separation for the empirical derived groups; (31)] was moderate (average Silhouette = 0.3, suggesting reasonable evidence of cluster structure in the data). The comparison between the largest cluster size (n = 1,555, 60.5%) and the smallest (n = 107, 4.2%) yielded a ratio of 14.53.
Figure 1 summarizes the composition of the final solution for the three-cluster model. The first panel contains the bar-graph with the relative importance of each indicator in the clustering, which reports how well each variable can differentiate the different derived clusters (the higher the importance of the measure, the less likely it is that the variation for the variable between clusters is due to chance and the more likely it is due to underlying differences). In this study, the general psychopathological state registered on the SCL-90-R questionnaire obtained the highest relative importance for clustering, followed by the TCI-R self-directedness and harm avoidance dimensions, and the GD severity measures (number of gambling activities, and SOGS-total score). The poorest relevance for clustering was obtained for the sample origin (Spanish vs. immigrant), civil status, substances use-abuse, chronological age, illness duration, TCI-R persistence scores, and age of GD onset. The table included in the right panel of Figure 1 contains the centroids for the indicators in the clustering (means for the quantitative variables and the percentage distribution for categorical variables), which summarizes the clusters patterns for this set of variables.
Cluster 1 contained 908 patients (35.5% of the sample) and it was characterized by featuring the oldest patients with the longest duration of GD, the highest GD severity, the most severe levels of general psychopathology or emotional distress (highest mean scores on the SCL-90R questionnaire), the highest levels in the personality traits harm avoidance and self-transcendence and the lowest levels in the personality domains of self-directedness and cooperativeness. This cluster has been labeled as “high emotional distress.”
Cluster 2, the largest empirical group with 1,555 patients (60.5% of the sample), was characterized by including the highest proportion of men, the lowest GD severity, the best psychopathological state (lowest means on the SCL-90R scales), the lowest levels in the personality traits novelty seeking and harm avoidance, and the highest mean scores in the personality scales reward dependence, persistence, self-directedness, and cooperativeness. This cluster has been labeled as “mild emotional distress.”
Cluster 3, with only 107 patients (4.2%), included the youngest participants with the lowest GD duration, the highest levels of education, the lowest prevalence of substance use-abuse, the most severe economical consequences related to the GD (mean bets per episode and cumulate debts due to gambling), and moderate levels in psychopathological state. This cluster has been labeled as “moderate emotional distress.”
Distribution of the Empirical Clusters During the Period of Data Recruitment
The line-chart in the Figure 2 shows the prevalence of each cluster in the study sample during the data recruitment period. As a whole, the most prevalent cluster during the time of data recruitment was cluster 2 (“mild emotional distress”), followed by cluster 1 (“high emotional distress”). A positive significant linear trend for clusters 1 (its presence increased from 29.4% in 2005 to 40.3% in 2015) and 3 (increases ranged from 1.7% in 2005 to 7.3% in 2015) was found, while cluster 2 obtained a negative significant linear trend (prevalence decreased from 68.9% in 2005 to 52.4% in 2015).
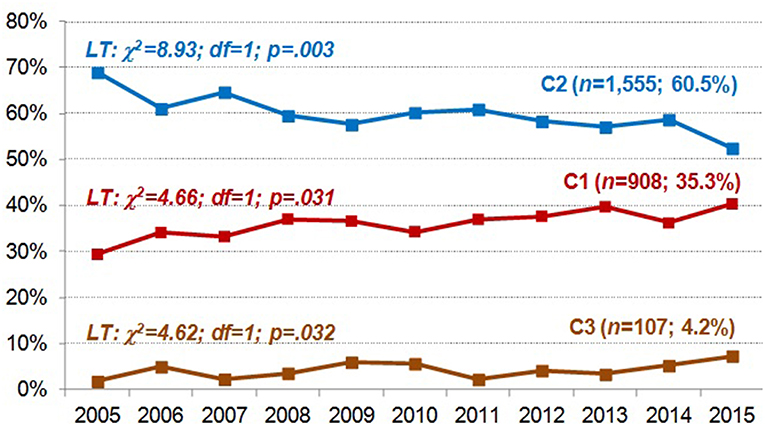
Figure 2. Distribution of the prevalence of each cluster during the data recruitment. LT, linear trend.
