- 1Neuroscience, La Jolla Laboratory, Astellas Research Institute of America LLC, San Diego, CA, United States
- 2Candidate Discovery Research Labs, DDR, Astellas Pharm Inc., Tsukuba, Japan
While the etiology of many neuropsychiatric disorders remains unknown, increasing evidence suggests that aberrant sensory processing plays a central role. For this class of disorders, which are characterized by affective, cognitive, and behavioral symptoms, electroencephalography remains the dominant tool for providing insight into the physiological and molecular underpinnings of the disease state and predicting the effectiveness of investigational new drugs. Within the spectrum of electrical activity present in the CNS, high-frequency oscillations in the gamma band are frequently altered in these patient populations. Measurement of gamma oscillation can be further classified into baseline and evoked, each of which offers a specific commentary on disease state. Baseline gamma analysis provides a surrogate of pharmacodynamics and predicting the time course effects of clinical candidate drugs, while alterations in evoked (time-locked) gamma power may serve as a disease biomarker and have utility in assessing patient response to new drugs. Together, these techniques offer complimentary methods of analysis for discrete realms of clinical and translational medicine. In terms of drug development, comprehensive analysis containing aspects of both baseline and evoked gamma oscillations may prove more useful in establishing better workflow and more accurate criteria for the testing of investigational new drugs.
Introduction
Electroencephalography (EEG) indirectly measures neuronal activity within the brain. The technique is widely used in neurology, cognitive science, and psychophysiological research. EEG is an attractive tool in the field of translational science, as it is a non-invasive procedure that offers insight into the cell- and system-level changes that underlie both the normal and disordered function of the CNS. Clinically, the technique allows study of the efficacy and impact of investigational new drugs on both a systems and population level.
While a significant fraction of EEG-based research focuses on relatively low-frequency oscillations (1–30 Hz), research into higher-frequency oscillations in the gamma band (30–200 Hz) have revealed alterations that are closely linked to various neuropsychiatric disorders (1–4), including schizophrenia and bipolar disorder. Numerous studies (in both humans and animal models) suggest that changes in gamma oscillation likely reflect the activity of parvalbumin-positive GABAergic interneuron populations (2, 5). This cell population plays an important role in sensory input and processing, and is of particular interest in diseases where these processes are altered. Specifically, it is hypothesized that alterations in gamma oscillations are indicative of an imbalance in excitation/inhibition (E/I) arising from the loss of function within the previously mentioned interneuron population that ultimately manifest as affective, cognitive, and behavioral changes. Alterations in gamma oscillation have been reported in schizophrenia (6–9), autism spectrum disorder (10), fragile X syndrome (11), bipolar disorder (12), major depressive disorder (13), and epilepsy (14). For these reasons, tracking these changes remains one of the most commonly employed techniques in recent drug discovery research for neuropsychiatric disorders (1, 15).
Measurement of gamma oscillation is typically performed in one of two ways. Baseline (resting) gamma measures native gamma oscillations in the absence of external stimuli. In contrast, evoked (time-locked) gamma is passive gamma oscillation elicited by auditory or visual cues. Oscillatory activity typically evoked during sensory/cognitive processing is characterized by band-limited modulations, which are a key feature of an oscillatory process. Especially, evoked gamma power is typically observed ~60–100 ms after stimulus (1). This is not normally observed in spontaneous or baseline activity. Baseline measures are generally more prone to artefacts. While the source of these artefacts is not always clear, researchers have identified significant contributions from muscle/saccadic artefacts towards high-frequency EEG activity, the removal of which has allowed better identification of the “true” signal from brain (16–18). The frequency, duration, and magnitude of the elicited activity provide a composite measurement of the subject's response to a one-time or ongoing series of sensory input. When considered collectively, the two approaches offer a somewhat unique, albeit overlapping commentary on the functional status of sensory processing within the CNS.
In a clinical context, gamma oscillation may be used either as proof of pharmacology or as a proof-of-concept tool. With regard to the former, EEG-based measurements are typically employed to track changes in brain activity, including gamma oscillation following the addition of an investigational new drug. Tracking these changes, usually through longitudinal monitoring of baseline EEG activity, provides a functional biomarker of drug targeting and activity. Alongside other more traditional pharmacokinetic/pharmacodynamic (PK/PD) and tolerability analyses, this approach provides guidance on trial design, dosing, and predicts clinical efficacy. For these reasons, this type of evaluation is generally incorporated relatively early in the clinical process, generally in healthy subjects. In proof-of-concept applications, changes in gamma oscillation need be accompanied by similar changes in surrogate endpoint(s) that comment directly on the disease (e.g., cognitive measurement, PANSS scale changes, etc.). In addition, some regard specific alterations in gamma band activity—usually Auditory Steady State Response (ASSR) deficits—as a potential disease biomarker for some neuropsychiatric disorders, as well as a means of stratifying patients for clinical trials, though further investigation of these claims is needed.
Gamma Oscillations in Understanding Disease Etiology
Studies of gamma band alterations have historically proven valuable in understanding the underpinnings of diseases with ostensible E/I imbalances. In animal models of neuropsychiatric disorder created by blunting NMDA signaling transduction in parvalbumin-positive interneurons baseline gamma power is increased, while evoked gamma power is decreased (5, 19). Similar results are observed in animals treated with NMDA antagonists (20, 21). This suggests two conclusions. First, the disruption in sensory input in the diseased state may be caused by a reduction in signal-to-noise ratio resulting from augmented baseline activity within interneurons. Second, NMDA-mediated signaling is directly implicated in maintaining E/I balance, as gamma alterations in animal models appear to mirror those of disease patients. While this last point is difficult to prove conclusively in patients, circumstantial evidence seems to support this working theory. For example, the addition of a GABA transporter inhibitor results in the increase in the evoked gamma power of healthy volunteers (22).
Gamma band changes have also contributed to the discovery that sensory balancing is not constrained to GABAergic neuron populations. Studies involving other neurotransmitters support the notion that the effects of E/I imbalance are more widespread than initially believed. Compounds targeting dopaminergic and norepinephrinergic neurons typically increase evoked gamma power (23, 24), indicating that these neurotransmitters can be indirectly surveilled using this measurement technique. Preclinical evidence in rodents also suggests that ASSR also changes in response to cholinergic neurotransmitter modulation (25). Based on these results, it stands to reason that ASSR could also be used a predictor for the efficacy of drugs targeting these classes of compounds.
The common role of the neural circuit mentioned above is their conserved function in sensory perception. Accordingly, imbalances in their regulation (either directly, or via manipulation of interneurons) could result in E/I imbalance. As one of the endophenotypes in neuropsychiatric disorders, dysfunction within these systems could manifest as false or warped sensory input in an affected individual. The monitoring of gamma oscillation as a means of commenting on this phenotype of neural function underscores the importance of this technique in dynamically characterizing the development of the disease from acute to a chronic state. Understanding the pathogenesis of etiologically challenging disorders is of critical importance in the development of next-generation neuropsychiatric drugs targeted to these pathways.
Gamma Oscillations in Drug Development
The development of new drugs encompasses a wide variety of topics, including the pharmacological profile (PK/PD, ADME, etc.) of each new drug and the ensuing response of the patient (ranging from cell- and molecular changes to the amelioration of symptoms and changes in clinical endpoints). Regarding the former, resting gamma measurement offers direct commentary on the activity of a particular cell population, in this case, inhibitory neurons involved in sensory processing. As these populations are the target for numerous drug development efforts, measuring baseline gamma response following administration of an investigational drug provides valuable retrospective information on dosing levels and therapeutic windows, acts as a useful secondary biomarker of efficacy and receptor occupancy, and can be used to corroborate PK/PD data for new compounds. Studies using baseline gamma are technically suited to capture these types of data, and have proven successful in this endeavor (26, 27).
Despite their success in this regard, the use of baseline gamma measurement in the evaluation of new CNS drugs does not necessarily extend beyond PK/PD assessments. Although abnormal resting gamma power was also identified as being connected to neuropsychiatric disorders and their preclinical animal models (21, 28), several studies have not identified this abnormality (29, 30). While this matter is not fully settled, recent evidence suggests that many psychiatric diseases, including schizophrenia and bipolar disorder, are rooted in abnormalities in sensory processing that relate to the disruption of evoked gamma response. As such, ASSR, rather than baseline gamma, is likely to be the superior measuring stick of clinical efficacy for diseases in this category.
Subsequent results have substantiated this hypothesis. In schizophrenia patients, abnormal 40-Hz ASSR has been reported in the prodromal state and has persisted into the chronic phase of the disease (6–9, 31–37). This relationship extends to clinical trials, where the effect size of ASSR to schizophrenia is reportedly over 0.5 (35). The close association between schizophrenia and evoked gamma power suggests it should be feasible to use this alteration as a proxy for proof of pharmacology in patient populations. This possibility is further buoyed by the observation that ASSR alterations in rodent models of psychiatric disease appear primarily in the gamma band range (21).
The consistent appearance of altered evoked gamma is both widespread and in support of the interneuron-mediated E/I imbalance theory. Abnormal evoked gamma is present in genetic models with impaired NMDA receptor function in parvalbumin-positive knockout animals (5, 38), as well as NMDA receptor-antagonized models using ketamine, phencyclidine, and MK-801 (20, 21, 39–44). In addition to supporting the broader hypothesis, these data demonstrate the role of evoked gamma measurement in establishing reliable translational validity from the preclinical to clinical development.
Combining resting and evoked gamma has also been used to increase understanding of disease features in situations where either method alone would be insufficient. When multiple NMDA receptor antagonists (memantine or ketamine) were given to healthy volunteers and schizophrenia patients, ASSR was enhanced in both populations (45, 46). Although this observation seems contradictory to NMDA receptor hypothesis for schizophrenia, this effect was echoed in rats, where enhanced ASSR was correlated with moderate NMDA receptor occupancy (20). This might be explained by the persistent reduction of neuronal firing of interneurons, i.e., GABAergic neurons, in the cortex, which might directly influence the mode of action through which gamma oscillation develops in ASSR. Thus, moderate NMDA receptor blockade biases the excitatory/inhibitory balance toward increased excitability, which could yield beneficial effects on brain function, similar to the antidepressive effect of ketamine (47). However, the effect of NMDA receptor antagonists on gamma power may not be explained solely by the disinhibition of GABAergic activity, as higher exposure of ketamine (and accordingly higher receptor occupancy) reduces ASSR signaling, which may reflect a collapse of cortical neuronal synchrony (20, 21).
These seemingly paradoxical results were resolved with additional examination of baseline gamma under similar conditions, which revealed that resting gamma was increased under the influence of the NMDA receptor antagonists. This observation presented another possibility, namely, that the observed ASSR disruptions in patient populations are caused by excess augmented baseline gamma activity. As mentioned previously, calculating ASSR involves the measurement of both the response to stimuli and resting state activity. In this case, an increase in baseline gamma levels—presumably a product of the inhibition of the GABA interneurons—decreases the signal-to-noise ratio, and reduces evoked gamma in the absence of any change in peak response. Indeed, additional studies (20, 21, 48) confirmed that baseline gamma power was augmented under these conditions, regardless of receptor occupancy. However, evoked gamma enables the detection of sensory processing dysfunction between healthy subject and patient endophenotypes because baseline gamma alterations remain difficult to detect in patients.