Comparisons Between Clusters
The last columns of Table 1 contain the results of the comparison between the groups for the variables analyzed in the study and defined as indicators in the clustering procedure.
Table 2 includes the gambling preferences and the comparisons between clusters. The preferred gambling activity was slot machines for clusters 1 and 2, while cluster 3 included a higher proportion of participants reporting online gambling, but also bingo, casinos, and land-based sport betting as their main preferences.
Discussion
The aim of this study was to explore the existence of distinct GD phenotypes in a large sample of patients seeking treatment for GD through clustering analysis, using a wide set of variables, including sociodemographic, gambling-related factors, general psychopathological state, and personality traits as indicators. We expected that different empirical clusters would emerge based on emotional distress or psychopathological state, measured using SCL-90R questionnaire.
In fact, in keeping with models proposing emotion-regulation processes to be strongly related to individual differences among GD patients (8, 32), the most relevant variable for the clustering analysis was emotional distress (SCL-90-R); followed by personality traits (TCI-R), and GD severity measures (number of gambling activities and SOGS-total score). Thus, three empirical clusters emerged defining psychopathological status as their main indicator: (a) Cluster 1 composed of more dysfunctional patients who reported high emotional distress; (b) Cluster 2 grouped patients with low distress levels; (c) Cluster 3 characterized by mainly young patients with medium distress levels.
From a clinical perspective, these findings could be understood taking into account that certain personality traits or domains could be associated with a greater or lesser degree of psychopathology or emotional distress, as shown in previous research (33–37). Specifically, according to the results, the personality trait most closely associated with psychopathology or emotional distress was harm avoidance. All the mentioned personality and emotional vulnerability characteristics make Cluster 1 especially complex from a therapeutic point of view, given that these patients present greater gambling severity, more vulnerability to stressful situations and specific personality characteristics that hinder their ability to cope with adverse situations. These personality traits are characterized by a greater tendency to anxiety, worry and insecurity, isolation, and disconnection from the environment, poor decision-making skills and planning skills (38). In this profile, therefore, gambling could be a dysfunctional habit acquired and maintained over time as a mechanism to avoid problems and difficulties in emotion regulation. Thus, it could represent a group of patients more inclined to present poor response to treatment, as various studies have previously shown (39–41). As such, they may require treatment programs that incorporate techniques to improve these aspects, favoring a better response to therapy (8).
Another interesting aspect to highlight from these results is that patients with the highest emotional distress and severity were the oldest patients with the longest GD duration, most of them included in the Cluster 1. This result would be in agreement with previous studies that have focused on exploring the clinical differences of patients diagnosed with GD, depending on age (42–44). The findings of these studies suggest that age is a strong moderating variable in the evolution of the disorder and that it has a relevant impact on the emotional aspects associated with gambling. In this sense, in the elderly, anxious-depressive symptoms would be related to medical illnesses and health problems, but also to stressful life situations that appear at this stage of life (e.g., retirement, reduced purchasing power, loneliness, loss of family or friends, etc.) (35, 45, 46).
Patients from our study also displayed different preferences in gambling activities, depending on the cluster that they were formed part of. Most of patients included in Cluster 1 and Cluster 2 preferred slot machines. In contrast, Cluster 3 was mainly composed of patients with a higher variety of gambling preferences, even though the most frequent gambling activity was again slot machines, these were nearly followed by online gambling. These results are in agreement with other studies having observed that online gamblers usually also engaged in land-based gambling, combining both modalities (47). Literature supports that online gambling is mainly practiced by young men (17–19), according to our results where Cluster 3 was composed of the youngest patients who also prefer new forms of gambling activities such as online gambling. The increase in the use of online gambling may be because of features that make it more convenient and accessible (15, 16), especially for young people. Relatedly, the rest of features of patients included in Cluster 3 also coincide with profile of online gamblers, being male, high levels of education, employed with good incomes, betting larger amounts of money, as well as diverse gambling preferences (17–19).