Understanding the synergistic analysis of baseline and evoked gamma remains a particularly challenging area of EEG research, as some evidence suggests that interpretation of these results may not be as straightforward as originally assumed. Grent-'t-Jong, T., et al., for example, demonstrated that a higher dose of S-ketamine (10 mg, bolus injection) also enhanced task-related gamma power in healthy subjects, while ketamine significantly increased PANSS scores (49). This suggests a dissociation of gamma power abnormalities observed during acute NMDA receptor hypofunction and in schizophrenia. The dissociation of evoked gamma power at a higher dose of NMDA receptor antagonist in these conditions suggests the need to carefully revisit the NMDAR hypothesis, particularly for acute (e.g., ketamine-induced) NMDAR hypofunction, to create a more holistic understanding of the molecular, cellular, and system-level interplay. Similarly, the true meaning of baseline gamma power modulation needs to be more fully explored in future studies.
Future Directions: Patient Stratification and Other Biomarkers
The repeated failures of investigational new drugs for neuropsychiatric disorders strongly suggest the need for new drug discovery approaches that target specific pathophysiological alterations shared in pre-stratified patient populations (50). Accumulated evidence demonstrates the excellent test-retest reliability of EEG measures (51–53), indicating the use of this method for identifying patient subpopulations that contain unique sensory processing deficits. A lack of patient stratification could affect the response rate among patients, reducing the overall efficacy and possibly contributing to a new drug's failure to achieve primary endpoints. Indeed, some research indicates that dopaminergic drugs show the bimodal response occurs based, at least in part, on the difference in the individual response before administration of the drug (54, 55).
The critical need for new and more useful biomarkers in neuropsychiatric disorders has also been well documented in the literature (56). The use of evoked gamma for biomarker-based stratification may be useful for a plethora of other neuropsychiatric disorders that display altered evoked gamma. This list includes schizophrenia, bipolar disorder (8, 12, 57–60), autism (61, 62), 22q11.2 deletion (63), and fragile X syndrome (11). For these diseases, incorporation of a prospective screen would aid in the selection of a more appropriate trial population and, in the case of ineffective compounds, possibly reveal a candidate's lack of efficacy at an earlier stage. It should be noted that the role(s) of gamma power abnormalities in the pathophysiology of each neuropsychiatric disorders needs to be carefully assessed.
Although the industry is still facing challenges regarding identification of potential responders in interventional trials, recent results may shed some light on new avenues of investigation into psychometric measures that identify biologically distinct subpopulations. For example, Swerdlow and Light propose treatment-sensitive individuals (i.e., responder) for cognitive training could be detected by the response of early auditory information processing (EAIP) after a single challenge dose of a pharmacologic agent (46, 64). Evoked gamma power and other measures of EIAP were enhanced with acute challenge of memantine, which is considered as retained plasticity in response to interventions. In a similar vein, Javitt et al. showed that there are (at least) two biologically distinct subgroups in schizophrenia that can be identified using tests for tone-matching and mismatch negativity (65, 66). Further, Light et al. proposed pre-dose mismatch negativity could predict response some interventions (51, 67). One recent effort to model EAIP using large patient datasets estimated that a microvolt change in the amplitude of mismatch negativity and P3a would produce substantial impact on improvement of cognition and psychosocial functioning, while impaired EAIP predicts poor functional outcomes resulting from impaired cognition and increased negative symptoms (68, 69). These findings support the rationale to identify the relevant patient subpopulations with EEG measures.
An obvious avenue to advancing patient stratification is to pair these emerging psychometric features with EEG measures to identify more biologically distinct subpopulations than previously thought possible. This work has been the focus of working groups like the Bipolar-Schizophrenia Network on Intermediate Phenotypes (B-SNIP), which focuses on studying the genetic, cognitive markers of these disorders. One of the resulting improvements in this area has been the selective pairing of investigational methods to create more precise diagnoses and improved detection (70). The earliest forms of this sort of paired analysis have already been applied to psychosis patients, and this approach, in somewhat different forms, could well be applied to other neuropsychiatric disorders.
Clinically, the lack of biomarker-based stratification and the failure to evaluate a compound's effect on appropriate biomarkers could create blind spots within clinical trials for this class of diseases. Based on the current understanding of these diseases, it is possible to argue that the selective, synergistic incorporation of gamma oscillation and other emerging biomarkers will result in improved trial design and revealed critical flaws (e.g., lack of efficacy) at an earlier stage.
Conclusions
Changes within the gamma band have been proven to be durable biomarkers for neuropsychiatric patient populations, ones that offer commentary on both disease state and response to trial medications. While baseline gamma is an excellent tool for measuring the dynamic changes within target cell populations that occur in response to investigational drugs, the approach has specific limitations in reflecting the disease state and providing good predictive validity when it comes to identifying and testing patient populations. In contrast, evoked gamma measurements offer a potentially better patient stratification biomarker and measure of acute responsiveness to an investigational new drug, but are more difficult to employ in more complex time-course experiments. Given these strengths and limitations, the obvious course of action is the strategic pairing of these approaches to maximize their usefulness in new drug validation and clinical trial design.