In more general terms, Cluster 3 seems to largely overlap with a subtype of gambler at least partially identified in previous research. On the one hand, this cluster is likely be responsible for the pattern of correlations between different types of high arousal, large-stake games (bets, casino games, skill-based games) categorized as Type I gambling in Navas et al. (48). On the other hand, GD patients with this profile (even after controlling for age and other potential confounders) are more likely to present high sensation- or novelty-seeking scores and stronger gambling-related cognitive distortions. At the same time, and somewhat counterintuitively, in these patients, general self-regulatory, and executive functioning, as well as high-order cognitive processing, tend to be well preserved (32, 49, 50). Integrity of high-order cognitive and regulatory processes is fully compatible with the fact that Cluster 3 GD patients present a higher level of education, higher self-directedness scores, and a lower risk of suffering from a comorbid addictive disorder. In spite of these seemingly “favorable” sociodemographic and neurocognitive features, gambling severity is by no means reduced in this profile. Actually, the variable cumulated amount of debts exceeded 94,000 euros in Cluster 3, while in the other groups, it did not reach 10,000 euros. Different studies point out that, in online gambling, spending money is much easier and faster; therefore, debts are acquired in a shorter period of time (14, 47).
Online gambling has brought about the appearance of a new GD phenotype, designated as Cluster 3 in our study. Although it includes fewer patients (only 4.2% of the total sample), Cluster 3 represents a completely new and differentiated type of GD patient, with respect to the previous subgroup identification studies (8, 10–12). We can postulate, seeing how the online gambling market is increasing and attracting young people, that this group will be more frequent in the near future. Therefore, it is necessary to identify indicators of good or bad response to treatment to be able to manage this new and growing phenotype. More specifically, novelty- and sensation-seeking—seemingly elevated in Cluster 3—have been observed to be among the best predictors of low treatment adherence and compliance (51–53). This finding is not only relevant to tailoring treatment plans, but it could also indicate that the low percentage of Cluster 3 patients in clinical sample could be partially due to the fact that a some potential patients with such a profile are less prone to seek or remain in treatment.
Finally, the progression of the clusters throughout time is information of clinical and therapeutic interest. It can be seen how Cluster 2 decreased significantly whereas Clusters 1 and 3 have increased rates. It could be possible that we are at a crucial point in terms of a change in the clinical profiles of GD individuals, which may require the development of new theoretical models and therapeutic strategies that adapt to these changes.
Limitations
The present study has the potential to improve current knowledge on GD subtypes and provides relevant information by describing three different clusters depending on different emotional alterations. However, this research has some limitations that need to be considered. First of all, our sample was principally made of males referred to a specialized unit that usually deals with severe cases of GD. For this reason, our results have to be taken with caution before being generalized to the general population. Future studies should be conducted to explore if the described subtypes are applicable to both sexes and include samples from other centers. Second, this is a cross-sectional study; therefore, no causality can be driven from the results. Future studies should test if the observed clusters could also predict treatment outcome.
Conclusion
Cluster formation was carried out mainly taking into account the psychopathological state or emotional distress, using the SCL-90-R. This variable allowed for differentiation of homogeneous groups or subtypes within the spectrum of the disorder. In agreement with Chamberlain (54), the identification of differentiated subtypes can contribute to a better understanding of GD from a clinical perspective. Characterizing phenotypes and endophenotypes will favor the design and implementation of increasingly specific interventions that improve response to treatment.
Author Contributions
SJ-M, RG, and FF-A designed the experiment and analyzed the data. SJ-M, RG, FF-A, TS, GM-B, NM-B, NA, MG-P, and ZA conducted the experiment and wrote a first draft of the manuscript. TS, GM-B, RS, JT, ML-M, TM-M, JP, JN, CS-M, AdP-G, VM-R, and JM further reviewed and modified the manuscript.