The dual use of both baseline and evoked gamma measurement presents additional complexities, primarily related to the difficulty of incorporating EEG measurements in a clinical context. Perhaps, the largest of these challenges is the standardization of methods. Biomarker measurements require well-developed protocols, and these must match precisely in order to create translatable results between different clinical groups. This is doubly important for distributed clinical trials with multiple sites, as well as creating study-to-study consistency. Additionally, the use of evoked gamma measurement in clinical applications must be undertaken strategically, particularly for longer (time course) experiments conducted on neuropsychiatric patients that may be prone to seizures and/or have difficulty remaining still for extended testing. Further efforts might be needed to optimize protocols to fit the demands of clinical trials.
In adapting these lessons for use in clinical trial design, several conclusions become apparent. Baseline gamma would proceed concomitantly with evoked gamma measurement in disease populations. This would inform decisions on trial design (dosing, etc.), while simultaneously stratifying a population of prospective patients for proof of concept study (Figure 1). After confirming the evoked gamma response in this stratified patient population, it is possible to proceed to phase two trials with increased confidence in both the design of the trial and the prospect of a more receptive patient population. As the use of baseline and evoked gamma measurements mature, we anticipate they will provide a pillar upon which to build robust translational tools and develop ever more reliable clinical protocols.
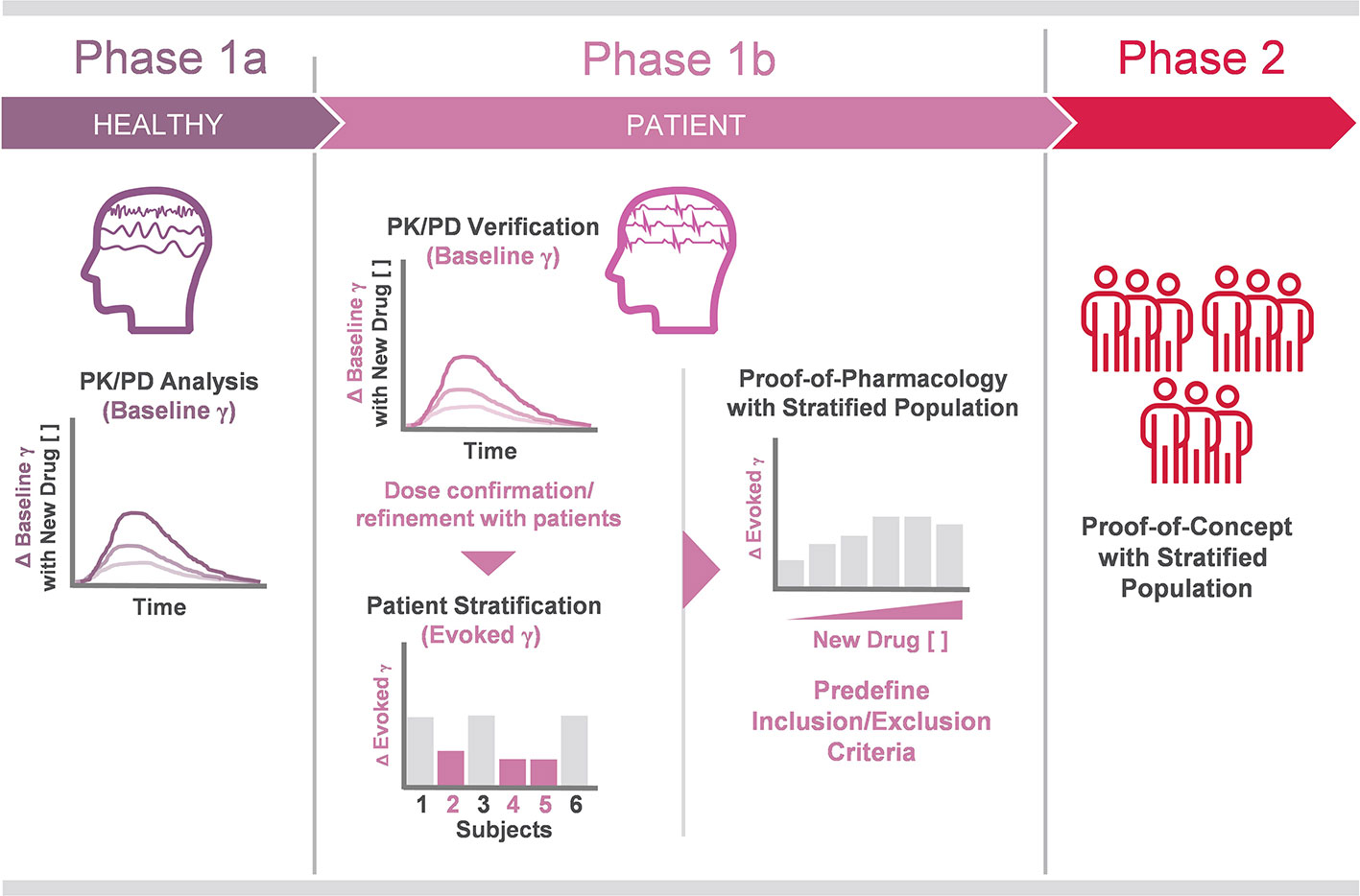
Figure 1 Proposed workflow of gamma oscillation analysis in future clinical trials. Phase 1a PK/PD analysis of investigational drugs targeting E/I imbalance. In the proposed trial design, baseline gamma alterations are tracked against investigational drugs concentrations in plasma, receptor occupancy, etc., in healthy volunteers. These results are confirmed in the patient population prior to stage-up. Phase 1b also includes patient population stratification, achieved in part by identification of blunted evoked gamma in ASSR testing. Phase 2 trial design is informed on dosing and time course insights gained in Phase 1a/b PK/PD testing, while candidate patient populations are defined (Phase 1b) before therapeutic response is evaluated using evoked gamma ASSR and other clinical endpoints in Phase 2.
Author Contributions
SH and TM wrote the manuscript. KT and MM participated in formative discussions and edited the manuscript.
Conflict of Interest
All authors are employees of Astellas Pharma Inc, which is involved in the development and sale of pharmaceutical products.
Acknowledgments
The authors wish to acknowledge Noah Walton for his assistance in assembling this manuscript.
Abbreviations
Auditory Steady State Response, (ASSR); Electroencephalography, (EEG); Early Auditory Information Processing, (EAIP); Excitatory/Inhibitory, (E/I); Pharmacokinetics/Pharmacodynamics, (PK/PD).
References
1. Gandal MJ, Edgar JC, Klook K, Siegel SJ. Gamma synchrony: towards a translational biomarker for the treatment-resistant symptoms of schizophrenia. Neuropharmacology (2012) 62(3):1504–18. doi: 10.1016/j.neuropharm.2011.02.007
2. Lewis DA, Curley AA, Glausier JR, Volk DW. Cortical parvalbumin interneurons and cognitive dysfunction in schizophrenia. Trends Neurosci (2012) 35(1):57–67. doi: 10.1016/j.tins.2011.10.004
3. Uhlhaas PJ, Pipa G, Neuenschwander S, Wibral M, Singer W. A new look at gamma? High- (>60 Hz) gamma-band activity in cortical networks: function, mechanisms and impairment. Prog Biophys Mol Biol (2011) 105(1-2):14–28. doi: 10.1016/j.pbiomolbio.2010.10.004
4. Uhlhaas PJ, Singer W. High-frequency oscillations and the neurobiology of schizophrenia. Dialogues Clin Neurosci (2013) 15(3):301–13.
5. Carlen M, Meletis K, Siegle JH, Cardin JA, Futai K, Vierling-Claassen D, et al. A critical role for NMDA receptors in parvalbumin interneurons for gamma rhythm induction and behavior. Mol Psychiatry (2012) 17(5):537–48. doi: 10.1038/mp.2011.31
6. Brenner CA, Krishnan GP, Vohs JL, Ahn WY, Hetrick WP, Morzorati SL, et al. Steady state responses: electrophysiological assessment of sensory function in schizophrenia. Schizophr Bull (2009) 35(6):1065–77. doi: 10.1093/schbul/sbp091
7. Light GA, Hsu JL, Hsieh MH, Meyer-Gomes K, Sprock J, Swerdlow NR, et al. Gamma band oscillations reveal neural network cortical coherence dysfunction in schizophrenia patients. Biol Psychiatry (2006) 60(11):1231–40. doi: 10.1016/j.biopsych.2006.03.055
8. Spencer KM, Salisbury DF, Shenton ME, McCarley RW. Gamma-band auditory steady-state responses are impaired in first episode psychosis. Biol Psychiatry (2008) 64(5):369–75. doi: 10.1016/j.biopsych.2008.02.021
9. Tada M, Nagai T, Kirihara K, Koike S, Suga M, Araki T, et al. Differential Alterations of Auditory Gamma Oscillatory Responses Between Pre-Onset High-Risk Individuals and First-Episode Schizophrenia. Cereb Cortex (2016) 26(3):1027–35. doi: 10.1093/cercor/bhu278
10. Rojas DC, Wilson LB. gamma-band abnormalities as markers of autism spectrum disorders. Biomark Med (2014) 8(3):353–68. doi: 10.2217/bmm.14.15
11. Ethridge LE, White SP, Mosconi MW, Wang J, Byerly MJ, Sweeney JA. Reduced habituation of auditory evoked potentials indicate cortical hyper-excitability in Fragile X Syndrome. Transl Psychiatry (2016) 6:e787. doi: 10.1038/tp.2016.48
12. Isomura S, Onitsuka T, Tsuchimoto R, Nakamura I, Hirano S, Oda Y, et al. Differentiation between major depressive disorder and bipolar disorder by auditory steady-state responses. J Affect Disord (2016) 190:800–6. doi: 10.1016/j.jad.2015.11.034
13. Fitzgerald PJ, Watson BO. Gamma oscillations as a biomarker for major depression: an emerging topic. Transl Psychiatry (2018) 8(1):177. doi: 10.1038/s41398-018-0239-y
14. Hermes D, Kasteleijn-Nolst Trenite DGA, Winawer J. Gamma oscillations and photosensitive epilepsy. Curr Biol (2017) 27(9):R336–8. doi: 10.1016/j.cub.2017.03.076
15. Modi ME, Sahin M. Translational use of event-related potentials to assess circuit integrity in ASD. Nat Rev Neurol (2017) 13(3):160–70. doi: 10.1038/nrneurol.2017.15
16. Akay M, Daubenspeck JA. Investigating the contamination of electroencephalograms by facial muscle electromyographic activity using matching pursuit. Brain Lang (1999) 66(1):184–200. doi: 10.1006/brln.1998.2030
17. Croft RJ, Barry RJ. Removal of ocular artifact from the EEG: a review. Neurophysiol Clin (2000) 30(1):5–19. doi: 10.1016/S0987-7053(00)00055-1
18. Hagemann D, Naumann E. The effects of ocular artifacts on (lateralized) broadband power in the EEG. Clin Neurophysiol (2001) 112(2):215–31. doi: 10.1016/s1388-2457(00)00541-1
19. Gandal MJ, Sisti J, Klook K, Ortinski PI, Leitman V, Liang Y, et al. GABAB-mediated rescue of altered excitatory-inhibitory balance, gamma synchrony and behavioral deficits following constitutive NMDAR-hypofunction. Transl Psychiatry (2012) 2:e142. doi: 10.1038/tp.2012.69