Funding
Financial support was received through the Ministerio de Economía y Competitividad (grant PSI2015-68701-R) and the Investigación subvencionada por la Delegación del Gobierno para el Plan Nacional sobre Drogas (2017I067). FIS PI14/00290, FIS PI17/01167, and 18MSP001 - 2017I067 received aid from the Ministerio de Sanidad, Servicios Sociales e Igualdad. CIBER Fisiología Obesidad y Nutrición (CIBERobn) and CIBER Salud Mental (CIBERSAM), both of which are initiatives of ISCIII. GM-B is supported by a predoctoral AGAUR grant (2018 FI_B200174), grant co-funded by the European Social Fund (ESF) ESF, investing in your future. With the support of the Secretariat for Universities and Research of the Ministry of Business and Knowledge of the Government of Catalonia. TM-M and ML-M are supported by a predoctoral Grant of the Ministerio de Educación, Cultura y Deporte (FPU16/02087 and FPU15/02911). JP and JN are supported by a grant from the Spanish Government (Ministerio de Economía y Competitividad, Secretaría de Estado de Investigación, Desarrollo e Innovación; Convocatoria 2017 de Proyectos I+D de Excelencia, Spain; co-funded by the Fondo Europeo de Desarrollo Regional, FEDER, European Union), with reference number PSI2017-85488-P. We also want to thank CERCA Programme/Generalitat de Catalunya for institutional support.
Conflict of Interest Statement
The authors declare that the research was conducted in the absence of any commercial or financial relationships that could be construed as a potential conflict of interest.
References
1. American Psychiatric Association. Diagnostic and Statistical Manual of Mental Disorders. 5th ed. Washington, DC: American Psychiatric Association (2013).
2. Calado F, Griffiths MD. Problem gambling worldwide: an update and systematic review of empirical research (2000-2015). J Behav Addict. (2016) 5:592–613. doi: 10.1556/2006.5.2016.073
3. Pavarin R, Fioritti A, Marani S, Gambini D, Turino E, Piazza A. Who are the subjects with gambling-related problems requiring treatment? a study in northern Italy. J Clin Med. (2018) 7:80. doi: 10.3390/jcm7040080
4. Molinaro S, Benedetti E, Scalese M, Bastiani L, Fortunato L, Cerrai S, et al. Prevalence of youth gambling and potential influence of substance use and other risk factors across 33 European countries: first results from the 2015 ESPAD study. Addiction. (2018) 113:1862–73doi: 10.1111/add.14275
5. Ronzitti S, Kraus SW, Hoff RA, Clerici M, Potenza MN. Problem-gambling severity, suicidality and DSM-IV Axis II personality disorders. Addict Behav. (2018) 82:142–50. doi: 10.1016/j.addbeh.2018.03.003
6. Blaszczynski A, Steel Z, Mcconaghy N. Impulsivity in pathological gambling: the antisocial impulsivist. Addiction. (1997) 92:75–87. doi: 10.1111/j.1360-0443.1997.tb03639.x
7. Steel Z, Blaszczynski A. The factorial structure of pathological gambling. J Gambl Stud. (1996) 12:3–20. doi: 10.1007/BF01533186
8. Blaszczynski A, Nower L. A pathways model of problem and pathological gambling. Addiction. (2002) 97:487–99. doi: 10.1046/j.1360-0443.2002.00015.x
9. Milosevic A, Ledgerwood DM. The subtyping of pathological gambling: a comprehensive review. Clin Psychol Rev. (2010) 30:988–98. doi: 10.1016/j.cpr.2010.06.013
10. Ledgerwood DM, Petry NM. Subtyping pathological gamblers based on impulsivity, depression, and anxiety. Psychol Addict Behav. (2010) 24:680–8. doi: 10.1037/a0019906
11. Moon M, Lister JJ, Milosevic A, Ledgerwood DM. Subtyping non-treatment-seeking problem gamblers using the pathways model. J Gambl Stud. (2017) 33:841–53. doi: 10.1007/s10899-016-9658-y
12. Alvarez-Moya EM, Jiménez-Murcia S, Aymamí MN, Gómez-Peña M, Granero R, Santamaría J, et al. Subtyping study of a pathological gamblers sample. Can J Psychiatry. (2010) 55:498–506. doi: 10.1177/070674371005500804
13. Griffiths M, Hayer T, Meyer G. Problem Gambling in Europe: Challenges, Prevention, and Interventions. Griffiths M, Hayer T, Meyer G, eds. New York, NY: Springer (2009).
14. Gainsbury SM, Russell A, Hing N, Wood R, Lubman D, Blaszczynski A. How the internet is changing gambling: findings from an Australian prevalence survey. J Gambl Stud. (2015) 31:1–15. doi: 10.1007/s10899-013-9404-7
15. Bonnaire C. [Internet gambling: what are the risks?]. Encephale. (2012) 38:42–9. doi: 10.1016/j.encep.2011.01.014
16. Effertz T, Bischof A, Rumpf H-J, Meyer C, John U. The effect of online gambling on gambling problems and resulting economic health costs in Germany. Eur J Heal Econ. (2018) 19:967–78. doi: 10.1007/s10198-017-0945-z.
17. Mallorquí-Bagué N, Fernández-Aranda F, Lozano-Madrid M, Granero R, Mestre-Bach G, Baño M, et al. Internet gaming disorder and online gambling disorder: clinical and personality correlates. J Behav Addict. (2017) 6:669–77. doi: 10.1556/2006.6.2017.078
18. Wood RT, Williams RJ. A comparative profile of the internet gambler: demographic characteristics, game-play patterns, and problem gambling status. N Media Soc. (2011) 13:1123–41. doi: 10.1177/1461444810397650
19. Gainsbury SM, Wood R, Russell A, Hing N, Blaszczynski A. A digital revolution: comparison of demographic profiles, attitudes and gambling behavior of internet and non-internet gamblers. Comput Human Behav. (2012) 28:1388–98. doi: 10.1016/j.chb.2012.02.024
20. Lesieur HR, Blume SB. The South Oaks Gambling Screen (SOGS): a new instrument for the identification of pathological gamblers. Am J Psychiatry. (1987) 144:1184–8. doi: 10.1176/ajp.144.9.1184
21. Báez C, Echeburúa E, Fernández-Montalvo J. Características demográficas, de personalidad y psicopatológicas de los jugadores patológicos de máquinas tragaperras en tratamiento: un estudio descriptivo. Clínica y Salud. (1994) 5:289–305.
22. Stinchfield R. Reliability, validity, and classification accuracy of a measure of DSM-IV diagnostic criteria for pathological gambling. Am J Psychiatry. (2003) 160:180–2. doi: 10.1176/appi.ajp.160.1.180
23. Jimenez-Murcia S, Stinchfield R, Alvarez-Moya E, Jaurrieta N, Bueno B, Granero R, et al. Reliability, validity, and classification accuracy of a Spanish translation of a measure of DSM-IV diagnostic criteria for pathological gambling. J Gambl Stud. (2009) 25:93–104. doi: 10.1007/s10899-008-9104-x
24. Cloninger CR, Przybeck TR, Svrakic DM, Wetzel RD. The Temperament and Character Inventory (TCI): A Guide to Its Development and Use. St Louis, MO: Center for Psychobiology of Personality, Washington University (1994).
25. Gutiérrez-Zotes JA, Bayón C, Montserrat C, Valero J, Labad A, Cloninger CR. Temperament and Character Inventory-Revised (TCI-R). Standardization and normative data in a general population sample. Actas Españolas Psiquiatr. (2004) 32:8–15.
26. Derogatis L. SCL-90-R. Administration, Scoring and Procedures Manual. Clinical Psychometric. Baltimore, MD (1990).
28. Jiménez-Murcia S, Aymamí-Sanromà M, Gómez-Peña M, Álvarez-Moya E, Vallejo J. Protocols de Tractament Cognitivoconductual pel joc Patològic i d'altres Addiccions no Tòxiques. Hospital Universitari de Bellvitge, Departament de Salut, Generalitat de Catalunya. Barcelona (2006).
29. Nylund KL, Asparouhov T, Muthén M&, Muthén BO. Deciding on the number of classes in latent class analysis and growth mixture modeling: a monte carlo simulation study. Struct Equ Model. (2007) 14:535–69. doi: 10.1080/10705510701575396
30. Finner H. On a monotonicity problem in step-down multiple test procedures. J Am Stat Assoc. (1993) 88:920–3. doi: 10.2307/2290782
31. Rousseeuw PJ. Silhouettes: a graphical aid to the interpretation and validation of cluster analysis. J Comput Appl Math. (1987) 20:53–65. doi: 10.1016/0377-0427(87)90125-7
32. Navas JF, Billieux J, Verdejo-García A, Perales JC. Neurocognitive components of gambling disorder: implications for policy, prevention, and treatment. In: Bowden-Jones H, Dickson C, Dunand C, Simon O, editors. Harm Reduction for Problem Gambling: A Public Health Approach. London: Routledge (2019).