20. Sivarao DV, Chen P, Senapati A, Yang Y, Fernandes A, Benitex Y, et al. 40 Hz Auditory Steady-State Response Is a Pharmacodynamic Biomarker for Cortical NMDA Receptors. Neuropsychopharmacology (2016) 41(9):2232–40. doi: 10.1038/npp.2016.17
21. Kozono N, Honda S, Tada M, Kirihara K, Zhao Z, Jinde S, et al. Auditory Steady State Response; nature and utility as a translational science tool. Sci Rep (2019) 9(1):8454. doi: 10.1038/s41598-019-44936-3
22. Frankle WG, Cho RY, Narendran R, Mason NS, Vora S, Litschge M, et al. Tiagabine increases [11C]flumazenil binding in cortical brain regions in healthy control subjects. Neuropsychopharmacology (2009) 34(3):624–33. doi: 10.1038/npp.2008.104
23. Albrecht MA, Price G, Lee J, Iyyalol R, Martin-Iverson MT. Dexamphetamine selectively increases 40 Hz auditory steady state response power to target and nontarget stimuli in healthy humans. J Psychiatry Neurosci (2013) 38(1):24–32. doi: 10.1503/jpn.110145
24. Minzenberg MJ, Yoon JH, Cheng Y, Carter CS. Sustained Modafinil Treatment Effects on Control-Related Gamma Oscillatory Power in Schizophrenia. Neuropsychopharmacology (2016) 41(5):1231–40. doi: 10.1038/npp.2015.271
25. Zhang J, Ma L, Li W, Yang P, Qin L. Cholinergic modulation of auditory steady-state response in the auditory cortex of the freely moving rat. Neuroscience (2016) 324:29–39. doi: 10.1016/j.neuroscience.2016.03.006
26. Sanacora G, Smith MA, Pathak S, Su HL, Boeijinga PH, McCarthy DJ, et al. Lanicemine: a low-trapping NMDA channel blocker produces sustained antidepressant efficacy with minimal psychotomimetic adverse effects. Mol Psychiatry (2014) 19(9):978–85. doi: 10.1038/mp.2013.130
27. Agbo F, Bui KH, Zhou D. Population pharmacokinetic analysis of lanicemine (AZD6765), an NMDA channel blocker, in healthy subjects and patients with major depressive disorder. J Clin Pharm Ther (2017) 42(5):539–46. doi: 10.1111/jcpt.12541
28. Hirano Y, Oribe N, Kanba S, Onitsuka T, Nestor PG, Spencer KM. Spontaneous Gamma Activity in Schizophrenia. JAMA Psychiatry (2015) 72(8):813–21. doi: 10.1001/jamapsychiatry.2014.2642
29. Grent-'t-Jong T, Rivolta D, Sauer A, Grube M, Singer W, Wibral M, et al. MEG-measured visually induced gamma-band oscillations in chronic schizophrenia: Evidence for impaired generation of rhythmic activity in ventral stream regions. Schizophr Res (2016) 176(2-3):177–85. doi: 10.1016/j.schres.2016.06.003
30. Uhlhaas PJ, Linden DE, Singer W, Haenschel C, Lindner M, Maurer K, et al. Dysfunctional long-range coordination of neural activity during Gestalt perception in schizophrenia. J Neurosci (2006) 26(31):8168–75. doi: 10.1523/JNEUROSCI.2002-06.2006
31. Brenner CA, Sporns O, Lysaker PH, O'Donnell BF. EEG synchronization to modulated auditory tones in schizophrenia, schizoaffective disorder, and schizotypal personality disorder. Am J Psychiatry (2003) 160(12):2238–40. doi: 10.1176/appi.ajp.160.12.2238
32. Kirihara K, Rissling AJ, Swerdlow NR, Braff DL, Light GA. Hierarchical organization of gamma and theta oscillatory dynamics in schizophrenia. Biol Psychiatry (2012) 71(10):873–80. doi: 10.1016/j.biopsych.2012.01.016
33. Kwon JS, O'Donnell BF, Wallenstein GV, Greene RW, Hirayasu Y, Nestor PG, et al. Gamma frequency-range abnormalities to auditory stimulation in schizophrenia. Arch Gen Psychiatry (1999) 56(11):1001–5. doi: 10.1001/archpsyc.56.11.1001
34. Teale P, Collins D, Maharajh K, Rojas DC, Kronberg E, Reite M. Cortical source estimates of gamma band amplitude and phase are different in schizophrenia. Neuroimage (2008) 42(4):1481–9. doi: 10.1016/j.neuroimage.2008.06.020
35. Thune H, Recasens M, Uhlhaas PJ. The 40-Hz Auditory Steady-State Response in Patients With Schizophrenia: A Meta-analysis. JAMA Psychiatry (2016) 73(11):1145–53. doi: 10.1001/jamapsychiatry.2016.2619
36. Tsuchimoto R, Kanba S, Hirano S, Oribe N, Ueno T, Hirano Y, et al. Reduced high and low frequency gamma synchronization in patients with chronic schizophrenia. Schizophr Res (2011) 133(1-3):99–105. doi: 10.1016/j.schres.2011.07.020
37. Vierling-Claassen D, Siekmeier P, Stufflebeam S, Kopell N. Modeling GABA alterations in schizophrenia: a link between impaired inhibition and altered gamma and beta range auditory entrainment. J Neurophysiol (2008) 99(5):2656–71. doi: 10.1152/jn.00870.2007
38. Kim T, Thankachan S, McKenna JT, McNally JM, Yang C, Choi JH, et al. Cortically projecting basal forebrain parvalbumin neurons regulate cortical gamma band oscillations. Proc Natl Acad Sci U S A (2015) 112(11):3535–40. doi: 10.1073/pnas.1413625112