33. Aymamí N, Granero R, Penelo E, Fernández-Aranda F, Krug I, Gunnard K, et al. Anger in pathological gambling: Clinical, psychopathological, and personality correlates. Span J Psychol. (2014) 17:1–12. doi: 10.1017/sjp.2014.40
34. Brunborg GS, Hanss D, Mentzoni RA, Molde H, Pallesen S. Problem gambling and the five-factor model of personality: a large population-based study. Addiction. (2016) 111:1428–35. doi: 10.1111/add.13388
35. Moragas L, Granero R, Stinchfield R, Fernández-Aranda F, Fröberg F, Aymamí N, et al. Comparative analysis of distinct phenotypes in gambling disorder based on gambling preferences. BMC Psychiatry. (2015) 15:86. doi: 10.1186/s12888-015-0459-0
36. Mann K, Lemenager T, Zois E, Hoffmann S, Nakovics H, Beutel M, et al. Comorbidity, family history and personality traits in pathological gamblers compared with healthy controls. Eur Psychiatry. (2017) 42:120–8. doi: 10.1016/j.eurpsy.2016.12.002
37. Vaddiparti K, Cottler LB. Personality disorders and pathological gambling. Curr Opin Psychiatry. (2017) 30:45–9. doi: 10.1097/YCO.0000000000000300
38. Cloninger C, Svrakic D, Przybeck T. A Psychobiological model of temperament and character. Arch Gen Psychiatry. (1993) 50:975–90. doi: 10.1001/archpsyc.1993.01820240059008
39. Menchón JM, Mestre-Bach G, Steward T, Fernández-Aranda F, Jiménez-Murcia S. An overview of gambling disorder: from treatment approaches to risk factors. F1000Research. (2018) 7:434. doi: 10.12688/f1000research.12784.1
40. Alvarez-Moya EM, Ochoa C, Jiménez-Murcia S, Aymamí MN, Gómez-Peña M, Fernández-Aranda F, et al. Effect of executive functioning, decision-making and self-reported impulsivity on the treatment outcome of pathologic gambling. J Psychiatry Neurosci. (2011) 36:165–75. doi: 10.1503/jpn.090095
41. Tominaga Y, Aomori T, Hayakawa T, Kijima N, Morisky DE, Takahashi K, et al. Possible associations of personality traits representing harm avoidance and self-directedness with medication adherence in Japanese patients with type 2 diabetes. J Pharm Heal Care Sci. (2018) 4:16. doi: 10.1186/s40780-018-0112-4
42. Granero R, Penelo E, Stinchfield R, Fernandez-Aranda F, Savvidou LG, Fröberg F, et al. Is pathological gambling moderated by age? J Gambl Stud. (2014) 30:475–92. doi: 10.1007/s10899-013-9369-6
43. Jiménez-Murcia S, Granero R, Tárrega S, Angulo A, Fernández-Aranda F, Arcelus J, et al. Mediational role of age of onset in gambling disorder, a path modeling analysis. J Gambl Stud. (2016) 32:327–40. doi: 10.1007/s10899-015-9537-y
44. Sauvaget A, Jiménez-Murcia S, Fernández-Aranda F, Fagundo AB, Moragas L, Wolz I, et al. Unexpected online gambling disorder in late-life: a case report. Front Psychol. (2015) 6:655. doi: 10.3389/fpsyg.2015.00655
45. Dennis CB, Davis TD, Chang J, McAllister C. Psychological vulnerability and gambling in later life. J Gerontol Soc Work. (2017) 60:471–86. doi: 10.1080/01634372.2017.1329764
46. Parke A, Griffiths M, Pattinson J, Keatley D. Age-related physical and psychological vulnerability as pathways to problem gambling in older adults. J Behav Addict. (2018) 7:137–45. doi: 10.1556/2006.7.2018.18
47. Gainsbury SM, Russell A, Blaszczynski A, Hing N. The interaction between gambling activities and modes of access: a comparison of internet-only, land-based only, and mixed-mode gamblers. Addict Behav. (2015) 41:34–40. doi: 10.1016/j.addbeh.2014.09.023