39. Ehrlichman RS, Gandal MJ, Maxwell CR, Lazarewicz MT, Finkel LH, Contreras D, et al. N-methyl-d-aspartic acid receptor antagonist-induced frequency oscillations in mice recreate pattern of electrophysiological deficits in schizophrenia. Neuroscience (2009) 158(2):705–12. doi: 10.1016/j.neuroscience.2008.10.031
40. Leishman E, O'Donnell BF, Millward JB, Vohs JL, Rass O, Krishnan GP, et al. Phencyclidine Disrupts the Auditory Steady State Response in Rats. PloS One (2015) 10(8):e0134979. doi: 10.1371/journal.pone.0134979
41. Saunders JA, Gandal MJ, Siegel SJ. NMDA antagonists recreate signal-to-noise ratio and timing perturbations present in schizophrenia. Neurobiol Dis (2012) 46(1):93–100. doi: 10.1016/j.nbd.2011.12.049
42. Schuelert N, Dorner-Ciossek C, Brendel M, Rosenbrock H. A comprehensive analysis of auditory event-related potentials and network oscillations in an NMDA receptor antagonist mouse model using a novel wireless recording technology. Physiol Rep (2018) 6(16):e13782. doi: 10.14814/phy2.13782
43. Sivarao DV, Frenkel M, Chen P, Healy FL, Lodge NJ, Zaczek R. MK-801 disrupts and nicotine augments 40 Hz auditory steady state responses in the auditory cortex of the urethane-anesthetized rat. Neuropharmacology (2013) 73:1–9. doi: 10.1016/j.neuropharm.2013.05.006
44. Sullivan EM, Timi P, Hong LE, O'Donnell P. Effects of NMDA and GABA-A Receptor Antagonism on Auditory Steady-State Synchronization in Awake Behaving Rats. Int J Neuropsychopharmacol (2015) 18(7):pyu118. doi: 10.1093/ijnp/pyu118
45. Plourde G, Baribeau J, Bonhomme V. Ketamine increases the amplitude of the 40-Hz auditory steady-state response in humans. Br J Anaesth (1997) 78(5):524–9. doi: 10.1093/bja/78.5.524
46. Light GA, Zhang W, Joshi YB, Bhakta S, Talledo JA, Swerdlow NR. Single-Dose Memantine Improves Cortical Oscillatory Response Dynamics in Patients with Schizophrenia. Neuropsychopharmacology (2017) 42(13):2633–9. doi: 10.1038/npp.2017.81
47. Farmer CA, Gilbert JR, Moaddel R, George J, Adeojo L, Lovett J, et al. Ketamine metabolites, clinical response, and gamma power in a randomized, placebo-controlled, crossover trial for treatment-resistant major depression. Neuropsychopharmacology (2020). doi: 10.1038/s41386-020-0663-6
48. Hiyoshi T, Kambe D, Karasawa J, Chaki S. Differential effects of NMDA receptor antagonists at lower and higher doses on basal gamma band oscillation power in rat cortical electroencephalograms. Neuropharmacology (2014) 85:384–96. doi: 10.1016/j.neuropharm.2014.05.037
49. Grent-'t-Jong T, Rivolta D, Gross J, Gajwani R, Lawrie SM, Schwannauer M, et al. Acute ketamine dysregulates task-related gamma-band oscillations in thalamo-cortical circuits in schizophrenia. Brain (2018) 141(8):2511–26. doi: 10.1093/brain/awy175
50. Matsumoto M, Walton NM, Yamada H, Kondo Y, Marek GJ, Tajinda K. The impact of genetics on future drug discovery in schizophrenia. Expert Opin Drug Discovery (2017) 12(7):673–86. doi: 10.1080/17460441.2017.1324419
51. Light GA, Swerdlow NR. Future clinical uses of neurophysiological biomarkers to predict and monitor treatment response for schizophrenia. Ann N Y Acad Sci (2015) 1344:105–19. doi: 10.1111/nyas.12730
52. McGinnity CJ, Riano Barros DA, Rosso L, Veronese M, Rizzo G, Bertoldo A, et al. Test-retest reproducibility of quantitative binding measures of [(11)C]Ro15-4513, a PET ligand for GABAA receptors containing alpha5 subunits. Neuroimage (2017) 152:270–82. doi: 10.1016/j.neuroimage.2016.12.038
53. Roach BJ, D'Souza DC, Ford JM, Mathalon DH. Test-retest reliability of time-frequency measures of auditory steady-state responses in patients with schizophrenia and healthy controls. NeuroImage Clin (2019) 23:101878. doi: 10.1016/j.nicl.2019.101878
54. Takeshita S, Ogura C. Effect of the dopamine D2 antagonist sulpiride on event-related potentials and its relation to the law of initial value. Int J Psychophysiol (1994) 16(1):99–106. doi: 10.1016/0167-8760(94)90046-9
55. Chavanon ML, Wacker J, Stemmler G. Paradoxical dopaminergic drug effects in extraversion: dose- and time-dependent effects of sulpiride on EEG theta activity. Front Hum Neurosci (2013) 7:117. doi: 10.3389/fnhum.2013.00117
56. Suhara T, Chaki S, Kimura H, Furusawa M, Matsumoto M, Ogura H, et al. Strategies for Utilizing Neuroimaging Biomarkers in CNS Drug Discovery and Development: CINP/JSNP Working Group Report. Int J Neuropsychopharmacol (2017) 20(4):285–94. doi: 10.1093/ijnp/pyw111