48. Navas JF, Billieux J, Perandrés-Gómez A, López-Torrecillas F, Cándido A, Perales JC. Impulsivity traits and gambling cognitions associated with gambling preferences and clinical status. Int Gambl Stud. (2017) 17:102–24. doi: 10.1080/14459795.2016.1275739
49. Perales JC, Navas JF, Ruiz de Lara CM, Maldonado A, Catena A. Causal learning in gambling disorder: beyond the illusion of control. J Gambl Stud. (2017) 33:705–17. doi: 10.1007/s10899-016-9634-6
50. Ruiz de Lara CM, Navas JF, Soriano-Mas C, Sescousse G, Perales JC. Regional grey matter volume correlates of gambling disorder, gambling-related cognitive distortions, and emotion-driven impulsivity. Int Gambl Stud. (2018) 18:195–216. doi: 10.1080/14459795.2018.1448427
51. Aragay N, Jiménez-Murcia S, Granero R, Fernández-Aranda F, Ramos-Grille I, Cardona S, et al. Pathological gambling: understanding relapses and dropouts. Compr Psychiatry. (2015) 57:58–64. doi: 10.1016/j.comppsych.2014.10.009
52. Jiménez-Murcia S, Granero R, Fernández-Aranda F, Arcelus J, Aymamí MN, Gómez-Peña M, et al. Predictors of outcome among pathological gamblers receiving cognitive behavioral group therapy. Eur Addict Res. (2015) 21:169–78. doi: 10.1159/000369528
53. Jara-Rizzo MF, Navas JF, Steward T, López-Gómez M, Jiménez-Murcia S, Fernández-Aranda F, et al. Impulsividad y conciencia del problema predicen la adherencia terapéutica y el abandono del tratamiento en el trastorno por juego de azar. Adicciones. (2018) 1041. doi: 10.20882/adicciones.1041
Keywords: gambling disorder, clustering, personality traits, psychopathology, severity
Citation: Jiménez-Murcia S, Granero R, Fernández-Aranda F, Stinchfield R, Tremblay J, Steward T, Mestre-Bach G, Lozano-Madrid M, Mena-Moreno T, Mallorquí-Bagué N, Perales JC, Navas JF, Soriano-Mas C, Aymamí N, Gómez-Peña M, Agüera Z, del Pino-Gutiérrez A, Martín-Romera V and Menchón JM (2019) Phenotypes in Gambling Disorder Using Sociodemographic and Clinical Clustering Analysis: An Unidentified New Subtype? Front. Psychiatry 10:173. doi: 10.3389/fpsyt.2019.00173
Received: 03 September 2018; Accepted: 08 March 2019;
Published: 29 March 2019.
Edited by:
Yasser Khazaal, Centre Hospitalier Universitaire Vaudois, SwitzerlandReviewed by:
Mercedes Lovrecic, National Institute for Public Health, SloveniaXiaohui Xu, Texas A&M Health Science Center, United States
Copyright © 2019 Jiménez-Murcia, Granero, Fernández-Aranda, Stinchfield, Tremblay, Steward, Mestre-Bach, Lozano-Madrid, Mena-Moreno, Mallorquí-Bagué, Perales, Navas, Soriano-Mas, Aymamí, Gómez-Peña, Agüera, del Pino-Gutiérrez, Martín-Romera and Menchón. This is an open-access article distributed under the terms of the Creative Commons Attribution License (CC BY). The use, distribution or reproduction in other forums is permitted, provided the original author(s) and the copyright owner(s) are credited and that the original publication in this journal is cited, in accordance with accepted academic practice. No use, distribution or reproduction is permitted which does not comply with these terms.
*Correspondence: Susana Jiménez-Murcia, c2ppbWVuZXpAYmVsbHZpdGdlaG9zcGl0YWwuY2F0