57. O'Donnell BF, Hetrick WP, Vohs JL, Krishnan GP, Carroll CA, Shekhar A. Neural synchronization deficits to auditory stimulation in bipolar disorder. Neuroreport (2004) 15(8):1369–72. doi: 10.1097/01.wnr.0000127348.64681.b2
58. Reite M, Teale P, Rojas DC, Reite E, Asherin R, Hernandez O. MEG auditory evoked fields suggest altered structural/functional asymmetry in primary but not secondary auditory cortex in bipolar disorder. Bipolar Disord (2009) 11(4):371–81. doi: 10.1111/j.1399-5618.2009.00701.x
59. Rass O, Krishnan G, Brenner CA, Hetrick WP, Merrill CC, Shekhar A, et al. Auditory steady state response in bipolar disorder: relation to clinical state, cognitive performance, medication status, and substance disorders. Bipolar Disord (2010) 12(8):793–803. doi: 10.1111/j.1399-5618.2010.00871.x
60. Oda Y, Onitsuka T, Tsuchimoto R, Hirano S, Oribe N, Ueno T, et al. Gamma band neural synchronization deficits for auditory steady state responses in bipolar disorder patients. PloS One (2012) 7(7):e39955. doi: 10.1371/journal.pone.0039955
61. Wilson TW, Hernandez OO, Asherin RM, Teale PD, Reite ML, Rojas DC. Cortical gamma generators suggest abnormal auditory circuitry in early-onset psychosis. Cereb Cortex (2008) 18(2):371–8. doi: 10.1093/cercor/bhm062
62. Edgar JC, Fisk CLT, Liu S, Pandey J, Herrington JD, Schultz RT, et al. Translating Adult Electrophysiology Findings to Younger Patient Populations: Difficulty Measuring 40-Hz Auditory Steady-State Responses in Typically Developing Children and Children with Autism Spectrum Disorder. Dev Neurosci (2016) 38(1):1–14. doi: 10.1159/000441943
63. Larsen KM, Pellegrino G, Birknow MR, Kjaer TN, Baare WFC, Didriksen M, et al. 22q11.2 Deletion Syndrome Is Associated With Impaired Auditory Steady-State Gamma Response. Schizophr Bull (2018) 44(2):388–97. doi: 10.1093/schbul/sbx058
64. Swerdlow NR, Bhakta SG, Light GA. Room to move: Plasticity in early auditory information processing and auditory learning in schizophrenia revealed by acute pharmacological challenge. Schizophr Res (2018) 199:285–91. doi: 10.1016/j.schres.2018.03.037
65. Lee M, Sehatpour P, Dias EC, Silipo GS, Kantrowitz JT, Martinez AM, et al. A tale of two sites: Differential impairment of frequency and duration mismatch negativity across a primarily inpatient versus a primarily outpatient site in schizophrenia. Schizophr Res (2018) 191:10–7. doi: 10.1016/j.schres.2017.07.032
66. Medalia A, Saperstein AM, Qian M, Javitt DC. Impact of baseline early auditory processing on response to cognitive remediation for schizophrenia. Schizophr Res (2019) 208:397–405. doi: 10.1016/j.schres.2019.01.012
67. Perez VB, Tarasenko M, Miyakoshi M, Pianka ST, Makeig SD, Braff DL, et al. Mismatch Negativity is a Sensitive and Predictive Biomarker of Perceptual Learning During Auditory Cognitive Training in Schizophrenia. Neuropsychopharmacology (2017) 42(11):2206–13. doi: 10.1038/npp.2017.25
68. Rissling AJ, Miyakoshi M, Sugar CA, Braff DL, Makeig S, Light GA. Cortical substrates and functional correlates of auditory deviance processing deficits in schizophrenia. NeuroImage Clin (2014) 6:424–37. doi: 10.1016/j.nicl.2014.09.006
69. Thomas ML, Green MF, Hellemann G, Sugar CA, Tarasenko M, Calkins ME, et al. Modeling Deficits From Early Auditory Information Processing to Psychosocial Functioning in Schizophrenia. JAMA Psychiatry (2017) 74(1):37–46. doi: 10.1001/jamapsychiatry.2016.2980
Keywords: electroencephalography (EEG), schizophrenia, bipolar disorder, autism spectrum disorder (ASD), evoked gamma, baseline gamma, Auditory Steady State Response (ASSR)
Citation: Honda S, Matsumoto M, Tajinda K and Mihara T (2020) Enhancing Clinical Trials Through Synergistic Gamma Power Analysis. Front. Psychiatry 11:537. doi: 10.3389/fpsyt.2020.00537
Received: 17 March 2020; Accepted: 26 May 2020;
Published: 10 June 2020.
Edited by:
Kiyoto Kasai, University of Tokyo, JapanReviewed by:
Kenji Hashimoto, Chiba University, JapanPeter Uhlhaas, University of Glasgow, United Kingdom
Copyright © 2020 Honda, Matsumoto, Tajinda and Mihara. This is an open-access article distributed under the terms of the Creative Commons Attribution License (CC BY). The use, distribution or reproduction in other forums is permitted, provided the original author(s) and the copyright owner(s) are credited and that the original publication in this journal is cited, in accordance with accepted academic practice. No use, distribution or reproduction is permitted which does not comply with these terms.
*Correspondence: Takuma Mihara, dGFrdW1hLm1paGFyYUBhc3RlbGxhcy5jb20=